DOI:
10.1039/D3NH00165B
(Minireview)
Nanoscale Horiz., 2023,
8, 1523-1528
Specific interaction based drug loading strategies
Received
29th April 2023
, Accepted 1st August 2023
First published on 4th August 2023
Abstract
Drug carriers have been commonly used for drug control release, enhancing drug efficacy and/or minimizing side-effects. However, it is still difficult to get a high loading efficiency when encapsulating super hydrophilic drugs with a narrow therapeutic index, such as many neurotoxins. Increasing the carrier proportion can improve drug loading to a certain degree, while the burst released drug when the formulation enters the body may cause overdose side-effects. Moreover, high-dose carriers themselves may increase the metabolic burden of the body. Hence, new drug carriers and/or loading strategies are urgently needed to promote the applications of these drugs. This minireview will introduce drug loading strategies based on specific interactions (between drugs and carriers) and will discuss the challenges and perspectives of these strategies. This work is expected to provide alternative inspiration for the delivery of hydrophilic drugs.
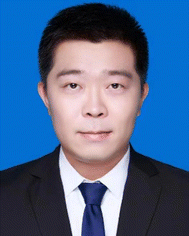
Tianjiao Ji
| Tianjiao Ji received his Ph.D. (2016) from the National Center for Nanoscience and Technology (NCNST) under the supervision of Prof. Guangjun Nie. He finished postdoctoral research under the supervision of Prof. Daniel Kohane (Boston Children's Hospital, Harvard Medical School). He is now a principal investigator at NCNST. His research interests focus on the construction of functional supramolecular assemblies for drug delivery and pain management. |
1. Introduction
Nanoscale drug delivery systems (DDSs) have been applied in the treatment of clinical diseases,1–6 especially in cancer therapy.3–6 Rationally designed drug carriers can improve the solubility of drugs,7,8 enhance drug targeting,9–12 increase drug penetration and/or realize drug-controlled release.13–17 Several types of materials have been investigated to construct DDSs, including organic materials such as phospholipids,18,19 polymers,20–22 biological membranes,23–25 viruses,26 and bacteria,27–29 and inorganic materials such as gold and silicon nanoparticles,30–33 as well as hybrid materials.34,35
Drugs can be loaded in/on the nanocarriers through several approaches. For example, most drugs can be physically encapsulated by liposomes or polymer nanospheres;19,20,34 poorly water soluble (hydrophobic) small-molecule drugs can be encapsulated in the hydrophobic regions of micelles and liposomes through hydrophobic forces;18,21 nucleic acid drugs can be complexed with positively charged carriers through electrostatic interactions;36–38 some drugs can also be conjugated with carriers through chemical bonds.39,40 However, for some super hydrophilic drugs with a narrow therapeutic index, such as tetrodotoxin (TTX),41 efficient loading is challenging due to their strong hydrophilicity, which makes them prone to leakage from carriers. Increasing the portion of carrier materials may improve the drug loading and slow down the spontaneous drug release in vitro, but the burst release in the initial stages when the formulation enters the body may cause overdose side-effects. In addition, a large amount of carrier materials may cause tissue inflammation and toxicity.20,42,43
In another case, peptide drugs with inherent targeting properties should not be encapsulated in the carriers, but conjugating them to the surface of carriers may reduce their targeting activity and efficacy.44–46 Besides, nucleic acid drugs may detach from the carriers during transportation in the body since the electrostatic adsorption is not stable in the complicated physiological environment.47,48 Therefore, new carriers and/or loading strategies need to be developed to achieve effective loading of these mentioned drugs.
In biological systems, most of the information exchanges occur based on the specific interactions between molecules. For example, enzymes recognize their corresponding substrate;49 DNA and RNA exchange information through complementary base pairing;50,51 the COVID-19 virus infects the host through the binding ACE2 receptor.52 Similarly, most drugs have specific binding regions in the targeting molecules.53–55 For example, doxorubicin (a chemotherapeutic drug), which inserts into the double-strand of DNA molecules,54 and lidocaine (a local anesthetic) binds the inner site of sodium ion channels.55 These specific interactions have inspired researchers to construct DDSs using peptide and nucleic acid motifs selected from drug targeting regions, which may achieve specific drug loading.56 This review will introduce two major specific interaction-based drug loading strategies (Scheme 1): (1) a strategy inspired by the interactions between drugs and their targets, and (2) a strategy inspired by the interactions from life processes, and this work is expected to provide insights for nanoscale DDS construction.
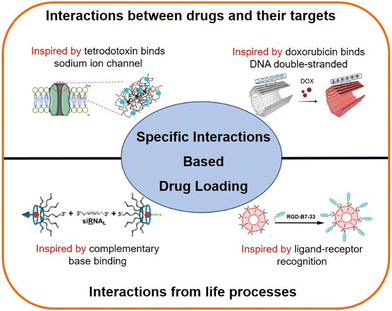 |
| Scheme 1 Schematic illustration of the content of this mini-review. The specific interactions for drug loading can be from (1) drugs and their targets, such as tetrodotoxin with a sodium channel and doxorubicin with DNA molecules, and (2) biomolecular recognition, such as complementary base binding and ligand–receptor recognition. | |
2. Construction of DDSs inspired by the interactions between drugs and their targets
Typically, the binding between drug molecules and their targets occurs due to a matching structural region,53 generating multiple supramolecular interactions such as hydrogen bonds, hydrophobic forces, and electrostatic interactions,54 which is crucial for achieving drug efficacy. Extracting motifs of the regions (e.g. peptides or nucleic acids) that interact with drugs to construct drug carriers, holds great possibility to achieve specific loading and sustained release of the drug. For instance, Ji et al. selected peptide sequences from the binding site of tetrodotoxin (TTX) (Fig. 1A and B) with a sodium ion channel protein to construct the drug carrier that can improve the TTX loading efficiency.56 However, since the selected peptide sequences were hydrophilic, directly mixing them with TTX in the solution resulted in low probability to generate supramolecular interactions, making it difficult to load and control release TTX. By modifying the binding sequences with hydrophobic domains (fatty acids or hydrophobic amino acids), the constructed amphiphilic peptides could self-assemble into nanofibers. The hypothesis was that the enrichment of the binding sequences to the nanoscale structure might facilitate supramolecular interactions between the binding (peptide) sequences and TTX (Fig. 1C). Nonetheless, the interactions between binding sequences could affect TTX loading, and only large volumes of hydrophobic modifications (modification with hydrophobic amino acids) (Fig. 1D) can effectively avoid excessive interactions of binding sequences themselves (as shown in Fig. 1E, with less β-sheet conformation detected using the circular dichroism spectrum), so that the TTX could bind to the nanostructures and then got the controlled release effect (Fig. 1F). Mutating key amino acids or scrambling the binding sequence would also affect the nanofibers to bind TTX, indicating that the nanofibers from the optimized group had specificity in binding TTX. In addition, the nanofibers could compete with TTX primary antigens in ELISA kits (in binding TTX) (Fig. 1G), which further showed the TTX binding belonged to a specific interaction. This designed nanocarrier could provide 16 h local anesthesia, which is seven times longer than that of free TTX, and the therapeutic index was significantly expanded as well.
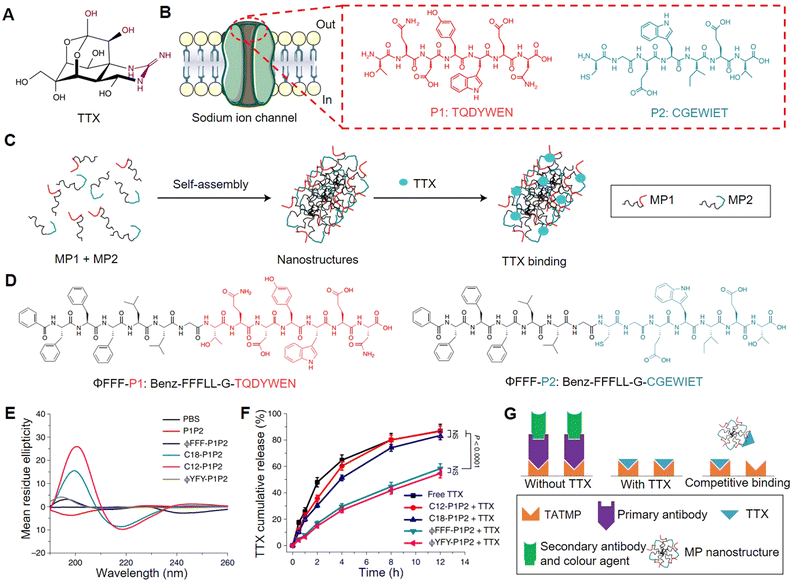 |
| Fig. 1 Ion channel derived nanocarrier design and its specific loading for tetrodotoxin (TTX). (A) Molecular structure of TTX. (B) Sodium ion channel and the binding site, key binding sequences (P1 and P2) with TTX. (C) Schematic illustration of the hydrophobic modified peptides (MP1 and MP2) self-assembly and TTX binding. (D) Molecular structures of the optimized amphiphilic peptide pair (ϕFFF-P1 and ϕFFF-P2). (E) Conformation of each peptide group detected using the circular dichroism spectrum. (F) TTX release profile of each formulation. (G) Competitive binding (peptide assembly with a TTX primary antibody) experiment carried out though ELISA kit. This figure has been adapted from ref. 56 with permission from Springer Nature, copyright 2021. | |
For more examples, Li team developed nanoparticles by assembling doxorubicin, siRNA and iron ions (Fig. 2A), enabling the efficient co-delivery of doxorubicin and nucleic acid drug (Fig. 2B and C).57 In this nanosystem, doxorubicin could stabilize the nanoparticle, possibly because of the specific interactions of nucleic acid molecules with doxorubicin. Similarly, Ding's group used a DNA origami nanosystem to effectively load doxorubicin (Fig. 2D) for cancer treatment.58
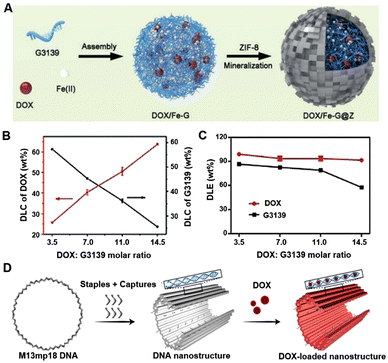 |
| Fig. 2 Nucleic acid materials for doxorubicin (DOX) loading. (A) Schematic illustration of the co-assembly construction. (B) Drug loading content (DLC) of DOX (red line) and G3139 (black line). (C) Drug loading efficiency (DLE) of DOX and G3139 in the NPs with different added ratios of DOX: G3139 during the assembly. (D) The construction of DOX loaded DNA origami structure. (A) and (B) have been adapted from ref. 57 with permission from Wiley-VCH, Copyright 2019, (D) has been adapted from ref. 58 with permission from Wiley-VCH Copyright 2020. | |
3. Drug carrier design inspired by specific molecular interactions from life processes
Almost all life processes rely on the specific molecular interactions among biomolecules in organisms, such as antibody–antigen recognition, and the complementary base pairing of nucleic acids.50,59,60 These interactions can be applied in the design of DDSs. For instance, B7-33 is a peptide drug having anti-fibrotic properties through targeting relaxin-receptors and block their interaction with TGF-β.61 However, its hydrophilicity and short half-life in the body limit the therapeutic efficacy. If B7-33 is encapsulated in a carrier, its targeting capability will be shielded, and hydrophobic modifications (such as conjugating with a fatty acid) may compromise its function. To overcome these issues, Zhang et al. added the cRGD sequence to the end of the B7-33 (forming RGD-B7-33), which did not affect the anti-fibrosis efficacy of B7-33.44 With the cRGD sequence, the RGD-B7-33 could be loaded onto the human umbilical vein endothelial cell (HUVEC) cell membrane derived nano-carriers through the specific interactions of cRGD with integrins (overexpressed on the HUVEC membrane) (Fig. 3A). This approach effectively prolonged the circulation time of B7-33 in the body, and improved its inhibition of fibrotic microenvironment caused by the activated liver stellate cells (Fig. 3B and C), and thereby effectively inhibiting pancreatic cancer liver metastasis.
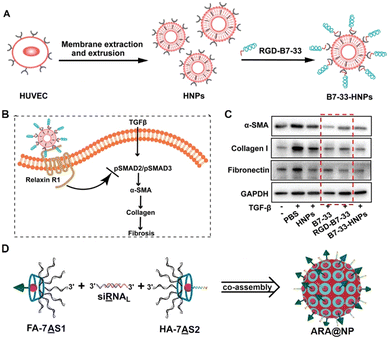 |
| Fig. 3 Construction of drug carriers inspired by specific molecular interactions from life processes. (A) Illustration of the construction of the ligand–receptor interaction-based therapeutic peptide (B7-33) delivery system (B7-33-HNPs). (B) The anti-fibrotic mechanism of B7-33-HNPs. (C) The in vitro anti-fibrotic efficacy evaluation of different formulations. The red dashed box showed that the function of B7-33 was not reduced after cRGD sequence modification. (D) Construction of the co-assembly from the specific interaction of branched antisense and siRNA. FA: folate for targeting; HA: influenza hemagglutinin peptide for endosomal escape. (A)–(C) have been adapted from ref. 44 with permission from Wiley-VCH, Copyright 2022, (D) has been adapted from ref. 62 with permission from Wiley-VCH, Copyright 2020. | |
Ding's group extended the 3′ end of siRNA with a specific base sequence that can interact specifically with the corresponding DNA sequence (pair with the siRNA) modified cyclodextrin, resulting in a multifunctional assembly (Fig. 3D).63 These nanoparticles could target tumors and achieve lysosomal escape. Subsequently, the DNA sequences of siRNA and mRNA antisense chains can work synergistically to silence the tumor-specific gene (PLK1), achieving the enhanced anti-tumor effect.
4. Discussion and perspectives
Previous reports have shown that nanomaterials containing toxin/protein receptors can be used to remove toxins/virus.64–69 For instance, Zhang's group coated neuronal cell membranes to PLGA nanoparticles to construct a nanosponge that specifically neutralized neurotoxins, because the neuronal cell membrane contained large amount of neurotoxin receptors (ion channel proteins).68 They also used nanosponges rich in ACE2 to neutralize the SARS-CoV-2 virus, significantly reducing the risk of host cells being invaded by the virus.69 Actually, these strategies hold the same theory of this mini-review.
Extracting the binding sequences to construct drug carriers seems a simplified strategy compared to preparing cell membrane derived nanomaterials. However, it poses several major challenges. Firstly, the conformation of the binding (peptide) sequences may be difficult to maintain after they are detached from the proteins, making it hard to establish a relatively strong supramolecular interaction with the drug. Secondly, even if the constructed nanocarrier can generate strong supramolecular interactions with the drug, the actual binding manners are extremely challenging to be directly characterized. Thirdly, the binding of the drug with its target may be contributed by multiple of peptide sequences, so that the constructed carrier (if only one or two binding sequences is included) cannot provide sufficient interactions to effectively load the drug. Fourthly, it is challenging to avoid interactions between peptide sequences themselves in the nanoscale space when constructing the drug carrier, and if the inner supramolecular interaction of the carrier is too strong, it would be hard for drugs to load on the carriers.
Despite these challenges, the existing work (highlighted in this review) has demonstrated the feasibility of constructing carriers based on specific interactions. We believe that well-designed experimental control groups (providing enough molecular candidates for screening), coupled with computer simulation (or artificial intelligence)-assisted optimization, can promote the applications of this drug loading strategy. By taking experiments, we can screen out the carrier with the best drug-controlled release effect by adjusting or mutating the peptide sequences; by using computer simulation, we can screen out the drug–carrier pair with the lowest binding energy by docking the drug molecule with the carrier molecule.
Of course, all the applications of DDSs must always follow the principles of safety and effectiveness. In fact, the binding of drugs with their targets, as well as the binding with carriers, are in a dynamic process after the DDSs are administered into the body. If the interactions of the drug with the carrier are too strong, the released drug may not reach the effective dose. In contrast, if the drug is released too rapidly (the interactions between the drug and the carrier are too weak), it may generate side effects. Therefore, the in vivo safety and effectiveness are the most important criteria for DDS evaluation.
Compared to carrier design based on drug–target interactions, using receptor–ligand inspiration for carrier design presents fewer challenges. The main challenge might be the scale-up production of the carrier, which is the common challenge faced by most nanoscale drug formulations. Besides, relatively strong supramolecular interactions would occur between two different types of drug molecules, and these two drugs could form nanostructures through self-assembly.70 This phenomenon probably is induced by some specific interactions between two drugs. However, most of these interactions are driven by hydrophobic forces. Thus, we did not include this field in this work.
In summary, this mini-review introduced a drug loading strategy that relies on specific interactions, which may offer feasible references for constructing the DDSs for some special drugs, such as the super hydrophilic drugs with narrow therapeutic index.
Conflicts of interest
The authors declare no financial/commercial conflicts of interests.
Acknowledgements
This work was supported by the National Key Research and Development Program of China (2021YFA0909900), the National Natural Science Foundation of China (32271391 and 82101286), and the Beijing Natural Science Foundation (Z220022).
References
- A. Akinc, M. A. Maier, M. Manoharan, K. Fitzgerald, M. Jayaraman, S. Barros, S. Ansell, X. Du, M. J. Hope, T. D. Madden, B. L. Mui, S. C. Semple, Y. K. Tam, M. Ciufolini, D. Witzigmann, J. A. Kulkarni, R. van der Meel and P. R. Cullis, Nat. Nanotechnol., 2019, 14, 1084–1087 CrossRef CAS PubMed.
- C. Wang, Y. Zhang and Y. Dong, Acc. Chem. Res., 2021, 54, 4283–4293 CrossRef CAS PubMed.
- Y. Barenholz, J. Controlled Release, 2012, 160, 117–134 CrossRef CAS PubMed.
- K. A. Autio, R. Dreicer, J. Anderson, J. A. Garcia, A. Alva, L. L. Hart, M. I. Milowsky, E. M. Posadas, C. J. Ryan, R. P. Graf, R. Dittamore, N. A. Schreiber, J. M. Summa, H. Youssoufian, M. J. Morris and H. I. Scher, JAMA Oncol., 2018, 4, 1344–1351 CrossRef PubMed.
- M. Yu, W. Yang, W. Yue and Y. Chen, Adv. Sci., 2022, 9, 2204335 CrossRef CAS.
- P. Zhang, Y. Xiao, X. Sun, X. Lin, S. Koo, A. V. Yaremenko, D. Qin, N. Kong, O. C. Farokhzad and W. Tao, Med, 2023, 4, 147–167 CrossRef CAS PubMed.
- K. M. Au, A. Z. Wang and S. I. Park, Sci. Adv., 2020, 6, eaaz9798 CrossRef CAS PubMed.
- X. Chen, Y. Cheng, Q. Pan, L. Wu, X. Hao, Z. Bao, X. Li, M. Yang, Q. Luo and H. Li, ACS Nano, 2023, 17, 3705–3722 CrossRef CAS PubMed.
- D. Zeng, Z. Ma, X. Zan, T. Luo, X. Wang, X. Gao and X. Fu, Chin. Chem. Lett., 2023 DOI:10.1016/j.cclet.2023.108433.
- S. Chen, Y. Zhong, W. Fan, J. Xiang, G. Wang, Q. Zhou, J. Wang, Y. Geng, R. Sun, Z. Zhang, Y. Piao, J. Wang, J. Zhuo, H. Cong, H. Jiang, J. Ling, Z. Li, D. Yang, X. Yao, X. Xu, Z. Zhou, J. Tang and Y. Shen, Nat. Biomed. Eng., 2021, 5, 1019–1037 CrossRef CAS PubMed.
- Q. Wan, B. Huang, T. Li, Y. Xiao, Y. He, W. Du, B. Z. Wang, G. F. Dakin, M. Rosenbaum, M. D. Goncalves, S. Chen, K. W. Leong and L. Qiang, Nat. Nanotechnol., 2022, 17, 1311–1321 CrossRef CAS PubMed.
- S. Waheed, Z. Li, F. Zhang, A. Chiarini, U. Armato and J. Wu, J. Nanobiotechnol., 2022, 20, 395 CrossRef PubMed.
- C. Zheng, D. Zhang, Y. Kong, M. Niu, H. Zhao, Q. Song, Q. Feng, X. Li and L. Wang, Exploration, 2023, 20220124 CrossRef.
- T. Ji, J. Lang, J. Wang, R. Cai, Y. Zhang, F. Qi, L. Zhang, X. Zhao, W. Wu, J. Hao, Z. Qin, Y. Zhao and G. Nie, ACS Nano, 2017, 11, 8668–8678 CrossRef CAS PubMed.
- W. Li, J. Chen, S. Zhao, T. Huang, H. Ying, C. Trujillo, G. Molinaro, Z. Zhou, T. Jiang, W. Liu, L. Li, Y. Bai, P. Quan, Y. Ding, J. Hirvonen, G. Yin, H. A. Santos, J. Fan and D. Liu, Nat. Commun., 2022, 13, 1262 CrossRef CAS PubMed.
- J. Ouyang, A. Xie, J. Zhou, R. Liu, L. Wang, H. Liu, N. Kong and W. Tao, Chem. Soc. Rev., 2022, 51, 4996–5041 RSC.
- N. Kong, R. Zhang, G. Wu, X. Sui, J. Wang, N. Y. Kim, S. Blake, D. De, T. Xie, Y. Cao and W. Tao, Proc. Natl. Acad. Sci. U. S. A., 2022, 119, e2112696119 CrossRef CAS.
- J. Lu, X. Liu, Y.-P. Liao, F. Salazar, B. Sun, W. Jiang, C. H. Chang, J. Jiang, X. Wang, A. M. Wu, H. Meng and A. E. Nel, Nat. Commun., 2017, 8, 1811 CrossRef PubMed.
- Y. Chen, Y. Zhang, B. Wang, Q. Fan, Q. Yang, J. Xu, H. Dai, F. Xu and C. Wang, ACS Nano, 2023, 17, 760–774 CrossRef CAS.
- Z. Kang, C. Wang, Z. Zhang, Q. Liu, Y. Zheng, Y. Zhao, Z. Pan, Q. Li, L. Shi and Y. Liu, Adv. Mater., 2022, 34, 2201945 CrossRef CAS.
- J. Wang, Z. Wang, G. Chen, Y. Wang, T. Ci, H. Li, X. Liu, D. Zhou, A. R. Kahkoska, Z. Zhou, H. Meng, J. B. Buse and Z. Gu, ACS Nano, 2021, 15, 4294–4304 CrossRef CAS PubMed.
- J. Ouyang, B. Deng, B. Zou, Y. Li, Q. Bu, Y. Tian, M. Chen, W. Chen, N. Kong, T. Chen and W. Tao, J. Am. Chem. Soc., 2023, 145, 12193–12205 CrossRef CAS PubMed.
- C.-M. J. Hu, L. Zhang, S. Aryal, C. Cheung, R. H. Fang and L. Zhang, Proc. Natl. Acad. Sci. U. S. A., 2011, 108, 10980–10985 CrossRef CAS PubMed.
- M. Ying, J. Zhuang, X. Wei, X. Zhang, Y. Zhang, Y. Jiang, D. Dehaini, M. Chen, S. Gu, W. Gao, W. Lu, R. H. Fang and L. Zhang, Adv. Funct. Mater., 2018, 28, 1801032 CrossRef PubMed.
- B. Bahmani, H. Gong, B. T. Luk, K. J. Haushalter, E. DeTeresa, M. Previti, J. Zhou, W. Gao, J. D. Bui, L. Zhang, R. H. Fang and J. Zhang, Nat. Commun., 2021, 12, 1999 CrossRef CAS PubMed.
- S. Kler, R. Zalk, A. Upcher and I. Kopatz, Nanoscale, 2022, 14, 11535–11542 RSC.
- Z. Xiang, G. Jiang, D. Fan, J. Tian, Z. Hu and Q. Fang, Nanoscale, 2020, 12, 13513–13522 RSC.
- H. Chen, M. Zhou, Y. Zeng, T. Miao, H. Luo, Y. Tong, M. Zhao, R. Mu, J. Gu, S. Yang and L. Han, Adv. Sci., 2022, 9, 2105854 CrossRef CAS PubMed.
- Y. Li, Q. Leng, Y. Zhang, S. Lin, Q. Wen, Y. Lu, K. Xiong, H. Shi, Y. Liu, S. Xiao, L. Zhao, J. Wu, Z. Qian and S. Fu, Chem. Eng. J., 2022, 438, 135566 CrossRef CAS.
- L. Wang, C. Zhang, T. Li, M. Duan, F. Xia, X. Li, C. Song, S. Pan, B. Liu and D. Cui, Nanoscale, 2019, 11, 22237–22242 RSC.
- S. Varela-Aramburu, C. Ghosh, F. Goerdeler, P. Priegue, O. Moscovitz and P. H. Seeberger, ACS Appl. Mater. Interfaces, 2020, 12, 43380–43387 CrossRef CAS PubMed.
- P. Mora-Raimundo, D. Lozano, M. Manzano and M. Vallet-Regí, ACS Nano, 2019, 13, 5451–5464 CrossRef CAS PubMed.
- B. Ruehle, D. L. Clemens, B.-Y. Lee, M. A. Horwitz and J. I. Zink, J. Am. Chem. Soc., 2017, 139, 6663–6668 CrossRef CAS PubMed.
- M. J. W. Evers, S. I. van de Wakker, E. M. de Groot, O. G. de Jong, J. J. J. Gitz-François, C. S. Seinen, J. P. G. Sluijter, R. M. Schiffelers and P. Vader, Adv. Healthcare Mater., 2022, 11, 2101202 CrossRef.
- C. Wang, Y. Yu, M. Irfan, B. Xu, J. Li, L. Zhang, Z. Qin, C. Yu, H. Liu and X. Su, Small, 2020, 16, 2002578 CrossRef CAS PubMed.
- J. Lang, X. Zhao, Y. Qi, Y. Zhang, X. Han, Y. Ding, J. Guan, T. Ji, Y. Zhao and G. Nie, ACS Nano, 2019, 13, 12357–12371 CrossRef CAS PubMed.
- X. Han, H. Zhang, K. Butowska, K. L. Swingle, M.-G. Alameh, D. Weissman and M. J. Mitchell, Nat. Commun., 2021, 12, 7233 CrossRef CAS PubMed.
- O. S. Fenton, K. J. Kauffman, J. C. Kaczmarek, R. L. McClellan, S. Jhunjhunwala, M. W. Tibbitt, M. D. Zeng, E. A. Appel, J. R. Dorkin, F. F. Mir, J. H. Yang, M. A. Oberli, M. W. Heartlein, F. DeRosa, R. Langer and D. G. Anderson, Adv. Mater., 2017, 29, 1606944 CrossRef PubMed.
- V. Basavalingappa, S. Bera, B. Xue, I. Azuri, Y. Tang, K. Tao, L. J. W. Shimon, M. R. Sawaya, S. Kolusheva, D. S. Eisenberg, L. Kronik, Y. Cao, G. Wei and E. Gazit, Nat. Commun., 2019, 10, 5256 CrossRef PubMed.
- C. Li, J. Lang, Y. Wang, Z. Cheng, M. Zu, F. Li, J. Sun, Y. Deng, T. Ji, G. Nie and Y. Zhao, Acta Pharm. Sin. B, 2023 DOI:10.1016/j.apsb.2023.03.024.
- Q. Liu, C. M. Santamaria, T. Wei, C. Zhao, T. Ji, T. Yang, A. Shomorony, B. Y. Wang and D. S. Kohane, Nano Lett., 2018, 18, 32–37 CrossRef CAS PubMed.
- C. Zhao, A. Liu, C. M. Santamaria, A. Shomorony, T. Ji, T. Wei, A. Gordon, H. Elofsson, M. Mehta, R. Yang and D. S. Kohane, Nat. Commun., 2019, 10, 2566 CrossRef.
- C. Weldon, T. Ji, M.-T. Nguyen, A. Rwei, W. Wang, Y. Hao, C. Zhao, M. Mehta, B. Y. Wang, J. Tsui, R. P. Marini and D. S. Kohane, ACS Nano, 2019, 13, 18–25 CrossRef CAS PubMed.
- L. Zhang, Z. Li, F. Wang, Q. Chen, M. Zu, X. Li, J. Wan, X. Yao, X. Lou, Y. Shi, Y. Sheng, M. Wang, J. Yang, X. Wang, Z. Qin and T. Ji, Adv. Funct. Mater., 2022, 32, 2208404 CrossRef CAS.
- L. Zhang, Y. Qi, H. Min, C. Ni, F. Wang, B. Wang, H. Qin, Y. Zhang, G. Liu, Y. Qin, X. Duan, F. Li, X. Han, N. Tao, L. Zhang, Z. Qin, Y. Zhao and G. Nie, ACS Nano, 2019, 13, 5091–5102 CrossRef CAS PubMed.
- Y. Wang, J. Zhan, J. Huang, X. Wang, Z. Chen, Z. Yang and J. Li, Interdiscip. Med., 2023, 1, e20220005 Search PubMed.
- Z.-G. Lu, J. Shen, J. Yang, J.-W. Wang, R.-C. Zhao, T.-L. Zhang, J. Guo and X. Zhang, Signal Transduction Targeted Ther., 2023, 8, 39 CrossRef CAS PubMed.
- Y. Liu, C.-F. Xu, S. Iqbal, X.-Z. Yang and J. Wang, Adv. Drug Delivery Rev., 2017, 115, 98–114 CrossRef CAS PubMed.
- H. Huang, D. Zhang, Y. Weng, K. Delaney, Z. Tang, C. Yan, S. Qi, C. Peng, P. A. Cole, R. G. Roeder and Y. Zhao, Sci. Adv., 2021, 7, eabe2771 CrossRef CAS PubMed.
- Y. Deng, Y. Tan, Y. Zhang, L. Zhang, C. Zhang, Y. Ke and X. Su, ACS Appl. Mater. Interfaces, 2022, 14, 34470–34479 CrossRef CAS PubMed.
- S. Hoshika, N. A. Leal, M.-J. Kim, M.-S. Kim, N. B. Karalkar, H.-J. Kim, A. M. Bates, N. E. Watkins, H. A. SantaLucia, A. J. Meyer, S. DasGupta, J. A. Piccirilli, A. D. Ellington, J. SantaLucia, M. M. Georgiadis and S. A. Benner, Science, 2019, 363, 884–887 CrossRef CAS PubMed.
- S. Gangadevi, V. N. Badavath, A. Thakur, N. Yin, S. De Jonghe, O. Acevedo, D. Jochmans, P. Leyssen, K. Wang, J. Neyts, T. Yujie and G. Blum, J. Phys. Chem. Lett., 2021, 12, 1793–1802 CrossRef CAS PubMed.
- M. Zafferani, C. Haddad, L. Luo, J. Davila-Calderon, L.-Y. Chiu, C. S. Mugisha, A. G. Monaghan, A. A. Kennedy, J. D. Yesselman, R. J. Gifford, A. W. Tai, S. B. Kutluay, M.-L. Li, G. Brewer, B. S. Tolbert and A. E. Hargrove, Sci. Adv., 2021, 7, eabl6096 CrossRef CAS PubMed.
- X. Qiao, S. Y. van der Zanden, D. P. A. Wander, D. M. Borras, J. Y. Song, X. Li, S. van Duikeren, N. van Gils, A. Rutten, T. van Herwaarden, O. van Tellingen, E. Giacomelli, M. Bellin, V. Orlova, L. G. J. Tertoolen, S. Gerhardt, J. J. Akkermans, J. M. Bakker, C. L. Zuur, B. Pang, A. M. Smits, C. L. Mummery, L. Smit, R. Arens, J. Li, H. S. Overkleeft and J. Neefjes, Proc. Natl. Acad. Sci. U. S. A., 2020, 117, 15182–15192 CrossRef CAS PubMed.
- H. Hermanns, M. W. Hollmann, M. F. Stevens, P. Lirk, T. Brandenburger, T. Piegeler and R. Werdehausen, Br. J. Anaesth., 2019, 123, 335–349 CrossRef CAS PubMed.
- T. Ji, Y. Li, X. Deng, A. Y. Rwei, A. Offen, S. Hall, W. Zhang, C. Zhao, M. Mehta and D. S. Kohane, Nat. Biomed. Eng., 2021, 5, 1099–1109 CrossRef CAS PubMed.
- B. Liu, F. Hu, J. Zhang, C. Wang and L. Li, Angew. Chem., Int. Ed., 2019, 58, 8804–8808 CrossRef CAS PubMed.
- Z. Wang, L. Song, Q. Liu, R. Tian, Y. Shang, F. Liu, S. Liu, S. Zhao, Z. Han, J. Sun, Q. Jiang and B. Ding, Angew. Chem., Int. Ed., 2021, 60, 2594–2598 CrossRef CAS PubMed.
- H. J. Sijben, G. Superti-Furga, A. P. Ijzerman and L. H. Heitman, Trends Pharmacol. Sci., 2022, 43, 358–361 CrossRef CAS PubMed.
- W. Ma, Y. Yang, J. Zhu, W. Jia, T. Zhang, Z. Liu, X. Chen and Y. Lin, Adv. Mater., 2022, 34, 2109609 CrossRef CAS PubMed.
- M. A. Hossain, M. Kocan, S. T. Yao, S. G. Royce, V. B. Nair, C. Siwek, N. A. Patil, I. P. Harrison, K. J. Rosengren, S. Selemidis, R. J. Summers, J. D. Wade, R. A. D. Bathgate and C. S. Samuel, Chem. Sci., 2016, 7, 3805–3819 RSC.
- J. Liu, X. Lu, T. Wu, X. Wu, L. Han and B. Ding, Angew. Chem., Int. Ed., 2021, 60, 1853–1860 CrossRef CAS PubMed.
- J. Liu, C. Chen, T. Wei, O. Gayet, C. Loncle, L. Borge, N. Dusetti, X. Ma, D. Marson, E. Laurini, S. Pricl, Z. Gu, J. Iovanna, L. Peng and X.-J. Liang, Exploration, 2021, 1, 21–34 CrossRef PubMed.
- C.-M. J. Hu, R. H. Fang, J. Copp, B. T. Luk and L. Zhang, Nat. Nanotechnol., 2013, 8, 336–340 CrossRef CAS PubMed.
- C.-M. J. Hu and L. Zhang, Nano Today, 2014, 9, 401–404 CrossRef CAS PubMed.
- L. Sun, D. Wang, I. Noh, R. H. Fang, W. Gao and L. Zhang, Angew. Chem., Int. Ed., 2023, e202301566 CAS.
- J. Zhou, N. Krishnan, Z. Guo, C. J. Ventura, M. Holay, Q. Zhang, X. Wei, W. Gao, R. H. Fang and L. Zhang, Sci. Adv., 2022, 8, eabq5492 CrossRef CAS PubMed.
- D. Wang, X. Ai, Y. Duan, N. Xian, R. H. Fang, W. Gao and L. Zhang, ACS Nano, 2022, 16, 19145–19154 CrossRef CAS PubMed.
- X. Ai, D. Wang, A. Honko, Y. Duan, I. Gavrish, R. H. Fang, A. Griffiths, W. Gao and L. Zhang, J. Am. Chem. Soc., 2021, 143, 17615–17621 CrossRef CAS PubMed.
- S. Fu, G. Li, W. Zang, X. Zhou, K. Shi and Y. Zhai, Acta Pharm. Sin. B, 2022, 12, 92–106 CrossRef CAS PubMed.
Footnote |
† These authors made equal contributions to this work. |
|
This journal is © The Royal Society of Chemistry 2023 |
Click here to see how this site uses Cookies. View our privacy policy here.