DOI:
10.1039/D2RA06467G
(Paper)
RSC Adv., 2023,
13, 5259-5265
Design of highly active substrates using molecular docking for microbial transglutaminase detection†
Received
13th October 2022
, Accepted 6th February 2023
First published on 13th February 2023
Abstract
The transglutaminase (TGase) family catalyzes a transamidation reaction between glutamine (Gln) and lysine (Lys) residues on protein substrates. Highly active substrates are important for cross-linking and modifying proteins of TGase. In the present work, high-activity substrates have been designed based on the principles of enzyme–substrate interaction, using microbial transglutaminase (mTGase) as a research model of the TGase family. Substrates with high activity were screened using a combination of molecular docking and traditional experiments. Twenty-four sets of peptide substrates all produced good catalytic activity with mTGase. FFKKAYAV as the acyl acceptor and VLQRAY as the acyl donor group had the best reaction efficiency with highly sensitive detection of 26 nM mTGase. In addition, the substrate grouping, KAYAV and AFQSAY, detected 130 nM mTGase under physiological conditions (37 °C, pH 7.4), producing 20-fold higher activity than the natural substrate, collagen. The experimental results confirmed the potential for design of high-activity substrates by a combination of molecular docking and traditional experiments under physiological conditions.
Introduction
Transglutaminases (TGase, EC 2.3.2.13) are a widely distributed group of enzymes that catalyze the acyl transfer between inter- or intramolecular lysine ε-amino groups and glutamate γ-carboxyamido groups to form heteropeptide bonds.1–5 The acyl donor is glutamine and the acyl acceptor is the ε-amino group of lysine.1–8 The accumulation of high molecular cross-linked product is found in a number of tissues, including skin, hair, blood clotting and wound healing.9–12 Monitoring TGase activity is important for clinical diagnosis because dysregulation of certain TGase activity involves major disruptions of cellular homoeostatic mechanisms, which is related to a number of human diseases, including neurodegenerative disease, metabolic disorders, cancers and fibrotic diseases.9–17 Not surprisingly, high activity substrates of TGase can reduce non-specific binding and substrate flooding affixation, which are significant for the detection of TGase activity, the diagnosis of related diseases and drug discovery.18–22 Currently, numerous works had been devoted to high activity TGase substrates that can compensate for the lack of specific coupling sites between enzymes and substrates.23–26 Base on screening commercial peptide libraries is the most commonly used method of substrate optimization.24–26 However, this traditional screening approach is time consuming and laborious to identify multitudinous compounds with rich composition, varied properties, and structural diversity. The design of effective catalytic substrates according to the structural characteristic has not been fully investigated, nor has studied the enzyme activity under the human physiological environment.
Molecular docking methodology aims to predict the structure of receptor ligand complexes and study the affinities of small molecules within the binding site of particular receptor targets. It is used as a standard computational tool in drug design for lead compound optimization.27–29 In recent years, many works have begun to use molecular docking technology to identify the interactions between enzymes and ligand, based on the enzyme structure and active site can be simulated by molecular docking software and optimal substrate-binding identified.27–31 Compared with traditional experimental techniques, molecular docking is a virtual technique that uses computer simulation to analyze the interaction between receptors and ligands, which is low-cost and labor-saving. In particular, the method of combining computational analysis and experimental assays is a reasonable strategy to achieve effectively evaluation of many protein reactions.
Here, designing high activity substrates have been employed to develop highly sensitive assay for detecting TGase based on molecular docking and experimental studies. Microbial transglutaminase (mTGase) as one of Ca2+ independent TGase is our research model for designing high activity substrates to improve the reactivity of enzyme. mTGase is thought to have a novel discoidal three-dimensional (3D) structure with a deep cleft at the edge.20,21 Cys64 occupies the main catalytic site and is located at the bottom of the cleft, covered by the α-helix. The left wall of the active site cleft maintains the enzyme's structural stability while the right wall has a flexibility which reduces the spatial site block between the enzyme and the substrate. Thus, the active site Cys64 is fully exposed to the solvent for rapid reaction with the substrate20–25. During catalysis, the mTGase active site has no preference for specific peptide sequence motifs, recognizing glutamine and lysine residues for cross-linking. Both glutamine (acyl donor) and lysine (acyl acceptor) residues are suitable substrates, producing different levels of reactivity. Therefore, mTGase has a higher reaction rate and broad substrate specificity relative to the mammalian TGases.23–25 The high sensitivity detection due to the highly active substrate is of great significance to the application of mTGase in food safety and emerging biomedical engineering.20,32–51 The current work used molecular docking and traditional experiments to develop high-sensitivity detection of mTGase assay, as follows: (1) peptides were designed and enzyme–substrate interaction simulated by Autodock-vina molecular docking software to allow selection of 6 highly reactive peptides as acyl donors combined with 4 acyl acceptors to produce 24 pairs of highly reactive mTGase substrate combinations; (2) enzyme activities with the 24 sets of substrates were measured and used to define the sensitivity of mTGase detection and identify the pair of substrate peptides with the best enzymatic reactivity; (3) effects of temperature and pH on the enzyme-catalyzed reaction were assessed and the peptide group with the best reactivity selected compared with the natural substrate under physiological conditions.
Materials and methods
Materials and reagents
mTGase was donated by Jiangsu Yiming Biological Products Co., Ltd. (Jiangsu, China) and purified using AKTA purifier (CE Healthcare, UK), see additional experimental section and Fig. S1 in ESI† for details. Twenty-two peptides, including one control peptide CP (CALNN) and twenty-one mTGase substrates (as shown in Table 1), were commercial synthesized by ChinaPeptides Ltd. (Shanghai, China). Collagen was purchased from Gentihold Science & Technology Co., Ltd (Beijing, China). BCA protein Assay kit and PageRuler Plus Prestained ladder were purchased from Thermo Fisher scientific Co., Ltd (Shanghai, China). Other chemicals were analytical grade. Milli-Q water (18.2 MΩ cm) was used in all experiments.
Table 1 Sequence of designed substrates
Acyl acceptor |
Sequence |
Hydrophobicity |
Isoelectric point |
Steric energy (kcal mol−1) |
PA1 |
KAYA |
−0.400 |
9.72 |
2711.120 |
PA2 |
KAYAV |
0.520 |
8.50 |
2766.581 |
PA3 |
FMKHKFV |
0.100 |
10.00 |
7118.663 |
PA4 |
FFKKAYAV |
0.537 |
9.70 |
191.764 |
Acyl donor |
Sequence |
Hydrophobicity |
Isoelectric point |
Steric energy (kcal mol−1) |
PD1 |
LQSP |
0.53 |
7 |
959.866 |
PD2 |
FLQG |
0.68 |
7 |
83.266 |
PD3 |
LLQG |
0.93 |
7 |
29.177 |
PD4 |
LLQGA |
1.10 |
5.52 |
32.441 |
PD5 |
LLQGP |
0.42 |
5.52 |
3496.919 |
PD6 |
LGQAAY |
0.483 |
5.52 |
65.780 |
PD7 |
PLQAVY |
0.567 |
5.95 |
1186.156 |
PD8 |
AFQAAY |
0.567 |
5.57 |
98.292 |
PD9 |
LVQRAY |
0.083 |
8.75 |
126.364 |
PD10 |
VLQRAY |
0.083 |
8.72 |
127.006 |
PD11 |
IGQSAY |
0.05 |
5.52 |
86.862 |
PD12 |
FYQAAY |
0.05 |
5.52 |
158.846 |
PD13 |
FWQAAY |
0.117 |
5.52 |
223.324 |
PD14 |
AFQSAY |
0.133 |
5.57 |
100.015 |
PD15 |
ILQRAY |
0.133 |
8.75 |
121.629 |
PD16 |
FMQSAY |
0.150 |
5.52 |
119.250 |
PD17 |
LPQAAY |
0.167 |
5.52 |
2203.871 |
Acyl donor |
Sequence |
Hydrophobicity |
Isoelectric point |
Steric energy (kcal mol−1) |
Control peptide (CP) |
CALNN |
0.22 |
5.25 |
533.6 |
Peptide substrate design
Acyl acceptors were based on literature reports and hydrophobicity modified to better suit the donor substrate.52–54 Acyl donors, tetrapeptides and pentapeptides mostly use excellent substrates reported in the literature.52,54 The hexapeptide was designed by taking Gln (Q) as the central residue, a peptide with a negative N-terminus and a positive C-terminus, which is according to the characteristics of amino acids near Gln (Q) from literature reports.55 Amino acids appearing at each position are shown in Table S1 in the ESI.† Acyl donor sequences were designed by permuting and combining amino acids at different positions. Hydrophobicities, isoelectric points and steric energy of peptides were tested on the ExPASy site (https://www.expasy.org/).
Molecular docking
Peptides were verified by AutoDock Vina,56 an open-source program available from the Centre of Computational Structural Biology at The Scripps Research Institute. The structure of mTGase used for docking is from Protein Data Bank (PDB), and the PDB ID of mTGase is 1iU4. The acyl donor with the strongest binding to mTGase, according to binding energy score, was selected. In the docking experiment, the number of modes used in the molecular docking is 20. The docking grid box spacing was set to 0.375 Å, the box was a cube of side length 40 grid points, which can cover all residue positions on the mTGase active sites, and is big enough for substrate docking. The active site center was set to −2.718, 22.138, −4.549 (x, y, z). Cys64, Asp255 and His274 were set as flexible fragments and docking within the active pocket evaluated using AutoDock Vina.56 Binding energies were accessed from the outputpdbqt file in the working directory. Pymol (an open-source molecular visualization system) was used to visualize the results of molecular docking.
Enzymatic reaction
Assessment of mTGase catalytic activity subjected to various substrates was completed by measuring changes in turbidity (optical density (OD) values at 600 nm) at 25 °C by TECAN Infinite200 PRO multifunctional enzyme standard (Tecan Trading AG, Switzerland).Various concentrations (0.0001, 0.001, 0.01, 0.1, 1 and 10 μM) of peptides PA1–PA4 were used as acyl acceptors and of PD6, PD8, PD10, PD12, PD14, PD15 as acyl donors. Acceptors and donors were paired one by one to form 24 pairs of reaction groups in 50 mM MES buffer, pH 6. Substrates were incubated with 26 μM mTGase at 40 °C for 30 min. Rates of gelation of 24 pairs of acceptor and donor peptides were measured by changes in OD600 values during various reaction times (0–60 min) when incubated with 26 μM mTGase at 40 °C. Activation of mTGase was assessed by using peptide pair PA4 + PD10 incubating with a range of mTGase concentrations (0.0026, 0.026, 0.26, 2.6, 26 and 260 μM) at 40 °C for 30 min. All these experiments were use CP (1 μM) as the negative control.
Optimal substrates under physiological conditions
The impact of pH and temperature change on enzyme activity with different substrates was investigated across the temperature range, 25–60 °C and the pH range, 5–9. In this assay, PBS was used instead of MES buffer to dissolve substrates because PBS can provide wider buffering range. Five peptide pairs, PA2 + PD14; PA3 + PD14; PA4 + PD10, PA4 + PD14, PA1 + PD14 and the natural substrate, collagen, were dissolved in 20 mM PBS to make 1 μM and 5 μM substrate concentrations and incubated with 26 μM mTGase. CP (1 μM) was used as the control. OD values at 600 nm were read after 60 min of reaction. Activation of mTGase under physiological conditions was assessed by incubating peptide pair, PA2 + PD14, with a range of mTGase concentrations (0.026, 0.13, 0.26, 1.3, 2.6, 13, 26 and 130 μM) in 20 mM PBS at 37 °C for 60 min. The natural substrate, collagen, was incubated with 0.26, 1.3, 2.6, 13, 26 and 130 μM mTGase in 20 mM PBS at 37 °C for 60 min. All these experiments used CP (1 μM) as the negative control.
Results and discussion
Peptide design and molecular docking
Acyl acceptor and acyl donor substrates were designed based on the structure of mTGase and with reference to enzyme–substrate interactions.52–55 The hydrophobicities, steric energy and isoelectric points of twenty-one peptides, including four acyl acceptors, PA1–PA4, and seventeen acyl donors, PD1–PD17, are shown in Table 1. Surface hydrophobicities of acceptors and donors were considered because only proteins with similar surface hydrophobicity are able to polymerize and crosslink.12,48 The smaller the steric energy, the easier it is for the acyl acceptor and acyl donor be in sufficient proximity for reaction and the higher the enzymatic reaction efficiency.48,52,55 Substrate requirements of mTGase towards acyl acceptors are considerably less strict than acyl donors due to the broad tolerance of the enzymes for the structural differences in acyl acceptors.12 For acyl acceptors, isoelectric points of acyl acceptors should be greater than the pH in the human body to ensure a positive charge and allow entry into the posterior part of the active pocket for biomedical engineering applications.48,50,57 mTGase is more selective for acyl donors, so 17 acyl donors were docked using AutoDock vina.12,56 The best scores of 17 conformation have been summarized in Table 2. The peptides achieving the best 6 conformational mean scores were PD6, PD8, PD12, PD14 PD10 and PD15. Based on the ranking of the best scores of 17 peptides, PD6, PD8, PD10, PD12, PD14, PD15 were selected as acyl donor substrates. Acyl acceptors, PA1–PA4, were used as acceptor substrates to pair with PD6, PD8, PD10, PD12, PD14, PD15 for enzymatic reactions, respectively. This result shows the ability of molecular docking technology to virtualize the recognition between the enzyme and substrate peptide designed on the basis of the active site and structural characteristics. According to the best score of docking results, the best substrate sequence was screened fast, which sets the foundation for further enzyme reaction experiments.
Table 2 Molecular docking of acyl donors
Acyl donors |
Sequence |
Score (kcal mol−1) |
1 |
LQSP |
−7.00 |
2 |
FLQG |
−6.29 |
3 |
LLQG |
−6.54 |
4 |
LLQGA |
−6.90 |
5 |
LLQGP |
−7.10 |
6 |
LGQAAY |
−8.24 |
7 |
PLQAVY |
−6.80 |
8 |
AFQAAY |
−8.01 |
9 |
LVQRAY |
−6.28 |
10 |
VLQRAY |
−7.32 |
11 |
IGQSAY |
−7.16 |
12 |
FYQAAY |
−7.83 |
13 |
FWQAAY |
−6.67 |
14 |
AFQSAY |
−7.58 |
15 |
ILQRAY |
−7.20 |
16 |
FMQSAY |
−6.66 |
17 |
LPQAAY |
−6.03 |
Enzymatic reactions of synthetic substrates
The four acyl acceptor and six acyl donor peptides showing strong binding to mTGase from molecular docking experiments were assayed for enzyme catalytic activity. Peptides were paired to form twenty-four groups as double substrate peptides for mTGase with CP as a negative control (as shown in Fig. 1).CALNN was used as CP due to there is no glutamine (acyl donor) and lysine (acyl acceptor) residues in the sequence can be recognized by mTGase active site for cross-linking. All peptide pairs reacted efficiently with mTGase, indicating entry into the mTGase reaction pocket and catalysis of the reaction between acyl donor and acyl acceptor producing cross-linking. Eight peptide pairs, PA4 + PD10, PA4 + PD15, PA3 + PD14, PA4 + PD14, PA3 + PD12, PA2 + PD14, PA1 + PD14 and PA3 + PD15, produced signal intensities for the catalyzed reaction which were more than 50% of the strongest signal (as shown in Fig. 1). For acyl receptors, PA4 is more easily recognized by mTGase, because it not only has the lowest steric energy but also two adjacent Lys (K) residue in the sequence which significantly increases enzymatic reactivity.58 For acyl donors, absolute binding energy values for PD10, PD12, PD14 and PD15 were all greater than 7 in molecular docking analyses. Thus, it can be concluded that all these acyl donors bound to the mTGase59 (as shown in Fig. 2 and Table 2). Enzymatic reactivities were also assessed for these peptide substrates. Pair PA4 + PD10 showed the highest activity. Enzymatic efficiency of acyl donor/acyl acceptor pairs in order of magnitude was: PA4 + PD10 > PA4 + PD15 > PA3 + PD14 > PA4 + PD14 > PA5 + PD12 > PA2 + PD14 > PA1 + PD14 > PA3 + PD15 (as shown in Fig. S2–S9 in the ESI†). These results conform to the comprehensive consideration of (1) the steric energy, (2) the distribution of active sites and (3) the principle that the more similar the hydrophobicity between acyl acceptor and acyl donor, the stronger the binding force and the higher the enzymatic reaction efficiency (as shown in Tables 1 and 2). The results of traditional experiments are consistent with the results of molecular docking, which confirms the reliability of molecular docking technology based on computer virtual.
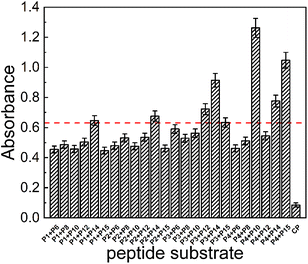 |
| Fig. 1 Four acyl acceptors and six acyl donors were paired one by one to form 24 pairs of reaction groups as double substrate peptides to react with mTGase in MES (50 mM, pH 6) buffer at 40 °C for 30 min. mTGase was present at a 26 μM concentration and the substrate pair at 1 μM. CALNN (CP) (1 μM) was used as a negative control. The red dotted line shows the location of 50% of the strongest OD600 value (PA4 + PD10). | |
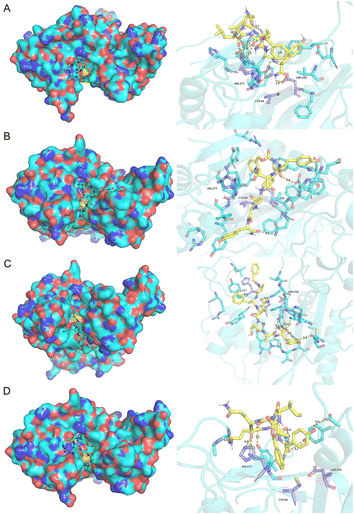 |
| Fig. 2 Molecular docking of acyl donors (A) PD10, (B) PD12, (C) PD14, (D) PD15 were visualized using Pymol. Acyl donors enter the active pocket (shown on the left) and the hydrogen bond interactions of the substrate with the active site residues Cys64, Asp255 and His274 (shown on the right). The distance (in angstroms) between atoms is shown by the dashed line. Absolute binding energy values for PD10, PD12, PD14 and PD15 were 7.32, 7.83, 7.58 and 7.20 kcal mol−1, respectively. | |
Use of peptides for quantitative detection of mTGase activity
The development of a highly sensitive mTGase detection assay would be of great value to avoid substrate flooding caused by nonspecific conjugation in biological samples. The cross-linking caused by mTGase catalyses acyl–transfer reactions will produce gels, which will lead to changes in the turbidity of the reaction solution. Therefore, the turbidity of the reaction solution can be used to characterize the efficiency of enzyme reaction.60 Experiments were designed to determine the detection limit of mTGase by monitoring the increase in gelation solution turbidity resulting from the gel formation using the newly-designed substrates. Enzymatic efficiencies of pairs, PA4 + PD10, PA4 + PD15, PA3 + PD14, PA4 + PD14, PA3 + PD12, PA2 + PD14, PA1 + PD14 and PA3 + PD15, are shown in Fig. 3(A). The signal intensity of the mixture of substrates and mTGase was increased by increasing reaction time and began to saturate after 30 min, indicating an effective gelation. To obtain a high sensitivity of assay, pair PA4 + PD10 was used as a peptide substrate in the following mTGase detection. As shown in Fig. 3(B), mTGase activity could be detected as low as 26 nM in the mTGase assay buffer. The detection signal was linear with respect to the logarithm of the mTGase concentration between 2.6 nM and 2.6 μM. This mTGase concentration is almost 3 orders of magnitude lower than those of 26 μM used in site specific conjugation in biomedical and bioengineering.19,60 Such a low concentration of mTGase would prevent non-specific binding, improve the efficiency of the enzymatic reaction, and achieve high-sensitivity detection of mTGase in the field of food safety. In addition, the experiment result confirms that the molecular docking-based assay could be used to design highly active substrates for the TGase family to improve the efficiency of cross-linking and protein modification, achieve high-sensitivity detection and diseases diagnosis, which will bring great benefits for biomedical applications.
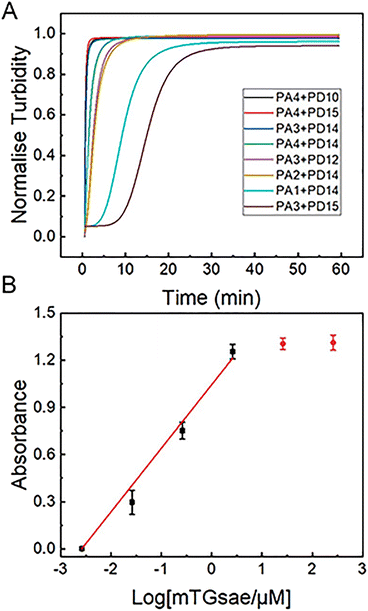 |
| Fig. 3 (A) Rate of gelation of 24 pairs of acceptor and donor peptides catalyzed by mTGase measured by changes in OD600 values after various reaction times (0–60 min). 26 μM mTGase and 1 μM peptide pair were present. (B) Logarithmic plots of the integrated OD600 values as a function of the concentration of mTGase (0.0026, 0.026, 0.26, 2.6, 26 and 260 μM) in MES buffer (50 mM, pH 6). The concentration of peptide pair, PA4 + PD10, was 1 μM. Average OD600 value of CP has been subtracted from signal value (as shown in Fig. 1). | |
Activity of designed substrates under physiological conditions
The impact of pH and temperature change on enzyme activity with the peptides have also been measured since the activity of substrates and the catalytic efficiency of mTGase is strongly dependent on its environment. In particular, 37 °C and pH 7.4 were emphasized since many diseases detection and the bioengineering applications need to be carried out under physiological conditions. The results of 5 groups of substrate peptides, PA4 + PD10, PA3 + PD14, PA4 + PD14, PA2 + PD14 and PA1 + PD14, and natural substrate collagen, across the pH range, 5–9 and temperature range, 25–60 °C were shown in Fig. 4 (PA3 + PD12, PA4 + PD15 and PA3 + PD15 were not studied, since the acyl donors PD12 and PD15 were unstable with pH change). Enzymatic activity with all pairs of substrates varied with temperature and pH, producing slightly stronger effects for the synthetic peptides than for the natural substrates. The peptide pair, P2 + P14, produced the highest enzyme activity under physiological conditions (37 °C, pH 7.4).
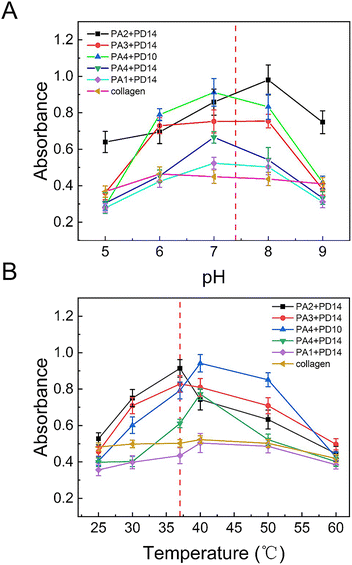 |
| Fig. 4 (A) The activity of mTGase with five peptide pairs, PA2 + PD14; PA3 + PD14; PA4 + PD10, PA4 + PD14, PA1 + PD14 and collagen, across pH 5 to 9. (B) The activity of mTGase with five peptide pairs, PA2 + PD14; PA3 + PD14; PA4 + PD10, PA4 + PD14, PA1 + PD14 and collagen, across temperature 25 °C to 60 °C. The concentration of mTGase was 26 μM. The concentration of PA2 + PD14, PA3 + PD14, PA4 + PD10, PA4 + PD14 and PA1 + PD14 was 1 μM in 20 mM PBS buffer. The concentration of collagen was 5 μM. The red dotted line shows the location of physiological conditions (37 °C and pH 7.4). Average OD600 value of CP has been subtracted from signal value (as shown in Fig. 1). | |
The synthetic peptide pair, P2 + P14, which produced the highest enzyme activity under physiological conditions, was compared with collagen, the preferred natural substrate for mTGase61,62 (as shown in Fig. 5). Under physiological conditions, the P2 + P14 substrate pair allowed detection of mTGase activity at concentrations as low as 130 nM. The signal intensity increased linearly with increasing mTGase concentration between 130 nM to 26 μM and saturation was observed above 26 μM. The detection limit of mTGase was 2.6 μM with a collagen substrate. Thus, mTGase activity showed a 20-fold enhancement with the synthetic peptide pair compared with collagen. The experiment demonstrates the potential for TGase-related disease diagnosis under physiological conditions. Meanwhile, after considering the unfavourable behaviours of peptides on pharmacokinetics (e.g. plasma stability and membrane permeability), this method can also provide a new idea for drug discovery. Furthermore, design of synthetic peptides by this method may replace natural protein substrates, excluding adverse effects of non-specific reactions and showing great promise for the field of biomedical engineering.
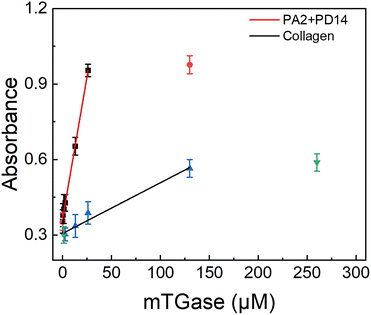 |
| Fig. 5 Plots of integrated OD600 values as a function of the concentration of mTGase (0.026, 0.13, 0.26, 1.3, 2.6, 13, 26 and 130 μM) incubated with P2 + P14, and mTGase (1.3, 2.6, 13, 26, 130 and 260 μM) incubated with collagen in 20 mM PBS at 37 °C for 60 min. The concentration of peptide pair P2 + P14 was 1 μM and of collagen 5 μM. Average OD600 value of CP has been subtracted from signal value (as shown in Fig. 1). | |
Conclusions
We have developed a high activity substrates design method based on molecular docking and experimental assay for sensitive profiling the activity of mTGase. The ability to design high activity substrates under physiological conditions provides a distinct advantage for mTGase assays. We have demonstrated the utility of the combination of molecular docking and traditional experiments in designing highly active enzyme substrates. The proof-of-concept study opens an avenue for further exploring highly sensitive detection method of TGase family, providing more accurate results for the diagnosis of related diseases and drug discovery. This approach will strongly supplement existing enzymatic techniques and provide a potential tool with a high throughput format for screening high activity substrates under physiological conditions.
Author contributions
Tao Li contributed to conception, design of the study and finalization of manuscript. Longhao Zou did a major part of molecular docking, data analysis, assisted in enzyme experiments and wrote the first draft of the manuscript. Xu Geng developed the major enzyme experiment and wrote sections of the manuscript. Zengqiang Li thoroughly reviewed the manuscript. All authors contributed to manuscript revision, read and approved the submitted version.
Conflicts of interest
The authors declare that the research was conducted in the absence of any commercial or financial relationships that could be construed as a potential conflict of interest.
Acknowledgements
They authors would like to express their gratitude to the science and technology development program of Jilin Province (No. 20210204187YY and No. 20200301029RQ) for financial support and to EditSprings (https://www.editsprings.cn) for the expert linguistic services provided.
References
- D. D. Clarke, M. J. Mycek, A. Neidle and H. Waelsch, Arch. Biochem. Biophys., 1959, 79, 338–354 CrossRef CAS.
- J. J. Pisano, J. S. Finlayson and M. P. Peyton, Science, 1968, 160, 892–893 CrossRef CAS PubMed.
- T. Kanaji, H. Ozaki, T. Takao, H. Kawajiri, H. Ide, M. Motoki and Y. Shimonishi, J. Biol. Chem., 1993, 268, 11565–11572 CrossRef CAS PubMed.
- S. Del Duca, S. Beninati and D. Serafini-Fracassini, Biochem. J., 1995, 305(Pt 1), 233–237 CrossRef CAS PubMed.
- R. N. Singh and K. Mehta, Eur. J. Biochem., 1994, 225, 625–634 CrossRef CAS PubMed.
- J. Zhang and Y. Masui, Mol. Reprod. Dev., 1997, 47, 302–311 CrossRef CAS PubMed.
- H. Yasueda, Y. Kumazawa and M. Motoki, Biosci., Biotechnol., Biochem., 1994, 58, 2041–2045 CrossRef CAS PubMed.
- E. G. Puszkin and V. Raghuraman, J. Biol. Chem., 1985, 260, 16012–16020 CrossRef CAS PubMed.
- L. Fesus, A. Madi, Z. Balajthy, Z. Nemes and Z. Szondy, Experientia, 1996, 52, 942–949 CrossRef CAS PubMed.
- V. Gentile, V. Thomazy, M. Piacentini, L. Fesus and P. J. Davies, J. Cell Biol., 1992, 119, 463–474 CrossRef CAS PubMed.
- R. A. Jones, B. Nicholas, S. Mian, P. J. Davies and M. Griffin, J. Cell Sci., 1997, 110(Pt 19), 2461–2472 CrossRef CAS PubMed.
- C. S. Greenberg, P. J. Birckbichler and R. H. Rice, FASEB J., 1991, 5, 3071–3077 CrossRef CAS PubMed.
- M. Lesort, J. Tucholski, M. L. Miller and G. V. W. Johnson, Prog. Neurobiol., 2000, 61, 439–463 CrossRef CAS PubMed.
- W. Dieterich, T. Ehnis, M. Bauer, P. Donner, U. Volta, E. O. Riecken and D. Schuppan, Nat. Med., 1997, 3, 797–801 CrossRef CAS PubMed.
- P. J. Birckbichler, G. R. Orr, E. Conway and M. K. Patterson, Jr., Cancer Res., 1977, 37, 1340–1344 CAS.
- R. N. Barnes, P. J. Bungay, B. M. Elliott, P. L. Walton and M. Griffin, Carcinogenesis, 1985, 6, 459–463 CrossRef CAS PubMed.
- M. Griffin, L. L. Smith and J. Wynne, Br. J. Exp. Pathol., 1979, 60, 653–661 CAS.
- N. M. Rachel and J. N. Pelletier, Biomolecules, 2013, 3, 870–888 CrossRef PubMed.
- S. E. Farias, P. Strop, K. Delaria, M. G. Casas, M. Dorywalska, D. L. Shelton, J. Pons and A. Rajpal, Bioconjugate Chem., 2014, 25, 240–250 CrossRef CAS PubMed.
- S. Jeger, K. Zimmermann, A. Blanc, J. Grunberg, M. Honer, P. Hunziker, H. Struthers and R. Schibli, Angew. Chem., Int. Ed., 2010, 49, 9995–9997 CrossRef CAS PubMed.
- P. Dennler, A. Chiotellis, E. Fischer, D. Bregeon, C. Belmant, L. Gauthier, F. Lhospice, F. Romagne and R. Schibli, Bioconjugate Chem., 2014, 25, 569–578 CrossRef CAS PubMed.
- S. Dickgiesser, M. Rieker, D. Mueller-Pompalla, C. Schroter, J. Tonillo, S. Warszawski, S. Raab-Westphal, S. Kuhn, T. Knehans, D. Konning, J. Dotterweich, U. A. K. Betz, J. Anderl, S. Hecht and N. Rasche, Bioconjugate Chem., 2020, 31, 1070–1076 CrossRef CAS PubMed.
- D. Fiebig, S. Schmelz, S. Zindel, V. Ehret, J. Beck, A. Ebenig, M. Ehret, S. Frols, F. Pfeifer, H. Kolmar, H. L. Fuchsbauer and A. Scrima, J. Biol. Chem., 2016, 291, 20417–20426 CrossRef CAS PubMed.
- Y. Sugimura, M. Hosono, F. Wada, T. Yoshimura, M. Maki and K. Hitomi, J. Biol. Chem., 2006, 281, 17699–17706 CrossRef CAS PubMed.
- Y. Sugimura, K. Yokoyama, N. Nio, M. Maki and K. Hitomi, Arch. Biochem. Biophys., 2008, 477, 379–383 CrossRef CAS PubMed.
- K. Hitomi, M. Kitamura and Y. Sugimura, Amino Acids, 2009, 36, 619–624 CrossRef CAS PubMed.
- X. Zheng, P. Pu, B. T. Ding, W. C. Bo, D. Y. Qin and G. Z. Liang, Food Chem., 2021, 362, 9 Search PubMed.
- E. Bilen, U. O. Ozmen, S. Cete, S. Alyar and A. Yasar, Chem.-Biol. Interact., 2022, 360, 14 CrossRef PubMed.
- M. J. M. Ridhwan, S. I. Abu Bakar, N. Abd Latip, N. Ab Ghani and N. H. Ismail, J. Comput. Biophys. Chem., 2022, 21, 259–285 CrossRef CAS.
- Y. L. Li, S. Y. Zhang, Z. J. Bao, N. Sun and S. Y. Lin, Innovative Food Sci. Emerging Technol., 2022, 76, 10 CrossRef.
- S. Taj, M. Ahmad and U. A. Ashfaq, Int. J. Biol. Macromol., 2022, 207, 507–521 CrossRef CAS PubMed.
- A. J. Gerrard, S. E. Fayle, P. A. Brown, K. H. Sutton, L. Simmons and I. Rasiah, J. Food Sci., 2001, 66, 782–786 CrossRef.
- P. Trespalacios and R. Pla, Food Chem., 2007, 100, 264–272 CrossRef CAS.
- X. F. Zhou, Y. R. Zheng, Y. Zhong, D. F. Wang and Y. Deng, Food Chem., 2022, 383, 14 CrossRef PubMed.
- M. Kieliszek and A. Misiewicz, Folia Microbiol., 2014, 59, 241–250 CrossRef CAS PubMed.
- J. Cortez, P. L. R. Bonner and M. Griffin, Enzyme Microb. Technol., 2004, 34, 64–72 CrossRef CAS.
- M. M. Taylor, L. Bumanlag, W. N. Marmer and E. M. Brown, J. Am. Leather Chem. Assoc., 2006, 101, 169–178 CAS.
- G. C. Du, L. Cui, Y. Zhu and J. Chen, Enzyme Microb. Technol., 2007, 40, 1753–1757 CrossRef CAS.
- M. H. Kamani, J. Semwal and A. M. Khaneghah, Colloids Surf., B, 2022, 211, 15 CrossRef PubMed.
- N. Doti, A. Caporale, A. Monti, A. Sandomenico, F. Selis and M. Ruvo, World J. Microbiol. Biotechnol., 2020, 36, 14 CrossRef PubMed.
- M. C. Echave, C. Pimenta-Lopes, J. L. Pedraz, M. Mehrali, A. Dolatshahi-Pirouz, F. Ventura and G. Orive, Int. J. Pharm., 2019, 562, 151–161 CrossRef CAS PubMed.
- C. V. L. Giosafatto, A. Fusco, A. Al-Asmar and L. Mariniello, Int. J. Mol. Sci., 2020, 21, 17 Search PubMed.
- D. Gupta, J. W. Santoso and M. L. McCain, Bioengineering, 2021, 8, 16 CrossRef PubMed.
- H. Schneider, L. Deweid, O. Avrutina and H. Kolmar, Anal. Biochem., 2020, 595, 13 CrossRef PubMed.
- X. Li and D. D. Fan, ACS Biomater. Sci. Eng., 2019, 5, 3523–3536 CrossRef CAS PubMed.
- Y. Zhu and J. Tramper, Trends Biotechnol., 2008, 26, 559–565 CrossRef CAS PubMed.
- P. Strop, Bioconjugate Chem., 2014, 25, 855–862 CrossRef CAS PubMed.
- S. K. Chan and T. S. Lim, Appl. Microbiol. Biotechnol., 2019, 103, 2973–2984 CrossRef CAS PubMed.
- C. W. Yung, L. Q. Wu, J. A. Tullman, G. F. Payne, W. E. Bentley and T. A. Barbari, J. Biomed. Mater. Res., Part A, 2007, 83, 1039–1046 CrossRef CAS PubMed.
- M. P. Savoca, E. Tonoli, A. G. Atobatele and E. A. M. Verderio, Micromachines, 2018, 9, 23 CrossRef PubMed.
- A. N. Zelikin, C. Ehrhardt and A. M. Healy, Nat. Chem., 2016, 8, 997–1007 CrossRef CAS PubMed.
- M. T. Gundersen, J. W. Keillor and J. N. Pelletier, Appl. Microbiol. Biotechnol., 2014, 98, 219–230 CrossRef CAS PubMed.
- A. Caporale, F. Selis, A. Sandomenico, G. S. Jotti, G. Tonon and M. Ruvo, Biotechnol. J., 2015, 10, 154–161 CrossRef CAS PubMed.
- L. Deweid, O. Avrutina and H. Kolmar, Methods Mol. Biol., 2019, 2012, 151–169 CrossRef CAS PubMed.
- J. H. Lee, C. Song, D. H. Kim, I. H. Park, S. G. Lee, Y. S. Lee and B. G. Kim, Biotechnol. Bioeng., 2013, 110, 353–362 CrossRef CAS PubMed.
- O. Troott and A. J. Olson, J. Comput. Chem., 2010, 31, 455–461 Search PubMed.
- U. Tagami, N. Shimba, M. Nakamura, K. Yokoyama, E. Suzuki and T. Hirokawa, Protein Eng., Des. Sel., 2009, 22, 747–752 CrossRef CAS PubMed.
- M. Malesevic, A. Migge, T. C. Hertel and M. Pietzsch, ChemBioChem, 2015, 16, 1169–1174 CrossRef CAS PubMed.
- L. L. Yang, D. Y. Li, Y. B. Zhang, M. Y. Zhu, D. Chen and T. D. Xu, Acta Pharmacol. Sin., 2012, 33, 41–48 CrossRef CAS PubMed.
- R. C. Deller, T. Richardson, R. Richardson, L. Bevan, I. Zampetakis, F. Scarpa and A. W. Perriman, Nat. Commun., 2019, 10, 1887–1897 CrossRef PubMed.
- J. G. Fernandez, S. Seetharam, C. Ding, J. Feliz, E. Doherty and D. E. Ingber, Tissue Eng., Part A, 2017, 23, 135–142 CrossRef CAS PubMed.
- R. N. Chen, H. O. Ho and M. T. Sheu, Biomaterials, 2005, 26, 4229–4235 CrossRef CAS PubMed.
|
This journal is © The Royal Society of Chemistry 2023 |