DOI:
10.1039/D3MO00089C
(Review Article)
Mol. Omics, 2023,
19, 607-623
Integrated multi-omics analyses of microbial communities: a review of the current state and future directions
Received
28th April 2023
, Accepted 3rd July 2023
First published on 3rd July 2023
Abstract
Integrated multi-omics analyses of microbiomes have become increasingly common in recent years as the emerging omics technologies provide an unprecedented opportunity to better understand the structural and functional properties of microbial communities. Consequently, there is a growing need for and interest in the concepts, approaches, considerations, and available tools for investigating diverse environmental and host-associated microbial communities in an integrative manner. In this review, we first provide a general overview of each omics analysis type, including a brief history, typical workflow, primary applications, strengths, and limitations. Then, we inform on both experimental design and bioinformatics analysis considerations in integrated multi-omics analyses, elaborate on the current approaches and commonly used tools, and highlight the current challenges. Finally, we discuss the expected key advances, emerging trends, potential implications on various fields from human health to biotechnology, and future directions.

Muzaffer Arıkan
| Muzaffer Arıkan holds a PhD degree in Molecular Biology and Genetics and works as an assistant professor in the Department of Medical Biology, School of Medicine at Istanbul Medipol University. His research focuses on understanding structure and function of microbial communities from various ecosystems through omics analyses. |
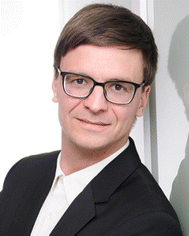
Thilo Muth
| Thilo Muth works as group leader of the eScience Section in the Department of Quality Infrastructure at the Federal Institute for Materials Research and Testing (BAM) in Berlin/Germany. One of his primary current research interests focuses on bioinformatic method development for multi-omics-based microbiome analysis. Besides providing data science-driven applications in materials science, his group develops advanced methods for analyzing and processing mass spectrometry-based data – especially with applications in metaproteomics and virus diagnostics. |
Introduction
Microbiomes, characteristic microbial communities inhabiting a specific environment with physio-chemical properties,1 are essential players of natural and managed ecosystems. Research efforts to understand microbiomes have been limited to culturing and microscopy for many decades, which have notable disadvantages, such as a lack of cultivation methods for most microorganisms and difficulties in evaluating community dynamics.2 To tackle these obstacles, molecular microbiology methods have been developed and applied to an ever-growing extent.
High-throughput omics technologies have greatly accelerated the advances in microbiome research.3 Although individual omics-based studies (e.g., amplicon sequencing, metagenomics, metatranscriptomics, metaproteomics, ‘meta-metabolomics’,4 hereafter referred to as metabolomics) provide valuable insights into the structural and functional characteristics, the combination of multiple omics data brings the advantage of revealing biological mechanisms and exploiting translational aspect of microbiomes.5–7 Specifically, integrating individual omics data enables a more comprehensive view of the flow of information -starting from DNA that is transcribed into RNA and finally translated into proteins that catalytically interconvert metabolites- in complex living systems.
In the last decade, there has been a massive accumulation in all omics data types due to the development of novel techniques, increasing availability of analysis platforms, and decreased experimental costs.8,9 At the same time, the increasing amount of microbiome data has unsurprisingly brought considerable challenges in replicability, robustness, reproducibility, and generalizability.10 Thus, there has been a considerable increase in the number of approaches and tools to overcome these challenges. Moreover, considering the differences and similarities of different omics data types, a variety of integrated analysis approaches and tools have been developed and applied in microbiome studies.
Recently, several reviews and perspectives on the multi-omics sciences have been published. For example, Zhang et al. (2023) focused their review on the application of multi-omics approaches, specifically in tumour microbiome research.11 Another review by Subramanian et al. (2020) provided a comprehensive overview of data repositories, tools/methods, and visualization platforms used in the analysis of multi-omics data, with a primary focus on bioinformatics aspects, and use cases in cancer diagnosis, prognosis, and treatment.12 Mallick et al. (2017) provided a thorough discussion on the experimental considerations for omics-based microbiome studies, listed bioinformatics analysis tools tailored explicitly for two mainstream omics types (metagenomics and metatranscriptomics), and briefly mentioned the challenges associated with integrated multi-omic analyses.13 In their perspective article, Nyholm et al. (2020) summarized the application of the holo-omics approach in biological research, specifically highlighting recent holo-omics use cases in host-microbiota interaction studies while prioritizing the exploration of applications across various fields over engaging in a debate about available tools and methods.14 In another review, Zhang et al. (2019) summarized and discussed the application of the meta-omics approaches in translational microbiome research by focusing on the recent multi-omics use cases in the microbiome field rather than debating the available tools and methods.9 On the other hand, Graw et al. (2021) focused on study design considerations and provided a summary of omics analysis types, while their discussion, although briefly mentioning integrative analysis tools, did not mainly elaborate on a microbiome-focused discussion.15
While the mentioned review articles addressed various aspects of the multi-omics analysis of microbiomes, they lack comprehensive coverage of certain crucial areas. In particular, there is a pressing need for a holistic overview that not only encompasses available mainstream omics analysis methods, their typical experimental and bioinformatic workflows, and main applications in the microbiome field but also addresses the integrated multi-omics analyses of microbial communities by delving into experimental considerations such as study design, sample collection, sample processing steps, and bioinformatics considerations. Furthermore, there is a distinct lack of microbiome-focused review articles thoroughly discussing the current integrated multi-omics analysis tools and the challenges associated with the integrative analysis of microbial communities. Lastly, there is a strong demand for an up-to-date, microbiome field-specific debate on the future perspectives of integrative multi-omics science to advance further investigations.
This review provides an overview of the most used omics analysis methods by presenting a brief historical context, a typical experimental and bioinformatic workflow, main applications, strengths, and limitations. Then, we focus on the integrated multi-omics analyses of microbial communities by covering both experimental considerations, such as study design, sample collection, and sample processing steps as well as bioinformatics considerations, such as handling different data types, assessing the computational requirements and selection of the integration approach and analysis tool. Next, we summarize the current challenges of the integrated multi-omics analyses, namely data heterogeneity, interpretability of the models, missing value imputation, compositionality, performance and scalability issues, and data availability and reproducibility. Finally, we address expected advances and potential solutions, the importance of international collaborations, and provide a foresight for the integrated multi-omics field.
We structure our review around a balance of both experimental and bioinformatics aspects, which would help the researchers comprehend the commonly used omics applications and gain insight into the opportunities and challenges posed by multi-omics methods particularly within the framework of the microbiome field.
Omics analysis types
Amplicon sequencing
The discussion about using molecular methods to study microbial diversity started more than 50 years ago and accelerated after rapid DNA sequencing methods were introduced.16 Subsequently, the small subunit ribosomal RNA gene was proposed as a marker gene that can be used to identify phylogenetic relationships among microorganisms.17 This approach has been accepted by the microbiology community and has become widespread over the years. However, especially in the second half of the 2000s, with the emergence of next-generation sequencing (NGS) technologies, microbial genomics approaches have gained increasing momentum18 and amplicon sequencing, widely used today, is carried out mainly using NGS platforms.19 Nowadays, amplicon sequencing has found broad use in microbiome studies in the last two decades, mainly centring on taxonomic profiling of microbial communities and investigation of changes in the composition (Fig. 1).
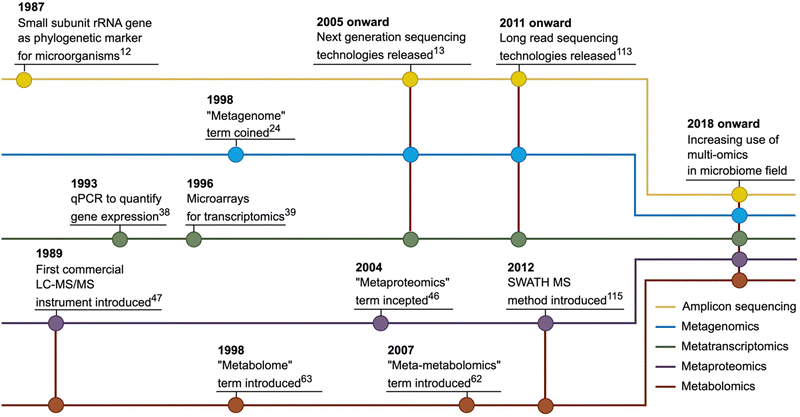 |
| Fig. 1 A brief historical timeline of the mainstream omics methods mentioned in this review. Line colours indicate omics analysis type: yellow for amplicon sequencing, blue for metagenomics, green for metatranscriptomics, purple for metaproteomics and red for metabolomics. Key developments in omics fields are highlighted by coloured circles. | |
As with all other omics-based microbiome studies, amplicon sequencing-based microbiome studies, should start with a clearly framed study design and description of the setting that would facilitate later steps. Sample collection which itself can already introduce biases,2 is the first step of amplicon sequencing-based analysis. Thus, essential factors such as sample characteristics, amount, location, and collection method should be evaluated before the sampling. The second step is the storage of the samples (immediate freezing after collection or using alternative preservative methods if freezers are unavailable20) as it is generally not possible to process the samples directly at the sampling site. Storage conditions may also affect the microbiome profiles.21,22 Next, DNA is extracted from the collected samples. DNA extraction can be performed using a variety of commercial kits or in-house protocols depending on the sample types, which are also known to affect the quality, quantity and profile of the extracted DNA, thus, downstream processes.23 The procedures continue with the library preparation for sequencing, which typically involves a two-step PCR approach (Fig. 2).24 First, specific gene regions of marker genes selected based on the target microbial group (e.g., 16S rRNA gene for bacteria and archaea, ITS regions for fungi) are amplified using universal primers. Then, amplified fragments from each sample are indexed with unique barcode combinations and added sequencing adapters for sequencing. Pooling and normalizations are the final steps before sequencing. It should be noted that samples should be sequenced along with extraction negative and no-template PCR controls to avoid spurious findings due to contamination. After NGS, sequence information from target marker gene regions is used for investigating the microbial community. Bioinformatic analyses of amplicon sequencing studies usually include diversity estimation and comparisons, differential abundance analysis, calculations of correlations between environmental parameters and community changes, network constructions and classification analyses. Details of each of these steps have been reviewed elsewhere.2,19
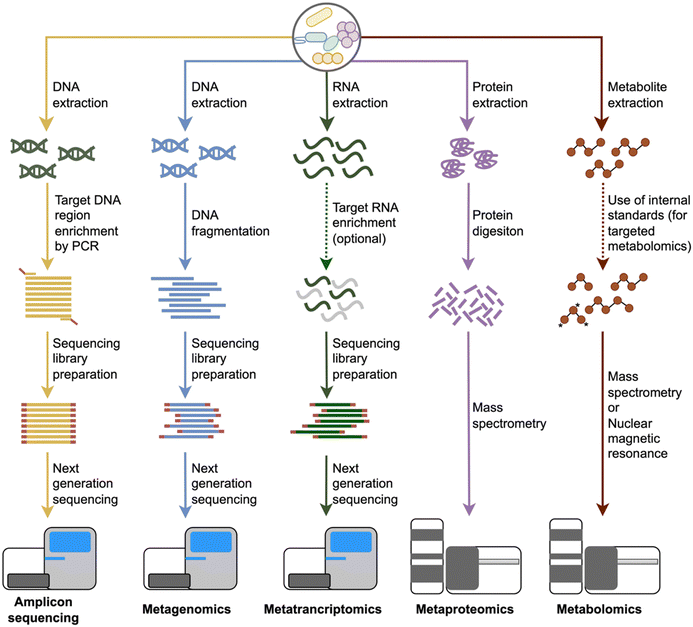 |
| Fig. 2 Experimental workflows for mainstream omics analysis types. Arrow colours indicate omics analysis type: yellow for amplicon sequencing, blue for metagenomics, green for metatranscriptomics, purple for metaproteomics and red for metabolomics. Amplicon sequencing involves a series of steps including DNA extraction, target DNA region enrichment, library preparation, and sequencing in an NGS instrument. On the other hand, metagenomics starts with DNA extraction and proceeds with random DNA fragmentation, library preparation, and sequencing in an NGS instrument. Metatranscriptomics, which focuses on RNA analysis, includes RNA extraction, optional target RNA enrichment, library preparation, and sequencing in an NGS instrument. Metaproteomics starts with protein extraction, followed by purification and digestion steps, and then analysis of the resulting peptides using mass spectrometry. Lastly, metabolomics involves the extraction of small metabolites, preparation for targeted or untargeted metabolomics analysis, and analysis using mass spectrometry or nuclear magnetic resonance instruments. | |
Although it undoubtedly alleviated many culturing problems, amplicon sequencing has its specific advantages and disadvantages. Wet laboratory advantages include applicability to low-biomass or heavily host DNA-contaminated samples, while PCR and primers biases can be listed as disadvantages.19 For the bioinformatics part, amplicon sequencing (particularly 16S rRNA gene amplicon sequencing) is a well-established method with comprehensive well-curated reference databases25 and an exhaustive list of analysis tools.26 On the other hand, as targeting only specific regions of marker genes does not provide information about the functional properties of the community, 16S rRNA gene amplicon sequencing studies are limited to taxonomic investigations -resolved down to the genus level- although some bioinformatics efforts on functional potential predictions were introduced in the last years.27,28 Lastly, the cost-effectiveness and scalability of this method still make it a preferred approach by many research groups, especially with limited budgets.
Metagenomics
The term metagenome was coined by Handelsman et al. (1998) to describe the collective genome of the microbial community in an environmental sample,29 while “metagenomics” refers to the analysis of this collective genetic material. Although the first studies in the field of sequencing-based metagenomic analysis (shotgun metagenomics) were based on the Sanger sequencing method, NGS technologies paved the way for significant advances. Two important studies published in 2004 presented the potential of shotgun metagenomics as a valuable method to better understand the structure and functional dynamics of microbial communities through analysis of whole genome information.30,31 The release of new sequencing platforms at the end of the 2000s has accelerated the progress of metagenomics applications.32 Recently, in addition to the decreasing sequencing costs, the introduction of new data analysis approaches such as metagenomic classification and profiling, microbial genome reconstructions from metagenomes and integration of metagenomic and metabolomic data to predict functional profile contributed to broadening use of this method.33 At present, shotgun metagenomics has an increasing use in the microbiome field for microbial community profiling, identification of microbial biomarkers, assessment of the functional potential of microbial community members and microbiome-based classifications of sample groups.
Shotgun metagenomics contains the same experiments at the study design, sampling, and DNA extraction steps with amplicon sequencing and thus shares the same pitfalls described above for these steps. However, the flow of procedures for shotgun metagenomics differentiates from amplicon sequencing after the DNA extraction step (Fig. 2). Instead of a PCR-based amplification of a specific genome region, shotgun metagenomics includes random fragmentation of DNA samples because most NGS systems have input fragment size limitations, followed by the addition of unique barcode and adapter sequences and sequencing.34 Then, bioinformatic tools are used to investigate microbiome composition, reconstruct the microbial genomes, determine functional potential, perform diversity analyses, and calculate the compositional and functional level associations between environmental parameters and microbiome alterations.35 Details of each of these steps have been reviewed elsewhere.19,36,37
Compared to amplicon sequencing, shotgun metagenomics is less subject to amplification biases,38 provides information on the metabolic potential and functional capabilities of microbial communities,39 and allows whole genome recovery and examinations of microbiome members.40 However, the main disadvantages of this method are computational requirements, higher susceptibility to contamination in low biomass samples, effects of host DNA contamination, data complexity, and analysis issues.33
Metatranscriptomics
Omics analysis types focusing on DNA as a target biomolecule, such as amplicon sequencing and metagenomics, have contributed significantly to a better understanding of the microbiome's role in various of ecological scenarios. However, the remaining fundamental limitation of these genomic methods is that the obtained results do not indicate the viability of cells or expression profiles of the detected genes.41,42 Metatranscriptomics has been defined as the characterization of transcriptional profiles in microbiome samples, and it thus affords insight into the activity of microbial communities. Although first applied using qPCR43 or hybridization-based approaches such as microarrays,44 shotgun metatranscriptomics via RNA-Seq has been established as the mainstream approach after developing NGS technologies due to the high diversity of microbiomes and lack of reference isolates.45 Metatranscriptomics is currently used for assessing microbial activity, determining microbiome-environment interactions through gene regulation dynamics, the association of gene expression profiles to phenotypes and phylogenomic analysis of microbiomes.
Metatranscriptomics experiments involve the extraction of RNA from microbiomes after the study design and sample collection steps described above. Since RNA is prone to degradation,46 extraction and storage conditions should be designed more cautiously. If feasible, sample storage in stabilizer solutions may be preferred to alleviate the degradation problem.47 Also, RNA-degrading enzyme contamination (e.g., by ribonucleases) should be avoided throughout the experiments.48 The second step of metatranscriptomics experiments generally comprises mRNA enrichment using polyA+ selection and rRNA depletion for eukaryotes and prokaryotes, respectively.49 Then, mRNA fragments are randomly fragmented, cDNA is synthesized, unique barcode and adapter sequences are ligated, and the prepared library is sequenced (Fig. 2). Bioinformatics analysis of metatranscriptomics data starts with the quality control and filtering steps as in amplicon sequencing and metagenomics. In the second step, clean reads can be aligned to reference sequences to determine transcript abundances. Alternatively, a de novo assembly approach can be applied by first assembling reads into contigs and then calculating the transcript abundances. Taxonomic and functional profiling based on transcript abundances can be performed to understand microbiome composition and activity. Statistical analyses can be employed to detect changes between different conditions. Details of experimental and bioinformatics analysis steps of metatranscriptomics have been reviewed elsewhere.45,49
The persisting challenges in metatranscriptomics studies include the difficulties in unbiased, high-quality RNA sample preparation,50 high host RNA contamination in some samples,49 lack of the optimization of data analysis steps,45 biological interpretation issues related to uncharacterized transcripts and integration with additional omics data such as metaproteomics.
Metaproteomics
Metaproteomics is the study of the entire protein content of microbiome samples51 and thus provides the opportunity to investigate the functionality of microbiomes. Although first commercial LC-MS/MS instrument was introduced in 198952 and there have been extensive studies in the early 2000s,53,54 metaproteomics has become widespread in the microbiome field since 2012, partly thanks to the advantages provided by significant technological advances that allowed more feasible and affordable metaproteomics studies.55 Moreover, the introduction of new metaproteomics data analysis tools56–58 and optimized workflows59,60 have accelerated metaproteomics-based microbiome investigations. Presently, metaproteomics is mainly used to determine microbial community structures based on protein biomass, the interactions between community members, specific substrate uses by community members, and expressed metabolism and physiology of the community.55
Shotgun metaproteomics, likely the most widely used technique to identify and quantify proteins in microbiome samples, typically includes the following main steps: Proteins are extracted from samples and purified. Purified proteins are digested into peptides using trypsin and peptides are separated by chromatography. Mass spectrometric analysis is applied to measure both intact peptide masses (MS1) and masses of peptide fragments (MS/MS) (Fig. 2). The MS/MS spectra are then compared against theoretical spectra calculated from an in silico digested protein database. Considerations for constructing a protein sequence database in metaproteomics have been reviewed in detail recently.61 The identified peptides are used to infer proteins in the samples. Taxonomic analysis, functional profiling and differential abundance analysis can be carried out at the peptide or protein level. Sample preparation methods and methodological considerations in metaproteomics studies are reviewed elsewhere.62,63
The main challenges related to metaproteomics applications are standardization, repeatability and reproducibility of sample preparation protocols,60,64 insufficient coverage and accuracy for detecting proteins from low abundant species in complex samples,63 unavailability of optimized protein sequence databases due to the lack of optimized database construction protocols and genomic sequences for many microbial community members,61 high computational demand,56 and data interpretation issues.65
Metabolomics
Focusing on small molecules with molecular weights less than 2000 Da (commonly substrates and products of enzymes),66 microbiome metabolomics provides a snapshot of the physiological state of a microbial ecosystem. Although it has been used for over two decades, the high-throughput analysis of total metabolite pool (metabolome67) derived from microbial samples has gained significant interest in recent years as it bridges microbial and genetic composition with phenotypes through metabolites.68 Metabolomics has found wide applications in different fields and has become a valuable tool in the microbiome field with the increasing number of translational microbiome studies. At present, the applications of metabolomics to the study of microbiomes include determining functions of unknown genes, characterizing environmental microbial communities and their functions by the association between microbes and metabolites, molecular epidemiology studies, the discovery of novel enzymes, and the identification of potential metabolite biomarkers.68
A typical metabolomics experiment includes the following main steps: Metabolites are extracted from microbiome samples using an extraction method of choice. Then, targeted or untargeted metabolomics analysis may be conducted depending on the study design and goals. Metabolites are routinely detected and quantified by either nuclear magnetic resonance (NMR) spectroscopy or mass spectrometry (MS) coupled with liquid chromatography (LC), gas chromatography (GC), or capillary electrophoresis (CE). NMR holds the advantage of being a direct quantification technique; however, it has a relatively low sensitivity and low throughput. In contrast, MS is a much more sensitive technique but suffers from technical challenges in quantification and its destructive nature.69 Specifically, a typical NMR-based metabolomic study usually provides information on 50–200 identified metabolites with concentrations >1 μM, while a typical LC-MS-based metabolomic study can return information on more than 1000 identified metabolites with concentrations of >10 to 100 nM.70 Considering the small overlap in their spectrums of detectable compounds, these two methods represent highly complementary metabolomics approaches.71 The bioinformatic analysis of metabolomics data starts with standard pre-processing steps, including quality control, noise reduction, feature identification and quantification. Finally, metabolites in the microbiome are chemically annotated, associated with other microbial features (e.g., abundances, genes, proteins), and linked to changes in microbial ecosystems.72 Tools, experimental approaches, and computational methods used in microbiome metabolomics are reviewed elsewhere.72,73
Emerging as a promising technology complementing sequencing-based approaches, metabolomics poses some limitations, such as reaching a limited number of metabolites due to sample preparation protocols despite their vast diversity,74 the predominance of unknown metabolites in untargeted metabolomics analysis,75 lack of comprehensive reference databases and bioinformatics tools,76 difficulties in the integration of metabolomics data with other omics data types.77
A summary of characteristics of omics analysis types is provided in Table 1.
Table 1 Characteristics of omics analysis types
Omics Analysis |
Target biomolecule |
Advantages |
Disadvantages |
Main pplications |
Amplicon Sequencing |
DNA |
• Applicable to low-biomass or heavily host DNA contaminated samples |
• PCR and primers biases |
• Taxonomic profiling of microbiomes |
• Well-established method with comprehensive and curated reference databases and a wide list of analysis tools |
• No information on functional properties |
• Investigation of changes in the microbiome composition |
• Cost-effective and scalable |
Metagenomics |
DNA |
• Less subject to amplification biases |
• High computational requirements |
• Taxonomic microbial community profiling |
• Information on the metabolic potential and functional capabilities |
• Susceptibility to contamination in low biomass samples |
• Identification of microbial biomarkers |
• Whole genome recovery |
• Affected of host DNA contamination |
• Assessment of functional potential of microbial community members |
• Data complexity and analyses issues |
• Microbiome-based classifications |
• No indication of the viability of cells or expression profiles of the detected genes |
Metatranscriptomics |
RNA |
• Allows analysis of expression profiles for the detected genes |
• Difficulties in unbiased, high quality RNA sample preparation |
• Determination of microbial activity |
• Determination of functional state of the microbial community |
• Affected by host RNA contamination |
• Analysis of microbiome-environment interactions through gene regulation dynamics |
• Detection of rapid microbial community responses |
• Lack of optimization for data analysis steps |
• Association of gene expression profiles to phenotypes |
• Biological interpretation issues related to uncharacterized transcripts |
• Phylogenomic analysis of microbiomes |
• Integration difficulties with other omics data |
Metaproteomics |
Protein |
• Provides the opportunity to investigate both taxonomic composition and the functionality of microbiomes |
• Standardization, repeatability, and reproducibility of sample preparation protocols |
• Determining microbial community structures based on protein biomass |
• Analysis of interactions between community members |
• Insufficient coverages and accuracy for detecting proteins from low abundant species in complex samples |
• Analysis of interactions between community members |
• Unavailability of optimized protein sequence databases due to lack of optimized database construction protocols and genomic sequences for many microbial community members |
• Investigating specific substrate uses by community members |
• High computational demand |
• Overview of expressed metabolism and physiology of the microbial community |
• Data interpretation issues |
• Identification of potential protein biomarkers |
Metabolomics |
Small Metabolites |
• Provides a snapshot of the physiological state of a microbial ecosystem |
• Limited number of detected metabolites |
• Determining functions of unknown genes |
• Lack of comprehensive reference databases and bioinformatics tools |
• Characterization of microbial communities and their functions by association between microbes and their metabolites |
• Difficulties in the integration of metabolomics data with other omics data types |
• Molecular epidemiology analysis |
• Identification of potential metabolite biomarkers |
Integrated multi-omics analyses of microbial communities
Experimental considerations
First and foremost, well-thought study design and appropriate methodology are crucial for the success of an integrated multi-omics study. Therefore, the study's scope and limitations should be clearly defined before any experimental work is conducted. If possible, it is good practice to work with a statistician and experts of each omics and integrated multi-omics as this would help avoiding common pitfalls considerably. The decision of which omics types to be employed in a multi-omics study should be made on a balance of maximization of information gain by each omics type, feasibility, and additional financial costs due to the extension of the multi-omics design.78,79 Another important consideration is the calculation and recruitment of an adequate number of samples for a multi-omics study. Unfortunately, there are only a few tools evaluating sample size and conducting power analysis for multi-omics studies. Tarazona et al. (2020) proposed the MultiPower method for power analysis and sample size estimations for multi-omics studies.80 Syed et al. (2021) described MOPower for multi-omics data simulation and power calculation.81 Hence, there is a strong need for new tools that can be employed for complex scenarios such as multiple study groups and considering the characteristics of microbiome multi-omics datasets. Here, it should also be noted that the same number of samples does not provide the same power for each omics analysis type, and targeting the same power for each omics analysis type results in a different number of samples.82 As these scenarios have different impacts on downstream steps such as the suitability of multi-omics integration tools, the advantages and disadvantages should be carefully examined. Also, as we summarized in previous sections, each omics analysis type has its limitations. Therefore, when it is impossible to avoid some technical limitations, a careful and detailed recording of the sample data and experimental procedures helps adjusting for the confounding effects during data analysis steps and improves re-usability of the data.
Sample type and amount are also among crucial parameters, as sample-specific structural features or availability may limit the application of all omics analysis types.83 Moreover, optimal sample handling and storage conditions differ between omics analysis types, as each type of target biomolecules has a different vulnerability profile. For example, it is known that RNA is more prone to degradation than DNA. Neglecting these profile differences during experimental procedures may introduce degradation-related sample composition changes and consequently cause the disappearance of true signals or lead to false associations. As performing sample preparations for each omics analysis separately may decrease the comparability of the results due to extraction method differences, simultaneous extraction protocols84,85 can be employed to reduce the potential biases. Also, universal references, biological and technical replicates, mock samples, and negative controls are important components of a successful multi-omics study with reproducible and robust results. Finally, performing a pilot study can provide a preliminary insight into the characteristics of the results expected to be obtained from integrated multi-omics analysis despite a potentially high variability due to the small sizes of these studies.
Bioinformatics considerations
Bioinformatics considerations should start from the preliminary phases of the study design, as the selected omics analysis combination and data characteristics of each employed omics type determine consequent bioinformatics analysis design. Firstly, the required/expected quality and quantity of data should be estimated for each omics type individually by considering the specific factors influencing the outcome of each analysis. For example, sequencing depth has a clear impact on the microbial community resolution obtained by metagenomics and metatranscriptomics86,87 and data quality profiles are known to vary according to the sequencing platforms. Here, if present, previously published datasets on the same sample type can also be instructive for determining data requirements for a successful study.
Computational requirements for storing and analysing each omics data are different and require certain preparations in advance. For example, the analysis of metagenomics data requires high computational power and processing time. Thus, access to high performance computing (HPC) clusters or cloud-based environments would facilitates the processing of metagenomics data.88 There is a continuous introduction of new technologies and data types expected to be added to the current omics data types which indicates the growing importance of HPC and cloud-based services.82
Selecting the most suitable integration, comparison and statistical analysis approaches is another key element for answering the research questions of a multi-omics study. Various integration approaches differ in both the way of processing the omics data combinations, generated outputs, and their interpretation of results.12,77,89 Previously published integrated multi-omics studies and proposed bioinformatics analysis protocols can provide immense help for familiarizing with the necessary analysis steps.
Moreover, employing the most appropriate multi-omics integration tool depends on the omics data types combined in the study. Although the number of multi-omics integration tools increases, only some of them are microbiome data-specific/supporting tools. However, only such specific tools allow appropriate handling of microbiome multi-omics data without neglecting its specific limitations.
Finally, the data availability and reproducibility of bioinformatics analysis protocols are of paramount importance for reducing the waste of time and effort, particularly in research. It is therefore important to follow international guidelines and principles to make data findable, accessible, interoperable and reusable.90,91 Accordingly, the design of bioinformatics analysis protocols should also include precise planning of data sharing and documentation.
Integrated multi-omics analysis tools for microbiome studies
This section presents an overview of the available tools for the integrated multi-omics analysis of microbiomes. However, our intention is not to offer an exhaustive list of the existing tools; instead, we aim to provide a starting point and initial guidance for the researchers interested in including integrated multi-omics analyses in their future microbiome research. Accordingly, for each tool listed below, we briefly describe its general features, discuss advantages and limitations, and give reproducible example studies from the microbiome field, which made both datasets and code are publicly available.
MOFA.
Multi-omics factor analysis (MOFA) performs a factor analysis to identify latent factors formed by co-varying features of different omics data modalities in an unsupervised manner and reveals the factors that explain the greatest variance in datasets.92 It supports both numerical (count and continuous) and binary data as input, handles missing values, and can be applied to integrate amplicon sequencing, metagenomics, metatranscriptomics, metaproteomics and metabolomics datasets. MOFA can integrate partially overlapping omics datasets which is a significant advantage considering the different number of samples for each omics analysis. However, as it depends on linear models, MOFA suffers from its poor ability to detect nonlinear correlations between and within omics datasets. In addition, data normalization and dimensionality reduction to reduce the differences between data modalities are critical for the model to work properly. Haak et al. (2021) employed MOFA to integrate the bacterial, fungal, and viral microbiota sequencing data from the faecal samples of critically ill patients with and without sepsis and healthy volunteers and highlighted transkingdom changes associated with the disease along with the contributions from each component.93 Recently, Mikaeloff et al. (2023) used MOFA to integrate amplicon sequencing, metabolomics, and lipidomics datasets and revealed the association of the intercorrelated microbiome-associated metabolites with the clinical parameters in people living with HIV.94
mixOmics.
mixOmics is a toolkit containing supervised and unsupervised multivariate analysis methodologies for the exploration, integration and visualization of multi-omics datasets.95 Particularly, DIABLO, a specific framework implemented in mixOmics, performs supervised integration of multi-omics assays to identify a subset of features discriminating phenotypes across datasets.96 DIABLO accepts multiple omics data types and has been successfully applied in several multi-omics studies. The main limitations of this tool are that it accepts only continuous data, requires omics datasets with completely overlapping samples and assumes a linear relationship between the features, which limits its performance in nonlinear associations. Liu et al. (2020) integrated transcriptomics, amplicon sequencing, and metabolomics data using DIABLO which showed the links between the changes in specific omics features in gut microbiota and the effects of intermittent fasting on diabetes-induced cognitive impairment.97 In another study, DIABLO was employed to perform an integrative analysis of the mutational profile, the metabolome, and the microbiota (amplicon sequencing) data to identify discriminatory latent variables between different dietary regimens.98
IMP.
The integrated meta-omic pipeline (IMP) provides a microbiome analysis workflow that allows the integrated analysis of metagenomics and metatranscriptomics data.99 Instead of accepting the matrix generated from each omics dataset individually as inputs, IMP includes both pre-processing of raw metagenomics and metatranscriptomics datasets and integrative analysis. The integrated approach of IMP is mainly based on the calculation of average metatranscriptomics to metagenomics depth of coverage ratios, relying on sequence identity. Hence, it provides a user-friendly, standardized workflow for investigating links between the composition and expression profiles of microbiomes. However, allowing integrated analysis of only these two omics types currently limits an even broader use of this pipeline. Herold et al. (2020) performed metagenomics, metatranscriptomics, metaproteomics, and metabolomics in a longitudinal study to examine the response of microbial communities in a biological wastewater treatment plant to disturbance.100 IMP was used in a combined analysis of metagenomics and metatranscriptomics datasets in this study while other methods were employed for the integrative analysis. More recently, de Nies et al. (2023) applied metagenomics and metatranscriptomics to the stool samples collected from COVID-19 patients and healthy controls and then conducted an integrative analysis with IMP to reveal the effect of the infection on the gut microbiome community and functions.101
gNOMO.
gNOMO is a bioinformatics pipeline specifically designed to process and analyse metagenomics, metatranscriptomics and metaproteomics data in an integrative manner.102 This pipeline accepts raw sequencing and spectra data as inputs and executes all analysis steps from pre-processing to integrative analysis and visualization. gNOMO integrates metagenomics and metatranscriptomics datasets based on assignments to the same functional categories, uses metagenomics and metatranscriptomics data to create a custom database for metaproteomics analysis and finally applies a pathway integration to track microbiome alterations in different levels. gNOMO pipeline also processes host organism data along with microbiome data which provides an essential advantage in host-microbiome investigations. The main limitations of this pipeline are that it does not support amplicon sequencing and metabolomics data, which would enable analysis of more multi-omics combinations. gNOMO was employed in an integrative multi-omics analysis of hindgut samples of Blattella germanica, a non-model the German cockroach species by using metagenomics, metatranscriptomics and metaproteomics datasets.102 Moreover, human gut microbiota samples were processed with this pipeline by combining metagenomics and metaproteomics data.102
mmvec.
Microbe–metabolite vectors (mmvec) uses a machine learning neural network to estimate the conditional probabilities of metabolites in the presence of a specific microorganism.103 Metabolite and microbe (amplicon sequencing or metagenomics) abundance tables are required as input tables for this tool. The main limitations of mmvec include the lack of a calculation method for statistical significance and confidence intervals for the strength of microbe–metabolite interactions, handling only count data and not allowing for adjustment of covariates. Allaband et al. (2021) used mmvec to combine amplicon sequencing and metabolomics data and predicted microbe–metabolite interactions contributing to the increased risk for heart disease seen in patients with obstructive sleep apnea.104
mCIA.
Multiple co-inertia analysis (mCIA) is an unsupervised analysis method to identify the relationships in multiple omics datasets.105 mCIA depends on the transformation of different omics datasets into comparable lower dimensional spaces through an ordination method and extraction of most variable omics features sharing similar trends across the datasets.106 A sparsity employing mCIA method (smCIA) has been recently introduced to improve the feature selection and interpretability of the mCIA models.105 mCIA method was used by Heintz-Buschart et al. (2016) for the integrative analysis of three omics datasets (metagenomics, metatranscriptomics and metaproteomics) to explore their relationships in the context of a case study of familial type 1 diabetes.107
MiBiOmics.
MiBiOmics offers both a web-based and standalone tool for the simultaneous analysis of up to three omics datasets.108 It includes correlation analysis using weighted gene correlation network analysis (WGCNA), dimensionality reduction for each omics dataset using mCIA and Procrustes, detection of significant associations between omics layers through a network-based approach, and extraction of the features associated with phenotypes. MiBiOmics is a user-friendly tool and allows for data transformation/normalizations to account for the compositionality aware. However, this tool has certain limitations, such as its use of univariate analysis which may lead to erroneous associations when dealing with highly collinear features, and the requirement for a complete overlap between different omics datasets.
COMBI.
Compositional omics model-based integration (COMBI) combines latent variable modelling and log-ratio link functions with mean-variance modelling to generate a new model for the integration of multi-omics datasets.109 COMBI accepts omics (amplicon sequencing, metagenomics, metatranscriptomics, metaproteomics and metabolomics) feature abundance tables as inputs and generates a model model-based joint visualization to highlight sample clusters and feature relationships. The advantages of this methods include accounting for the compositionality in omics data and handling covariates. Yet, the interpretating the relationships between features from different compositional datasets can be quite challenging in this method.
PALM.
The pipeline for the analysis of longitudinal multi-omics data (PALM) applies temporal normalization using continuous curve alignment and uses dynamic Bayesian networks (DBNs) to reconstruct a unified model. Then, the interactions between the features from different omics datasets are predicted to infer microbiome changes in the microbiomes over time.110 PALM provides information on host-microbiome interactions via the integration of metagenomics, metatranscriptomics, metabolomics, and host transcriptomics. PALM is designed to process longitudinal microbiome multi-omics data and thus answers a particular need in microbiome field. However, the lack of comprehensive databases of interactions between the features of different omics layers and detailed documentation limit the broad use of this tool.
The main steps of the bioinformatics workflow for each omics type, along with the characteristics of the integrated multi-omics tools discussed earlier, are summarized in Fig. 3.
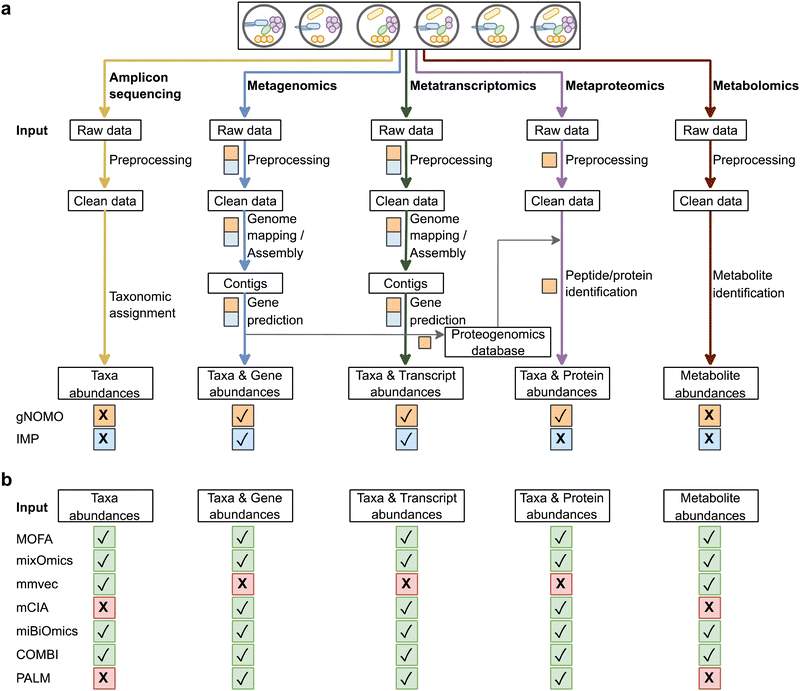 |
| Fig. 3 Main bioinformatics workflow steps for mainstream omics analysis types and integrated multi-omics analysis tools. (a) The main steps of the bioinformatics workflow are displayed, accompanied by two integrated multi-omics analysis tools that accept raw omics data as input. Arrow colours indicate omics analysis type: yellow for amplicon sequencing, blue for metagenomics, green for metatranscriptomics, purple for metaproteomics and red for metabolomics. The tool that can perform each step is indicated by coloured squares next to arrows: gNOMO is represented by yellow, while IMP is represented by blue. (b) Integrated multi-omics analysis tools that accept feature abundance tables as input. For each tool, the accepted and non-accepted omics data types are indicated by coloured squares. Green squares indicate that the tool accepts the corresponding data type, while red squares indicate that it does not. | |
To cover key aspects, strengths, and limitations of the different approaches thoroughly, we further discuss the step-by-step process of multi-omics data analysis through an imaginary dataset containing samples collected at different stages of the fermentation process of artisanal cheese production, thus specifically focusing on the role of microbial communities involved in the fermentation process. As previously mentioned, firstly, the choice of omics combination should align with the study's specific goals. For example, to comprehensively understand the role of certain members of a microbial community and their products, researchers can employ a combination of metagenomics, metatranscriptomics, and metaproteomics. This integrated approach enables the analysis of taxonomic abundance, gene expression, and protein profiles, thereby providing a holistic view of the alterations in the microbial community throughout the fermentation process. Fig. 3 illustrates a typical workflow for this type of study, depicting the sequential steps involved. The raw omics data acquired from these analyses can be directly inputted into either the gNOMO or IMP pipelines, depending on the specific types of omics data being processed. The gNOMO pipeline can handle metagenomics, metatranscriptomics, and metaproteomics data, while the IMP pipeline specifically accepts metagenomics and metatranscriptomics data. Both pipelines effectively process the raw omics data and perform pre-processing, normalization, integration, and visualization steps. They generate comparisons of abundance for matched features across different omics layers, thereby facilitating the identification of taxonomic and functional changes within the microbial community. By employing visualization methods such as KronaPlot or Pathview, researchers can also explore abundance changes in various taxa, transcripts, and proteins between different phenotypes. This would not only reveal potential compositional changes in the microbial community but also shed light on changes in gene expression and protein profiles, providing valuable information about the fermentation process.
Moreover, the list of integrated multi-omics analysis tools that accept feature abundance tables as input is provided in Fig. 3. Each tool is designed to support different combinations of omics data, providing researchers with a range of options based on their specific requirements. By performing initial data analysis steps (i.e., pre-processing and generation of feature abundance table) separately for each omics type, researchers can leverage the capabilities of various tools to gain deeper insights into their data. For identifying the most variable feature groups across omics layers and exploring their potential relationship with certain phenotypes, tools like MOFA, mixOmics, MiBiOmics, mmvec and mCIA can be employed. These tools offer advanced analytical techniques that enable researchers to uncover patterns and associations within multi-omics datasets. For instance, if the researchers are interested in studying metabolites influencing flavour development and their relationship with specific microbial community members, they can apply amplicon sequencing and metabolomics, perform initial data analysis steps separately for each data type and obtain feature abundance tables and then use mmvec to identify the top co-occurring microbes and metabolites, providing valuable information about microbe–metabolite interactions. In longitudinal designs, PALM emerges as a valuable tool facilitating the study of temporal changes and providing researchers with a comprehensive understanding of the alterations over time. Furthermore, COMBI is a tool that allows for the investigation of different omics features through joint visualization. By integrating data from multiple omics types, COMBI facilitates the determination of associations between different omics features and phenotypes. Overall, by allowing the analysis of multi-omics data, these tools could help improve cheese quality, understand the factors influencing flavour development, and guide the selection of starter cultures or fermentation conditions.
Table 2 provides a summary of the strengths and limitations of each tool, accompanied by links to tutorials and code resources for further insights into the data processing and output visualization techniques.
Table 2 Characteristics of integrated multi-omics analysis tools described in this review
Tool |
Strengths |
Limitations |
Link |
Ref. |
MOFA |
• Ability to integrate partially overlapping omics datasets |
• Poor ability of detecting nonlinear correlations |
https://github.com/bioFAM/MOFA
|
92
|
mixOmics |
• Customizable, well-implemented tool with extensive documentation |
• Poor ability of detecting nonlinear correlations |
https://mixomics.org
|
95
|
• Low computational demand |
• Accepts only continuous data |
• Requires complete overlap between omics datasets |
IMP |
• Standardized workflow including all data analysis steps |
• Does not support amplicon sequencing, metaproteomics and metabolomics data |
https://r3lab.uni.lu/web/imp
|
99
|
• High computational demand |
gNOMO |
• Standardized workflow including all data analysis steps |
• Does not support amplicon sequencing and metabolomics data |
https://gitlab.com/gaspilleura/gnomo
|
102
|
• Allows simultaneous analysis host data |
• High computational demand |
mmvec |
• Accounts for the compositionality |
• Does not calculate statistical significance and confidence intervals |
https://github.com/biocore/mmvec
|
103
|
• Handles only count data |
• Does not adjust for covariates |
mCIA |
• Does not require common set of features for all omics datasets |
• Poor ability of handling sparse data |
https://bioconductor.org/packages/release/bioc/html/omicade4.html
|
105
|
• Does not support amplicon sequencing and metabolomics data |
MiBiOmics |
• Easy to use (web-based and standalone versions available) |
• Calculates univariate correlation |
https://gitlab.univ-nantes.fr/combi-ls2n/mibiomics
|
108
|
• Compositionality-aware |
• Requires complete overlap between omics datasets |
COMBI |
• Accounts for the compositionality |
• Difficulty of interpretation of the relationships between features from different datasets |
https://www.bioconductor.org/packages/release/bioc/html/combi.html
|
109
|
PALM |
• Works with longitudinal multi-omics datasets |
• Incomplete databases of interactions between omics layers |
https://github.com/DaniRuizPerez/PALM-Public-Respository
|
110
|
• No detailed documentation |
Challenges of the integrated multi-omics analyses
Multi-omics analyses have become increasingly common in the microbiome field, yet they present some important challenges that need to be addressed in the future. The main challenges include heterogeneity of multi-omics data, compositionality, missing value problem, difficulties in biological interpretations, performance and scalability of the analysis tools, data availability, and reproducibility issues.
Multi-omics data is generated using different analysis platforms which bring resolution differences and technique-inherent biases as sources of data heterogeneity. The heterogeneity of multi-omics datasets causes different statistical power for each omics analysis type, eventually affecting the integration and interpretation efforts.80 Therefore, power and sample number estimation is not an easy task for multi-omics studies. There are two main approaches to alleviate the effects of data heterogeneity in omics analyses. The first approach ensures the same number of samples across all analyses, which inevitably results in different statistical power and false negative results. The second approach maintains consistent statistical power for each omics type despite varying sample sizes, which imposes the necessity of using specific integrative analysis tools (such as MOFA) that can handle partially overlapping datasets. Other approaches to handle heterogeneity across multi-omics datasets include employing pre-processing steps, including sample eliminations before integration or applying power enhancement approaches for the same features across datasets during integrative analysis.111 However, these approaches only partially solve the heterogeneity-related issues and bring new complications.
Another critical challenge in multi-omics analyses arises from the compositionality of the sequencing and mass spectrometry data, which means these measurements do not provide absolute abundances; instead, the relative abundances of target biomolecules, which are not independent, are presented with an arbitrary sum constraint.103,112,113 Hence, this compositional nature of multi-omics microbiome data can confound downstream statistical analyses and cause spurious inferences of interaction.114 Although the number of the tools considering compositionality has been increasing, particularly in genomics, there is a strong need for compositional data analysis tools for facilitating integrated multi-omics analysis.
Omics technology applications in the microbiome field inevitably suffer from the missing value problem with different underlying causes.82 As missing data hampers a reliable and efficient integrated multi-omics analysis, appropriate missing value handling/imputation processes are necessary.92,115 There are already some multi-omics analysis tools and pre-processing protocols before integrative analysis addressing this issue. However, comprehensive studies systematically evaluating the effects of these approaches on the results are needed to correctly examine the consequences of these applications on the results obtained from the integrated multi-omics analysis.
Drawing biological interpretations from integrated multi-omics analyses can be challenging, mainly due to the complex nature of microbial ecosystems and the need for comprehensive reference databases and knowledge of analysed biomolecules. In addition, some omics analyses, such as metabolomics, suffer from a high percentage of unknown biomolecules detected in samples which substantially limits the translational success in microbiome studies.72 The broader use of omics technologies and collaborative efforts would be valuable to circumvent these limitations.
Development of easy-to-access, secure, and efficient data storage and management strategies and high-performance, scalable analysis tools are critical to keeping pace with the exponential growth of microbiome multi-omics data. In addition, availability of the data from conducted multi-omics studies, standardization of experimental and bioinformatics protocols and increasing reproducibility of the analyses are vital topics that demand attention in the future.
Future perspectives
As in all other biological and medical fields, the microbiome field has benefited from the rapid progress of multi-omics applications in the last decade. However, thus far, primarily large research groups with a previous experience of omics technologies have been applying these analyses while wider microbiology community has yet to start using them due to a lack of know-how and current high costs. Therefore, projecting the developments in the multi-omics sciences into the future based on the experience with amplicon sequencing in the microbiome field, which has become a routine application now, we expect a broadening use will essentially depend on both the development of standardized sample preparation protocols with extensive documentations and the improvement of the novel cost-effective analysis techniques. At this point, we emphasize that user feedback would be critically important for the enhanced usability of protocols and tools. In addition, developing and spreading the novel analysis techniques proposed to address the current challenges (such as long-read sequencing for DNA/RNA targeting approaches,116–118 and DIA for metaproteomics119,120) would contribute to the advances in the field. Furthermore, increasing comparability between different studies via standardizing wet lab and bioinformatics protocols would boost the discovery and applications. Finally, developing new integration strategies considering the limitations of each omics data type and allowing further integration with other data types, such as clinical tests, are promising research topics of the multi-omics sciences. We summarize several example studies applying integrated multi-omics analyses of microbiomes in Table 3.
Table 3 Example studies for integrated multi-omics of microbiomes
Study |
Biome |
Omics types conducted |
Integration tool |
Key findings |
Lloyd-Price et al.121 |
Human |
Metagenomics |
Hierarchical All-against-All association testing122 |
Identified relationships between the molecular features of gut microbiome during inflammatory bowel disease activity via a longitudinal multi-omic study design and determined microbial, biochemical and host factors associated with disease dynamics. |
Metatranscriptomics |
Metaproteomics |
Metabolomics |
Viromics |
Zhang et al.123 |
Human |
Amplicon sequencing |
mixOmics (DIABLO) |
Provided potential novel biomarkers for detection of colorectal cancer by integrating gut microbiome and metabolome data. |
Metabolomics |
Sun et al.7 |
Animal |
Metagenomics |
Hmisc124 |
Revealed the molecular interactions in the organs that are central to digestion, metabolism and milk production in dairy cows and reported a biomarker for low milk production in corn stover-fed cows |
Metatranscriptomics |
Metabolomics |
Ichihashi et al.125 |
Plant-Soil |
Amplicon sequencing |
WGCNA126 |
Reported soil organic nitrogen generated by soil solarization as one of the significant factors that can boost crop productivity. |
Ionomics |
Metabolomics |
Herold et al.100 |
Wastewater |
Metagenomics |
IMP, Procrustes, cor function in R |
Provided a comprehensive characterization of microbial ecosystem in a biological wastewater treatment plant and presented significant insights into phenotypic plasticity and niche complementarity lipid-accumulating microbiomes. |
Metatranscriptomics |
Metaproteomics |
Metabolomics |
Insufficient sample sizes are among the main problems for microbiome studies, which result in inconsistent findings between different studies.127 Thus, international collaborations would promote employing large cohorts and increase the robustness of the results in the multi-omics studies in the microbiome field. There have already been large projects on multi-omic studies of the human microbiome, such as the Integrative Human Microbiome Project3 and The National Microbiome Data Collaborative,128 and international initiatives for comprehensive assessments and best practice identifications for single omics protocols through multi-laboratory comparisons.60,129,130 New international collaborations focusing on the integrated multi-omics analysis of both human and environmental microbial communities are needed in the future to provide best practices and propose solutions for the current limitations.
Another critical aspect of multi-omics analyses of microbiomes is cost, as this determines the financial feasibility of their use in microbiome studies. Although we have been witnessing a continuous decrease in the costs of omics applications, these applications are still not cost-effective for many research groups around the world. There has been a sharp decrease in the costs of DNA/RNA sequencing technologies which tends to stabilize unless new cost-effective technologies create a new wave. We expect a similar decrease for metaproteomics and metabolomics applications in the coming years as the scientific community has started to employ these techniques more in the last few years.
Multi-omics analyses of microbial communities necessitate interactions between different disciplines which would be among the driving forces for these studies. Also, using automated systems for experimental procedures and employing innovative computational methodologies such as machine learning and artificial intelligence in multi-omics studies would help interpret the results of multi-omics studies and disentangle complex interactions in microbial ecosystems.
Conclusions
Despite their current limitations, integrated multi-omics analyses are finding increasing use in the microbiome field, as they provide deeper insight into dynamics and interactions of microbial ecosystems when compared with single omics analyses. Future advances and potential solutions to the current challenges are expected to accelerate the impact of multi-omics on the microbiome field and facilitate its clinical translation such as in better disease diagnosis, personalized medicine, preventative and therapeutic interventions, and biotechnological applications such as bioremediation, sustainable agriculture, and farming.
In this review, we summarized experimental and bioinformatics aspects of both individual omics types and integrated multi-omics analyses. In addition, we presented various available multi-omics integration tools in microbiome research with example studies. Moreover, we discussed the main current challenges, future perspectives, and potential implications. Overall, our review highlights current concepts, opportunities and challenges posed by multi-omics methods in the microbiome field.
Author contributions
MA and TM conceived the idea. MA drafted and wrote the manuscript. TM reviewed and edited the manuscript. Both authors approved the final version of the manuscript.
Conflicts of interest
There are no conflicts to declare.
Acknowledgements
Muzaffer Arıkan is a recipient of the Scientific and Technological Research Council of Turkey (TUBITAK), BIDEB 2219-International Postdoctoral Research Fellowship.
References
- G. Berg, D. Rybakova, D. Fischer, T. Cernava, M.-C. C. Vergès, T. Charles, X. Chen, L. Cocolin, K. Eversole, G. H. Corral, M. Kazou, L. Kinkel, L. Lange, N. Lima, A. Loy, J. A. Macklin, E. Maguin, T. Mauchline, R. McClure, B. Mitter, M. Ryan, I. Sarand, H. Smidt, B. Schelkle, H. Roume, G. S. Kiran, J. Selvin, R. S. C. de Souza, L. van Overbeek, B. K. Singh, M. Wagner, A. Walsh, A. Sessitsch and M. Schloter, Microbiome, 2020, 8, 103 CrossRef PubMed.
- L. W. Hugerth and A. F. Andersson, Front. Microbiol., 2017, 8, 1–22 CrossRef PubMed.
- L. M. Proctor, H. H. Creasy, J. M. Fettweis, J. Lloyd-Price, A. Mahurkar, W. Zhou, G. A. Buck, M. P. Snyder, J. F. Strauss, G. M. Weinstock, O. White and C. Huttenhower, Nature, 2019, 569, 641–648 CrossRef PubMed.
- P. J. Turnbaugh, R. E. Ley, M. Hamady, C. M. Fraser-Liggett, R. Knight and J. I. Gordon, Nature, 2007, 449, 804 CrossRef CAS PubMed.
- R. A. T. Mars, Y. Yang, T. Ward, M. Houtti, S. Priya, H. R. Lekatz, X. Tang, Z. Sun, K. R. Kalari, T. Korem, Y. Bhattarai, T. Zheng, N. Bar, G. Frost, A. J. Johnson, W. van Treuren, S. Han, T. Ordog, M. Grover, J. Sonnenburg, M. D’Amato, M. Camilleri, E. Elinav, E. Segal, R. Blekhman, G. Farrugia, J. R. Swann, D. Knights and P. C. Kashyap, Cell, 2020, 182, 1460–1473 CrossRef CAS PubMed.
- C. H. Park, C. Hong, A. Lee, J. Sung and T. H. Hwang, iScience, 2022, 25, 103956 CrossRef CAS PubMed.
- H. Z. Sun, H. Z. Sun, M. Zhou, O. Wang, Y. Chen, J. X. Liu and L. L. Guan, Bioinformatics, 2020, 36, 2530–2537 CrossRef CAS PubMed.
- A. Alyass, M. Turcotte and D. Meyre, BMC Med. Genomics, 2015, 8, 1–12 CrossRef PubMed.
- X. Zhang, L. Li, J. Butcher, A. Stintzi and D. Figeys, Microbiome, 2019, 7, 154 CrossRef PubMed.
- P. D. Schloss, mBio, 2018, 9, e00525–18 CrossRef PubMed.
- N. Zhang, S. Kandalai, X. Zhou, F. Hossain and Q. Zheng, iMeta, 2023, 2, 1–26 CrossRef.
- I. Subramanian, S. Verma, S. Kumar, A. Jere and K. Anamika, Bioinform. Biol. Insights, 2020, 14, 117793221989905 CrossRef PubMed.
- H. Mallick, S. Ma, E. A. Franzosa, T. Vatanen, X. C. Morgan and C. Huttenhower, Genome Biol., 2017, 18, 228 CrossRef PubMed.
- L. Nyholm, A. Koziol, S. Marcos, A. B. Botnen, O. Aizpurua, S. Gopalakrishnan, M. T. Limborg, M. T. P. Gilbert and A. Alberdi, iScience, 2020, 23, 101414 CrossRef CAS PubMed.
- S. Graw, K. Chappell, C. L. Washam, A. Gies, J. Bird, M. S. Robeson and S. D. Byrum, Mol. Omi., 2021, 17, 170–185 RSC.
- F. Sanger and A. Coulson, J. Mol. Biol., 1975, 94, 441 CrossRef CAS PubMed.
- C. R. Woese, Microbiol. Rev., 1987, 51, 221–271 CrossRef CAS PubMed.
-
Ş. Ari and M. Arikan, Plant Omics: Trends and Applications, Springer International Publishing, Cham, 2016, pp. 109–135 Search PubMed.
- Y. X. Liu, Y. Qin, T. Chen, M. Lu, X. Qian, X. Guo and Y. Bai, Protein Cell, 2021, 12, 315–330 CrossRef PubMed.
- D. Kim, C. E. Hofstaedter, C. Zhao, L. Mattei, C. Tanes, E. Clarke, A. Lauder, S. Sherrill-Mix, C. Chehoud, J. Kelsen, M. Conrad, R. G. Collman, R. Baldassano, F. D. Bushman and K. Bittinger, Microbiome, 2017, 5, 52 CrossRef PubMed.
- S. V. Jenkins, K. B. Vang, A. Gies, R. J. Griffin, S.-R. Jun, I. Nookaew and R. P. M. Dings, BMC Microbiol., 2018, 18, 227 CrossRef CAS PubMed.
- J. M. Choo, L. E. X. Leong and G. B. Rogers, Sci. Rep., 2015, 5, 16350 CrossRef CAS PubMed.
- C. Allaband, D. McDonald, Y. Vázquez-Baeza, J. J. Minich, A. Tripathi, D. A. Brenner, R. Loomba, L. Smarr, W. J. Sandborn, B. Schnabl, P. Dorrestein, A. Zarrinpar and R. Knight, Clin. Gastroenterol. Hepatol., 2019, 17, 218–230 CrossRef PubMed.
- E. J. de Muinck, P. Trosvik, G. D. Gilfillan, J. R. Hov and A. Y. M. Sundaram, Microbiome, 2017, 5, 68 CrossRef PubMed.
- C. Quast, E. Pruesse, P. Yilmaz, J. Gerken, T. Schweer, P. Yarza, J. Peplies and F. O. Glöckner, Nucleic Acids Res., 2013, 41, 590–596 CrossRef PubMed.
- J. Galloway-Peña and B. Hanson, Dig. Dis. Sci., 2020, 65, 674–685 CrossRef PubMed.
- F. Wemheuer, J. A. Taylor, R. Daniel, E. Johnston, P. Meinicke, T. Thomas and B. Wemheuer, Environ. Microbiomes, 2020, 15, 1–12 CrossRef PubMed.
- G. M. Douglas, V. J. Maffei, J. R. Zaneveld, S. N. Yurgel, J. R. Brown, C. M. Taylor, C. Huttenhower and M. G. I. Langille, Nat. Biotechnol., 2020, 38, 685–688 CrossRef CAS PubMed.
- J. Handelsman, M. R. Rondon, S. F. Brady, J. Clardy and R. M. Goodman, Chem. Biol., 1998, 5, R245–9 CrossRef CAS PubMed.
- G. W. Tyson, J. Chapman, P. Hugenholtz, E. E. Allen, R. J. Ram, P. M. Richardson, V. V. Solovyev, E. M. Rubin, D. S. Rokhsar and J. F. Banfield, Nature, 2004, 428, 37–43 CrossRef CAS PubMed.
- J. C. Venter, K. Remington, J. F. Heidelberg, A. L. Halpern, D. Rusch, J. A. Eisen, D. Wu, I. Paulsen, K. E. Nelson, W. Nelson, D. E. Fouts, S. Levy, A. H. Knap, M. W. Lomas, K. Nealson, O. White, J. Peterson, J. Hoffman, R. Parsons, H. Baden-Tillson, C. Pfannkoch, Y.-H. Rogers and H. O. Smith, Science, 2004, 304, 66–74 CrossRef CAS PubMed.
- M. Kim, K.-H. Lee, S.-W. Yoon, B.-S. Kim, J. Chun and H. Yi, Genomics Inform., 2013, 11, 102 CrossRef PubMed.
- H.-J. Gwak, S. J. Lee and M. Rho, J. Microbiol., 2021, 59, 233–241 CrossRef PubMed.
- C. Quince, A. W. Walker, J. T. Simpson, N. J. Loman and N. Segata, Nat. Biotechnol., 2017, 35, 833–844 CrossRef CAS PubMed.
- S. Hiraoka, C. Yang and W. Iwasaki, Microbes Environ., 2016, 31, 204 CrossRef PubMed.
- T. Thomas, J. Gilbert and F. Meyer, Microb. Inf. Exp., 2012, 2, 3 CrossRef PubMed.
- F. P. Breitwieser, J. Lu and S. L. Salzberg, Briefings Bioinf., 2017, 1–15 Search PubMed.
- M. B. Jones, S. K. Highlander, E. L. Anderson, W. Li, M. Dayrit, N. Klitgord, M. M. Fabani, V. Seguritan, J. Green, D. T. Pride, S. Yooseph, W. Biggs, K. E. Nelson and J. C. Venter, Proc. Natl. Acad. Sci. U. S. A., 2015, 112, 14024–14029 CrossRef CAS PubMed.
- M. Kumar, B. Ji, K. Zengler and J. Nielsen, Nat. Microbiol., 2019, 4, 1253–1267 CrossRef CAS PubMed.
- Y. Zhou, M. Liu and J. Yang, Microbiol. Res., 2022, 260, 127023 CrossRef CAS PubMed.
- M. Shakya, C. C. Lo and P. S. G. Chain, Front. Genet., 2019, 10, 1–10 CrossRef PubMed.
- M. J. Gosalbes, A. Durbán, M. Pignatelli, J. J. Abellan, N. Jiménez-Hernández, A. E. Pérez-Cobas, A. Latorre and A. Moya, PLoS One, 2011, 6, e17447 CrossRef CAS PubMed.
- R. Higuchi, C. Fockler, G. Dollinger and R. Watson, Nat. Biotechnol., 1993, 11, 1026–1030 CrossRef CAS PubMed.
- C. Simon and R. Daniel, Appl. Environ. Microbiol., 2011, 77, 1153–1161 CrossRef CAS PubMed.
- Y. Zhang, K. N. Thompson, T. Branck, Y. Yan, L. H. Nguyen, E. A. Franzosa and C. Huttenhower, Annu. Rev. Biomed. Data Sci., 2021, 4, 279–311 CrossRef PubMed.
- X. C. Morgan and C. Huttenhower, Gastroenterology, 2014, 146, 1437–1448 CrossRef CAS PubMed.
- P. Micke, M. Ohshima, S. Tahmasebpoor, Z.-P. Ren, A. Östman, F. Pontén and J. Botling, Lab. Invest., 2006, 86, 202–211 CrossRef CAS PubMed.
- U. Nagalakshmi, K. Waern and M. Snyder, Curr. Protoc. Mol. Biol., 2010, 89, 4–11 Search PubMed.
- S. Bashiardes, G. Zilberman-Schapira and E. Elinav, Bioinf. Biol. Insights, 2016, 10, 19–25 CAS.
- I. Gallego Romero, A. A. Pai, J. Tung and Y. Gilad, BMC Biol., 2014, 12, 42 CrossRef PubMed.
- P. Wilmes and P. L. Bond, Environ. Microbiol., 2004, 6, 911–920 CrossRef CAS PubMed.
- Y. V. Zhang, B. Wei, Y. Zhu, Y. Zhang and M. H. Bluth, Clin. Lab. Med., 2016, 36, 635–661 CrossRef PubMed.
- R. J. Ram, N. C. Verberkmoes, M. P. Thelen, G. W. Tyson, B. J. Baker, R. C. Blake, M. Shah, R. L. Hettich and J. F. Banfield, Science, 2005, 308, 1915 CrossRef CAS PubMed.
- N. C. Verberkmoes, A. L. Russell, M. Shah, A. Godzik, M. Rosenquist, J. Halfvarson, M. G. Lefsrud, J. Apajalahti, C. Tysk, R. L. Hettich and J. K. Jansson, ISME J., 2009, 3, 179–189 CrossRef CAS PubMed.
- M. Kleiner, mSystems, 2019, 4, e00115–19 CrossRef CAS PubMed.
- T. Muth, B. Y. Renard and L. Martens, Expert Rev. Proteomics, 2016, 13, 757–769 CrossRef CAS PubMed.
- R. Sajulga, C. Easterly, M. Riffle, B. Mesuere, T. Muth, S. Mehta, P. Kumar, J. Johnson, B. A. Gruening, H. Schiebenhoefer, C. A. Kolmeder, S. Fuchs, B. L. Nunn, J. Rudney, T. J. Griffin and P. D. Jagtap, PLoS One, 2020, 15, e0241503 CrossRef CAS PubMed.
- H. Schiebenhoefer, T. Van Den Bossche, S. Fuchs, B. Y. Renard, T. Muth and L. Martens, Expert Rev. Proteomics, 2019, 16, 375–390 CrossRef CAS PubMed.
- R. Heyer, K. Schallert, A. Büdel, R. Zoun, S. Dorl, A. Behne, F. Kohrs, S. Püttker, C. Siewert, T. Muth, G. Saake, U. Reichl and D. Benndorf, Front. Microbiol., 2019, 10, 1–20 CrossRef PubMed.
- T. Van Den Bossche, B. J. Kunath, K. Schallert, S. S. Schäpe, P. E. Abraham, J. Armengaud, M. Ø. Arntzen, A. Bassignani, D. Benndorf, S. Fuchs, R. J. Giannone, T. J. Griffin, L. H. Hagen, R. Halder, C. Henry, R. L. Hettich, R. Heyer, P. Jagtap, N. Jehmlich, M. Jensen, C. Juste, M. Kleiner, O. Langella, T. Lehmann, E. Leith, P. May, B. Mesuere, G. Miotello, S. L. Peters, O. Pible, P. T. Queiros, U. Reichl, B. Y. Renard, H. Schiebenhoefer, A. Sczyrba, A. Tanca, K. Trappe, J.-P. Trezzi, S. Uzzau, P. Verschaffelt, M. von Bergen, P. Wilmes, M. Wolf, L. Martens and T. Muth, Nat. Commun., 2021, 12, 7305 CrossRef CAS PubMed.
- J. A. Blakeley-Ruiz and M. Kleiner, Comput. Struct. Biotechnol. J., 2022, 20, 937–952 CrossRef CAS PubMed.
- B. J. Kunath, G. Minniti, M. Skaugen, L. H. Hagen, G. Vaaje-Kolstad, V. G. H. Eijsink, P. B. Pope and M. Arntzen, Adv. Exp. Med. Biol., 2019, 1073, 187–215 CrossRef CAS PubMed.
- X. Zhang and D. Figeys, J. Proteome Res., 2019, 18, 2370–2380 CrossRef CAS PubMed.
- D. L. Tabb, L. Vega-Montoto, P. A. Rudnick, A. M. Variyath, A. L. Ham, D. M. Bunk, L. E. Kilpatrick, D. D. Billheimer, R. K. Blackman, H. L. Cardasis, S. A. Carr, K. R. Clauser, J. D. Jaffe, K. A. Kowalski, T. A. Neubert, F. E. Regnier, B. Schilling, T. J. Tegeler, M. Wang, P. Wang, J. R. Whiteaker, L. J. Zimmerman, S. J. Fisher, B. W. Gibson, C. R. Kinsinger, M. Mesri, H. Rodriguez, S. E. Stein, P. Tempst, A. G. Paulovich, D. C. Liebler and C. Spiegelman, J. Proteome Res., 2010, 9, 761–776 CrossRef CAS PubMed.
- J. Armengaud, Environ. Microbiol., 2022, 115–125 Search PubMed.
- C. M. Grim, G. T. Luu and L. M. Sanchez, FEMS Microbiol. Lett., 2019, 366, 1–11 CrossRef PubMed.
- H. Tweeddale, L. Notley-Mcrobb and T. Ferenci, J. Bacteriol., 1998, 180, 5109–5116 CrossRef CAS PubMed.
- A. Bauermeister, H. Mannochio-Russo, L. V. Costa-Lotufo, A. K. Jarmusch and P. C. Dorrestein, Nat. Rev. Microbiol., 2022, 20, 143–160 CrossRef CAS PubMed.
- P. Giraudeau, Magn. Reson. Chem., 2017, 55, 61–69 CrossRef CAS PubMed.
- A.-H. Emwas, R. Roy, R. T. McKay, L. Tenori, E. Saccenti, G. A. N. Gowda, D. Raftery, F. Alahmari, L. Jaremko, M. Jaremko and D. S. Wishart, Metabolites, 2019, 9, 123 CrossRef CAS PubMed.
- D. K. Wissenbach, K. Oliphant, U. Rolle-Kampczyk, S. Yen, H. Höke, S. Baumann, S. B. Haange, E. F. Verdu, E. Allen-Vercoe and M. von Bergen, Int. J. Med. Microbiol., 2016, 306, 280–289 CrossRef CAS PubMed.
- A. Bhosle, Y. Wang, E. A. Franzosa and C. Huttenhower, Curr. Opin. Microbiol., 2022, 70, 102195 CrossRef CAS PubMed.
- D. Ye, X. Li, J. Shen and X. Xia, TrAC, Trends Anal. Chem., 2022, 148, 116540 CrossRef CAS.
- S. Alseekh, A. Aharoni, Y. Brotman, K. Contrepois, J. D’Auria, J. Ewald, J. C. Ewald, P. D. Fraser, P. Giavalisco, R. D. Hall, M. Heinemann, H. Link, J. Luo, S. Neumann, J. Nielsen, L. Perez de Souza, K. Saito, U. Sauer, F. C. Schroeder, S. Schuster, G. Siuzdak, A. Skirycz, L. W. Sumner, M. P. Snyder, H. Tang, T. Tohge, Y. Wang, W. Wen, S. Wu, G. Xu, N. Zamboni and A. R. Fernie, Nat. Methods, 2021, 18, 747–756 CrossRef CAS PubMed.
- I. Gertsman and B. A. Barshop, J. Inherited Metab. Dis., 2018, 41, 355–366 CrossRef CAS PubMed.
- D. K. Barupal, S. Fan and O. Fiehn, Curr. Opin. Biotechnol, 2018, 54, 1–9 CrossRef CAS PubMed.
- Z. Cai, R. C. Poulos, J. Liu and Q. Zhong, iScience, 2022, 25, 103798 CrossRef CAS PubMed.
- T. M. Santiago-Rodriguez and E. B. Hollister, Semin. Perinatol., 2021, 45, 151456 CrossRef PubMed.
- S. Graw, K. Chappell, C. L. Washam, A. Gies, J. Bird, M. S. Robeson and S. D. Byrum, Mol. Omi., 2021, 17, 170–185 RSC.
- S. Tarazona, L. Balzano-Nogueira, D. Gómez-Cabrero, A. Schmidt, A. Imhof, T. Hankemeier, J. Tegnér, J. A. Westerhuis and A. Conesa, Nat. Commun., 2020, 11, 1–13 CrossRef PubMed.
- H. Syed, G. W. Otto, D. Kelberman, C. Bacchelli and P. L. Beales, bioRxiv, 2021, 12(19), 473339 Search PubMed.
- S. Tarazona, A. Arzalluz-Luque and A. Conesa, Nat. Comput. Sci., 2021, 1, 395–402 CrossRef.
- F. R. Pinu, D. J. Beale, A. M. Paten, K. Kouremenos, S. Swarup, H. J. Schirra and D. Wishart, Metabolites, 2019, 9, 76 CrossRef CAS PubMed.
- E. S. Nakayasu, C. D. Nicora, A. C. Sims, K. E. Burnum-Johnson, Y.-M. Kim, J. E. Kyle, M. M. Matzke, A. K. Shukla, R. K. Chu, A. A. Schepmoes, J. M. Jacobs, R. S. Baric, B.-J. Webb-Robertson, R. D. Smith and T. O. Metz, mSystems, 2016, 1, e00043–16 CrossRef PubMed.
-
H. Roume, A. Heintz-Buschart, E. E. L. Muller and P. Wilmes, Sequential isolation of metabolites, RNA, DNA, and proteins from the same unique sample, Elsevier Inc., vol. 531, 1st edn, 2013 Search PubMed.
- J. Pereira-Marques, A. Hout, R. M. Ferreira, M. Weber, I. Pinto-Ribeiro, L. J. Van Doorn, C. W. Knetsch and C. Figueiredo, Front. Microbiol., 2019, 10, 1–9 CrossRef PubMed.
- F. J. Stewart, E. A. Ottesen and E. F. Delong, ISME J., 2010, 4, 896–907 CrossRef CAS PubMed.
- J. M. Abuin, N. Lopes, L. Ferreira, T. F. Pena and B. Schmidt, PLoS One, 2020, 15, 1–20 CrossRef PubMed.
- T. M. Santiago-Rodriguez and E. B. Hollister, Semin. Perinatol., 2021, 45, 151456 CrossRef PubMed.
-
M. D. Wilkinson, M. Dumontier, I. J. Aalbersberg, G. Appleton, M. Axton, A. Baak, N. Blomberg, J. W. Boiten, L. B. da Silva Santos, P. E. Bourne, J. Bouwman, A. J. Brookes, T. Clark, M. Crosas, I. Dillo, O. Dumon, S. Edmunds, C. T. Evelo, R. Finkers, A. Gonzalez-Beltran, A. J. G. Gray, P. Groth, C. Goble, J. S. Grethe, J. Heringa, P. A. C. Hoen, R. Hooft, T. Kuhn, R. Kok, J. Kok, S. J. Lusher, M. E. Martone, A. Mons, A. L. Packer, B. Persson, P. Rocca-Serra, M. Roos, R. van Schaik, S. A. Sansone, E. Schultes, T. Sengstag, T. Slater, G. Strawn, M. A. Swertz, M. Thompson, J. Van Der Lei, E. Van Mulligen, J. Velterop, A. Waagmeester, P. Wittenburg, K. Wolstencroft, J. Zhao and B. Mons, Sci. Data, 2016, 3, 1–9 Search PubMed.
- T. Cernava, D. Rybakova, F. Buscot, T. Clavel, A. C. McHardy, F. Meyer, F. Meyer, J. Overmann, B. Stecher, A. Sessitsch, M. Schloter, G. Berg, P. Arruda, T. Bartzanas, T. Kostic, P. I. Brennan, B. B. Biazotti, M. C. Champomier-Verges, T. Charles, M. Coakley, P. Cotter, D. Cowan, K. D’Hondt, I. Ferrocino, K. Foterek, G. Herrero-Corral, C. Huitema, J. Jansson, S. J. Liu, P. Malloy, E. Maguin, L. Markiewicz, R. Mcclure, A. Moser, J. Roovers, M. Ryan, I. Sarand, B. Schelkle, A. Meisner, U. Schurr, J. Selvin, E. Tsakalidou, M. Wagner, S. Wakelin, W. Wiczkowski, H. Winkler, J. Xiao, C. J. Bunthof, R. S. C. de Souza, Y. Sanz, L. Lange and H. Smidt, Environ. Microbiomes, 2022, 17, 1–10 CrossRef PubMed.
- R. Argelaguet, B. Velten, D. Arnol, S. Dietrich, T. Zenz, J. C. Marioni, F. Buettner, W. Huber and O. Stegle, Mol. Syst. Biol., 2018, 14, 1–13 CrossRef PubMed.
- B. W. Haak, R. Argelaguet, C. M. Kinsella, R. F. J. Kullberg, J. M. Lankelma, M. Deijs, M. Klein, M. F. Jebbink, F. Hugenholtz, S. Kostidis, M. Giera, T. B. M. Hakvoort, W. J. de Jonge, M. J. Schultz, T. van Gool, T. van der Poll, W. M. de Vos, L. M. van der Hoek and W. J. Wiersinga, mSystems, 2021, 6, e01148–20 CrossRef CAS PubMed.
- F. Mikaeloff, M. Gelpi, R. Benfeitas, A. D. Knudsen, B. Vestad, J. Høgh, J. R. Hov, T. Benfield, D. Murray, C. G. Giske, A. Mardinoglu, M. Trøseid, S. D. Nielsen and U. Neogi, eLife, 2023, 12, 1–25 CrossRef PubMed.
- F. Rohart, B. Gautier, A. Singh and K.-A. Le Cao, PLoS Comput. Biol., 2017, 13, e1005752 CrossRef PubMed.
- A. Singh, C. P. Shannon, B. Gautier, F. Rohart, M. Vacher, S. J. Tebbutt and K. A. L. Cao, Bioinformatics, 2019, 35, 3055–3062 CrossRef CAS PubMed.
- Z. Liu, X. Dai, H. Zhang, R. Shi, Y. Hui, X. Jin, W. Zhang, L. Wang, Q. Wang, D. Wang, J. Wang, X. Tan, B. Ren, X. Liu, T. Zhao, J. Wang, J. Pan, T. Yuan, C. Chu, L. Lan, F. Yin, E. Cadenas, L. Shi, S. Zhao and X. Liu, Nat. Commun., 2020, 11, 855 CrossRef CAS PubMed.
- T. Dapa, R. S. Ramiro, M. F. Pedro, I. Gordo and K. B. Xavier, Cell Host Microbe, 2022, 30, 183–199 CrossRef CAS PubMed.
- S. Narayanasamy, Y. Jarosz, E. E. L. Muller, A. Heintz-Buschart, M. Herold, A. Kaysen, C. C. Laczny, N. Pinel, P. May and P. Wilmes, Genome Biol., 2016, 17, 260 CrossRef PubMed.
- M. Herold, S. Martínez Arbas, S. Narayanasamy, A. R. Sheik, L. A. K. Kleine-Borgmann, L. A. Lebrun, B. J. Kunath, H. Roume, I. Bessarab, R. B. H. Williams, J. D. Gillece, J. M. Schupp, P. S. Keim, C. Jäger, M. R. Hoopmann, R. L. Moritz, Y. Ye, S. Li, H. Tang, A. Heintz-Buschart, P. May, E. E. L. Muller, C. C. Laczny and P. Wilmes, Nat. Commun., 2020, 11, 581 CrossRef PubMed.
- L. de Nies, V. Galata, C. Martin-Gallausiaux, M. Despotovic, S. B. Busi, C. J. Snoeck, L. Delacour, D. P. Budagavi, C. C. Laczny, J. Habier, P. C. Lupu, R. Halder, J. V. Fritz, T. Marques, E. Sandt, M. P. O’Sullivan, S. Ghosh, V. Satagopam, R. Krüger, G. Fagherazzi, M. Ollert, F. Q. Hefeng, P. May and P. Wilmes, Microbiome, 2023, 11, 46 CrossRef PubMed.
- M. Muñoz-Benavent, F. Hartkopf, T. Van Den Bossche, V. C. Piro, C. García-Ferris, A. Latorre, B. Y. Renard and T. Muth, NAR: Genomics Bioinf., 2020, 2, 1–13 Search PubMed.
- J. T. Morton, A. A. Aksenov, L. F. Nothias, J. R. Foulds, R. A. Quinn, M. H. Badri, T. L. Swenson, M. W. Van Goethem, T. R. Northen, Y. Vazquez-Baeza, M. Wang, N. A. Bokulich, A. Watters, S. J. Song, R. Bonneau, P. C. Dorrestein and R. Knight, Nat. Methods, 2019, 16, 1306–1314 CrossRef CAS PubMed.
- C. Allaband, A. Lingaraju, C. Martino, B. Russell, A. Tripathi, O. Poulsen, A. C. Dantas Machado, D. Zhou, J. Xue, E. Elijah, A. Malhotra, P. C. Dorrestein, R. Knight, G. G. Haddad and A. Zarrinpar, mSystems, 2021, 6, 1–17 CrossRef PubMed.
- C. Meng, B. Kuster, A. C. Culhane and A. M. Gholami, BMC Bioinf., 2014, 15, 1–13 Search PubMed.
- E. J. Min and Q. Long, BMC Bioinf., 2020, 21, 1–12 CrossRef PubMed.
- A. Heintz-Buschart, P. May, C. C. Laczny, L. A. Lebrun, C. Bellora, A. Krishna, L. Wampach, J. G. Schneider, A. Hogan, C. de Beaufort and P. Wilmes, Nat. Microbiol., 2016, 2, 16180 CrossRef CAS PubMed.
- J. Zoppi, J.-F. Guillaume, M. Neunlist and S. Chaffron, BMC Bioinf., 2021, 22, 6 CrossRef PubMed.
- S. Hawinkel, L. Bijnens, K.-A. L. Cao and O. Thas, NAR: Genomics Bioinf., 2020, 2, 1–12 Search PubMed.
- D. Ruiz-Perez, J. Lugo-Martinez, N. Bourguignon, K. Mathee, B. Lerner, Z. Bar-Joseph and G. Narasimhan, mSystems, 2021, 6, e01105–20 CrossRef CAS PubMed.
- M. Krassowski, V. Das, S. K. Sahu and B. B. Misra, Front. Genet., 2020, 11, 1–17 CrossRef PubMed.
- G. B. Gloor, J. M. Macklaim, V. Pawlowsky-Glahn and J. J. Egozcue, Front. Microbiol., 2017, 8, 1–6 CrossRef PubMed.
- L. Sisk-Hackworth and S. T. Kelley, NAR: Genomics Bioinf., 2020, 2, 1–8 CAS.
- D. Jiang, C. R. Armour, C. Hu, M. Mei, C. Tian, T. J. Sharpton and Y. Jiang, Front. Genet., 2019, 10, 1–19 CrossRef CAS PubMed.
- M. Song, J. Greenbaum, J. Luttrell, W. Zhou, C. Wu, H. Shen, P. Gong, C. Zhang and H. W. Deng, Front. Genet., 2020, 11, 1–15 CrossRef PubMed.
- D. G. Maghini, E. L. Moss, S. E. Vance and A. S. Bhatt, Nat. Protoc., 2021, 16, 458–471 CrossRef CAS PubMed.
- L. Ciuffreda, H. Rodríguez-Pérez and C. Flores, Comput. Struct. Biotechnol. J., 2021, 19, 1497–1511 CrossRef CAS PubMed.
- S. L. Amarasinghe, S. Su, X. Dong, L. Zappia, M. E. Ritchie and Q. Gouil, Genome Biol., 2020, 21, 30 CrossRef PubMed.
- S. Pietilä, T. Suomi and L. L. Elo, ISME Commun., 2022, 2, 51 CrossRef.
- L. C. Gillet, P. Navarro, S. Tate, H. Röst, N. Selevsek, L. Reiter, R. Bonner and R. Aebersold, Mol. Cell. Proteomics, 2012, 11, 1–17 CrossRef PubMed.
- J. Lloyd-Price, C. Arze, A. N. Ananthakrishnan, M. Schirmer, J. Avila-Pacheco, T. W. Poon, E. Andrews, N. J. Ajami, K. S. Bonham, C. J. Brislawn, D. Casero, H. Courtney, A. Gonzalez, T. G. Graeber, A. B. Hall, K. Lake, C. J. Landers, H. Mallick, D. R. Plichta, M. Prasad, G. Rahnavard, J. Sauk, D. Shungin, Y. Vázquez-Baeza, R. A. White, J. Braun, L. A. Denson, J. K. Jansson, R. Knight, S. Kugathasan, D. P. B. McGovern, J. F. Petrosino, T. S. Stappenbeck, H. S. Winter, C. B. Clish, E. A. Franzosa, H. Vlamakis, R. J. Xavier and C. Huttenhower, Nature, 2019, 569, 655–662 CrossRef CAS PubMed.
- A. R. Ghazi, K. Sucipto, A. Rahnavard, E. A. Franzosa, L. J. McIver, J. Lloyd-Price, E. Schwager, G. Weingart, Y. S. Moon, X. C. Morgan, L. Waldron and C. Huttenhower, Bioinformatics, 2022, 38, I378–I385 CrossRef PubMed.
- S.-L. Zhang, L.-S. Cheng, Z.-Y. Zhang, H.-T. Sun and J.-J. Li, Pharmacol. Res., 2023, 188, 106633 CrossRef CAS PubMed.
-
F. E. Harrell Jr, 2019.
- Y. Ichihashi, Y. Date, A. Shino, T. Shimizu, A. Shibata, K. Kumaishi, F. Funahashi, K. Wakayama, K. Yamazaki, A. Umezawa, T. Sato, M. Kobayashi, M. Kamimura, M. Kusano, F.-S. Che, M. O’Brien, K. Tanoi, M. Hayashi, R. Nakamura, K. Shirasu, J. Kikuchi and N. Nihei, Proc. Natl. Acad. Sci. U. S. A., 2020, 117, 14552–14560 CrossRef CAS PubMed.
- P. Langfelder and S. Horvath, BMC Bioinf., 2008, 9, 559 CrossRef PubMed.
- D. Rothschild, S. Leviatan, A. Hanemann, Y. Cohen, O. Weissbrod and E. Segal, PLoS One, 2022, 17, 11–15 CrossRef PubMed.
- E. M. Wood-Charlson, F. Anubhav, D. Auberry, H. Blanco, M. I. Borkum, Y. E. Corilo, K. W. Davenport, S. Deshpande, R. Devarakonda, M. Drake, W. D. Duncan, M. C. Flynn, D. Hays, B. Hu, M. Huntemann, P. E. Li, M. Lipton, C. C. Lo, D. Millard, K. Miller, P. D. Piehowski, S. Purvine, T. B. K. Reddy, M. Shakya, J. C. Sundaramurthi, P. Vangay, Y. Wei, B. E. Wilson, S. Canon, P. S. G. Chain, K. Fagnan, S. Martin, L. A. McCue, C. J. Mungall, N. J. Mouncey, M. E. Maxon and E. A. Eloe-Fadrosh, Nat. Rev. Microbiol., 2020, 18, 313–314 CrossRef CAS PubMed.
- F. Meyer, A. Fritz, Z. L. Deng, D. Koslicki, T. R. Lesker, A. Gurevich, G. Robertson, M. Alser, D. Antipov, F. Beghini, D. Bertrand, J. J. Brito, C. T. Brown, J. Buchmann, A. Buluç, B. Chen, R. Chikhi, P. T. L. C. Clausen, A. Cristian, P. W. Dabrowski, A. E. Darling, R. Egan, E. Eskin, E. Georganas, E. Goltsman, M. A. Gray, L. H. Hansen, S. Hofmeyr, P. Huang, L. Irber, H. Jia, T. S. Jørgensen, S. D. Kieser, T. Klemetsen, A. Kola, M. Kolmogorov, A. Korobeynikov, J. Kwan, N. LaPierre, C. Lemaitre, C. Li, A. Limasset, F. Malcher-Miranda, S. Mangul, V. R. Marcelino, C. Marchet, P. Marijon, D. Meleshko, D. R. Mende, A. Milanese, N. Nagarajan, J. Nissen, S. Nurk, L. Oliker, L. Paoli, P. Peterlongo, V. C. Piro, J. S. Porter, S. Rasmussen, E. R. Rees, K. Reinert, B. Renard, E. M. Robertsen, G. L. Rosen, H. J. Ruscheweyh, V. Sarwal, N. Segata, E. Seiler, L. Shi, F. Sun, S. Sunagawa, S. J. Sørensen, A. Thomas, C. Tong, M. Trajkovski, J. Tremblay, G. Uritskiy, R. Vicedomini, Z. Wang, Z. Wang, Z. Wang, A. Warren, N. P. Willassen, K. Yelick, R. You, G. Zeller, Z. Zhao, S. Zhu, J. Zhu, R. Garrido-Oter, P. Gastmeier, S. Hacquard, S. Häußler, A. Khaledi, F. Maechler, F. Mesny, S. Radutoiu, P. Schulze-Lefert, N. Smit, T. Strowig, A. Bremges, A. Sczyrba and A. C. McHardy, Nat. Methods, 2022, 19, 429–440 CrossRef CAS PubMed.
- A. Sczyrba, P. Hofmann, P. Belmann, D. Koslicki, S. Janssen, J. Dröge, I. Gregor, S. Majda, J. Fiedler, E. Dahms, A. Bremges, A. Fritz, R. Garrido-Oter, T. S. Jørgensen, N. Shapiro, P. D. Blood, A. Gurevich, Y. Bai, D. Turaev, M. Z. Demaere, R. Chikhi, N. Nagarajan, C. Quince, F. Meyer, M. Balvočiutė, L. H. Hansen, S. J. Sørensen, B. K. H. Chia, B. Denis, J. L. Froula, Z. Wang, R. Egan, D. Don Kang, J. J. Cook, C. Deltel, M. Beckstette, C. Lemaitre, P. Peterlongo, G. Rizk, D. Lavenier, Y. W. Wu, S. W. Singer, C. Jain, M. Strous, H. Klingenberg, P. Meinicke, M. D. Barton, T. Lingner, H. H. Lin, Y. C. Liao, G. G. Z. Silva, D. A. Cuevas, R. A. Edwards, S. Saha, V. C. Piro, B. Y. Renard, M. Pop, H. P. Klenk, M. Göker, N. C. Kyrpides, T. Woyke, J. A. Vorholt, P. Schulze-Lefert, E. M. Rubin, A. E. Darling, T. Rattei and A. C. McHardy, Nat. Methods, 2017, 14, 1063–1071 CrossRef CAS PubMed.
|
This journal is © The Royal Society of Chemistry 2023 |
Click here to see how this site uses Cookies. View our privacy policy here.