DOI:
10.1039/D3NA00180F
(Review Article)
Nanoscale Adv., 2023,
5, 4354-4367
Machine learning assisted-nanomedicine using magnetic nanoparticles for central nervous system diseases
Received
21st March 2023
, Accepted 24th July 2023
First published on 28th July 2023
Abstract
Magnetic nanoparticles possess unique properties distinct from other types of nanoparticles developed for biomedical applications. Their unique magnetic properties and multifunctionalities are especially beneficial for central nervous system (CNS) disease therapy and diagnostics, as well as targeted and personalized applications using image-guided therapy and theranostics. This review discusses the recent development of magnetic nanoparticles for CNS applications, including Alzheimer's disease, Parkinson's disease, epilepsy, multiple sclerosis, and drug addiction. Machine learning (ML) methods are increasingly applied towards the processing, optimization and development of nanomaterials. By using data-driven approach, ML has the potential to bridge the gap between basic research and clinical research. We review ML approaches used within the various stages of nanomedicine development, from nanoparticle synthesis and characterization to performance prediction and disease diagnosis.
Introduction
The field of nanomedicine has grown rapidly over the past few decades. Various smart nanomaterials have been developed, and their small sizes (smaller than mammalian and bacterial cells and larger than biological macromolecules such as antibody/protein) and unique functionalities enabled and improved various therapeutic and diagnostic applications such as drug delivery and disease diagnostics. Among these nanomaterials, magnetic nanoparticles (MNPs) show unique functionalities based on their magnetic properties, including magnetic guidance, heat generation under an alternating magnetic field, and image contrasts in magnetic-based medical imaging systems (Fig. 1).1,2 Magnetic guidance significantly enhances nanocarriers' drug and gene delivery capacities by applying an external magnetic field gradient.3 The heat generation capacity allows thermal treatment of cancer, a less invasive treatment with potentially minimum side effects compared to conventional cancer treatments such as surgery and chemotherapy.4 The image contrasts generated by MNPs using magnetic resonance imaging (MRI) and Magnetic Particle Imaging (MPI) allow early disease diagnosis. MPI is a new imaging modality which directly detects MNPs based on their magnetic properties. The nonlinear magnetic curves of MNPs exhibit higher harmonics under an oscillating magnetic field, which are detected as signals.5
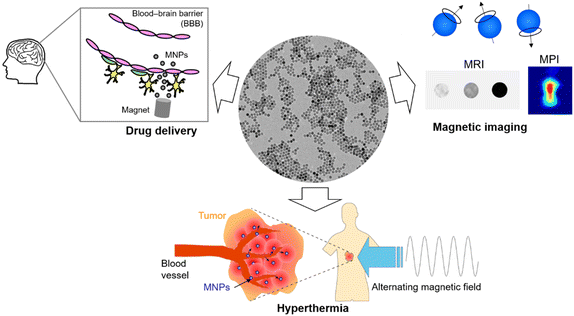 |
| Fig. 1 Biomedical applications of magnetic nanoparticles. | |
MNPs tailored to target specific disease sites or specific cells enable disease diagnostics and visualization of disease progression. MNPs are especially beneficial for the diagnosis and treatment of central nervous system (CNS) diseases, which affect the functions of the brain and spinal cord. CNS diseases, such as Alzheimer's disease, Parkinson's disease, epilepsy, multiple sclerosis, and CNS cancers (glioblastoma, meningioma, etc.), have caused a severe burden on medical systems and caregivers. The treatments for CNS diseases are challenging as only limited therapeutic agents reach the brain. The primary obstacle to delivering therapeutic agents to the brain is the blood–brain barrier (BBB), an interface between the circulating blood and the brain.6 The BBB, composed of tight junctions between endothelial cells, is a protective barrier restricting the influx of molecules from the bloodstream in to brain.7 The BBB limits the permeation of toxins as well as beneficial therapeutic agents, leading to poor delivery and limited therapeutic effects. MNPs possess promising functionality to enhance not only magnetic targeting but also transmigration across the BBB due to their magnetic guidance capacity.8,9 This unique function enables efficient brain-targeting for drug delivery, local thermal treatment, as well as diagnosis of CNS diseases using MRI and MPI.
Although MNPs have shown excellent potential for CNS-targeted applications, many obstacles exist in translating basic research to clinical settings. Machine learning (ML) is a powerful technique that learns from data sets and identifies correlations among data.10 This data-driven approach can be used to process various types of data and develop predictive models, which help identify critical information to bridge the gap between basic research and clinical research, including nanoparticle design, a more efficient synthesis process, enhanced BBB transmigration, and disease diagnosis based on image classification.
This review covers the synthesis, surface modification, and various biomedical applications of MNPs as well as ML approaches used at different stages of nanomedicine research. We will further discuss the recent work on MNPs developed for CNS diseases, including CNS/brain cancers, Alzheimer's disease, Parkinson's disease, epilepsy, multiple sclerosis, and drug addiction.
Magnetic nanoparticles
Magnetic properties
Most MNPs developed for biomedical applications possess unique superparamagnetic properties, which differ from the magnetic properties of bulk materials. Bulk ferrite materials (including iron oxide) are ferrimagnetic, in which magnetic moments align in an antiparallel arrangement. The opposing moments in this antiparallel arrangement do not cancel out; thus, ferrimagnetic materials show a net magnetic moment. The magnetization of ferrimagnetic materials shows hysteresis behavior with non-zero coercivity and remanent magnetization (Fig. 2a). In contrast, ferrite nanomaterials with sizes below the critical size exhibit superparamagnetic property, in which the coercivity and remanent magnetization become zero at the temperature above the blocking temperature due to thermal fluctuation (Fig. 2b).11 Superparamagnetic nanoparticles are preferred for biomedical applications over ferrimagnetic particles due to the zero remanent magnetization achieving better colloidal stability.
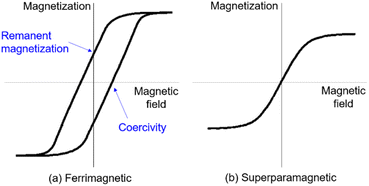 |
| Fig. 2 The hysteresis loops of (a) ferrimagnetic materials and (b) superparamagnetic materials. | |
Synthesis
Iron oxide NPs (IONPs) have been primarily used for biomedical applications among various MNPs with different compositions due to their large magnetization and biocompatibility.12 IONPs can be synthesized by various methods including coprecipitation, thermal decomposition, microemulsion, and hydrothermal approaches. The most widely used synthesis method is coprecipitation which involves coprecipitation of Fe2+ and Fe3+ ions under basic conditions.13,14 The mixture of Fe2+ and Fe3+ solutions with a 1
:
2 molar ratio is coprecipitated by adding an alkaline solution at room or higher temperature. This reaction results in solution turning black. The morphology and magnetic properties of the resulting IONPs vary based on different parameters, including the types of salt employed, salt concentration, reaction temperature, and pH of solution during the reaction. The coprecipitation method is very simple and generates IONPs with a hydrophilic surface, beneficial for biomedical applications. The major drawback of this method is the broad polydispersity of synthesized NPs.
Thermal decomposition is another widely used method to synthesize IONPs. The decomposition of iron precursors, including iron oleate, iron pentacarbonyl, and iron acetylacetonate under high temperatures produces highly monodispersed IONPs.15 This method involves the decomposition of iron precursors in organic solvents, and the high-temperature reaction is carried out in the presence of surfactant in an inert atmosphere. The morphology of the NPs can be controlled by adjusting the precursor concentration, surfactant employed, reaction temperature, and time.16 The thermal decomposition method synthesizes IONPs with a hydrophobic surface, which is not dispersible in water or buffer. Therefore, phase transfer or surface coating is required for use in biological studies.
To avoid the phase transfer step, another synthesis method called hydrothermal synthesis was developed to synthesize size-controlled IONPs with a hydrophilic surface. Hydrothermal synthesis crystalizes iron oxide from Fe ions in an aqueous solution under a high vapor pressure condition in addition to high temperature. The NPs sizes are controlled by altering the precursor concentration and the composition of the reaction solvent.17
Microemulsion-mediated methods have also been used to synthesize various NPs with precise shape and size control.18 Microemulsion, composed of water, oil, and surfactant, contains nanodroplets dispersed in another solvent. Water-in-oil (W/O) microemulsion retains water nanodroplets dispersed in the oil phase, and the water droplets are used as a nano-sized reactor for nanoparticle synthesis. Since the reaction occurs within the nanodroplets, the morphology of NPs can be controlled based on the size and shape of the nanodroplets. Their size and shape are controlled by varying parameters such as the type of surfactant and water-to-surfactant molar ratio. Size and shape-controlled IONPs are synthesized using the microemulsion method in conjunction with other synthesis methods, including coprecipitation.19
Surface coating and functionalization
MNPs intrinsically aggregate due to their magnetic property and sizes in the nano-range. Colloidal stability is critical for maintaining their hydrodynamic sizes, surface-to-volume ratios, and the desired magnetic properties for various biomedical applications. The surface coating of MNPs helps improve colloidal stability, and further functionalizing them with biological molecules for disease and cell-specific targeting. The materials widely used for surface coating include citric acid, silane agents, surfactants, and polymers. Citric acid or sodium citrate has been used as a capping agent during MNP synthesis as well as post-synthesis. Citrate coating significantly improves colloidal stability and affects the hydrodynamic sizes of MNPs by introducing negative charges on the surface, which induces repulsive force between each particle.20 Silane coupling agents are frequently used to coat inorganic NPs and introduce organic compounds to the NP's surface. An amine silane, (3-aminopropyl)triethoxylsilane (APTES)-coated MNPs form Fe–O–Si bond on the NPs surface, and the terminal amine groups are used for further functionalization with biological molecules.21,22 Various polymers are used to improve the colloidal stability of MNPs by adding a physical layer on the surface of the nanoparticles. Polyethylene glycol (PEG) is one of the most commonly used polymers for the surface coating of NPs. PEG protects NPs by providing steric hindrance, which prevents the adsorption of serum proteins and aggregation between nanoparticles in biological media.23 These features make PEG-coated NPs stealthy to the immune system and give extended blood circulation time.24,25 Moreover, PEG can be tailored with a variety of terminal functional groups based on the applications, including carboxylic, amine, and biotin, which can be used for surface binding as well as surface functionalization of NPs. Cationic polymers are often used for the surface coating of MNPs for gene delivery applications.26 The most popular choice is polyethylenimine (PEI), a cationic polymer used as a transfection agent. The positive charge of PEI-coated NPs attracts negatively charged DNA to the NP surface through electrostatic interaction and promotes binding to cells that contain an anionic phospholipid membrane.27 Moreover, MNPs with hydrophobic surfaces require ligand exchange or surface coating to introduce water dispersibility. Ligand exchange removes organic surfactants by replacing the surfactants with another ligand that exhibits higher adsorption affinity to the NPs.28 Small molecules including citric acid, APTES, and dopamine have been used for the ligand exchange of hydrophobic MNPs.29 Contrary to ligand exchange, amphiphilic polymers which contain both hydrophobic and hydrophilic components are used to cap hydrophobic MNPs via hydrophobic interactions. The hydrophobic component of amphiphilic polymers including poly(maleic anhydride-alt-1-octadecene) and Pluronics binds to the MNPs surface and exposes hydrophilic components outside.30 Mitochondria-targeted IONPs, namely Mito-Magneto were made by coating IONPs with triphenylphosphonium cation moieties through ligand exchange from oleic acid-capped IONPs.31,32
Surface functionalization of MNPs with biological molecules is an important step for applications that require disease, cell, or biomarker-specific binding. Various biological molecules have been functionalized, including antibodies, proteins, and other ligands that target specific disease biomarkers. These biological molecules are bound on MNPs by using the surface coating materials as an intermediate layer. The functional end groups of surface coating materials and biological molecules are permanently bound via chemical couplings.33,34 The biological molecules functionalized on MNPs for brain applications include transferrin, lactoferrin, and cell-penetrating peptides (CPPs), which are known to promote transcytosis across the BBB. Transferrin receptors are overexpressed on brain capillary endothelial cells forming the BBB; therefore, transferrin and lactoferrin bind to the receptor and help NPs cross the BBB.35 CPPs are a set of peptides that facilitate cellular internalization by utilizing their ability to cross cell membranes.36 Among various CPPs, transactivator of transcription (TAT)-conjugated NPs have shown transcytosis capabilities for brain targeting.37 MNPs can also be integrated with virus capsids to form a traceable vehicle for brain targeting.38
Hybrid magnetic nanomaterials and vortex structures
Smart magnetic NPs with additional functions such as plasmonic property, electric property, biodegradability, and swelling property have been developed by combining magnetic NPs with organic or other inorganic materials. Magnetoliposomes combining MNPs and liposomes (Fig. 3a,39) are another type of drug delivery carriers. Liposomes are lipid bilayer vesicles that can hold hydrophilic drugs in their inner aqueous phase and hydrophobic drugs in the lipid bilayer and release these drugs in a sustained manner.40 The addition of magnetic NPs to these drug carriers provides multifunctionality including enhanced targeting capacity using magnetic guidance,41 controlled drug release using magnetic hyperthermia or magnetic stimulation,42 and imaging contrasts in MRI.43 Rao et al. synthesized magnetoliposomes encapsulating neuromodulators for the manipulation of targeted neural populations.44 The neuromodulator release controlled remotely by an external magnetic field achieved increased social preference in mice. Magnetic nanomicelles encapsulating MNPs within micelles have been reported for various biomedical applications. Vitol et al. synthesized temperature-responsive magnetic nanomicelles containing platinum complex for cancer treatment. The combination of magnetic targeting and controlled pro-drug release into a cancer cell via hyperthermia enabled high-precision delivery to a single cell.45 Magnetic nanogels (Fig. 3b,46) are organic/inorganic hybrid materials with drug delivery capacities. Nanogels, nano-sized hydrogels composed of crosslinked polymer networks, are emerging nanomaterials with biocompatibility, good drug-loading capacity, and shrinking/swelling properties.47,48 They are composed of various polymers, and their physicochemical properties can be fine-tuned by varying the compositions of polymers and crosslinkers. Nanogels prepared from biodegradable polymers and temperature/pH-responsive polymers are used for sustained drug release and controlled drug release, respectively.49 Similar to magnetoliposomes, MNPs significantly enhance the drug delivery capabilities of nanogels by adding magnetic guidance capacity, controlled drug release upon magnetic stimulation, and imaging capacity.50 MNPs were also encapsulated successfully in the core of polymer-lipid hybrid nanocarriers for in vivo theranostics applications (Fig. 3c).51
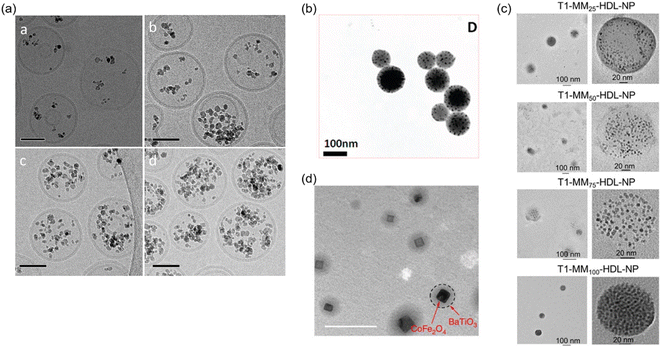 |
| Fig. 3 (a) Cryo-transmission electron microscope (TEM) images of magnetoliposomes with different amounts of MNPs (scale bars, 50 nm). Reproduced with permission from ref. 39. Copyright (2012) John Wiley & Sons, Ltd. (b) A TEM image of magnetic nanogels. Reproduced with permission from ref. 46. Copyright (2010) American Chemical Society. (c) TEM characterization of mitochondria-targeted HDL mimicking-NPs with various amounts (25, 50, 75, and 100%) of Mito-Magneto feeds inside the hydrophobic core of T-HDL-NPs. Reproduced with permission from ref. 51. Copyright (2020) American Chemical Society. (d) A TEM image of magnetoelectric nanoparticles (Scale bar, 100 nm). Reproduced with permission from ref. 57. Copyright (2013) Macmillan Publishers Limited. | |
Core–shell NPs containing MNPs and additional inorganic materials are promising for image-guided therapy and theranostics. In addition to magnetic properties, core–shell NPs possess multifunctionalities combining other properties such as plasmonic and electric properties. Plasmonic nanomaterials provide image contrasts in multiple imaging modalities including X-ray CT52 and photoacoustic imaging53 as well as light-activated thermal therapy.54 The integration of magnetic and plasmonic features in a single structure gives great opportunities for various image-guided therapies. The most common structure for this type of NP is a magnetic core and gold shell. The gold shell provides biocompatibility, stability, and adjustable plasmonic properties based on the shell morphology.
Magnetoelectric NPs (MENPs)55 are another type of core–shell NPs which not only integrate magnetic and electric properties in a single structure but also show a magnetoelectric effect. The magnetoelectric effect is a unique property coupling magnetic and electric properties. The electric polarization of magnetoelectric NPs can be controlled by an external magnetic field. Our group has applied this unique property for controlled drug delivery applications. Drugs were released from MENPs composed of CoFe2O4 core-BaTiO3 shell (Fig. 3d) by manipulating the electrostatic interaction between the nanoparticles and drugs bound on nanoparticle surface.56–58
In addition, magnetic vortex structures have been developed for various biomedical applications including cancer treatment and neuromodulation. Some micro- and nanoscale magnetic discs and dots have shown vortex configuration, which is a closed circular magnetization.59 The magneto-mechanical force induced by the magnetic vortex structures under an alternating magnetic field achieved the destruction of brain cancer cells.60 Gregurec et al. utilized magnetic vortex nanodiscs for neuromodulation. The mechanosensory neurons decorated with the nanodiscs were stimulated by applying an alternating magnetic field and the activation of ion channels were observed.61
Machine learning-assisted synthesis and characterization
Nanoparticle synthesis and characterization are tedious processes that involves various parameters for optimization. ML-assisted approaches help speed up these processes and improve their performances in the targeted applications. ML is a subset of artificial intelligence that learns and identifies patterns from data and develop models to solve problems, including prediction and classification. ML algorithms are generally classified into supervised and unsupervised learning. In supervised learning, ML models are trained using input datasets labeled for output, and the trained models are used to predict the outputs of the new unlabeled data. On the contrary, unsupervised learning uses unlabeled datasets to identify hidden patterns.62 Most commonly used ML algorithms include regression, k-nearest neighbor (KNN), decision tree, random forest, and support vector machine (SVM) that are classified as supervised learning algorithms, as well as artificial neural networks (ANN), which mimic neural networks in the human brain. In contrast to ANN, neural networks with deeply nested architecture are classified as deep learning (DL). More specific examples will be discussed in detail below and schematic illustrations of ML and DL algorithms were show in Fig. 4.
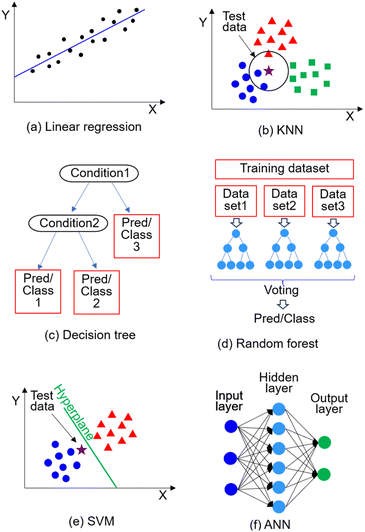 |
| Fig. 4 Schematic illustration of (a) linear regression, (b) KNN, (c) decision tree, (d) random forest, (e) SVM, and (f) ANN. | |
Linear regression is one of ML's most basic supervised learning algorithms, which is used to predict continuous values by fitting a linear correlation between input data and output. Linear regression models are applied to make predictions in various fields, such as the price in real estate and weather forecasts, as well as a mortality prediction of patients associated with the disease symptoms.63
KNN is a simple algorithm that is mainly used for classification. The data is classified based on the neighboring training dataset by calculating the distance between the test data and the neighboring training dataset, identifying K nearest neighbors (dataset), and identifying the class of the K nearest neighbors. KNN performs all calculations during the testing phase; therefore, the computational cost is high when the dataset is large.64
The decision tree is an algorithm used for regression and classification by continuously splitting data based on certain conditions and forming a tree-like structure. The internal node, branch, and leaf node of the tree structure represent the conditions tested, the outcome of the test such as true/false, and the final prediction or decision, respectively.
Random forest is a supervised learning algorithm that combines multiple decision trees in parallel by using a randomly selected subset of data for each decision tree and aggregating their decisions. The subset of data is selected for each tree by a random sampling technique called bootstrap, which allows multiple samplings from the same dataset. The aggregation process (bagging or bootstrap aggregating) in random forest overcomes the over-fitting problem that decision tree models have by reducing the variance.65
SVM is another supervised learning algorithm that identifies the boundary between the data points from different classes called a hyperplane and routinely used in genomics. The data points closest to the hyperplane and the distance between those points from each class are called support vectors and margin, respectively. SVM identifies the hyperplane that maximizes the margin. To solve non-linear problems, the kernel method that adds additional dimensions are used to transform the data into a linear problem.66
ANN mimics neural networks in the human brain by simulating interconnected artificial neurons (nodes) which transmit signals between nodes. ANN consists of multiple layers, including an input layer where the input data is received, an output layer where the result is given, and hidden layers between input and output layers where the data is processed. Within the hidden layer, weights and bias are used to amplify/diminish the signals. The neural network with many hidden layers that construct a deeply nested structure is called a deep neural network.67
Various models have been developed to predict nanoparticle properties related to biomedical applications. The hydrodynamic sizes (colloidal sizes) and surface properties of NPs significantly affect the biodistribution and circulation time. Shalaby et al. developed an ANN model to predict the hydrodynamic sizes of polymeric nanoparticles and drug encapsulation efficiency by using the molecular weight of the polymer, the drug-to-polymer ratio, and the number of polymer blocks.68 Drug encapsulation/loading was also predicted by utilizing other algorithms including Gaussian Processes (a type of supervised machine learning method).69 AI-assisted image processing approaches have been applied to analyze the size, shape, and agglomeration of MNPs. Since the magnetic properties of MNPs largely depend on the particle size and shape, the unsupervised ML method was used to analyze the distributions of NP size and shape. The classification was performed on transmission electron microscopy (TEM) images containing large numbers of NPs with different shapes and image magnifications using the hierarchical clustering method.70 The agglomeration state of MNPs was also analyzed using an ML-based image segmentation tool.71 Agglomerate analysis of MNPs was successfully performed on NP suspension in cell culture media.
Some ML and DL models have been developed to predict the magnetic properties of magnetic materials. The saturation magnetization, coercivity, and magnetostriction of magnetic nanocrystalline alloys were predicted by various models trained using algorithms such as SVMs, KNNs, and random forests. Among other models, the random forest model achieved better prediction of the magnetic properties based on the compositions.72 The magnetic properties of MNPs, including saturation magnetization and magnetic anisotropy, were also predicted using a neural network and random forest.73
Biomedical applications
Magnetic nanoparticles-based drug delivery system
The magnetic force exerted on MNPs under external field gradients allow targeted therapy and drug delivery to the body tissues, which are difficult to reach with traditional nanocarriers, including the brain.74,75 This magnetic guidance feature significantly reduces side effects and allows efficient targeted therapy with enhanced therapeutic efficacy. Our group demonstrated that MNPs-based nanomaterials including magneto-electric nanoparticles (MENPs) and magnetic core–gold shell nanoparticles enhance BBB transmigration in the presence of a permanent magnet.9,57 MENPs were delivered to the brain of a baboon using the static magnetic field of the MRI without adverse effects.76 In another study, our group showed that MNPs can be incorporated in micro/nanogels for targeted therapy to the brain.77 These magnetic nanogels find various applications for neurological disorders and biomedical applications.78,79
The magneto-thermal effect of MNPs is used to achieve controlled drug release.80 Thermal energy cleaves non-covalent bonds as well as thermally-sensitive covalent bonds at a temperature above a certain range.81 This cleavage results in the release of drugs bound on the MNPs surface upon stimulation with an alternating magnetic field. The magneto-thermal effect can also be combined with temperature-sensitive liposomes, gels and polymers, including poly(N-isopropylacrylamide) and Pluronics. These temperature-sensitive materials change their physical properties when the temperature is elevated.82 By incorporating MNPs into temperature-sensitive nanocarriers, the nanocarriers achieve controlled release capacity of encapsulated drugs upon heat generation of MNPs triggered by an external magnetic field.80 These magnetic nanogels find various applications for various neurological disorders and biomedical applications.78,79
Hyperthermia
MNPs generate heat by hysteresis loss and relaxation losses, which are further classified into Brownian relaxation and Néel relaxation.83 Hysteresis loss is based on the hysteresis between external magnetic fields and the magnetization of MNPs induced by the external fields. The magnetization lags compared to the external fields, and this energy is dissipated in the form of heat. When the particle sizes decrease, MNPs exhibit superparamagnetic properties with closed hysteresis. MNPs in this size range generate heat primarily by relaxation losses as there's no contribution from hysteresis loss. Relaxation losses are associated with the relaxation of magnetic moments and the delay in this relaxation. Brownian relaxation and Néel relaxation involve the relaxation of magnetic moments derived from the motion of entire particles and the rotation of magnetic moments, respectively.83
Imaging
MRI contrast is derived from the relaxation of hydrogen protons in body tissues. MRI contrast agents enhance contrasts by shortening the longitudinal (T1) or transverse (T2) relaxation time of hydrogen protons nearby.84 Although MNPs are primarily used as T2 contrast agents which provide negative contrasts, recent reports demonstrated the potential of ultra-small superparamagnetic nanoparticles as T1 contrast agents which provide positive contrasts.85 Although commercially available contrast agents based on iron oxide NPs are no longer available in the US, ultra-small iron oxide NPs with a T1-contrast have been developed as an alternative to the Gd complex.86 MPI signal is generated from time-varying magnetization responses of MNPs under external magnetic fields.87 Contrary to MRI, MPI only images MNPs without background signals from tissues.88 This feature makes MPI suitable for molecular imaging. Various monodispersed MNPs with different size variations have been synthesized to improve the MPI signals.89,90 MRI and MPI combined with MNPs-based contrast agents/imaging probes have shown promising results for early disease diagnostics due to enhanced image contrasts. Functionalized MNPs have been developed for the early detection of various medical conditions, including different types of cancers91 and CNS diseases.92
Magnetic nanoparticles for brain diseases
MNPs have been explored significantly for therapeutic and diagnostic applications. Especially they have shown potential for brain diseases theranostics. In the present section, we will highlight the role of MNPs in various brain diseases. It will give an overview of the recent progress made in the few major brain diseases.
Brain tumor
A brain tumor can generally be defined as an abnormal growth of cells in the brain or skull of humans. This can be benign or malignant. All brain tumors are a serious threat and can eventually damage and spread to other parts of the brain and body. Various modalities have continuously been discovered for improved treatment and diagnosis. In this regard, MNPs have been extensively explored for drug delivery to the brain and treatment of brain tumors.93,94 Magnetic field-guided therapy has been successfully utilized to deliver drugs within the brain tumor by externally-controlled magnetic systems.95 However, MNPs have continuously been challenged for their toxicity and retention within the tumor site.
Glioblastoma multiforme (GBM) is a malignant type of CNS tumor.96 It is one of the most common brain tumors and has a moderate survival rate.97 The treatment is accompanied by huge challenges such as (a) acquiring resistance to radiation therapies and chemotherapies;98 (b) recurrence of the tumor at the nearby sites of the tumor; (c) surgical procedures involved with adjuvant chemotherapy;99 (d) neurologic discrepancies and systemic toxicities with these therapies;100 (e) hindrance to cross BBB by various drugs.101 These challenges associated with the treatment of GBM tumors require novel approaches for a greater impact on patient survival and quality of life. Interestingly, a study by Lee et al.102 used MNPs with free thiol end groups to enhance the accumulation of these particles in the brain. This study suggests that MNPs coated with bi-functional poly(ethylene glycol) form a large cluster near the brain tumor where an external magnetic field is applied, allowing an enhanced accumulation of MNPs in the rat brain tumor model. This surface modification enhanced the delivery of doxorubicin, suggesting a potential therapeutic application of such NPs. One of the very interesting therapies using MNPs known as ferroptosis therapy (FT) was reported by Chen et al.103 In this therapy, they hypothesized that the FT could be improved by utilizing the chemistries of the Fenton reaction, which accelerates the reaction by producing an increased concentration of all the reactants (Fe2+, Fe3+, and H2O2) in cancer cells. Cisplatin (CDDP)-loaded Fe3O4/Gd2O3 hybrid nanoparticles conjugated with lactoferrin and arginylglycylaspartic acid (RGD) dimer were used for FT of orthotopic brain tumor. These particles demonstrated a transport across the BBB and showed internalization in cancer cells, accompanied by the generation of reactive oxygen species to induce cancer cell death. This study showed the capability of the NPs to inhibit tumor growth.103 Remarkably, the replacement of MNPs-based therapeutics for brain tumor treatment and diagnosis with classical chemotherapy and radiotherapy has been highlighted due to the improved drug delivery aspects achieved by magnetic hyperthermia using MNPs.104 Recent progress demonstrates magnetic hyperthermia in clinical trials for the tumors associated with the brain and prostate. MNPs are used as intratumoral hyperthermia agents under an alternating magnetic field within the safe range for the patients. Future research should be focused on the development of the MNPs with improved heating efficiency in vivo which prevents the loss of magnetic properties of the NPs under physiological conditions.105
Alzheimer's disease
Alzheimer's disease (AD) is a type of neurological disorder that affects memory, thoughts, and behavior. This disease grows slowly and reaches severe conditions that can interfere with the individual's daily activities. This disease is challenged by delayed diagnosis and long treatment regimens. The late diagnosis of AD leads to irreversible neuronal damage and malfunctioning. In this regard, MNPs have been explored for decades as contrast agents. A few studies demonstrate the use of MNPs based nanoconjugate bound to antiferritin antibody, which targets the high levels of ferritin protein present in the areas of high-accumulated amyloid plaques in the brain. These studies showed that the nanoconjugates recognize and bind to the ferritin protein in the subiculum area of AD transgenic mice.106 One of the major improvements for better AD diagnosis is the liquid biopsy of AD biomarkers, including tau proteins and amyloid-β peptides (Aβs). A recent study by Li et al.107 shows the utilization of antibiofouling polymer polyethylene glycol-block-allyl glycidyl ether (PEG-b-AGE) coated MNPs. These MNPs suppressed non-specific interactions of various serum proteins, which was the cause of the false-positive diagnosis. Moreover, the antibody-conjugated antibiofouling MNPs showed improved specificity and sensitivity (80–90%) compared to the antibody-conjugated micron beads (Fig. 5). These particles showed better detection of the Aβs and tau proteins from the human whole blood samples than antibody-conjugated Dynabeads (∼20%). Cerebral amyloid angiopathy (CAA) has gained a lot of attention due to the deposition of Aβ proteins at the walls of cerebral vasculature which leads to recurrent hemorrhagic strokes. A study reports the use of theranostic nanovehicles consisting of Magnevist® (MRI contrast agent) which was conjugated to the polymer chitosan and loaded with cyclophosphamide, functionalized with F(ab′)2 fragment of anti-amyloid antibody IgG4. These theranostic nanovehicles were capable of targeting cerebrovascular amyloid and treating cerebrovascular inflammation and can also be used as MRI contrast agents.108 Overall, MNPs have emerged as potential candidates for AD diagnostic and treatments.109–111
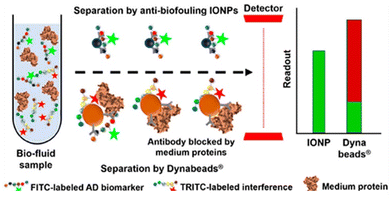 |
| Fig. 5 Antibiofouling magnetic nanoparticles for liquid biopsy of Alzheimer's disease. Reprinted with permission from ref. 107. Copyright (2019) American Chemical Society. | |
Parkinson's disease
Parkinson's disease (PD) is a brain disorder that leads to disorientation in walking and talking, disbalance, stiffness, and loss of coordination. It is the second most common neurodegenerative disorder.112 PD patients face challenges due to a lack of effective drugs and diagnostic methods. Few drugs are known for their treatment, such as Levodopa. Although Levodopa has shown better treatment outcomes than other conventional drugs, it has severe limitations, such as lower transport across the BBB and poor bioavailability. Nanomaterials have shown great potential to transport anti-PD drugs across the BBB.113 Moreover, there is much need for effective diagnostic tools for early diagnosis. In many studies, MNPs have been used as an efficient diagnostic tool for detecting and separating important biomarkers associated with the pathogenesis of PD. A recent study showed that monoclonal antibodies with MNPs detect plasma or serum α-synuclein levels via immunomagnetic reduction.114 Gene therapy is also one of the modalities utilized to effectively target and alter the expression of α-synuclein, a protein associated with PD pathology. Niu et al. synthesized N-isopropylacrylamide derivative-immobilized MNPs carrying short hairpin RNA (shRNA) plasmid, which interferes with α-synuclein.115 An effective repair was observed by using the multifunctional MNPs carrying shRNA in a PD model. These research studies reveal the important role of MNPs which can be utilized for effective repair in a PD model and for determining the mechanisms and signal transduction pathways associated with PD. Another study by Niu et al.115 utilized multifunctional superparamagnetic NPs carrying the shRNA α-synuclein (α-syn) which is the major component of the Lewy bodies. These Lewy bodies are considered the main pathological characteristics of PD. This study utilizes the functionalized superparamagnetic NPs for effective repair in a Parkinson's model (Fig. 6). MNPs have also been utilized to immobilize various inhibitors such as Monoamine oxidase. These studies demonstrate the efficient screening of inhibitors from complex mixtures, which can identify new anti-PD compounds from medicinal plants.116
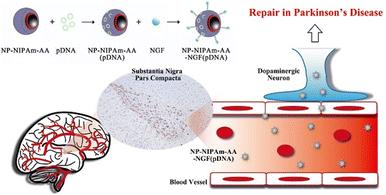 |
| Fig. 6 Multifunctional magnetic nanoparticles loaded with alpha-synuclein RNAiPlasmid utilized in a Parkinson's disease model. Reproduced reprinted under the creative commons attribution 4.0 International from ref. 115. | |
Seizure
Epilepsy is considered a major neurological disorder characterized by abnormal brain activity that causes seizures and leads to loss of awareness. Although antiepileptic drugs have been used to treat epilepsy in most cases, they are ineffective in controlling seizure attack in some patient populations. Thus, there is a need to develop better therapeutic options for epilepsy. MNPs have found great interest in developing improved nanocarriers for antiepileptic drugs and natural herbal compounds. Quercetin is a well-known natural compound recognized for its antioxidant properties and anticonvulsant characteristics. However, this compound has faced some criticism in studies. At low doses 10 mg kg−1, it prolonged the onset of seizures, while at high dosages, 40 mg kg−1 was not able to prevent pentylenetetrazole (PTZ) induced seizure.117 Moreover, other studies showed that quercetin possesses a weak and short-term anti-convulsant potential.118 The fabrication of the quercetin-conjugated Fe3O4–β-cyclodextrin (βCD) nanoparticles showed better performance, such as reduced seizure behavior, and astrocyte activation in a PTZ-induced kindling model as compared to the free quercetin.119 Recent research shows the use of MNPs for accurate brain mapping. This technique is based on creating superparamagnetic aggregates in the epileptic foci due to the increased electrical and magnetic activities. This study presents the potential of aggregates for enhanced tissue contrasts in MRI for an improved resection of epileptic foci (Fig. 7).120 Future studies should be implemented for novel nanotheranostics strategies where MNPs can be utilized wisely as an improved and efficient primary care tool for epilepsy.121
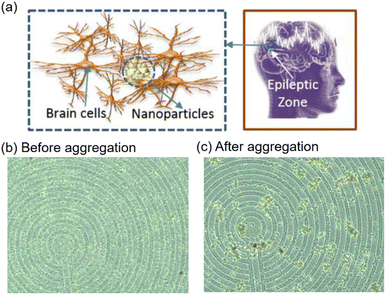 |
| Fig. 7 (a) Schematic illustration of MNPs aggregation in an epileptic zone. (b) MNPs above the micro coil before applying an electromagnetic field. (c) Aggregation of MNPs after applying an electromagnetic field. Reproduced reprinted under the creative commons attribution 4.0 International from ref. 120. | |
Multiple sclerosis
Multiple sclerosis (MS) is a common demyelinating disease observed in humans, where the myelin sheath that protects neurons is damaged and significantly affects nerve cells of the brain and spinal cord.122 MNPs have been employed primarily to monitor the disease in MS, as well as to treat it in conjunction with imaging. One method employed to treat MS is to implant oligodendrocytes which produce myelin. In one study, Chari et al. have shown MNPs-mediated transfection (magnetofection) for gene transfer of reporter and therapeutic genes in oligodendrocyte precursor cells. They used static and oscillating magnetic fields in tissue models of rat brain slices, demonstrating the potential of magnetofection.123 In another study with a similar idea, monocytes labeled with ultrasmall MNPs were tested in rat MS models for MRI using the magnetoelectroporation technique.124 In addition to myelin loss, MS is often associated with increased inflammation causing a significant disturbance in BBB. MNPs have been employed in humans, to image neuroinflammation using MRI. Studies found that superparamagnetic nanoparticles were sensitive in identifying neuroinflammation compared to gadolinium-based contrast agents.125 Ultrasmall MNPs enhancement in MRI was associated with a higher loss of brain tissue structure.126 Trafficking of cells, especially lymphocytes and monocytes that transmigrate BBB, in MS patients was explored initially using blood samples isolated from MS patients. Cells were isolated and treated with Ferridex, then imaged using MRI to show the feasibility of this technology in vivo.127 Experimental autoimmune encephalomyelitis (EAE) based animal models were employed to mimic many aspects of human MS. MNPs were used to detect migration of encephalitogenic T cells into the CNS to provide information on these cells' role in the development of lesions.128–136 In one study, MNPs were administered intravenously and examined via MRI followed by ex vivo examination of the brain using sonography. Both results showed that MNPs can be employed for detection of CNS lesions by specific macrophage imaging.128 Frank et al. used polylysine tagged MNPs to track the migration of encephalitogenic T cells using in vivo and ex vivo MR studies.129 Breckwoldt et al. used the FDA-approved MNPs, ferumoxytol in mice model of EAE to show that noninvasive MR imaging can be used to track immune response as biomarkers to monitor inflammatory conditions.135 PLGA-PEG-coated MNPs loaded into T-lymphocytes showed T-cells entry into the brain.136
Addiction
Addiction to abuse drugs has been a significant problem facing many countries in the world.137–142 Opioid and abuse drug-related deaths have seen a significant increase in the last decade. Abuse drugs such as nicotine, cocaine, methamphetamine, morphine, heroin, and opioids exert various immunomodulatory effects in the body, but many of them cause serious effects on the brain by inducing apoptosis, inflammation, or oxidative stress in brain cells including neurons, microglia, and astrocytes. Further, if the patient has a brain-related disease, these effects of drug abuse exacerbate. Most of the research literature covering MNPs and addiction has been conducted in the context of diseases such as HIV143–145 and Alzheimer's disease.146,147 Delivering neuroprotectants that counter the effects of abused drugs is often a challenge due to the poor ability of these drugs to cross the BBB. Nair and coworkers have published multiple articles related to MNPs in the context of drug abuse materials.148–150 In one article, they coated 60 nm diameter MNPs with brain-derived neurotropic factor (BDNF), a neuron-resuscitating agent, and demonstrated such nanocarriers can be directed to cross BBB and deliver the neuroprotective agent BDNF. They showed to reduce the morphine-induced apoptosis in PBMCs, and restore the spine density in SKNMC cells thus reducing the neurotoxicity through their in vitro studies.148 In another study, the same authors examined the effects of morphine, in the context of HIV-1 using 25–40 nm-sized MNPs coated with a potent morphine antagonist CTOP, a BBB impermeable peptide (D-Pen-Cys-Tyr-DTrp-Orn-Thr-Pen-Thr-NH2). The CTOP-MNPs showed protection of SKNMC neuronal dendrites and spine morphology under morphine exposure either in the presence or absence of HIV infection.150 In another example, a cocaine antagonist, BD1063 using MENPs loaded liposomes were tested in cell models.151 One of the main advantages of using MNPs is their imaging capacities and image-guided drug delivery. Jacobs et al. used pharmacologic MRI (phMRI) techniques to show a decrease in cerebral blood volume in the C57BL/6 mouse brain after using cocaine, a psychotropic substance using three different MNP-based contrast agents.152,153 Liu and coworkers have delivered 18–30 nm-sized MNPs conjugated single-stranded oligodeoxynucleotides (sODN) via intracerebroventricular (ICV), intraperitoneal (i.p.), and ophthalmic (OTRD) delivery routes to C57BL/6 mice, and imaged with MRI to understand the gene action in neural cell migration, drug addiction, and neurological disorders.154 Moreover, carboxyl-coated magnetic beads of micrometer size were employed for sensing-related applications using colorimetric methods to detect methamphetamine and cocaine by using illicit drug-binding DNA aptamers and DNA–DNA hybridization techniques155 to potentially employ them for detection of these drugs in the waste water.
ML-driven biomedical applications of magnetic nanoparticles
ML-driven drug delivery system
The delivery efficiencies of nanocarriers depend on various parameters of the NPs, including particle sizes, surface charges, hydrophilicity and hydrophobicity, and intrinsic properties of NPs. Lin et al. used ML and DL models to predict the delivery efficiencies of NPs to tumors in tumor-bearing mice.156 Different models were trained using classic models, ensemble models, SVMs, and neural networks on data sets, including physicochemical properties of NPs as well as targeting strategies, cancer types, and tumor models. Among other models, the DL model was shown to provide better prediction, with the nanoparticles' zeta potential and core materials being significant parameters. Moreover, ML and DL techniques have been used to predict BBB permeability of small molecules. Due to the ability of the BBB to limit the permeation of toxins, it also limits the delivery of therapeutic agents to the brain. The prediction of BBB permeability helps identify molecules capable of crossing the BBB and design the nanocarriers for brain delivery of molecules, which cannot cross the BBB. Various prediction models for BBB permeability have been tested, including SVM,157 Recurrent Neural Network (RNN),158 and a hybrid model combining SVM and graph convolutional network.159 These models were primarily trained based on molecular descriptors, which are mathematical representations of the physical and chemical features of the tested molecules.
ML-driven hyperthermia
Various MNPs with different compositions (e.g., Fe3O4, NiFe2O4, CoFe2O4) have been studied as heat generators for hyperthermia.12,160 Since the heat generation of MNPs largely depends on their magnetic properties, various parameters such as composition, size, and morphology of MNPs need to be optimized to achieve sufficient heating to treat cancer cells. Coïsson et al. used ML to predict the heat generation of MNPs with different magnetic properties under varying conditions, such as the intensity and frequency of external magnetic fields.73 The ML models were trained using a neural network and random forest on data sets including nanoparticle diameters, external magnetic field, saturation magnetization, and magnetic anisotropy as input data and coercive field, magnetic remanence, and hysteresis loop area as output data. A more precise heat generation prediction was achieved using a random forest model. Moreover, MNPs functionalized with targeting ligands have shown excellent anti-cancer thermal effects in vitro and in vivo.161
ML-driven imaging
ML has been applied in the field of Radiology to improve medical image processing and analysis as well as diagnostic accuracy. In particular, convolutional neural networks (CNNs) and RNNs have been used for image segmentation and registration, which are the processes of delineating different anatomical structures and aligning multiple images, respectively.162 Moreover, ML-assisted image segmentation identified and quantified MNPs-labeled islet organoids differentiated from stem cells in the MPI images. This study used the K-means++ clustering algorithm to differentiate the labeled organoids from the background contrasts and provide 3D image segmentation in a diabetic mouse model.163 DL method was also used to quantify the concentration and distribution of MNPs based on MR images. The DL model achieved more accurate quantification for a wide range of MNPs concentration compared to traditional methods.164 In addition to image segmentation and registration, ML and DL models have been applied for image classification, which helps detect and diagnose diseases based on medical images. Crimi et al. developed a two-tiered classification model for the analysis of multiple sclerosis (MS). The MS lesions were identified based on the MR images enhanced with an iron oxide-based contrast agent using the model.165 Moreover, various image classification models identified different CNS diseases, including brain tumors and Alzheimer's disease.166,167
Conclusion and future perspective
The present review summarizes various biomedical applications of MNPs, ML approaches used at different stages of nanomedicine research, and the noteworthy progress in MNP-based therapeutic and diagnostic applications for major CNS diseases. Various ML models developed to support nanoparticle characterization and predict properties related to biomedical applications were discussed. Moreover, the therapeutic and diagnostic tools based on MNPs utilized for early diagnosis of brain tumors, AD, Parkinson's disease, multiple sclerosis, and drug addiction were described. The utilization of MNPs as theranostic agents have been successfully highlighted for various brain diseases. Additionally, when MNPs are used as nanofillers in various nanocarriers such as liposomes, nanogels, dendrimers, polymeric nanoparticles etc. they enhance physicochemical properties and targeting ability by functioning as cross-linkers connecting polymeric backbones.168 Although the unique and improved functionalization of MNPs has come up with breakthrough research, MNP-based applications are still in their infancy state in some areas including seizure and drug addiction, and there are inconsistent reports on the MNPs' efficiency, especially in clinical studies.
ML has been utilized and proven to be an efficient approach for making predictions and classifications in various fields. In nanomedicine, there have been significant advancements in ML-assisted toxicity and cellular internalization prediction of various NPs.169–171 ML and DL-based image analysis effectively performed disease diagnosis on medical images. Internet-of-medical-things (IoMT) was suggested to be an effective strategy for disease management when combined with sensors.172 However, ML models have not been extensively developed to analyze MNP-based biomedical applications including the prediction of magnetic targeting efficacy, heat generation of MNPs, as well as MNPs' efficiencies towards CNS therapeutics and diagnostics. By incorporating ML, we will be able to predict optimal MNP characteristics including the composition, size, and surface coating for drug delivery systems, hyperthermia, MRI, and MPI. Moreover, ML will be able to assist with MNPs characterization by predicting/classifying the analytical properties possibly without traditional lab equipment. This combined area of MNPs and ML research shows a huge potential to promote further clinical translation. The challenges associated with the MNPs, such as toxicity, the generation of free radicals and increased oxidative stress, and interference with liver metabolism, can be addressed wisely by alteration of the surface and functionalization. This review covers the study highlighting the improved properties of MNPs for better treatment and diagnosis of many diseases of CNS. These studies give a broad overview that despite various challenges associated with MNPs for clinical translation to humans, the modified MNPs are a potential candidate.
Conflicts of interest
Authors declare no conflict of interest.
Acknowledgements
This work was supported by National Institute of Health (NIH) grants. M. N. thanks support from National Institutes of Health (NIH) grants DA052271, DA042706, DA040537, DA037838, DA034547, and Florida Department of Health grant 8AZ04. A. V. thanks the support from FIU Foundation, grant number AWD0011094.
Notes and references
- Q. A. Pankhurst, J. Connolly, S. K. Jones and J. Dobson, J. Phys. D: Appl. Phys., 2003, 36, 167–181 CrossRef.
- E. A. Rozhkova, Adv. Mater., 2011, 23, H136–H150 CrossRef CAS PubMed.
- V. V. Mody, A. Cox, S. Shah, A. Singh, W. Bevins and H. Parihar, Appl. Nanosci., 2014, 4, 385–392 CrossRef CAS.
- X. Liu, Y. Zhang, Y. Wang, W. Zhu, G. Li, X. Ma, Y. Zhang, S. Chen, S. Tiwari, K. Shi, S. Zhang, H. M. Fan, Y. X. Zhao and X.-J. Liang, Theranostics, 2020, 10, 3793–3815 CrossRef CAS PubMed.
- B. Gleich and J. Weizenecker, Nature, 2005, 435, 1214–1217 CrossRef CAS PubMed.
- P. Ballabh, A. Braun and M. Nedergaard, Neurobiol. Dis., 2004, 16, 1–13 CrossRef CAS PubMed.
- Q. He, J. Liu, J. Liang, X. Liu, W. Li, Z. Liu, Z. Ding and D. Tuo, Cells, 2018, 7, 24 CrossRef PubMed.
- A. Kaushik, R. D. Jayant, R. Nikkhah-Moshaie, V. Bhardwaj, U. Roy, Z. Huang, A. Ruiz, A. Yndart, V. Atluri, N. El-Hage, K. Khalili and M. Nair, Sci. Rep., 2016, 6, 25309 CrossRef CAS PubMed.
- A. Tomitaka, H. Arami, A. Raymond, A. Yndart, A. Kaushik, R. D. Jayant, Y. Takemura, Y. Cai, M. Toborek and M. Nair, Nanoscale, 2017, 9, 764–773 RSC.
- M. Alber, A. Buganza Tepole, W. R. Cannon, S. De, S. Dura-Bernal, K. Garikipati, G. Karniadakis, W. W. Lytton, P. Perdikaris, L. Petzold and E. Kuhl, NPJ Digit. Med., 2019, 2, 115 CrossRef PubMed.
- Q. Chen and Z. J. Zhang, Appl. Phys. Lett., 1998, 73, 3156–3158 CrossRef CAS.
- A. Tomitaka, M. Jeun, S. Bae and Y. Takemura, J. Magn., 2011, 16, 164–168 CrossRef.
- M. C. Mascolo, Y. Pei and T. A. Ring, Materials, 2013, 6, 5549–5567 CrossRef CAS PubMed.
- H.-C. Roth, S. P. Schwaminger, M. Schindler, F. E. Wagner and S. Berensmeier, J. Magn. Magn. Mater., 2015, 377, 81–89 CrossRef CAS.
- M. Unni, A. M. Uhl, S. Savliwala, B. H. Savitzky, R. Dhavalikar, N. Garraud, D. P. Arnold, L. F. Kourkoutis, J. S. Andrew and C. Rinaldi, ACS Nano, 2017, 11, 2284–2303 CrossRef CAS PubMed.
- R. Hufschmid, H. Arami, R. M. Ferguson, M. Gonzales, E. Teeman, L. N. Brush, D. Browning and K. M. Krishnan, Nanoscale, 2015, 7, 11142–11154 RSC.
- S. Ge, X. Shi, K. Sun, C. Li, J. R. Baker, M. M. Banaszak Holl and B. G. Orr, J. Phys. Chem. C, 2009, 113, 13593–13599 CrossRef CAS PubMed.
- G. Zhang, Y. Liao and I. Baker, Mater. Sci. Eng., C, 2010, 30, 92–97 CrossRef PubMed.
- S. Santra, R. Tapec, N. Theodoropoulou, J. Dobson, A. Hebard and W. Tan, Langmuir, 2001, 17, 2900–2906 CrossRef CAS.
- L. Li, K. Y. Mak, C. W. Leung, K. Y. Chan, W. K. Chan, W. Zhong and P. W. T. Pong, Microelectron. Eng., 2013, 110, 329–334 CrossRef CAS.
- Y. Liu, Y. Li, X.-M. Li and T. He, Langmuir, 2013, 29, 15275–15282 CrossRef CAS PubMed.
- B. K. Sodipo and A. A. Aziz, Ultrason. Sonochem., 2018, 40, 837–840 CrossRef CAS PubMed.
- C. Fang, N. Bhattarai, C. Sun and M. Zhang, Small, 2009, 5, 1637–1641 CrossRef CAS PubMed.
- L. Guerrini, R. A. Alvarez-Puebla and N. Pazos-Perez, Materials, 2018, 11 Search PubMed.
- J. S. Suk, Q. Xu, N. Kim, J. Hanes and L. M. Ensign, Adv. Drug Delivery Rev., 2016, 99, 28–51 CrossRef CAS PubMed.
- S. Majidi, F. Zeinali Sehrig, M. Samiei, M. Milani, E. Abbasi, K. Dadashzadeh and A. Akbarzadeh, Artif. Cells, Nanomed., Biotechnol., 2016, 44, 1186–1193 CAS.
- S. S. Rohiwal, N. Dvorakova, J. Klima, M. Vaskovicova, F. Senigl, M. Slouf, E. Pavlova, P. Stepanek, D. Babuka, H. Benes, Z. Ellederova and K. Stieger, Sci. Rep., 2020, 10, 4619 CrossRef CAS PubMed.
- X. Wang, X. Wang, X. Bai, L. Yan, T. Liu, M. Wang, Y. Song, G. Hu, Z. Gu, Q. Miao and C. Chen, Nano Lett., 2019, 19, 8–18 CrossRef CAS PubMed.
- K. Davis, B. Cole, M. Ghelardini, B. A. Powell and O. T. Mefford, Langmuir, 2016, 32, 13716–13727 CrossRef CAS.
- G. C. Lavorato, J. C. Azcárate, M. B. Rivas Aiello, J. M. Orozco Henao, P. Mendoza Zélis, M. Ceolin, E. Winkler, M. H. Fonticelli and C. Vericat, Appl. Surf. Sci., 2021, 570, 151171 CrossRef CAS.
- B. Banik, B. W. Askins and S. Dhar, Nanoscale, 2016, 8, 19581–19591 RSC.
- B. Banik and S. Dhar, Curr. Protoc. Cell Biol., 2018, 76, 25 Search PubMed.
- R. P. Friedrich, J. Zaloga, E. Schreiber, I. Y. Tóth, E. Tombácz, S. Lyer and C. Alexiou, Nanoscale Res. Lett., 2016, 11, 297 CrossRef PubMed.
- S. H. Liao, C. H. Liu, B. P. Bastakoti, N. Suzuki, Y. Chang, Y. Yamauchi, F. H. Lin and K. C. Wu, Int. J. Nanomed., 2015, 10, 3315–3327 CrossRef CAS PubMed.
- A. J. Clark and M. E. Davis, Proc. Natl. Acad. Sci., 2015, 112, 12486 CrossRef CAS PubMed.
- S. Stalmans, N. Bracke, E. Wynendaele and B. Gevaert, PLoS One, 2015, 10, e0139652 CrossRef PubMed.
- B. Dos Santos Rodrigues, S. Lakkadwala, T. Kanekiyo and J. Singh, Int. J. Nanomed., 2019, 14, 6497–6517 CrossRef PubMed.
- J. Yun, A. M. Sonabend, I. V. Ulasov, D.-H. Kim, E. A. Rozhkova, V. Novosad, S. Dashnaw, T. Brown, P. Canoll, J. N. Bruce and M. S. Lesniak, J. Clin. Neurosci., 2012, 19, 875–880 CrossRef CAS PubMed.
- B. Garnier, S. Tan, S. Miraux, E. Bled and A. R. Brisson, Contrast Media Mol. Imaging, 2012, 7, 231–239 CrossRef CAS PubMed.
- M.-K. Lee, Pharmaceutics, 2020, 12 Search PubMed.
- M. Babincová, V. Altanerová, M. Lampert, Č. Altaner, E. Machová, M. Š. rámka and P. Babinec, Z. Naturforsch., C, 2000, 55, 278–281 CrossRef PubMed.
- R. Spera, F. Apollonio, M. Liberti, A. Paffi, C. Merla, R. Pinto and S. Petralito, Colloids Surf., B, 2015, 131, 136–140 CrossRef CAS PubMed.
- S. J. Soenen, G. V. Velde, A. Ketkar-Atre, U. Himmelreich and M. De Cuyper, Wiley Interdiscip. Rev.: Nanomed. Nanobiotechnol., 2011, 3, 197–211 CAS.
- S. Rao, R. Chen, A. A. LaRocca, M. G. Christiansen, A. W. Senko, C. H. Shi, P.-H. Chiang, G. Varnavides, J. Xue, Y. Zhou, S. Park, R. Ding, J. Moon, G. Feng and P. Anikeeva, Nat. Nanotechnol., 2019, 14, 967–973 CrossRef CAS PubMed.
- E. A. Vitol, E. A. Rozhkova, V. Rose, B. D. Stripe, N. R. Young, E. E. W. Cohen, L. Leoni and V. Novosad, Adv. Mater. Interfaces, 2014, 1, 1400182 CrossRef.
- S. R. Deka, A. Quarta, R. Di Corato, A. Falqui, L. Manna, R. Cingolani and T. Pellegrino, Langmuir, 2010, 26, 10315–10324 CrossRef CAS PubMed.
- Y. Yin, B. Hu, X. Yuan, L. Cai, H. Gao and Q. Yang, Pharmaceutics, 2020, 12 Search PubMed.
- A. Vashist, A. Kaushik, A. Vashist, J. Bala, R. Nikkhah-Moshaie, V. Sagar and M. Nair, Drug discovery today, 2018, 23, 1436–1443 CrossRef CAS PubMed.
- S. Hajebi, N. Rabiee, M. Bagherzadeh, S. Ahmadi, M. Rabiee, H. Roghani-Mamaqani, M. Tahriri, L. Tayebi and M. R. Hamblin, Acta Biomater., 2019, 92, 1–18 CrossRef CAS PubMed.
- B. Sung, M.-H. Kim and L. Abelmann, Bioeng. Transl. Med., 2021, 6, e10190 CAS.
- B. Banik, B. Surnar, B. W. Askins, M. Banerjee and S. Dhar, ACS Appl. Mater. Interfaces, 2020, 12, 6852–6862 CrossRef CAS PubMed.
- Y. C. Dong, M. Hajfathalian, P. S. N. Maidment, J. C. Hsu, P. C. Naha, S. Si-Mohamed, M. Breuilly, J. Kim, P. Chhour, P. Douek, H. I. Litt and D. P. Cormode, Sci. Rep., 2019, 9, 14912 CrossRef PubMed.
- W. Li and X. Chen, Nanomedicine, 2015, 10, 299–320 CrossRef CAS PubMed.
- J. B. Vines, J.-H. Yoon, N.-E. Ryu, D.-J. Lim and H. Park, Front. Chem., 2019, 7, 167 CrossRef CAS PubMed.
- N. Kolishetti, A. Vashist, A. Y. Arias, V. Atluri, S. Dhar and M. Nair, Mol. Aspects Med., 2021, 101046, DOI:10.1016/j.mam.2021.101046.
- A. Kaushik, A. Yndart, V. Atluri, S. Tiwari, A. Tomitaka, P. Gupta, R. D. Jayant, D. Alvarez-Carbonell, K. Khalili and M. Nair, Sci. Rep., 2019, 9, 3928 CrossRef PubMed.
- M. Nair, R. Guduru, P. Liang, J. Hong, V. Sagar and S. Khizroev, Nat. Commun., 2013, 4, 1707 CrossRef PubMed.
- N. Kolishetti, A. Vashist, A. Y. Arias, V. Atluri, S. Dhar and M. Nair, Mol. Aspects Med., 2022, 83, 101046 CrossRef CAS PubMed.
- K. L. Metlov and K. Y. Guslienko, J. Magn. Magn. Mater., 2002, 242–245, 1015–1017 CrossRef CAS.
- D.-H. Kim, E. A. Rozhkova, I. V. Ulasov, S. D. Bader, T. Rajh, M. S. Lesniak and V. Novosad, Nat. Mater., 2010, 9, 165–171 CrossRef CAS PubMed.
- D. Gregurec, A. W. Senko, A. Chuvilin, P. D. Reddy, A. Sankararaman, D. Rosenfeld, P.-H. Chiang, F. Garcia, I. Tafel, G. Varnavides, E. Ciocan and P. Anikeeva, ACS Nano, 2020, 14, 8036–8045 CrossRef CAS PubMed.
- K. G. Liakos, P. Busato, D. Moshou, S. Pearson and D. Bochtis, Sensors, 2018, 18, 2674 CrossRef PubMed.
- Y. Gao, G.-Y. Cai, W. Fang, H.-Y. Li, S.-Y. Wang, L. Chen, Y. Yu, D. Liu, S. Xu, P.-F. Cui, S.-Q. Zeng, X.-X. Feng, R.-D. Yu, Y. Wang, Y. Yuan, X.-F. Jiao, J.-H. Chi, J.-H. Liu, R.-Y. Li, X. Zheng, C.-Y. Song, N. Jin, W.-J. Gong, X.-Y. Liu, L. Huang, X. Tian, L. Li, H. Xing, D. Ma, C.-R. Li, F. Ye and Q.-L. Gao, Nat. Commun., 2020, 11, 5033 CrossRef CAS PubMed.
-
K. Taunk, S. De, S. Verma and A. Swetapadma, 2019 International Conference on Intelligent Computing and Control Systems (ICCS), Madurai, India, 2019, pp. 1255–1260 Search PubMed.
- P. O. Gislason, J. A. Benediktsson and J. R. Sveinsson, Pattern Recognit. Lett., 2006, 27, 294–300 CrossRef.
- S. Huang, N. Cai, P. P. Pacheco, S. Narrandes, Y. Wang and W. Xu, Cancer Genomics, 2018, 15, 41 CAS.
- C. Janiesch, P. Zschech and K. Heinrich, Electron. Mark., 2021, 31, 685–695 CrossRef.
- K. S. Shalaby, M. E. Soliman, L. Casettari, G. Bonacucina, M. Cespi, G. F. Palmieri, O. A. Sammour and A. A. El Shamy, Int. J. Nanomed., 2014, 9, 4953–4964 CAS.
- R. M. Hathout and A. A. Metwally, Eur. J. Pharm. Biopharm., 2016, 108, 262–268 CrossRef CAS PubMed.
- H. Wen, J. M. Luna-Romera, J. C. Riquelme, C. Dwyer and S. L. Y. Chang, Nanomaterials, 2021, 11(10), 2706 CrossRef CAS PubMed.
- M. Ilett, J. Wills, P. Rees, S. Sharma, S. Micklethwaite, A. Brown, R. Brydson and N. Hondow, J. Microsc., 2020, 279, 177–184 CrossRef CAS PubMed.
- Y. Wang, Y. Tian, T. Kirk, O. Laris, J. H. Ross, R. D. Noebe, V. Keylin and R. Arróyave, Acta Mater., 2020, 194, 144–155 CrossRef CAS.
- M. Coïsson, G. Barrera, F. Celegato, P. Allia and P. Tiberto, APL Mater., 2022, 10, 081108 CrossRef.
- K. T. Al-Jamal, J. Bai, J. T.-W. Wang, A. Protti, P. Southern, L. Bogart, H. Heidari, X. Li, A. Cakebread, D. Asker, W. T. Al-Jamal, A. Shah, S. Bals, J. Sosabowski and Q. A. Pankhurst, Nano Lett., 2016, 16, 5652–5660 CrossRef CAS PubMed.
- S. D. Kong, J. Lee, S. Ramachandran, B. P. Eliceiri, V. I. Shubayev, R. Lal and S. Jin, J. Controlled Release, 2012, 164, 49–57 CrossRef CAS PubMed.
- A. Kaushik, J. Rodriguez, D. Rothen, V. Bhardwaj, R. D. Jayant, P. Pattany, B. Fuentes, H. Chand, N. Kolishetti, N. El-Hage, K. Khalili, N. S. Kenyon and M. Nair, ACS Appl. Bio Mater., 2019, 2, 4826–4836 CrossRef CAS PubMed.
-
A. Vashist, A. Kaushik and N. Madhavan, US Pat., 10344100, 2019 Search PubMed.
- A. Vashist, V. Atluri, A. Raymond, A. Kaushik, T. Parira, Z. Huang, A. Durygin, A. Tomitaka, R. Nikkhah-Moshaie and A. Vashist, Front. Bioeng. Biotechnol., 2020, 8, 315 CrossRef PubMed.
-
A. Vashist, A. Ghosal, E. Sharmin, A. Vashist, R. Dua, V. Bhardwaj, A. Tomatika, R. D. Jayant, S. Uthaman and M. Calderon, Nanogels for Biomedical Applications, Royal Society of Chemistry, 2017 Search PubMed.
- D. Mertz, O. Sandre and S. Bégin-Colin, Biochim. Biophys. Acta, 2017, 1861, 1617–1641 CrossRef CAS PubMed.
- J. F. Liu, B. Jang, D. Issadore and A. Tsourkas, Wiley Interdiscip. Rev.: Nanomed. Nanobiotechnol., 2019, 11, e1571 Search PubMed.
- Y. K. Kim, E.-J. Kim, J. H. Lim, H. K. Cho, W. J. Hong, H. H. Jeon and B. G. Chung, Nanoscale Res. Lett., 2019, 14, 77 CrossRef PubMed.
- A. E. Deatsch and B. A. Evans, J. Magn. Magn. Mater., 2014, 354, 163–172 CrossRef CAS.
- Y.-D. Xiao, R. Paudel, J. Liu, C. Ma, Z.-S. Zhang and S.-K. Zhou, Int. J. Mol. Med., 2016, 38, 1319–1326 CrossRef CAS PubMed.
- H. Wei, O. T. Bruns, M. G. Kaul, E. C. Hansen, M. Barch, A. Wi, G. Adam, H. Ittrich, A. Jasanoff, P. Nielsen and M. G. Bawendi, Proc. Natl. Acad. Sci., 2017, 114, 2325–2330 CrossRef CAS PubMed.
- Y. Bao, J. A. Sherwood and Z. Sun, J. Mater. Chem. C, 2018, 6, 1280–1290 RSC.
- L. M. Bauer, S. F. Situ, M. A. Griswold and A. C. S. Samia, J. Phys. Chem. Lett., 2015, 6, 2509–2517 CrossRef CAS PubMed.
- X. Y. Zhou, Z. W. Tay, P. Chandrasekharan, E. Y. Yu, D. W. Hensley, R. Orendorff, K. E. Jeffris, D. Mai, B. Zheng, P. W. Goodwill and S. M. Conolly, Curr. Opin. Chem. Biol., 2018, 45, 131–138 CrossRef CAS PubMed.
- A. Tomitaka, H. Arami, S. Gandhi and K. M. Krishnan, Nanoscale, 2015, 7(40), 16890–16898 RSC.
- R. M. Ferguson, K. R. Minard and K. M. Krishnan, J. Magn. Magn. Mater., 2009, 321, 1548–1551 CrossRef CAS PubMed.
- Z. Bakhtiary, A. A. Saei, M. J. Hajipour, M. Raoufi, O. Vermesh and M. Mahmoudi, Nanotechnology, Biology and Medicine, 2016, 12, 287–307 CrossRef CAS PubMed.
- S. Luo, C. Ma, M.-Q. Zhu, W.-N. Ju, Y. Yang and X. Wang, Front. Cell. Neurosci., 2020, 14 CrossRef CAS PubMed.
- M. Wankhede, A. Bouras, M. Kaluzova and C. G. Hadjipanayis, Expert Rev. Clin. Pharmacol., 2012, 5, 173–186 CrossRef CAS PubMed.
- L. L. Israel, A. Galstyan, E. Holler and J. Y. Ljubimova, J. Controlled Release, 2020, 320, 45–62 CrossRef CAS PubMed.
- H. Gandhi, A. K. Sharma, S. Mahant and D. N. Kapoor, Ther. Delivery, 2020, 11, 97–112 CrossRef CAS PubMed.
- F. Hanif, K. Muzaffar, K. Perveen, S. M. Malhi and S. U. Simjee, Asian Pac. J. Cancer Prev., 2017, 18, 3–9 Search PubMed.
- A. Jordan, R. Scholz, P. Wust, H. Fähling and R. Felix, J. Magn. Magn. Mater., 1999, 201, 413–419 CrossRef CAS.
- D. S. Backos, C. C. Franklin and P. Reigan, Biochem. Pharmacol., 2012, 83, 1005–1012 CrossRef CAS PubMed.
- R. Stupp, W. P. Mason, M. J. Van Den Bent, M. Weller, B. Fisher, M. J. Taphoorn, K. Belanger, A. A. Brandes, C. Marosi and U. Bogdahn, N. Engl. J. Med., 2005, 352, 987–996 CrossRef CAS PubMed.
- P. J. Loehrer Sr, Y. Feng, H. Cardenes, L. Wagner, J. M. Brell, D. Cella, P. Flynn, R. K. Ramanathan, C. H. Crane and S. R. Alberts, J. Clin. Oncol., 2011, 29, 4105 CrossRef PubMed.
- M. Tajes, E. Ramos-Fernández, X. Weng-Jiang, M. Bosch-Morato, B. Guivernau, A. Eraso-Pichot, B. Salvador, X. Fernandez-Busquets, J. Roquer and F. J. Munoz, Mol. Membr. Biol., 2014, 31, 152–167 CrossRef CAS PubMed.
- K. Lee, A. E. David, J. Zhang, M. C. Shin and V. C. Yang, J. Ind. Eng. Chem., 2017, 54, 389–397 CrossRef CAS.
- Z. Shen, T. Liu, Y. Li, J. Lau, Z. Yang, W. Fan, Z. Zhou, C. Shi, C. Ke, V. I. Bregadze, S. K. Mandal, Y. Liu, Z. Li, T. Xue, G. Zhu, J. Munasinghe, G. Niu, A. Wu and X. Chen, ACS Nano, 2018, 12, 11355–11365 CrossRef CAS PubMed.
-
C. Caizer and M. Rai, Magnetic Nanoparticles in Human Health and Medicine: Current Medical Applications and Alternative Therapy of Cancer, 2021, pp. 355–379 Search PubMed.
- H. Gavilán, S. K. Avugadda, T. Fernández-Cabada, N. Soni, M. Cassani, B. T. Mai, R. Chantrell and T. Pellegrino, Chem. Soc. Rev., 2021, 50, 11614–11667 RSC.
- T. Fernández, A. Martínez-Serrano, L. Cussó, M. Desco and M. Ramos-Gómez, ACS Chem. Neurosci., 2018, 9, 912–924 CrossRef PubMed.
- Y. Li, E. Lim, T. Fields, H. Wu, Y. Xu, Y. A. Wang and H. Mao, ACS Biomater. Sci. Eng., 2019, 5, 3595–3605 CrossRef CAS PubMed.
- E. K. Agyare, K. M. Jaruszewski, G. L. Curran, J. T. Rosenberg, S. C. Grant, V. J. Lowe, S. Ramakrishnan, A. K. Paravastu, J. F. Poduslo and K. K. Kandimalla, J. Controlled Release, 2014, 185, 121–129 CrossRef CAS PubMed.
- K. L. Viola, J. Sbarboro, R. Sureka, M. De, M. A. Bicca, J. Wang, S. Vasavada, S. Satpathy, S. Wu and H. Joshi, Nat. Nanotechnol., 2015, 10, 91–98 CrossRef CAS PubMed.
- H. Skaat, E. Corem-Slakmon, I. Grinberg, D. Last, D. Goez, Y. Mardor and S. Margel, Int. J. Nanomed., 2013, 8, 4063 Search PubMed.
- L. O. Sillerud, N. O. Solberg, R. Chamberlain, R. A. Orlando, J. E. Heidrich, D. C. Brown, C. I. Brady, T. A. Vander Jagt, M. Garwood and D. L. Vander Jagt, J. Alzheimer's Dis., 2013, 34, 349–365 CAS.
- M. Ozansoy and A. N. Başak, Mol. Neurobiol., 2013, 47, 460–465 CrossRef CAS PubMed.
- E. Ghazy, A. Rahdar, M. Barani and G. Z. Kyzas, J. Mol. Struct., 2021, 1231, 129698 CrossRef CAS.
- C.-W. Chang, S.-Y. Yang, C.-C. Yang, C.-W. Chang and Y.-R. Wu, Front. Neurol., 2020, 10, 1388 CrossRef PubMed.
- S. Niu, L.-k. Zhang, L. Zhang, S. Zhuang, X. Zhan, W.-y. Chen, S. Du, L. Yin, R. You, C.-H. Li and Y.-Q. Guan, Theranostics, 2017, 7, 344–356 CrossRef CAS PubMed.
- X. Jiang, Y. Yuan, L. Chen, Y. Liu, M. Xiao, Y. Hu, Z. Chun and X. Liao, Microchem. J., 2019, 146, 1181–1189 CrossRef CAS.
- F. Sefil, I. Kahraman, R. Dokuyucu, H. Gokce, A. Ozturk, O. Tutuk, M. Aydin, U. Ozkan and N. Pinar, Int. J. Clin. Exp. Med., 2014, 7, 2471 Search PubMed.
- D. Nieoczym, K. Socała, G. Raszewski and P. Wlaź, Prog. Neuro-Psychopharmacol. Biol. Psychiatry, 2014, 54, 50–58 CrossRef CAS PubMed.
- M. Hashemian, M. Ghasemi-Kasman, S. Ghasemi, A. Akbari, M. Moalem-Banhangi, L. Zare and S. R. Ahmadian, Int. J. Nanomed., 2019, 14, 6481–6495 CrossRef CAS PubMed.
- M. Z. Pedram, A. Shamloo, A. Alasty and E. Ghafar-Zadeh, Sensors, 2015, 15, 24409–24427 CrossRef CAS PubMed.
- P. Shende and R. Trivedi, Nano Sel., 2021, 2, 1277–1290 CrossRef CAS.
- H. N. Lemus, A. E. Warrington and M. Rodriguez, Neurol. Clin., 2018, 36, 1–11 CrossRef PubMed.
- S. I. Jenkins, M. R. Pickard, N. Granger and D. M. Chari, ACS Nano, 2011, 5, 6527–6538 CrossRef CAS PubMed.
- R. D. Engberink, S. M. van der Pol, P. Walczak, A. van der Toorn, M. A. Viergever, C. D. Dijkstra, J. W. Bulte, H. E. de Vries and E. L. Blezer, Mol. Imaging, 2010, 9, 268–277 CrossRef PubMed.
- A. Neuwelt, N. Sidhu, C. A. Hu, G. Mlady, S. C. Eberhardt and L. O. Sillerud, AJR, Am. J. Roentgenol., 2015, 204, W302–W313 CrossRef PubMed.
- A. Maarouf, J. C. Ferré, W. Zaaraoui, A. Le Troter, E. Bannier, I. Berry, M. Guye, L. Pierot, C. Barillot, J. Pelletier, A. Tourbah, G. Edan, B. Audoin and J. P. Ranjeva, Mult. Scler., 2016, 22, 1032–1039 CrossRef CAS PubMed.
- J. C. Sipe, M. Filippi, G. Martino, R. Furlan, M. A. Rocca, M. Rovaris, A. Bergami, J. Zyroff, G. Scotti and G. Comi, Magn. Reson. Imaging, 1999, 17, 1521–1523 CrossRef CAS PubMed.
- R. A. Linker, A. Kroner, T. Horn, R. Gold, M. Maurer and M. Bendszus, AJNR Am. J. Neuroradiol., 2006, 27, 1225–1229 CAS.
- S. A. Anderson, J. Shukaliak-Quandt, E. K. Jordan, A. S. Arbab, R. Martin, H. McFarland and J. A. Frank, Ann. Neurol., 2004, 55, 654–659 CrossRef PubMed.
- M. Rausch, P. Hiestand, D. Baumann, C. Cannet and M. Rudin, Magn. Reson. Med., 2003, 50, 309–314 CrossRef CAS PubMed.
- M. Rausch, P. Hiestand, C. A. Foster, D. R. Baumann, C. Cannet and M. Rudin, J. Magn. Reson. Imaging, 2004, 20, 16–24 CrossRef PubMed.
- Y. P. Hsiao, C. H. Huang, Y. C. Lin and T. R. Jan, Int. J. Nanomed., 2019, 14, 1229–1240 CrossRef CAS PubMed.
- J. M. Millward, J. Schnorr, M. Taupitz, S. Wagner, J. T. Wuerfel and C. Infante-Duarte, ASN Neuro, 2013, 5, e00110 CrossRef PubMed.
- M. Hunger, E. Budinger, K. Zhong and F. Angenstein, J. Magn. Reson. Imaging, 2014, 39, 1126–1135 CrossRef PubMed.
- K. Kirschbaum, J. K. Sonner, M. W. Zeller, K. Deumelandt, J. Bode, R. Sharma, T. Kruwel, M. Fischer, A. Hoffmann, M. Costa da Silva, M. U. Muckenthaler, W. Wick, B. Tews, J. W. Chen, S. Heiland, M. Bendszus, M. Platten and M. O. Breckwoldt, Proc. Natl. Acad. Sci. U. S. A., 2016, 113, 13227–13232 CrossRef CAS PubMed.
- M. M. D'Elios, A. Aldinucci, R. Amoriello, M. Benagiano, E. Bonechi, P. Maggi, A. Flori, C. Ravagli, D. Saer, L. Cappiello, L. Conti, B. Valtancoli, A. Bencini, L. Menichetti, G. Baldi and C. Ballerini, RSC Adv., 2018, 8, 904–913 RSC.
- J. Moreno-Rius, Neurosci. Biobehav. Rev., 2019, 107, 238–251 CrossRef CAS PubMed.
- L. Gravitz, Nature, 2019, 573, S20–S21 CrossRef CAS PubMed.
- N. P. Coussens, G. S. Sittampalam, S. G. Jonson, M. D. Hall, H. E. Gorby, A. P. Tamiz, O. B. McManus, C. C. Felder and K. Rasmussen, J. Pharmacol. Exp. Ther., 2019, 371, 396–408 CrossRef CAS PubMed.
- S. C. Wang, Y. C. Chen, C. H. Lee and C. M. Cheng, Int. J. Mol. Sci., 2019, 20(17), 4294 CrossRef CAS PubMed.
- C. J. Browne, A. Godino, M. Salery and E. J. Nestler, Biol. Psychiatry, 2020, 87, 22–33 CrossRef CAS PubMed.
- G. F. Koob, Biol. Psychiatry, 2020, 87, 44–53 CrossRef CAS PubMed.
- R. D. Bruce, Top Antivir. Med., 2018, 26, 89–92 Search PubMed.
- C. O. Cunningham, H. V. Kunins, R. J. Roose, R. T. Elam and N. L. Sohler, J. Gen. Intern. Med., 2007, 22, 1325–1329 CrossRef PubMed.
- T. F. Kresina, L. Eldred, R. D. Bruce and H. Francis, AIDS, 2005, 19(3), S221–S226 CrossRef CAS PubMed.
- J. Barg, M. Belcheva, J. Rowinski, A. Ho, W. J. Burke, H. D. Chung, C. A. Schmidt and C. J. Coscia, Brain Res., 1993, 632, 209–215 CrossRef CAS PubMed.
- M. Muhlbauer, J. C. Metcalf Jr, J. T. Robertson, G. Fridland and D. M. Desiderio, Biomed. Chromatogr., 1986, 1, 155–158 CrossRef CAS PubMed.
- S. Pilakka-Kanthikeel, V. S. Atluri, V. Sagar, S. K. Saxena and M. Nair, PLoS One, 2013, 8, e62241 CrossRef CAS PubMed.
- V. Sagar, V. S. Atluri, S. Pilakka-Kanthikeel and M. Nair, Mol. Brain, 2016, 9, 57 CrossRef PubMed.
- V. Sagar, S. Pilakka-Kanthikeel, V. S. Atluri, H. Ding, A. Y. Arias, R. D. Jayant, A. Kaushik and M. Nair, J. Biomed. Nanotechnol., 2015, 11, 1722–1733 CrossRef CAS PubMed.
-
M. Nair, S. Tiwari and A. Y. Arias, US Pat. 10213507, 2019.
- T. A. Perles-Barbacaru, D. Procissi, A. V. Demyanenko and R. E. Jacobs, NMR Biomed., 2012, 25, 498–505 CrossRef CAS PubMed.
- T. A. Perles-Barbacaru, D. Procissi, A. V. Demyanenko, F. S. Hall, G. R. Uhl and R. E. Jacobs, Neuroimage, 2011, 55, 622–628 CrossRef CAS PubMed.
- P. K. Liu and C. H. Liu, Methods Mol. Biol., 2011, 711, 363–377 CrossRef CAS PubMed.
- K. Mao, J. Ma, X. Q. Li and Z. G. Yang, Sci. Total Environ., 2019, 688, 771–779 CrossRef CAS PubMed.
- Z. Lin, W. C. Chou, Y. H. Cheng, C. He, N. A. Monteiro-Riviere and J. E. Riviere, Int. J. Nanomed., 2022, 17, 1365–1379 CrossRef PubMed.
- Y. Yuan, F. Zheng and C.-G. Zhan, AAPS J., 2018, 20, 54 CrossRef PubMed.
- S. Alsenan, I. Al-Turaiki and A. Hafez, Comput. Biol. Chem., 2020, 89, 107377 CrossRef CAS PubMed.
- T.-H. Yu, B.-H. Su, L. C. Battalora, S. Liu and Y. J. Tseng, Briefings Bioinf., 2022, 23, bbab377 CrossRef PubMed.
- M. Jeun, S. Bae, A. Tomitaka, Y. Takemura and K. H. Park, Appl. Phys. Lett., 2009, 95, 082501 CrossRef.
- A. S. Garanina, V. A. Naumenko, A. A. Nikitin, E. Myrovali, A. Y. Petukhova, S. V. Klimyuk, Y. A. Nalench, A. R. Ilyasov, S. S. Vodopyanov, A. S. Erofeev, P. V. Gorelkin, M. Angelakeris, A. G. Savchenko, U. Wiedwald, A. G. Majouga Dr and M. A. Abakumov, Nanomedicine, 2020, 25, 102171 CrossRef CAS PubMed.
- J.-G. Lee, S. Jun, Y.-W. Cho, H. Lee, G. B. Kim, J. B. Seo and N. Kim, Korean J. Radiol., 2017, 18, 570–584 CrossRef PubMed.
- A. Sun, H. Hayat, S. Liu, E. Tull, J. O. Bishop, B. F. Dwan, M. Gudi, N. Talebloo, J. R. Dizon, W. Li, J. Gaudet, A. Alessio, A. Aguirre and P. Wang, Front. Cell Dev. Biol., 2021, 9, 704483 CrossRef PubMed.
- G. d. Maggiora, C. Castillo-Passi, W. Qiu, S. Liu, C. Milovic, M. Sekino, C. Tejos, S. Uribe and P. Irarrazaval, IEEE Trans. Pattern Anal. Mach. Intell., 2022, 44, 143–153 Search PubMed.
- A. Crimi, O. Commowick, A. Maarouf, J.-C. Ferré, E. Bannier, A. Tourbah, I. Berry, J.-P. Ranjeva, G. Edan and C. Barillot, PLoS One, 2014, 9, e93024 CrossRef PubMed.
- K. Hassan Ali, J. Wu, M. Muhammad and M. Muhammad Umer, Math. Biosci. Eng., 2020, 17, 6203–6216 Search PubMed.
- Y. AbdulAzeem, W. M. Bahgat and M. Badawy, Neural. Comput. Appl., 2021, 33, 10415–10428 CrossRef.
- D. Podstawczyk, M. Nizioł, P. Szymczyk, P. Wiśniewski and A. Guiseppi-Elie, Addit. Manuf., 2020, 34, 101275 CAS.
- I. Furxhi, F. Murphy, M. Mullins and C. A. Poland, Toxicol. Lett., 2019, 312, 157–166 CrossRef CAS PubMed.
- Y. Huang, X. Li, J. Cao, X. Wei, Y. Li, Z. Wang, X. Cai, R. Li and J. Chen, Environ. Int., 2022, 164, 107258 CrossRef CAS PubMed.
- R. Ali, M. Balamurali and P. Varamini, Int. J. Mol. Sci., 2022, 23(24), 16070 CrossRef CAS PubMed.
- A. Kaushik, Expert Opin. Drug Delivery, 2021, 18, 531–534 CrossRef CAS PubMed.
|
This journal is © The Royal Society of Chemistry 2023 |
Click here to see how this site uses Cookies. View our privacy policy here.