DOI:
10.1039/D2NA00823H
(Review Article)
Nanoscale Adv., 2023,
5, 2375-2393
Recent advances in lab-on-a-chip systems for breast cancer metastasis research
Received
19th November 2022
, Accepted 26th March 2023
First published on 27th March 2023
Abstract
Breast cancer is the leading cause of cancer-related deaths in women. Multiple molecular subtypes, heterogeneity, and their ability to metastasize from the primary site to distant organs make breast cancer challenging to diagnose, treat, and obtain the desired therapeutic outcome. As the clinical importance of metastasis is dramatically increasing, there is a need to develop sustainable in vitro preclinical platforms to investigate complex cellular processes. Traditional in vitro and in vivo models cannot mimic the highly complex and multistep process of metastasis. Rapid progress in micro- and nanofabrication has contributed to soft lithography or three-dimensional printing-based lab-on-a-chip (LOC) systems. LOC platforms, which mimic in vivo conditions, offer a more profound understanding of cellular events and allow novel preclinical models for personalized treatments. Their low cost, scalability, and efficiency have resulted in on-demand design platforms for cell, tissue, and organ-on-a-chip platforms. Such models can overcome the limitations of two- and three-dimensional cell culture models and the ethical challenges involved in animal models. This review provides an overview of breast cancer subtypes, various steps and factors involved in metastases, existing preclinical models, and representative examples of LOC systems used to study and understand breast cancer metastasis and diagnosis and as a platform to evaluate advanced nanomedicine for breast cancer metastasis.
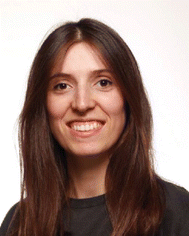 Burcu Firatligil-Yildirir | Burcu Firatligil-Yildirir is a postdoctoral researcher at the Faculty of Engineering and Natural Sciences, Tampere University, Finland. She received her Ph.D. in Molecular Biology and Genetics in 2021 from the Izmir Institute of Technology, Turkey, and M.Sc. in Biomedicine and Biotechnology from the University of Veterinary Medicine, Vienna, Austria. Her research interests include breast cancer metastasis, lab-on-a-chip platforms, and cell and tissue engineering. |
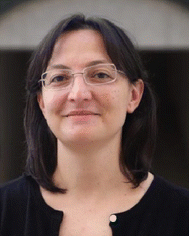 Özden Yalcin-Ozuysal | Ozden Yalcin-Ozuysal is an associate professor at the Molecular Biology and Genetics Department, Izmir Institute of Technology, Izmir, Turkey. She received her Ph.D. in 2009 from the Faculty of Biology and Medicine, University of Lausanne, Switzerland. She conducted her postdoctoral research at the École Polytechnique Fédérale de Lausanne. Her research expertise and interests include breast cancer metastasis, lab-on-a-chip technology, and molecular signaling. |
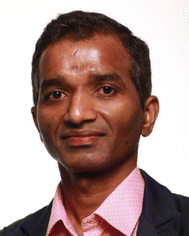 Nonappa | Nonappa is an Associate Professor at the Faculty of Engineering and Natural Sciences at Tampere University, Finland. He received his Ph.D. in 2008 from the Indian Institute of Science, Bangalore, India. He conducted his postdoctoral research at the University of Jyväskylä and Aalto University, Finland. His research interests include precision nanomaterials, colloids, hydrogels, in vitro breast cancer models, extracellular matrix design and cryogenic transmission electron microscopy. |
1. Introduction
Breast cancer is the most frequently diagnosed cancer type and is one of the leading causes of cancer-related deaths among women.1,2 According to the latest GLOBOCAN (Global Cancer Observatory) report, breast cancer is the most common cancer type in 159 out of 185 countries, with ∼12% contribution to the total cancer incidence in 2020.3 In most cases, primary breast cancer is curable in around 70–80% of patients if an early-stage diagnosis and treatments are performed. However, intertumoral (i.e., patient-to-patient) and intratumoral (i.e., within a patient's tumor) heterogeneity and multiple molecular subtypes significantly affect breast cancer's prognosis, treatment, and therapeutic outcome.4 Gene expression profile approaches have revealed several intrinsic molecular subtypes of breast cancer (Fig. 1).5 Each molecular subtype has different prognosis and metastatic properties (Fig. 1b). This has led to the development of different treatment strategies.6 The classification of breast cancer subtypes is related to hormone receptor (estrogen receptor (ER), progesterone receptor (PR), the cell proliferation marker (Ki67), and human epidermal growth factor receptor 2 (HER2)) expression patterns observed in breast cancer cells.7 The intrinsic molecular subtypes of breast cancer are categorized as (i) luminal A (ER+/PR+/HER2− and Ki67 low), (ii) luminal B (ER+/PR+/HER2−/+ and Ki67 high), (iii) HER2-enriched (ER−/PR−/HER2+) and (iv) triple negative/basal-like (ER−/PR−/HER2−) (Fig. 1).8 The bilayer structure of breast epithelium consists of luminal cells and an outer layer of basal cells surrounded by a basement membrane and stroma. In human breast cancers, although most cases are thought to be of luminal origin,9,10 there is still a debate on the origin as both luminal and basal progenitor cells can give rise to different tumor subtypes,11 which increases breast cancer heterogeneity.
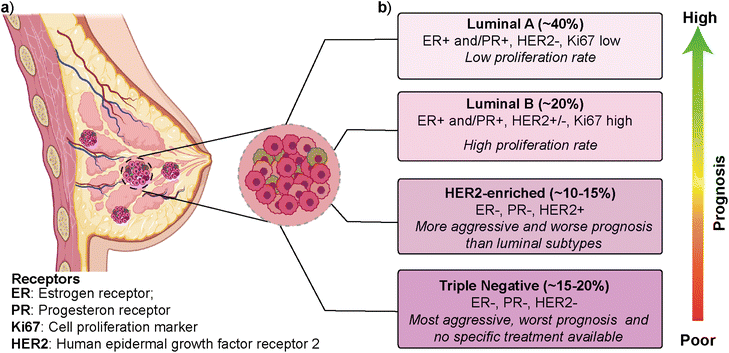 |
| Fig. 1 The mammary gland and molecular subtypes of breast cancer. (a) Schematic representation of the breast duct. (b) Molecular classification of breast cancer subtypes exhibiting their prevalence and associated hormone receptors. (a) was created using https://Biorender.com. | |
Luminal A type has a low-level cellular proliferation with the best prognosis by having low levels of the Ki67 marker. On the other hand, the luminal B type has a higher proliferation rate.12,13 The overexpression of the HER2 marker in the HER2-enriched subtype leads to a more aggressive phenotype and worse prognosis than the luminal subtype. The triple-negative breast cancer (TNBC), which is basal-like, is the most aggressive molecular subtype and is observed in 15–20% of all breast cancer cases.14,15 TNBC subtype generally shows a higher cellular proliferation rate with a higher risk of metastasis and recurrence.16,17 Luminal and HER2-enriched subtypes respond to hormone and HER2- targeted therapies, respectively. On the other hand, there is no specific treatment approach for TNBC that would worsen its prognosis.
2. Breast cancer metastasis
Cancer metastasis is a process in which cancer cells migrate from the primary tumor site to distant organs or secondary sites (Fig. 2).18 It is a highly complex and multistep process responsible for more than 90% of cancer-associated deaths.19 Despite substantial advancements in early detection of breast cancer, many patients have metastasis at the time of their first diagnosis.20 Around 50% of the patients first diagnosed with primary breast cancer eventually develop metastasis, leading to poor prognosis.20 In breast cancer, the most prevalent secondary sites are the lung, brain, bone, and liver (Fig. 2). Recent surveillance, epidemiology, and end results (SEER)-based studies revealed that 30–60% of breast cancer patients have metastases in the bone, 21–32% in the lungs, 15–32% in the liver, and 4–10% in the brain.21,22
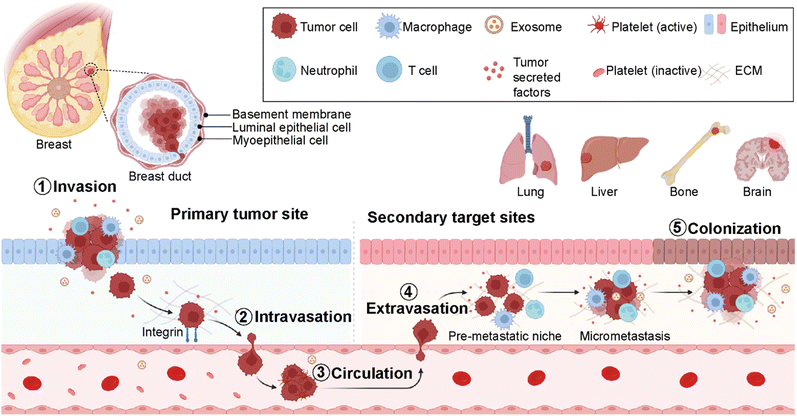 |
| Fig. 2 Breast cancer metastatic cascade. Schematic representation of key components involved in breast cancer metastasis from the primary tumor site to secondary target sites- lung, liver, bone, and brain. Figures were created using https://Biorender.com. | |
Importantly, the preferred metastatic sites are often associated with the specific molecular subtype of primary breast cancer.23,24 The luminal A and B breast cancer subtypes have the tendency to metastasize to the bone (58.5%). On the other hand, HER2-enriched breast cancers mainly give rise to liver metastases (31.7% for ER-/PR- and 25.7% for ER+/PR+ cases). Furthermore, HER2-enriched and TNBC subtypes have a higher propensity to metastasize to lung sites compared to HER2-negative breast cancers accounting for 21.2% and 32.1%, respectively. The propensity of different breast cancer subtypes to give rise to different target organ metastases is associated with the microenvironments at primary (breast tissue) and secondary (distant target organ) tumor sites.25,26
The cell–cell and cell–extracellular matrix (ECM) interactions between tumor cells and the microenvironment of distant organs initiate metastatic cascades. The development of metastasis is initiated by migration and local invasion of cancer cells into the surrounding stroma, followed by their intravasation into the vascular system (Fig. 2). Once in blood circulation, some cancer cells adhere to blood vessel walls and extravasate into new cellular surroundings in the target organ site. The cancer cells adapt to and ultimately succeed in the secondary site's foreign or distant environmental conditions to form metastatic colonization.27 Therefore, it is critical to understand the formation of microenvironments for primary and secondary sites and their molecular differences at every stage of breast cancer. This is crucial for the determination of an earlier diagnosis and the development of personalized medicine.28
Current methods such as the Boyden chamber and wound healing assay used to study cancer cell migration, cell invasion and cell–cell interactions are laborious and cost-intensive. Therefore, advanced in vitro platforms for understanding disease and providing diagnostic strategies become vital. Early diagnosis of disease at the molecular level using point-of-care platforms such as microfluidic technology is gaining pivotal preference.29 These emerging bio- and nanotechnological platforms offer more reliable and repeatable approaches with a high throughput screening.30 Point-of-care platforms such as lateral flow tests and chip-based assays have the potential to provide accurate diagnosis and disease monitoring.31 More importantly, the combination of biomarkers and microfluidic platforms allows rapid and early detection of molecular mechanisms behind different stages of breast cancer metastasis. Here, we review (i) the current knowledge of how specific components are vital for in vivo breast cancer metastasis, (ii) how these components and processes can be mimicked in advanced in vitro microfluidic technology, and (iii) how new technologies are used to study and detect different steps of breast cancer metastasis, diagnosis, drug discovery and nanomedicine. Specifically, we will emphasize various lab-on-a-chip (LOC)-based platforms.
2.1. Key players in the breast cancer metastasis cascade
The metastasis of breast cancer cells is closely associated with the microenvironment of the primary and secondary cancer sites. The target site (secondary site) microenvironment is a well-organized site for the colonization of tumor cells and their migration and spreading (Fig. 2). These tumor-induced microenvironments are known as pre-metastatic niches (PMNs).32 The composition and structure of PMNs are complex and different from those of primary tumor regions. Therefore, sufficient interactions between tumor cells and the microenvironment are critical for the survival of tumor cells. This environment consists of an extracellular matrix (ECM), host stromal cells including immune cells such as macrophages, neutrophils and T-cells, secreted factors (tumor-secreted factors, exosomes, etc.), and proteins such as growth factors, matrix metalloproteinases (MMPs), pro-inflammatory cytokines- S100A8 and S100A9, blood and lymph vessels that make the region inevitable for tumor initiation, progression, and metastasis (Fig. 2).33 Breast cancer metastasis is a non-random process related to the distribution of breast cancer cells to certain target organs. This phenomenon is known as metastatic organotropism or organ-specific metastasis. It is mediated by several factors, including tumor-intrinsic elements, the communication between tumor cells and the target-site microenvironment, and target organ-specific niches.34 Emerging studies reveal that primary tumor regions not only include cancer cells but also represent an altered surrounding environment. This altered stroma in the primary site called a tumor microenvironment (TME) is one of the key players in tumor initiation, development, and progression. The composition and organization of the extracellular matrix (ECM) and the cellular milieu are critical factors within the TME to foster tumor growth and spread to neighboring or distant sites.35 Therefore, it is decisive to investigate the roles of different cell populations, including immune cells, adipocytes, fibroblasts, and endothelial cells in the breast TME. These components mediate cancer cell dissemination either directly by secreting soluble and non-soluble mediators or indirectly by performing ECM deposition or remodeling.36,37
2.2. Cell-to-cell interactions
One of the main factors regulating the metastatic spread of breast cancer cells is the cooperation between cancer and stromal cells, where chemokine/chemokine receptor interactions play critical roles.38 Different chemokine receptor expression patterns found in breast cancer cells mediate various mechanisms during the metastasis process. For instance, the upregulation of chemokine receptor type 4 (CXCR4) and chemokine receptor type 7 (CCR7) in breast cancer cells is pointedly involved in pseudopodia formation.39 Pseudopodia are membrane protrusions promoting cancer cell dissemination.
This results in chemotactic and invasive responses of breast cancer cells within the body. In addition, the signaling of chemokine receptor type 2 (CXCR2) receptors is essential for the proliferation and colonization of breast cancer cells in the bone.40 The source of ligands for the chemokine receptors on breast cancer cells is mainly found within the pre-metastatic niches of their target tissues, which facilitates the movement of cancer cells to the secondary target tissues.38 Specifically, the interactions of stromal cell-derived factor 1 or C-X-C motif chemokine ligand 12 (CXCL12)/CXCR4 for breast cancer metastasis to the lung and C-X-C motif chemokine ligand 5 (CXCL5)/CXCR2 axis for breast cancer colonization in the bone site have been reported.38,40
2.3. The extracellular matrix
The extracellular matrix (ECM) is considered an essential part of both TMEs and PMNs. The ECM is mainly produced and organized by stromal cells residing within it. The ECM provides a dynamic environment that affects cellular responses, including proliferation, differentiation, and invasion. This dynamic environment is mainly created by biophysical and biochemical properties of the ECM, such as stiffness, topography, and solubility of the matrix and direct or indirect cues regulated by niche-promoting molecular components.41 Specifically, the niche-promoting protein components of the ECM have been defined as the matrisome, mainly composed of structural ECM proteins, secreted factors, and ECM regulators.42,43 The correlation between the increase in the expression of matrisomal components and increased mortality was reported in several studies.44 In addition, changes in ECM stiffness and structure have been associated with the alteration of cellular mechanotransduction, which is the conversion of mechanical stimuli into biochemical and cellular responses.41,45 These changes have been implied in tumor progression and metastasis.46 The stiffness of the ECM can stimulate intracellular signaling pathways to regulate cellular behavior. Breast cancer cells, for instance, can notice the increase in ECM stiffness and react by forming increased traction forces, especially via actomyosin and cytoskeleton contractility, promoting their invasion towards the bone microenvironment.47 The complex structure represented by the ECM is composed of fibrous proteins, glycoproteins, proteoglycans, and non-proteoglycan polysaccharides. Each component is crucial in providing mechanical strength, promoting cellular migration, and maintaining ECM assembly or cell signaling.48 Collagen is the most abundant structural fibrous protein of the ECM. It has several subtypes overexpressed in cancer stem cells (CSCs) and tumor-educated host stromal cells. Cancer progression is facilitated by increased collagen deposition and crosslinking performed by cancer-associated fibroblasts (CAFs) through the activation of focal adhesion kinase (FAK) and YAP/TAZ (transcriptional co-regulators).49 Glycoproteins such as fibronectin and laminin are involved in the ECM cohesive network formation by promoting adhesion between cells and ECM components. Other ECM elements, proteoglycans, and polysaccharides play roles in the assembly and buffering of the physical stress of the ECM, respectively.48 Overall, the changes in TMEs and PMNs caused by cell–cell and cell–ECM interactions create a charming environment for the survival of cancer cells at secondary sites after their metastasis. The coordinated work between cancer cells and stromal cells, together with their secreted factors and ECM components, opens the gate for the metastasis of breast cancer cells to their target organs. Therefore, to study breast cancer metastasis, in vitro models that can mimic these complex heterotypic interactions in metastasis cascades are required.
3. Breast cancer metastasis models
Despite an exponentially increasing number of research papers published on cancer biology, our knowledge of deeply understanding this complex disease is restricted. Investigating the complex physiopathology of the disease is crucial to evaluate anti-cancer drugs and eventually come up with specifically targeted personalized treatments. Several in vitro and in vivo models ranging from basic two-dimensional (2D) cell culture systems to more complex animal models have been utilized to investigate breast cancer metastasis (Fig. 3).50 Traditional 2D cell culture systems have long been applied in cancer research. These systems, however, do not accurately mimic physiological circumstances. Therefore, they cannot be translated into clinical approaches. Specifically, in cancer research, 2D models are unable to test heterotypic cell–cell and cell–ECM interactions and communication and, thus, cannot recapitulate the tumor and target site microenvironments.51 These limitations prompted the development of a three-dimensional (3D) cell culture system. 3D culture models bridge the gaps between 2D systems and animal-based models. The accuracy and flexibility of the cell culture are mainly improved by 3D culture conditions. 3D culture models provide physiologically relevant information by resembling native in vivo conditions. Several studies have revealed significant differences in the expression levels of hormone receptors on different breast cancer cells cultured in 2D or 3D culture systems, which consequently led to different responses to targeted and non-targeted chemotherapeutic drugs.52
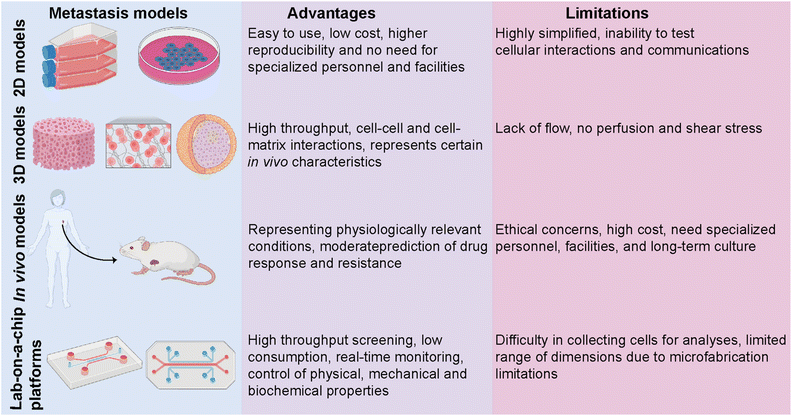 |
| Fig. 3 Comparison of different metastasis models. The advantages and limitations of in vitro 2D and 3D culture systems and in vivo animal-based models along with lab-on-a-chip platforms, are represented. Images were created using https://Biorender.com. | |
In vivo tumor characteristics such as dormancy, hypoxia, invasion and apoptotic phenotype, and drug resistance are better mimicked by 3D-cultured breast cancer cells.51,52 However, despite having several advantages over 2D and animal models, simple 3D culture systems are limited in their ability to mimic the physiological conditions of the microenvironment since they lack spatiotemporal control. In addition to traditional 3D culture approaches, patient-derived organoids (PDOs) and patient-derived explant culture (PDEC) models have been commonly used as 3D ex vivo approaches for studying cancer progression.10,53,54 PDOs carry the advantages of both 3D spheroids and primary cell lines representing the information of the patient.53 Therefore, PDOs can capture the heterogeneity of the parental tumor as well as maintain the genomic and phenotypic features of the tumor of origin, which enables them to become revolutionary preclinical models for personalized medicine.54 On the other hand, tissue-specific constraints, requirement for expertise on specific organoid establishment and the need for tissue- and patient-specific efficiency in the establishment and growth of organoids may restrict their use in cancer metastasis and new drug development.
In vivo animal models can serve as alternatives for 2D and 3D systems by overcoming most of the limitations. One of the most studied in vivo models in cancer research is patient-derived xenografts (PDXs) involving the implantation of a patient's tumor cells or tissue into immunocompromised mice.55 PDXs have been developed as a convenient model, especially for translational cancer research, as they retain the architecture and genomic information of the original tumor. PDX models serve as potential tools for de novo identification of molecular mechanisms and drug resistance, identifying new breast cancer biomarkers, and evaluating experimental therapeutic approaches. Yet, PDXs suffer from limitations, including ethical concerns, high cost, and loss of the contribution of human cells to the parental tumor biology due to the invasion of mouse stromal cells over time. PDX models, indeed, mostly represent the TNBC subtype and thus require more improvements in establishing the other molecular subtypes of breast cancer.56,57
The limitations of in vitro and in vivo models have prompted the development of lab-on-a-chip (LOC) technology as a novel in vitro platform. LOC systems offer a more native-like design complexity that can deepen our understanding of breast cancer biology. Being a highly heterogeneous disease whose progression and dissemination are driven by complex interactions between cellular, genetic and epigenetic factors, breast cancer needs more focused research and a controlled, real-time monitoring provided by LOC technology as a real proxy in vivo. Co-culturing tumor and non-tumor cells together with soluble and secreted factors, applicable mechanical features, and rich extracellular matrix (ECM) content is principally encouraged by LOC platforms. Therefore, modeling, monitoring, and investigating the key events of breast cancer progression are well performed in LOC platforms, where they prevail over traditional approaches.
4. Lab-on-a-chip technology in cancer research
Lab-on-a-chip (LOC) technology is a powerful approach that can integrate different functions including the manipulation of samples and their detection and/or quantification in a single platform. LOC systems offer an integrated platform to mimic physiologically relevant conditions by combining microfluidic technology and cell, tissue, or organ culture and therefore, offer opportunities to study complex mechanisms underlying breast cancer and its metastasis using pre-defined architectures and conditions representative of the in vivo environment.58,59
LOC has its origin in microfluidics, which allows an extremely low amount of fluid processing using highly controlled microchannels.60 LOC technology provides a controlled supply of nutrients, gases, and drugs by laminar flow with a high-throughput screening but low consumption of the reagents.
This advanced technology uses microfluidics science to manipulate fluids at microscale levels within channels that are micrometers in size. Recently, LOC-based in vitro platforms have gained considerable attention to study various aspects of breast cancer metastasis (Table 1). Examples include modeling the early stage of breast cancer to investigate molecular players in disease progression,61 studying cancer immunotherapies to identify new therapeutic approaches,62 and mimicking the metastatic cascade to determine critical insights in each step of metastasis.63 The design complexity achieved in LOC platforms offers insights into specific and effective approaches for the treatment of cancer. Recent attempts have also shown that complex architecture, physiologically relevant flow control and real-time 3D imaging offer crucial insights into breast cancer metastasis in co-cultured TNBC-like cell lines. For example, Chi et al. introduced a three-layered microfluidic platform called L-TumorChip.64 The PDMS-based system allowed the controlled formation and investigation of tumor microvasculature and tumor-stromal microenvironments (Fig. 4a and b).64 The L-TumorChip offers flow control in a physiologically acceptable range. The top channel was lined with monolayer HMVEC cells and cancer cells encapsulated in Matrigel were filled in the bottom chamber. Controlled cellular communication was mediated via the middle porous PDMS membrane. The effects of stromal cells such as normal and cancer-associated fibroblasts (CAFs), endothelial cells and mesenchymal stem cells on the doxorubicin treated MDA-MB-231 cells are investigated. These approaches collectively represent the system's potential for drug screening, thus leading to the development of new therapeutic drugs.
Table 1 Lab-on-a-chip (LOC) systems used for breast cancer metastasis research
Applications |
Cell types |
Approaches |
References |
Migration and invasions |
MCF-7, MDA-MB-231, and SUM-159PT |
Subtype-specific invasion of breast cancer cells |
Moon et al.67 |
MDA-MB-231, breast CAFs, and normal HMFs |
The roles of different cellular milieus and ECMs on breast cancer invasion |
Lugo-Cintron et al.68 |
Macrophages (RAW 264.7 and MDMs), MDA-MB-231, PC3, and MDA-MB-4355 |
Macrophage-induced breast cancer cell migration |
Li et al.70 |
MDA-MB-231, WI-38, BRL3A, and MCF10A |
Invasion/chemotaxis preferences to different homing sites |
Firatligil-Yildirir et al.71 |
Invasion and extravasation |
MDA-MB-231 and hMVECs |
Study on steps involved in extravasation |
Jeon et al.73 |
MDA-MB-231, hMVECs, hBM-MSC, and osteoblast-differentiated hBM-MSCs |
Organ-specific extravasation of breast cancer cells |
Jeon et al.74 |
MDA-MB-231, HUVECs, and MLO-Y4 |
The role of mechanical stimuli on breast cancer metastasis to the bone |
Mei et al.75 |
MDA-MB-231, WI-38, BRL3A, MCF10A, and HUVECs |
Extravasation preferences to different homing sites: lung, liver, or breast |
Firatligil-Yildirir et al.71 |
Modeling metabolic and biochemical properties |
MCF-7 |
Behavioral changes of breast cancer cells under hypoxic conditions |
Grist et al.79 |
MCF-10A, MCF-7, MDA-MB-231, HUVECs, and NHLF |
Metastasis-related gene expression and extravasation under hypoxic conditions |
Song et al.80 |
Breast cancer diagnosis and treatment |
CTCs from HER2-enriched patient samples, AU-565, and RAMOS |
Quantification of CTCs in blood samples with a 3D-flow optofluidic chip |
Pedrol et al.86 |
MCF-7, MDA-MB-231, SK-BR-3, and NFs |
Detection of breast cancer derived exosomes |
Fang et al.87 |
Blood samples from breast cancer patients |
One-step isolation of CTCs by the negative enrichment approach |
Lee et al.88 |
Nanomedicine |
MCF-7 and ASCs |
Evaluation of PDT efficiency in a 3D breast cancer tissue model |
Yang et al.93 |
MDA-MB-434 |
Study and the prediction of nanoparticle distribution in breast cancer tissue |
Albanese et al.94 |
BT549 and T47D |
Nanoparticle-based drug delivery approaches |
Chen et al.96 |
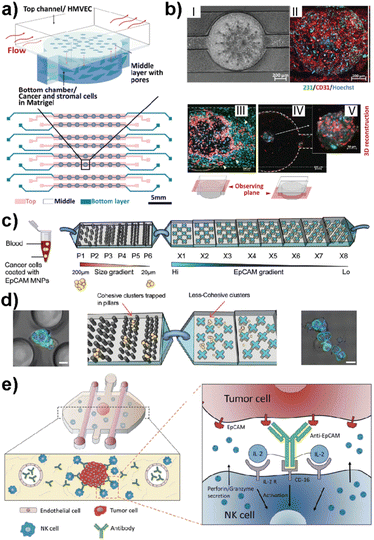 |
| Fig. 4 Representative LOC models used in cancer research. (a) A three-layered microfluidic platform called L-TumorChip in which cancer and stromal cells were added to the bottom channel within Matrigel and endothelial cells to the top channel. (b) HMVEC monolayer and cell migration patterns of MDA-MB-231 and HMVEC in L-TumorChip, (I) phase contrast image, (II) CD-31 immunostained images, (III) images of MDA-MB-231 and HMVEC at day 7 with the focal plane at the top channel, (IV) image taken with the focal plane at the bottom chamber, and (V) 3D reconstructed spheroid of MDA-MB-231/HMVEC. Reproduced with permission from ref. 64. (c) The design of a bimodular microfluidic device called PillarX to capture circulating tumor cells (CTCs). The pillar part of the device captures CTCs according to size, while the X part captures due to the EpCAM surface marker. (d) Clusters are trapped in pillars and less cohesive ones pass to the X device to be captured using their surface marker expression levels. Reproduced with permission from ref. 65. (e) Ductal carcinoma in situ (DCIS) model to investigate molecular and metabolic breast cancer biology research. The immunotherapies and cell cytotoxicity were studied in the platform using the immune cell recruitment, penetration, and permeability of the solid tumors to therapeutic antibodies. Reproduced with permission from ref. 62 © 2018 Taylor & Francis Group, LLC. | |
Ayuso et al. developed one of the first LOC-based in vitro models that mimics ductal carcinoma in situ (DCIS).61 The design allowed the generation of a hypoxia and nutrient starved microenvironment by DCIS cells which, furthermore, allowed selective targeting of hypoxic DCIS cells. In another study, the same model was used to study natural killer (NK) cell immunotherapies and antibody-dependent cell cytotoxicity using MCF-7 spheroids embedded in a collagen matrix (Fig. 4e).62 Nagaraju et al. developed a LOC-based vascular model to study the breast cancer metastasis cascade.63 This model revealed an enhanced invasion of MDA-MB-231 cells into the stroma in the presence of vasculature. Microfluidics also allow functionalized architectures with tunable shape, size, and geometry. Such designs can be used for rapid detection of metastatic cells based on their size and mechanical deformation. Green et al. recently reported a bimodular microfluidic device called PillarX (Fig. 4c and d).65 The design consists of pillared structures for size gradient based isolation and magnetic X-shaped structures for EpCAM gradient based isolation. This device allowed the isolation of single and clusters of CTCs from whole blood samples using functionalized magnetic nanoparticles (Fig. 4c and d). This device efficiently profiles and captures CTCs from whole blood which might be the basis of an effective prognostic tool for cancer.
In the following sections, we highlight the application of LOC platforms for investigating primary and secondary tumor sites and tissue-specific preferences of breast cancer cells and developing precision therapeutics.
5. Lab-on-a-chip technology to study breast cancer metastasis
5.1. Modelling cell migration and invasion
Cell migration and invasion are the key events observed at the beginning of breast cancer progression. Cancer cells possess different dissemination patterns from their primary site, either with individual or collective cell migration mechanisms. These mechanisms are related to various molecular events, including cell–ECM interactions through integrins and cell–cell adhesion and communication mediated by adhesion receptors and gap junctions. The collective cell migration and invasion mechanisms are predominantly observed in breast cancer, especially invasive ductal carcinoma (IDC) and invasive lobular carcinoma (ILC), contributing to its distant metastasis. These cellular dynamics of breast cancer cells are actively regulated by the tumor microenvironment leading to molecular and functional changes within the residing cells. Accordingly, the modulation of the TME in terms of ECM modifications, biochemical and molecular cell parameters, as well as cellular and molecular interactions is one of the key actors driving cancer phenotype and progression.66
Therefore, improving breast cancer treatments critically depends on understanding breast cancer progression, the role of the local tumor microenvironment and subtype-specific characteristics of breast cancer. LOC platforms have been used to investigate the contribution of different cell types, ECM components, chemokines and growth factors to cancer cell migration and invasiveness. Moon et al. studied the subtype-specific invasion potential of breast cancer cells using an in vitro invasive ductal carcinoma-on-a-chip (IDC-on-a-chip) platform (Fig. 5a and b).67 In this mammary duct-mimicking platform, a breast cancer cell duct was formed and surrounded by a 3D collagen matrix. The developed IDC-on-chip model is well suited to mimic cellular growth and local invasion of cell lines corresponding to luminal A subtype (MCF-7) and TNBC subtype (MDA-MB-231 and SUM-159PT). In the platform, TNBC cell lines revealed different invasive characteristics. Notably, higher invasiveness was observed in SUM-159PT cells with collective cell migration and matrix degradation. On the other hand, as expected, MCF-7 showed non-invasive characteristics indicating the capacity of the IDC-on-chip platform to assess subtype-specific invasive characteristics. However, the complexity of the TME was not mimicked to explore the differences in the invasive characteristics of breast cancer cells, especially towards different target sites specific to each subtype. Lugo-Cintron et al. investigated the importance of the TME on the invasion capacity of MDA-MB-231 cells using a 3D microfluidic co-culture system (Fig. 5c and d).68 Their study specifically focused on the effect of fibroblasts and different ECM protein compositions on the migration of human breast cancer cell line. MDA-MB-231 cells when co-cultured with breast cancer-associated fibroblasts (CAFs) or normal human mammary fibroblasts (HMFs), showed an increased invasion capacity into the fibronectin-rich collagen matrix compared to the collagen-only control. This study demonstrates the relevance of the platform to observe the contribution of different cellular components and matrix compositions to breast cancer invasion.
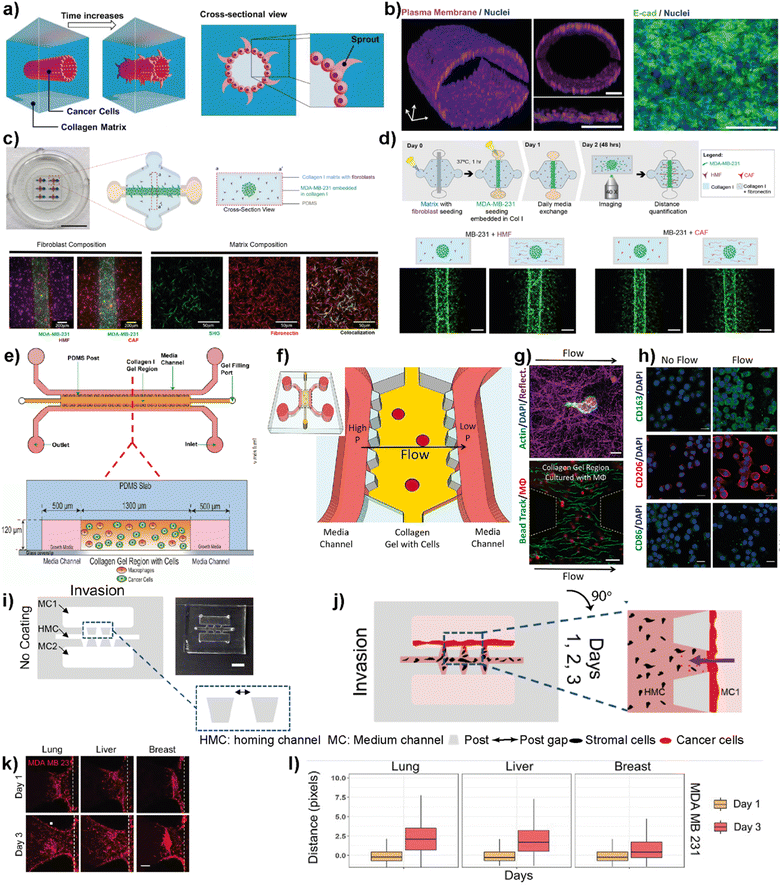 |
| Fig. 5 Representative examples showing LOC platforms used to model cell migration and invasion. (a) Schematic representation of the IDC-on-a-chip model used to study subtype-specific invasion potential of breast cancer cells. (b) MCF-7 lumen structure and cross-sectional images in the IDC-on-a-chip platform showing the plasma membrane (magenta), nuclei (blue) and E-cadherin immunostaining (green) of the MCF-7 duct. Reproduced with permission from ref. 67 Copyright: © 2020 Moon et al. under the terms of the Creative Commons Attribution License. (c) 3D co-culture model (top) to study the effect of TME on the invasion capacity of MDA-MB-231 cells focusing on fibroblasts and different ECM protein compositions (scale bar: 10 mm) and images (bottom) showing MDA-MB-231 co-cultures with normal fibroblasts, cancer-associated fibroblasts and a collagen I matrix containing fibronectin. (d) Schematics showing cell seeding, media exchanges and imaging after cell migration in a 3D co-culture platform (top) and images showing the effect of ECM on the co-culture of cancer cells (bottom). Reproduced with permission from ref. 68 Copyright © 2020 by the authors under the terms and conditions of the Creative Commons Attribution (CC BY) license. (e) Schematics of a LOC system used to study the role of macrophages in the migration and movements of breast cancer cells. The macrophages (green) and cancer cells (red) were embedded in 3D collagen matrices (orange) to form a suitable TME within the platform. Reproduced with permission from ref. 69 Copyright © 2016 American Association for Cancer Research. (f) Schematics of a LOC system used to study the effect of interstitial flow on macrophage polarization. (g) Fluorescent images showing the interaction of macrophages (top) with the collagen I matrix (scale bar 10 μm) and quantification of interstitial flow in the LOC system (bottom) using bead tracking (scale bar 50 μm). (h) Immunofluorescent images showing the effect of flow on the expression of M1 and M2 markers. Reproduced with permission from ref. 70 © 2018 Li et al. under the Creative Commons License (http://creativecommons.org/licenses/by-nc-sa/3.0). Published by The American Society for Cell Biology. (i and j) Schematics and photogram of the LOC platform used to study breast cancer invasion chemotaxis. The invasion/chemotaxis behaviors of breast cancer cells (red) towards in vitro generated homing target sites (represented by black colored homing cells) – lung, liver or breast are shown within the IC-chip platform (scale bar: 5 mm). (k and l) Results showing the ability of the LOC platform to observe the differences in the invasion preferences of breast cancer cells to lung, liver or breast environments. Reproduced with permission from ref. 71 Copyright © 2021 Wiley Periodicals LLC. | |
Macrophages residing within the tumor microenvironment are the key promoters of cancer cell dissemination. They have been shown to increase cancer cell migration and invasion, especially by enhancing the migration speed and continuity in 3D collagen matrices. Li et al. used a microfluidic 3D platform to study how macrophage-secreted TNF-α and TGFβ1 affect the dynamics of cancer cell migration (Fig. 5e).69 In this study, the authors used a PDMS-based microfluidic platform and 3D collagen I matrix. Using this platform, the cell migration dynamics of MDA-MB-231, PC3 and MDA-MB-435S cell lines in the presence of macrophages was investigated. It was found that in the presence of Raw 264.7 macrophages as well as primary macrophages such as human monocyte-derived macrophages (MDMΦ) and murine bone marrow-derived macrophages, cell migration speed and directedness increased in all three cancer cell lines. More importantly, a similar increase in speed and directedness was observed when macrophages were cultured without direct physical contact (but communicated via secreted paracrine factors) with cancer cells. Macrophages also upregulated the expression of MMP1 and MT-MMP in cancer cells. The cytokines, TNF-α and TGFβ1, which are released from macrophages to the TME, were identified as the key regulators to enhance the cancer cell migration dynamics including total speed and directedness.
The same LOC platform was also used to mimic the interstitial fluid flow reported in tumors under pathophysiological conditions to study the response of macrophages (Fig. 5f–h).70 Interstitial flow is known to affect cancer cell and fibroblast migration. Using this platform, the cell migration dynamics of cancer cells including metastatic breast cancer cell line, MDA-MB-231, were investigated and it was shown that interstitial flow is a critical regulator of immune modulation in the TME. Firatligil-Yildirir et al. utilized organ-on-a-chip platforms to investigate the invasion/chemotaxis behavior of TNBC cells (Fig. 5i–l).71 The modular LOC platform allows the generation of different homing sites including lung, liver and breast microenvironments simulated in a Matrigel matrix. It was shown that MDA-MB-231 TNBC cells preferred to invade the lung site compared to the liver or breast microenvironments. The results indicate the ability of the LOC platform to determine the invasion/chemotaxis phenotype of MDA-MB-231 cells qualitatively and quantitatively.
5.2. Modeling intravasation and extravasation
The complex metastasis process involves the invasion of cancer cells into blood vessels to enter blood circulation, followed by the exit from the circulation at the specific secondary loci (Fig. 2). These intravasation and extravasation steps are mainly directed by the adhesion molecules on the surface of cancer cells that support their attachment to the vascular endothelium.72 During each step, cancer cells secrete cytokines to induce vascular hyperpermeability.72 This allows the transmigration of cancer cells through the vascular endothelial barrier. Therefore, understanding the mechanisms involved in the breakage of vascular integrity at the primary and secondary loci and how cancer cells can modulate vascular properties is of great interest in developing novel therapeutic approaches. In this sense, different breast tumor-on-chip platforms have been modelled to gain insights into these interconnected processes. Jeon et al. investigated the critical steps of extravasation using a three-channel LOC platform (Fig. 6a and b).73 The platform allowed the investigation of cancer cell adhesion to the endothelial barrier and their trans-endothelial migration through the vascular monolayer and proliferation at secondary target sites. In their platform, the center channel was used to form an endothelium barrier with human microvascular endothelial cells (hMVECs). Once an intact monolayer was formed, MDA-MB-231 breast cancer cells were also introduced into the same central channel. The other two channels were used for media supply, and they were connected to the center channel through chambers where 3D collagen-based extracellular space was formed. This system enabled detailed visualization of extravasation steps and the proliferation rate of MDA-MB-231 cells at the collagen matrix after their extravasation across the intact endothelial barrier. In another study, the same group utilized a 3D microfluidic system to evaluate the organ-specific extravasation of breast cancer cells into in vitro-generated microvascularized bone-mimicking matrices (Fig. 6c).74 The microvascular network was created within the LOC model with the coculture of endothelial and human bone marrow mesenchymal stem cell-derived (hBM-MSC) mural cells. The coculturing resulted in more branched vascular structures. Besides, osteoblast-differentiated hBM-MSCs were seeded together with the aforementioned cells to form a bone-mimicking microenvironment within the platform. This study revealed a functional in vitro model to gain insights into breast cancer extravasation by monitoring the flow, adhesion, and metastasis of metastatic MDA-MB-231 cells through human microvascular networks. Mei et al. introduced a metastasis on-a-chip model where breast cancer bone metastasis was mimicked by applying physiologically relevant mechanical stimuli to osteocytes.75 Bone fluid flow stimulation, together with the intercellular communication between osteocytes, endothelial cells, and breast cancer cells, was integrated into the platform. The combination enabled physiologically relevant conditions to study the roles of mechanical stimuli in breast cancer bone metastasis. Humayun et al. used a PDMS-based LOC platform as a human organotypic vascularized microfluidics model to study breast cancer cell extravasation.76 The platform allowed the investigation of cancer–vasculature interactions and the effect of secreted factors during breast cancer cell extravasation (Fig. 6d–h). The results indicated that IL-6, MMP-3 and IL-8 paracrine signaling either independently or in combination induced the disruption of the vascular network, degradation of the basement membrane and cancer cell extravasation. Therefore, the organotypic model has the potential to provide crucial insights on how cancer–vascular interactions are performed for breast cancer cells to extravasate leading to the evaluation of therapeutic agents that prevent cancer extravasation. Firatligil-Yildirir et al. investigated the extravasation capacities of MDA-MB-231 cells towards in vitro generated lung, liver and breast homing sites in a LOC platform (Fig. 6i).71 Notably, an intact endothelial monolayer was formed by HUVEC-C cell lines while tissue-specific fibroblasts generated lung, liver and breast target sites. Consistent with the clinical data, MDA-MB-231 cells preferred to extravasate more to the lung microenvironment than to other homing sites providing a relevant platform that can distinguish and determine different metastatic phenotypes of breast cancer cells.
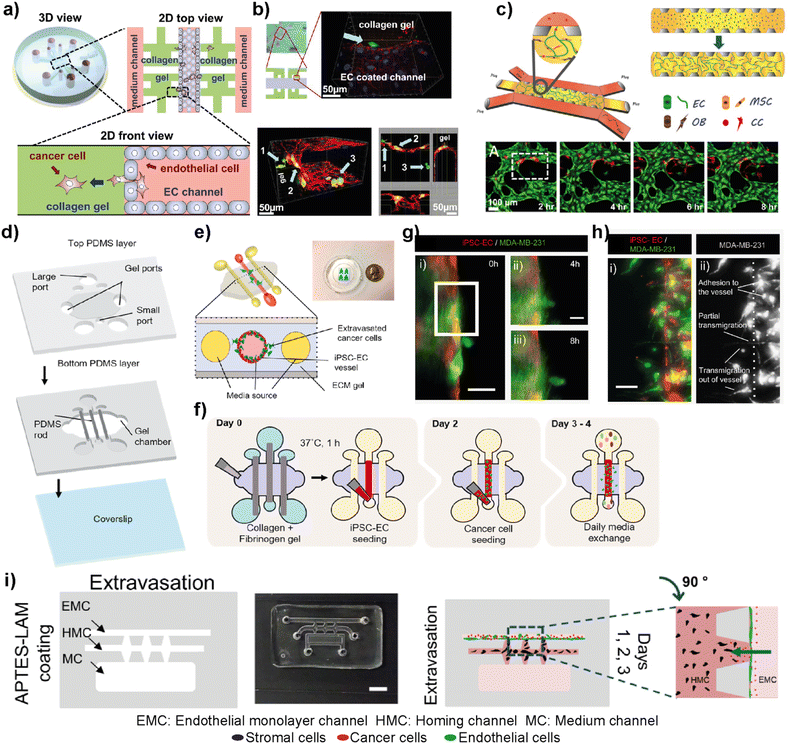 |
| Fig. 6 Representative examples showing LOC platforms used to model intravasation and extravasation processes. (a) Schematic representation of the LOC system used for detailed visualization of extravasation steps and the proliferation rate of MDA-MB-231 cells at the collagen matrix after their extravasation across the intact endothelial barrier. (b) Transmigration of cancer cells (green) across the endothelium and extravasation into the collagen matrix (top) and a confocal scan showing different locations of cancer cells (bottom). Reproduced with permission from ref. 73 Copyright ©2013 Jeon et al. under the terms of the Creative Commons Attribution License. (c) Schematic representation of the LOC system evaluating the organ-specific extravasation capacity of breast cancer cells into bone-mimicking matrices (top) and real-time monitoring (bottom) of extravasation of cancer cells (red) introduced into the vascular network (HUVECs stained with green). Reproduced with permission from ref. 74 Copyright © 2015 National Academy of Sciences. (d) A human organotypic vascularized model to study breast cancer extravasation and cancer–vascular interactions. (e) Cross-section view of the model mimicking the blood vessel structure with the ECM around it and showing the extravasation of cancer cells across the vessel. (f) Experimental workflow for the BCC extravasation model. Fluorescent images of MDA-MB-231 cells (g) extravasating at 4 h (ii) and 8 h (iii) and (h) taking different morphologies during extravasation such as adhesion to the internal surfaces of the vessel, partial transmigration, and full transmigration out of the vessels (i and ii). Reproduced with permission from ref. 76 Copyright © 2021 Elsevier B.V. (i) In the EX-chip platform, the extravasation capacity of MDA-MB-231 cells to in vitro generated lung, liver and bone sites was determined (scale bar: 5 mm). Reproduced with permission from ref. 71 Copyright © 2021 Wiley Periodicals LLC. | |
5.3. Modeling metabolic and biochemical properties
Hypoxia, the reduction of oxygen availability, is one of the key stimuli for invasion, metastasis, and drug resistance.77 Hypoxia observed within the tumor, especially in solid tumors such as breast cancer, is an effective factor for tumor growth and progression.78 Therefore, several lab-on-a-chip platforms were developed to explore the effects of hypoxic conditions on breast cancer progression and its response to chemotherapeutic drugs. In this context, Grist et al. investigated the roles of hypoxia in the behaviors of MCF7 breast cancer cells within 3D spheroids under spatiotemporal oxygen control in a microfluidic system.79 The demonstrated LOC platform provided physiologically relevant hypoxia conditions with precise control of oxygen concentration and real-time visualization of cell responses to tunable oxygen profiles. Swelling and shrinking dynamics of tumor spheroids, as well as their ability to uptake the doxorubicin drug during hypoxic exposure (0–10% O2) were reported. Therefore, the study provides information about the utility and potential of the platform to monitor tumor models under dynamic, time-varying, and/or controlled oxygen conditions during doxorubicin treatment.
Song et al. used a lab-on-a-chip platform to evaluate breast cancer extravasation under different oxygen conditions.80 MCF10A, MCF-7 and MDA-MB-231 cells were pre-conditioned by hypoxia and then seeded into the microvascular network formed by HUVECs and NHLFs (normal human lung fibroblasts) within the LOC platform. Under hypoxic conditions, breast cancer cells showed increased levels of hypoxia-inducible factor 1α (HIF-1α), which triggers cancer progression by regulating the expression of genes for cancer invasion and metastasis.81 Therefore, the platform used in this study enables the investigation of changes in breast cancer cell morphology and viability as well as their extravasation dynamics and aggressiveness within in vitro 3D microvasculature under hypoxia.
5.4. Lab-on-a-chip models for breast cancer diagnosis and treatment
Blood circulation transports circulating tumor cells (CTCs), tumor-derived exosomes, cell-free circulating tumor DNA (ctDNA), and RNA, which are essential for tumor growth and metastasis. Therefore, liquid biopsies have gained attention as they have the potential to identify cancer biomarkers to help in early detection and predicting cancer progression and response to therapies.82–85 Several microfluidic platforms have been developed to identify and isolate cancer cells and cancer cell-derived products from liquid biopsies of patients' blood for cancer prognosis and diagnosis. Pedrol et al. designed a 3D-flow focusing optofluidic chip to quantify CTCs in blood samples from metastatic HER2 breast cancer (Fig. 7a).86 The chip allows the confinement of a single cell in a flow-focusing channel integrated with multiple optical fibers. The microfluidic chip was integrated with single-mode and multimode optical fibers. Single-mode pumping fibers were placed perpendicular to the flow channels and multimode fluorescence collecting fibers were placed at an angle of 45° to the flow channel (Fig. 7b).
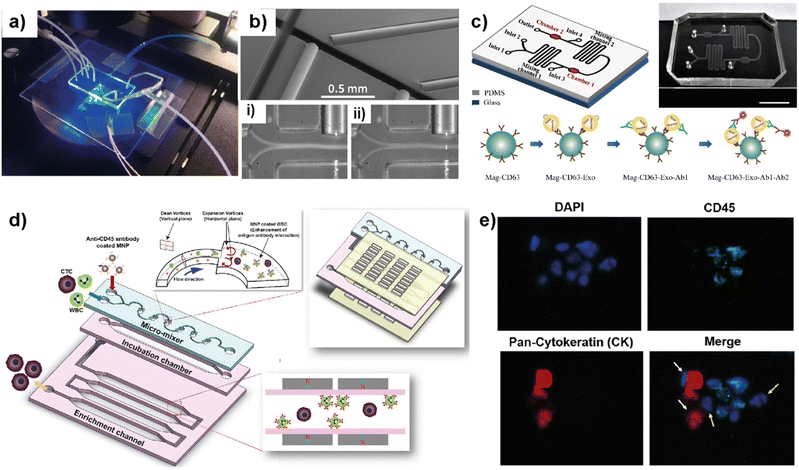 |
| Fig. 7 Representative examples showing LOC platforms used for exosome and CTC isolation from liquid biopsies. (a) Photograph of a 3D flow focusing optofluidic chip. (b) Microscopy image of an optofluidic chip showing irradiating and collecting fibers. Reproduced with permission from ref. 86 Copyright © 2017, Pedrol et al. under the terms of the Creative Commons CC BY license. (c) Schematics of multichannel microfluidic chip design for exosome capture and detection and photographs of the microfluidic chip (scale bar 1 cm). Reproduced with permission from ref. 87 Copyright © 2017 Fang et al. under the terms of the Creative Commons Attribution License. (d) Schematic diagram showing different compartments and components used in a μ-MixMACS chip for one-step CTC isolation. (e) Fluorescent images of primary breast cancer cells separated using the μ-MixMACS chip with DAPI positive, CD45 negative and pan-cytokeratin positive characteristics. Reproduced with permission from ref. 88 Copyright © 2016 Elsevier B.V. | |
The integrated chip allows fluorescence quantification of CTCs from breast cancer liquid samples with signals belonging to HER2 and EpCAM (epithelial cell adhesion molecule) receptors. Using AU-565 (HER2 positive and EpCAM positive) and RAMOS (HER2 negative and EpCAM negative) cell lines it was shown that the results obtained using an integrated optofluidic chip are similar to those obtained in flow cytometry. This type of platform is crucial to define different metastatic degrees and progression of real patient samples leading to production of a platform for early monitoring of tumors for early diagnosis.
Fang et al. designed a LOC platform to detect breast cancer-derived exosomes from patient plasma using CD63 antibody-conjugated magnetic nanoparticles (Mag-CD63).87 The unique multichannel chip consists of immunomagnetic particle collection chambers, mixing channels, inlets and one outlet (Fig. 7c). In this study, three types of breast cancer cell lines; MCF7, MDA-MB-231 and SK-BR-3 were used along with normal fibroblasts (NFs) as a control. The microfluidic device is capable of immunocapturing exosomes under cell culture conditions as well as from patient samples. Furthermore, immunofluorescent staining allowed the detection and quantification of tumor-specific antigens. It was further shown that EpCAM-positive exosomes are higher in breast cancer patient-based plasma compared to healthy control samples. Most of the LOC methods utilize EpCAM to detect CTCs. Due to their heterogeneity and rarity in the bloodstream, the EpCAM-based method may often be unable to capture the whole CTC population and estimate the correct number. In this context, Lee et al. demonstrated a one-step isolation of CTCs using an integrated LOC platform (Fig. 7d and e).88 Instead of routinely using surface markers and considering the CTC size, they performed the negative enrichment approach that selectively removes white blood cells (WBCs). The LOC platform consists of a microfluidic mixer to facilitate the interaction between CD45-conjugated magnetic nanoparticles (MNPs) and white blood cells (WBCs), an incubation chamber to stabilize MNP–WBC conjugation and a magnetic-activated cell sorting module to capture MNP-coated WBCs and elute CTCs. Using breast cancer blood samples of patients who received adjuvant therapy, the authors demonstrated that the negative enrichment provided by the integrated platform minimizes any CTC loss during isolation.
5.5. Lab-on-a-chip platforms for nanomedicine
Nanomedicine is an emerging technology that includes the use of nanostructured materials in applications including cancer diagnosis, imaging, targeted drug delivery and therapeutics.89 This technology has the power to provide improved bioavailability, direct targeting to diseased cells and dose–response compared to other conventional therapeutic methods.90 On the other hand, certain nanoparticles have been shown to have adverse effects by accelerating breast cancer invasion and extravasation when injected intravenously in animal models.91 Therefore, despite having potential benefits and promising therapeutic benefits in preclinical approaches, nanomedicine technology needs a more detailed evaluation to achieve clinical success.92 LOC platforms have been used to perform a more effective evaluation of nanomaterials to be used in clinical practices.
Yang et al. developed an 8-chamber microfluidic system to evaluate the efficiency of gold nanoparticles for photodynamic therapy (PDT) in a 3D breast cancer tissue model (Fig. 8a–c).93 To generate a 3D breast cancer model, breast cancer cells (MCF7) and adipose-derived stromal cells (ASCs) were used. Polyethyleneimine-coated cationic gold nanoparticles (45 ± 12 nm) along with a photosensitizer were injected into the LOC system with a continuous flow to study the PDT efficiency. After irradiating the breast cancer tissue model containing gold nanoparticles using a broadband light source for 24 h, the distribution profiles of nanoparticles within the tissues and morphological changes were evaluated. This study demonstrates that the microfluidic-based breast cancer model has the potential to provide PDT efficiency evaluation by investigating the flow of nanoparticles and thereby monitoring cancer tissue progression. Albanese et al. designed a tumor-on-a-chip platform to investigate the distribution of synthetic carriers within 3D interstitial spaces formed by breast cancer cells embedded in extracellular matrices.94 MDA-MB-435 spheroids were used as a tumor model and fluorescently labelled PEGylated gold nanoparticles of different sizes were used to study tissue accumulation. The 3D cancer tissue microenvironment, the diameters of nanoparticles and their efficiency at targetting receptors and flow conditions are evaluated. It was shown that the presence of nanoparticles inside the spheroid interstitial space was size dependent, with 40 and 70 nm NPs visible within 10 min. On the other hand, larger NPs were excluded from the interstitial spaces. Furthermore, by comparing passive and active targeting, it was found that receptor targeting increased the tissue accumulation of NPs. The in vitro results were further supported by intravenously injecting the NPs into a tumor-bearing animal model. The obtained results indicate the accuracy of the platform to study and then predict nanoparticle distribution in breast cancer tissue providing a useful monitoring method for nanoparticles prior to their use in clinics. Wang et al. reported a tumor-vasculature-on-a-chip (TVOC) model to study the extravasation and accumulation of nanoparticles and understand the effect of enhanced permeation and retention (EPR). The TVOC platform allowed coculture of HUVECs in its top channels and a bottom channel with human ovarian cancer cell (SKOV3) spheroids. This allowed the creation of an endothelial barrier and a 3D tumor environment with a dense ECM, to study extravasation of 20 and 70 kDa dextran, liposomes, or polymer-based nanoparticles and their accumulation in tumor tissues (Fig. 8d).95 The endothelial barrier and dense ECM of tumor sites created in the TVOC model enable the prediction of the transport efficacy of NPs and their tumor accumulation making the microfluidic model powerful for the evaluation of nanoparticles.
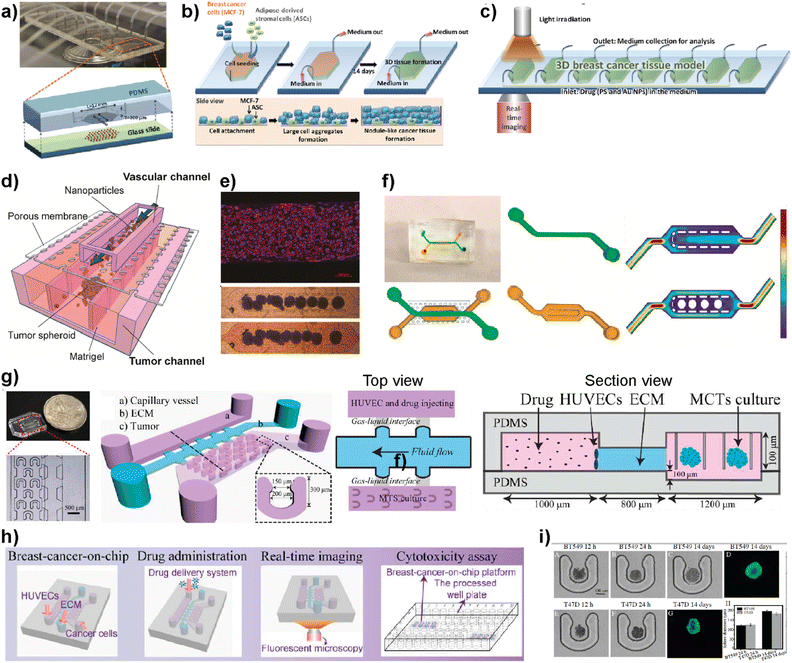 |
| Fig. 8 Examples showing the use of LOC platforms in nanomedicine. (a) Design of an 8-chamber microfluidic platform for photodynamic therapy. (b) Schematics showing 3D breast cancer tissue generation. (c) Schematic image showing the experimental setup of photodynamic therapy evaluation using a 3D breast cancer tissue model formed within the microfluidic platform. Reproduced with permission from ref. 93 Copyright © 2015, Royal Society of Chemistry. (d) Schematic illustration of a tumor-vasculature on-chip (TVOC) model including two layers of microchannels and a membrane between them. The upper channel was designed for modeling 3D tumor vasculature, while the bottom one is for modeling the tumor tissue. (e) A confocal image of a HUVEC monolayer stained with VE-cadherin (red) and nucleus (blue) and microscopy images showing the spheroids in the bottom channel. (f) Design of the top and bottom channels of the TVOC model. The top channel allows for culturing of endothelial cells with steady medium perfusion, while the bottom channel was designed to trap tumor spheroids with its central region. Reproduced with permission from ref. 95 Copyright © 2018 American Chemical Society. (g) Photograph and schematic illustration of a 3D breast cancer-on-chip platform monitoring the transport of nanoparticles. (h) Schematic representation of drug delivery, real-time imaging and cytotoxicity assay. (i) Brightfield and fluorescence images of term culture and spheroid formation inside the chip. Reproduced with permission from ref. 96 Copyright © 2018 Elsevier B.V. | |
Chen et al. demonstrated a breast tumor model on a chip having multicellular tumor spheroids, microvessel walls and an ECM to evaluate nanoparticle-based drug delivery approaches (Fig. 8g–i).96 The evaluation of the drug delivery system was performed using a functionalized carbon dot-based drug delivery system in TNBC and non-TNBC spheroids generated using BT549 and T47D cell lines, respectively. The system designed by the authors provides real-time monitoring for the transport of nanoparticles across the vessel wall and gives information about their penetration ability into tumor spheroids within the platform. This novel 3D breast-cancer-on-chip platform revealed in the study provides both the real-time dynamic flow of nanoparticles and in situ cytotoxicity assessment in a single platform, providing an accurate and cost-effective screening model for better preclinical drug screening.
5.6. Multi-organ-on-chip platforms for breast cancer research
Simple LOC platforms offer cell, tissue or organ specific disease models. However, to understand human physiology, cross-organ communication and complex nature of diseases, advanced multi-organ design is needed. Therefore, multiorgan-on-a-chip (multi-OoC) technology has become one of the important disease models in human health research.97 Multi-OoC includes multiple tissues or organs either in a single platform or interconnected individual platforms. The connection of different engineered organ models allows the mimicking of complex human physiology and systemic diseases to develop body-on-a-chip (BOC)-based personalized treatments (Fig. 9).98 Beyond disease complexity, multi-OoC allows for better understanding of drug metabolism and therapeutic outcomes.99–103 Ronaldson-Bouchard et al. developed an interorgan platform in which matured human heart, bone, liver, and skin tissues were formed and connected to each other through a recirculating vascular flow. The design allowed the study of interdependent organ functions that are typically observed in vivo.99 In the platform, each tissue was separated by an endothelial barrier that allowed the culture of each tissue under its own optimized culture conditions and the communication between tissues through secreted cytokine, exosomes, and/or circulating cells. These conditions formed in the multi-OoC platform enable the recapitulation of pharmacokinetics (PK)/pharmacodynamics (PD) profiles of doxorubicin and the elaboration of miRNA biomarkers of cardiotoxicity associated with doxorubicin. Therefore, this multi-OoC platform may lead to the development of personalized models for systemic diseases and the testing of new treatments. Another study was performed by Aleman et al. where a multi-site metastasis-on-a-chip (MOC) device was explained (Fig. 9d).102 Different bioengineered 3D organoids including colorectal cancer, liver, lung and endothelial constructs were located in the platform so that they could connect with each other, and fluid flow was applied. Their study showed that colorectal cancer cells grow in their primary site, move to the other chambers where liver and lung constructs are placed and start growing in these target sites under recirculating fluid flow. Importantly, this multi-site MOC platform may lead to the study of detailed molecular mechanisms underlying metastatic preferences of cancer cells in a single platform.
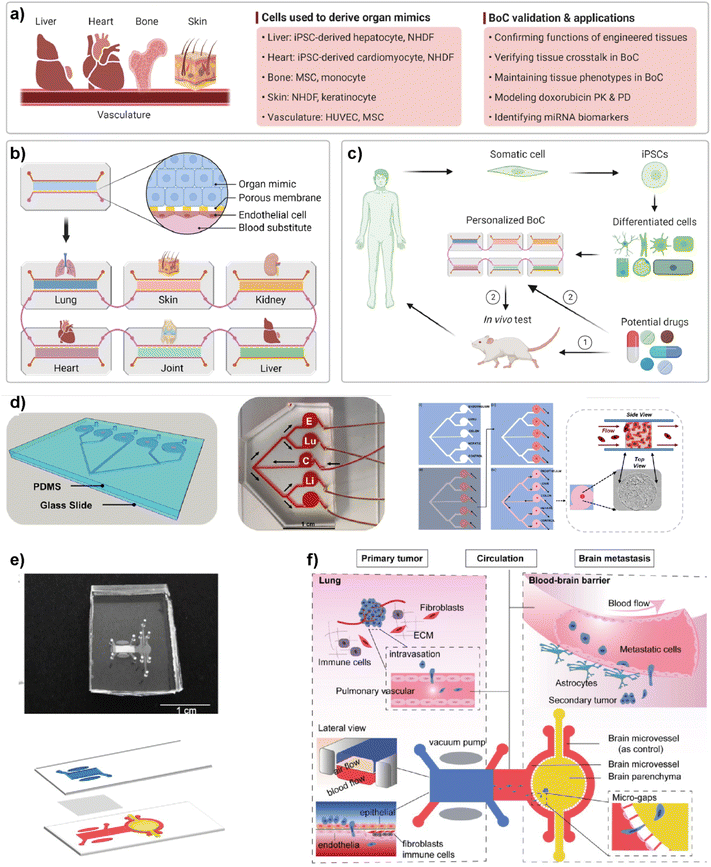 |
| Fig. 9 Representative examples of multi-organ-on-a-chip (multi-OoC) platforms. (a–c) show approaches to multi-OoC systems based on cell-derived organ mimics and body-on-a-chip (BoC) validation. Reproduced with permission from ref. 98 Copyright: © 2022 Li et al. under the terms of the Creative Commons Attribution License. (d) 3D CAD visualization of a metastasis-on-a-chip (MoC) platform, fluid flow overview (C-CRC chamber, Lu-Lung, and E-endothelial), schematics showing sequential patterning and top and side views of MoC. Reproduced with permission from ref. 102 Copyright © 2019 Elsevier B.V. (e) Image of a multi-organ chip (top) designed to study lung cancer metastasis to the brain and its schematic illustration (bottom). (f) Schematic illustrations showing various components and the process used in lung cancer metastasis to the brain. Reproduced with permission from ref. 103 © 2019 Wiley Periodicals, Inc. | |
Liu et al. introduced a multi-organ microfluidic platform allowing the study of brain metastasis of lung cancer (Fig. 9e and f).103 The MOC platform combines a primary tumor (lung cancer) site and metastasis organ (brain) with a functional blood–brain barrier (BBB). Through this approach, the growth at the primary site, extravasation across the BBB, and metastasis to the brain environment were monitored. Different lung cancer cell lines were used to verify the platform and different metastatic capacities were observed among cell lines. More importantly, an elevated expression of the aldo-keto reductase family 1 B10 (AKR1B10) protein was detected in the cells that were being metastasized to the brain parenchyma. Therefore, the introduced multi-organ platform may be used as a feasible approach to studying the pathogenesis of brain metastasis and detecting potential diagnostic biomarkers.
6. Current status and limitations
Lab-on-a-chip platforms developed in recent years have demonstrated great potential as advanced preclinical models for improved diagnosis and personalized therapeutics. This emerging technology offers a physiologically relevant tumor model by providing 3D constructs mimicking in vivo tumor microenvironments. Furthermore, it offers the co-culturing of different cell types residing within the TME of the primary tumor and/or PMNs of secondary target sites. The ability of these platforms to monitor cell–cell and/or cell–matrix interactions in real time enables understanding of metastasis phenomena, drug development and screening. Here, some of the recently developed LOC platforms used in monitoring breast cancer progression, identifying the key players contributing to breast cancer metastasis and evaluating their roles in early diagnosis have been discussed. Because of their ability to mimic the complexity of in vivo conditions, LOC platforms allow more realistic, reliable, and accurate models to study the mechanisms underlying breast cancer and its dissemination to a variety of organs. Therefore, these platforms compensate for conventional 2D and 3D in vitro models and reduce the need for animal model-based testing for effective anticancer therapies. Due to their design flexibility, LOC platforms can be used from basic research to more complex preclinical and clinical settings. LOC technology is currently incorporated into drug development, disease modeling, preclinical trials, pharmacokinetics, and therapeutics testing. Simpler systems are mainly being used during mid-preclinical trials for the discovery and formulation, while more complex multi-OoC models are required for the prediction of efficacy and toxicity of candidate drugs. Therefore, LOC systems, alone or coupled with an analytical or a mathematical model, provide crucial steps to connect basic science research and clinical studies.
However, it should be addressed that LOC platforms still have several challenges to consider before adapting to clinics. The fabrication of the platforms requires expertise and experienced engineering techniques to develop proper networks and architectures similar to those of tumor physiology. Specifically for LOC platforms considering breast cancer progression, it is challenging to integrate different cell types and tissues for mimicking breast metastatic target sites as most of them need different culture media treatments. Furthermore, the availability of primary cells such as primary breast cancer-associated fibroblasts (CAFs) and tissue samples from breast cancer patients is quite limited which might affect the development of more relevant in vivo-like constructs. It is worth noting that there exist challenges to conducting processes such as the detection of cancer-related cells and their separation and isolation along with the analysis within a single platform. Furthermore, one of the main challenges LOC platforms face is the accuracy of these models to predict and identify the human response to existing and/or new anticancer drugs. More extensive collaborations should be performed between different research fields and clinicians to test a large number of patient samples with different molecular signatures to promote the platforms as advanced preclinical tools.
Overall, despite the current challenges, lab-on-a-chip platforms continue to gain more attention regarding their efficiency and applicability for studying breast cancer metastasis and diagnosis leading to the development of personalized medicine by bridging preclinical and clinical approaches.
Author contributions
N. N. and B. F.-Y. together conceived the contents and structure of the review. B. F.-Y. prepared the first version of the manuscript and revised it together with N. N. and O. Y. O. All authors contributed to preparing the final version of the manuscript.
Conflicts of interest
There are no conflicts to declare.
Acknowledgements
The authors acknowledge the Academy of Finland for project funding (No. 352900) and Flagship on Photonics Research and Innovation (PREIN).
Notes and references
- N. Harbeck, F. Penault-Llorca, J. Cortes, M. Gnant, N. Houssami, P. Poortmans, K. Ruddy, J. Tsang and F. Cardoso, Nat. Rev. Dis. Primers, 2019, 5, 66 CrossRef PubMed
.
- K. L. Britt, J. Cuzick and K.-A. Phillips, Nat. Rev. Cancer, 2020, 20, 417–436 CrossRef CAS PubMed
.
- H. Sung, J. Ferlay, R. L. Siegel, M. Laversanne, I. Soerjomataram, A. Jemal and F. Bray, Ca-Cancer J. Clin., 2021, 71, 209–249 CrossRef PubMed
.
- F. Lüond, S. Tiede and G. Christofori, Br. J. Cancer, 2021, 125, 164–175 CrossRef PubMed
.
- F. Andre and L. Pusztai, Nat. Clin. Pract. Oncol., 2006, 3, 621–632 CrossRef PubMed
.
- A. Hennigs, F. Riedel, A. Gondos, P. Sinn, P. Schirmacher, F. Marmé, D. Jäger, H.-U. Kauczor, A. Stieber, K. Lindel, J. Debus, M. Golatta, F. Schütz, C. Sohn, J. Heil and A. Schneeweiss, BMC Cancer, 2016, 16, 734 CrossRef PubMed
.
- C. M. Perou, T. Sørlie, M. B. Eisen, M. van de Rijn, S. S. Jeffrey, C. A. Rees, J. R. Pollack, D. T. Ross, H. Johansen, L. A. Akslen, O. Fluge, A. Pergamenschikov, C. Williams, S. X. Zhu, P. E. Lonning, A. L. Børresen-Dale, P. O. Brown and D. Botstein, Nature, 2000, 406, 747–752 CrossRef CAS PubMed
.
- X. Dai, H. Cheng, Z. Bai and J. Li, J. Cancer, 2017, 8, 3131 CrossRef PubMed
.
- S. M. Hein, S. Haricharan, A. N. Johnston, M. J. Toneff, J. P. Reddy, J. Dong, W. Bu and Y. Li, Oncogene, 2016, 35, 1461–1467 CrossRef CAS PubMed
.
- P. M. Munne, L. Martikainen, I. Räty, K. Bertula, Nonappa, J. Ruuska, H. Ala-Hongisto, A. Peura, B. Hollmann, L. Euro, K. Yavuz, L. Patrikainen, M. Salmela, J. Pokki, M. Kivento, J. Väänänen, T. Suomi, L. Nevalaita, M. Mutka, P. Kovanen, M. Leidenius, T. Meretoja, K. Hukkinen, O. Monni, J. Pouwels, B. Sahu, J. Mattson, H. Joensuu, P. Heikkilä, L. L. Elo, C. Metcalfe, M. R. Junttila, O. Ikkala and J. Klefström, Nat. Commun., 2021, 12, 6967 CrossRef CAS PubMed
.
- J. Kim, R. Villadsen, T. Sørlie, L. Fogh, S. Z. Grønlund, A. J. Fridriksdottir, I. Kuhn, F. Rank, V. T. Wielenga, H. Solvang, P. A. W. Edwards, A.-L. Børresen-Dale, L. Rønnov-Jessen, M. J. Bissell and O. W. Petersen, Proc. Natl. Acad. Sci. U. S. A., 2012, 109, 6124–6129 CrossRef CAS PubMed
.
- G. Ciriello, R. Sinha, K. A. Hoadley, A. S. Jacobsen, B. Reva, C. M. Perou, C. Sander and N. Schultz, Breast Cancer Res. Treat., 2013, 141, 409–420 CrossRef CAS PubMed
.
- P. Poudel, G. Nyamundanda, Y. Patil, M. C. U. Cheang and A. Sadanandam, Breast Cancer Res. Treat., 2019, 5, 21 Search PubMed
.
- X. Dai, H. Cheng, Z. Bai and J. Li, J. Cancer, 2017, 8, 3131–3141 CrossRef PubMed
.
- G. K. Malhotra, X. Zhao, H. Band and V. Band, Cancer Biol. Ther., 2010, 10, 955–960 CrossRef PubMed
.
- J. R. E. Fernandez, B. L. Eckhardt, J. Lee, B. Lim, T. Pearson, R. S. Seitz, D. R. Hout, B. L. Schweitzer, T. J. Nielsen, O. R. Lawrence, Y. Wang, A. Rao and N. T. Ueno, PLoS One, 2020, 15, e0231953 CrossRef PubMed
.
- P. Zagami and L. A. Carey, Breast Cancer Res. Treat., 2022, 8, 95 CAS
.
- P. S. Steeg, Nat. Rev. Cancer, 2016, 16, 201–218 CrossRef CAS PubMed
.
- D. Wirtz, K. Konstantopoulos and P. C. Searson, Nat. Rev. Cancer, 2011, 11, 512–522 CrossRef CAS PubMed
.
- A. I. Riggio, K. E. Varley and A. L. Welm, Br. J. Cancer, 2021, 124, 13–26 CrossRef PubMed
.
- S. Kimbung, N. Loman and I. Hedenfalk, Semin. Cancer Biol., 2015, 35, 85–95 CrossRef CAS PubMed
.
- M. Yousefi, R. Nosrati, A. Salmaninejad, S. Dehghani, A. Shahryari and A. Saberi, Cell. Oncol., 2018, 41, 123–140 CrossRef CAS PubMed
.
- H. Kennecke, R. Yerushalmi, R. Woods, M. C. Cheang, D. Voduc, C. H. Speers, T. O. Nielsen and K. Gelmon, J. Clin. Oncol., 2010, 28, 3271–3277 CrossRef PubMed
.
- C. Bartmann, M. Wischnewsky, T. Stüber, R. Stein, M. Krockenberger, S. Häusler, W. Janni, R. Kreienberg, M. Blettner, L. Schwentner, A. Wöckel and J. Diessner, Arch. Gynecol. Obstet., 2017, 295, 211–223 CrossRef PubMed
.
- E. Daneberg, H. Bardwell, V. R. T. Zanotelli, E. Provenzano, S.-F. Chin, O. M. Rueda, A. Green, E. Rakha, S. Aparicio, I. O. Ellis, B. Bodenmiller, C. Caldas and H. R. Ali, Nat. Genet., 2022, 54, 660–669 CrossRef PubMed
.
- M.-Z. Jin and W.-L. Jin, Signal Transduction Targeted Ther., 2020, 5, 166 CrossRef PubMed
.
- J. Fares, M. Y. Fares, H. H. Khachfe, H. A. Salhab and Y. Fares, Signal Transduction Targeted Ther., 2020, 5, 28 CrossRef PubMed
.
- N. Pashayan, A. C. Antoniou, U. Ivanus, L. J. Esserman, D. F. Easton, D. French, G. Sroczynski, P. Hall, J. Cuzick, D. G. Evans, J. Simard, M. Garcia-Closas, R. Schmutzler, O. Wegwarth, P. Pharoah, S. Moorthie, S. D. Montgolfier, C. Baron, Z. Herceg, C. Turnbull, C. Balleyguier, P. G. Rossi, J. Wesseling, D. Ritchie, M. Tischkowitz, M. Broeders, D. Reisel, A. Metspalu, T. Callender, H. de Koning, P. Devilee, S. Delaloge, M. K. Schmidt and M. Widschwendter, Nat. Rev. Clin. Oncol., 2020, 17, 687–705 CrossRef PubMed
.
- Y. Manmana, T. Kubo and K. Otsuka, TrAC, Trends Anal. Chem., 2021, 135, 116160 CrossRef CAS
.
- D. Caballero, S. Kaushik, V. M. Correlo, J. M. Oliveira, R. L. Reis and S. C. Kundu, Biomaterials, 2017, 149, 98–115 CrossRef CAS PubMed
.
- A. Joshi, A. Vishnu, T. Sakorikar, A. M. Kamal, J. S. Vaidya and H. J. Pandya, Nanoscale Adv., 2021, 3, 5542–5564 RSC
.
- H. Peinado, H. Zhang, I. R. Matei, B. Costa-Silva, A. Hoshino, G. Rodrigues, B. Psaila, R. N. Kaplan, J. F. Bromberg, Y. Kang, M. J. Bissell, T. R. Cox, A. J. Giaccia, J. T. Erler, S. Hiratsuka, C. M. Ghajar and D. Lyde, Nat. Rev. Cancer, 2017, 17, 302–317 CrossRef CAS PubMed
.
- C. F. Monteiro, C. A. Custódio and J. F. Mano, Adv. Ther., 2019, 2, 1800108 CrossRef
.
- Y. Gao, I. Bado, H. Wang, W. Zhang, J. M. Rosen and X. H.-F. Zhang, Dev. Cell, 2019, 49, 375–391 CrossRef CAS PubMed
.
- I. Primac, E. Maquoi, S. Blacher, R. Heljasvaara, J. V. Deun, H. Y. H. Smeland, A. Canale, T. Louis, L. Stuhr, N. E. Sounni, D. Cataldo, T. Pihlajaniemi, C. Pequeux, O. D. Wever, D. Gulberg and A. Noel, J. Clin. Invest., 2019, 129, 4609–4628 CrossRef CAS PubMed
.
- Y. Attieh, A. G. Clark, C. Grass, S. Richon, M. Pocard, P. Mariani, N. Elkhatib, T. Betz, B. Gurchenkov and D. M. Vignjevic, J. Cell Biol., 2017, 216, 3509–3520 CrossRef CAS PubMed
.
- R. Lappano, M. Talia, F. Cirillo, D. C. Rigiracciolo, D. Scordamaglia, R. Guzzi, A. M. Miglietta, E. M. D. Francesco, A. Belfiore, A. H. Sims and M. Maggiolini, J. Exp. Clin. Cancer Res., 2020, 39, 153 CrossRef CAS PubMed
.
- B. S. Hill, A. Sarnella, G. DÁvino and A. Zannetti, Semin. Cancer Biol., 2020, 60, 202–203 CrossRef PubMed
.
- A. Muller, B. Homey, H. Soto, N. Ge, D. Catron, M. E. Buchanan, T. McClanahan, E. Murphy, W. Yuan, S. N. Wagner, J. L. Barrera, A. Mohar, E. Verástegui and A. Zlotnik, Nature, 2001, 410, 50–56 CrossRef CAS PubMed
.
- R. Romero-Moreno, K. J. Curtis, T. R. Coughlin, M. C. Miranda-Vergara, S. Dutta, A. Natarajan, B. A. Facchine, K. M. Jackson, L. Nystrom, J. Li, W. Kaliney, G. L. Niebur and L. E. Littlepage, Nat. Commun., 2019, 10, 4404 CrossRef PubMed
.
- A. M. Høye and J. T. Erler, Am. J. Physiol.: Cell Physiol., 2016, 310, C955–C967 CrossRef PubMed
.
- A. Naba, K. R. Clauser, S. Hoersch, H. Liu, S. A. Carr and R. O. Hynes, Mol. Cell. Proteomics, 2021, 11, M111.014647 CrossRef PubMed
.
- R. O. Hynes and A. Naba, Cold Spring Harbor Perspect. Biol., 2012, 3, a004903 Search PubMed
.
- M. W. Pickup, J. K. Mouw and V. M. Weaver, EMBO Rep., 2014, 15, 1243–1253 CrossRef CAS PubMed
.
- E. K. Paluch, C. M. Nelson, N. Biais, B. Fabry, J. Moeller, B. L. Pruitt, C. Wollnik, G. Kudryasheva, F. Rehfeldt and W. Federle, BMC Biol., 2015, 13, 47 CrossRef PubMed
.
- T. R. Cox and J. T. Erler, Clin. Cancer Res., 2014, 20, 3637–3643 CrossRef CAS PubMed
.
- M. Kalli and T. Stylianopoulos, Front. Oncol., 2018, 8, 55 CrossRef PubMed
.
- S. Nallanthighal, J. P. Heisrman and D.-J. Cheon, Front. Cell Dev. Biol., 2019, 7, 86 CrossRef PubMed
.
- J. Z. Kechagia, J. Ivaska and P. Roca-Cusachs, Nat. Rev. Mol. Cell Biol., 2019, 20, 457–473 CrossRef CAS PubMed
.
-
(a) B. Weigelt, J. L. Peterse and L. J. van't Veer, Nat. Rev. Cancer, 2005, 5, 591–602 CrossRef CAS PubMed
;
(b) Y. Imamura, T. Mukohara, Y. Shimono, Y. Funakoshi, N. Chayahara, M. Toyoda, N. Kiyota, S. Takao, S. Kono, T. Nakatsura and H. Minami, Oncol. Rep., 2015, 33, 1837–1843 CrossRef CAS PubMed
;
(c) J. C. Fontoura, C. Viezzer, F. G. dos Santos, R. A. Ligabue, R. Weinlich, R. D. Puga, D. Antonow, P. Severino and C. Bonorino, Mater. Sci. Eng., C, 2020, 107, 110264 CrossRef CAS PubMed
.
- C. Jubelin, J. Muñoz-Garcia, L. Griscom, D. Cochonneau, E. Ollivier, M.-F. Heymann, F. M. Vallette, L. Oliver and D. Heymann, Cell Biosci., 2022, 12, 155 CrossRef PubMed
.
-
(a) E. Koedoot, L. Wolters, M. Smid, P. Stoilov, G. A. Burger, B. Herpers, K. Yan, L. S. Price, J. W. M. Martens, S. E. Le Dévédec and B. van de Water, Sci. Rep., 2021, 7259 CrossRef CAS PubMed
;
(b) P. Boix-Montesinos, P. M. Soriano-Teruel, A. Armiñán, M. Orzáez and M. J. Vicent, Adv. Drug Delivery Rev., 2021, 173, 306–330 CrossRef CAS PubMed
.
- L. Liu, L. Yu, Z. Li, W. Li and W. Huang, J. Transl. Med., 2021, 19, 40 CrossRef CAS PubMed
.
- J. Wang, C. Chen, L. Wang, M. Xie, X. Ge, S. Wu, Y. He, X. Mou, C. Ye and Y. Sun, Front. Oncol., 2022, 12, 872531 CrossRef PubMed
.
- Y. S. DeRose, K. M. Gligorich, G. Wang, A. Georgelas, P. Bowman, S. J. Courdy, A. L. Welm and B. E. Welm, Curr. Protoc. Pharmacol., 2013, 60, 14.23.1–14.23.43 Search PubMed
.
- M. D. Matossian, A. A. Giardina, M. K. Wright, S. Elliott, M. M. Loch, K. Nguyen, A. H. Zea, F. H. Lau, K. Moroz, A. I. Riker, S. D. Jones, E. C. Martin, B. A. Bunnell, L. Miele, B. M. Collins-Burow and M. E. Burow, Women's Health Rep., 2020, 1, 383–392 CrossRef PubMed
.
- J. R. Whittle, M. T. Lewis, G. J. Lindeman and J. E. Visvader, Breast Cancer Res., 2015, 17, 17 CrossRef PubMed
.
- J. El-Ali, P. K. Sorger and K. F. Jensen, Nature, 2006, 442, 403–411 CrossRef CAS PubMed
.
- J. M. Ayuso, M. Virumbrales-Muñoz, J. M. Lang and D. J. Beebe, Nat. Commun., 2022, 13, 3086 CrossRef CAS PubMed
.
- G. M. Whitesides, Nature, 2006, 442, 368–373 CrossRef CAS PubMed
.
- J. M. Ayuso, A. Gillette, K. Lugo-Cintrón, S. Acevedo-Acevedo, I. Gomez, M. Morgan, T. Heaster, K. B. Wisinski, S. P. Palecek, M. C. Skala and D. J. Beebe, EBioMedicine, 2018, 37, 144–157 CrossRef PubMed
.
- J. M. Ayuso, R. Truttschel, M. M. Gong, M. Humayun, M. Virumbrales-Munoz, R. Vitek, M. Felder, S. D. Gillies, P. Sondel, K. B. Wisinski, M. Patankar, D. J. Beebe and M. C. Skala, OncoImmunology, 2019, 8, 1553477 CrossRef PubMed
.
- S. Nagaraju, D. Truong, G. Mouneimne and M. Nikkhah, Adv. Healthcare Mater., 2018, 7, 1701257 CrossRef PubMed
.
- C. W. Chi, Y. H. Lao, A. H. R. Ahmed, E. C. Benoy, C. Li, Z. Dereli-Korkut, B. M. Fu, K. W. Leong and S. Wang, Adv. Healthcare Mater., 2020, 9, e2000880 CrossRef PubMed
.
- B. J. Green, M. Marazzini, B. Hershey, A. Fardin, Q. Li, Z. Wang, G. Giangreco, F. Pisati, S. Marchesi, A. Disanza, E. Frittoli, E. Martini, S. Magni, G. V. Beznoussenko, C. Vernieri, R. Lobefaro, D. Parazzoli, P. Maiuri, K. Havas, M. Labib, S. Sigismund, P. P. Di Fiore, R. H. Gunby, S. O. Kelley and G. Scita, Small, 2022, 18, 2206567 CrossRef CAS PubMed
.
- V. Salvatore, G. Teti, S. Focaroli, M. C. Mazzotti, A. Mazzotti and M. Falconi, Oncotarget, 2017, 8, 9608–9616 CrossRef PubMed
.
- H. Moon, N. Ospina-Muñoz, V. Noe-Kim, Y. Yang, B. D. Elzey, S. F. Konieczny and B. Han, PLoS One, 2020, 15, e0234012 CrossRef CAS PubMed
.
- K. M. Lugo-Cintron, M. M. Gong, J. M. Ayuso, L. A. Tomko, D. J. Beebe, M. Virumbrales-Muñoz and S. M. Ponik, Cancers, 2020, 12, 1173 CrossRef CAS PubMed
.
- R. Li, J. D. Hebert, T. A. Lee, H. Xing, A. Boussommier-Calleja, R. O. Hynes, D. A. Lauffenburger and R. D. Kamm, Cancer Res., 2017, 77, 279–290 CrossRef CAS PubMed
.
- R. Li, J. C. Serrano, H. Xing, T. A. Lee, H. Azizgolshani, M. Zaman and R. D. Kamm, Mol. Biol. Cell, 2018, 29, 1927–1940 CrossRef CAS PubMed
.
- B. Firatligil-Yildirir, G. Bati-Ayaz, I. Tahmaz, M. Bilgen, D. Pesen-Okvur and O. Yalcin-Ozuysal, Biotechnol. Bioeng., 2021, 118, 3799–3810 CrossRef CAS PubMed
.
- A. K. Shenoy and J. Lu, Mol. Cell. Oncol., 2017, 4, e126067 Search PubMed
.
- J. S. Jeon, I. K. Zervantonakis, S. Chung, R. D. Kamm and J. L. Charest, PLoS One, 2013, 8, e56910 CrossRef CAS PubMed
.
- J. S. Jeon, S. Bersini, M. Gilardi, G. Dubini, J. L. Charest, M. Moretti and R. D. Kamm, Proc. Natl. Acad. Sci. U. S. A., 2015, 112, 214–219 CrossRef CAS PubMed
.
- X. Mei, K. Middleton, D. Shim, Q. Wan, L. Xu, Y. V. Ma, D. Devadas, N. Walji, L. Wang, E. W. K. Young and L. You, Integr. Biol., 2019, 11, 119–129 CrossRef PubMed
.
- M. Humayun, J. M. Ayuso, R. A. Brenneke, M. Virumbrales Muñoz, K. Lugo-Cintrón, S. Kerr, S. M. Ponik and D. J. Beebe, Biomaterials, 2021, 270, 120640 CrossRef CAS PubMed
.
- R. Wang, I. Godet, Y. Yang, S. Salman, H. Lu, Y. Lyu, Q. Zuo, Y. Want, Y. Zhu, C. Chen, J. He, D. M. Gilkes and G. L. Semenza, Proc. Natl. Acad. Sci. U. S. A., 2021, 118, e2024490118 Search PubMed
.
- J. Araos, J. P. Sleeman and B. K. Garvalov, Clin. Exp. Metastasis, 2018, 35, 563–599 CrossRef CAS PubMed
.
- S. M. Grist, S. S. Nasseri, L. Laplantine, J. C. Schmok, D. Yao, J. Hua, L. Chrostowski and K. C. Cheung, Sci. Rep., 2019, 9, 17782 CrossRef PubMed
.
- J. Song, A. Miermont, C. T. Lim and R. D. Kamm, Sci. Rep., 2018, 8, 17949 CrossRef CAS PubMed
.
- D. M Gilkes, P. Chaturvedi, S. Bajpai, C. C. Wong, H. Wei, S. Pitcairn, M. E. Hubbi, D. Wirtz and G. L. Semenza, Cancer Res., 2013, 73, 3285–3286 CrossRef PubMed
.
- J. C. M. Wan, C. Massie, J. Garcia-Corbacho, F. Mouliere, J. D. Brenton, C. Caldas, S. Pacey, R. Baird and N. Rosenfeld, Nat. Rev. Cancer, 2017, 17, 223–238 CrossRef CAS PubMed
.
- A. B. Silveira, F.-C. Bidard, M.-L. Tanguy, E. Girard, O. Trédan, C. Dubot, W. Jacot, A. Goncalves, M. Debled, C. Levy, J.-M. Ferrero, C. Jouannaud, M. Rios, M.-A. Mouret-Reynier, F. Dalenc, C. Hego, A. Rampanou, B. Albaud, S. Baulande, F. Berger, J. Lemonnier, S. Renault, I. Desmoulins, C. Proudhon and J.-Y. Pierga, Breast Cancer Res. Treat., 2021, 7, 115 Search PubMed
.
- C. Alix-Panabieres and K. Pantel, Clin. Chem., 2013, 59, 110–118 CrossRef CAS PubMed
.
- C. Alix-Panabieres and K. Pantel, Lab Chip, 2014, 14, 57–62 RSC
.
- E. Pedrol, M. Garcia-Algar, J. Massons, M. Nazarenus, L. Guerrini, J. Martinez, A. Rodenas, A. Fernandez-Carrascal, M. Aguilo, L. G. Estevez, I. Calvo, A. Olana-Daza, E. Garcia-Rico, F. Diaz and R. A. Alvarez-Puebla, Sci. Rep., 2017, 7, 3677 CrossRef PubMed
.
- S. Fang, H. Tian, X. Li, D. Jin, X. Li, J. Kong, C. Yang, X. Yang, Y. Lu, Y. Luo, B. Lin, W. Niu and T. Liu, PLoS One, 2017, 12, e0175050 CrossRef PubMed
.
- T. Y. Lee, K. Hyun, S. Kim and H. Jung, Sens. Actuators, B, 2017, 238, 1144–1150 CrossRef CAS
.
- J. Shi, P. W. Kantoff, R. Wooster and O. C. Farokhzad, Nat. Rev. Cancer, 2017, 17, 20–37 CrossRef CAS PubMed
.
- R. van der Meel, E. Sulheim, Y. Shi, F. Kiessling, W. J. M. Mulder and T. Lammers, Nat. Nanotechnol., 2019, 14, 1007–1017 CrossRef CAS PubMed
.
- F. Peng, M. I. Setyawati, J. K. Tee, X. Ding, J. Wang, M. E. Nga, H. K. Ho and D. T. Leong, Nat. Nanotechnol., 2019, 14, 279–286 CrossRef CAS PubMed
.
- Y. S. Zhang, Y.-N. Zhang and W. Zhang, Drug Discovery Today, 2017, 22, 1392–1399 CrossRef CAS PubMed
.
- Y. Yang, X. Yang, J. Zou, C. Jia, Y. Hu, H. Du and H. Wang, Lab Chip, 2015, 15, 735–744 RSC
.
- A. Albanese, A. K. Lam, E. A. Sykes, J. V. Rocheleau and W. C. Chan, Nat. Commun., 2013, 4, 2718 CrossRef PubMed
.
- H.-F. Wang, R. Ran, Y. Liu, Y. Hui, B. Zeng, D. Chen, D. A. Weitz and C. X. Zhao, ACS Nano, 2018, 12, 11600–11609 CrossRef CAS PubMed
.
- Y. Chen, D. Gao, Y. Wang, S. Lin and Y. Jiang, Anal. Chim. Acta, 2018, 1036, 97–106 CrossRef CAS PubMed
.
- N. Picollet-D'hahan, A. Zuhowaska, I. Lemeunier and S. L. Gac, Trends Biotechnol., 2021, 39, 788–810 CrossRef PubMed
.
- Z. A. Li and R. S. Tuan, Stem Cell Res. Ther., 2022, 31, 431 CrossRef PubMed
.
- K. Ronaldson-Bouchard, D. Teles, K. Yeager, D. N. Tavakol, Y. Zhao, A. Chramiec, S. Tagore, M. Summers, S. Stylianos, M. Tamargo, B. M. Lee, S. P. Halligan, E. H. Abaci, Z. Guo, J. Jackow, A. Pappalardo, J. Shih, R. K. Soni, S. Sonar, C. German, A. M. Christiano, A. Califano, K. K. Hirschi, C. S. Chen, A. Przekwas and G. Vunjak-Novakovic, Nat. Biomed. Eng., 2022, 6, 351–371 CrossRef CAS PubMed
.
- A. Herland, B. M. Maoz, D. Das, M. R. Somayaji, R. Prantil-Baun, R. Novak, M. Cronce, T. Huffstater, S. S. F. Jeanty, M. Ingram, A. Chalkiadaki, D. B. Chou, S. Marquez, A. Delahanty, S. Jalili-Firoozinezhad, Y. Milton, A. Sontheimer-Phelps, B. Swenor, O. Levy, K. K. Parker, A. Przekwas and D. E. Ingber, Nat. Biomed. Eng., 2020, 4, 421–436 CrossRef CAS PubMed
.
- Z. Li, Z. Lin, S. Liu, H. Yagi, X. Zhang, L. Yocum, M. Romero-Lopez, C. Rhee, M. J. Makarcyzk, I. Yu, E. N. Li, M. R. Fritch, Q. Gao, K. B. Goh, B. O'Donnell, T. Hao, P. G. Alexander, B. Mahadik, J. P. Fisher, S. B. Goodman, B. A. Bunnell, R. S. Tuan and H. Lin, Adv. Sci., 2022, 9, 2105909 CrossRef CAS PubMed
.
- J. Aleman and A. Skardal, Biotechnol. Bioeng., 2019, 116, 936–944 CrossRef CAS PubMed
.
- W. Liu, J. Song, X. Du, Y. Zhou, Y. Li, R. Li, L. Lyu, Y. He, J. Hao, J. Ben, W. Wang, H. Shi and Q. Wang, Acta Biomater., 2019, 91, 195–208 CrossRef CAS PubMed
.
|
This journal is © The Royal Society of Chemistry 2023 |