Multifaceted mutational immunotherapeutic approach to design therapeutic mAbs to combat monkeypox disease via integrated screening algorithms and antibody engineering†
Received
17th April 2023
, Accepted 15th June 2023
First published on 16th June 2023
Abstract
After a multi-country outbreak, the monkeypox (MPX) disease was designated a global public health emergency on July 23, 2022. Some antiviral medications tailored to the smallpox virus are currently being used to treat the disease. There is no specific treatment for the MPX disease with minimal negligible side effects. The engineering of antibodies has increased dramatically since the US Food and Drug Administration (US FDA) approved the first therapeutic monoclonal antibody (mAb) in 1986. mAbs have revolutionized biomedical research and have been used with remarkable precision for avoiding undesirable consequences. So, in this study, mAbs from the Thera-SAbDab (Therapeutic Structural Antibody Database) were screened using the ClusPro protein–protein docking server against the critical enzymes of the monkeypox virus (thymidine kinase, methyltransferase, D9 decapping enzyme, and RNA polymerase). Based on the predicted ClusPro docking score, binding affinity (ΔG), dissociation constant (Kd), and physiochemical properties, the best two mAbs (eculizumab and vofatamab) were designated for further investigation. Furthermore, the CUPSAT server and PyMol mutagenesis wizard were employed to generate a mutant pool (up to triple mutant through permutation combinations) and investigate the binding affinity of the candidate mAbs following point mutation. Eventually, the mAbs eculizumab mutant (L: L92F) and vofatamab mutant (L: H94T, L: Q96V) were identified as the most effective and promising inhibitors targeting all four MPXV enzymes, based on molecular dynamics (MD) simulations and MD trajectory assessment. In the future, in vitro and in vivo experiments on promising mAbs identified and developed by us could aid virus neutralization in MPXV-infected patients.
Design, System, Application
This study's molecular design strategy involved screening a database of therapeutic monoclonal antibodies (mAbs) against the critical enzymes of the monkeypox virus using protein–protein docking servers and mutagenesis methods. The objective was to identify mAbs with high binding affinity and antiviral efficacy that could be further developed for use as inhibitors in infected patients. This strategy represents a promising and effective method for designing targeted therapeutics for viral infections. In this instance, the desired system functionality was identifying the most effective monoclonal antibodies (mAbs) against the MPXV. The design constraints required screening many monoclonal antibodies (mAbs) and generating a pool of mutants for testing. The study used a combination of computational techniques, such as protein–protein docking, mutagenesis, and molecular dynamics simulations, to surmount these limitations and identify the most promising inhibitors. This work has the potential for immediate implementation in developing targeted therapies for patients infected with the monkeypox virus. Eculizumab and vofatamab, the two most effective mAbs identified in this study, could neutralize the virus and enhance patient outcomes. This study demonstrates the promise of integrating computational and experimental approaches to molecular design and optimization, with implications for viral therapeutics.
|
1. Introduction
The monkeypox virus, a neglected zoonotic virus endemic to West and Central Africa, has recently sparked significant international interest because of the global 2022 monkeypox outbreak.1,2 This sporadic infection has primarily been reported in African countries since its first nonhuman case in 1958 in Denmark3 and the first human case in 1970 in the Democratic Republic of Congo.4 It has dispersed to non-endemic countries due to the travel or importation of infected animals. The first outbreak in the summer of 2003 in the United States, caused by pet prairie dogs imported from Ghana, established monkeypox as a disease of global public health concern. Since its first outbreak, reports of it have been made in various endemic and non-endemic nations, with the largest outbreak in Nigeria in 2017.5 As of January 11, 2023, the Centers for Disease Control and Prevention (CDC) has confirmed 8447 cases in 110 countries, including 103 countries that have never previously reported monkeypox.6
The monkeypox virus is a member of the Poxviridae family of double-stranded deoxyribonucleic acid viruses. The Poxviridae family of viruses, which includes smallpox, vaccinia, and cowpox viruses, is the most important known vertebrate virus family, affecting humans and a wide range of animals such as birds, reptiles, insects, mammals, etc. The family is divided into two subfamilies: Chordopoxvirinae (which includes 18 genera and 52 species) and Entomopoxvirinae (with four genera and 30 species). Monkeypox, with a broad host range, belongs to the Orthopoxvirus genus of the Chordopoxvirinae subfamily.7 Human monkeypox has a clinical presentation similar to ordinary smallpox but is less fatal, with a characteristic rash preceded by mild prodromal symptoms and case fatality rates of around 10%. Because orthopoxviruses share genetic and antigenic characteristics, an immune response to any orthopoxvirus-caused illness reduces the likelihood of infection with other orthopoxviruses.8 Smallpox vaccination has been shown to protect against monkeypox. After smallpox was eradicated in the 1970s, discontinuing the smallpox vaccine gradually reduced cross-immunity and antibodies against other orthopoxviruses.8,9
Monkeypox viruses spread from animals to humans via bites, blood, bodily fluids, or the consumption of improperly cooked infected animals.10 In human-to-human transmission, the virus is transmitted through direct face-to-face or mucocutaneous lesion contact or, less frequently, through air droplets. It can also be passed from mother to child during pregnancy through the fluids of an infected person or virus-contaminated items such as clothing and bedding.11 Most cases in the current outbreak are concentrated in Europe and the western hemisphere. Cases are most common among people under 40, born after the smallpox vaccination campaign was discontinued, indicating a lack of cross-protective immunity.12 Males also have a higher prevalence of monkeypox cases. However, the precise reason for this is still unknown.
Concerning the treatment, previous research indicates that smallpox vaccination may have a protective function against the monkeypox virus, thereby improving patient clinical manifestations.13 Most patients with monkeypox recover without medical treatment, and those experiencing gastrointestinal symptoms like vomiting and diarrhoea require oral/intravenous rehydration to reduce fluid losses.14 Tecovirimat, brincidofovir, and cidofovir antiviral compounds and their combinations may effectively treat monkeypox infections. The effectiveness of these medications against monkeypox in people has yet to be studied, even though they were approved for treating smallpox based on animal models.15 Therapeutic antibodies have emerged as a predominant class of new drugs developed recently. At least 570 therapeutic mAbs have been studied in clinical trials by commercial companies worldwide, and 79 therapeutic mAbs have been approved by the United States Food and Drug Administration (US FDA) and are currently on the market. mAbs produced by B cells because of their high specificity to target antigens could be novel therapeutics with progressively fewer adverse effects.16
Homology modelling, also known as comparative modelling, is based on the biological premise that when two sequences have a high degree of identity or resemblance, they are homologous.17 Homology modelling predicts the three-dimensional structure of a query protein using template protein sequence alignment. Homology modelling typically includes the four processes listed as follows: template identification, sequence alignment, model creation, and model improvement.18 The Ramachandran plot is calculated after the 3D structure has been modelled to check the accuracy of the models based on the fraction of residues that fall within the Ramachandran plot's authorized range. PROCHECK is another tool for confirming the precision of a protein model. Proteins interact with one another rather than acting separately to carry out biological functions. However, it is difficult to directly observe them in action and obtain objective, definitive evidence of their association.19 Considering these methodologies, homology modelling can be an incredible strategy in drug development.20,21 Homology modelling is significant in drug discovery because it accurately predicts the 3D structure of the therapeutic target, allowing for the rational design of small molecule inhibitors for the target protein. Researchers can identify possible binding sites and design therapeutic candidates if an accurate model of the target protein has been developed.22,23
Similarly, homology modelling aids in designing and optimizing therapeutic antibodies in antibody engineering. Researchers can comprehend the binding interactions and pinpoint the key residues that regulate the antibody–antigen by developing antibody–antigen complex models. This information is subsequently utilized to develop antibodies with increased affinity, specificity, and stability, enhancing therapeutic efficacy.24
In our study, the therapeutic targets for mAbs were RNA polymerase, mRNA decapping enzyme, thymidine kinase, and mRNA (nucleoside-O2′-)-methyltransferase. (1) RNA polymerase is a promising therapeutic target for treating monkeypox. Because monkeypox viruses are not packaged with RNA, they must generate the RNA required for protein production during translation.25 These RNA polymerases differ from human polymerases because they lack proofreading exonuclease activity,26 resulting in a high error rate in viral mRNA synthesis, contributing to viral mutation and evolution. They also have endonuclease activity, which cleaves host cell pre-mRNA, causing the host cell to shut down and allowing the virus to take over its replication functions. The distinct properties of poxvirus RNA polymerase make it a promising target.27 (2) Decapping the protective 5′-7-methylguanosine (m7G) cap and poly (A) tail of mRNA is a necessary step in the degradation process. The degradation of mRNA by decapping enzymes inhibits host protein synthesis, limiting the accumulation of viral double-stranded RNA. Two mRNA decapping enzymes, D9 expressed early in infection and D10 expressed after viral DNA replication, control and encode the entire decapping process.28 (3) Thymidine kinase (TMPK) is a magnesium kinase that catalyzes the conversion of thymidine 5′-phosphate (dTMP) to thymidine 5′-diphosphate (dTDP). This dTDP is an essential DNA building block; inhibiting the TMPK activity can prevent viral infection.29,30 (4) The MPXV 2′-O MTase has been implicated in the methylation of initial nucleotides during the mature MPXV cap (cap-1) at the 2′-O ribose. VP39 catalyzes this enzyme, also known as mRNA (nucleoside-O2′-)-methyltransferase. To prevent the emergence of innate immune responses, Poxviridiae virus immature caps (cap-0) must be transformed into mature caps by adding another methyl group at the 2′-O ribose site. Methyltransferase is necessary for cap-0 synthesis.31 The orthopoxviruses' remarkable conservation of VP39 shows that this protein has a crucial influence on the viral lifecycle and is, therefore, a prospective therapeutic target.32
Considering the therapeutic efficacy of mAbs in recent years, we screened therapeutic mAbs to identify potential mAbs against MPXV. We mutated these potential mAbs to improve their efficacy against clinically selected essential targets to propose effective treatment options. Furthermore, mAbs have several advantages over traditional small molecule inhibitors, including higher specificity and a longer half-life, which can result in longer-term therapeutic efficacy. In our research study, we have optimized the design of some potential mAbs for maximum effectiveness and a promising therapy for treatment against the MPX disease (Fig. 1).
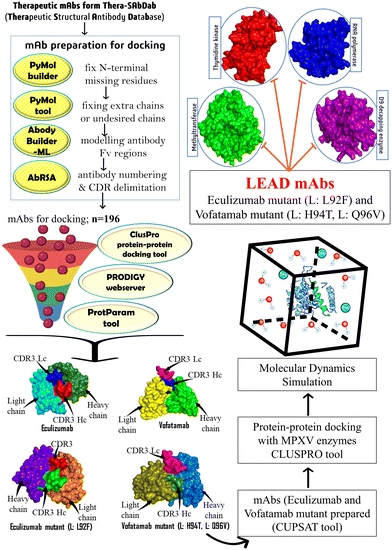 |
| Fig. 1 The methodology utilized to identify and develop potential therapeutic mAbs targeting MPXV's essential enzymes. | |
2. Methodology
2.1 Retrieval of essential enzymes and therapeutic monoclonal antibodies
In this study, the essential enzymes from MPXV were accessed from the NCBI reference genome accession number ON563414.3, which contains the whole genome of MPXV isolate USA_2022_MA001 (https://www.ncbi.nlm.nih.gov/nuccore/ON563414.3/). From the MPXV complete genome, the protein sequence of the four essential enzymes mentioned in the introduction section was retrieved in the FASTA format for further study.
Structures of therapeutic monoclonal antibodies (mAbs) were retrieved from the Thera-SAbDab, i.e., Therapeutic Structural Antibody Database (https://opig.stats.ox.ac.uk/webapps/therasabdab) (accessed on November 25, 2022).33 As a therapeutic format, the option to search therapeutics by attributes and all monoclonals was chosen, and the search was limited to known structures only to extract the structures of therapeutic mAbs from the Thera-SAbDab. Therapeutic mAb structures whose sequences in the database were available in the correct format were retrieved in pdb format from Thera-SAbDab.
2.2 Protein 3D structure prediction, refinement, model quality assessment, and structural validation
The crystal structure of the selected MPXV enzymes was not present in the Protein Data Bank (https://www.rcsb.org/). So, protein sequences in the FASTA format were retrieved from NCBI and modelled using the I-TASSER (Iterative Threading ASSEmbly Refinement) tool. I-TASSER is a protein structure and function prediction web server and is used to obtain the 3D structure of the proteins. The server is accessible for academic use with the institutional email addresses at https://zhanggroup.org/I-TASSER/.34
The GalaxyRefine (https://galaxy.seoklab.org/refine) web server was used to improve the local and global quality of the predicted model. The developers have successfully tested the accuracy of the refinement tool in the CASP10 model.35 GalaxyRefine uses the protein structure in the pdb format as the input sequence.
2.3 Therapeutic monoclonal antibody preparation for molecular interaction studies
The structures of monoclonal antibodies recovered from the Thera-SAbDab are not in the appropriate format, as some have missing residues, and the majority have additional residues. The PyMol standalone tool and PyMol builder were used to alter the residues of the mAbs in order to achieve the correct structure of the antibodies in the appropriate format. The mAbs whose tertiary (3D) structures are unavailable in the Protein Data Bank were modelled using the ABodyBuilder-ML (https://opig.stats.ox.ac.uk/webapps/newsabdab/sabpred/abodybuilder/). The PyMol requires the 3D structures of the mAbs in the pdb format as an input file, whereas the sequences of both heavy and light chains were submitted to the ABodyBuilder-ML tool for modelling the mAbs.36 The AbRSA webserver (https://cao.labshare.cn/AbRSA/) was utilized for antibody numbering and delimiting the complementary determining regions (CDRs) that establish the direct connection with antigen binding.37
2.4 Molecular interaction study between potential MPXV enzymes and therapeutic mAbs
Docking procedures are classified into two types: direct and template based. The direct docking method-based ClusPro 2.0 (https://cluspro.bu.edu/) protein–protein docking webserver was employed in this study. The ClusPro server proceeds through three key phases: (1) sampling billions of conformations for rigid body docking using the PIPER docking algorithm, premised on the FFT (fast Fourier transform) correlation technique. In this strategy, the first protein is arranged on a fixed grid called a receptor, while the second protein is placed on a movable grid called a ligand. This results in the rigorous sampling of billions of the two interacting proteins' conformations, and the interaction energy between the protein and ligand is depicted as a correlation function at each grid point;38 (2) clustering of the lowest possible energy structures based on root-mean-square deviation to obtain the most likely largest cluster model; and (3) structural improvement of the final models through energy minimization and refinement. In this way, FFT is used for protein–protein docking and efficient calculations of energy functions without prior knowledge about the proteins.39,40
During docking, the models retrieved from the GalaxyRefine web server in the pdb format were employed as the receptors, while the monoclonal antibodies (mAbs) served as the ligands. Specific molecular interaction analyses were carried out for each mAb by including the active site residues in the attraction section of the advanced options. CDR3 sequences of both the heavy and light chains were furnished for the ligand, which have previously been predicted using the AbRSA web server.37
2.5 Estimation of binding affinity (ΔG) and dissociation constant (Kd)
The structural characterization and interpretation of protein–protein interactions are critical for a comprehensive understanding of the functionality and diseases, including therapeutic advancement.41 As a result, the web-based PRODIGY (PROtein binDIng enerGY) server (https://wenmr.science.uu.nl/prodigy/) was leveraged to precisely anticipate the binding affinity (ΔG) and dissociation constant (Kd) between the vital MPXV enzymes and the mAbs. The PRODIGY server predicts protein–protein complex binding affinity based on structural features. It considers interfacial interactions that correspond with experimental binding affinity. It combines interacting and non-interacting surface features to make reliable predictions. The PRODIGY's prediction approach employs Pearson's correlation with a coefficient of 0.73 between the predicted and measured binding affinity and has a low root-mean-square error (1.89 kcal mol−1), assuring good prediction model accuracy. It is reliable for researching protein–protein interactions and determining binding affinities.41
The PRODIGY web server requires the protein–protein complex in the pdb format and the chain IDs of both proteins involved in complex formation to compute the ΔG and Kd at 25.0 °C. The PyMol standalone tool was used to determine the chain IDs of heavy and light chains of mAbs, which have been submitted to interactor 1, while the chain ID of the other protein (MPXV enzyme) was given to interactor 2. In addition, a job ID and email address were required to submit the task to the PRODIGY server.42
2.6 Estimation of physiochemical properties
The ProtParam tool (https://web.expasy.org/protparam/) provided by the Swiss Institute of Bioinformatics (SIB) was used to predict the intrinsic physiochemical properties of the mAbs. The predicted properties are the theoretical pI, GRAVY (grand average of hydropathicity) index, MW, half-life, instability, and aliphatic index. These expected characteristics essentially reflect the stability of the predicted entity. Based on the N-end rule, which correlates a protein's half-life to the type of its N-terminal residue, the server evaluates the half-life for three model species, i.e., human reticulocytes, yeast, and E. coli. However, the hydropathy values of all amino acid residues are added, and the GRAVY is calculated by dividing the total number of residues by the results.43,44
2.7 Construction of the mutant library and evaluation of the binding affinities
The parameters used to select the top two mAbs were based on the obtained results, like the ClusPro docking score, binding affinity, and the dissociation constant. Various physiochemical properties of the mAbs, like the theoretical pI, GRAVY, MW, half-life, and instability index as well as the aliphatic index, were also taken into consideration for finalizing the top two mAbs (eculizumab and vofatamab) for further studies. Next, our primary objective was to generate a mutant library of these mAbs to enhance overall binding affinity against the MPXV targets of interest. For that, a web-based CUPSAT (Cologne University Protein Stability Analysis Tool) server (https://cupsat.tu-bs.de/) was utilized to analyze and anticipate the changes in protein stability. The server executes the probable mutation for every residue with multiple standard amino acids. The server's predictive model examines the microenvironment around the mutant sites using amino acids' atomic potential and the torsion angle dispersion. Following the prediction of stabilizing and advantageous mutant residues using the CUPSAT server, PyMol's protein mutagenesis wizard, the standalone tool, was employed to generate the mutant library for future investigation.44,45
2.8 Validation of in silico enzymatic inhibition through molecular dynamics (MD) simulations
WebGro for biomolecular simulations (https://simlab.uams.edu/), developed and managed by UAMS (University of Arkansas for Medical Sciences), was used to perform the molecular dynamics simulations. WebGro employs the GROMACS (GROningen MAchine for Chemical Simulations) package, which is freely available and extensively adopted for biomolecular simulation systems.46,47 Throughout MD analysis, the GROMOS96 43a1 forcefield was chosen since it aids in critically evaluating proteins and their folding; hence, it assists in evaluating bonding and non-bonding interactions.48 The SPC water model consisting of triatomic particles and a triclinic solvent box was included. Na+ and Cl− ions inside the PBCs (periodic boundary conditions) were harnessed to modulate and neutralize the solvent box. The steepest descent method was employed to determine whether there have been any steric clashes between the MPXV enzymes and the mAbs during the protein energy minimization process. This algorithm progressively alters the atomic coordinates to obtain the molecules toward the minimal point.49 Furthermore, an NPT (constant temperature, constant pressure) ensemble was applied to maintain the biomolecular system constantly at 300 K and one atmospheric pressure (1 atm). The constant temperature of the NPT ensemble maintains thermal behaviour and mimics biological conditions. MD production runs enduring 50 ns in triplicate were performed by applying the leapfrog algorithm of integrating equations of motion. Finally, MD trajectories were analyzed by plotting the root-mean-square-deviation (RMSD), root-mean-square-fluctuations (RMSF), radius of gyration (Rg), solvent-accessible surface area (SASA), and hydrogen bonding.50
3. Results and discussion
3.1 Screening and recruitment of vital MPXV enzymes and therapeutic mAbs
The essential enzymes of MPXV were selected from the GenBank (Accession ON563414.3) (https://www.ncbi.nlm.nih.gov/genbank/), a genetic sequence database of the National Institute of Health (NIH).51 After a critical literature search, four MPXV enzymes were selected, and their protein sequences were retrieved as an RNA polymerase subunit (RPO19) (Accession URK20553.1), mRNA D9 decapping enzyme (E9R) (Accession URK20543.1), thymidine kinase (L2R) (Accession URK20523.1), and mRNA (nucleoside-O2′-)-methyltransferase (VP39) (Accession URK20524.1).
In this study, we seek therapeutic mAbs to combat the MPXV disease. Therefore, the Thera-SAbDab, a database of therapeutic structural antibodies, was selected as the starting point of our investigation. The Thera-SAbDab (updated weekly) keeps a record of all the physicochemical characteristics, including structural information of antibodies and nanobodies that the World Health Organization (WHO) has acknowledged.33 As of November 25, 2022, the Thera-SAbDab contained 202 mAbs, but data regarding six mAbs were unavailable. As a result, 196 mAbs were shortlisted for further analysis.
3.2 Structure prediction, refinement, model quality assessment, and structural validation
The I-TASSER web server developed and maintained by the Zhang Lab, University of Michigan, was employed for protein structure prediction. For precise structure and function prediction, the server uses cutting-edge algorithms. The server computed the C-score, TM-score, and estimated RMSD which are three independent scoring functions for the predicted model. An essential scoring function to assess the model's quality is the C-score, which has a confidence range of −5 to 2. The higher the C-score, the more accurate the model will be. Both the TM-score and RMSD are used to determine structural similarity, which is employed to assess the structural reliability of the predicted model (Table 1; Fig. 2).
Table 1 Summarised basic structural information such as the protein accession number, C-score, TM-score, and estimated RMSD of essential enzymes
S. no. |
MPXV enzymes as drug targets |
C-score |
TM-score |
RMSD |
1 |
RNA polymerase (URK20553.1) |
−1.56 |
0.52 ± 0.15 |
8.3 ± 4.5 Å |
2 |
mRNA decapping (URK20543.1) |
1.38 |
0.91 ± 0.06 |
2.8 ± 2.0 Å |
3 |
Thymidine kinase (URK20523.1) |
0.95 |
0.84 ± 0.08 |
3.2 ± 2.3 Å |
4 |
mRNA (2′-O)-methyltransferase (URK20524.1) |
−0.25 |
0.68 ± 0.12 |
7.0 ± 4.1 Å |
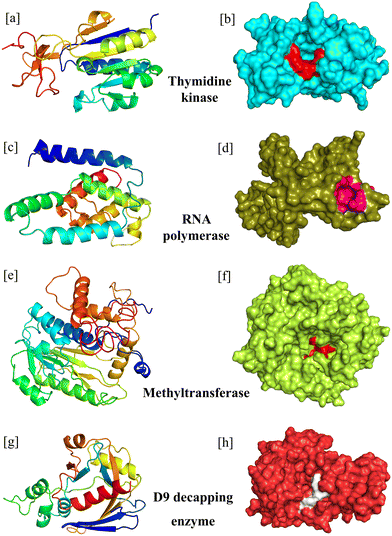 |
| Fig. 2 The modelled structures of the MPXV enzymes in schematic form and surface form (active site residues are highlighted in different colours). (a and b) Thymidine kinase, (c and d) RNA polymerase, (e and f) methyltransferase, and (g and h) D9 decapping enzyme. | |
The model was further refined using the GalaxyRefine webserver to acquire the native structure. The tool initially rebuilds the side chain and then repacks it, and then structural relaxation is achieved through repeated structural perturbation and molecular dynamics simulation. Upon refinement, the GalaxyRefine webserver delivers the top five refined models based on various criteria, such as Rama favoured, bad rotamers, clash score, MolProbity, RMSD, and GDT-HA. We chose the revised model based on the Ramachandran favoured score and RMSD to extract the best-refined model (Table S1†).
3.3 Fabrication of therapeutic monoclonal antibodies for molecular interaction studies
The PyMol tool aids in the excision of residues and unnecessary chains; meanwhile, the PyMol builder facilitates the incorporation of missing residues at the N-terminus of the mAbs. The mAbs were extracted in their correct sequential form in the pdb format. ABodyBuilder-ML, a machine learning-based antibody Fv modelling tool, constructs models of mAbs with structures that seem to be previously unavailable. Using a deep-learning paradigm, this tool uses the ABlooper to generate CDR loops.36 The most critical region of the mAbs, i.e., CDR3, was confirmed via the AbRSA tool.42 During the molecular interaction analyses, these CDR3 sequences of the mAbs were considered the active site residues for interaction studies. However, the active site residues of the essential enzymes were identified through a literature search (Table 2).
Table 2 Active site residues of essential enzymes and their references
S. no. |
MPXV enzymes |
Active site residues |
Ref. |
1 |
RNA polymerase |
L90, P96, T105, M106, Q108, M110, V113 |
52
|
2 |
mRNA decapping enzyme |
E16, R50, F54, D151, Y158 |
53
|
3 |
Thymidine kinase |
F14, S15, G16, K17, S18, F113, I157, I159 |
54
|
4 |
mRNA (nucleoside-O2′-)-methyltransferase |
Catalytic triad: K41, D138, K175 |
55
|
3.4 Potential MPXV enzymes and therapeutic mAbs: a molecular interaction analysis
Molecular interaction analysis was performed to screen out the best mAbs as potential multi-enzymatic inhibitors of MPXV. All the processed mAbs (n = 196) obtained from the Thera-SAbDab were virtually screened against the four essential enzymes of MPXV by defining the active site residues of receptors and ligands in the ClusPro web server. Additionally, we have included all the mAbs (n = 25) with scores under −1400 kcal mol−1 because a lower docking score indicates a more vital interaction between the receptor and ligand. Since many more factors can be used to screen the mAbs for possible MPXV inhibitors, the threshold, in this case, was set at a lowest value of −1400 kcal mol−1. The range selected for the ClusPro docking score was between −1407.3 kcal mol−1 (lowest) and −2381.8 kcal mol−1 (highest), and the highest docking score was shown by apitegromab against the RNA polymerase (−2381.8 kcal mol−1), while the lowest docking score was reported by two mAbs bevacizumab and ivonescimab which was −1407.3 kcal mol−1 for the D9 decapping enzyme (Table 3).
Table 3 CDS (ClusPro docking score) in kcal mol−1 of the essential enzymes of the monkeypox virus and the top 25 therapeutic monoclonal antibodies
S. no. |
Therapeutic mAbs |
Docking score of the mAbs with essential enzymes of the monkeypox virus |
Thymidine kinase |
Methyltransferase |
D9 decapping enzyme |
RNA polymerase |
CDS (kcal mol−1) |
CDS (kcal mol−1) |
CDS (kcal mol−1) |
CDS (kcal mol−1) |
1 |
Abelacimab |
−2009.5 |
−1810.7 |
−1667.2 |
−1835.9 |
2 |
Afasevikumab |
−1813.2 |
−1413.9 |
−1451.8 |
−1647.8 |
3 |
Apitegromab |
−2115.2 |
−1798.5 |
−1994.3 |
−2381.8 |
4 |
Bevacizumab |
−1912.4 |
−1554.4 |
−1407.3 |
−1553.8 |
5 |
Carlumab |
−1578.8 |
−1450.3 |
−1453.2 |
−1784.3 |
6
|
Eculizumab
|
−1980.8
|
−1703.2
|
−1668.8
|
−1833.8
|
7 |
Erenumab |
−1889.2 |
−1591.9 |
−1813.1 |
−2188.3 |
8 |
Erlizumab |
−1984.1 |
−1657.1 |
−1484.6 |
−2124.3 |
9 |
Fulranumab |
−2197.6 |
−1634.7 |
−1899.6 |
−2169.0 |
10 |
Gedivumab |
−2061.2 |
−1713.8 |
−1746.9 |
−2112.8 |
11 |
Idarucizumab |
−1728.6 |
−1483.9 |
−1426.0 |
−1913.0 |
12 |
Ivonescimab |
−1912.4 |
−1554.4 |
−1407.3 |
−1824.4 |
13 |
Lexatumumab |
−1824.9 |
−1418.4 |
1515.1 |
−1716.4 |
14 |
Ligelizumab |
−1997.8 |
−1687.1 |
−1591.4 |
−2039.6 |
15 |
Ofatumumab |
−1584.6 |
−1449.1 |
−1432.5 |
−1719.2 |
16 |
Ranibizumab |
−1986.6 |
−1543.8 |
−1530.4 |
−1874.6 |
17 |
Regdanvimab |
−1968.1 |
−1553.5 |
−1711.7 |
−2055.4 |
18 |
Sudubrilimab |
−1745.7 |
−1401.8 |
−1466.7 |
−1680.9 |
19 |
Tamrintamab |
−1848.9 |
−1494.7 |
−1514.0 |
−2033 |
20 |
Tarcocimab |
−1986.6 |
−1543.8 |
−1516.4 |
−1874.6 |
21 |
Tralokinumab |
−1872.9 |
−1526.2 |
−1541.4 |
−1764.9 |
22 |
Urelumab |
−1497.1 |
−1479.5 |
−1502.5 |
−1627.7 |
23
|
Vofatamab
|
−2058.8
|
−1843.2
|
−2229.2
|
−2008.4
|
24 |
Volagidemab |
−1809.0 |
1518.4 |
−2007.8 |
−2007.7 |
25 |
Zinlirvimab |
−1728.9 |
−1551.1 |
−1629.0 |
−1821.8 |
Finally, we utilized GRAMM, an FFT-based protein–protein docking web server, to determine whether the ligand docking sites (binding sites) are conserved. Some of the binding sites have been identified to be conserved, such as: (1) thymidine kinase – ARG26, ARG27, and GLY47; (2) methyltransferase – ASN255 and ARG292; and (3) D9 decapping enzyme – ARG51, SER61, LYS156, and HIS33 (Table S2†).
3.5 Estimation of binding affinity (ΔG) and dissociation constant (Kd)
Other screening variables, such as the binding affinity and dissociation constant calculation, are included to obtain a prospective multitargeted mAb.56 The receptor–ligand (protein–protein) complex interaction strength is significantly associated with the interface contacts. In addition to interface interactions, non-interface contacts like polar and charged residues play an essential role in the binding affinity.57 Consequently, the PRODIGY server's predictor model employs a generalized regression model of interface contacts and some parameters of non-interface surfaces.57,58 The tool marks the residues or atoms in contact if the distance between the two atoms is within 5.5 Å. Similarly, the formula for the calculation of the dissociation constant is:
ΔG = RT ln Kd |
where
R = ideal gas constant (kcal K−1 mol−1),
T = temperature (K),
ΔG = free energy
For the top-hit mAbs mentioned in Table 3, the binding affinity and dissociation constant were computed with each of the four targets (thymidine kinase, methyltransferase, D9 decapping enzyme, and RNA polymerase). Two mAbs (bevacizumab and ivonescimab with the methyltransferase) had the highest ΔG of −18.1 kcal mol−1, while gedivumab with the RNA polymerase had the lowest ΔG of −8.4 kcal mol−1. The highest Kd was observed for vofatamab with the RNA polymerase, 1.10 × 10−7 at 25.0 °C, whereas the least Kd was anticipated for mAbs bevacizumab and ivonescimab with the methyltransferase, 5.40 × 10−14 (Table 4).
Table 4 Predicted ΔG (binding affinity) in kcal mol−1 and Kd (dissociation constant) at 25.0 °C between the monoclonal antibodies and the MPXV enzymes
S. no. |
AA residues of mAb |
Therapeutic mAbs |
Binding energy and dissociation constant of the essential MPXV enzymes |
Thymidine kinase |
Methyltransferase |
D9 decapping enzyme |
RNA polymerase |
ΔG |
K
d (M) |
ΔG |
K
d (M) |
ΔG |
K
d (M) |
ΔG |
K
d (M) |
1 |
232 |
Abelacimab |
−17.3 |
2.00 × 10−13 |
−16.0 |
1.80 × 10−12 |
−10.3 |
2.90 × 10−8 |
−12.3 |
9.80 × 10−10 |
2 |
231 |
Afasevikumab |
−12.1 |
1.30 × 10−9 |
−15.0 |
9.10 × 10−12 |
−12.7 |
5.00 × 10−10 |
−13.8 |
7.20 × 10−11 |
3 |
235 |
Apitegromab |
−11.4 |
4.00 × 10−9 |
−09.9 |
5.10 × 10−8 |
−13.1 |
2.40 × 10−10 |
−11.8 |
2.20 × 10−9 |
4 |
230 |
Bevacizumab |
−16.4 |
8.90 × 10−13 |
−18.1 |
5.40 × 10−14 |
−12.2 |
1.10 × 10−9 |
−12.1 |
1.30 × 10−9 |
5 |
228 |
Carlumab |
−11.3 |
5.60 × 10−9 |
−14.4 |
2.60 × 10−11 |
−15.7 |
3.10 × 10−12 |
−12.7 |
5.20 × 10−10 |
6
|
229 |
Eculizumab
|
−14.8
|
1.30 × 10
−11
|
−15.7
|
2.80 × 10
−12
|
−14.9
|
1.10 × 10
−11
|
−12.8
|
3.90 × 10
−10
|
7 |
240 |
Erenumab |
−11.0 |
8.40 × 10−9 |
−10.7 |
1.40 × 10−8 |
−14.3 |
3.10 × 10−11 |
−10.2 |
3.60 × 10−8 |
8 |
231 |
Erlizumab |
−17.3 |
2.00 × 10−13 |
−13.9 |
5.90 × 10−11 |
−12.9 |
3.20 × 10−10 |
−14.8 |
1.50 × 10−11 |
9 |
230 |
Fulranumab |
−15.1 |
7.80 × 10−12 |
−12.0 |
1.60 × 10−9 |
−14.6 |
2.00 × 10−11 |
−13.8 |
7.70 × 10−11 |
10 |
234 |
Gedivumab |
−13.0 |
3.10 × 10−10 |
−11.7 |
2.50 × 10−9 |
−10.3 |
2.70 × 10−8 |
−08.4 |
6.40 × 10−7 |
11 |
234 |
Idarucizumab |
−13.1 |
2.40 × 10−10 |
−12.9 |
3.50 × 10−10 |
−13.9 |
6.70 × 10−11 |
−12.9 |
3.50 × 10−10 |
12 |
230 |
Ivonescimab |
−16.4 |
8.90 × 10−13 |
−18.1 |
5.40 × 10−14 |
−13.3 |
1.80 × 10−10 |
−12.4 |
8.40 × 10−10 |
13 |
229 |
Lexatumumab |
−15.7 |
2.80 × 10−12 |
−17.5 |
1.40 × 10−13 |
−15.7 |
2.90 × 10−12 |
−11.2 |
6.10 × 10−9 |
14 |
230 |
Ligelizumab |
−15.0 |
9.30 × 10−12 |
−14.5 |
2.10 × 10−11 |
−15.3 |
6.50 × 10−12 |
−13.3 |
1.60 × 10−10 |
15 |
229 |
Ofatumumab |
−13.5 |
1.30 × 10−10 |
−15.5 |
4.30 × 10−12 |
−09.8 |
6.70 × 10−8 |
−12.0 |
1.60 × 10−9 |
16 |
230 |
Ranibizumab |
−13.4 |
1.40 × 10−10 |
−12.7 |
5.00 × 10−10 |
−12.4 |
8.60 × 10−10 |
−13.1 |
2.40 × 10−10 |
17 |
238 |
Regdanvimab |
−14.1 |
4.30 × 10−11 |
−12.2 |
1.10 × 10−9 |
−11.9 |
2.00 × 10−9 |
−12.3 |
9.10 × 10−10 |
18 |
225 |
Sudubrilimab |
−11.7 |
2.70 × 10−9 |
−18.1 |
5.20 × 10−14 |
−14.6 |
1.90 × 10−11 |
−13.1 |
2.40 × 10−10 |
19 |
231 |
Tamrintamab |
−14.3 |
3.10 × 10−11 |
−14.1 |
4.40 × 10−11 |
−11.6 |
3.00 × 10−9 |
−12.1 |
1.30 × 10−9 |
20 |
230 |
Tarcocimab |
−13.4 |
1.40 × 10−10 |
−12.7 |
5.00 × 10−10 |
−15.5 |
4.50 × 10−12 |
−13.1 |
2.40 × 10−10 |
21 |
230 |
Tralokinumab |
−13.1 |
2.50 × 10−10 |
−11.4 |
4.40 × 10−9 |
−12.1 |
1.30 × 10−9 |
−12.6 |
5.80 × 10−10 |
22 |
230 |
Urelumab |
−13.5 |
1.20 × 10−10 |
−12.1 |
1.40 × 10−9 |
−12.7 |
4.60 × 10−10 |
−12.2 |
1.20 × 10−9 |
23
|
234 |
Vofatamab
|
−09.9
|
5.10 × 10
−8
|
−14.1
|
4.60 × 10
−11
|
−14.5
|
2.40 × 10
−11
|
−09.5
|
1.10 × 10
−7
|
24 |
235 |
Volagidemab |
−11.9 |
2.00 × 10−9 |
−09.7 |
7.30 × 10−8 |
−14.9 |
1.20 × 10−11 |
−10.7 |
1.30 × 10−8 |
25 |
240 |
Zinlirvimab |
−13.0 |
2.90 × 10−10 |
−12.5 |
7.00 × 10−10 |
−13.2 |
2.00 × 10−10 |
−12.3 |
9.80 × 10−10 |
3.6 Estimation of physiochemical properties
The ProtParam server was used to estimate the physical and chemical characteristics of all the mAbs with favourable ClusPro docking scores against the MPXV enzymes. For the mAbs we investigated, the instability index ranged from 31.5 (volagidemab) to 50.53 (zinlirvimab). Generally, a protein's stability index should be under 40, indicating stability; however, an index higher than 40 indicates that the protein is unstable.59,60 According to the instability index, 12 mAbs are stable and have an instability index under 40; 14 mAbs have been classified as unstable because their instability index was estimated to be greater than 40. Although the typical pI values for antibodies are around 8 and 9, the range of theoretical pI reported in this investigation was from 5.23 (eculizumab) to 9.1 (erenumab).61 According to several studies, the isoelectric point for antibodies estimated by the cIEF lies between 5.7 and 9.62 Also, the modified antibody fragments with cationic residues increase the pI by 1 unit and hence increase the plasma clearance, whereas the Fab modification through anionic residues will lower the pI by 2 units and result in decreased plasma clearance.63 The grand average of hydropathicity ranged between −0.121 and −0.429, whereas the aliphatic index for the mAbs examined ranged between 59.78 and 77.48. To choose the best monoclonal antibodies from the Thera-SAbDab, another parameter, ‘estimated status’, was considered. The mAbs with active status have been selected for additional research, whereas other mAbs with withdrawn, discontinued, and no further development status were discarded. From our screened mAbs, 14 were still in use, 6 were no longer being developed, and 2 had no further development. Finally, after examining each criterion mentioned earlier, we identified the two mAbs with the best ClusPro docking scores, binding affinities, dissociation constants, and physiochemical attributes. Eculizumab and vofatamab were the resultant mAbs. They both meet our screening criteria, are stable, and have active status. Interestingly, these selected mAbs, eculizumab and vofatamab, have lower pI values of 5.23 and 5.86, respectively, which may also decrease the clearance of mAbs from the blood plasma (Tables 3–5).
Table 5 Several significant physiochemical parameters of therapeutic mAbs evaluated using the ProtPartam server; A = active, D = discontinued, NFD = no further development
S. no. |
Therapeutic mAbs |
Estimated status |
Physiochemical properties (ProtParam server) |
pI |
Estimated half-life |
Instability index |
Aliphatic index |
GRAVY |
Stability |
Mammalian reticulocytes |
Yeast (min) |
E. coli (h) |
1 |
Abelacimab |
A |
7.77 |
0.8 hours |
10 |
10 |
40.92 |
68.92 |
−0.282 |
Unstable |
2 |
Afasevikumab |
D |
8.38 |
1.0 hours |
30 |
>10 |
49.46 |
72.25 |
−0.258 |
Unstable |
3 |
Apitegromab |
A |
6.99 |
0.8 hours |
10 |
10 |
43.83 |
71.32 |
−0.294 |
Unstable |
4 |
Bevacizumab |
A |
7.06 |
1.0 hours |
30 |
>10 |
36.43 |
61.87 |
−0.331 |
Stable |
5 |
Carlumab |
D |
5.72 |
0.8 hours |
10 |
10 |
41.94 |
73.6 |
−0.168 |
Unstable |
6
|
Eculizumab
|
A
|
5.23
|
0.8 hours
|
10
|
10
|
31.78
|
68.08
|
−0.276
|
Stable
|
7 |
Erenumab |
NFD |
9.1 |
0.8 hours |
10 |
10 |
43.75 |
70.21 |
−0.264 |
Unstable |
8 |
Erlizumab |
D |
8.34 |
1.0 hours |
30 |
>10 |
36.31 |
62.86 |
−0.429 |
Stable |
9 |
Fulranumab |
D |
7.87 |
1.0 hours |
30 |
>10 |
48.83 |
73.35 |
−0.215 |
Unstable |
10 |
Gedivumab |
D |
8.64 |
1.0 hours |
30 |
>10 |
42.75 |
77.48 |
−0.169 |
Unstable |
11 |
Idarucizumab |
NFD |
8.58 |
0.8 hours |
10 |
10 |
41.24 |
79.91 |
−0.121 |
Unstable |
12 |
Ivonescimab |
A |
7.06 |
1.0 hours |
30 |
>10 |
36.43 |
61.87 |
−0.331 |
Stable |
13 |
Lexatumumab |
D |
7.87 |
1.0 hours |
30 |
>10 |
36.90 |
69.39 |
−0.348 |
Stable |
14 |
Ligelizumab |
A |
5.64 |
0.8 hours |
10 |
10 |
45.73 |
59.78 |
−0.313 |
Unstable |
15 |
Ofatumumab |
A |
8.35 |
1.0 hours |
30 |
>10 |
44.06 |
72.45 |
−0.312 |
Unstable |
16 |
Ranibizumab |
A |
6.34 |
1.0 hours |
30 |
>10 |
37.07 |
63.57 |
−0.325 |
Stable |
17 |
Regdanvimab |
A |
8.3 |
0.8 hours |
10 |
10 |
26.33 |
74.92 |
−0.268 |
Stable |
18 |
Sudubrilimab |
A |
7.88 |
1.0 hours |
30 |
>10 |
40.69 |
66.76 |
−0.200 |
Unstable |
19 |
Tamrintamab |
A |
6.36 |
0.8 hours |
10 |
10 |
47.06 |
67.58 |
−0.350 |
Unstable |
20 |
Tarcocimab |
A |
6.34 |
1.0 hours |
30 |
>10 |
37.07 |
63.57 |
−0.325 |
Stable |
21 |
Tralokinumab |
A |
5.86 |
0.8 hours |
10 |
10 |
32.27 |
66.91 |
−0.327 |
Stable |
22 |
Urelumab |
A |
5.89 |
0.8 hours |
10 |
10 |
37.63 |
74.22 |
−0.274 |
Stable |
23
|
Vofatamab
|
A
|
5.86
|
1.0 hours
|
30
|
>10
|
30.44
|
67.91
|
−0.262
|
Stable
|
24 |
Volagidemab |
A |
8.8 |
0.8 hours |
10 |
10 |
50.53 |
68.38 |
−0.410 |
Unstable |
25 |
Zinlirvimab |
A |
8.64 |
0.8 hours |
10 |
10 |
31.50 |
71.46 |
−0.340 |
Stable |
3.7 Mutant library preparation and evaluation of the binding affinities
The CUPSAT webserver predicted a stabilized and favourable residual mutation in eculizumab and vofatamab. In search of novel mAbs with higher binding affinities and docking scores, the protein mutagenesis wizard tool of PyMol was employed to generate the mutant pool. The CUPSAT produced many datasets for stabilizing and favourable mutants; as a result, the predicted ΔΔG score threshold was adjusted to 0.2 kcal mol−1. Under this limit, five mutants were listed from both mAbs and through permutation combinations, up to triple mutant libraries were prepared (n = 25 for each mAb) (Table S3†).
The mutant library was then screened using the ClusPro web server against the targets: thymidine kinase, methyltransferase, D9 decapping enzyme, and RNA polymerase. The mAbs, eculizumab and vofatamab, were designated positive controls for their respective mutant libraries. The eculizumab docking scores for thymidine kinase, methyltransferase, D9 decapping enzyme, and RNA polymerase were −1980.8, −1703.2, −1668.8, and −1833.8 kcal mol−1, respectively. The eculizumab mutant, L: L92F has a docking score of −2057.3, −1758.7, −1579.7, and −1866.0 kcal mol−1 for thymidine kinase, methyltransferase, D9 decapping enzyme, and RNA polymerase, respectively.
Furthermore, the docking scores for vofatamab against the targets thymidine kinase, methyltransferase, D9 decapping enzyme, and RNA polymerase were −2058.8, −1843.2, −2229.2, and −2008.4 kcal mol−1, respectively. However, the vofatamab mutant, L: H94T, L: Q96V, has an excellent docking score of −2048.5, −1851.8, −2249.5, and −2016.3 kcal mol−1 for thymidine kinase, methyltransferase, D9 decapping enzyme, and RNA polymerase, respectively. There were many more mutants with docking scores relatively higher than the positive control for single or double enzymes (targets), but none could target all four targets with considerably higher docking scores than the positive control (Table 6).
Table 6 The top five best mutant mAbs with high ClusPro docking scores
S. no. |
MPXV essential enzymes |
ClusPro docking score (kcal mol−1) |
Mutant library |
Thymidine kinase |
Methyltransferase |
D9 decapping enzyme |
RNA polymerase |
1
|
Eculizumab (positive control)
|
−1980.8
|
−1703.2
|
−1668.8
|
−1833.8
|
2 |
H: R98L |
−1990.9 |
−1729.4 |
−1672.7 |
−1819.7 |
3
|
L: L92F
|
−2057.3
|
−1758.7
|
−1579.7
|
−1866.0
|
4 |
H: R98L, H: F101L |
−2057.3 |
−1635.2 |
−1540.6 |
−1781.8 |
5 |
L: L92F, H: R98L |
−2022.8 |
−1698.6 |
−1644.5 |
−1876.1 |
6 |
H: R98L, H: F101L, L: L92F |
−2007.2 |
−1703.1 |
−1590.2 |
−1831.0 |
|
1
|
Vofatamab (positive control)
|
−2058.8
|
−1843.2
|
−2229.2
|
−2008.4
|
2 |
L: Q90A |
−2051.5 |
−1843.0 |
−2249.1 |
−2008.6 |
3 |
L: H94T |
−2044.2 |
−1844.2 |
−2267.4 |
−2012.4 |
4 |
L: Q90A, L: Q96V |
−2046.9 |
−1846.2 |
−2268.2 |
−2018.0 |
5 |
L: Q90A, L: H94T |
−2038.1 |
−1843.5 |
−2309.1 |
−2019.3 |
6
|
L: H94T, L: Q96V
|
−2048.5
|
−1851.8
|
−2249.5
|
−2016.3
|
3.8 Molecular dynamics simulations to validate in silico enzymatic inhibition
The MD simulation provides the behaviour of biomolecules in the virtual physiological environment for the MPXV enzymes thymidine kinase, methyltransferase, D9 decapping enzyme, and RNA polymerase in association with the mAbs eculizumab and vofatamab. The MD simulation of the eculizumab mutant (L: L92F) and vofatamab mutant (L: H94T, L: Q96V) was also performed while eculizumab and vofatamab were considered as positive controls for their mutants. The MD simulation was conducted in triplicate, and the results were compared to confirm our findings. GraphPad Prism 9 was used to plot the RMSD, RMSF, Rg, SASA, and hydrogen bonding using output files in the xvg format. Upon data extraction, data of three replicates for each dataset were placed in adjacent sub-columns in the Y-axis and plotted by applying only the mean values for datasets.64,65
The RMSD of the parental mAbs and their mutants associated with the MPXV enzymes was calculated to ensure structural changes during the MD simulation. The initially provided structures for MD simulation were used as reference structures, and the distance was calculated by superimposing the atomic coordinates of simulated structures onto reference structures.66 With thymidine kinase, methyltransferase, and D9 decapping enzyme, the average RMSD scores for the mAbs and their mutants were under 0.40 nm, whereas the average RMSD scores for the mAbs with RNA polymerase were between 0.53 nm and 0.67 nm. Moderately mutant mAbs were more stable than the parent mAbs regarding the RMSD (Fig. 3).
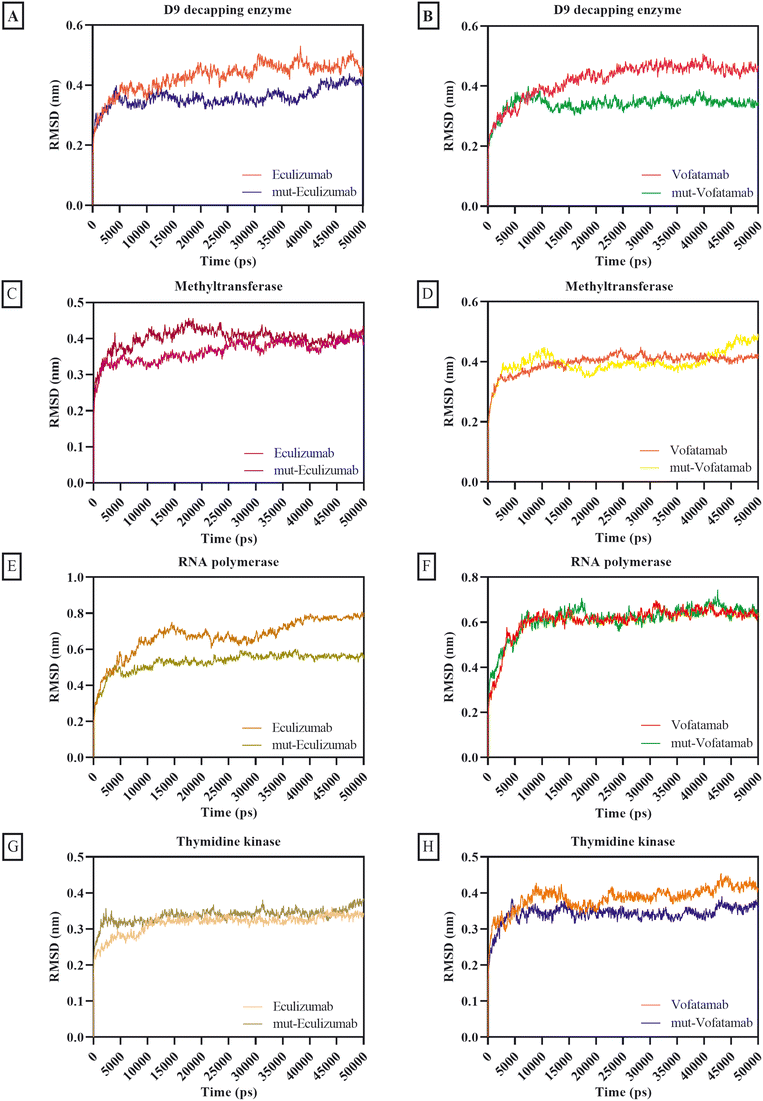 |
| Fig. 3 RMSD plots of D9 decapping enzyme with (A) eculizumab and eculizumab mutant (L: L92F), (B) vofatamab mutant (L: H94T, L: Q96V); methyltransferase with (C) eculizumab and eculizumab mutant (L: L92F), (D) vofatamab mutant (L: H94T, L: Q96V); RNA polymerase with (E) eculizumab and eculizumab mutant (L: L92F), (F) vofatamab mutant (L: H94T, L: Q96V), and thymidine kinase with (G) eculizumab and eculizumab mutant (L: L92F), (H) vofatamab mutant (L: H94T, L: Q96V). | |
Although RMS fluctuation and RMSD have different interpretations, both are virtually equivalent in numerical calculations in biomolecular simulation because calculations involve similar mathematical procedures. RMSD displays positional changes within the overall structure during simulations, while RMS fluctuation quantifies the average deviation of individual residues from their mean position. RMS fluctuation and RMSD play an essential role in determining the stability of complexes.67,68 The average RMS fluctuations for each of the complexes in this investigation ranged from 0.68 nm to 0.18 nm. However, it was fascinating to observe that both mutant mAbs were more stable in combination with the vital MPXV enzymes than the mAbs eculizumab and vofatamab (Fig. 4).
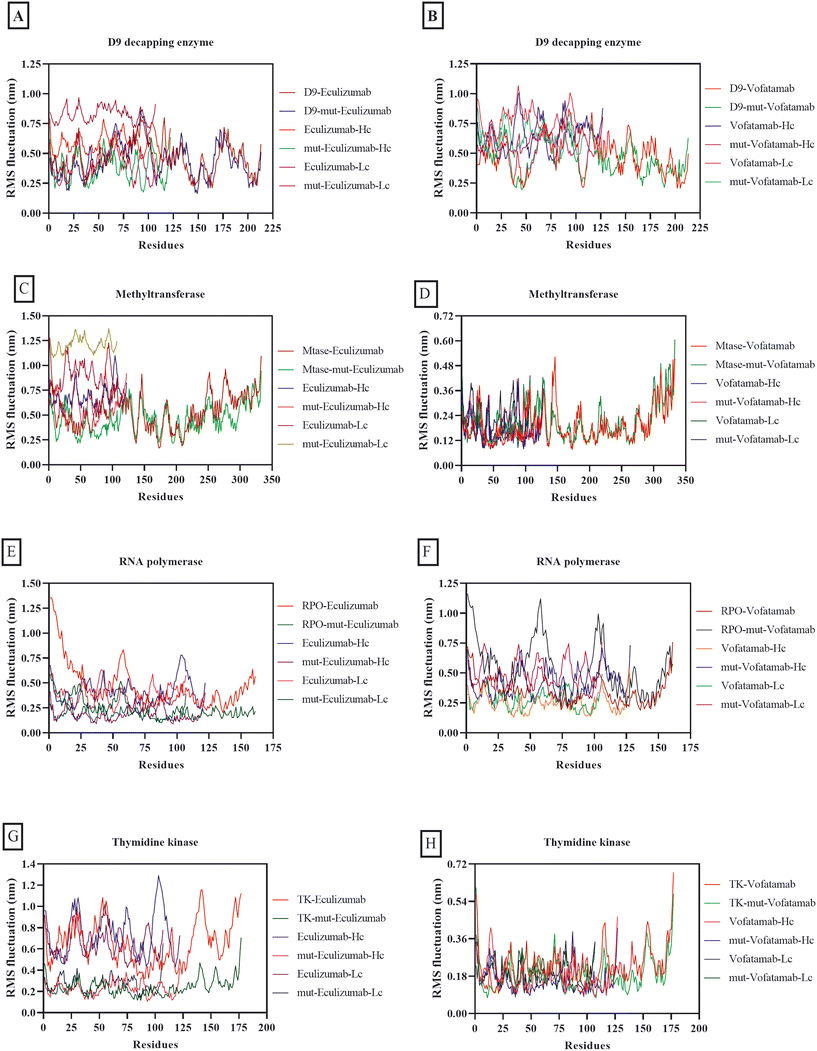 |
| Fig. 4 RMS fluctuation plots of the D9 decapping enzyme with (A) eculizumab and eculizumab mutant (L: L92F), (B) vofatamab mutant (L: H94T, L: Q96V); methyltransferase with (C) eculizumab and eculizumab mutant (L: L92F), (D) vofatamab mutant (L: H94T, L: Q96V); RNA polymerase with (E) eculizumab and eculizumab mutant (L: L92F), (F) vofatamab mutant (L: H94T, L: Q96V), and thymidine kinase with (G) eculizumab and eculizumab mutant (L: L92F), (H) vofatamab mutant (L: H94T, L: Q96V). | |
The atom dispersion around the protein axis was determined during dynamics using the radius of gyration.69 The behaviour of all complexes was satisfactory based on the average Rg calculations, while the D9 decapping enzyme complexes exhibit somewhat unstable behaviour. Additionally, the Rg of the mutant mAbs was superior to that of the native mAbs (Fig. 5).
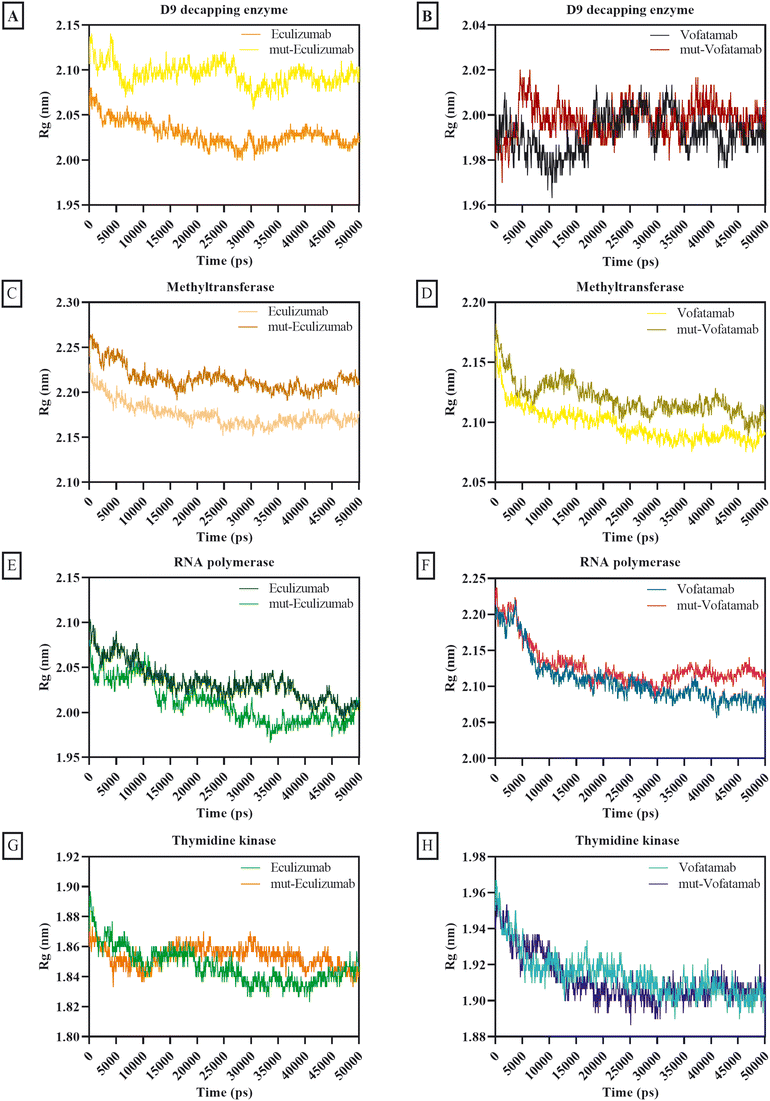 |
| Fig. 5 Radius of gyration plots of the D9 decapping enzyme with (A) eculizumab and eculizumab mutant (L: L92F), (B) vofatamab mutant (L: H94T, L: Q96V); methyltransferase with (C) eculizumab and eculizumab mutant (L: L92F), (D) vofatamab mutant (L: H94T, L: Q96V); RNA polymerase with (E) eculizumab and eculizumab mutant (L: L92F), (F) vofatamab mutant (L: H94T, L: Q96V), and thymidine kinase with (G) eculizumab and eculizumab mutant (L: L92F), (H) vofatamab mutant (L: H94T, L: Q96V). | |
The solvent's accessibility to the surface area of the atoms involved in the mAb and protein complex was verified by the SAS area. In addition to how hydrophobic and hydrophilic residues interact with the solvent, surface tension around the complex and solvent influences the SASA value.70 Except for the complexes with methyltransferase (SAS area ranges between 233.85 nm2 and 239.67 nm2), the average SAS area for the mAbs and mutant mAbs with MPXV enzymes was less than 197.83 nm2. Overall, the findings of the different mAbs for the SAS area exhibit remarkable consistency, but minimal fluctuations are also observed in some mAbs (Fig. 6).
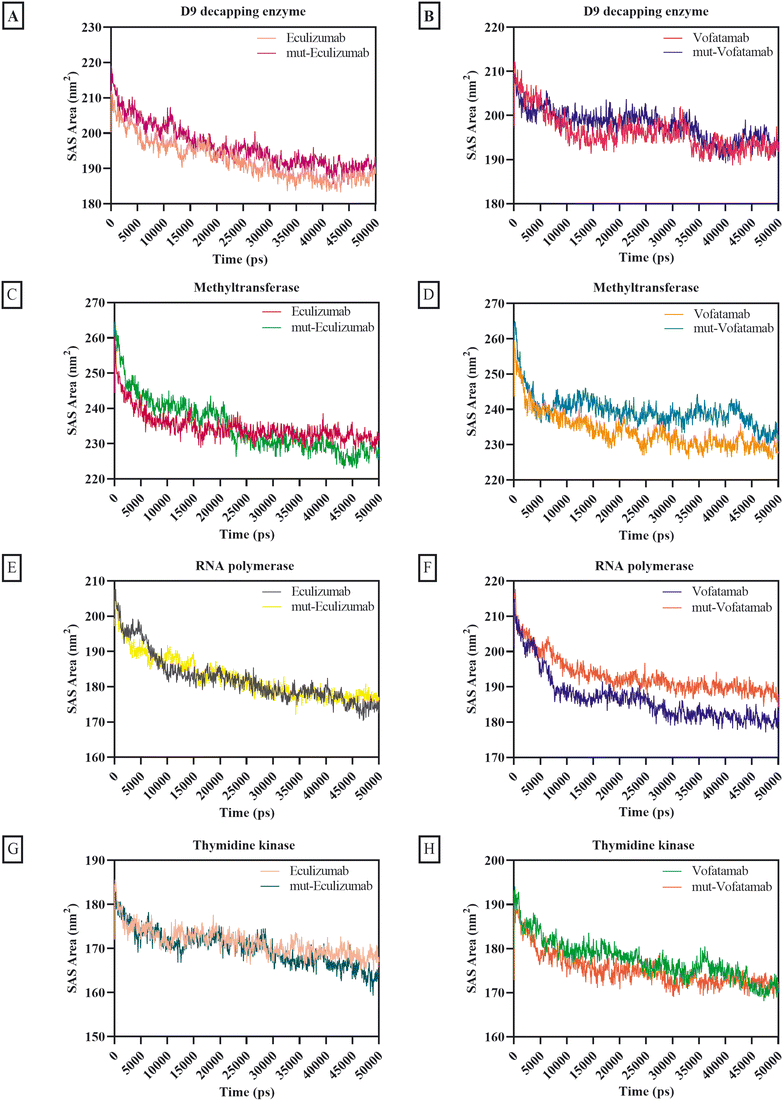 |
| Fig. 6 SAS area plots of D9 decapping enzyme with (A) eculizumab and eculizumab mutant (L: L92F), (B) vofatamab mutant (L: H94T, L: Q96V); methyltransferase with (C) eculizumab and eculizumab mutant (L: L92F), (D) vofatamab mutant (L: H94T, L: Q96V); RNA polymerase with (E) eculizumab and eculizumab mutant (L: L92F), (F) vofatamab mutant (L: H94T, L: Q96V), and thymidine kinase with (G) eculizumab and eculizumab mutant (L: L92F), (H) vofatamab mutant (L: H94T, L: Q96V). | |
One of the most crucial interactions in terms of stability is hydrogen bonding (between 5 and 30 kJ per mole); in this case, the hydrogen atom is attached to the more electronegative donor atom.65,71 Hydrogen bonding interactions sustain the complex and can impact the structure's dynamic behaviour. Since hydrogen bonds can occur between complementary residues, they also play a role in protein–protein recognition and binding specificity. The quantity and strength of hydrogen bonds between protein–protein complexes affect the stability. Intense and frequent hydrogen bonds can help the complex resist conformational changes and disturbances and prevent the complex from dissociating or suffering from significant structural changes.72,73
The usual number of hydrogen bonds formed by all the mAbs with RNA polymerase and thymidine kinase ranged from 1004.2 to 1120.25, whereas the average number of hydrogen bonds formed by all the mAbs with methyltransferase and D9 decapping enzyme was relatively high (between 1201.57 and 1584.89) (Fig. 7).
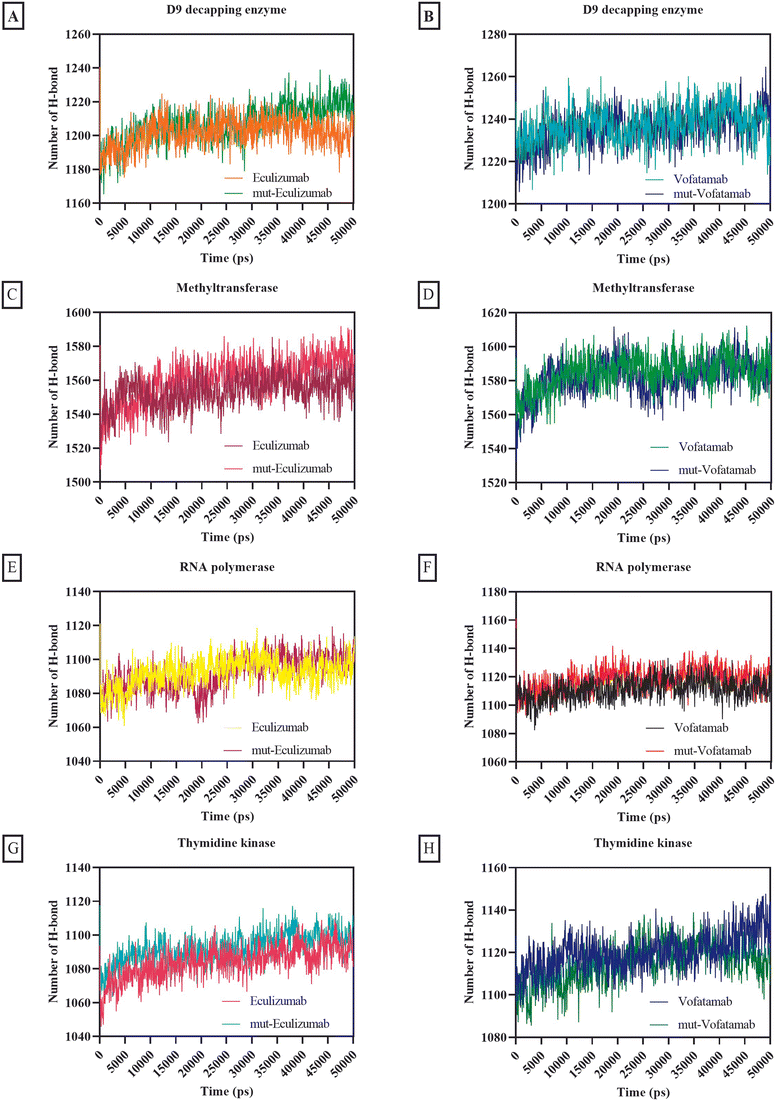 |
| Fig. 7 Graphical representation of formation and breakage of hydrogen bonds during the MD simulation of the D9 decapping enzyme with (A) eculizumab and eculizumab mutant (L: L92F), (B) vofatamab mutant (L: H94T, L: Q96V); methyltransferase with (C) eculizumab and eculizumab mutant (L: L92F), (D) vofatamab mutant (L: H94T, L: Q96V); RNA polymerase with (E) eculizumab and eculizumab mutant (L: L92F), (F) vofatamab mutant (L: H94T, L: Q96V), and thymidine kinase with (G) eculizumab and eculizumab mutant (L: L92F), (H) vofatamab mutant (L: H94T, L: Q96V). | |
Certain MD plots did not achieve stability during the 50 ns molecular dynamics simulation. To overcome this issue, we used a triple dynamics strategy, conducting three different simulations and averaging the results from these MD simulations to generate the plots. This method minimizes the influence of any temporary fluctuations or artefacts encountered in individual simulations, resulting in a more trustworthy depiction for the behaviour of the system.
4. Conclusion
Antibody engineering has significantly evolved since the US FDA approved the first therapeutic mAb in 1986. Because of their greater specificity and fewer side effects, mAbs have recently taken over as the most used class of medication. This study offers an excellent framework for therapeutic mAb engineering that will enhance the effectiveness and development of new therapeutic mAbs. Here, the sequences for the key enzymes of MPXV were first extracted and then the mAbs from the Thera-SAbDab.
The library is screened using the ClusPro web server, and the PRODIGY web server supports binding affinity predictions and the dissociation constant. Two mAbs (eculizumab and vofatamab) were found to be the top-performing mAbs from the Thera-SAbDab across a variety of screening methods. Using an antibody engineering tool and a mutational immunotherapeutic approach, novel mAbs were designed. The observations of molecular dynamics simulations demonstrate that the most effective and promising inhibitors were the mAbs eculizumab mutant (L: L92F) and vofatamab mutant (L: H94T, L: Q96V) (Fig. 8).
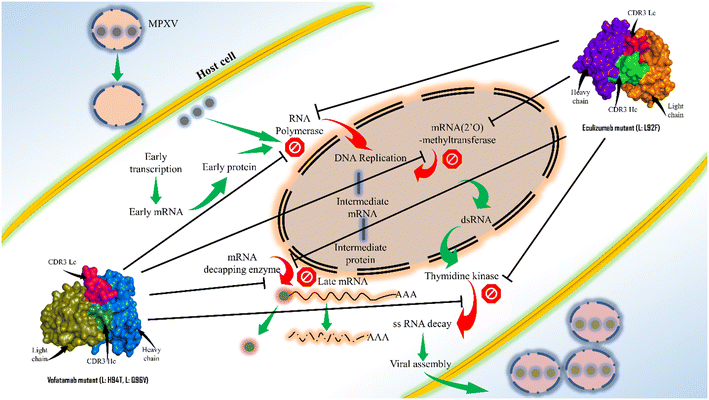 |
| Fig. 8 Concluding illustration of the proposed mAbs targeting the essential MPXV enzymes involved in the MPXV replication. | |
Data availability
The data used to support the findings of this study are included in the article.
Ethics approval
This is an observational study; no ethical approval is required.
Author contributions
Satyendra Singh: conceptualization, data curation, software analysis, methodology, writing – original draft, review & editing, and validation. Abhishek Rao: writing – original draft. Anshuman Mishra: writing – original draft. Amit Mishra: writing – original draft. Vijay Kumar Prajapati: conceptualization, supervision, writing – original draft, and finalizing the draft.
Conflicts of interest
The authors have declared no competing interests.
Acknowledgements
SS is thankful to UGC and the Central University of Rajasthan for providing a university fellowship. VKP is thankful to the Central University of Rajasthan for providing a computational facility.
References
-
European Center for Disease Control, Epidemiological update: monkeypox outbreak, Available at: https://www.ecdc.europa.eu/en/news-events/epidemiological-update-monkeypox-outbreak, Accessed May 31 2022 Search PubMed.
-
World Health Organization, Multi-country monkeypox outbreak: situation update, Available at: https://www.who.int/emergencies/disease-outbreak-news/item/2022-DON392, Accessed June 15 2022 Search PubMed.
- P. Magnus, E. K. Andersen, K. B. Petersen and A. Birch-Andersen, A pox-like disease in cynomolgus monkeys, Acta Pathol. Microbiol. Scand., 1959, 46(2), 156–176 CrossRef.
- J. G. Breman, R. Kalisa, M. V. Steniowski, E. Zanotto, A. I. Gromyko and I. Arita, Human monkeypox, 1970-79, Bull. W. H. O., 1980, 58(2), 165–182 CAS.
- J. Kaler, A. Hussain, G. Flores, S. Kheiri and D. J. C. Desrosiers, Monkeypox: a comprehensive review of transmission, pathogenesis, and manifestation, Cureus, 2022, 14(7), e26531 Search PubMed.
- Mpox Outbreak Global Map, Accessed: January 11, 2023, https://www.cdc.gov/poxvirus/monkeypox/response/2022/world-map.html, 2023.
- G. P. Oliveira, R. A. L. Rodrigues, M. T. Lima, B. P. Drumond and J. S. Abrahão, Poxvirus Host Range Genes and Virus-Host Spectrum: A Critical Review, Viruses, 2017, 9(11), 331 CrossRef PubMed.
- E. Petersen, A. Kantele, M. Koopmans, D. Asogun, A. Yinka-Ogunleye and C. Ihekweazu,
et al., Human Monkeypox: Epidemiologic and Clinical Characteristics, Diagnosis, and Prevention, Infect. Dis. Clin. North Am., 2019, 33(4), 1027–1043 CrossRef PubMed.
- S. Singh, A. Rao, K. Kumar, A. Mishra and V. K. Prajapati, Translational vaccinomics and structural filtration algorithm to device multiepitope vaccine for catastrophic monkeypox virus, Comput. Biol. Med., 2023, 153, 106497 CrossRef CAS PubMed.
- K. Sharma, S. Akre, S. Chakole and M. B. Wanjari, Monkeypox: An Emerging Disease, Cureus, 2022, 14(9), e29393 Search PubMed.
- E. Alakunle, U. Moens, G. Nchinda and M. I. Okeke, Monkeypox Virus in Nigeria: Infection Biology, Epidemiology, and Evolution, Viruses, 2020, 12(11), 1257 CrossRef CAS PubMed.
- E. Petersen, A. Kantele, M. Koopmans, D. Asogun, A. Yinka-Ogunleye and C. Ihekweazu,
et al., Human monkeypox: epidemiologic and clinical characteristics, diagnosis, and prevention, Infect. Dis. Clin. North Am., 2019, 33(4), 1027–1043 CrossRef PubMed.
- E. Hammarlund, M. W. Lewis, S. V. Carter, I. Amanna, S. G. Hansen and L. I. Strelow,
et al., Multiple diagnostic techniques identify previously vaccinated individuals with protective immunity against monkeypox, Nat. Med., 2005, 11(9), 1005–1011 CrossRef CAS PubMed.
- M. G. Reynolds, A. M. McCollum, B. Nguete, R. Shongo Lushima and B. W. Petersen, Improving the Care and Treatment of Monkeypox Patients in Low-Resource Settings: Applying Evidence from Contemporary Biomedical and Smallpox Biodefense Research, Viruses, 2017, 9(12), 380 CrossRef PubMed.
- J. G. Rizk, G. Lippi, B. M. Henry, D. N. Forthal and Y. Rizk, Prevention and Treatment of Monkeypox, Drugs, 2022, 82(9), 957–963 CrossRef CAS PubMed.
- R.-M. Lu, Y.-C. Hwang, I. J. Liu, C.-C. Lee, H.-Z. Tsai and H.-J. Li,
et al., Development of therapeutic antibodies for the treatment of diseases, J. Biomed. Sci., 2020, 27(1), 1 CrossRef CAS PubMed.
- L. Tan, J. Yao, L. Lei, K. Xu, F. Liao and S. Yang,
et al., Emergence of a Novel Recombinant Pseudorabies Virus Derived From the Field Virus and Its Attenuated Vaccine in China, Front. Vet. Sci., 2022, 9, 872002 CrossRef PubMed.
- S. Dong, J. Sun, Z. Mao, L. Wang, Y. L. Lu and J. Li, A guideline for homology modeling of the proteins from newly discovered betacoronavirus, 2019 novel coronavirus (2019-nCoV), J. Med. Virol., 2020, 92(9), 1542–1548 CrossRef CAS PubMed.
- A. K. Rajendran, D. Sankar, S. Amirthalingam, H. D. Kim, J. Rangasamy and N. S. Hwang, Trends in mechanobiology guided tissue engineering and tools to study cell-substrate interactions: a brief review, Biomater. Res., 2023, 27(1), 55 CrossRef CAS PubMed.
- C. Dequeker, Y. Mohseni Behbahani, L. David, E. Laine and A. Carbone, From complete cross-docking to partners identification and binding sites predictions, PLoS Comput. Biol., 2022, 18(1), e1009825 CrossRef CAS PubMed.
- A. Singh, T. Dauzhenka, P. J. Kundrotas, M. J. Sternberg and I. A. Vakser, Application of docking methodologies to modeled proteins, Proteins: Struct., Funct., Bioinf., 2020, 88(9), 1180–1188 CrossRef CAS PubMed.
- V. K. Vyas, R. D. Ukawala, M. Ghate and C. Chintha, Homology modeling a fast tool for drug discovery: current perspectives, Indian J. Pharm. Sci., 2012, 74(1), 1–17 CrossRef CAS PubMed.
- M. Batool, B. Ahmad and S. Choi, A Structure-Based Drug Discovery Paradigm, Int. J. Mol. Sci., 2019, 20(11), 2783 CrossRef CAS PubMed.
- D. M. Mason, S. Friedensohn, C. R. Weber, C. Jordi, B. Wagner and S. M. Meng,
et al., Optimization of therapeutic antibodies by predicting antigen specificity from antibody sequence via deep learning, Nat. Biomed. Eng., 2021, 5(6), 600–612 CrossRef CAS PubMed.
- A. A. Rabaan, A. H. Abas, T. E. Tallei, M. A. Al-Zaher, N. M. Al-Sheef, Fatimawali, E. Z. Al-Nass, E. A. Al-Ebrahim, Y. Effendi, R. Idroes and M. F. Alhabib,
et al., Monkeypox outbreak 2022: What we know so far and its potential drug targets and management strategies, J. Med. Virol., 2023, 95(1), e28306 CrossRef CAS PubMed.
- D. A. Steinhauer, E. Domingo and J. J. Holland, Lack of evidence for proofreading mechanisms associated with an RNA virus polymerase, Gene, 1992, 122(2), 281–288 CrossRef CAS PubMed.
- K. H. Choi, Viral polymerases, Adv. Exp. Med. Biol., 2012, 726, 267–304 CrossRef CAS PubMed.
- J. K. Peters, R. W. Tibble, M. Warminski, J. Jemielity and J. D. Gross, Structure of the poxvirus decapping enzyme D9 reveals its mechanism of cap recognition and catalysis, Structure, 2022, 30(5), 721–732.e4 CrossRef CAS PubMed.
- Q. Cui, W. S. Shin, Y. Luo, J. Tian, H. Cui and D. Yin, Thymidylate kinase: an old topic brings new perspectives, Curr. Med. Chem., 2013, 20(10), 1286–1305 CrossRef CAS PubMed.
- D. Topalis, B. Collinet, C. Gasse, L. Dugué, J. Balzarini and S. Pochet,
et al., Substrate specificity of vaccinia virus thymidylate kinase, FEBS J., 2005, 272(24), 6254–6265 CrossRef CAS PubMed.
- J. L. Hyde and M. S. Diamond, Innate immune restriction and antagonism of viral RNA lacking 2′-O methylation, Virology, 2015, 479–480, 66–74 CrossRef CAS PubMed.
- C. M. Byrd, T. C. Bolken, A. M. Mjalli, M. N. Arimilli, R. C. Andrews and R. Rothlein,
et al., New class of orthopoxvirus antiviral drugs that block viral maturation, J. Virol., 2004, 78(22), 12147–12156 CrossRef CAS PubMed.
- M. I. J. Raybould, C. Marks, A. P. Lewis, J. Shi, A. Bujotzek and B. Taddese,
et al., Thera-SAbDab: the Therapeutic Structural Antibody Database, Nucleic Acids Res., 2019, 48(D1), D383–D388 CrossRef PubMed.
- J. Yang and Y. Zhang, I-TASSER server: new development for protein structure and function predictions, Nucleic Acids Res., 2015, 43(W1), W174–W181 CrossRef CAS PubMed.
- L. Heo, H. Park and C. Seok, GalaxyRefine: Protein structure refinement driven by side-chain repacking, Nucleic Acids Res., 2013, 41(Web Server issue), W384–W388 CrossRef PubMed.
- B. Abanades, G. Georges, A. Bujotzek and C. M. Deane, ABlooper: fast accurate antibody CDR loop structure prediction with accuracy estimation, Bioinformatics, 2022, 38(7), 1877–1880 CrossRef CAS PubMed.
- L. Li, S. Chen, Z. Miao, Y. Liu, X. Liu and Z. X. Xiao,
et al., AbRSA: a robust tool for antibody numbering, Protein Sci., 2019, 28(8), 1524–1531 CrossRef CAS PubMed.
- E. Katchalski-Katzir, I. Shariv, M. Eisenstein, A. A. Friesem, C. Aflalo and I. A. Vakser, Molecular surface recognition: determination of geometric fit between proteins and their ligands by correlation techniques, Proc. Natl. Acad. Sci. U. S. A., 1992, 89(6), 2195–2199 CrossRef CAS PubMed.
- D. Kozakov, D. R. Hall, B. Xia, K. A. Porter, D. Padhorny and C. Yueh,
et al., The ClusPro web server for protein–protein docking, Nat. Protoc., 2017, 12(2), 255–278 CrossRef CAS PubMed.
- I. T. Desta, K. A. Porter, B. Xia, D. Kozakov and S. Vajda, Performance and Its Limits in Rigid Body Protein-Protein Docking, Structure, 2020, 28(9), 1071–1081.e3 CrossRef CAS PubMed.
- L. C. Xue, J. P. Rodrigues, P. L. Kastritis, A. M. Bonvin and A. Vangone, PRODIGY: a web server for predicting the binding affinity of protein–protein complexes, Bioinformatics, 2016, 32(23), 3676–3678 CrossRef CAS PubMed.
- M. Panda, E. Kalita, S. Singh, K. Kumar, A. Rao and V. K. Prajapati, MiRNA-SARS-CoV-2 dialogue and prospective anti-COVID-19 therapies, Life Sci., 2022, 120761 CrossRef CAS PubMed.
-
J. M. Walker, The proteomics protocols handbook, Springer, 2005 Search PubMed.
- P. Chouhan, S. Singh, V. Sharma and V. K. Prajapati, Anti-IL-10 Antibody Humanization by SDR Grafting with Enhanced Affinity to Neutralize the Adverse Response of Interleukin-10, Int. J. Pept. Res. Ther., 2022, 28(5), 1–14 Search PubMed.
- S. M. Danilov, M. S. Wade, S. L. Schwager, R. G. Douglas, A. B. Nesterovitch and I. A. Popova,
et al., A novel angiotensin I-converting enzyme mutation (S333W) impairs N-domain enzymatic cleavage of the anti-fibrotic peptide, AcSDKP, PLoS One, 2014, 9(2), e88001 CrossRef PubMed.
- M. J. Abraham, T. Murtola, R. Schulz, S. Páll, J. C. Smith and B. Hess,
et al., GROMACS: High performance molecular simulations through multi-level parallelism from laptops to supercomputers, SoftwareX, 2015, 1-2, 19–25 CrossRef.
- B. Naik, N. Gupta, R. Ojha, S. Singh, V. K. Prajapati and D. J. Prusty, High throughput virtual screening reveals SARS-CoV-2 multi-target binding natural compounds to lead instant therapy for COVID-19 treatment, Int. J. Biol. Macromol., 2020, 160, 1–17 CrossRef CAS PubMed.
- L. Pol-Fachin, C. L. Fernandes and H. Verli, GROMOS96 43a1 performance on the characterization of glycoprotein conformational ensembles through molecular dynamics simulations, Carbohydr. Res., 2009, 344(4), 491–500 CrossRef CAS PubMed.
- B. J. Jaidhan, P. S. Rao and A. Apparao, Energy minimization and conformation analysis of molecules using steepest descent method, Int. J. Comput. Sci. Inf. Technol., 2014, 5(3), 3525–3528 Search PubMed.
- B. Naik, V. S. K. Mattaparthi, N. Gupta, R. Ojha, P. Das, S. Singh, V. K. Prajapati and D. Prusty, Chemical system biology approach to identify multi-targeting FDA inhibitors for treating COVID-19 and associated health complications, J. Biomol. Struct. Dyn., 2021, 1–25 Search PubMed.
- D. A. Benson, M. Cavanaugh, K. Clark, I. Karsch-Mizrachi, D. J. Lipman and J. Ostell,
et al., GenBank, Nucleic Acids Res., 2012, 41(D1), D36–D42 CrossRef PubMed.
- H. N. Altayb, Fludarabine, a Potential DNA-Dependent RNA Polymerase Inhibitor, as a Prospective Drug against Monkeypox Virus: A Computational Approach, Pharmaceuticals, 2022, 15(9), 1129 CrossRef CAS PubMed.
- J. K. Peters, R. W. Tibble, M. Warminski, J. Jemielity and J. D. Gross, Structure of the poxvirus decapping enzyme D9 reveals its mechanism of cap recognition and catalysis, Structure, 2022, 30(5), 721–732.e4 CrossRef CAS PubMed.
- K. El Omari, N. Solaroli, A. Karlsson, J. Balzarini and D. K. Stammers, Structure of vaccinia virus thymidine kinase in complex with dTTP: insights for drug design, BMC Struct. Biol., 2006, 6(1), 22 CrossRef PubMed.
- P.-Y. Colin and P. A. Dalby, Functional and computational identification of a rescue mutation near the active site of an mRNA methyltransferase, Sci. Rep., 2020, 10(1), 21841 CrossRef CAS PubMed.
- S. Singh and V. K. Prajapati, Exploring actinomycetes natural products to identify potential multi-target inhibitors against Leishmania donovani, 3 Biotech, 2022, 12(9), 1–22 Search PubMed.
- P. L. Kastritis, J. P. G. L. M. Rodrigues, G. E. Folkers, R. Boelens and A. M. J. J. Bonvin, Proteins Feel More Than They See: Fine-Tuning of Binding Affinity by Properties of the Non-Interacting Surface, J. Mol. Biol., 2014, 426(14), 2632–2652 CrossRef CAS PubMed.
- A. Vangone and A. M. J. J. Bonvin, Contacts-based prediction of binding affinity in protein–protein complexes, eLife, 2015, 4, e07454 CrossRef PubMed.
- S. Enany, Structural and functional analysis of hypothetical and conserved proteins of Clostridium tetani, J. Infect. Public Health., 2014, 7(4), 296–307 CrossRef PubMed.
- K. Guruprasad, B. V. B. Reddy and M. W. Pandit, Correlation between stability of a protein and its dipeptide composition: a novel approach for predicting in vivo stability of a protein from its primary sequence, Protein Eng., Des. Sel., 1990, 4(2), 155–161 CrossRef CAS PubMed.
- C. A. Boswell, D. B. Tesar, K. Mukhyala, F.-P. Theil, P. J. Fielder and L. A. Khawli, Effects of charge on antibody tissue distribution and pharmacokinetics, Bioconjugate Chem., 2010, 21(12), 2153–2163 CrossRef CAS PubMed.
- Y. Tang, P. Cain, V. Anguiano, J. J. Shih, Q. Chai and Y. Feng, Impact of IgG subclass on molecular properties of monoclonal antibodies, mAbs, 2021, 13(1), 1993768 CrossRef PubMed.
- H. Kobayashi, N. Le, I.-s. Kim, M.-K. Kim, J.-E. Pie and D. Drumm,
et al., The pharmacokinetic characteristics of glycolated humanized anti-Tac Fabs are determined by their isoelectric points, Cancer Res., 1999, 59(2), 422–430 CAS.
- S. Singh, K. Kumar, M. Panda, A. Srivastava, A. Mishra and V. K. Prajapati, High-throughput virtual screening of small-molecule inhibitors targeting immune cell checkpoints to discover new immunotherapeutics for human diseases, Mol. Diversity, 2022, 1–23 Search PubMed.
-
V. Sharma, S. Singh, T. S. Ratnakar and V. K. Prajapati, Immunoinformatics and reverse vaccinology methods to design peptide-based vaccines, Advances in Protein Molecular and Structural Biology Methods, Elsevier, 2022, pp. 477–487 Search PubMed.
-
I. Kufareva and R. Abagyan, Methods of protein structure comparison, Methods in molecular biology, Clifton, NJ, 2012, vol. 857, pp. 231–257 Search PubMed.
- S. A. Sehgal, S. Mannan and S. Ali, Pharmacoinformatic and molecular docking studies reveal potential novel antidepressants against neurodegenerative disorders by targeting HSPB8, Drug Des., Dev. Ther., 2016, 10, 1605–1618 CrossRef CAS PubMed.
- R. Ojha, N. Gupta, B. Naik, S. Singh, V. K. Verma and D. Prusty,
et al., High throughput and comprehensive approach to develop multiepitope vaccine against minacious COVID-19, Eur. J. Pharm. Sci., 2020, 151, 105375 CrossRef CAS PubMed.
- S. Falsafi-Zadeh, Z. Karimi and H. Galehdari, VMD DisRg: New User-Friendly Implement for calculation distance and radius of gyration in VMD program, Bioinformation, 2012, 8(7), 341 CrossRef PubMed.
- W. M. Berhanu and U. H. Hansmann, Side-chain hydrophobicity and the stability of Aβ16–22 aggregates, Protein Sci., 2012, 21(12), 1837–1848 CrossRef CAS PubMed.
- I. Chikalov, P. Yao, M. Moshkov and J.-C. Latombe, Learning probabilistic models of hydrogen bond stability from molecular dynamics simulation trajectories, BMC Bioinf., 2011, 12(1), S34 CrossRef PubMed.
- C. N. Pace, H. Fu, K. Lee Fryar, J. Landua, S. R. Trevino and D. Schell,
et al., Contribution of hydrogen bonds to protein stability, Protein Sci., 2014, 23(5), 652–661 CrossRef CAS PubMed.
- D. Li, B. Ji, K. C. Hwang and Y. Huang, Strength of hydrogen bond network takes crucial roles in the dissociation process of inhibitors from the HIV-1 protease binding pocket, PLoS One, 2011, 6(4), e19268 CrossRef CAS PubMed.
|
This journal is © The Royal Society of Chemistry 2023 |
Click here to see how this site uses Cookies. View our privacy policy here.