DOI:
10.1039/D3LC00204G
(Paper)
Lab Chip, 2023,
23, 2664-2682
Investigating the effects of arginine methylation inhibitors on microdissected brain tumour biopsies maintained in a miniaturised perfusion system†
Received
9th March 2023
, Accepted 3rd May 2023
First published on 3rd May 2023
Abstract
Arginine methylation is a post-translational modification that consists of the transfer of one or two methyl (CH3) groups to arginine residues in proteins. Several types of arginine methylation occur, namely monomethylation, symmetric dimethylation and asymmetric dimethylation, which are catalysed by different protein arginine methyltransferases (PRMTs). Inhibitors of PRMTs have recently entered clinical trials to target several types of cancer, including gliomas (NCT04089449). People with glioblastoma (GBM), the most aggressive form of brain tumour, are among those with the poorest quality of life and likelihood of survival of anyone diagnosed with cancer. There is currently a lack of (pre)clinical research on the possible application of PRMT inhibitors to target brain tumours. Here, we set out to investigate the effects of clinically-relevant PRMT inhibitors on GBM biopsies. We present a new, low-cost, easy to fabricate perfusion device that can maintain GBM tissue in a viable condition for at least eight days post-surgical resection. The miniaturised perfusion device enables the treatment of GBM tissue with PRMT inhibitors ex vivo, and we observed a two-fold increase in apoptosis in treated samples compared to parallel control experiments. Mechanistically, we show thousands of differentially expressed genes after treatment, and changes in the type of arginine methylation of the RNA binding protein FUS that are consistent with hundreds of differential gene splicing events. This is the first time that cross-talk between different types of arginine methylation has been observed in clinical samples after treatment with PRMT inhibitors.
1. Introduction
Gliomas, arising from glial cells, are the most prevalent malignancy of the central nervous system (CNS). Gliomas can be subcategorised into oligodendroglioma, ependymoma and astrocytoma, dependent upon the malignant cell type.1 Astrocytoma are the most frequent CNS tumours, accounting for almost 80% of all malignant primary brain tumours.2,3 The most aggressive, but also the most common form of astrocytoma is glioblastoma (GBM).4 GBM was recently reclassified by the WHO as an adult-type diffuse glioma, of which there are several subtypes, including giant cell glioblastoma, gliosarcoma and epithelioid glioblastoma.1 Molecular features include isocitrate dehydrogenase (IDH) and histone-3 wildtype status, as well as potential telomerase reverse transcriptase (TERT) promoter mutation, chromosome +7/−10 and endothelial growth factor receptor (EGFR) amplification. GBM has a universally poor prognosis due to its highly proliferative nature. GBM is mostly diagnosed in older adults (55–64 years);5,6 and the average survival of people diagnosed with GBM is short (12–15 months after diagnosis),7 with a 1 year survival rate of 37.2%, and a 5 year survival rate of 5.1%.5 Exposure to high doses of ionising radiation is the only confirmed risk factor for GBM.8,9 An example is exposure to intracranial radiation for therapeutic reasons, and there is an association with patient age and radiation dose/volume.10,11 Additionally, a small percentage (<5%) of people with gliomas may have a germline predisposition.12
Histologically, GBM presents as a tumour with neoplastic cells having astrocytic characteristics, necrosis and/or endothelial proliferation.13 The diagnosis of GBM is initially driven by neuroimaging, followed by biopsy or resection of tumour tissue, towards the grading and characterisation of the tumour following histological and genetic analysis.14 Current clinical treatment options after maximal brain tumour resection are limited to radio- and chemotherapy (temozolomide, TMZ) regimens. This standard-of-care increases survival by about two months,15 however, recurrence is almost inevitable16,17 and GBM is currently the subject of intense preclinical and clinical research.
In fact, many models to investigate brain tumours have been described, including the use of simple, 2-D cell culture of GBM-derived cell lines (e.g. U87-MG cells), and spheroids grown from cell lines or primary GBM cells, where cell morphology and behaviour can be modelled in 3-D. Tumour-on-chip models, where tumour tissues (sliced or micro-dissected), extracted from patients, are maintained in (micro)fluidic devices, have gained much traction over the past two decades. These (micro)fluidic devices enable the continuous flow of nutrients and the removal of waste, while maintaining the tumour microstructure and microenvironment ex vivo, and are thought to better resemble some of the properties of the tumour microenvironment in vivo.18 Work in this field has included the maintenance and investigation of a range of normal and diseased tissues, e.g. ovarian cancer,19 breast cancer,20 lung cancer,21,22 rectal cancer,23 thyroid,24 head and neck25–28 and brain tissues.29–32 Recently, our group has reported the study of 128 GBM biopsies from 33 patients, using a first-generation brain tumour chip.33 Other groups have reported the co-culture of brain tumour cells or tissues on-chip with immune cells,34 micro-glial cells,35 HUVEC cells,36 as well as more complex microphysiological systems that provide a biomimetic microenvironment for brain cells through inclusion of endothelial cells, pericytes, glial cells and neurons.37,38 Clinically, efforts to understand factors determining survival focus on combining TMZ with other anticancer agents; on understanding the genomic drivers of recurrence;39,40 on the development of new drugs41,42 and on surpassing radiation resistance.43
Protein methylation is a protein post-translational modification that consists of the transfer of methyl groups from S-adenosyl-L-methionine (SAM) onto specific arginine or lysine residues.44,45 The enzymes responsible for arginine methylation are known as protein arginine methyltransferases (PRMTs). There are three types of PRMTs, each responsible for a different arginine methylation end-product: type I PRMTs lead to asymmetric dimethylarginine (aDMA); type II PRMTs produce symmetric dimethylarginine (sDMA); and type III PRMTs form monomethyl arginine (mMA) only. mMA is produced by all three PRMT types and is often seen as a stable intermediate product in type I and II PRMT reactions. Type I PRMTs include PRMT1, −2, −3, −4, −6 and −8. PRMT5 and −9 are type II PRMTs. PRMT7 is the only type III PRMT identified to-date.46 It is accepted that most of the arginine methyltransferase activity in mammalian cells can be attributed to PRMT1.47 The distinct arginine methylation modifications cause differential effects on protein functions and cell biology, including regulating cell proliferation and apoptosis.
In fact, it is well established that the dysregulation of PRMT activity can lead to cancer.48 For this reason, cell-permeable PRMT inhibitors have been developed over the past decade and have been investigated as possible therapeutic measures for the treatment of cancer in cell and animal models. Importantly, PRMT inhibitors are currently in clinical trials by major pharmaceutical companies to target a range of tumours (at least 11 active or recruitment trials as per September 2022),49,50 including GBM as expansion cohorts.51 Two such PRMT inhibitors include MS023 and GSK3368715, both targeting type I PRMTs. MS023 is a potent and selective inhibitor, binding to the substrate binding site of type I PRMTs,52 with IC50 values ranging from 4 to 119 nM. GSK3368715 is a potent and reversible, SAM-non-competitive inhibitor, with IC50 values in the low nM range, which has been shown to inhibit tumour growth or cause tumour regression in in vivo models.49 GSK3368715 was entered into phase I clinical trials in 2018 for refractory diffuse large B-cell lymphoma and other selected solid tumours.49 Of note, inhibitors targeting different types of PRMTs can have synergistic effects.49,53,54 This can be explained, at least in part, by the observation that inhibiting (or knocking down) specific PRMTs often leads to substrate scavenging by other PRMTs.55,56 However, this so-called ‘cross-talk’ between PRMTs has only been described in cell models (including brain tumour cells57), and its clinical relevance remains uncertain.
We and others have found that hundreds of proteins are modified by arginine methylation in the brain.58,59 However, the effects of PRMT inhibitors on brain tumours have not yet been fully investigated. Our research question was thus: what is the effect of PRMT inhibitors on GBM? We set out to answer this question by investigating the effects of clinically-relevant PRMT inhibitors on patient GBM biopsies that were maintained in perfusion devices.
2. Materials and methods
2.1. Cell lines, brain tumour samples and ethical considerations
This project was approved by the Yorkshire & The Humber South Yorkshire Research Ethics Committee (13/YH/0238) and by the NIHR (IRAS 131630). Surplus GBM tissue, which was not required for histopathological analyses, was collected from the Hull University Teaching Hospitals NHS Trust, with written informed consent from patients. Patients and samples were recruited between 2018 and 2022. Consented patients had brain tumours believed to be GBM (primary or recurrent), at the time of initial diagnosis, as indicated by neuroimaging. All patients had been given dexamethasone (Dex) twice per day, at the time of initial diagnosis and through imaging. Standard procedure was that patients received 8 mg Dex for at least 48 hours, which was then reduced to between 2–4 mg until the day of surgery. Dose was then increased back to 8 mg on the day of surgery for 48 hours, and then again reduced to 2 mg. Patients may or may not have undergone prior treatment with TMZ and radiotherapy, dependent upon whether the tumour was recurrent. Resected GBM tissue samples were transported in 10 ml Dulbecco's Modified Eagle's Medium (DMEM) (with 4500 mg L−1 glucose, L-glutamine, sodium bicarbonate and phenol red; without sodium pyruvate and HEPES) (plus 10% FCS, 1 mM streptomycin/ampicillin, 1 mM sodium pyruvate) (Merck, Dorset) in an insulated box and put in the miniaturised perfusion system within two hours of resection. Pathology results (e.g. MGMT promoter methylation status) were not known at the time of experimental set up.
Patient-derived cell lines (GBM63 and GBM58) were acquired from the Leeds Neuropathology Research Tissue Bank (NHS Research Ethics Committee code 20/YH/0109). These were derived from GBM tissue from patients that consented for the use of their tissue in research, and specifically for the generation of in vitro models. They were derived in serum free conditions.
2.2. Perfusion device and maintenance of spheroids and GBM tissue
The perfusion device was a custom-designed polymethyl methacrylate (PMMA) flow cell, consisting of three individual in-house laser-cut (LS6840, HPC Laser, UK) pieces, assembled together by hand and bonded using chloroform (Fig. 1A). The inlet and outlet were 4 mm in diameter, to enable direct interfacing with Luer to barb tubing connectors. The internal chamber, where the sample was placed, was also 4 mm in diameter and the central piece held a tissue retaining layer with 37 × 100 μm holes to maintain the tissue position, while allowing the flow of media to occur. The chamber was connected to a 0.22 μm-filtered 20 ml syringe, containing media (DMEM plus 10% FCS, 1 mM streptomycin/ampicillin, 1 mM sodium pyruvate), and either dimethyl sulfoxide (DMSO), or pharmaceutical agents (TMZ or arginine methylation inhibitors as appropriate), delivered through 1/32′′ Tygon Silicone tubing (Cole Parmer) via female/male elbow Luer connects (Ibidi). The same tubing was connected to the outlet of the perfusion device to allow effluent drainage (Fig. 1A).
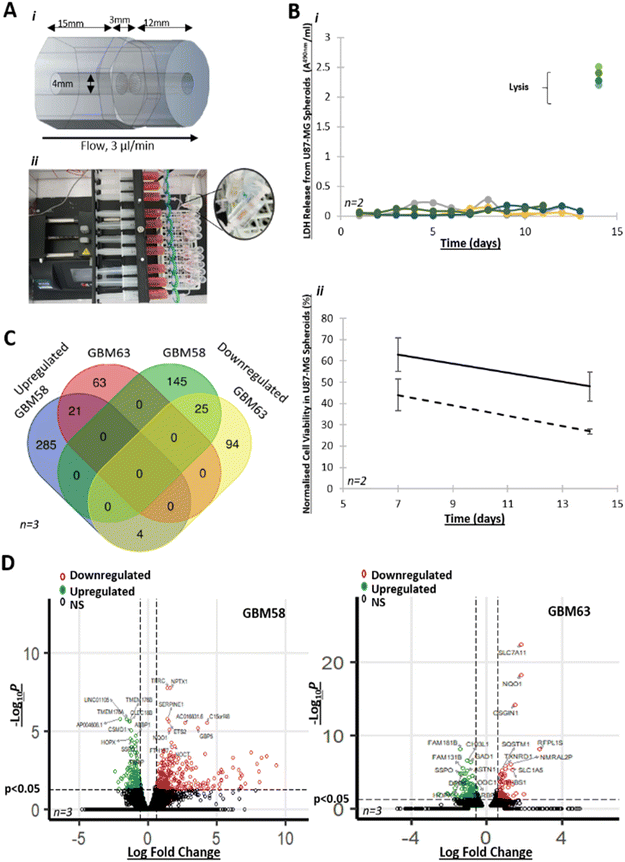 |
| Fig. 1 A new perfusion system for the investigation of GBM cell line spheroids Ai) the simple, ‘in-house,’ microfabricated perfusion device was comprised of three pieces of polymethyl methacrylate (PMMA), fused together with chloroform. The central section contained a frit to allow media flow to the effluent chamber (right) whilst retaining the biopsy in the inlet chamber (left). This choice of perfusion devices reduces the requirement for speciality fabrication equipment, thereby overcoming common barriers of expertise and cost that prevent the widespread implementation of additional, valuable research models. ii) Ten devices were routinely set up in parallel to enable dynamic throughput. Devices were connected to 20 ml syringes with a 0.33 μm filter and loaded onto a Harvard Apparatus PHD Ultra syringe pump (or equivalent), via 1/32′′ Tygon silicone material tubing with female/male elbow Luer connects (Ibidi). An effluent tube was similarly connected to the opposite end of the perfusion device and effluent collected in a 15 ml centrifuge tube. The entire system sat within a purpose-built incubator at 37 °C. Calculated Reynold No. was 0.5 × 10−2 with shear stress calculated at 4.9 × 10−5 dyne per cm2. Bi) Cytotoxicity assay (LDH) of U87-MG spheroids with end-stage lysis, over 13 days. Four individual technical replicates are shown. ii) MTS assay indicates cell viability of 62% and 44% in U87-MG spheroids in the device, treated with 10 μM (solid line) and 100 μM TMZ (dashed line) respectively (n = 2, ± SEM), normalised to untreated controls. C) RNA-seq data indicating shared significant (p < 0.05) DEGs (log2 fold change > 0.585) in spheroids from the GBM primary cell line GBM58 and GBM63, maintained for 3 days in the perfusion device vs. GBM58 and GBM63 spheroids maintained for 3 days in a static culture (n = 3). D) Volcano plots highlighting top 10 significant (p < 0.05) DEGs (log2 fold change > 0.585) in spheroids from the GBM primary cell lines GBM58 and GBM63, maintained for 3 days in the perfusion device vs. spheroids maintained for 3 days in a static culture (n = 3). | |
GBM primary cell lines were maintained in B27-, human basic fibroblast growth factor- and epidermal growth factor-supplemented Neurobasal medium (Fisher Scientific) for a maximum of 11 passages (GBM58 cell line) and 7 passages (GBM63 cell line). U87-MG cells were maintained in supplemented DMEM medium. All spheroids were generated in round-bottomed 96 well plate Ultra-Low Attachment (ULA) (Corning Costar, UK) using an initial cell density of 3 × 104 cells/well and cultured in ULA plates for 10 days prior to experiments. Medium was changed every 3 days.
Brain tumour biopsies were microdissected, upon receipt from the hospital, into ca. 20 mg (±10%) samples. Biopsies were turned out onto a 20 mm petri dish (Corning) in a class II biological hood. Taking care to avoid areas of cauterised tissue, visible necrosis and blood vessels, tissue was manually microdissected by a neurosurgeon using single use, sterile Swann–Morton curved blade no. 2 scalpels and forceps (ESI† Video S1). Samples were generally spherical, around 3 mm in diameter (for an approximate volume of 14 mm3). Microbiopsies were then weighed using a microbalance and biopsies trimmed, using the scalpel, until they reached the standardised weight. ‘Pre-perfusion’ control samples were immediately frozen in optimal cutting temperature medium (OCT, TissueTek®) in 2-methylbutane (Honeywell) pre-chilled in liquid nitrogen, to prevent the formation of ice crystals, and stored at −80 °C. The remaining samples were randomly placed into the perfusion device chambers within two hours of resection (one 20 mg biopsy per device). Devices had been pre-sterilised with 70% ethanol and pre-filled with medium, ensuring absence of air pockets.
Devices containing either spheroids or GBM biopsies were attached to the tubing/syringe system (see above) and loaded onto a Harvard Apparatus PHD-ULTRA syringe pump, which was programmed to infuse media at a flow rate of 2–3 μl min−1. Effluent was collected into 15 ml centrifuge tubes for each 24 hour period, over eight days. Effluents were stored at 4 °C short term (maximum of eight days), or at −80 °C long-term.
2.3. Immunohistochemistry
Pre- and post-perfusion control and treated GBM tissue samples were flash frozen with OCT (TissueTek®) in 2-methylbutane (Honeywell) pre-chilled in liquid nitrogen, and stored at −80 °C before cryostat (Leica Microsystems, Germany) sectioning onto poly-L-lysine coated slides at 8 μm thickness. Sections were fixed in ice-cold 100% methanol for 10 minutes, and washed with tris buffered-saline (TBS, pH 7.6). Endogenous peroxidase activity was blocked in 3% H2O2 in absolute methanol for 15 minutes. Slides were loaded onto Sequenza™ racks and incubated with normal horse serum according to the Vectastain Elite kit protocol (Vector Laboratories). This was followed by incubation with avidin and then biotin solutions (Vector Laboratories), to prevent non-specific binding of reagents. Slides were washed with TBS between different reagent additions. Sections were incubated overnight at 4 °C with antibodies against the apoptosis marker poly(ADP-ribose) polymerase (cleaved-PARP Asp214, D6E10, Cell Signalling #5625S) in TBS (1
:
100 dilution). After washes in TBS, the secondary antibody was added according to the manufacturer's instructions, then with streptavidin ABC reagent, before placing in double-distilled water (ddH2O) and staining with 3,3-diaminobenzidine and H2O2 dissolved in ddH2O, for 5 minutes. Sections were counterstained for 15 seconds with Harris haematoxylin (Merck, Dorset), blued in tap water for 1 minute and then dehydrated in increasing grades of ethanol (70%, 90%, 100%) for 1 minute each and submerged twice in Histoclear (Histochoice®), each for 1 minute. Sections were mounted using glass coverslips (VWR) and Histomount (National Diagnostics).
Microscopy images were taken using an Olympus IX71 microscope, on the brightfield setting, phase 2, ×40 magnification and using CellSens software 1.18. Positive staining was quantified using Cell Profiler 4.1.3. An antigen expression index was calculated for each condition, for each biological replicate, using the ratio of positive cells to total cells. This value was made relative to the control samples (DMSO) by dividing it by the average control antigen expression index. Statistical analysis was performed using R 4.1.2.
2.4. Lactate dehydrogenase (LDH) assays
LDH assays were performed on the effluents collected for each 24 hour period and stored at 4 °C. Assays were performed on the last day of effluent collection, using the Cytotoxicity Detection KitPLUS (Roche). Effluents (50 μl) were analysed in duplicate in a 96-well plate (Corning), with an equal volume of catalyst-dye solution (1
:
45). Two wells in each plate also contained medium-only. After 30 minutes at 37 °C in the absence of light, 1 M HCl (25 μl) was added to stop the reaction. Absorbance was recorded at 495 nm, correcting at 690 nm, using the BioTek™ ELx800™ absorbance microplate reader with Gen5 software 1.08. The medium-only absorbance was subtracted from effluent sample absorbance and the average absorbance for each sample was calculated and normalised per mg of starting tissue weight.
2.5. MTS assays
U87-MG spheroids were transferred from perfusion devices to a clear, flat-bottomed 96-well plate (Corning), using a p1000 pipette to prevent mechanical stress. Spheroids (in 100 μl growth medium) were incubated with CellTiter 96 Aqueous One Solution reagent (Promega) for 1 hour at 37 °C, 5% CO2. Plates were shaken at 300 rpm for 2 minutes before transfer of the supernatant to a clear, flat-bottomed 96-well plate (Corning). Absorbance was recorded at 490 nm using a Biotek Synergy HTSpectrophotometer.
2.6. Cytokine analysis
Cytokine profiling in effluents was performed using the Human XL Cytokine Array Detection kit (R&D Systems), which includes 105 cytokines. Effluents from two control (untreated) devices were combined, at three different time points (48, 96 and 192 hours) to offset any effects of intratumour heterogeneity and sample size distribution. To prevent non-specific protein binding, membranes were blocked for one hour before incubating overnight at 4 °C with effluents diluted in array buffer 6 (8
:
7). Three time points were assessed, that is, effluents corresponding to the second day, the fourth day and the eighth day of incubation in the perfusion device. After washing in the provided wash buffer, membranes were incubated with an antibody detection cocktail for 1 hour, and then with streptavidin-HRP for 30 minutes, before visualising using the ChemiDoc™ Imaging system (Bio-Rad), with Clarity™ ECL Western substrate (Bio-Rad). Densitometry was analysed using R&D-approved software, HLImage++ Quick Spots (Western Vision Software, 2021) and statistics performed using R 4.1.2.
2.7. Western blot
Protein lysates were produced from GBM biopsies both pre-perfusion (i.e. fresh biopsies from surgery) and post-perfusion (i.e. GBM samples after 8 day incubation in the perfusion device). Tissue was homogenised in 1% Triton X-100 and phosphate buffered saline (PBS) plus protease inhibitors (Sigma) using a Dounce homogeniser. Crude lysates were incubated on a rotating wheel for 1 hour at 4 °C and centrifuged at 13
000 × g. The aqueous layer was siphoned off as the protein lysate, taking care not to disturb the nucleic acid and cell debris pellet and total protein concentrations were quantified using a bicinchoninic acid assay (BCA) (Sigma), according to the manufacturer's instructions.
Protein lysates were boiled with Laemmli sample buffer and proteins (20–50 μg) were resolved through 10% SDS-PAGE run at 120 V. Transfer to a nitro-cellulose membrane (ECL Hybond) was performed at 30 V overnight, before blocking in 5% milk (Marvel) in TBS (w/v) solution for 1 hour. Membranes were washed twice in TBS with 1% Tween-20 (TBST) before incubation at 4 °C overnight in 1% milk in TBST (w/v) containing 1
:
1000 dilutions of primary antibodies (#13522, #13222 or #8015 for aDMA, sDMA and mMA, respectively, all from Cell Signalling Technologies) as appropriate. Binding was detected using horseradish peroxidase (HRP)-conjugated goat anti-rabbit antibody (Dako #P0448, 1
:
5000 dilution), using Clarity™ ECL Western substrate (Bio-Rad) and imaged as previously described. Densitometry analysis was performed in ImageJ and protein band intensity was normalised to loading controls (actin, GAPDH) bands.
2.8. Methyl-SILAC, and immunopurification and mass spectrometry analysis of methylated peptides
We utilised heavy methyl SILAC (hmSILAC) to label proteins modified by arginine methylation in brain tumour biopsies maintained in the perfusion device, through adaptation of previously published protocols.55 Briefly, two devices containing control (DMSO) tumour pieces from the same biopsy were connected to medium containing, respectively, light methionine and heavy (13CD3) methionine (100 μM). In parallel, two devices containing tumour pieces from the same biopsy (D17) were treated with MS023 (100 nM) and connected to medium containing light methionine and heavy methionine as before. After eight days of perfusion, the tissues were snap-frozen in liquid nitrogen. Tissue (30 mg) lysis was done in 500 μL of urea lysis buffer (20 mM HEPES, 9 M Urea and protease inhibitors) and the lysates were sonicated for 5 minutes at 30 second intervals. The lysates were then cleared by centrifugation at 20
000 × g for 15 minutes and the supernatant transferred to a clean tube. Lysates from control tissue incubated with 13CD3 and from the MS023-treated sample incubated with light Met were pooled into ‘pool 1’ at a 1
:
1 protein concentration ratio. Lysates from control tissue incubated with light Met and from the MS023-treated sample incubated with 13CD3 were separately pooled (‘pool 2’). Prior to mass spectrometry analysis and to increase sensitivity of the analysis towards methylated peptides, the samples were enriched with peptides modified by mMA using immunoprecipitation and anti-mMA antibodies as previously described.59,60 Following purification, peptides were separated by liquid chromatography (50 cm C18 EN PepMap column) and analysed on-line using an Orbitrap Fusion Tribrid mass spectrometer using a Waters mClass UPLC. Resulting LC–MS chromatograms were peak picked and searched against the human subset of the SwissProt database (October 2019) using PEAKS Studio X. The number of missed cleavages considered was set to four with trypsin specified as the enzyme. Cys carbamidomethylation was set as fixed modification, and Met oxidation and arginine methylation were set as variable modifications. False discovery rate was set at 0.05.
2.9. RNA-sequencing analysis
Spheroids were retrieved from the ULA plate or perfusion device and transferred to RNAse free centrifuge tubes for RNA extraction. For tissue work, post-perfusion samples (control and 1 μM GSK3368715-treated) were snap frozen in liquid nitrogen and stored at −80 °C until use. Of note, tissues maintained in the same conditions (either treated or control) were pooled from 2–3 miniaturised devices, for each of the biopsies (n = 4 biopsies, D31 to D34). TRIzol™ (ThermoFisher Scientific) was added to the spheroids or tissue biopsies and samples were homogenised using a Dounce homogeniser. Chloroform was added 1
:
5 to the lysate, tubes inverted gently for 15 seconds and then incubated for 3 minutes on ice. Samples were centrifuged at 12
000 × g for 15 minutes at 4 °C. Isopropanol was added 1
:
2 to the upper, colourless, aqueous phase and samples were incubated overnight at −80 °C before centrifuging at 12
000 × g for 10 minutes at 4 °C.
The RNA pellet was washed twice in 75% ethanol before centrifuging for 5 minutes, at 7500 × g at 4 °C and the supernatant discarded each time. The RNA pellet was air dried before resuspending in RNase-free water through incubation at 55 °C. Total RNA was then analysed to yield the transcriptomic profile of the cells and tissues in the two different conditions (for cell work, cells grown in the perfusion device vs. under static conditions and for biopsy work, control vs. 1 μM GSK3368715-treated tissues). Library preparation and RNA sequencing were done by Novogene Co. Ltd. (Cambridge, UK) using the Illumina NovaSeq 6000 platform at a depth of 34–60 million reads per sample. Briefly, the HISAT2 algorithm was used for read alignment and FPKM was used to normalise gene expression.61 Differential gene expression was determined from read counts using the DESeq2 R package.62 Gene expression change in either cells in the perfusion device, or treated tissues, compared to cells grown in static conditions, or to control tissues in the perfusion device, respectively, was log2 transformed, and the calculated p-values were adjusted using the Benjamini–Hochberg correction for false discovery rate (padj). Genes significantly (padj ≤ 0.05) up- or down-regulated at least 1.5-fold (log2 fold change FC = ±0.585) with a gene symbol/ID annotation were used in subsequent analysis. Gene Ontology (GO) enrichment and Kyoto Encyclopedia of Genes and Genomes (KEGG) pathway enrichment were done using ShinyGO63 and GOrilla.64 RNA-sequencing data have been deposited in NCBI's Gene Expression Omnibus (GEO-https://www.ncbi.nlm.nih.gov/geo/) and are accessible through the GEO Series accession number (GSE226721).
2.10. Statistical methods.
Statistical analysis was performed using R 4.1.2. The specific statistical tests used are acknowledged in the legends to the figures.
3. Results and discussion
The aim of this work was to investigate the effect of PRMT inhibitors on GBM, a disease with clearly unmet clinical needs. Building on our extensive experience with tumour-on-chip approaches, we set out to design a a novel, miniaturised perfusion system that enabled the investigation of GBM cells and tissue at the molecular and cell biology levels.
3.1. A new perfusion device that enables the maintenance of primary GBM cell line spheroids
First, we tested our new perfusion system design using GBM cell spheroids. We incubated U87-MG cell spheroids in the perfusion device for two weeks and we did not observe any increase in LDH release during that period, suggesting that cell membrane integrity was maintained throughout (Fig. 1Bi). We then treated U87-MG spheroids with TMZ in the perfusion device and we observed a decrease in cell viability after one- and two-weeks' treatment compared to control (Fig. 1Bii).
To assess how GBM spheroids responded to incubation in the device at the molecular level, we compared gene expression profiles of spheroids from two primary, patient-derived, cell lines (GBM58 and GBM63)65 that were maintained in the perfusion device for 72 hours versus spheroids cultured in static conditions. We found 637 unique DEGs (369 upregulated and 264 downregulated, padj < 0.05, FC > 1.5) between spheroids maintained in the flow system, compared to static conditions (Fig. 1C and D). GO term enrichment analysis of DEGs identified GO terms associated with cell migration, cell–cell communications (e.g. proinflammatory cytokines), sugar metabolism, transcription factor activity, extracellular matrix (ECM) interactions and defence mechanisms, as well as the regulation of cell death and oxidative stress, in either GBM58 or GBM63 spheroids grown in the perfusion device vs. spheroids grown in static cultures (ESI† Fig. S1 and Table S1). These results show that GBM spheroids responded to the continuous flow of the perfusion system through regulating key cancer pathways, including GBM.66–69 Therefore, our system provided a useful platform and a research opportunity to investigate GBM in greater detail.
3.2. Maintaining fresh GBM biopsies in a viable condition in the laboratory
Next, we investigated our model for the maintenance of GBM biopsies from patients. We recruited tissue donors undergoing brain tumour surgery for suspected GBM. During surgery, surplus brain tumour tissues not required for histopathological analysis were collected and transported to our laboratory, where biopsies were maintained in miniaturised devices (Fig. 2A). Through the analysis of cell integrity, proliferation and apoptosis markers, we clearly show below that biopsies remained viable for at least eight days in the device under continuous flow of medium.
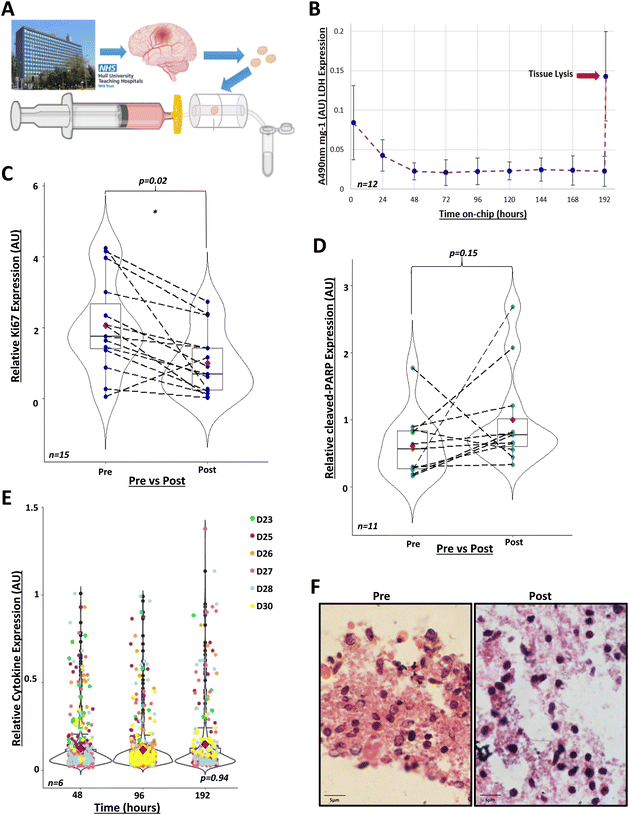 |
| Fig. 2 GBM tissue can be maintained in the perfusion system in viable conditions for 8 days. A) GBM tissue was collected from the Hull Royal Infirmary during surgery and taken to the University of Hull campus. Tissue was dissected into 20 mg ± 10% samples. Each sample was placed into an individual perfusion device pre-filled with medium. Effluent was collected every 24 hours. B) Lactate dehydrogenase (LDH) activity was measured in the effluent, collected every 24 hours over 8 days, via absorbance at 490 nm (A490nm) (n = 12, D8, D12, D14, D18–D21, D30, D31, D33–D35, see Table 1). LDH activity decreased to 0.02 AU (absorbance units)/mg ± 0.011 at 48 hours and fluctuated minimally (F = 3.64, df = 1, p = 0.06) between 0.02–0.025 AU mg−1 for the remaining 8 days, these fluctuations correlated with syringe refilling with medium at day 4. After 8 days, tissues were lysed to assess remaining LDH within the biopsies (n = 10), leading to a peak reading at 0.145 AU mg−1 ± 0.057 (indicated by red arrow) (F = 63, df = 1, p = 1.32 × 10−7, compared to 48 hours). One-way ANOVA performed using R 4.2.0 after data transformation. C) Immunohistochemistry of GBM tissue pre- and post-perfusion, with proliferative marker Ki67 (n = 15, D12, D14, D15, D18–D24, D30, D31, D33–D35, see Table 1), relative to the post-perfusion control. Analysis was performed using R 4.1.2., using the Mann–Whitney U paired wilcox.test function for comparison of medians (w = 168 p = 0.023). Mean Ki67 expression pre-perfusion was 2.06-fold ± 1.30 higher than post-perfusion (red diamonds). Dashed lines indicate paired patient samples. D) Immunohistochemistry of GBM tissue pre- and post-perfusion with the apoptotic marker, cleaved-PARP (n = 11, D19-D28 and D30), normalised to post-perfusion values. Analysis was performed using R 4.1.2., using the Mann–Whitney U paired wilcox.test function for comparison of medians (w = 38, p = 0.151). Mean cleaved-PARP expression pre-perfusion was 0.609 ± 0.474-fold lower than post-perfusion (red diamonds). Dashed lines indicate paired patient samples. E) Cytokine expression in combined duplicate effluents of control samples (n = 6) after 48, 96 and 192 hours in the perfusion device in arbitrary units (AU). Kruskal-Wallis test performed in R 4.1.2 (X2 = 0.134, df = 2, p-value = 0.935). F) Haematoxylin and eosin staining of GBM tissue pre- and 8 days post-perfusion. Images taken using Olympus IX71 Inverted microscope, using CellSens software 1.18 (magnification ×100). | |
Firstly, we showed that cell integrity was maintained during incubation. We collected daily samples of the effluent and analysed LDH activity. Following the initial 24 hours in the device, when elevated LDH levels were due to tissue disruption during resection and handling, LDH activity remained low for the duration of the experiments (Fig. 2B). Tissue lysis at the end of the incubation period demonstrated a peak of LDH. These are well-known patterns that indicate that cell plasma membranes were intact during tissue incubation in the device.24,26–28,70–72
Secondly, we showed that the cytokines released by GBM tissues in the device remained the same for the duration of the experiment. We assayed the levels of 105 cytokines in the effluents corresponding to days 2, 4 and 8 of incubation. A reduction in the number of cytokines or decreased levels of cytokines released by tissues could indicate cell death. On average, 63 cytokines, out of the 105 in the microarray, were routinely detectable and quantifiable in effluents and the top-10 most abundant were interleukin-8 (IL-8), Serpin E1, Osteopontin, Chitinase 3-like 1, vascular endothelial growth factor (VEGF), matrix metalloproteinase-9 (MMP-9), macrophage migration inhibitory factor (MIF), monocyte chemoattractant protein-1 (MCP1), insulin-like growth factor binding protein-2 (IGFBP-2) and extracellular matrix metalloproteinase inducer (EMMPRIN). We found no statistically significant changes in the profiles of cytokine secretion over time (Fig. 2E and S2†), which again supports the notion that brain tumour tissues were maintained in a viable condition in the perfusion device.
Thirdly, we showed that brain tumour cells did not undergo apoptosis and even proliferated after eight days in the perfusion device. We used immunohistochemistry to compare the levels of cell proliferation and apoptosis markers between paired samples of fresh biopsies (‘pre-perfusion’) and tissues that had been maintained for eight days in the perfusion device (‘post-perfusion’). We found that post-perfusion samples conserved ca. 40% of the levels of the cell proliferation marker Ki67, compared with pre-perfusion samples (Fig. 2C). This was in line with our expectation of a decrease in the proliferative capacity of brain tumour cells eight days after surgical resection. To rule out that this reduction was associated with cell death, we compared the levels of the apoptosis marker cleaved-PARP between pre-perfusion and post-perfusion samples. We found no statistically significant difference in the levels of brain tumour cell apoptosis in 11 paired post-perfusion vs. pre-perfusion samples (Fig. 2D), meaning that incubation in the device did not reprogramme cells to undergo death.
Finally, H&E staining, assessed by a neuropathologist, showed that tissue architecture was maintained post-perfusion (Fig. 2F and S3†). Collectively, these data clearly show that our perfusion system maintained GBM biopsies in a viable condition for at least eight days after resection.
3.3. GSK3368715 causes apoptosis of GBM tissues
Having shown that GBM biopsies could be maintained in our perfusion system for eight days, we tested the hypothesis that treatment of GBM tissue with PRMT inhibitors leads to tumour cell death. For each of 10 patient samples, we ran parallel devices where 1 μM GSK3368715 was included in the medium feeding 3–4 devices, run alongside 3–4 controls (DMSO, maximum 0.0004% or 56 μM) from the same GBM biopsy. We assessed the effect of treatment on cell apoptosis through measuring the expression of cleaved-PARP using immunohistochemistry. We found a statistically significant increase in the expression of cleaved-PARP in samples treated with GSK3368715, compared to DMSO, untreated controls (Fig. 3A and C). This indicated that GSK3368715 caused brain tumour cell death (apoptosis) in a specific manner.
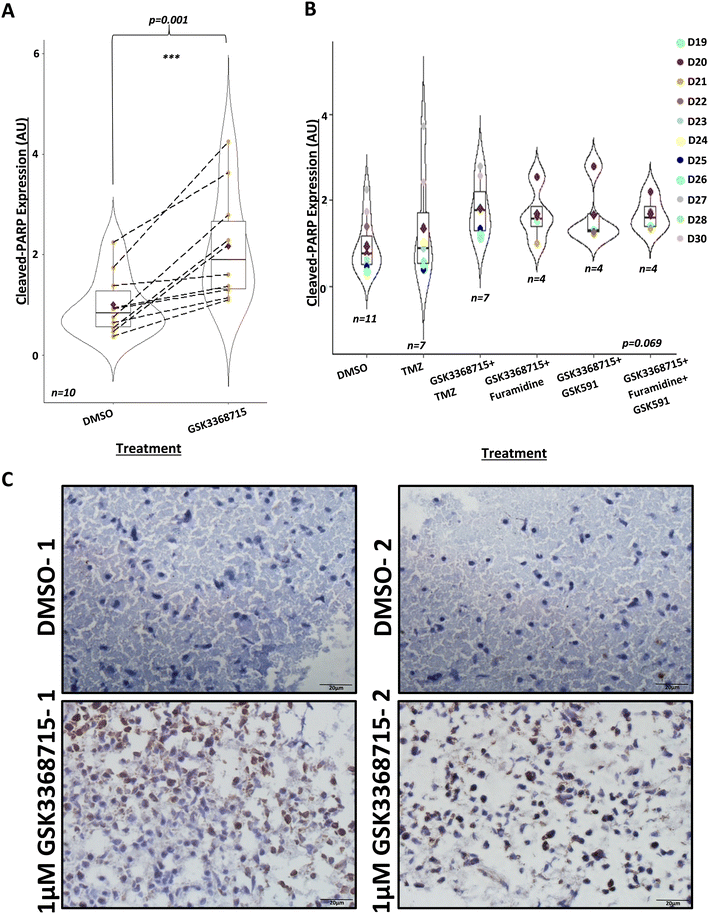 |
| Fig. 3 PRMT inhibition by GSK715 induces apoptosis in GBM tissue after 8 days. A) Immunohistochemistry of GBM tissue 8 days post-perfusion, treated with DMSO control or 1 μM GSK3368715, with apoptotic marker, cleaved-PARP (n = 10, D19–D23, D25–D28 and D30, see Table 1). Analysis was performed using R 4.1.2., using the paired t-test function. Mean cleaved-PARP expression in 1 μM GSK3368715-treated GBM tissue increased 2.17 ± 1.1-fold compared to cleaved-PARP expression in parallel control samples, as indicated by the red diamonds. Median cleaved-PARP expression in 1 μM GSK3368715-treated samples: t = −4.52, df = 9, p = 0.001. B) Immunohistochemistry of GBM tissue 8 days post-perfusion, treated with: DMSO (control, n = 11), 10 μM TMZ (n = 7), 1 μM GSK3368715 + 10 μM TMZ (n = 7), 1 μM GSK3368715 + 1 μM furamidine (n = 4), 1 μM GSK3368715 + 1 μM GSK591 (n = 4), or 1 μM GSK3368715 + 1 μM furamidine + 1 μM GSK591 (n = 4), with apoptotic marker, cleaved-PARP, relative to the post-perfusion DMSO control. Analysis was performed using R 4.1.2 using the Kruskal_test function. The overall statistical significance was borderline (statistic = 10.2, df = 5, p = 0.069), due to the co-treatments with GSK3368715, but there was no evidence of synergies with other PRMT inhibitors or TMZ (compare with panel 3A). C) Representative IHC images from two 8 day post-perfusion DMSO controls and 1 μM GSK3368715-treated chips (D30), taken on Olympus IX71 Inverted microscope, using CellSens Entry v1.18, magnification ×40. | |
Given previous observations that inhibitors targeting different types of PRMTs can have synergistic effects,49,53,54 we investigated any synergies between GSK3368715 (a type I PRMT inhibitor) and other type I and type II PRMT inhibitors in leading towards increased brain tumour cell death. However, there were no evident synergies with any of the combined treatments (Fig. 3B). We additionally combined GSK3368715 with TMZ. In patients with MGMT promoter methylation, TMZ is recognised to cause DNA damage through the methylation of guanine at O6. The resulting O6-meG is genotoxic due to its subsequent nucleotide mispairing with thymine instead of cytosine during DNA replication. The generation of DNA breaks, produced when mismatch repair (MMR) enzymes attempt to cleave the O6-meG adduct induces G2/M phase cell cycle arrest and ultimately cell death.73,74 Given that the inhibition of PRMTs impairs DNA damage repair mechanisms,75,76 we hypothesised that the combined treatment of TMZ and GSK3368715 would lead to synergistic effects against GBM. To test this hypothesis, we collected seven biopsies and exposed them for eight days to the combined treatment of TMZ (10 μM) and GSK3368715 (1 μM) while maintained in the device. We did not observe any significant enhancement of GSK3368715-driven cell apoptosis upon TMZ co-treatment (Fig. 3B), however, a possible explanation for this was the fact that six out of the seven biopsies came back as negative for methylation of the MGMT promoter (Table 1). Additionally, here we used 10 μM TMZ as a lower-end clinically relevant concentration;77–79 the possibility remains that higher but relevant TMZ doses (e.g. 30–100 μM) cause GBM tissue apoptosis and synergise with PRMT1 inhibitors in doing so. Finally, we have done experiments at the eight-day end-point only, and it is conceivable that longer incubation times may lead to synergistic effects between GSK336715 and other PRMT inhibitors or TMZ.
Table 1 Clinical data from 40 donors (D). Anonymised sample IDs were allocated to each donor. During routine histopathological analysis, it was noted whether the tumour is primary or recurrent. Molecular markers of interest included isocitrate dehydrogenase (IDH) mutation status; O(6)-methylguanine-DNA methyltransferase (MGMT) promoter methylation status; epidermal growth factor receptor (EGFR) amplification and telomerase reverse transcriptase (TERT) promoter mutation status. ‘+’ indicates mutation or presence of molecular marker, ‘—’ indicates wildtype, or absence of molecular marker. Clinical and histopathological data were not known at the time of tumour setup in the perfusion device. Samples D12, D18, D29 and D38 were collected before the change in GBM classification in 2021, and were therefore retained in this study
Sample ID |
Sex |
Type |
IDH |
MGMT |
EGFR |
TERT |
D1 |
F |
|
— |
— |
|
|
D2 |
M |
|
— |
+ |
|
|
D3 |
M |
|
— |
— |
|
+ |
D4 |
M |
|
— |
— |
|
|
D5 |
M |
|
— |
— |
|
+ |
D6 |
M |
|
— |
— |
|
|
D7 |
F |
|
— |
+ |
|
|
D8 |
M |
|
— |
— |
|
|
D9 |
F |
|
— |
— |
|
|
D10 |
M |
|
— |
— |
|
+ |
D11 |
M |
Recurrent |
— |
— |
— |
|
D12 |
F |
|
+ |
— |
— |
|
D13 |
M |
|
— |
— |
|
|
D14 |
M |
|
— |
— |
— |
+ |
D15 |
F |
|
— |
+ |
— |
— |
D16 |
M |
|
— |
|
|
|
D17 |
F |
|
— |
+ |
|
+ |
D18 |
F |
Recurrent |
+ |
— |
|
+ |
D19 |
M |
Primary |
— |
+ |
|
|
D20 |
M |
Primary |
— |
+ |
|
+ |
D21 |
F |
Primary |
— |
+ |
|
— |
D22 |
F |
Primary |
— |
+ |
— |
— |
D23 |
M |
Primary |
— |
— |
|
+ |
D24 |
M |
Primary |
— |
|
|
|
D25 |
M |
Primary |
— |
+ |
|
|
D26 |
M |
Recurrent |
— |
— |
|
|
D27 |
F |
Primary |
— |
— |
|
+ |
D28 |
M |
Primary |
— |
— |
|
|
D29 |
M |
|
+ |
+ |
|
|
D30 |
M |
Recurrent |
— |
— |
|
|
D31 |
M |
Primary |
— |
— |
|
+ |
D32 |
M |
Recurrent |
— |
— |
|
+ |
D33 |
M |
Primary |
— |
+ |
|
+ |
D34 |
M |
Primary |
— |
|
|
|
D35 |
|
|
|
|
|
|
D36 |
F |
|
— |
|
— |
+ |
D37 |
M |
Primary |
— |
|
|
|
D38 |
F |
Recurrent |
+ |
— |
|
+ |
D39 |
M |
Primary |
— |
— |
|
+ |
D40 |
M |
|
— |
+ |
|
|
Taken together, these data show, firstly, that our perfusion system enables the investigation of new drugs, and combinations of them, against GBM. We routinely set up ten devices in parallel containing tissue coming from the same biopsy and we used 2–4 technical replicates (i.e. 2–4 devices per condition, including control). Therefore, a maximum of five combinations of treatments/concentrations could be investigated using ten parallel devices. The limitation of our approach towards becoming a higher-throughput screening tool was brain tumour tissue availability, and future drug screening efforts, including towards e.g. the optimisation of PRMT inhibitor concentrations, could include multiplexed microfluidics strategies using brain tumour tissue slices.80,81 Secondly, our results warrant further (pre)clinical research into the use of GSK3368715, or next-generation type I PRMT inhibitors, to cause apoptosis of GBM tissue. This is supported by previous observations of the anti-tumour effects of GSK3368715 in cell lines and animal models.49,50
3.4. Cross-talk between asymmetric and symmetric arginine methylation in clinical samples
Cross-talk between different types of PRMTs has not yet been established in clinical samples, mainly because of the difficulty of treating tissue ex vivo with PRMT inhibitors for a long enough period of time. To assess whether our perfusion system could shed light onto this standing question, we treated biopsies with 100 nM MS023 for eight days while maintained in the devices and analysed the patterns of mMA, aDMA and sDMA in these samples. As expected, we observed that treatment with the type I PRMT inhibitor MS023 generally led to reduced protein aDMA (ESI† Fig. S4). Of note, treatment also led to the observation of new or more intense proteins bands recognised by antibodies targeting mMA and sDMA (Fig. 4A, B and S5†).
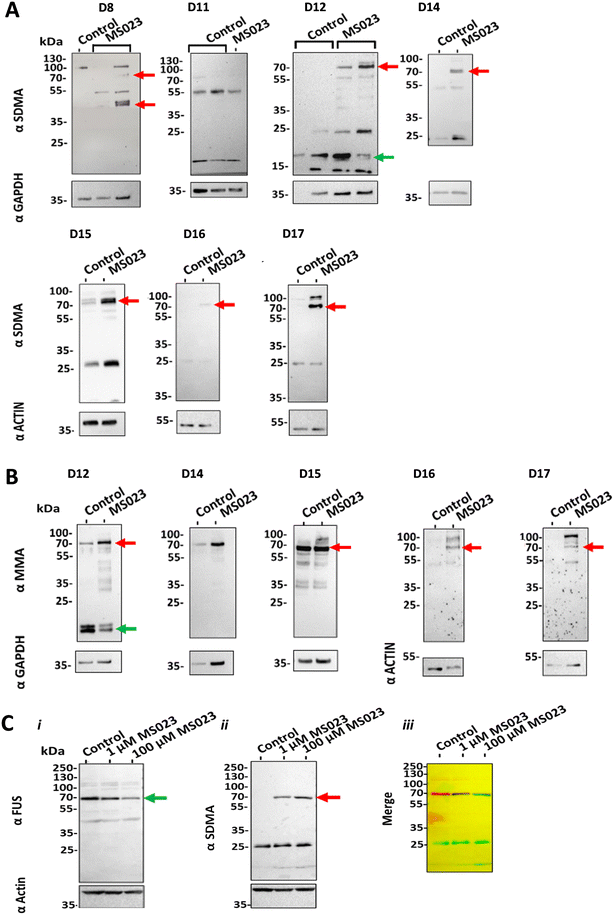 |
| Fig. 4 Treatment with a type I PRMT inhibitor leads to cross-talk with type II PRMTs A) Western blots of GBM samples treated with MS023 alongside controls (no treatment). Seven GBM samples from different donors (D codes) are shown, each treated with 100 nM MS023 for eight days (192 hours). The intensity of a 70 kDa protein band recognised by SDMA-specific antibodies increased in 6 out of the 7 samples analysed (red arrows). B) Western blot analysis of five GBM samples, each treated with 100 nM MS023 for eight days. The intensity of a 70 kDa protein band recognised by MMA-specific antibodies increased in 4 out of the 5 samples analysed (red arrows). We also observed that the intensity of other SDMA and MMA marks decreased in one of the samples (D12, green arrows). GAPDH or actin were used as loading controls. C) Western blot showing the overlap between the protein bands recognised by the FUS (i, left) and SDMA antibodies (ii, middle). The merged image (iii, right) was coloured green for FUS signals and red for SDMA signals, with overlaps in black. Actin was used as a loading control. | |
To further understand the nature of these cross-talk events, we set out to identify the major proteins modified by mMA and sDMA after treatment of GBM tissue with MS023. Using a 13CD3 methyl-SILAC design and mass spectrometry, we identified a total of 316 peptides bearing light- and 19 peptides bearing heavy-mMA modifications in brain tumour lysates (133 and 10 peptides labelled with light- and heavy-mMA groups, respectively, in ‘pool 1’, and 183 and 9 peptides with light- and heavy-mMA groups, respectively, in ‘pool 2’, ESI† Table S2). One such arginine methylation site, R394 in the RNA-binding protein FUS, showed the light/heavy mMA signature of being mono-methylated only in samples treated with MS023, and not in controls, that is, a light mMA modification in ‘pool 1’ in the absence of a heavy mMA modification, and a heavy mMA modification in ‘pool 2’ in the absence of a light mMA modification (Table 2). To test the hypothesis that the 70 kDa sDMA protein band in samples treated with the type I PRMT inhibitor MS023 (red arrows in Fig. 4A) was due to symmetric dimethylation of FUS, we analysed protein lysates using western blot. We found that the protein bands recognised by the sDMA and FUS antibodies overlapped (Fig. 4C).
Table 2 Identification of FUS peptides labelled with 13CD3 while GBM samples were maintained in the perfusion device. Pool 1: lysates from samples treated with light Met and MS023, pooled with lysates from control samples treated with heavy Met. Pool 2: lysates from samples treated with heavy Met and MS023, pooled with lysates from control samples treated with light Met. FUS position indicates the arginine residue identified as methylated in the FUS sequence. Expected MW is the molecular weight of the peptides that would be expected in the absence of any methylation. Observed MW is the actual molecular weight of the peptides, including light or heavy methyl additions. Mass error is the difference between the observed MW and the theoretical molecular weight for modified peptides. −10
log
P indicates the probability of the identification, please note that a −10
log
P of 13 equates to p < 0.05. The methylation site localisation probability is given as the AScore in the last column – the AScore is the −10
log
P of the site localisation probability
Pool |
FUS peptide (P35637) |
FUS position |
Expected MW |
Observed MW |
Mass error |
−10 log P |
AScore |
1 |
GGYGGGGSGGGGR(+14.02)GGFPSGGGGGGGQQR |
407 |
2250.9862 |
2265.002 |
−0.5 |
34.93 |
R13:19.24 |
1 |
GGPMGR(+14.02)GGYGGGGSGGGGR(+14.02) |
394 & 407 |
1549.6803 |
1577.7117 |
1.1 |
24.35 |
R6:1000; R19:1000 |
2 |
GGRGR(+18.04)GGPMGR(+18.04)GGYGGGGSGGGGR |
388 & 394 |
2032.9469 |
2069.0227 |
−0.4 |
24.5 |
R11:5.09 |
Taken together, these results show, firstly, that our perfusion system enables the biochemical modification of proteins in GBM tissue maintained in the devices. Although the number of heavy-labelled peptides was low, this is proof-of-concept that our model preserves cell metabolism (necessary for the synthesis of the methyl donor SAM from 13CD3-Met) and endogenous enzyme activity, and can therefore facilitate molecular investigations of brain tissues ex vivo. Secondly, our results show that cross-talk between type I and type II PRMTs does occur in clinical samples. This is important because PRMT inhibitors are in clinical trials, and cross-talk events could lead to possible off-target side effects. Indeed, the monitoring of any side-effects of PRMT inhibitors is a recognised need,82 in particular in the light of recent investigations of the effect of PRMT inhibitors on platelets83 and the termination of several clinical trials using PRMT1 inhibitors, including GSK3368715 (e.g. NCT03666988, NCT03854227).
FUS is a DNA/RNA binding protein that plays well-known roles in the response to DNA damage and in the regulation of transcription, including alternative RNA splicing. FUS is expressed at high levels in GBM compared with normal tissue, and its expression is associated with poor differentiation, poor prognosis and greater metastasis.84,85 Loss of FUS leads to reduced non-homologous end joining and homologous recombination, as well as to DNA damage.86,87 FUS has been implicated in the pathogenesis of GBM through interactions with microRNAs and long non-coding RNAs (LINC00470 (ref. 88) and ADAMTS9-AS2).89 FUS is thought to regulate the long non-coding RNA TSLNC8 and the microRNA miR-10b05p to ultimately block the Hippo signalling pathway and promote malignancy in glioma cells.90 FUS positively regulates the transcription of SMO-193a.a, a circular RNA that encodes for the Smoothened homolog (SMO) protein, which transduces hedgehog signalling to promote proliferation and self-renewal.91 FUS has also been shown to promote angiogenesis in GBM through upregulation of the circular RNA circ_002136, forming part of a positive feedback loop involving miR-138-5p, SOX13 and SPON2.92
FUS is well-known to be methylated by PRMT1 at several sites,93,94 including at R394.95 Arginine methylation of FUS is associated with the activation of survivin and the inhibition of apoptosis.96 Arginine methylation is generally known to regulate RNA binding proteins interactions with RNA.97 It is tempting to speculate that PRMT1 inhibition leads to the symmetric methylation of FUS and that this contributes to changes in FUS–RNA interactions and to the observed increase in cell apoptosis after treatment (Fig. 3A). It is known that aDMA of FUS can induce cytoplasm–nucleus translocations98 and regulate FUS–protein interactions,99 however, the specific role of sDMA of FUS is unclear. Other groups have found, using cell lines, that the arginine methylation status of FUS changes to sDMA after treatment with PRMT1 inhibitors, including at R394.49,53 FUS is also known to be methylated by PRMT5,100 which facilitates the binding of FUS to p62, a protein involved in autophagy.101 In summary, the switch to sDMA in FUS could contribute to the mechanisms underlying the effects of PRMT inhibitors on GBM cell death. This hypothesis is supported by recent observations of changes in the dimethylation patterns of FUS in cells under stress (oxidative stress, heat stress),102 however, it is not consistent with the lack of synergistic effects between type I and type II PRMT inhibitors in causing cell death (Fig. 3B). It is possible that longer treatment with higher PRMT inhibitor concentrations may result in observable synergistic effects towards increased cell apoptosis. However, this would also push the limits of lab-on-a-chip systems to maintain tissue viability for extended periods of time, and of the clinical relevance of PRMT inhibitor concentrations used.
3.5. Treatment with PRMT inhibitors can induce major changes in the transcriptomic profiles of GBM tissues
To better understand the effects of treating GBM biopsies with PRMT inhibitors, we analysed the transcriptome of biopsies treated with GSK3368715 (1 μM). We analysed four biopsies (D31–D34, Table 1) and performed RNAseq analysis of tissues treated for eight days in the perfusion system, compared to controls (i.e. no treatment). A total of 36
459 transcripts were sequenced at depths between 34 and 60 million reads per sample. Not surprisingly, we observed that the variance between samples was best explained by interpatient heterogeneity (Fig. 5A). A paired analysis found a total of 16
869 DEGs (FC > 1.5) between treated and control samples (Fig. 5B and C). Consistent with the PCA results, the vast majority of DEGs were in samples D32 and D33. It is tempting to speculate that these two samples seemed to respond to treatment with GSK3368715, as opposed to D31 and D34, and further work is warranted to investigate the hypothesis that tissues can be classified between ‘respondent’ and ‘non-respondent’ to PRMT inhibitor treatment. Alternatively, the large number of DEGs in treated samples D32 and D33 could stem from intratumour heterogeneity, although we had controlled for this by pooling tissues from 2–3 devices together from each condition (control or treated).
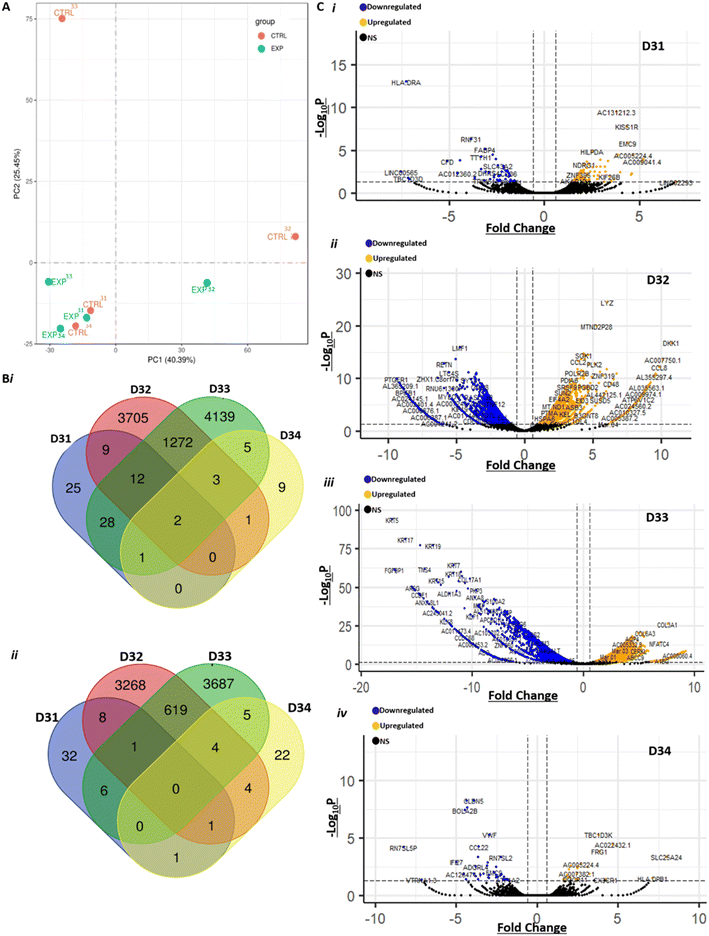 |
| Fig. 5 RNA-seq analysis reveals major effects of treatment with GSK3368715 at a transcriptomic scale. A) Principal component analysis (PCA) of gene expression levels in control (‘CTRL’) and 1 μM-GSK3368715-treated (‘EXP’) GBM tissues, n = 4 (D31-D34). Bi) Venn diagram showing the number of upregulated DEGs in each of the four treated samples, compared to each control counterpart. ii) Venn diagram showing the number of downregulated DEGs in each of the four treated samples, compared to each control counterpart. C) Gene expression after RNA-seq analysis of GBM patient samples, 8 days post-perfusion and treated with 1 μM GSK3368715 vs. DMSO control. Volcano plots created using the EnhancedVolcano package in R4.1.2. Blue data points indicate significantly (padj < 0.05) downregulated (fold change <−1.5) differentially expressed genes (DEGs), yellow data points indicate significantly upregulated (fold change >1.5) DEGs and black data points indicate non-significant gene expression in donor samples i) D31, ii) D32, iii) D33 and iv) D34. | |
For the purpose of analyses of DEGs in the current work, we decided to perform GO term analysis in DEGs identified in two or more treated tissues. In downregulated DEGs, we found large and highly significant enrichments in GO terms associated with the ribosome and translation (ESI† Fig. S6 and Table S3). This indicates decreased protein synthesis capacity after treatment, which is consistent with the observation of cell apoptosis. In upregulated DEGs, we found modest enrichment in terms related to transcription, cell–cell communication, adhesion, migration and cell surface (ESI† Fig. S7 and Table S3). We also found a total of 6171 genes undergoing alternative splicing events in treated compared to control samples (Fig. 6A), which is compatible with a mechanism where changes in the arginine methylation pattern of FUS, a member of the spliceosome, upon GSK3368715 treatment contribute to alternate gene splicing. Mutation of arginine methylation sites in FUS is associated with neurodegenerative diseases, such as amyotrophic lateral sclerosis, and leads to widespread intron retention in RNA-binding proteins.103 Similarly, FUS knockdown leads to hundreds of alternative splicing events in human and mouse brains.104,105 Changes in the arginine methylation patterns of FUS, for example through inhibition of type I PRMTs with GSK3368715, also lead to splicing defects,49,100 largely exon skipping as in our data set,44 including through the regulation of FUS cellular localisation.98,106
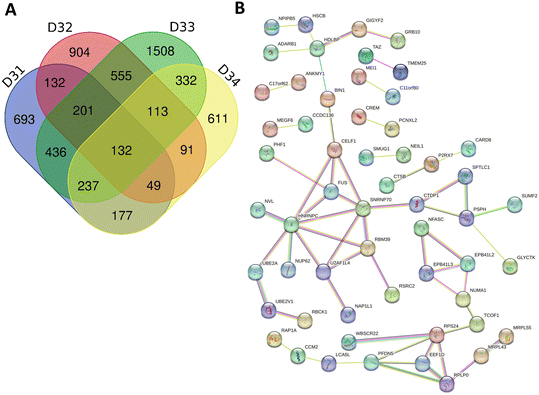 |
| Fig. 6 Treatment with GSK3368715 results in hundreds of alternative splicing events A) Venn diagram showing the number of alternatively spliced genes in each of the four 1 μM GSK3368715 treated samples (D31–D34), compared to each DMSO control counterpart. B) STRING analysis of connections between the genes found to be differentially alternatively spliced in four samples, and FUS. Out of the 132 genes and FUS, only those displaying connections are shown. | |
There are hundreds of known FUS gene splicing targets.104 We asked how many of these were detected in our gene splicing dataset. There were more differential gene-splicing events in D32 and D33 samples but, in contrast to our DEG analysis, also a considerable number in D31 and D34 samples. For consistency, we decided to focus on those events conserved in 2 or more samples. Out of the 2455 genes undergoing differential alternative splicing in two or more treated samples compared to control, 136 were known FUS gene splicing targets (more than expected by chance, χ2 = 7.16, p = 0.007) and 91 had previously been observed after treatment of cell lines with GSK3368712 (structurally similar to GSK3368715).44 STRING network visualisation of the alternatively spliced genes was done, for clarity, on the 132 events conserved in the four samples (Fig. 6B). We found enrichment in annotated keywords related to alternative splicing (KW-0025, 92 of the 132 proteins in our data set vs. a total of 10
179 in the human genome, FDR 3.92e-06) (ESI† Table S4). The broader GO analysis of conserved alternative splicing events in two or more samples revealed enrichment in terms related to DNA damage, RNA processing and cell death (ESI† Table S5). Taken together, our results provide a mechanism to explain how GSK3368715 causes apoptosis of GBM biopsies (Fig. 3A), that is, through modifying RNA processing, gene splicing and protein translation, which are well-known regulators of apoptosis in GBM.107–110
4. Conclusions
The highlights from our work are, first, a novel perfusion device that maintained GBM cell spheroids (Fig. 1) and brain tumour tissue samples in viable conditions for at least eight days, as judged by proliferation, apoptosis, LDH, cytokine and H&E analyses (Fig. 2). Second, our data show that PRMT inhibitors caused a two-fold increase in apoptosis of GBM tissues, compared with the parallel, untreated control (Fig. 3). This opens the door to the use of PRMT inhibitors in the clinical setting against brain tumours, especially as PRMT inhibitors have already been trialled against other cancers. Third, we present clear evidence of cross-talk between aDMA inhibition and the occurrence of protein mMA and sDMA, for the first time in clinical samples (Fig. 4). Finally, we show that treatment of GBM tissues led to hundreds of alternative splicing events (Fig. 6), which were consistent with changes in the arginine methylation status of FUS, and to thousands of DEGs with enrichment in GO terms relevant to apoptosis (Fig. 5). In conclusion, we have advanced the understanding of the effects of PRMT inhibitors on GBM, a disease very much in need of novel treatment strategies, by developing a miniaturised perfusion device that enables the testing of new drugs against brain tumours with timeframes compatible with patient clinical management. Results also suggest that our model can be useful to identify respondents and non-respondents to drugs and therefore the most appropriate and effective treatments against individual tumours at specific times towards precision medicine.
Author contributions
Conceptualization: SA, CR, VG, JG, PBA. Data curation: AB, SFS, IH, AM, LF, CJS, KCWV, ISS, LFS, JG, MAW, PBA. Formal analysis: AB, SFS, IH, AM, LF, CJS, KCWV, ISS, LFS, JG, MAW, PBA. Funding acquisition: AB, SA, CR, VG, LFS, JG, MAW, PBA. Investigation: All. Methodology: All. Resources: SA, CR, LFS, VG, JG, MAW, PBA. Software: AB, SFS, IH, AM, LF, SD, KCWV, VG, LFA, MAW. Supervision: KCWV, ISS, VG, LFS, JG, MAW, PBA. Validation: KCWV, ISS, VG, LFS, JG, MAW, PBA. Writing – original draft: AB, SFS, PBA. Writing – review & editing: All.
Conflicts of interest
There are no conflicts to declare.
Acknowledgements
AB, VG, JG, MW, LFS and PBA are very grateful for a PhD studentship from Yorkshire's Brain Tumour Charity. AB, VG, JG, MW and PBA acknowledge a separate grant from Yorkshire's Brain Tumour Charity (Grant number 21001). SFS, JG and PBA acknowledge a PhD studentship from the Allam Scholarships programme. Mass spectrometry analysis was performed by Dr Adam Dowle and Dr Tony Larson at the Centre for Excellence in Mass Spectrometry at the University of York. We would like to acknowledge Leeds Neuropathology RTB [funded by Yorkshire's Brain Tumour Charity and OSCAR's Paediatric Brain Tumour Charity] as the source of patient derived cell lines. We are grateful to Kath Bulmer for outstanding technical assistance.
Notes and references
- D. N. Louis, A. Perry, P. Wesseling, D. J. Brat, I. A. Cree, D. Figarella-Branger, C. Hawkins, H. K. Ng, S. M. Pfister, G. Reifenberger, R. Soffietti, A. von Deimling and D. W. Ellison, Neuro. Oncol., 2021, 23, 1231–1251 CrossRef CAS PubMed.
- J. A. Schwartzbaum, J. L. Fisher, K. D. Aldape and M. Wrensch, Nat. Clin. Pract. Neurol., 2006, 2, 494–503 CrossRef PubMed ; quiz 491 p following 516.
- P. Y. Wen, M. Weller, E. Q. Lee, B. M. Alexander, J. S. Barnholtz-Sloan, F. P. Barthel, T. T. Batchelor, R. S. Bindra, S. M. Chang, E. A. Chiocca, T. F. Cloughesy, J. F. DeGroot, E. Galanis, M. R. Gilbert, M. E. Hegi, C. Horbinski, R. Y. Huang, A. B. Lassman, E. Le Rhun, M. Lim, M. P. Mehta, I. K. Mellinghoff, G. Minniti, D. Nathanson, M. Platten, M. Preusser, P. Roth, M. Sanson, D. Schiff, S. C. Short, M. J. B. Taphoorn, J. C. Tonn, J. Tsang, R. G. W. Verhaak, A. von Deimling, W. Wick, G. Zadeh, D. A. Reardon, K. D. Aldape and M. J. van den Bent, Neuro. Oncol., 2020, 22, 1073–1113 CrossRef CAS PubMed.
- O. G. Taylor, J. S. Brzozowski and K. A. Skelding, Front. Oncol., 2019, 9, 963 CrossRef PubMed.
- Q. T. Ostrom, H. Gittleman, P. Farah, A. Ondracek, Y. Chen, Y. Wolinsky, N. E. Stroup, C. Kruchko and J. S. Barnholtz-Sloan, Neuro. Oncol., 2013, 15 Suppl 2, ii1-56 Search PubMed.
- E. Ladomersky, D. M. Scholtens, M. Kocherginsky, E. A. Hibler, E. T. Bartom, S. Otto-Meyer, L. Zhai, K. L. Lauing, J. Choi, J. A. Sosman, J. D. Wu, B. Zhang, R. V. Lukas and D. A. Wainwright, Front. Pharmacol., 2019, 10, 200 CrossRef CAS PubMed.
- P. Zhu, X. L. Du, G. Lu and J. J. Zhu, Oncotarget, 2017, 8, 44015–44031 CrossRef PubMed.
- P. D. Inskip, R. E. Tarone, E. E. Hatch, T. C. Wilcosky, W. R. Shapiro, R. G. Selker, H. A. Fine, P. M. Black, J. S. Loeffler and M. S. Linet, N. Engl. J. Med., 2001, 344, 79–86 CrossRef CAS PubMed.
- M. L. Bondy, M. E. Scheurer, B. Malmer, J. S. Barnholtz-Sloan, F. G. Davis, D. Il'yasova, C. Kruchko, B. J. McCarthy, P. Rajaraman, J. A. Schwartzbaum, S. Sadetzki, B. Schlehofer, T. Tihan, J. L. Wiemels, M. Wrensch, P. A. Buffler and C. Brain Tumor Epidemiology, Cancer, 2008, 113, 1953–1968 CrossRef PubMed.
- G. Prasad and D. A. Haas-Kogan, Expert Rev. Neurother., 2009, 9, 1511–1517 CrossRef CAS PubMed.
- A. Lanese, E. Franceschi and A. A. Brandes, Oncol. Ther., 2018, 6, 105–108 CrossRef PubMed.
- F. D'Angelo, M. Ceccarelli, Tala, L. Garofano, J. Zhang, V. Frattini, F. P. Caruso, G. Lewis, K. D. Alfaro, L. Bauchet, G. Berzero, D. Cachia, M. Cangiano, L. Capelle, J. de Groot, F. DiMeco, F. Ducray, W. Farah, G. Finocchiaro, S. Goutagny, C. Kamiya-Matsuoka, C. Lavarino, H. Loiseau, V. Lorgis, C. E. Marras, I. McCutcheon, D. H. Nam, S. Ronchi, V. Saletti, R. Seizeur, J. Slopis, M. Sunol, F. Vandenbos, P. Varlet, D. Vidaud, C. Watts, V. Tabar, D. E. Reuss, S. K. Kim, D. Meyronet, K. Mokhtari, H. Salvador, K. P. Bhat, M. Eoli, M. Sanson, A. Lasorella and A. Iavarone, Nat. Med., 2019, 25, 176–187 CrossRef PubMed.
- R. V. Lukas, D. A. Wainwright, E. Ladomersky, S. Sachdev, A. M. Sonabend and R. Stupp, Oncology, 2019, 33, 91–100 Search PubMed.
- J. M. Bark, A. Kulasinghe, B. Chua, B. W. Day and C. Punyadeera, Br. J. Cancer, 2020, 122, 295–305 CrossRef PubMed.
- R. Stupp, M. E. Hegi, W. P. Mason, M. J. van den Bent, M. J. Taphoorn, R. C. Janzer, S. K. Ludwin, A. Allgeier, B. Fisher, K. Belanger, P. Hau, A. A. Brandes, J. Gijtenbeek, C. Marosi, C. J. Vecht, K. Mokhtari, P. Wesseling, S. Villa, E. Eisenhauer, T. Gorlia, M. Weller, D. Lacombe, J. G. Cairncross, R. O. Mirimanoff, R. European Organisation for, T. Treatment of Cancer Brain, G. Radiation Oncology and G. National Cancer Institute of Canada Clinical Trials, Lancet Oncol., 2009, 10, 459–466 CrossRef CAS PubMed.
- M. Glas, B. H. Rath, M. Simon, R. Reinartz, A. Schramme, D. Trageser, R. Eisenreich, A. Leinhaas, M. Keller, H. U. Schildhaus, S. Garbe, B. Steinfarz, T. Pietsch, D. A. Steindler, J. Schramm, U. Herrlinger, O. Brustle and B. Scheffler, Ann. Neurol., 2010, 68, 264–269 Search PubMed.
- R. Stupp, W. P. Mason, M. J. van den Bent, M. Weller, B. Fisher, M. J. Taphoorn, K. Belanger, A. A. Brandes, C. Marosi, U. Bogdahn, J. Curschmann, R. C. Janzer, S. K. Ludwin, T. Gorlia, A. Allgeier, D. Lacombe, J. G. Cairncross, E. Eisenhauer, R. O. Mirimanoff, R. European Organisation for, T. Treatment of Cancer Brain, G. Radiotherapy and G. National Cancer Institute of Canada Clinical Trials, N. Engl. J. Med., 2005, 352, 987–996 CrossRef CAS PubMed.
- X. Liu, Q. Su, X. Zhang, W. Yang, J. Ning, K. Jia, J. Xin, H. Li, L. Yu, Y. Liao and D. Zhang, Biosensors, 2022, 12, 1045 CrossRef CAS PubMed.
- M. Astolfi, B. Peant, M. A. Lateef, N. Rousset, J. Kendall-Dupont, E. Carmona, F. Monet, F. Saad, D. Provencher, A. M. Mes-Masson and T. Gervais, Lab Chip, 2016, 16, 312–325 RSC.
- F. Gioiella, F. Urciuolo, G. Imparato, V. Brancato and P. A. Netti, Adv. Healthcare Mater., 2016, 5, 3074–3084 CrossRef CAS PubMed.
- B. A. Hassell, G. Goyal, E. Lee, A. Sontheimer-Phelps, O. Levy, C. S. Chen and D. E. Ingber, Cell Rep., 2017, 21, 508–516 CrossRef CAS PubMed.
- X. Yang, K. Li, X. Zhang, C. Liu, B. Guo, W. Wen and X. Gao, Lab Chip, 2018, 18, 486–495 RSC.
- A. D. Rodriguez, L. F. Horowitz, K. Castro, H. Kenerson, N. Bhattacharjee, G. Gandhe, A. Raman, R. J. Monnat, R. Yeung, R. C. Rostomily and A. Folch, Lab Chip, 2020, 20, 1658–1675 RSC.
- A. Riley, H. Jones, J. England, D. Kuvshinov, V. Green and J. Greenman, Oncol. Lett., 2021, 22, 780 CrossRef CAS PubMed.
- V. L. Green, A. Michno, J. Greenman and N. D. Stafford, Results Immunol., 2011, 2, 1–6 Search PubMed.
- R. Bower, V. L. Green, E. Kuvshinova, D. Kuvshinov, L. Karsai, S. T. Crank, N. D. Stafford and J. Greenman, Future Sci. OA, 2017, 3, FSO174 CrossRef CAS PubMed.
- R. Cheah, R. Srivastava, N. D. Stafford, A. W. Beavis, V. Green and J. Greenman, Int. J. Oncol., 2017, 51, 1227–1238 CrossRef CAS PubMed.
- S. M. Hattersley, D. C. Sylvester, C. E. Dyer, N. D. Stafford, S. J. Haswell and J. Greenman, Ann. Biomed. Eng., 2012, 40, 1277–1288 CrossRef PubMed.
- A. J. Blake, T. M. Pearce, N. S. Rao, S. M. Johnson and J. C. Williams, Lab Chip, 2007, 7, 842–849 RSC.
- Y. Choi, M. A. McClain, M. C. LaPlaca, A. B. Frazier and M. G. Allen, Biomed. Microdevices, 2007, 9, 7–13 CrossRef PubMed.
- T. C. Chang, A. M. Mikheev, W. Huynh, R. J. Monnat, R. C. Rostomily and A. Folch, Lab Chip, 2014, 14, 4540–4551 RSC.
- A. N. Cho, Y. Jin, Y. An, J. Kim, Y. S. Choi, J. S. Lee, J. Kim, W. Y. Choi, D. J. Koo, W. Yu, G. E. Chang, D. Y. Kim, S. H. Jo, J. Kim, S. Y. Kim, Y. G. Kim, J. Y. Kim, N. Choi, E. Cheong, Y. J. Kim, H. S. Je, H. C. Kang and S. W. Cho, Nat. Commun., 2021, 12, 4730 CrossRef CAS PubMed.
- F. Olubajo, S. Achawal and J. Greenman, Transl. Oncol., 2020, 13, 1–10 CrossRef PubMed.
- X. Cui, C. Ma, V. Vasudevaraja, J. Serrano, J. Tong, Y. Peng, M. Delorenzo, G. Shen, J. Frenster, R. T. Morales, W. Qian, A. Tsirigos, A. S. Chi, R. Jain, S. C. Kurz, E. P. Sulman, D. G. Placantonakis, M. Snuderl and W. Chen, eLife, 2020, 9, e52253 CrossRef CAS PubMed.
- D. M. Leite, B. Z. Baskovic, P. Civita, C. Neto, M. Gumbleton and G. J. Pilkington, FASEB J., 2020, 34, 1710–1727 CrossRef CAS PubMed.
- T. Amemiya, N. Hata, M. Mizoguchi, R. Yokokawa, Y. Kawamura, R. Hatae, Y. Sangatsuda, D. Kuga, Y. Fujioka, K. Takigawa, Y. Akagi, K. Yoshimoto, K. Iihara and T. Miura, Mol. Biol. Rep., 2021, 48, 395–403 CrossRef CAS PubMed.
- I. Pediaditakis, K. R. Kodella, D. V. Manatakis, C. Y. Le, S. Barthakur, A. Sorets, A. Gravanis, L. Ewart, L. L. Rubin, E. S. Manolakos, C. D. Hinojosa and K. Karalis, iScience, 2022, 25, 104813 CrossRef CAS PubMed.
- I. Pediaditakis, K. R. Kodella, D. V. Manatakis, C. Y. Le, C. D. Hinojosa, W. Tien-Street, E. S. Manolakos, K. Vekrellis, G. A. Hamilton, L. Ewart, L. L. Rubin and K. Karalis, Nat. Commun., 2021, 12, 5907 CrossRef CAS PubMed.
- F. S. Varn, K. C. Johnson, J. Martinek, J. T. Huse, M. P. Nasrallah, P. Wesseling, L. A. D. Cooper, T. M. Malta, T. E. Wade, T. S. Sabedot, D. Brat, P. V. Gould, A. Woehrer, K. Aldape, A. Ismail, S. K. Sivajothi, F. P. Barthel, H. Kim, E. Kocakavuk, N. Ahmed, K. White, I. Datta, H. E. Moon, S. Pollock, C. Goldfarb, G. H. Lee, L. Garofano, K. J. Anderson, D. Nehar-Belaid, J. S. Barnholtz-Sloan, S. Bakas, A. T. Byrne, F. D'Angelo, H. K. Gan, M. Khasraw, S. Migliozzi, D. R. Ormond, S. H. Paek, E. G. Van Meir, A. M. E. Walenkamp, C. Watts, T. Weiss, M. Weller, K. Palucka, L. F. Stead, L. M. Poisson, H. Noushmehr, A. Iavarone, R. G. W. Verhaak and G. Consortium, Cell, 2022, 185, 2184–2199 e2116 CrossRef CAS PubMed.
- F. P. Barthel, K. C. Johnson, F. S. Varn, A. D. Moskalik, G. Tanner, E. Kocakavuk, K. J. Anderson, O. Abiola, K. Aldape, K. D. Alfaro, D. Alpar, S. B. Amin, D. M. Ashley, P. Bandopadhayay, J. S. Barnholtz-Sloan, R. Beroukhim, C. Bock, P. K. Brastianos, D. J. Brat, A. R. Brodbelt, A. F. Bruns, K. R. Bulsara, A. Chakrabarty, A. Chakravarti, J. H. Chuang, E. B. Claus, E. J. Cochran, J. Connelly, J. F. Costello, G. Finocchiaro, M. N. Fletcher, P. J. French, H. K. Gan, M. R. Gilbert, P. V. Gould, M. R. Grimmer, A. Iavarone, A. Ismail, M. D. Jenkinson, M. Khasraw, H. Kim, M. C. M. Kouwenhoven, P. S. LaViolette, M. Li, P. Lichter, K. L. Ligon, A. K. Lowman, T. M. Malta, T. Mazor, K. L. McDonald, A. M. Molinaro, D. H. Nam, N. Nayyar, H. K. Ng, C. Y. Ngan, S. P. Niclou, J. M. Niers, H. Noushmehr, J. Noorbakhsh, D. R. Ormond, C. K. Park, L. M. Poisson, R. Rabadan, B. Radlwimmer, G. Rao, G. Reifenberger, J. K. Sa, M. Schuster, B. L. Shaw, S. C. Short, P. A. S. Smitt, A. E. Sloan, M. Smits, H. Suzuki, G. Tabatabai, E. G. Van Meir, C. Watts, M. Weller, P. Wesseling, B. A. Westerman, G. Widhalm, A. Woehrer, W. K. A. Yung, G. Zadeh, J. T. Huse, J. F. De Groot, L. F. Stead, R. G. W. Verhaak and G. Consortium, Nature, 2019, 576, 112–120 CrossRef CAS PubMed.
- S. Valtorta, A. Lo Dico, I. Raccagni, D. Gaglio, S. Belloli, L. S. Politi, C. Martelli, C. Diceglie, M. Bonanomi, G. Ercoli, V. Vaira, L. Ottobrini and R. M. Moresco, Oncotarget, 2017, 8, 113090–113104 CrossRef PubMed.
- S. P. C. Hsu, J. S. Kuo, H. C. Chiang, H. E. Wang, Y. S. Wang, C. C. Huang, Y. C. Huang, M. S. Chi, M. P. Mehta and K. H. Chi, Oncotarget, 2018, 9, 6883–6896 CrossRef PubMed.
- C. T. Stackhouse, J. C. Anderson, Z. Yue, T. Nguyen, N. J. Eustace, C. P. Langford, J. Wang, J. R. T. Rowland, C. Xing, F. M. Mikhail, X. Cui, H. Alrefai, R. E. Bash, K. J. Lee, E. S. Yang, A. B. Hjelmeland, C. R. Miller, J. Y. Chen, G. Y. Gillespie and C. D. Willey, JCI Insight, 2022, 7, e148717 CrossRef PubMed.
- D. O. Onwuli and P. Beltran-Alvarez, Amino Acids, 2016, 48, 641–651 CrossRef CAS PubMed.
- R. S. Blanc and S. Richard, Mol. Cell, 2017, 65, 8–24 CrossRef CAS PubMed.
- C. I. Zurita-Lopez, T. Sandberg, R. Kelly and S. G. Clarke, J. Biol. Chem., 2012, 287, 7859–7870 CrossRef CAS PubMed.
- J. Tang, A. Frankel, R. J. Cook, S. Kim, W. K. Paik, K. R. Williams, S. Clarke and H. R. Herschman, J. Biol. Chem., 2000, 275, 7723–7730 CrossRef CAS PubMed.
- J. Jarrold and C. C. Davies, Trends Mol. Med., 2019, 25, 993–1009 CrossRef CAS PubMed.
- A. Fedoriw, S. R. Rajapurkar, S. O'Brien, S. V. Gerhart, L. H. Mitchell, N. D. Adams, N. Rioux, T. Lingaraj, S. A. Ribich, M. B. Pappalardi, N. Shah, J. Laraio, Y. Liu, M. Butticello, C. L. Carpenter, C. Creasy, S. Korenchuk, M. T. McCabe, C. F. McHugh, R. Nagarajan, C. Wagner, F. Zappacosta, R. Annan, N. O. Concha, R. A. Thomas, T. K. Hart, J. J. Smith, R. A. Copeland, M. P. Moyer, J. Campbell, K. Stickland, J. Mills, S. Jacques-O'Hagan, C. Allain, D. Johnston, A. Raimondi, M. P. Scott, N. Waters, K. Swinger, A. Boriack-Sjodin, T. Riera, G. Shapiro, R. Chesworth, R. K. Prinjha, R. G. Kruger, O. Barbash and H. P. Mohammad, Cancer Cell, 2019, 36, 100–114 e125 CrossRef CAS PubMed.
- A. Fedoriw, L. Shi, S. O'Brien, K. N. Smitheman, Y. Wang, J. Hou, C. Sherk, S. Rajapurkar, J. Laraio, L. J. Williams, C. Xu, G. Han, Q. Feng, M. T. Bedford, L. Wang, O. Barbash, R. G. Kruger, P. Hwu, H. P. Mohammad and W. Peng, Cancer Immunol. Res., 2022, 10, 420–436 CrossRef CAS PubMed.
- S. F. Samuel, A. Barry, J. Greenman and P. Beltran-Alvarez, Amino Acids, 2021, 53, 489–506 CrossRef CAS PubMed.
- M. S. Eram, Y. Shen, M. Szewczyk, H. Wu, G. Senisterra, F. Li, K. V. Butler, H. U. Kaniskan, B. A. Speed, C. Dela Sena, A. Dong, H. Zeng, M. Schapira, P. J. Brown, C. H. Arrowsmith, D. Barsyte-Lovejoy, J. Liu, M. Vedadi and J. Jin, ACS Chem. Biol., 2016, 11, 772–781 CrossRef CAS PubMed.
- J. Y. Fong, L. Pignata, P. A. Goy, K. C. Kawabata, S. C. Lee, C. M. Koh, D. Musiani, E. Massignani, A. G. Kotini, A. Penson, C. M. Wun, Y. Shen, M. Schwarz, D. H. Low, A. Rialdi, M. Ki, H. Wollmann, S. Mzoughi, F. Gay, C. Thompson, T. Hart, O. Barbash, G. M. Luciani, M. M. Szewczyk, B. J. Wouters, R. Delwel, E. P. Papapetrou, D. Barsyte-Lovejoy, C. H. Arrowsmith, M. D. Minden, J. Jin, A. Melnick, T. Bonaldi, O. Abdel-Wahab and E. Guccione, Cancer Cell, 2019, 36, 194–209 e199 CrossRef CAS PubMed.
- G. Gao, L. Zhang, O. D. Villarreal, W. He, D. Su, E. Bedford, P. Moh, J. Shen, X. Shi, M. T. Bedford and H. Xu, Nucleic Acids Res., 2019, 47, 5038–5048 CrossRef CAS PubMed.
- D. Musiani, J. Bok, E. Massignani, L. Wu, T. Tabaglio, M. R. Ippolito, A. Cuomo, U. Ozbek, H. Zorgati, U. Ghoshdastider, R. C. Robinson, E. Guccione and T. Bonaldi, Sci. Signaling, 2019, 12, eaat8388 CrossRef CAS PubMed.
- N. G. Hartel, B. Chew, J. Qin, J. Xu and N. A. Graham, Mol. Cell. Proteomics, 2019, 18, 2149–2164 CrossRef PubMed.
- S. F. Samuel, A. J. Marsden, S. Deepak, F. Rivero, J. Greenman and P. Beltran-Alvarez, Proteomes, 2018, 6(4), 44 CrossRef CAS PubMed.
- D. O. Onwuli, L. Rigau-Roca, C. Cawthorne and P. Beltran-Alvarez, Proteomics: Clin. Appl., 2017, 11, 1 Search PubMed.
- A. Guo, H. Gu, J. Zhou, D. Mulhern, Y. Wang, K. A. Lee, V. Yang, M. Aguiar, J. Kornhauser, X. Jia, J. Ren, S. A. Beausoleil, J. C. Silva, V. Vemulapalli, M. T. Bedford and M. J. Comb, Mol. Cell. Proteomics, 2014, 13, 372–387 CrossRef CAS PubMed.
- D. O. Onwuli, S. F. Samuel, P. Sfyri, K. Welham, M. Goddard, Y. Abu-Omar, M. Loubani, F. Rivero, A. Matsakas, D. M. Benoit, M. Wade, J. Greenman and P. Beltran-Alvarez, Int. J. Cardiol., 2019, 282, 76–80 CrossRef PubMed.
- A. Mortazavi, B. A. Williams, K. McCue, L. Schaeffer and B. Wold, Nat. Methods, 2008, 5, 621–628 CrossRef CAS PubMed.
- S. Anders and W. Huber, Genome Biol., 2010, 11, R106 CrossRef CAS PubMed.
- S. X. Ge, D. Jung and R. Yao, Bioinformatics, 2020, 36, 2628–2629 CrossRef CAS PubMed.
- E. Eden, R. Navon, I. Steinfeld, D. Lipson and Z. Yakhini, BMC Bioinf., 2009, 10, 48 CrossRef PubMed.
- L. Cen, B. L. Carlson, M. A. Schroeder, J. L. Ostrem, G. J. Kitange, A. C. Mladek, S. R. Fink, P. A. Decker, W. Wu, J. S. Kim, T. Waldman, R. B. Jenkins and J. N. Sarkaria, Neuro. Oncol., 2012, 14, 870–881 CrossRef CAS PubMed.
- Y. T. Yeung, K. L. McDonald, T. Grewal and L. Munoz, Br. J. Pharmacol., 2013, 168, 591–606 CrossRef CAS PubMed.
- D. Zagzag, Y. Lukyanov, L. Lan, M. A. Ali, M. Esencay, O. Mendez, H. Yee, E. B. Voura and E. W. Newcomb, Lab. Invest., 2006, 86, 1221–1232 CrossRef CAS PubMed.
- F. Torrisi, C. Alberghina, S. D'Aprile, A. M. Pavone, L. Longhitano, S. Giallongo, D. Tibullo, M. Di Rosa, A. Zappala, F. P. Cammarata, G. Russo, M. Ippolito, G. Cuttone, G. Li Volti, N. Vicario and R. Parenti, Biomedicines, 2022, 10(4), 806 CrossRef CAS PubMed.
- O. Uchenunu, M. Pollak, I. Topisirovic and L. Hulea, J. Mol. Endocrinol., 2019, 62, R83–R103 CAS.
- S. D. Carr, V. L. Green, N. D. Stafford and J. Greenman, Otolaryngol.--Head Neck Surg., 2014, 150, 73–80 CrossRef PubMed.
- S. M. Hattersley, C. E. Dyer, J. Greenman and S. J. Haswell, Lab Chip, 2008, 8, 1842–1846 RSC.
- A. Dawson, C. Dyer, J. Macfie, J. Davies, L. Karsai, J. Greenman and M. Jacobsen, Biomicrofluidics, 2016, 10, 064101 CrossRef CAS PubMed.
- B. M. Alexander, N. Pinnell, P. Y. Wen and A. D'Andrea, J. Neurooncol., 2012, 107, 463–477 CrossRef CAS PubMed.
- T. Kanzawa, I. M. Germano, T. Komata, H. Ito, Y. Kondo and S. Kondo, Cell Death Differ., 2004, 11, 448–457 CrossRef CAS PubMed.
- M. Urulangodi and A. Mohanty, J. Cell Commun. Signaling, 2020, 14, 31–45 CrossRef PubMed.
- C. Dominici, N. Sgarioto, Z. Yu, L. Sesma-Sanz, J. Y. Masson, S. Richard and N. J. Raynal, Clin. Epigenet., 2021, 13, 54 CrossRef CAS PubMed.
- X. Xu, F. Stockhammer, A. Schmitt, R. Casalegno-Garduno, A. Enders, J. Mani, C. F. Classen, M. Linnebacher, M. Freund and M. Schmitt, Int. J. Oncol., 2012, 40, 764–772 CAS.
- M. S. Bobola, D. D. Kolstoe, A. Blank and J. R. Silber, Mol. Cancer Ther., 2010, 9, 1208–1218 CrossRef CAS PubMed.
- J. Portnow, B. Badie, M. Chen, A. Liu, S. Blanchard and T. W. Synold, Clin. Cancer Res., 2009, 15, 7092–7098 CrossRef CAS PubMed.
- J. M. de Hoyos-Vega, A. M. Gonzalez-Suarez and J. L. Garcia-Cordero, Microsyst. Nanoeng., 2020, 6, 40 CrossRef CAS PubMed.
- L. F. Horowitz, A. D. Rodriguez, Z. Dereli-Korkut, R. Lin, K. Castro, A. M. Mikheev, R. J. Monnat Jr., A. Folch and R. C. Rostomily, npj Precis. Oncol., 2020, 4, 12 CrossRef CAS PubMed.
- H. Hu, K. Qian, M. C. Ho and Y. G. Zheng, Expert Opin. Invest. Drugs, 2016, 25, 335–358 CrossRef CAS PubMed.
- A. J. Marsden, D. R. J. Riley, A. Barry, J. S. Khalil, B. A. Guinn, N. T. Kemp, F. Rivero and P. Beltran-Alvarez, ACS Pharmacol. Transl. Sci., 2021, 4, 1567–1577 CrossRef CAS PubMed.
- D. Xiong, Y. B. Wu, C. Jin, J. J. Li, J. Gu, Y. F. Liao, X. Long, S. Q. Zhu, H. B. Wu, J. J. Xu and J. Y. Ding, Oncol. Lett., 2018, 16, 1791–1800 Search PubMed.
- H. Zhu, T. Zheng, J. Yu, L. Zhou and L. Wang, Biomed. Pharmacother., 2018, 105, 789–797 CrossRef CAS PubMed.
- H. Wang, W. Guo, J. Mitra, P. M. Hegde, T. Vandoorne, B. J. Eckelmann, S. Mitra, A. E. Tomkinson, L. Van Den Bosch and M. L. Hegde, Nat. Commun., 2018, 9, 3683 CrossRef PubMed.
- H. Deng, K. Gao and J. Jankovic, Nat. Rev. Neurol., 2014, 10, 337–348 CrossRef CAS PubMed.
- C. Liu, Y. Zhang, X. She, L. Fan, P. Li, J. Feng, H. Fu, Q. Liu, Q. Liu, C. Zhao, Y. Sun and M. Wu, J. Hematol. Oncol., 2018, 11, 77 CrossRef PubMed.
- Y. Yan, Z. Xu, X. Chen, X. Wang, S. Zeng, Z. Zhao, L. Qian, Z. Li, J. Wei, L. Huo, X. Li, Z. Gong and L. Sun, Front. Cell Dev. Biol., 2019, 7, 217 CrossRef PubMed.
- Y. Yang, X. Liu, J. Zheng, Y. Xue, L. Liu, J. Ma, P. Wang, C. Yang, D. Wang, L. Shao, X. Ruan and Y. Liu, Mol. Oncol., 2020, 14, 2936–2959 CrossRef CAS PubMed.
- X. Wu, S. Xiao, M. Zhang, L. Yang, J. Zhong, B. Li, F. Li, X. Xia, X. Li, H. Zhou, D. Liu, N. Huang, X. Yang, F. Xiao and N. Zhang, Genome Biol., 2021, 22, 33 CrossRef CAS PubMed.
- Z. He, X. Ruan, X. Liu, J. Zheng, Y. Liu, L. Liu, J. Ma, L. Shao, D. Wang, S. Shen, C. Yang and Y. Xue, J. Exp. Clin. Cancer Res., 2019, 38, 65 CrossRef PubMed.
- J. Rappsilber, W. J. Friesen, S. Paushkin, G. Dreyfuss and M. Mann, Anal. Chem., 2003, 75, 3107–3114 CrossRef CAS PubMed.
- K. Du, S. Arai, T. Kawamura, A. Matsushita and R. Kurokawa, Biochem. Biophys. Res. Commun., 2011, 404, 991–996 CrossRef CAS PubMed.
- E. Massignani, R. Giambruno, M. Maniaci, L. Nicosia, A. Yadav, A. Cuomo, F. Raimondi and T. Bonaldi, Mol. Cell. Proteomics, 2022, 21, 100243 CrossRef CAS PubMed.
- P. K. Jaiswal, A. Goel and R. D. Mittal, Indian J. Med. Res., 2015, 141, 389–397 CrossRef PubMed.
- M. Maniaci, F. L. Boffo, E. Massignani and T. Bonaldi, Front. Mol. Biosci., 2021, 8, 688973 CrossRef CAS PubMed.
- M. L. Tradewell, Z. Yu, M. Tibshirani, M. C. Boulanger, H. D. Durham and S. Richard, Hum. Mol. Genet., 2012, 21, 136–149 CrossRef PubMed.
- S. Qamar, G. Wang, S. J. Randle, F. S. Ruggeri, J. A. Varela, J. Q. Lin, E. C. Phillips, A. Miyashita, D. Williams, F. Strohl, W. Meadows, R. Ferry, V. J. Dardov, G. G. Tartaglia, L. A. Farrer, G. S. K. Schierle, C. F. Kaminski, C. E. Holt, P. E. Fraser, G. Schmitt-Ulms, D. Klenerman, T. Knowles, M. Vendruscolo and P. St George-Hyslop, Cell, 2018, 173, 720–734 e715 CrossRef CAS PubMed.
- M. Chitiprolu, C. Jagow, V. Tremblay, E. Bondy-Chorney, G. Paris, A. Savard, G. Palidwor, F. A. Barry, L. Zinman, J. Keith, E. Rogaeva, J. Robertson, M. Lavallee-Adam, J. Woulfe, J. F. Couture, J. Cote and D. Gibbings, Nat. Commun., 2018, 9, 2794 CrossRef PubMed.
- T. E. Rusten and H. Stenmark, Nat. Cell Biol., 2010, 12, 207–209 CrossRef CAS PubMed.
- Q. Wang, Z. Li, S. Zhang, Y. Li, Y. Wang, Z. Fang, Y. Ma, Z. Liu, W. Zhang, D. Li, C. Liu and M. Ye, Proc. Natl. Acad. Sci. U. S. A., 2022, 119, e2205255119 CrossRef CAS PubMed.
- J. Humphrey, N. Birsa, C. Milioto, M. McLaughlin, A. M. Ule, D. Robaldo, A. B. Eberle, R. Krauchi, M. Bentham, A. L. Brown, S. Jarvis, C. Bodo, M. G. Garone, A. Devoy, G. Soraru, A. Rosa, I. Bozzoni, E. M. C. Fisher, O. Muhlemann, G. Schiavo, M. D. Ruepp, A. M. Isaacs, V. Plagnol and P. Fratta, Nucleic Acids Res., 2020, 48, 6889–6905 CrossRef CAS PubMed.
- T. Nakaya, P. Alexiou, M. Maragkakis, A. Chang and Z. Mourelatos, RNA, 2013, 19, 498–509 CrossRef CAS PubMed.
- B. Rogelj, L. E. Easton, G. K. Bogu, L. W. Stanton, G. Rot, T. Curk, B. Zupan, Y. Sugimoto, M. Modic, N. Haberman, J. Tollervey, R. Fujii, T. Takumi, C. E. Shaw and J. Ule, Sci. Rep., 2012, 2, 603 CrossRef PubMed.
- M. J. Finelli, K. X. Liu, Y. Wu, P. L. Oliver and K. E. Davies, Hum. Mol. Genet., 2015, 24, 3529–3544 CrossRef CAS PubMed.
- F. M. Meliso, C. G. Hubert, P. A. Favoretto Galante and L. O. Penalva, Hum. Genet., 2017, 136, 1129–1141 CrossRef PubMed.
- A. Dome, M. Dymova, V. Richter and G. Stepanov, Int. J. Mol. Sci., 2022, 23(16), 9272 CrossRef CAS PubMed.
- S. Mukherjee, U. Kundu, D. Desai and P. P. Pillai, J. Mol. Neurosci., 2022, 72, 2188–2206 CrossRef CAS PubMed.
- S. Ponnala, K. K. Veeravalli, C. Chetty, D. H. Dinh and J. S. Rao, PLoS One, 2011, 6, e26191 CrossRef CAS PubMed.
Footnotes |
† Electronic supplementary information (ESI) available. See DOI: https://doi.org/10.1039/d3lc00204g |
‡ Contributed equally as first authors. |
§ Current address: Department of Materials and Environmental Chemistry, Stockholm University. |
¶ Contributed equally as corresponding authors. |
|
This journal is © The Royal Society of Chemistry 2023 |