DOI:
10.1039/D2RA08049D
(Paper)
RSC Adv., 2023,
13, 8882-8889
A simple array integrating machine learning for identification of flavonoids in red wines†
Received
17th December 2022
, Accepted 6th March 2023
First published on 16th March 2023
Abstract
Bioactive flavonoids, the major ingredients of red wines, have been proven to prevent atherosclerosis and cardiovascular disease due to their anti-inflammatory and anti-oxidant activity. However, flavonoids have proven challenging to identify, even when multiple approaches are combined. Hereby, a simple array was constructed to detect flavonoids by employing phenylboronic acid modified perylene diimide derivatives (PDIs). Through multiple non-specific interactions (hydrophilic, hydrophobic, charged, aromatic, hydrogen-bonded and reversible covalent interactions) with flavonoids, the fluorescence of PDIs can be modulated, and variations in intensity can be used to create fingerprints of flavonoids. This array successfully discriminated 14 flavonoids of diverse structures and concentrations with 100% accuracy, based on patterns in fluorescence intensity modulation, via optimized machine learning algorithms. As a result, this array demonstrated the parallel detection of 8 different types and origins of red wines with a high accuracy, revealing the excellent potential of the sensor array in food mixtures detection.
1 Introduction
Wine is an alcoholic drink typically made from fermented grapes. Differences in grape varieties and fermentation strains are the primary factors that lead to wines with different styles and flavors. Flavonoids are important natural polyphenols that exist as plant metabolites and are the major ingredients of red wines.1–5 Due to their potential antioxidant, anti-inflammatory, anti-viral and anti-carcinogenic activities, flavonoids have been investigated for use in the foods, pharmaceuticals, and cosmetics industry and other fields.6,7 Flavonoids with different modified structures diversify in their bioactivities, leading to realistic demand for their qualitative and quantitative discrimination, especially in the food industry and medical research.8–10
Conventional qualitative and quantitative analytic methods for flavonoids mainly rely on nuclear magnetic resonance (NMR), high-performance liquid chromatography (HPLC), liquid chromatography mass spectrometry (LC-MS), UV-vis spectroscopy, electrogenerated chemiluminescence, etc.11–15 However, most of these methods require costly instruments, complicated and time-consuming operations, and fairly involved sample preparation, resulting in low detection efficiency. It is hard for a single method to discriminate flavonoids with the same molecular weight or similar structures, while a combination of methods will undoubtedly increase the testing time and cost. Moreover, flavonoids are mostly found in mixed samples with multiple components, such as in red wines, and conventional techniques are in fact extremely challenging to detect such mixtures. Therefore, it is of interest to develop a new method for rapidly identifying flavonoids in complex environments, particularly those with similar structures or uniform molecular weights.
Array-based sensing employs mimics of the mammalian olfactory and gustatory systems in which artificial arrays of cross-reactive receptors are employed. The signal difference generated by the interaction between the analytes and the sensing elements can be analyzed and processed by suitable algorithms (pattern recognition), and finally expressed as a visual fingerprint. As pattern recognition is the most crucial data analysis for array-based sensing, machine learning (ML) algorithms can prove to be extremely useful in analyzing such data. ML involves a collection of algorithms and models that learns a mathematical model based on a part of the data, to make predictions on the other sections of the data.
Sensor arrays based on optical signals are successfully applied to the rapid detection of analytes due to their powerful recognition capabilities.16 Our team has developed a series of sensor arrays that can detect wines, whiskies, tea, fruit juices, bacteria and antibiotics, etc.17–23 Recently, we successfully constructed a poly(para-aryleneethynylene)s-based sensor array that accurately identified white wines, and discovered that flavonoids are the primary causes of fluorescence quenching.17 Therefore, achieving highly sensitive and parallel detection of various types of flavonoids will greatly facilitate the detection of wines.
Herein we created a simple three-element (phenylboronic acid modified perylene diimide derivatives) fluorescence sensor array capable of discriminating 14 flavonoids with various structures and concentrations. Multiple benzene rings are contained within the skeleton of these flavonoids (Fig. S1†). The array achieves fluorescent signal changes through non-specific interactions (hydrophilic, hydrophobic, electrostatic, aromatic, hydrogen bonding, etc.) between perylene diimide derivatives (PDIs) and the flavonoids, in combination with data processing by different machine learning algorithms to create visual fingerprints of flavonoids (Fig. 1). The ultimate objective is to enable the identification of red wines of different categories, origins and brands, and to exhibit the great potential of this approach for the analysis of food mixtures.
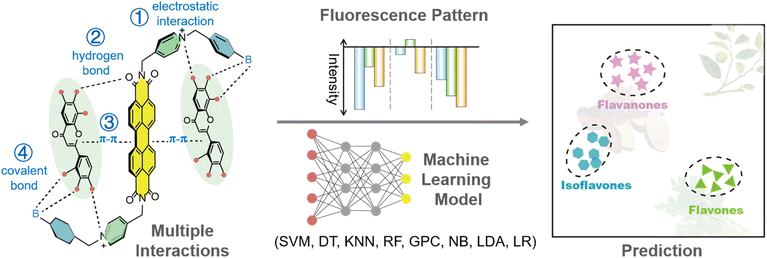 |
| Fig. 1 Plausible mechanism diagram of the sensor array based on boronic acid modified PDIs for the discrimination of flavonoids via machine learning algorithms. | |
2 Experimental
2.1 Reagents and chemicals
All chemicals were obtained from commercial sources and used directly without further purification. 3,4,9,10-Perylenetetracarboxylic acid anhydride (PTCDA) was purchased from https://leyan.com/. 4-(Bromomethyl)phenylboronic acid, 3-(bromomethyl)phenylboronic acid, 2-(bromomethyl)phenylboronic acid, 4-(aminomethyl)pyridine, apigenin, baicalein, genistein, puerarin, daidzein, hesperidin, naringenin, naringin hydrate were purchased from Energy Chemical. Flavone was purchased from Macklin. Methyl hesperidin, neohesperidin, were purchased from Psaitong. Sinensetin, tangeretin, nobiletin, were purchased from Chengdu Biopurify Phytochemicals Ltd. All other reagents, unless mentioned otherwise, were of analytical reagent grade.
2.2 Instrumentation
The fluorescence values were recorded on a SpectraMaxR ID3 Multi-Mode Microplate Reader (Molecular Devices, California, USA) at room temperature. The 96-well plates were produced from Costar (3590, USA). 1H NMR and 13C NMR spectra were recorded at room temperature on Bruker Avance III HD 300 and Bruker Avance III NEO 400. MS spectra were recorded on a Waters 2690 Separations Module and MassLynx analysis software.
2.3 Chemical synthesis of fluorescent sensor array
Synthesized procedures and characterization data were described in ESI (Fig. S2–S14†).
2.3.1 Synthesis of PDI-1. A mixture of compound 1 (0.51 g, 1.30 mmol), compound 2 (0.52 g, 4.81 mmol) and imidazole (25.10 g, 0.37 mol) was added in a 100 mL round bottom flask, then stirred for 48 h in 130 °C under nitrogen. After cooling down to room temperature, added distilled water under stirring, separated the precipitate, filter with suction, and wash the precipitate with a large amount of distilled water to obtain dark red solid 3 (0.64 g), yield: 88%. 1H NMR (300 MHz, CF3COOD) δ = 9.19–8.86 (m, 12H), 8.44 (d, J = 5.5 Hz, 4H), 6.05 (s, 4H).Compound 3 (102 mg, 0.178 mmol) and compound 4 (122 mg, 0.568 mmol) were added to a 50 mL eggplant-shaped flask, protected by nitrogen. Then 7.0 mL of N-methylpyrrolidone (NMP) was added, the reaction was stirred 48 h in 100 °C. After the reaction was over, cool to room temperature, remove most of the organic solvents by rotary evaporation, add the reaction solution dropwise to chloroform, precipitate the precipitate, filter with suction, wash the filter cake with chloroform, and dry the filter cake in vacuo to obtain a red-black solid PDI-1 132 mg, yield: 88%. 1H NMR (300 MHz, DMSO-d6) δ = 9.19 (d, J = 6.4 Hz, 4H), 8.60 (d, J = 8.5 Hz, 4H), 8.33 (d, J = 7.8 Hz, 4H), 8.25 (d, J = 6.5 Hz, 4H), 7.83 (d, J = 7.8 Hz, 4H), 7.49 (d, J = 7.8 Hz, 4H), 5.85 (s, 4H), 5.55 (s, 4H). 13C NMR (75 MHz, DMSO-d6) δ 163.09, 157.68, 145.04, 136.47, 135.31, 133.84, 131.08, 128.45, 128.15, 126.76, 125.01, 124.54, 122.34, 63.18, 43.21. MS: [M–2Br]2+ calculated 842.27, [(M–2Br)/2]+ found 420.9, C50H36B2N4O82+.
2.3.2 Synthesis of PDI-2. The synthesis method is similar to the synthesis of PDI-1. 130 mg of dark red solid was obtained, yield: 88%. 1H NMR (300 MHz, DMSO-d6) δ = 9.15 (d, J = 6.4 Hz, 4H), 8.78–8.59 (m, 4H), 8.40 (d, J = 7.9 Hz, 4H), 8.25 (d, J = 6.5 Hz, 5H), 7.89–7.80 (m, 4H), 7.56 (dt, J = 7.8, 1.5 Hz, 2H), 7.41 (t, J = 7.5 Hz, 2H), 5.83 (s, 4H), 5.55 (s, 4H). 13C NMR (75 MHz, DMSO-d6) δ 163.04, 157.57, 145.00, 135.41, 134.90, 133.91, 133.75, 130.94, 128.77, 126.78, 124.89, 124.54, 122.21, 63.42, 42.87. MS: [M–2Br]2+ calculated 842.27, [(M–2Br)/2]+ found 421.0, C50H36B2N4O82+.
2.3.3 Synthesis of PDI-3. The synthesis method is similar to the synthesis of PDI-1. Obtained 124 mg of red solid, yield: 84%. 1H NMR (300 MHz, DMSO-d6) δ = 8.92 (dd, J = 25.6, 7.5 Hz, 8H), 8.52 (d, J = 7.5 Hz, 8H), 8.21 (d, J = 6.6 Hz, 4H), 7.80 (dd, J = 7.0, 2.0 Hz, 2H), 7.45 (td, J = 7.2, 1.7 Hz, 4H), 7.26 (dd, J = 7.2, 1.7 Hz, 2H), 5.99 (s, 4H), 5.55 (s, 4H). 13C NMR (100 MHz, DMSO-d6) δ 163.37, 157.59, 144.95, 138.26, 135.94, 134.41, 131.45, 130.95, 129.94, 128.90, 126.21, 124.74, 122.99, 63.42, 43.45. MS: [M–2Br]2+ calculated 842.27, [(M–2Br)/2]+ found 421.1, C50H36B2N4O82+.
2.3.4 Preparation of fluorescent sensor array. Three PDI derivatives in dimethyl sulfoxide (DMSO) were prepared as a stock solution (1.0 × 10−3 mol L−1), and diluted with DI water to a final concentration of 1.0 × 10−5 mol L−1, for the construction of the fluorescent sensor array.
2.4 The discrimination of flavonoids
For flavonoids discrimination, 190 μL PDIs solution was mixed with 10 μL flavonoids with a certain concentration. The response of fluorescence signal was obtained by a SpectraMaxR ID3 Multi-Mode Microplate Reader. The obtained data were processed using linear discriminant analysis (LDA) and machine learning.
2.5 Anti-interference experiments
D-Glucose and D-fructose were dissolved in DMSO to obtain a sugar stock solution (D-glucose 1.5 g L−1 and D-fructose 3.5 g L−1, respectively). Similarly, tartaric acid and malic acid were dissolved to prepare an acid solution (tartaric acid 3.0 g L−1 and malic acid 2.0 g L−1, respectively). 10 mM flavonoid stock solution was diluted with sugar or acid solution to make 1 mM flavonoid test solution. For the anti-interference experiment, 190 μL PDIs solution was mixed with 10 μL flavonoids (1 mM). The fluorescent intensity was recorded at emission maximum (550 nm) and the raw data matrix was subjected to linear discriminant analysis (LDA).
2.6 The detection of red wines
Eight commercially available red wines (Fig. 6B) were selected for detecting experiments. Due to the strong quenching ability of red wine, the red wine sample was diluted 5000 times with DI water, then wines (50 μL) were added to the suspension of PDIs aqueous solution (50 μL, 10 μM), and the analysis process was the same as the above-described sensing procedure.
2.7 Data processing and analysis
All data were processed using python 3.2.2, and the machine learning models used were derived from scikit-learn. https://scikit-learn.org/stable/index.html.
Eight machine learning algorithms, including support vector machine (SVM), decision tree (DT), K-nearest neighbor (KNN), random forest (RF), Gaussian process classifier (GPC), naïve bayes (NB), logistic regression (LR) and linear discriminant analysis (LDA) were used in the data processing and the exact code has been put into the ESI.†
3 Results and discussion
3.1 Design of fluorescent sensor array
The cationic water-soluble fluorescent sensor elements modified with phenylboronic acid were prepared through reported literature with minor modifications.24–26 To improve the water-solubility and recognition capability of the sensor elements, pyridinium ammonium salt group and phenylboronic acid group with different substitution positions were introduced (Fig. 2A). First, flavonoids contain multiple benzene rings that can easily form π–π interactions with PDI fluorophores of highly conjugated systems and change their self-assembly patterns.27–31 Second, flavonoids contain multiple hydroxyl and methoxy substituents, which are capable of forming hydrogen bonds and reversible covalent bonds with boronic acid moieties.32–35 Further, cationic character of quaternary ammonium groups, which may form an electrostatic effect with flavonoids, is introduced simultaneously at both ends of the symmetrical PDI to strengthen recognition ability. Finally, the substitution position of the recognition group (boronic acid) is adjusted to affect the binding ability between the sensor elements to the analytes, resulting in differential responses.
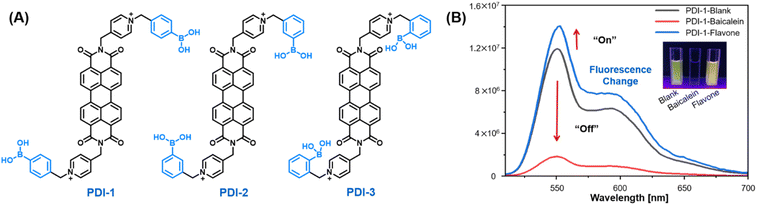 |
| Fig. 2 (A) Structures of the PDIs employed for constructing sensor array, (B) fluorescence change of PDI-1 (10 μM, aqueous solution) after adding baicalein and flavone. | |
3.2 Optical properties
As is evident from the UV-vis and fluorescence spectrum of sensor elements in Fig. S15A,† three sensor elements exhibited similar absorption (470 nm, 500 nm and 543 nm) and emission (550 nm and 593 nm) properties due to the same conjugated skeletal structure.
3.3 Verify the feasibility of the designed array
Baicalein and flavone with evident structural variations were chosen to preliminarily verify the feasibility of the designed array by testing the fluorescence intensity changes of PDI-1 after adding flavonoids. In Fig. 2B, baicalein containing three hydroxyl groups quenched the fluorescence of PDI, whereas flavone without hydroxyl groups increased the fluorescence of PDI. The apparently differentiated fluorescence responses stimulated us to explore more structural types of flavonoids.
3.4 Discrimination of 14 flavonoids
14 different commercially available flavonoids from three subgroups which were based on their structural differences and similarities were selected as tested analytes, aiming at exploring the structure–function relationship and the regularity in discriminating flavonoids, and forecasting the species of the tested flavonoids (Fig. 3). Although many types of flavonoids have been discovered, the accurate differentiation of flavonoids with the same molecular weight or similar structures has remained challenging through a single method. Fluorescence intensity changes (550 nm) were recorded and represented as (I − I0)/I0. I and I0 were defined as the fluorescence intensity of sensor element with and without flavonoids, respectively.
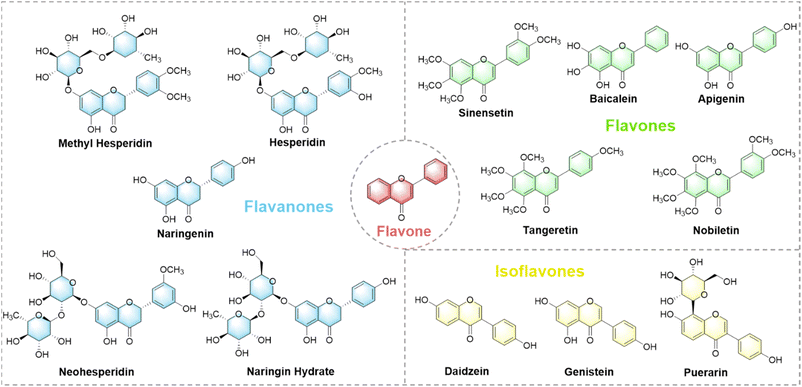 |
| Fig. 3 Structures, classification of the investigated 14 flavonoids. | |
Fig. 4A shows that the addition of flavonoids resulted in a significant fluorescence intensity change of the sensor elements. Most flavonoids showed fluorescence quenching, with flavones demonstrating the most remarkable fluorescence decrease. We speculate this might be attributed to the non-specific interactions (electrostatic interactions, hydrogen bonding, covalent bonding and π–π interactions) between PDIs and flavonoids, leading to further aggregation of the self-assembly of PDI. Thus, greater aggregation leads to fluorescence quenching (Fig. S15B†). Moreover, hydrophobic flavone with weak non-specific interactions might insert into the hydrophobic region of self-assembled PDIs, destroying the rigidity of self-assembly, and leading to enhanced fluorescence intensity (Fig. S15B,† left). Meanwhile, the various sites of the boronic acid substitutions can lead to differences in the binding and assembly of flavonoids.
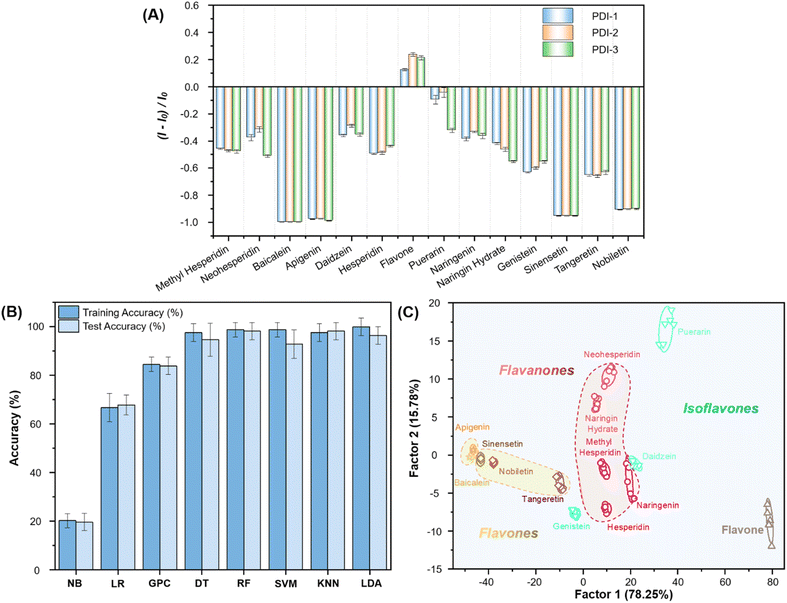 |
| Fig. 4 (A) Fluorescence response pattern ((I − I0)/I0) obtained by PDIs (10 μM, aqueous solution) treated with flavonoids (50 μM). Each value is the average of six independent measurements; each error bar shows the standard error (SE) of these measurements, (B) comparison of prediction accuracies for 14 types of flavonoids by employing different models including NB, LR, GPC, DT, RF, SVM, KNN and LDA on the training set and test set, (C) LDA 2D plot for the first two factors obtained with an array of the PDIs (10 μM, aqueous solution) with 95% confidence ellipses. Each point represents the response pattern for a single analyte in the array. | |
The fluorescence intensity changes of three sensor elements towards 14 flavonoids were obtained and subjected to different machine learning techniques to obtain a suitable analysis method,36–42 and the results are summarized in Fig. 4B. Among these eight algorithms, RF, KNN and LDA showed high accuracy in training and prediction models.43–47 We divided our data sets at a ratio of 6
:
4 (∼60% training and ∼40% testing). Their accuracies were RF (98.8% training, 98.2% prediction), KNN (97.6% training, 98.2% prediction) and LDA (100% training, 96.4% prediction), respectively.
Apart from its excellent accuracy, LDA is also a fantastic visualization tool, allowing us to clearly observe the fingerprint features of each analyte.16,48 As shown in Fig. 4C, LDA converts the training matrix (3 sensor elements × 14 flavonoids × 6 replications) into canonical scores according to their Mahalanobis distance. The first two canonical factors represented 94.03% of the total variation. The canonical scores were clustered into 14 groups. All flavonoids were differentiated to generate their unique fingerprints. Meanwhile, we observed that the fingerprints of 14 flavonoids were independently clustered according to their structural similarity. Flavones were located on the left-hand side of the plot, flavanones were in the middle, while isoflavones were located on the right-hand side of the plot (Tables S1–S4†). Generally, the more complex the mechanism of action, the better the discrimination effect on the same type of analytes. Therefore, compared with the highly specific probe, it can usually act with a wide range of substances. Thus, our sensor array is theoretically capable of identifying multiple flavonoids as well as analogues.
3.5 Detection of different concentrations of single flavonoids
With these results in hand, we further applied such arrays to the semi-quantitative detection of flavonoids. Tangeretin and hesperidin of different concentrations and different proportions were selected for testing. The fluorescence intensity (550 nm) changes of sensor elements were recorded in Fig. S16.† The training matrix (3 sensor elements × tangeretin/hesperidin, 5 concentrations × 6 replicates) was converted into five canonical scores with LDA. As shown in Fig. 5A and B, the concentrations were linearly mapped in the LDA plot, clear discrimination dependence on the concentration of tangeretin and hesperidin were observed. The blind test achieved 100% accuracy (Tables S5–S10†). The results suggested that the array should allow for rigorous semi-quantitative detection. Additionally, different proportions of tangeretin and hesperidin mixtures can be easily distinguished with 100% accuracy (Fig. 5C and Tables S1–S13†). These experiments demonstrated the great potential of our sensor array in discriminating flavonoids with different compositions, contents and mixing states in real-life applications.
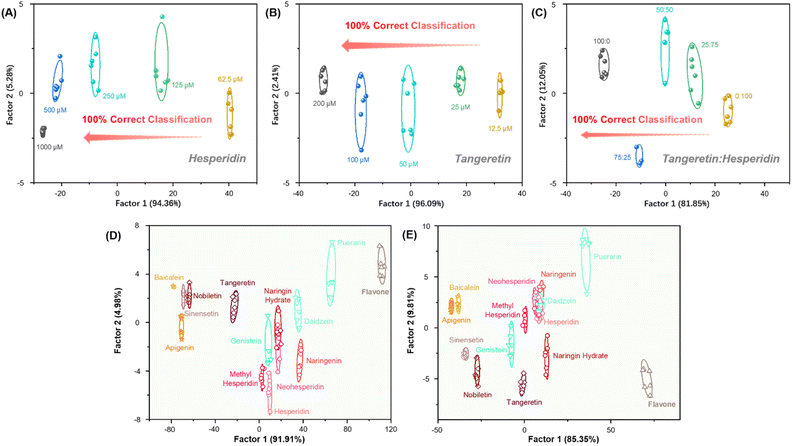 |
| Fig. 5 (A) LDA diagrams of different concentrations of hesperidin, (B) LDA diagrams of different concentrations of tangeretin, (C) LDA diagrams of tangeretin and hesperidin in different proportions, (D) LDA diagrams for the discrimination of 14 flavonoids (50 μM) in the presence of carbohydrates (5 g L−1), (E) LDA diagrams for the discrimination of 14 flavonoids (50 μM) in the presence of carboxylic acids (5 g L−1). | |
3.6 Anti-interference tests for the detection of flavonoids
We further investigated the anti-interference capability of our array. Flavonoids are the main components of red wines, which also contain other components, such as carbohydrates and organic acids. We performed anti-interference experiments by mimicking the other major components of red wines. First, carbohydrates (D-glucose and D-fructose, 5 g L−1) were selected as interferents to investigate the effect on the detection system. LDA converted the training matrix (3 sensor elements × 14 flavonoids × 6 replicates, Fig. 5D and S17A†) into canonical scores according to their Mahalanobis distance, and the sensor array can still accurately distinguish between 14 flavonoids with an accuracy of 93%. Meanwhile, blind tests showed 95% accuracy. Furthermore, the array was still able to distinguish between 14 flavonoids in the presence of carboxylic acids (tartaric acid and malic acid, 5 g L−1), with model and prediction accuracies of 92% and 91%, respectively (Fig. 5E and S17B†). Therefore, our sensor array possesses excellent anti-interference capability, indicating the potential of sensor array for applications in complicated environments (Tables S14–S19†).
3.7 Application in real samples
To evaluate the applicability of this PDI-based sensor array in real samples, eight red wines of various types and brands were selected for the study (Fig. 6B). Cabernet Sauvignon blended wine (wine 1, wine 2), Tempranillo wine (wine 3, wine 4), Syrah wine (wine 5), Merlot wine (wine 6, wine 7) and Pinot Noir wine (wine 8) were selected for fluorescence detection analysis to evaluate the practicality of the sensor array (Fig. 6B). The fluorescence intensity changes of three sensor elements towards 8 wines were obtained (Fig. 6A and Tables S20–S22†). The data was analyzed by 8 machine learning algorithms (Fig. 6C), GPC (98% training, 91% prediction) and LDA (98% training, 91% prediction) showed high accuracy in training and prediction models. 8 red wines were grouped by grape varieties on LDA diagrams (Fig. 6D and S18†). It is worth noting that the fingerprints of red wines can be clustered according to the similarity of the grape types that were fermented. The above experimental results showed that the designed sensor array has tremendous potential in wine analysis and quality control.
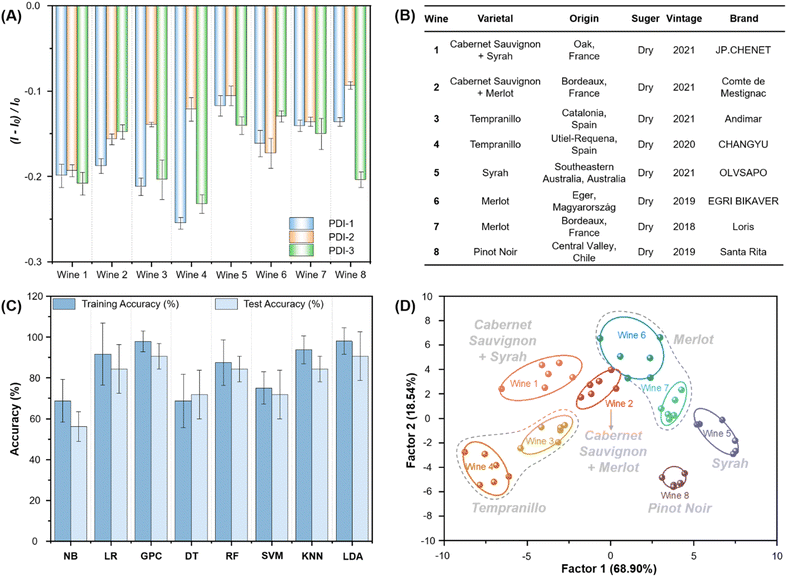 |
| Fig. 6 (A) Fluorescence response pattern ((I − I0)/I0) obtained by PDIs (10 μM, aqueous solution) treated with 8 red grape wines. Each value is the average of six independent measurements; each error bar shows the standard error (SE) of these measurements, (B) detailed information of the eight commercial red wines used in this study, (C) comparison of prediction accuracies for 8 red grape wines by employing different models including NB, LR, GPC, DT, RF, SVM, KNN and LDA on the training set and test set, (D) LDA 2D plot for the first two factors obtained with an array of the PDIs (10 μM, aqueous solution) with 95% confidence ellipses. Each point represents the response pattern for a single wine to the array. | |
4 Conclusions
In summary, we have successfully constructed a PDI-based fluorescence sensor array that enabled the rapid and highly sensitive identification of 14 flavonoids through a rational structural design strategy. With the addition of flavonoids, the supramolecular assembly abilities of PDI could be disturbed, leading to sensitive changes in fluorescence intensity. In combination with machine learning algorithms, our designed sensor array can qualitatively and quantitatively discriminate 14 kinds of flavonoids with 100% accuracy. Furthermore, our designed array possessed exquisite ability in distinguishing eight red wines, demonstrating that the combination of sensor arrays and machine learning algorithms accelerate the development of food mixtures detection, even when the exact ingredients of the mixtures are not known.
Author contributions
J. Q., H. W. and Y. X. contributed equally. J. H., W. W., F. L. and L. L. designed research; J. Q., H. W., Y. X., F. S., J. L. and S. Y. performed and verified experiments; J. H., F. L., J. Q., H. W., Y. X., F. S., S. Y., H. H., C. S., L. L., and J. L. discussed and analyzed the data; J. H., F. L., H. H., W. W., C. S., J. Q., H. W., Y. X. and H. H. wrote the paper.
Conflicts of interest
There are no conflicts to declare.
Acknowledgements
The authors thank Dr Hui-Min Xu from The Public Laboratory Platform at China Pharmaceutical University for assistance with NMR techniques.
Notes and references
- M. Labadie, G. Vallin, A. Petit, L. Ring, T. Hoffmann, A. Gaston, A. Potier, W. Schwab, C. Rothan and B. Denoyes, J. Agric. Food Chem., 2020, 68, 6927–6939 CrossRef CAS PubMed.
- A. P. Umali, S. E. LeBoeuf, R. W. Newberry, S. Kim, L. Tran, W. A. Rome, T. A. Tian, D. Taing, J. Hong, M. Kwan, H. Heymann and E. V. Anslyn, Chem. Sci., 2011, 2, 439–445 RSC.
- B. Heneka, H. Rimpler, A. Ankli, O. Sticher, S. Gibbons and M. Heinrich, Phytochemistry, 2005, 66, 649–652 CrossRef CAS PubMed.
- W. Li, Y. Gao, J. Zhao and Q. Wang, J. Agric. Food Chem., 2007, 55, 8478–8484 CrossRef CAS PubMed.
- M. Søltoft, J. Nielsen, K. Holst Laursen, S. Husted, U. Halekoh and P. Knuthsen, J. Agric. Food Chem., 2010, 58, 10323–10329 CrossRef PubMed.
- W. P. Xi, J. F. Lu, J. P. Qun and B. N. Jiao, J. Food Sci. Technol., 2017, 54, 1108–1118 CrossRef CAS PubMed.
- D. Barreca, C. Bisignano, G. Ginestra, G. Bisignano, E. Bellocco, U. Leuzzi and G. Gattuso, Food Chem., 2013, 141, 1481–1488 CrossRef CAS PubMed.
- A. Nakajima and Y. Ohizumi, Int. J. Mol. Sci., 2019, 20, 3380 CrossRef CAS PubMed.
- A. Ullah, S. Munir, S. L. Badshah, N. Khan, L. Ghani, B. G. Poulson, A. H. Emwas and M. Jaremko, Molecules, 2020, 25, 5243 CrossRef CAS PubMed.
- A. Nakajima, Y. Ohizumi and K. Yamada, Clin. Psychopharmacol. Neurosci., 2014, 12, 75–82 CrossRef PubMed.
- J. Y. Zhang, Q. Zhang, H. X. Zhang, Q. Ma, J. Q. Lu and Y. J. Qiao, J. Agric. Food Chem., 2012, 60, 9023–9034 CrossRef CAS PubMed.
- J. Lei, Y. Xue, Y. M. Liu and X. Liao, Chem. Cent. J., 2017, 11, 13 CrossRef CAS PubMed.
- J. Y. Zhang, J. Q. Lu, Q. Zhang, L. Dai, Y. Liu, P. F. Tu and Y. J. Qiao, J. Chromatogr. Sci., 2014, 52, 103–114 CAS.
- N. Sharma, V. Khajuria, S. Gupta, C. Kumar, A. Sharma, N. A. Lone, S. Paul, S. R. Meena, Z. Ahmed, N. K. Satti and M. K. Verma, ACS Omega, 2021, 6, 30241–30259 CrossRef CAS PubMed.
- H. M. Merken and G. R. Beecher, J. Agric. Food Chem., 2000, 48, 577–599 CrossRef CAS PubMed.
- Z. Li and K. S. Suslick, Acc. Chem. Res., 2021, 54, 950–960 CrossRef CAS PubMed.
- J. Han, M. Bender, K. Seehafer and U. H. F. Bunz, Angew. Chem., Int. Ed., 2016, 55, 7689–7692 CrossRef CAS PubMed.
- J. S. Han, C. Ma, B. H. Wang, M. Bender, M. Bojanowski, M. Hergert, K. Seehafer, A. Herrmann and U. H. F. Bunz, Chem, 2017, 2, 817–824 CAS.
- J. S. Han, B. H. Wang, M. Bender, K. Seehafer and U. H. F. Bunz, Analyst, 2017, 142, 537–543 RSC.
- B. H. Wang, J. S. Han, M. Bender, S. Hahn, K. Seehafer and U. H. F. Bunz, ACS Sens., 2018, 3, 504–511 CrossRef CAS PubMed.
- J. S. Han, B. H. Wang, M. Bender, K. Seehafer and U. H. F. Bunz, ACS Appl. Mater. Interfaces, 2016, 8, 20415–20421 CrossRef CAS PubMed.
- J. S. Han, H. R. Cheng, B. H. Wang, M. S. Braun, X. B. Fan, M. Bender, W. Huang, C. Domhan, W. Mier, T. Lindner, K. Seehafer, M. Wink and U. H. F. Bunz, Angew. Chem., Int. Ed., 2017, 56, 15246–15251 CrossRef CAS PubMed.
- J. S. Han, B. H. Wang, M. Bender, S. Kushida, K. Seehafer and U. H. F. Bunz, ACS Appl. Mater. Interfaces, 2017, 9, 790–797 CrossRef CAS PubMed.
- F. Rigodanza, E. Tenori, A. Bonasera, Z. Syrgiannis and M. Prato, Eur. J. Org. Chem., 2015, 2015, 5060–5063 CrossRef CAS.
- D. Ozdal, N. P. Aydinlik, J. B. Bodapati and H. Icil, Photochem. Photobiol. Sci., 2017, 16, 262–270 CrossRef CAS PubMed.
- C. Huang, S. Barlow and S. R. Marder, J. Org. Chem., 2011, 76, 2386–2407 CrossRef CAS PubMed.
- P. Singh, P. Sharma, N. Kaur, L. S. Mittal and K. Kumar, Anal. Methods, 2020, 12, 3560–3574 RSC.
- H. Zhao, S. Hussain, X. Y. Liu, S. L. Li, F. T. Lv, L. B. Liu and S. Wang, Chem.–Eur. J., 2019, 25, 9834–9839 CrossRef CAS PubMed.
- D. Dong, Q. Li, W. Hou and H. Zhang, J. Mol. Struct., 2020, 1199, 127002 CrossRef CAS.
- R. Roy, N. R. Sajeev, V. Sharma and A. L. Koner, ACS Appl. Mater. Interfaces, 2019, 11, 47207–47217 CrossRef CAS PubMed.
- T. L. Mako, J. M. Racicot and M. Levine, Chem. Rev., 2019, 119, 322–477 CrossRef CAS PubMed.
- G. Q. Fang, H. Wang, Z. C. Bian, J. Sun, A. Q. Liu, H. Fang, B. Liu, Q. Q. Yao and Z. Y. Wu, RSC Adv., 2018, 8, 29400–29427 RSC.
- T. Li and Y. Liu, Anal. Chem., 2021, 93, 7029–7036 CrossRef CAS PubMed.
- X. T. Zhang, S. Wang and G. W. Xing, ACS Appl. Mater. Interfaces, 2017, 9, 3368–3375 CrossRef CAS PubMed.
- R. Wang, Z. C. Bian, D. X. Zhan, Z. Y. Wu, Q. Q. Yao and G. M. Zhang, Dyes Pigm., 2021, 185, 108885 CrossRef CAS.
- W. P. Walters and R. Barzilay, Acc. Chem. Res., 2021, 54, 263–270 CrossRef CAS PubMed.
- H. He, S. Yan, D. Y. Lyu, M. X. Xu, R. Q. Ye, P. Zheng, X. Y. Lu, L. Wang and B. Ren, Anal. Chem., 2021, 93, 3653–3665 CrossRef CAS PubMed.
- K. P. Yin, S. Q. Wu, H. Zheng, L. Gao, J. F. Liu, C. L. Yang, L. W. Qi and J. J. Peng, Langmuir, 2021, 37, 5321–5328 CrossRef CAS PubMed.
- S. Maruyama, K. Ouchi, T. Koganezawa and Y. Matsumoto, ACS Comb. Sci., 2020, 22, 348–355 CrossRef CAS PubMed.
- F. Strieth-Kalthoff, F. Sandfort, M. H. S. Segler and F. Glorius, Chem. Soc. Rev., 2020, 49, 6154–6168 RSC.
- J. F. Joung, M. Han, J. Hwang, M. Jeong, D. H. Choi and S. Park, JACS Au, 2021, 1, 427–438 CrossRef CAS PubMed.
- F. Li, J. S. Han, T. Cao, W. Lam, B. E. Fan, W. Tang, S. J. Chen, K. L. Fok and L. X. Li, Proc. Natl. Acad. Sci. U. S. A., 2019, 116, 11259–11264 CrossRef CAS PubMed.
- P. Zhao, Y. Wu, C. Feng, L. Wang, Y. Ding and A. Hu, Anal. Chem., 2018, 90, 4815–4822 CrossRef CAS PubMed.
- R. Xie, P. Yang, J. Liu, X. Zou, Y. Tan, X. Wang, J. Tao and P. Zhao, Talanta, 2021, 231, 122366 CrossRef CAS PubMed.
- H. Fang, H. L. Wu, T. Wang, W. J. Long, A. Q. Chen, Y. J. Ding and R. Q. Yu, Food Chem., 2021, 342, 128235 CrossRef CAS PubMed.
- Z. Wang, Y. Niu, T. Vashisth, J. Li, R. Madden, T. S. Livingston and Y. Wang, Hortic. Res., 2022, 9, uhac145 CrossRef PubMed.
- Z. Sun, Y. Z. Fan, S. Z. Du, Y. Z. Yang, Y. Ling, N. B. Li and H. Q. Luo, Anal. Chem., 2020, 92, 7273–7281 CrossRef CAS PubMed.
- L. Mitchell, C. Shen, H. C. Timmins, S. B. Park and E. J. New, ACS Sens., 2021, 6, 1261–1269 CrossRef CAS PubMed.
|
This journal is © The Royal Society of Chemistry 2023 |
Click here to see how this site uses Cookies. View our privacy policy here.