DOI:
10.1039/D3NA00260H
(Paper)
Nanoscale Adv., 2023,
5, 4873-4880
Progress in magnetic particle imaging signal and iron quantification methods in vivo – application to long circulating SPIONs
Received
21st April 2023
, Accepted 17th August 2023
First published on 18th August 2023
Abstract
The strengths of Magnetic Particle Imaging (MPI) lay in its sensitivity, quantitative nature, and lack of signal attenuation for Superparamagnetic Iron Oxide Nanoparticles (SPION). These advantages make MPI a powerful tool for the non-invasive monitoring of tracer behaviour over time. With more MPI studies emerging, a standardized method for determining the boundaries of a region of interest (ROI) and iron quantification is crucial. The current approaches are inconsistent, making it challenging to compare studies, hindering MPI progression. Here we showcase three different ROI selection methods for the quantification of iron in vivo and ex vivo. Healthy mice were intravenously administered a long circulating tracer, never before applied in MPI, and the ROI methods were tested for their ability to accurately quantify the total signal present, in addition to the accumulation of the tracer in individual organs. We discuss how the quantified iron amount can be vastly altered based on the choice of ROI, the importance of the standard curve and the challenges associated with each method. Lastly, the user variability and accuracy of each method was compared by 3 independent users to ensure their consistency and lack of bias.
Introduction
Magnetic Particle Imaging (MPI) has risen as a powerful imaging modality due to its high sensitivity and lack of signal depth attenuation. The quantitative nature of MPI is a favourable attribute, with the signal generated from Superparamagnetic Iron Oxide Nanoparticles (SPION) directly correlating to the amount of iron present. This quantification allows for the monitoring of tracer behaviour in vivo across various applications such as cancer imaging, cell tracking and inflammation imaging.1–4 With the growth in MPI usage, agreement on a reliable and user-independent method to process and quantify MPI signals from 2D and 3D images is crucial. The majority of in vivo MPI studies describe either a region of interest (ROI) determined manually or by growing an ROI from a seed of the maximum signal, the quantified signal is then converted to an iron amount by using a standard curve.5–8 However, there is often minimal description of how the borders of the manual ROI is determined, how large the seed is grown to or how the standard curve is generated, making the current standard of analysis user-dependent and difficult for study comparison. Furthermore, other studies do not quantify the signal at all or directly compare the MPI signal, which is inaccurate when comparing different tracers as their sensitivities vary.9,10
Sehl et al., described how the quantification of iron can differ based on the defined ROI and sample volume.11 This is particularly true in situations where small amounts of iron are distributed in single large volumes. The study compares the following four methods: (1) an ROI grown at a scaling factor of 0.5 times the maximum value within the signal of interest. (2) A line profile was drawn through the maximum signal and the maximum distance between the curvature was multiplied by a scaling factor of 2 to determine the diameter of a circular ROI. (3) All images were analysed with the largest circular ROI determined from method 2. And finally, (4) the standard deviation of system noise (SD) was determined from an empty sample holder, then a ROI segment was generated with values five times the SD. For in vitro and in vivo quantification, the study found methods 3 and 4 to have the greatest accuracy in quantifying the iron, establishing two powerful and consistent methods. The study focused on quantifying a single signal generated from ferucarbotran-labelled stem cells in vivo. However, the methods do not explore the feasibility of quantifying signals from multiple and separate areas within the animal while the tracer is in circulation.
The current class of MPI tracers clear rapidly through the hepatic system within minutes, resulting in a strong liver signal only and therefore does not present a challenge for analysis.4,5 However, as these tracers continue to improve through metal doping and polymer coatings, their circulation will extend and produce more complex MPI images.12,13 Further, in the case of intravenously (IV) administered SPION, the tracer is distributed across the vasculature and different organs, increasing the signal's bleed and making it difficult to accurately determine the size of a ROI and therefore quantify the amount of iron. To support the growth of MPI research, it is important to establish a precise, reproducible and universal analysis method that is user-independent for accurate iron quantification based on MPI signals throughout the entire body and individual organs. Standardized methods can help enhance the field of MPI, leading to more accurate comparison between studies and different tracers.
Expanding on the work by Sehl et al., we report the analysis of a long circulating SPIONs and its quantification in organs. We apply three different ROI selection methods that are most suited for signal quantification of 3D MPI scans in vivo and ex vivo. Methods 1 and 3 are used to quantify the total amount of iron in vivo in healthy mice following IV administration of a long circulating SPION; while method 2 expands on method 1 to discriminate individual organs in vivo. Method 1 sets an ROI based on the image noise characteristics or SD while Method 3 draws an ROI across the entire animal. Method 2 applies custom freehand drawn ROIs within the image to monitor the tracers' dynamics within organs over time. For iron quantification of ex vivo MPI scans of the animal's organs, methods 1 and 3 were used. Our methods describe how to accurately quantify the total signal for particles in circulation based on the chosen ROI and standard curve, reiterating the importance of method standardization.
Materials and methods
mPEG Coated PrecisionMRX® SPIONs (mPEG NP) were provided courtesy of Imagion Biosystems. mPEG NPs are spherical, 24–26 nm in core size and coated in a monolayer of oleic acid, amphiphilic polymer and methoxypolyethylene glycol (9.43 mg of Fe/ml). DLS measurements were performed to determine the hydrodynamic size (nm), polydisperse index (PDI) and surface charge (zeta potential in mV).
MPI relaxation and signal standard curve
To generate a standard curve a 9-well phantom bed was used with each well holding 10 μl of serially diluted iron amounts of mPEG NP in water (100 μg–5 μg). 2D and 3D MPI scans of the phantom were performed using a Magnetic Insight Momentum CT scanner with the standard sequence and isotropic acquisition. Each of the three ROI selection methods mentioned in the following section were used to measure the MPI signals of each sample. The iron amounts and corresponding signal were then plotted on a linear regression to produce the standard curves.
Relaxometry scans of the mPEG NP were performed to measure the sensitivity and resolution using the RELAX module on the momentum MPI, automatically generating Point Spread Function (PSF) data. Resolution (mm) corresponds to the Full-Width Half-Maximum value (FWHM) divided by the gradient. Sensitivity (a.u./μg) is the peak signal of the PSF adjusted to the amount of iron.
MPI in vivo measurements
All animal procedures were approved by the Alfred Research Alliance Animal Ethics Committee, Monash University (approval E/8208/2022/M). Ten-week-old female C57BL/6 mice were injected through the tail vein with 0.5 mg Fe of mPEG NP (n = 4). MPI scans were performed on the Magnetic Insight Momentum Scanner 1, 6 and 24 hours post-tracer administration, using the standard sequence, isotropic acquisition, and in 3D mode with 21 projections. During the scan, mice were under isoflurane anesthesia (2–3%) with breathing maintained at 50–80 breaths per minute. Body temperature was monitored using a rectal fibre optic thermal probe and maintained with a supply of warm air. Fiducials were prepared in plastic tubing with 2% and 4% of the injected mPEG NP dose (9.43 μg and 18.86 μg). Following the final scan, animals were euthanized and 2D standard sequence, isotropic acquisition ex vivo MPI scans of the liver, spleen, kidney, heart, lung, and muscle were performed. In vivo images are presented as maximum intensity projections while ex vivo images are single 2D images.
ROI analysis methods
All image analysis was completed on MagImage Image Analysis Software (Magnetic Insight). Signals were quantified as a sum of scalar values which is the mean MPI signal (a.u.) within the ROI multiplied by the number of pixels. The values were then converted to iron amounts using the corresponding standard curve.
Method 1: ROI threshold set by image noise characteristics (continuation of method 4 by Sehl, et al.)
Using the in vivo 3D image, a profile line was placed on a blank region within the field of view (FOV); in between the edge of the image and the animal. The highest signal in that region was determined to be the standard deviation of system noise (SD). To determine the lowest true MPI signal (Signalmin), the SD was multiplied by scaling factor k, where k = 3, 5, or 10.14 Using the threshold tool, a segment ROI was created capturing all voxels greater than Signalmin. This was repeated for each individual scan. For ex vivo samples, the ROI was set directly on the 2D images.
Method 2: ROI threshold set by image noise characteristics and freehand discrimination
For quantification of individual organs in vivo, a mask was created based on the Signalmin and Signalmax using k = 5. The mask segment was then converted to a 2D image, therefore creating a single image with only true MPI signals and no background. A freehand ROI was then drawn around the individual distinguishable organs (liver, heart, and carotid artery).
Method 3: freehand drawn ROI
The in vivo 3D image was converted to a Sum of Projection Image (SPI) were the total signal at every voxel across each projection was summed to create a single 2D image. A freehand ROI was then drawn across the entire animal, as defined by the user, measuring the total signal. For ex vivo samples, the ROI was drawn directly on the 2D images.
User variability
For user variability, the data was analysed by three different users individually across the three methods in vivo (liver region for method 2) and in the liver for ex vivo scans. The pairwise difference of the means was measured between users and the average difference between them were quantified. In addition, the average of the standard error of measurement (SEM) and the coefficient of variance (CoV) between users mean values was calculated.
Statistical analysis
All statistical analysis was performed on GraphPad Prism v9. Differences across the timepoints were assessed by a One-Way ANOVA Test followed by Tukey's multiple comparison test. Difference between both timepoints and methods were assessed by a Two-Way ANOVA followed by Šídák's multiple comparisons test. A P-Value ≤ 0.05 was considered statistically significant. Standard curves were interpolated as simple linear regression passing through X and Y = 0.
Results and discussion
Two MPI signal standard curves were generated based on the methods described (Fig. 1a and b). Both approaches demonstrated a strong linear relationship between the signal quantified and amount of iron (r2 > 0.99). Method 1 and 2 use the same standard curve. The specific sensitivity and resolution values (Table 1) of the mPEG NP was determined by the PSF generated from the MPI RELAX module (Fig. 1d and e). DLS was also used to characterize the NP properties (Table 1).
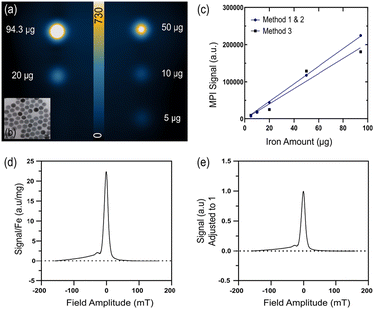 |
| Fig. 1 a) Phantom MPI scan with known amounts of mPEG NP (94.3 μg–5 μg). Scale bar unit (a.u.). (b) Representative TEM of core NP. (c) Standard curves of known iron amounts and corresponding MPI signal generated for each of the 3 ROI methods. (d–e) PSF derived from MPI RELAX scan of mPEG NP. (d) MPI sensitivity of mPEG NP with signal normalized to iron amount. (e) Resolution of mPEG NP with calculated from the full-width half-maximum value of the PSF. | |
Table 1 DLS and MPI characterization of mPEG Coated PrecisionMRX® SPION
Core size (nm) |
24–25 |
Hydrodynamic size (nm) |
80–90 |
Polydisperse index (PDI) |
<0.10 |
Zeta potential (mV) |
−15 to −10 |
MPI sensitivity (a.u./mg Fe) |
≈25 |
MPI resolution (mm) |
≈2.30 |
As MPI research gains more momentum and in vivo applications grow, it is critical to define analysis procedures for accurate comparison between various pathological studies and the development of different tracers. Currently a standardized method does not exist primarily due to the ongoing challenge of defining the borders of the ROI. Analysis of MPI scans increase in complexity between subcutaneous and intravenous injections, particularly when a SPION is in circulation and is distributed across a large volume, unlike a tracer that immediately clears into the liver producing a single signal. Further, although the MPI signal is directly correlated to the amount of iron present, without a precise and consistent ROI selection method the amount of iron can be grossly over or under-estimated, as we will demonstrate in this study. Finally, the sensitivity of a SPION can widely vary with surface modifications and therefore its quantification, making it important to know a tracers sensitivity value before in vivo experimentation.
Biodistribution of mPEG NP
Three ROI selection methods were compared in healthy animals IV administered mPEG NP, with their biodistribution monitored across three different timepoints in vivo (Fig. 2a) and ex vivo in the major organs (Fig. 2b). Visually, at the one hour timepoint the tracer's presence was evident across the animal's circulatory system including the abdominal aorta, caudal aorta and carotid arteries and was also present in the heart, lungs, liver, and spleen. The signal in the heart indicates the high blood pool and ongoing circulation of the tracer. However, in the following timepoints, the particle cleared from circulation into the liver and spleen through the hepatic system, a well characterized behaviour of SPION.15,16 These findings were similarly found in the ex vivo scans, indicated by tracer accumulation in the liver, spleen, and kidney, all organs involved in SPION clearance. Minimal presence of mPEG NP in the heart and lung tissue, and no presence in the muscle tissue, was observed. The mPEG NP presented here are a polymeric coated SPION, which aids in significantly extending the circulation time, continuing 6 hours after administration. This is substantially longer than many reported SPION, completely clearing into the liver within ten minutes such as ferucarbotran, commercially available as Vivotrax.5 In addition to a tracer's resolution, longer circulating particles make it easier to distinguish between organs, allowing for more effective imaging applications and analysis of tracer dynamics over time.
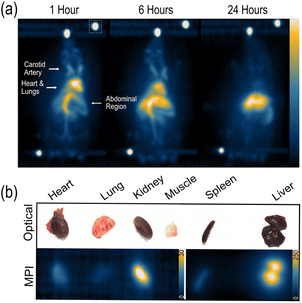 |
| Fig. 2 (a) Maximum intensity projection of healthy mice intravenously administered mPEG NP across 3 different time points. Dashed white box represents fiducials. (b) Representative 2D ex vivo MPI scans and optical image of mice organs 24 hours post IV. Note scale difference between the two images to best visually present signal. Scale bar unit (a.u.). | |
In vivo quantification using method 1
A crucial finding of this study is to match the MPI acquisition settings of the in vivo scan to that of the standard curve's, otherwise the acquired signals will be of different magnitudes and quantification will be inaccurate. Although we apply the following methods on 3D MPI scans, they can be replicated on 2D scans as long as the standard curve is created identically. However, 3D scans are more powerful in distinguishing the tracer's distribution in different areas and provides more information than a 2D scan on the relative distance between signals. The 3D scan can also be used to manually outline an area slice by slice, creating a 3D volume.17 This could be useful for detecting signals from areas separate to the primary signal of the heart and liver, such as a tumour. Outlining the borders of the organs from a co-registered CT scan on an MPI scan is another potential method for accurate ROI delineation, similar to PET analysis.18,19 However, this method will take time to gain popularity as most MPI scanners are yet to have a CT scanner installed.
Method 1 was first applied to quantify the total signal generated from the animal, plotted as sum of scalar value then converted to iron using its corresponding standard curve (Fig. 3). Sehl et al. calculated SD from a background scan of blank sample and applying that value to the in vivo scan. This method works well for a short circulating SPION that is localised in a discrete area. A longer circulating SPION creates issues with this analysis step due to signal bleed. All SPIONs display some amount of signal bleed, which is closely related to the properties of the particle itself. When a SPION is circulating, the signal bleed is spread across the entire image, and not just around a discrete area. In many cases the signal bleed is above the k = 5 value, resulting in over quantification if the Sehl et al. method 4 is followed. Our application for circulating SPIONs is to determine the background signal from within the acquisition image to adjust for the large amount of signal bleed. Using the in vivo 3D image, a profile line was placed on the blank region of the field of view (FOV); in between the edge of the image and the animal. The highest signal in that region was determined to be the standard deviation of system noise (SD) (Fig. 3a).
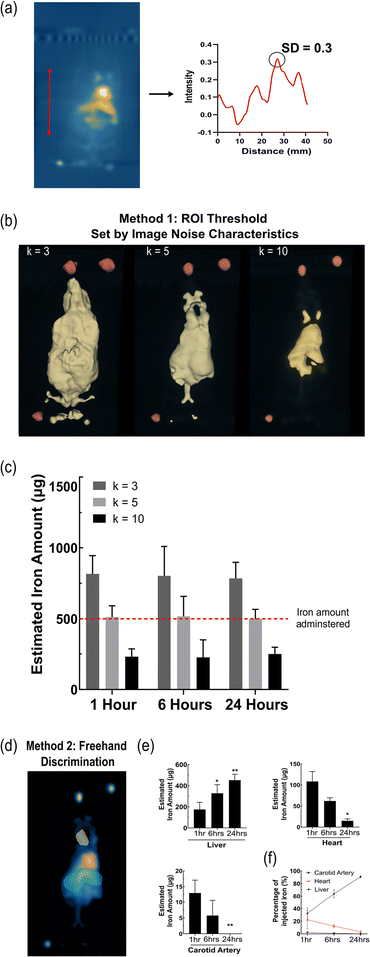 |
| Fig. 3 (a) Demonstration of profile line and determined SD value. (b) Representative images of ROI selection method 1 using k = 3, 5, 10 for whole body in vivo quantification. (c) Quantified MPI signal across the whole body and at each timepoint using method 1. (d) Representative images of ROI selection method 2 over carotid artery (yellow), heart (red) and liver (blue). (e) Quantified MPI signal in respective organ areas across 3 timepoints. (f) Quantified percentage change of MPI signal in the liver, heart, and carotid artery area in relation to whole signal using method 1 and 2. Data presented as mean ± SD (n = 4). P-Values in comparison to timepoint 1 hour. * P-value < 0.05, ** P-value < 0.01. | |
Three different scaling values were tested (k = 3, 5, 10) to determine the most accurate threshold size of the segment applied (Fig. 3b and c). K = 3 approximated 60% of the iron amount injected creating a large ROI, including increased SPION bleed and noise. While a k = 10 estimated 50% less, producing a smaller ROI and acquiring the least amount of signal. The most accurate signal and iron estimation was with a k value of 5, a 2–3% difference. A scaling value of k = 5 provided the best measurement of signal to noise ratio and is most closely aligned with the Rose Criterion model, generating the most accurate quantification of the total body signal.14,20 It was also apparent that across the 3 time points there was no significant difference in the amount of tracer present systemically, although mostly having cleared from circulation into the liver. Only k = 5 was able to accurately quantify the total injected iron dose across the timepoints, unlike k = 3 introducing increased bleed or k = 10 acquiring minimal true signal. A major challenge of method 1, however, is its inability to determine the signal from individual clusters given only a single ROI is generated. This is primarily related to the tracer's resolution and the ability to resolve separate signals from different organs and overlapping larger vessels. Our results are consistent with Sehl et al. in that k = 5 gives the most accurate quantification of iron.
In vivo quantification using method 2
For method 2, which expands on method 1, a 2D mask was generated from the 3D segment using the scaling factor k = 5 (Fig. 3d). The mask creates an image with only true MPI signals, offering the freedom to select any ROI and monitor the same region over time. Individual ROIs could be custom drawn across distinguishable organs; the liver, heart and carotid artery and the same sized ROIs can be maintained across the three timepoints for consistency, with slight adjustments for the shift of the animal (Fig. 3e). As expected, there was a significant increase in the amount of tracer accumulating in the liver area after 1 hour through to the 24 hour timepoint. Conversely, the signals from the heart and carotid artery decreased over time, as less of the particles were circulating. At 1 hour, 32% of the tracer is in the liver, 2.4% in the carotid artery and 22% in the heart, accounting for 57% of the tracer with the remainder still in ongoing circulation. By 24 hours, 90% of the total iron content is in the liver area, with negligible amounts in the carotid artery and 3% in the heart (Fig. 3e and f). It is important to note that the selected ROI gives an estimate to the amount of tracer present in that area at a given time however, it does not discreetly quantify the amount of tracer within the organ. An ongoing challenge is defining the precise borders of the organs the MPI image. Therefore, here the “liver” describes the abdominal region which may also include the spleen, kidneys and surrounding vasculature. The “heart” and “carotid artery” act as rough estimates for the amount of tracer in the blood pool that is circulating. As mentioned, the resolution of the tracer plays an important role in this aspect. The distinct accumulation and greater resolution a tracer has, makes defining the ROI considerably more reliable given the borders can be more effectively determined. As a result, a clear limitation of this method is the possible inter-user variability causing variation in the signal quantified. One good practice is to define a ROI at an early timepoint when blood circulation is at its highest and apply the same ROI over time. Another method would be to compare the signals at the ROI as a percentage of the whole signal from the animal, monitoring the change over time (Fig. 3f). It provides a valid method when comparing the biodistribution properties of different tracers and, as we demonstrate, the liver and heart could be used to indicate the clearance rate of the tracer from circulation. Describing the accumulation in organs as percentages ensures the amount quantified is adjusted to true amount of SPION injected and still in circulation.
In vivo quantification using method 3
Method 3 adopts a more straightforward approach, by simply converting the 3D image to a 2D SPI (Fig. 4a). The total signal was measured by using a single freehand drawn ROI, which greatly overestimated the total iron by over 200% and was more challenging to distinguish organs from each other (Fig. 4b). Method 3 is highly user dependent and is not reliable to retrieve the total injected dose.
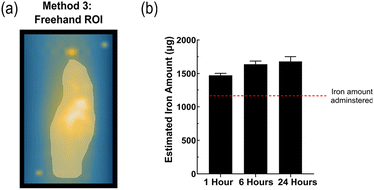 |
| Fig. 4 (a) Representative images of ROI selection method 3. (b) Quantified MPI signal across the whole body and at each timepoint using method 2. Data presented as mean ± SD (n = 4). | |
Ex vivo quantification using method 1 and method 3
Ex vivo MPI scans on the organs were performed to provide an additional quantification of the tracer biodistribution in individual organs and to compare to the in vivo quantification. During the scan, it is paramount that individual organs are placed far enough to resolve the individual MPI signals generated and ensure the signals do not overlap or bleed into one another, therefore impacting the generated ROI. As a result, the spleen and the liver were scanned separately to the remainder of the organs. When looking at the smaller organs with less iron accumulation, the difference between methods was apparent where method 1 generates a smaller ROI and overall measures less signal than method 3 (Fig. 5a). For method 1, scaling factors of k = 3 and 5 were used to create the ROI, whereas with k = 10 the borders of the threshold were too minor to detect any signal. The scaling factor of k = 3 captured more signal than k = 5, but fails to generate ROI for all organs, as clearly depicted in the lung and heart sections (Fig. 5a and b). With less iron in a given organ, the signal generated is very close to the systems SD, generating a smaller ROI and less estimated iron. This difference was less apparent in the liver, spleen and kidney (at 24 hours) where the signal is much greater than background (Fig. 5b). The signals in these three organs also showed an expected increase in the amount of iron from 6 to 24 hours, validating the in vivo findings. The amount of iron quantified in the liver is 91% and 85% to the quantified iron amount in vivo for k = 3 and k = 5 respectively. For the ex vivo data, method 1 and 2 varied slightly in the quantified iron amount due to the difference in the ROI size, although less variation than the in vivo findings. With method 3, the amount of iron quantified in the liver correlated to 70% that of the in vivo data and the sum of iron in all the organs correlated to nearly 80% of the total retrieved signal from the animal (Fig. 5c). The signal from the heart and lung were quite low and close to background resulting in less detection using method 1 than method 2, although potentially detecting more noise.
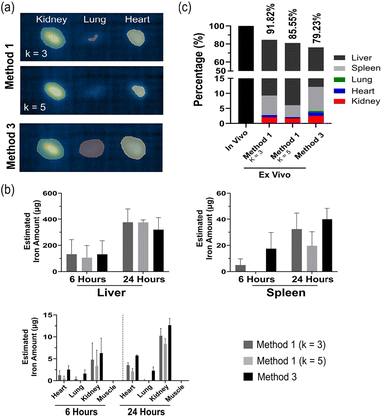 |
| Fig. 5 (a) Representative MPI images and generated ROI using method 1 with k = 3 or 5 and method 2. (b) Quantified iron amount in the liver, spleen, heart, lung, kidney, and muscle at 6 and 24 hours post IV using methods 1 and 3. Data presented as mean ± SD (n = 4). (c) Sum of iron in individual organs for each method as percentage of total iron in vivo at 24 hours. | |
User variability
The data was analysed by three different users individually to evaluate the user variability across the three methods in vivo and ex vivo at the 6 hour timepoint (Fig. 6). Three key measures were calculated for reproducibility; the average difference in quantification (μg); the standard error of measurement (SEM); and the coefficient of variance (CoV)% (Table 2).
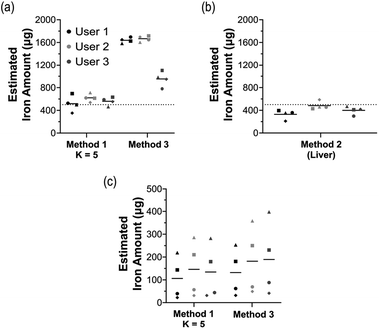 |
| Fig. 6 Variability of methods between 3 independent users. Individual values and mean value presented. Calculated for (a) method 1 (k = 5), method 3 in vivo (b) method 2 in vivo in the liver region and (c) method 1 (k = 5), method 3 ex vivo in the liver. | |
Table 2 User variability across ROI selection methods for in vivo and ex vivo iron quantification. Reported average difference in mean (μg), SEM and CoV (%)
ROI method |
In vivo
|
Ex vivo (liver) |
1 (K = 5) |
2 (Liver) |
3 |
1 (K = 5) |
3 |
Avg. diff. mean (μg) |
68.62 |
102.46 |
475.73 |
29.62 |
38.34 |
SEM |
51.82 |
76.96 |
404.25 |
16.76 |
25.48 |
CoV (%) |
9.16 |
19.10 |
28.48 |
13.02 |
15.21 |
Method 1 (k = 5) demonstrated much lower variation than method 3 and was the most accurate in its quantification of the total iron in vivo. The borders of the ROI in method 3 are highly influenced by the user, introducing the greater variation. Method 2, although similarly a freehand method, had a lower variation than method 3 likely due to the ROI being drawn on only true MPI signals rather than the entire scan. For ex vivo findings, the variation across the methods is quite similar. However, method 3 did have slightly higher variation, again due to the freehand nature of the method.
Limitations
A limitation of this study is the length of time each method takes to perform, especially with larger numbered data sets, as well as the inter-user variability with methods 2 and 3. Method 1 does minimize this issue by defining limits for the threshold segment value. As mentioned, defining ROI specifically around different organs can be challenging however with additional validation of iron uptake through post-mortem tissue analysis using techniques such as inductively coupled plasma optical emission spectroscopy (ICP-OES), mass spectrometry (ICP-MS) and transmission electron microscopy (TEM) the correct borders can be determined, although possibly technically challenging to distinguish endo- from exogenous iron.20
The automation of analysis through the generation of algorithms and machine learning is clearly a powerful tool that should be utilized across all imaging applications. K-means++ for accurate ROI delineation and segmentation, first introduced in 2021 by Hayat and Sun, enables automated and rapid throughput for MPI quantification.21,22 The algorithm automates the creation of segment ROI from the image and quantifies the iron using a standard curve model, as described in this study. The algorithm is useful in its automation of an analysis method similar to method 1 described in this study. However, the study was only applied on in vivo MPI scans with signals generated from a singular region and is limited by the tracer's resolution between different islands. Further, the use of an algorithm may restrict the spread of a universal MPI analysis method to those with computational backgrounds.
Conclusions
Ultimately, we present a very long circulating SPION, outcompeting many of the current reported MPI tracers. Given its elongated circulation, the mPEG NP allows for distinct observation of tracer in the heart, carotid artery and liver. We believe that method 1 using k = 5 is the most optimal for signal quantification of tracers in circulation, providing a consistent and accurate estimate of the total iron administered. Throughout our study we found the importance of measuring the SD on each scan as the bleed and noise introduced from the tracer can greatly influence this value. Measuring the SD from a blank scan with no tracer does not accurately represent the noise found when a tracer is in circulation. A combination of method 1 and 2 can be used to quantify the amount of iron in multiple regions, giving the user freedom to select different organs or areas in vivo and monitor the tracer's behavior in that region over time. Method 3 captures excess noise and error, resulting in gross overestimations in addition to being highly influenced by the users. This showcases the inability to simply free-hand draw an ROI over the entire FOV to quantify total iron in vivo. Similarly, individual ROI around organs cannot be directly drawn on the MPI scan in vivo. Method 1 should first be used to define the true MPI signal followed by the individual ROIs over organs, as we demonstrate in method 2. There was some variation between users in method 2 given the borders of the liver cannot be precisely determined, further reiterating the challenge of directly quantifying the accumulation of iron within organs in vivo. We suggest that method 2 be used to monitor the relative change in tracer accumulation within a region, such as the liver area, rather than distinct uptake. Consistency can also be enhanced by applying equally sized ROI over various time points; ensuring stable voxels doesn't influence the signal detected in specific organs.
For ex vivo MPI scans method 3 created larger ROIs than method 1, therefore quantifying more iron. However overall, the variation between users using method 1 and 3 for liver quantification ex vivo was relatively low and showed consistent quantification. This consistency is most likely due to the smaller size of the ROI and the ease in defining the borders since there is less bleed.
As the current class of SPION continue to improve their resolution and tissue specific binding, method 1 will also be capable of quantifying iron uptake in the different organs that create distinct segments rather than a single one. Our study demonstrates that threshold-based ROI segmentation set by SD is ideal for the analysis of 3D MPI scans and of long circulating SPION. This method most accurately quantifies the amount of iron, is the least user dependent, and is consistent across different timepoints. We encourage MPI users to clearly define their chosen analysis method and adopt a standardized method. This will significantly strengthen MPI research, allowing for greater reproducibility and accurate tracer comparison.
Author contributions
JT performed the experiments and analyzed the data. JT, RB and KA designed experiments, interpreted results, and wrote the manuscript. KA supervised the study.
Conflicts of interest
There are no conflicts to declare.
Acknowledgements
The authors acknowledge the scientific and technical assistance of the National Imaging Facility (NIF), a National Collaborative Research Infrastructure Strategy (NCRIS) capability at Monash Biomedical Imaging (MBI), a Technology Research Platform at Monash University. We also thank Imagion Biosystems for providing the mPEG NP, TEM of the material and its characterization by DLS. The authors acknowledge the contributions of Ms. Siqi Li on the user variability analysis. JT acknowledges the support received through the Australian Government Research Training Program.
References
- S. J. Park, S. R. Han, Y. H. Kang, E. J. Lee, E. G. Kim, H. Hong, J. C. Jeong, M. S. Lee, S. H. Lee and D. Y. Song, Int. J. Nanomed., 2022, 17, 3711–3722 CrossRef PubMed.
- A. Remmo, N. Lowa, O. Kosch, D. Eberbeck, A. Ludwig, L. Kampen, C. Gruttner and F. Wiekhorst, Cells, 2022, 11 Search PubMed.
- A. Rivera-Rodriguez, L. B. Hoang-Minh, A. Chiu-Lam, N. Sarna, L. Marrero-Morales, D. A. Mitchell and C. M. Rinaldi-Ramos, Nanotheranostics, 2021, 5, 431–444 CrossRef PubMed.
- E. Y. Yu, M. Bishop, B. Zheng, R. M. Ferguson, A. P. Khandhar, S. J. Kemp, K. M. Krishnan, P. W. Goodwill and S. M. Conolly, Nano Lett., 2017, 17, 1648–1654 CrossRef CAS PubMed.
- P. Keselman, E. Y. Yu, X. Y. Zhou, P. W. Goodwill, P. Chandrasekharan, R. M. Ferguson, A. P. Khandhar, S. J. Kemp, K. M. Krishnan, B. Zheng and S. M. Conolly, Phys. Med. Biol., 2017, 62, 3440–3453 CrossRef CAS PubMed.
- A. P. Khandhar, P. Keselman, S. J. Kemp, R. M. Ferguson, P. W. Goodwill, S. M. Conolly and K. M. Krishnan, Nanoscale, 2017, 9, 1299–1306 RSC.
- R. Orendorff, A. J. Peck, B. Zheng, S. N. Shirazi, R. Matthew Ferguson, A. P. Khandhar, S. J. Kemp, P. Goodwill, K. M. Krishnan, G. A. Brooks, D. Kaufer and S. Conolly, Phys. Med. Biol., 2017, 62, 3501–3509 CrossRef CAS PubMed.
- B. Zheng, T. Vazin, P. W. Goodwill, A. Conway, A. Verma, E. U. Saritas, D. Schaffer and S. M. Conolly, Sci. Rep., 2015, 5, 14055 CrossRef PubMed.
- H. Arami, E. Teeman, A. Troksa, H. Bradshaw, K. Saatchi, A. Tomitaka, S. S. Gambhir, U. O. Hafeli, D. Liggitt and K. M. Krishnan, Nanoscale, 2017, 9, 18723–18730 RSC.
- Y. Du, X. Liu, Q. Liang, X. J. Liang and J. Tian, Nano Lett., 2019, 19, 3618–3626 CrossRef CAS PubMed.
- O. C. Sehl, B. Tiret, M. A. Berih, A. V. Makela, P. W. Goodwill and P. J. Foster, Int. J. Magn. Part. Imaging, 2022, 8, 1 Search PubMed.
- F. Liu, H. Wu, B. Peng, S. Zhang, J. Ma, G. Deng, P. Zou, J. Liu, A. T. Chen, D. Li, S. Bellone, A. D. Santin, J. Moliterno and J. Zhou, Nano Lett., 2021, 21, 8111–8118 CrossRef CAS PubMed.
- F. Vurro, M. Gerosa, A. Busato, M. Muccilli, E. Milan, J. Gaudet, P. Goodwill, J. Mansfield, E. Forlin, A. Negri, F. Gherlinzoni, G. Morana, M. Gottardi, P. Matteazzi, M. Wintermark, A. Speghini and P. Marzola, Cancers, 2022, 14 Search PubMed.
- A. E. Burgess, J. Opt. Soc. Am. A, 1999, 16, 633–646 CrossRef CAS PubMed.
- D. Pouliquen, J. J. Le Jeune, R. Perdrisot, A. Ermias and P. Jallet, Magn. Reson. Imaging, 1991, 9, 275–283 CrossRef CAS PubMed.
- R. Weissleder, D. D. Stark, B. L. Engelstad, B. R. Bacon, C. C. Compton, D. L. White, P. Jacobs and J. Lewis, AJR, Am. J. Roentgenol., 1989, 152, 167–173 CrossRef CAS PubMed.
- K. M. Parkins, K. P. Melo, Y. Chen, J. A. Ronald and P. J. Foster, Nanoscale, 2021, 13, 6016–6023 RSC.
- S. Hu, E. A. Hoffman and J. M. Reinhardt, IEEE Trans. Med. Imaging, 2001, 20, 490–498 CrossRef CAS PubMed.
- R. M. Rangayyan, R. H. Vu and G. S. Boag, J. Digit. Imaging, 2008, 21(1), S134–147 CrossRef PubMed.
- A. Balfourier, E. Tsolaki, L. Heeb, F. H. L. Starsich, D. Klose, A. Boss, A. Gupta, A. Gogos and I. K. Herrmann, Small Methods, 2023, 7, e2201061 CrossRef PubMed.
- H. Hayat, A. Sun, H. Hayat, S. Liu, N. Talebloo, C. Pinger, J. O. Bishop, M. Gudi, B. F. Dwan, X. Ma, Y. Zhao, A. Moore and P. Wang, Mol. Imaging Biol., 2021, 23, 18–29 CrossRef PubMed.
- A. Sun, H. Hayat, S. Liu, E. Tull, J. O. Bishop, B. F. Dwan, M. Gudi, N. Talebloo, J. R. Dizon, W. Li, J. Gaudet, A. Alessio, A. Aguirre and P. Wang, Front. Cell Dev. Biol., 2021, 9, 704483 CrossRef PubMed.
|
This journal is © The Royal Society of Chemistry 2023 |
Click here to see how this site uses Cookies. View our privacy policy here.