DOI:
10.1039/D2LC00856D
(Paper)
Lab Chip, 2023,
23, 1561-1575
Virtual-freezing fluorescence imaging flow cytometry with 5-aminolevulinic acid stimulation and antibody labeling for detecting all forms of circulating tumor cells†
Received
16th September 2022
, Accepted 3rd January 2023
First published on 10th January 2023
Abstract
Circulating tumor cells (CTCs) are precursors to cancer metastasis. In blood circulation, they take various forms such as single CTCs, CTC clusters, and CTC–leukocyte clusters, all of which have unique characteristics in terms of physiological function and have been a subject of extensive research in the last several years. Unfortunately, conventional methods are limited in accurately analysing the highly heterogeneous nature of CTCs. Here we present an effective strategy for simultaneously analysing all forms of CTCs in blood by virtual-freezing fluorescence imaging (VIFFI) flow cytometry with 5-aminolevulinic acid (5-ALA) stimulation and antibody labeling. VIFFI is an optomechanical imaging method that virtually freezes the motion of fast-flowing cells on an image sensor to enable high-throughput yet sensitive imaging of every single event. 5-ALA stimulates cancer cells to induce the accumulation of protoporphyrin (PpIX), a red fluorescent substance, making it possible to detect all cancer cells even if they show no expression of the epithelial cell adhesion molecule, a typical CTC biomarker. Although PpIX signals are generally weak, VIFFI flow cytometry can detect them by virtue of its high sensitivity. As a proof-of-principle demonstration of the strategy, we applied cancer cells spiked in blood to the strategy to demonstrate image-based detection and accurate classification of single cancer cells, clusters of cancer cells, and clusters of a cancer cell(s) and a leukocyte(s). To show the clinical utility of our method, we used it to evaluate blood samples of four breast cancer patients and four healthy donors and identified EpCAM-positive PpIX-positive cells in one of the patient samples. Our work paves the way toward the determination of cancer prognosis, the guidance and monitoring of treatment, and the design of antitumor strategies for cancer patients.
1. Introduction
Circulating tumor cells (CTCs) are malignant cells that originate from a primary tumor, circulate in the bloodstream, migrate to a secondary site, and become seeds for the subsequent growth of additional tumors.1 This cancer spread or metastasis is known to be a major cause of cancer-related deaths. The presence and number of CTCs are thought to be associated with prognosis and may be involved in the intermediate stage of tumor metastasis.2,3 Efforts have been made to identify and characterize CTCs in cancer patients due to their tremendous potential for the diagnosis and treatment of cancer via liquid biopsy.4 In the circulatory system, CTCs take various forms such as single CTCs, CTC clusters, CTC–leukocyte clusters, and CTC–platelet clusters, all of which have unique characteristics in terms of physiological function and have been a subject of extensive research for the past several years.5–7 Recent reports show that CTC clusters and CTC–neutrophil clusters from cancer patients have higher metastatic potential than single CTCs.5,7 Therefore, the capture and analysis of all forms of CTCs in liquid biopsy can provide us with a bigger picture of the metastatic process and could advance our understanding of the biology of metastasis and improve the management of therapies for cancer patients.
Unfortunately, conventional methods are limited in accurately analyzing the highly heterogeneous nature of CTCs for the following reasons. First, CTCs are rare and make up only a small minority of cells circulating in a patient's blood, while CTC clusters and CTC–leukocyte clusters are even rarer than single CTCs, making it highly challenging to detect and isolate a statistically significant number of these cells and clusters.4 Second, the size of CTCs is highly heterogeneous, making it difficult to isolate them based on size-dependent separation methods8,9 such as filtration10–12 and microfluidic methods.13–16 Specifically, these methods could miss some CTCs that have a comparable size with other cell types such as leukocytes. Third, a general biomarker for detecting CTCs has yet to be identified. CellSearch, the first commercial CTC isolation platform approved by the US FDA, is an antibody-based method for detecting CTCs expressing epithelial markers such as the epithelial cell adhesion molecule (EpCAM) and cytokeratins (CKs), but not CD45 (common leukocyte antigen).17 Unfortunately, the invasive potential of CTCs through their epithelial–mesenchymal transition (EMT) cannot be identified by this antibody-based method because they do not always express these epithelial markers.1 Thus, the ability to detect and isolate all forms of CTCs, including CTC–leukocyte clusters from whole blood, is expected to significantly advance our understanding of the pathophysiology of tumor metastasis and further exploit the potential of liquid biopsy.
Recently, Matsusaka et al. have developed another CTC biomarker that overcomes the above CTC identification problem.18 This approach is photodynamic detection based on the accumulation of photosensitizing molecules induced by 5-aminolevulinic acid (5-ALA) in malignant cells. 5-ALA is a precursor of porphyrin in heme synthesis that induces intracellular accumulation of protoporphyrin IX (PpIX) in cancer cells due to their unique metabolic activity.19 Here, PpIX is a fluorescent substance that emits red fluorescence at a peak wavelength of 635 nm when excited by violet light near 400 nm, suggesting that red fluorescence from PpIX is a potential biomarker for CTC detection. It should be noted that 5-ALA-based photodynamic detection has been widely used for diagnosing various solid cancers,20–23 further supporting this potential. However, 5-ALA-induced PpIX fluorescence is generally weak, rendering conventional imaging flow cytometry techniques for PpIX-based CTC detection highly challenging.
In this article, we present an effective strategy for simultaneously analyzing all forms of CTCs in blood by virtual-freezing fluorescence imaging (VIFFI) flow cytometry24 with 5-ALA stimulation and antibody labeling. VIFFI is an optomechanical imaging method recently developed by Mikami et al.,24 which enables high-throughput acquisition of microscopy-grade images by virtually freezing the motion of fast-flowing cells (1 m s−1) on an image sensor to effectively increase the exposure time. Therefore, VIFFI combined with 5-ALA stimulation and antibody labeling overcomes the problems of the previous methods and enables high-throughput, blur-free, and sensitive fluorescence imaging of every single event (e.g., single cell, cell cluster, cell debris) in blood. In this work, we used VIFFI flow cytometry demonstrated in our earlier report24 as a basis, but introduced a sheath-less cell focusing method, namely elasto-inertial cell focusing,25–27 to increase the efficiency of cell introduction into a microfluidic channel. As a proof-of-principle demonstration of the strategy, we applied cancer cells spiked in blood to the strategy to perform VIFFI flow cytometry of 5-ALA stimulated cells with attenuated EpCAM expression at an event rate of ∼750 events per second (eps) with a signal-to-noise ratio (SNR) of ∼4.4 for PpIX fluorescence. Using the morphological features of cells or cell clusters, we classified events into single cancer cells, cancer cell cluster, and clusters of a cancer cell(s) and a leukocyte(s), resulting in an estimated classification accuracy of ∼99%, ∼80%, and ∼76%, respectively. Furthermore, spiking experiments with various concentrations of spiked cancer cells showed that the sensitivity of our strategy was ∼0.29 with a false positive rate of ∼1.6 × 10−5. These results indicate that VIFFI flow cytometry combined with 5-ALA stimulation made it possible to accurately detect and analyze heterogeneous cancer cell forms based on fluorescence images. Finally, we used the method to test blood samples drawn from four breast cancer patients and four healthy donors. In one of the patient samples, 21 cells positive for both EpCAM and PpIX were identified. With these results, our work paves the way toward accurate detection and enumeration of single CTCs, CTC clusters, and CTC–leukocyte clusters altogether in a large heterogeneous population of blood cells, thereby assisting the determination of prognosis, the guidance and monitoring of treatment, and the design of antitumor strategies for cancer patients.
2. Materials and methods
2.1. Overview
As shown in Fig. 1(a), the procedure of VIFFI flow cytometry with 5-ALA-based cancer detection consists of three steps: (i) sample preparation, (ii) image acquisition, and (iii) image analysis. In the sample preparation step, blood is drawn from a patient or healthy donor, followed by the extraction of a peripheral blood mononuclear cell (PBMC) fraction from the blood using a density gradient purification method (see section 2.3 for details). In addition, HT-29, a human colorectal cancer cell line, and HT-1080, a human fibrosarcoma cell line, are prepared for spiking experiments (see section 2.4 for details). The PBMCs, HT-29 cells, and HT-1080 cells are stimulated with 5-ALA and stained with anti-EpCAM and anti-CD45 antibodies (see section 2.5 for details). 5-ALA stimulation enables us to image cells with attenuated EpCAM expression [see static fluorescence-microscopy images shown in Fig. 1(b)]. Then, as a preparation step of image acquisition, a viscoelastic solution is added to the prepared cell suspensions to focus cells at the center of a microfluidic channel for elasto-inertial cell focusing [see section 2.6 for details; see Fig. 1(c) for a captured image of flowing cells obtained by a commercially available high-speed camera]. During the image acquisition step, cells are imaged using a VIFFI flow cytometer24 [see section 2.2 for details; see Fig. 1(d) for the schematic of the VIFFI flow cytometer; see Fig. 1(e) for an image library taken by VIFFI flow cytometry]. After this step, obtained images are analyzed and evaluated based on fluorescence intensities and cellular morphologies to find single cancer cells and clusters (see section 2.7 for details).
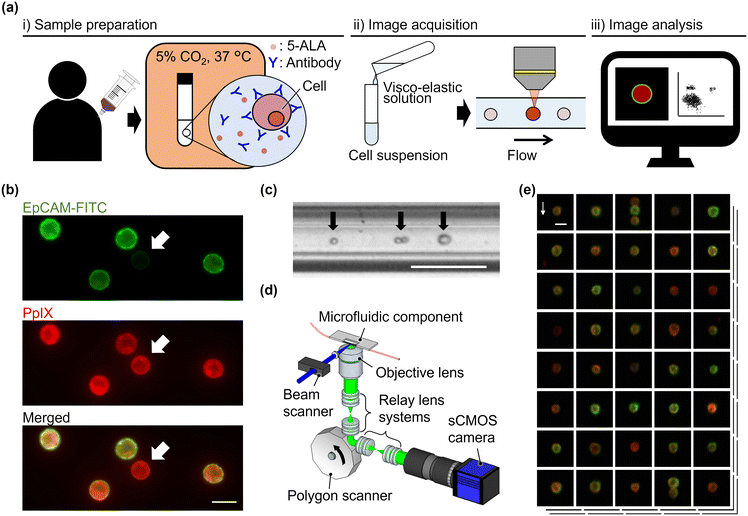 |
| Fig. 1 Schematic of VIFFI flow cytometry with 5-ALA stimulation and antibody labelling. (a) Overview of the procedure. (b) Fluorescence images of HT-29 cells taken by a commercially available microscope. Top, EpCAM-FITC channel image; middle, PpIX channel image; bottom, merged image. Arrows, a cell with attenuated EpCAM expression; scale bar, 20 μm. (c) Bright-field image of HT-29 cells flowing at 1 m s−1 in a microchannel with elasto-inertial focusing. Single cells and a cell cluster (pointed by black arrows) flow at the center of the microchannel. Scale bar, 100 μm. (d) Illustration of the major components of a VIFFI flow cytometer. (e) Library of representative images of HT-29 cells acquired by VIFFI flow cytometry. Green, EpCAM-FITC; red, PpIX; blue, CD45-PE; scale bar, 20 μm; arrow, flow direction (1 m s−1). | |
2.2. VIFFI flow cytometry
VIFFI is an optomechanical imaging method that virtually freezes the motion of fast-flowing cells on an image sensor and sensitively acquires their blur-free fluorescence images24 [see Fig. 1(d)]. This is enabled by precisely canceling the motion of cells with a flow-controlled microfluidic component, a speed-controlled polygon scanner, and a series of timing control circuits. A light-sheet excitation beam scanner is used to scan over the entire field of view during the exposure time of the image sensor. The timings of the image sensor's exposure, the excitation beam's illumination, and localization with respect to the rotation angle of the polygon scanner are precisely synchronized. By virtue of these elements, the virtual-freezing scheme effectively achieves ∼1000 times longer signal integration time on the image sensor, thereby enabling high-resolution (∼700 nm) fluorescence imaging of cells flowing at a speed of 1 m s−1. In this article, two excitation lasers with wavelengths of 405 nm (LBX-405-300-CSB-PP, Oxxius) and 488 nm (Genesis CX 488/2000 STM, Coherent) were used to excite PpIX and antibody-conjugated fluorophores, respectively. Two dichroic mirrors (ff560-FDi01-25×36, edge wavelength: 560 nm, Semrock; #34-741, edge wavelength: 605 nm, Edmund) were used to split the collected fluorescence light from fluorescein isothiocyanate (FITC), phycoerythrin (PE), and PpIX. An objective lens (UPLANSAPO10×, NA = 0.40, Olympus) and tube lens (U-TLU, f = 180 mm, Olympus) were used to form images on a scientific complementary metal-oxide-semiconductor (sCMOS) camera (PCO edge 5.5).
2.3. Extraction of PBMC fractions
PBMCs were isolated from whole-blood samples using an iodixanol (OptiPrep, Abbott Diagnostics Technologies AS, Norway)-based density gradient purification method, which is a common procedure as a preparation step for CTC detection.28–31 Specifically, 9–10 mL of whole blood was first collected using ethylenediaminetetraacetic acid (EDTA) tubes from six healthy donors at the University of Tokyo Hospital and four breast cancer patients ranging from stages I–III at the University of Tsukuba Hospital (see Table S1† for details). In a previous study, detecting five CTCs in a 7.5 mL blood sample was set as a threshold for discriminating patients breast cancer or colorectal cancer who were more likely to experience metastasis earlier than others.32 Using this threshold as a guideline, we collected ∼10 mL of blood so that we had a sufficient volume for each sample to detect CTCs. Second, each EDTA-treated blood was resuspended in 2.7 mL of 40% (w/v) iodixanol. Third, 5 mL of each prepared blood was overlaid on the iodixanol solution, which was adjusted to a density of 1.078 g mL−1. Fourth, 0.5 mL of phosphate-buffered saline (PBS) was overlaid on the top of each sample. Fifth, the samples were centrifuged at 700g for 20 min at room temperature. Sixth, a PBMC fraction was collected from each sample. Each PBMC fraction was transferred to a tube and then washed twice with PBS by centrifugation at 300g for 5 min at room temperature. Afterward, the obtained PBMC fractions were assessed with an automated hematology analyzer (XN-9100, Sysmex, Japan). The constituents of the prepared PBMC fractions are shown in Table S2.† This study was approved by the Institutional Ethics Committee of the University of Tokyo [No. 11049-(12)] and the University of Tsukuba (No. H30-120). The healthy donors and patients provided written informed consent.
2.4. Preparation of cancer cells and their clusters
HT-29 cells and HT-1080 cells were purchased from ATCC as human colorectal adenocarcinoma cells and human fibrosarcoma cells, respectively. HT-29 cells were cultured at 37 °C under 5% CO2 with humidification in Dulbecco's modified Eagle medium (DMEM) (FUJIFILM Wako Pure Chemical) supplemented with 10% fetal bovine serum (S1780-500, biowest), and 50 U mL−1 penicillin and 50 μg mL−1 streptomycin (FUJIFILM Wako Pure Chemical). HT-1080 cells were cultured at 37 °C under 5% CO2 with humidification in Eagle's minimum essential medium (EMEM) (Sigma) supplemented with 10% fetal bovine serum (S1780-500, biowest), and 50 U mL−1 penicillin and 50 μg mL−1 streptomycin (FUJIFILM Wako Pure Chemical). Additionally, we prepared cluster-induced cells by seeding cells onto low-cell-adhesion 35 mm dishes (MS-9035X, PrimeSurface, Sumitomo Bakelite) one day before imaging experiments, followed by one-day incubation. To make the variety of the cell cluster formation wider, we seeded cells with two cell concentrations of 1 × 104 cells per mL and 5 × 104 cells per mL. Here, we used DMEM for HT-29 cells and EMEM for HT-1080 cells.
2.5. 5-ALA stimulation and antibody staining
We first prepared cell suspensions at a concentration of 2.5 × 106 cells per mL as follows. For imaging cancer cells (see sections 3.2 and 3.3 for details), we prepared four cell suspensions: a 100
:
1 mixture of HT-29 cells and cluster-induced HT-29 cells (see section 2.4 for details) in DMEM, a 100
:
1 mixture of HT-1080 cells and cluster-induced HT-1080 cells (see section 2.4 for details) in EMEM, PBMCs in DMEM, PBMCs in EMEM. For rare cell detection experiments (see section 3.4 for details), 15 PBMC fractions from a healthy donor and one HT-29 cell suspension were prepared. For imaging cells in clinical samples (see section 3.5 for details), PBMC fractions from healthy donors and cancer patients were separately suspended in DMEM. 5-ALA (AL-05-1, Cosmo Bio) was added to each of the cell suspensions at a concentration of 3 mM, which were incubated at 37 °C for 1 hour afterward. Here, the concentration was selected to avoid potential cell toxicity.33 After the incubation, cells were washed with PBS and immunolabeled with FITC-conjugated anti-EpCAM antibody and PE-conjugated anti-CD45 antibody at 37 °C for 30 min. Then, the cells were washed and suspended in PBS at a final concentration of 5 × 105 cells per mL for VIFFI flow cytometry.
For imaging experiments of 1
:
1 mixtures (see section 3.3 for details), two of the cell suspensions were mixed right before the imaging experiments. Specifically, a 1
:
1 mixture of HT-29 cells and PBMCs was prepared by mixing the HT-29 suspension and the in-DMEM PBMC suspension; a 1
:
1 mixture of HT-1080 cells and PBMCs was prepared by mixing the HT-1080 suspension and the in-EMEM PBMC suspension.
For rare cell detection experiments (see section 3.4 for details), nine cell suspensions with spiked cancer cells were prepared by spiking ∼50, ∼500, and ∼1000 HT-29 cells in ∼105 PBMCs (three samples per condition). Six PBMC suspensions without spiked cancer cells were also prepared for negative controls. Specifically, a HT-29 suspension was diluted to reach a concentration of 5 × 104 cells per mL. An appropriate volume of the suspension containing a desired number of cancer cells (i.e., ∼50, ∼500, or ∼1000 HT-29 cells) was added into a ∼1 mL PBMC suspension with ∼105 PBMCs. The number of spiked HT-29 was estimated by preparing reference samples using the same protocol as that for the spiked samples, staining live cells with calcein AM, and counting the number of cells in each suspension. The enumeration of cells in each suspension was performed by using a 96-well plate and imaging the entire area of each well bottom with a commercially available fluorescence microscope (Nikon Instruments, ECLIPSE Ti2).
2.6. Elasto-inertial focusing
We employed elasto-inertial focusing25–27 for aligning cells at the center of a microchannel. Specifically, we used a home-made microfluidic component and hyaluronic acid (HA) sodium salt medium (1.01–1.8 MDa; Lifecore Biomedical) for focusing cells in VIFFI flow cytometry. As shown in Fig. S1,† the microfluidic component consists of a square glass capillary tube with an 80 μm inner-diameter and a 160 μm outer-diameter (8508-050, VitroCom), two polyether ether ketone (PEEK) tubings with a 500 μm inner-diameter (NPK-008, Nirei Industry Co., Ltd.), a home-made aluminum substrate, and paste (AX038, Cemedine). The construction process of the microfluidic component was based on the following three steps. First, each of the two ends of the glass capillary tube, which works as a microchannel, were inserted into PEEK tubing. Second, the connection points of the PEEK tubings and the glass capillary tube were glued for sealing and fixing onto the aluminum substrate. Finally, the microchannel was left to dry overnight. The two PEEK tubings work as adapters to connect the glass capillary tube to a syringe and a waste tank. A cell-containing HA sodium salt medium was prepared as follows. First, HA sodium salt powder was dissolved into PBS solution at a concentration of 2000 parts per million (ppm). Second, the dissolved fluid was mixed with cell suspension at a 1
:
1 volume ratio so that the final HA sodium salt concentration was 1000 ppm. Right before the VIFFI flow cytometry, the medium was mixed gently to avoid a gravitational deposition. Then, the medium was delivered to the microchannel via a syringe pump (Harvard apparatus 11 Elite) at a flow rate of 160 μL min−1, which corresponds to a flow speed of 1 m s−1. Based on these conditions, cells flow at the center of the microchannel due to the effect of elasto-inertial focusing25–27 (see Fig. S2†).
2.7. Image processing
The VIFFI flow cytometer acquired images with a field of view of 130 μm × 1600 μm, which may contain multiple objects (e.g., cells, cell clusters, and cell debris). Objects in each image were recognized as individual events based on their fluorescence signals. Specifically, a binary image of each field-of-view image was generated; the contours of objects in the field of view were detected based on the binary image; the center of mass of each object contour was calculated based on image moments of the object contour. Then each object was cropped into square-shape images such that the center of mass of the object contour was located at the center of the cropped image. For each event, we generated an image mask of the whole object as follows. We first overlayed three fluorescence channel images of EpCAM-FITC, CD45-PE, and PpIX into one single gray-scale image. Then, we converted the gray-scaled image to a binary image using a common threshold throughout all cropped images, generating an image mask of whole objects. Using the image mask, we calculated the average fluorescence intensities of each fluorescence channel. Specifically, for each fluorescence image channel, we calculated the integrated fluorescence intensities by summing up fluorescence intensity values of pixels within the image mask, followed by subtracting the background signal intensity and compensating for the crosstalk of fluorescence intensities among the three fluorescence channels. Here, we defined the background signal intensity to be subtracted from each image as the product of the image mask area and the average background signal intensity per pixel, the latter of which was calculated using a blank image. The crosstalk was compensated based on the fluorescence spectra of the fluorophores and the cut-off wavelength of the dichroic mirrors. The average fluorescence intensity of each event was calculated by dividing the integrated fluorescence intensity by the image mask area. Furthermore, using the image mask we generated a convex-hull mask and calculated the circularity of each event based on the convex-hull mask. Here, the circularity was used for the identification of single cells and cell clusters, since cell clusters have a lower circularity. Note, in a cropped image with an event having multiple objects, the circularity of the largest object was calculated whereas, in the case of events that have a combination of cancer cell(s) and leukocyte(s) that flow separately, the event was removed from the population to be analyzed.
2.8. Imaging sensitivity
To evaluate the sensitivity of the VIFFI flow cytometer, we used the SNR of the ith fluorescence channel as follows:
Here, f denotes the conversion factor of the sCMOS camera (0.46 electrons/count); r denotes the readout noise of the sCMOS camera (1.7 root-mean-square electrons); bj denotes the average background level of the jth fluorescence channel, which is given by subtracting the average signal intensity per pixel of the jth-channel blank images acquired in the dark from that of images acquired during a cell imaging experiment; sj denotes the average fluorescence signal of the jth fluorescence channel, which is given by subtracting the background level of the jth fluorescence channel from the average fluorescence intensity per pixel within a mask area; αi,j denotes a crosstalk compensation factor for the ith fluorescence channel.
3. Results and discussion
3.1. Overview
We performed four types of imaging flow cytometry experiments to validate our strategy. First, we conducted imaging flow cytometry experiments using three relatively homogenous cell samples: an HT-29 cell suspension, HT-1080 cell suspension, and PBMC suspension. These experiments aimed to verify the strategy's capability of detecting PpIX signals and imaging small cancer cells. Second, we performed imaging flow cytometry experiments using two types of cell-spiked blood samples: a 1
:
1 mixture of HT-29 cells and PBMCs in D-MEM and a 1
:
1-mixture of HT-1080 cells and PBMCs in E-MEM. Each sample was prepared by mixing the two cell suspensions right before imaging experiments. These experiments aimed to demonstrate the strategy's capability of identifying cancer cells spiked in blood and recognizing the morphological features of cell clusters. Third, we performed rare cell detection using spiked samples of HT-29 cells at various concentrations in 1 mL PBMC suspensions to evaluate the sensitivity of our method as a function of the false positive rate. Finally, we experimentally demonstrated the detection of EpCAM-positive PpIX-positive cells in patient blood samples to show the clinical potential of our method. Details of the experiments are described in sections 3.2–3.5.
3.2. Imaging of cancer cells and PBMCs
To validate the 5-ALA-based cancer cell detection with VIFFI flow cytometry, we separately imaged HT-29 cells, HT-1080 cells, and PBMCs. The event rate and duration time of the imaging experiments were ∼276 eps and ∼15 s, ∼353 eps and ∼23 s, and ∼178 eps and ∼14 s, respectively. We first processed these images to evaluate average fluorescence intensities for each event. We then confirmed that the images (especially those of PpIX) provided fluorescence SNRs high enough to analyze cellular shape and position. Finally, we evaluated the capability of detecting small cancer cells.
Fig. 2(a) shows scatter plots of average fluorescence intensities of the cells. HT-29 cells, HT-1080 cells, and PBMCs were well separated by the combination of EpCAM-FITC and CD45-PE signals or the combination of EpCAM-FITC and PpIX signals (see the left two panels). Both HT-29 and HT-1080 cells had a higher PpIX intensity compared with PBMCs, showing that not only EpCAM-positive cells (HT-29 cells) but also EpCAM-negative cells (mainly HT-1080 cells) can be detected with PpIX fluorescence intensity (see the bottom-left and top-right panels). These results reinforce the usefulness of 5-ALA stimulation for detecting cancer cells and that PpIX signals induced by 5-ALA stimulation are detectable using the VIFFI flow cytometer. Furthermore, in Fig. 2(b), we summarized the classification results of each population based on the thresholds we set in the histograms of Fig. 2(a). The classification results were found to be reasonable. Specifically, first, ∼100% of both HT-29 and HT-1080 cells were classified as CD45 negative, while ∼100% of PBMCs were classified as CD45 positive. Second, ∼87% of HT-29 cells were recognized as EpCAM-positive cells while the rest were negative, which can be explained by EMT that takes place in some HT-29 cells.34 Third, ∼100% of HT-1080 cells were regarded as EpCAM-negative cells, which is reasonable since HT-1080 is a mesenchymal cell line. Fourth, ∼100% of HT-29 cells and ∼96% of HT-1080 cells were regarded as PpIX-positive cells, which can be interpreted as almost all cancer cells being active. Lastly, ∼15% of the PBMCs were PpIX-positive cells, which can be explained by the presence of monocytes with CD14 expression that are known to accumulate PpIX when treated with 5-ALA.35 These results show that our VIFFI flow cytometer has the ability to detect fluorescence signals from EpCAM-FITC, CD45-PE, and PpIX correctly.
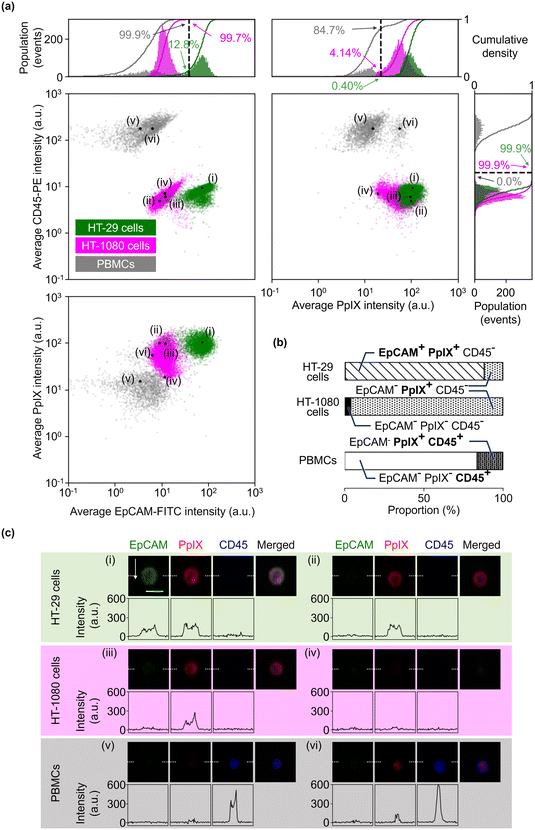 |
| Fig. 2 Fluorescence intensities and representative images of HT-29 cells, HT-1080 cells, and PBMCs separately imaged. (a) Scatter plots and histograms with the cumulative densities of events in average fluorescence intensities. The dashed lines are determined thresholds for each fluorescence detection channel. The black dots labelled as (i)–(vi) correspond to the events shown in fluorescence images of (c). (b) Proportions of events in EpCAM/PpIX/CD45 positive/negative. (c) Fluorescence images of the cells at the marked points labelled as (i)–(iv) in the scatter plots with profiles of fluorescence intensity along the white dashed lines in the images. Scale bar, 20 μm; arrow, flow direction (1 m s−1). | |
Fig. 2(c) shows representative fluorescence images at the points marked in the scatter plots in Fig. 2(a). Events (i), (iii), and (v) were extracted as typical images that were selected from the highest density region in all channels of fluorescence signals of HT-29 cells, HT-1080 cells, and PBMCs, respectively. Events (ii), (iv), and (vi) were extracted as comparisons of the corresponding events [events (i), (iii), and (v), respectively]. Event (i) images clearly show EpCAM expression on the surface of the cell and PpIX accumulation in the cytosol with an SNR of 5.2 and 5.9, respectively. The PpIX fluorescence image shows a low-intensity region in the center of the cell, which looks like a nucleus. Event (ii) images show that EpCAM-FITC signals were around a baseline, indicating that the color crosstalk compensation between the EpCAM channel and the CD45 channel works well. Event (iii) images show PpIX signals with an SNR of 6.1, while event (iv) images show them at around the baseline. Events (v) and (vi) images show CD45-PE signals with an SNR of 8.1 and 8.2, respectively. Notably, event (v) images show that the color crosstalk from the CD45 channel to the other two channels was well compensated. The peak value of the SNR distribution (see Fig. S3†) appeared at ∼5.2 for EpCAM-channel images of HT-29 cells, at ∼4.4 for PpIX-channel images of HT-1080 cells, and at ∼8.0 for CD45-channel images of PBMCs. These results show that all three channels have a sensitivity high enough to not only recognize cellular shape and position, but also intracellular molecule distribution.
We further evaluated the obtained datasets by measuring the size of HT-29 cells and PBMCs based on EpCAM-FITC signals and CD45-PE signals, respectively. As shown in Fig. 3(a), PBMCs were smaller than HT-29 cells, but HT-29 cells having a size similar to PBMCs were also present. Specifically, we recognized 122 events in the region of <250 μm2 in the HT-29 population. As shown in Fig. 3(b) (see Fig. S4† for images of all the events in the region of <250 μm2), ∼40% of these events (i.e., 49 events) were recognized as cells, while the rest were debris. Assuming that we isolated cancer cells from a mixture of HT-29 cells and PBMCs with a threshold of 250 μm2, ∼98% of PBMCs would be excluded while ∼1.6% of HT-29 cells would be lost. These results indicate that VIFFI flow cytometry can detect cancer cells as small as leukocytes that could be missed with size-dependent CTC isolation methods8,9 such as filtration methods10–12 and microfluidic methods.13–16
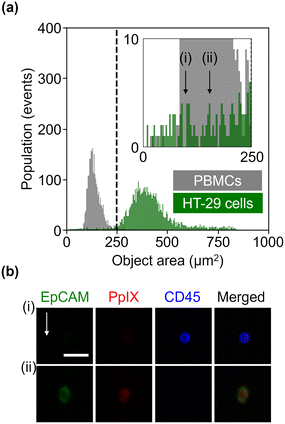 |
| Fig. 3 Size of HT-29 cells and PBMCs. (a) Histograms of events in the object area. (b) Representative fluorescence images of a leukocyte (i) and HT-29 cell (ii), each of which has an area of 100 μm2 and 156 μm2, respectively. Scale bar, 20 μm; arrow, flow direction (1 m s−1). | |
3.3. Imaging of mixtures of cancer cells and PBMCs
To simulate the characterization of cancer cells in blood, we performed imaging of a 1
:
1 mixture of HT-29 cells and PBMCs and that of HT-1080 cells and PBMCs. The event rate and duration time of the imaging experiments were ∼587 eps and ∼80 s and ∼750 eps and ∼160 s, respectively. All the combinations of fluorescence intensity scatter plots of these mixtures are shown in Fig. S5 and S6.† Among them, two scatter plots are shown in Fig. 4: the scatter plot of EpCAM-FITC and CD45-PE for the mixture of HT-29 cells and PBMCs and the scatter plot of PpIX and CD45-PE for the mixture of HT-1080 cells and PBMCs. This is because these two scatter plots show a different feature from those shown in Fig. 2(a). Specifically, the scatter plot for the mixture of HT-29 cells and PBMCs shows a subpopulation in the region of both EpCAM and CD45 positive [Fig. 4(a)]. This double positive subpopulation consists of heterogeneous clusters of an HT-29 cell(s) and a leukocyte(s) as shown in the event (i) image. The scatter plot for the mixture of HT-1080 cells and PBMCs shows a subpopulation in the region of both PpIX and CD45 positive as shown in Fig. 4(b). This double-positive subpopulation was predominated by leukocytes with PpIX accumulation similar to Fig. 2(a) [see event (i) image in Fig. 4(b) for a representative image], however, the subpopulation contained a heterogeneous cluster of an HT-1080 cell(s) and a leukocyte(s) [see event (ii) image in Fig. 4(b) for a representative image]. Therefore, these two types of events cannot be differentiated based on fluorescence intensity alone.
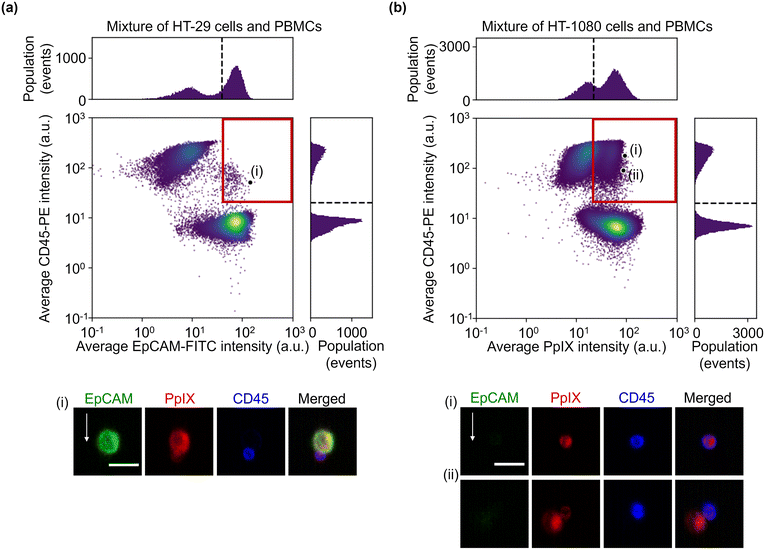 |
| Fig. 4 Fluorescence intensities and representative images of the mixture samples. (a) Scatter plot and histograms of events in EpCAM-FITC and CD45-PE intensity together with an image of cells at the marked point labelled as (i) in the scatter plot. (b) Scatter plot and histograms of events in PpIX intensity and CD45-PE intensity together with images of cells at the marked points labelled as (i) and (ii) in the scatter plot. Dashed lines, thresholds determined in Fig. 2; red squares, double positive region; scale bars, 20 μm; arrow, flow direction (1 m s−1). | |
To overcome this problem, we exploited image analysis. Specifically, we examined the localization of CD45-PE in fluorescence images to screen out events consisting of leukocytes only from heterogeneous clusters of an EpCAM-negative cancer cell(s) and a leukocyte(s). Here, to demonstrate this strategy, we used images of the mixture of HT-1080 cells and PBMCs. We first gated both CD45 and PpIX positive events and then generated two types of image masks for each event: one represents the whole object region and the other represents the CD45-positive region. Based on these masks, we quantified the coverage area ratio of the CD45-positive mask region to the whole mask region for each event. As shown in Fig. 5, there were two populations in the coverage area ratio of the CD45-positive region to the whole object region. Events consisting of a leukocyte(s) were found in the population with a higher cover ratio, while events containing heterogeneous clusters were found in the other population. These results show that the imaging capability enables us to distinguish heterogeneous clusters from the huge background of PpIX-positive leukocytes. We set 48% of the coverage area ratio as a threshold to separate events consisting of only leukocyte(s) and events consisting of a heterogeneous cluster for further cluster analysis.
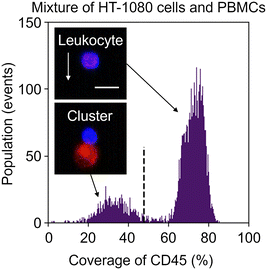 |
| Fig. 5 Histograms of events of the mixture of HT-1080 cells and PBMCs in the coverage area ratios of the CD45-positive region to the whole region. Insets, fluorescence images of a leukocyte, and a heterogeneous cluster of a leukocyte and cancer cell. Green, EpCAM-FITC; red, PpIX; blue, CD45-PE; dashed line, a threshold to separate events consisting of only leukocyte(s) and events consisting of a heterogeneous cluster(s); scale bar, 20 μm; arrow, flow direction (1 m s−1). | |
To further show the advantage of imaging flow cytometry over intensity-based analysis, we characterized diverse forms of cancer cell clusters. As a preparation step, we excluded events consisting of only leukocytes using a gating strategy based on the coverage area ratio of CD45 shown in Fig. 5 (see Fig. S7† for details of the gating strategy), followed by calculating the circularity and the integrated CD45-PE intensity of the gated cells for each event. Then, we used the circularity as a simple and typical morphological feature to find clusters. Fig. 6(a) shows the scatter plot of the circularity and integrated CD45-PE intensity of cells in the mixture of HT-29 cells and PBMCs. The red boxes in this figure display the gating areas which classify events as single cancer cells, cancer-cell clusters, and clusters of a cancer cell(s) and leukocyte(s). Based on the gating criteria, rare and unique events were found. Specifically, events of singlet [event (i)], doublet [event (ii)], and triplet [events (iii) and (iv)] cancer cells were found based on the circularity. Heterogeneous clusters of an EpCAM-positive and -negative cancer cell(s) were also found in them [events (ii) and (iv)]. Furthermore, heterogeneous clusters of a cancer cell(s) and a leukocyte(s) were found in the higher CD45-PE intensity region (≳3 × 104 a.u.) [events (v) and (vi)]. Notably, PpIX signal detection enabled us to recognize event (vi) as a cluster event. The classification accuracy values based on the gating criteria were estimated to be ∼99% for single cells, ∼76% for cancer cell clusters, and ∼75% for clusters of a cancer cell(s) and a leukocyte(s). Here, we obtained these classification accuracy values by a sampling test: we selected a dataset containing 1000 events that consecutively flowed in the microchannel, gated events using the same gating strategy shown in Fig. S7,† (see Fig. S8† for the scatter plots of the 1000 events), and manually examined and labeled the events true or false (see Fig. S9† for all gated event images).
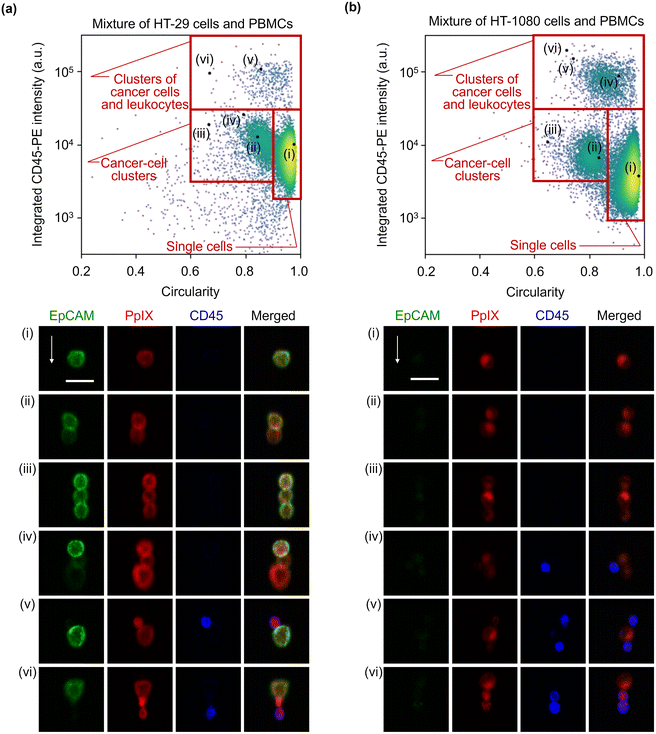 |
| Fig. 6 Morphology-based classification of the cancer cells/clusters in the 1 : 1 mixture of cancer cells and PBMCs. Scatter plots of events in the circularity and integrated CD45-PE intensity together with images of cells at the marked points labelled as (i)–(vi) in the scatter plots. Gating areas are shown with red boxes. (a) Mixture of HT-29 cells and PBMCs. (b) Mixture of HT-1080 cells and PBMCs. Scale bars, 20 μm; arrows, flow direction (1 m s−1). | |
To clearly show that our method is capable of detecting and characterizing EpCAM-negative cancer cells, we also analyzed cancer cells and clusters of the mixture of HT-1080 cells and PBMCs. Similar to Fig. 6(a) and (b) shows that a singlet [event (i)], a doublet [event (ii)], and a triplet [event (iii)] of cancer cell(s) were found based on the circularity; heterogeneous clusters were found in the higher CD45-PE intensity region (≳3 × 104 a.u.) [events (iv)–(vi)]. Furthermore, by virtue of its imaging capability, a finer classification of clusters was made possible. Specifically, event (iv) shows that all cancer cells and a leukocyte adhered to each other, while events (v) and (vi) show three cells adhered in a row. Finally, the classification accuracy values based on the gating areas shown in this figure were evaluated using the sampling test, which is the same procedure as in Fig. 6(a), resulting in ∼99% for single cells, ∼83% for cancer cell clusters, and ∼77% for clusters of a cancer cell(s) and a leukocyte(s) (see Fig. S10 and S11† for a 1000-event scatter plots with the gating strategy and their gated-event images). These results indicate that 5-ALA-based VIFFI flow cytometry provides rich information about cancer cells in blood such as morphologically different forms of mesenchymal CTCs, CTCs after EMT, and various patterns of cell clusters.
Interestingly, the major axis of almost all clusters was aligned with the flow direction as shown in the images of Fig. 6. This orientation alignment was caused by the force induced by elasto-inertial focusing and resulted in the reduced probability that clustered cells were located out of the focal plane.
3.4. Rare cell detection experiments
To quantitatively evaluate the capability of detecting minor populations of cells with our strategy, we performed rare cell detection using samples of HT-29 cells spiked in 1 mL PBMC suspensions at various concentrations. We first acquired images of cells in the spiked samples and then gated events for possible cancer cells by the following strategies: events with a coverage area ratio of the CD45-positive region to the whole object region (see section 3.3 for details) smaller than 48% were gated; events were gated using two parameters: the area of the whole mask region and CD45-PE intensity (see Fig. S12† for the gating strategy); then events were gated with various EpCAM-FITC intensity thresholds and/or PpIX intensity thresholds.
Fig. 7(a) shows the capture efficiency of spiked cancer cells using the EpCAM-based gating or PpIX-based gating. Here, the capture efficiency was defined as the ratio of the population of detected HT-29 cells in the whole population to that of the spiked HT-29 cells. The capture efficiencies ranged from 0 to 0.62 for the sample spiked with ∼1000 cells and from 0.007 to 0.53 for the sample spiked with ∼500 cells depending on the threshold values of the gating. On the other hand, the capture efficiencies of samples spiked with ∼50 cells ranged from 0.04 to 2.19. Notably, the capture efficiency with low threshold values (= 0.1 and 10) exceeded 1 due to the background of false positive events, indicating the difficulty of identifying cancer cells as rare as ∼50 cells.
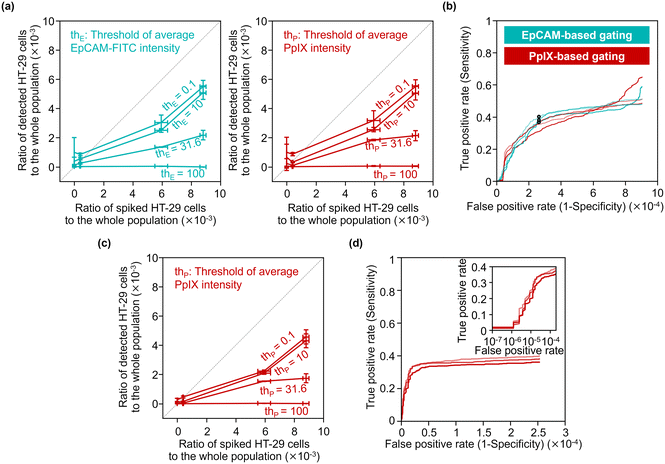 |
| Fig. 7 Rare cell detection with our strategy. (a) Statistical analysis of our strategy's capture efficiency for various concentrations of spiked HT-29 cells. Three samples were tested for each spike condition and six samples were tested for negative controls. The horizontal deviations were evaluated by separately prepared reference samples for spiking (see Materials and methods). (b) EpCAM/PpIX-based ROC curves without PpIX/EpCAM-based gating. Circles, the points where the thresholds of EpCAM intensity was 15.8 a.u. (c) Statistical analysis of the capture efficiency for various concentrations of spiked cancer cells with EpCAM-based gating using a EpCAM-FITC-intensity threshold of 15.8 a.u. (d) PpIX-based ROC curve with EpCAM-based gating using an EpCAM-FITC-intensity threshold of 15.8 a.u. Inset, a zoom-in of the graph shown as a semi-log scale. | |
We evaluated the true positive rate (sensitivity) and false positive rate (1-specificity) in cancer-cell detection with various gating thresholds of EpCAM-FITC or PpIX intensity by using the dataset of the samples spiked with ∼500 cells (the corresponding ratio of the spiked cells to the whole population was ∼6 × 10−3). Fig. 7(b) shows receiver operating characteristics (ROC) curves of EpCAM-based detection without PpIX-based gating and those of PpIX-based detection without EpCAM-based gating. As shown in this graph, the sensitivity increased with an increase in the false positive rate, saturating at ∼0.5 when the false positive rate was over ∼5 × 10−4. The false positive rate would be ∼2.5 × 10−4 by choosing a sensitivity of ∼0.38 as a representative value.
To use a higher specificity (i.e., lower false positive rate) for cancer-cell detection, we applied both of EpCAM-based and PpIX-based gating. For the EpCAM-based gating, we used a fixed threshold of EpCAM-FITC intensity of 15.8 a.u. (the points marked in Fig. 7(b) with circles). For the PpIX-based gating, we used various thresholds. Fig. 7(c) shows the capture efficiency of spiked cancer cells based on this gating strategy. The capture efficiencies were sacrificed in the samples spiked with ∼1000 cells or ∼500 cells, while the capture efficiencies in the sample spiked with ∼50 cells showed ∼1 or less, indicating that false positive events decreased. Fig. 7(d) shows ROC curves of cancer cell detection using the dataset of the samples spiked with ∼500 cells. This graph indicates that the sensitivity was maintained while the false positive rate was improved. The false positive rate would be ∼1.6 × 10−5 by choosing a sensitivity of ∼0.29 as a representative value.
3.5. Demonstration with clinical samples
To show the clinical potential of our method, namely for CTC detection, we experimentally demonstrated the detection of EpCAM-positive PpIX-positive cells from blood samples of four breast cancer patients and four healthy donors. The event rate and duration time of the imaging experiments were ∼1000 eps and >1000 s, respectively, resulting in the number of events detected being >106 (see Table S3† for details). As preparation for the experiment, we used the same gating strategy used in rare cell detection experiments (see section 3.4). Among the gated events in each sample, EpCAM-positive PpIX-positive events were enumerated with a fixed threshold of EpCAM-FITC intensity (15.8 a.u.) and a variable threshold of PpIX intensity. Here the fixed threshold of EpCAM-FITC intensity is the same threshold that was used for Fig. 7(c). As shown in Fig. 8, with a PpIX threshold of 100, 21 EpCAM-positive PpIX-positive events were found in one of the patient samples. These 21 events were as rare as ∼0.001% in the detected population (∼2.64 × 106 events) of the patient's sample. On the other hand, no such double-positive events were found in the samples of the other three patients and four healthy donors. These results are consistent with previous studies in which the CTC-detection ratios with non-metastatic breast cancer patients at stages I–III were found to be ∼27%,36 ∼20%,37 and ∼20%.38
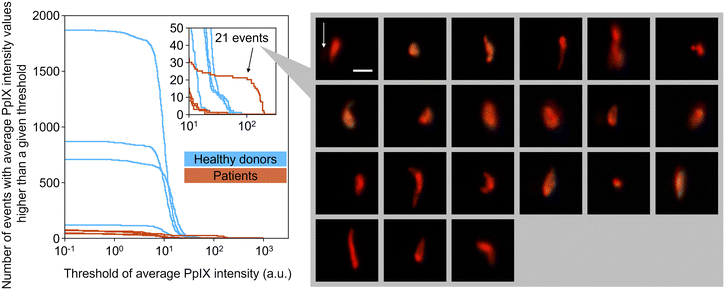 |
| Fig. 8 Number of events with PpIX intensity values higher than a given threshold. Top-right inset, a zoomed part of the main graph. Right inset, images of the events gated by the PpIX-intensity threshold of 100. Green, EpCAM-FITC; red, PpIX; blue, CD45-PE in fluorescence images. Scale bar, 20 μm; arrow, flow direction (1 m s−1). | |
4. Conclusions
In this article, to validate the strategy for simultaneously analyzing all forms of CTCs in blood, we experimentally demonstrated the detection and characterization of cancer cells spiked in PBMC fractions using VIFFI flow cytometry with 5-ALA stimulation and antibody labeling. Our results show that our VIFFI flow cytometer has the capability to sensitively detect the fluorescence signals of PpIX that were accumulated in cytosol through heme synthesis stimulated by 5-ALA (SNR = ∼4.4). The event rate of the VIFFI flow cytometer in the imaging experiments was ∼750 eps, which is sufficient for examining 5–20 million leukocytes in a PBMC fraction extracted from ∼10 mL blood39 within a few hours. Based on these images, not only cancer cells and their clusters, but also clusters of a cancer cell(s) and a leukocyte(s) were accurately discriminated from leukocyte events by evaluating fluorescence intensities and analyzing localizations of CD45. Our strategy enabled us to classify diverse forms of cancer cells/clusters based on spatial information of fluorescence images, as well as to examine the presence and distributions of stained molecules (EpCAM and CD45) and fluorescent metabolites (PpIX) in every single component (cell or debris) of a heterogeneous cluster. The spiking experiments show that the sensitivity of our strategy was ∼0.29 with a false positive rate of ∼1.6 × 10−5. Furthermore, clinical sample tests show that 21 EpCAM-positive PpIX-positive cells were found in one of the breast cancer patient samples. Finally, our strategy is different from and somewhat advantageous over previously demonstrated methods of blood cell identification using bright-field imaging flow cytometry with deep learning40–43 in that it does not require a supervised learning process and can screen unknown cells or cell clusters.
The capability of our strategy can be improved in multiple directions. First, the optimization of the conditions in the cell staining process can improve the sensitivity and specificity [i.e., 1 − (false positive rate)]. Especially, the concentration of 5-ALA to induce PpIX can be further optimized. As shown in the spiking experiments, some leukocytes show PpIX positive, which contributes to an increase of the false positive rate. Second, the image analysis with multiple morphological features and/or deep-learning algorithms can improve the sensitivity, specificity, and the accuracy of cluster detection. In this article, we used circularity as a simple and typical morphological feature to find clusters containing only a few cells since they have more elongated morphologies when compared to those of rounded single cells, however, this method can potentially miss some clusters with circularly aggregated cells. Third, the implementation of three-dimensional (3D) imaging into the VIFFI flow cytometer can improve the accuracy of analyzing clusters. This is because 3D imaging can solve the fundamental problem of two-dimensional (2D) imaging flow cytometry: if component cells are out of the focal plane, they cannot be imaged properly. Although there are a few reports about 3D imaging flow cytometry,44,45 it is challenging to use 3D imaging in CTC detection because the event rate is highly restricted by the throughput of the data acquisition and analysis. If high-throughput 3D imaging flow cytometry is developed, it is expected to be impactful.
Our next step would be to use it on large-scale clinical blood samples for a deeper understanding of cancer metastasis as well as for assisting the determination of prognosis, the guidance and monitoring of treatment, and the design of antitumor strategies for cancer patients. Specifically, extensive testing of patients having various cancer types and stages with the strategy could elucidate the correlation between cancer types/stages and the types of cancer cells/clusters. In addition, long-term monitoring of cancer patients could clarify their responses to drug treatments. Furthermore, sorting of CTCs using image-activated cell sorting46–51 would provide further insights into their physiological mechanisms through post analyses (e.g., single cell-RNA sequencing, whole-genome sequencing, and single-cell metabolomics analyses). For instance, the difference in gene expression profiling of various forms of CTC clusters could be investigated to identify signaling pathways that contribute to their formation and metastasis.5,52–55 Also, induction and elimination rates on diverse forms of CTC clusters upon drug treatment could be evaluated to potentially contribute to personalized medicine and guidance of treatment.56–59
Author contributions
Hiroki Matsumura: methodology (equal); software (lead); formal analysis (equal); investigation (lead); data curation (lead); writing – original draft (lead); visualization (equal). Larina Tzu-Wei Shen: methodology (equal); investigation (equal); resources (equal); writing – original draft (equal). Akihiro Isozaki: conceptualization (equal); formal analysis (equal); data curation (equal); writing – original draft (equal); visualization (equal); supervision (equal); project administration (lead); funding acquisition (equal). Hideharu Mikami: conceptualization (equal); software (equal); formal analysis (equal); data curation (equal); writing – review & editing (equal); supervision (equal); project administration (equal). Dan Yuan: methodology (equal); writing – review & editing (equal). Taichi Miura: investigation (equal); writing – review & editing (equal). Yuto Kondo: validation (equal); investigation (equal); data curation (equal); writing – review & editing (equal); visualization (equal). Tomoko Mori: investigation (equal); writing – review & editing (equal). Yoshika Kusumoto: investigation (equal); resources (equal); writing – review & editing (equal). Masako Nishikawa: methodology (equal); investigation (equal); resources (equal); writing – original draft (equal). Atsushi Yasumoto: Methodology (equal); investigation (equal); resources (equal); writing – review & editing (equal). Aya Ueda: investigation (equal); writing – review & editing (equal). Hiroko Bando: investigation (equal); writing – review & editing (equal). Hisato Hara: investigation (equal); writing – review & editing (equal). Yuhong Liu: investigation (equal); writing – review & editing (equal). Yunjie Deng: investigation (equal); writing – review & editing (equal). Masahiro Sonoshita: writing – review & editing (equal); funding acquisition (equal). Yutaka Yatomi: methodology (equal); resources (equal); writing – review & editing (equal). Keisuke Goda: conceptualization (equal); methodology (equal); resources (equal); writing – original draft (equal); writing – review & editing (lead); visualization (equal); supervision (equal); project administration (equal); funding acquisition (equal). Satoshi Matsusaka: conceptualization (equal); methodology (equal); resources (equal); writing – review & editing (equal); supervision (lead); project administration (equal); funding acquisition (equal).
Conflicts of interest
H. Mi. and K. G. are inventors on a patent covering the VIFFI microscope. K. G. is an inventor on a patent covering the data analysis and display method. K. G. is a shareholder of CYBO.
Acknowledgements
We thank Dr. Maik Herbig for helpful discussions about image analysis related to this research. This work is supported by JSPS Core-to-Core Program JPJSCCA20190007, JSPS KAKENHI grant numbers 19H05633, 20H00317, and 21H01778, White Rock Foundation, G-7 Scholarship Foundation, JST START Program JPMJST2115, and AMED 22ak0101163h0002. D. Y. is a recipient of the JSPS postdoctoral fellowship with the support of a Grant-in-Aid for Scientific Research.
References
- J. Fares, M. Y. Fares, H. H. Khachfe, H. A. Salhab and Y. Fares, Signal Transduction Targeted Ther., 2020, 5, 28 CrossRef PubMed.
- S. J. Cohen, C. J. A. Punt, N. Iannotti, B. H. Saidman, K. D. Sabbath, N. Y. Gabrail, J. Picus, M. Morse, E. Mitchell, M. C. Miller, G. V. Doyle, H. Tissing, L. W. M. M. Terstappen and N. J. Meropol, J. Clin. Oncol., 2008, 26, 3213–3221 CrossRef PubMed.
- F. C. Bidard, D. J. Peeters, T. Fehm, F. Nolé, R. Gisbert-Criado, D. Mavroudis, S. Grisanti, D. Generali, J. A. Garcia-Saenz, J. Stebbing, C. Caldas, P. Gazzaniga, L. Manso, R. Zamarchi, A. F. de Lascoiti, L. De Mattos-Arruda, M. Ignatiadis, R. Lebofsky, S. J. van Laere, F. Meier-Stiegen, M. T. Sandri, J. Vidal-Martinez, E. Politaki, F. Consoli, A. Bottini, E. Diaz-Rubio, J. Krell, S. J. Dawson, C. Raimondi, A. Rutten, W. Janni, E. Munzone, V. Carañana, S. Agelaki, C. Almici, L. Dirix, E. F. Solomayer, L. Zorzino, H. Johannes, J. S. Reis-Filho, K. Pantel, J. Y. Pierga and S. Michiels, Lancet Oncol., 2014, 15, 406–414 CrossRef PubMed.
- C. Alix-Panabières and K. Pantel, Cancer Discovery, 2021, 11, 858–873 CrossRef PubMed.
- B. M. Szczerba, F. Castro-Giner, M. Vetter, I. Krol, S. Gkountela, J. Landin, M. C. Scheidmann, C. Donato, R. Scherrer, J. Singer, C. Beisel, C. Kurzeder, V. Heinzelmann-Schwarz, C. Rochlitz, W. P. Weber, N. Beerenwinkel and N. Aceto, Nature, 2019, 566, 553–557 CrossRef CAS PubMed.
- X. Jiang, K. H. K. Wong, A. H. Khankhel, M. Zeinali, E. Reategui, M. J. Phillips, X. Luo, N. Aceto, F. Fachin, A. N. Hoang, W. Kim, A. E. Jensen, L. V. Sequist, S. Maheswaran, D. A. Haber, S. L. Stott and M. Toner, Lab Chip, 2017, 17, 3498–3503 RSC.
- M. Giuliano, A. Shaikh, H. C. Lo, G. Arpino, S. De Placido, X. H. Zhang, M. Cristofanilli, R. Schiff and M. V. Trivedi, Cancer Res., 2018, 78, 845–852 CrossRef CAS PubMed.
- L. Wang, Y. Li, J. Xu, A. Zhang, X. Wang, R. Tang, X. Zhang, H. Yin, M. Liu, D. D. Wang, P. P. Lin, L. Shen and J. Dong, Cancer Lett., 2018, 412, 99–107 CrossRef CAS PubMed.
- H. Ito, H. Inoue, S. Kimura, T. Ohmori, F. Ishikawa, K. Gohda and J. Sato, Int. J. Oncol., 2014, 45, 227–234 CrossRef CAS PubMed.
- J. A. Awe, J. Saranchuk, D. Drachenberg and S. Mai, Urol. Oncol.: Semin. Orig. Invest., 2017, 35, 300–309 CrossRef PubMed.
- H. K. Lin, S. Zheng, A. J. Williams, M. Balic, S. Groshen, H. I. Scher, M. Fleisher, W. Stadler, R. H. Datar, Y.-C. Tai and R. J. Cote, Clin. Cancer Res., 2010, 16, 5011–5018 CrossRef CAS PubMed.
- M. Boya, T. Ozkaya-Ahmadov, B. E. Swain, C.-H. Chu, N. Asmare, O. Civelekoglu, R. Liu, D. Lee, S. Tobia, S. Biliya, L. D. McDonald, B. Nazha, O. Kucuk, M. G. Sanda, B. B. Benigno, C. S. Moreno, M. A. Bilen, J. F. McDonald and A. F. Sarioglu, Nat. Commun., 2022, 13, 3385 CrossRef CAS PubMed.
- Y. Chen, P. Li, P. H. Huang, Y. Xie, J. D. Mai, L. Wang, N. T. Nguyen and T. J. Huang, Lab Chip, 2014, 14, 626–645 RSC.
- M. Dhar, J. N. Lam, T. Walser, S. M. Dubinett, M. B. Rettig and D. Di Carlo, Proc. Natl. Acad. Sci. U. S. A., 2018, 115, 9986–9991 CrossRef CAS PubMed.
- M. Dhar, J. Wong, A. Karimi, J. Che, C. Renier, M. Matsumoto, M. Triboulet, E. B. Garon, J. W. Goldman, M. B. Rettig, S. S. Jeffrey, R. P. Kulkarni, E. Sollier and D. Di Carlo, Biomicrofluidics, 2015, 9, 064116 CrossRef PubMed.
- M. Alunni-Fabbroni and M. T. Sandri, Methods, 2010, 50, 289–297 CrossRef CAS PubMed.
- W. J. Allard, J. Matera, M. C. Miller, M. Repollet, M. C. Connelly, C. Rao, A. G. J. Tibbe, J. W. Uhr and L. W. M. M. Terstappen, Clin. Cancer Res., 2004, 10, 6897–6904 CrossRef PubMed.
- S. Matsusaka, M. Kozuka, H. Takagi, H. Ito, S. Minowa, M. Hirai and K. Hatake, Cancer Lett., 2014, 355, 113–120 CrossRef CAS PubMed.
- J. C. Kennedy, S. L. Marcus and R. H. Pottier, J. Clin. Laser Med. Surg., 1996, 14, 289–304 CrossRef CAS PubMed.
- E. Endlicher and H. Messmann, Gastrointest. Endosc. Clin. North Am., 2004, 14, 475–485 CrossRef PubMed.
- C.-F. Jiang, C.-Y. Wang and C.-P. Chiang, J. Biomed. Opt., 2011, 16, 076006 CrossRef PubMed.
- R. Baumgartner, R. M. Huber, H. Schulz, H. Stepp, K. Rick, F. Gamarra, A. Leberig and C. Roth, J. Photochem. Photobiol., B, 1996, 36, 169–174 CrossRef CAS PubMed.
- C. J. Kelty, N. J. Brown, M. W. R. Reed and R. Ackroyd, Photochem. Photobiol. Sci., 2002, 1, 158–168 CrossRef CAS PubMed.
- H. Mikami, M. Kawaguchi, C. Huang, H. Matsumura, T. Sugimura, K. Huang, C. Lei, S. Ueno, T. Miura, T. Ito, K. Nagasawa, T. Maeno, H. Watarai, M. Yamagishi, S. Uemura and S. Ohnuki, Nat. Commun., 2020, 11, 1162 CrossRef CAS PubMed.
- E. J. Lim, T. J. Ober, J. F. Edd, S. P. Desai, D. Neal, K. W. Bong, P. S. Doyle, G. H. McKinley and M. Toner, Nat. Commun., 2014, 5, 4120 CrossRef CAS PubMed.
- S. Yang, J. Y. Kim, S. J. Lee, S. S. Lee and J. M. Kim, Lab Chip, 2011, 11, 266–273 RSC.
- D. Yuan, Q. Zhao, S. Yan, S. Y. Tang, G. Alici, J. Zhang and W. Li, Lab Chip, 2018, 18, 551–567 RSC.
- J. Zhang, K. Chen and Z. H. Fan, Adv. Clin. Chem., 2016, 75, 1–31 Search PubMed.
- M. Vismara, C. Reduzzi, M. Silvestri, F. Murianni, G. Lo Russo, O. Fortunato, R. Motta, D. Lanzoni, F. Giovinazzo, P. Miodini, S. Pasquali, P. Suatoni, U. Pastorino, L. Roz, G. Sozzi, V. Cappelletti and G. Bertolini, Clin. Chem., 2022, 68, 691–701 CrossRef PubMed.
- P. Zhu, M. L. Stanton, E. P. Castle, R. W. Joseph, D. L. Adams, S. Li, P. Amstutz, C. M. Tang and T. H. Ho, J. Transl. Med., 2016, 14, 198 CrossRef PubMed.
- H. Li, Q. H. Meng, H. Noh, I. S. Batth, N. Somaiah, K. E. Torres, X. Xia, R. Wang and S. Li, Cancer Lett., 2017, 403, 216–223 CrossRef CAS PubMed.
- M. Cristofanilli, G. T. Budd, M. J. Ellis, A. Stopeck, J. Matera, M. C. Miller, J. M. Reuben, G. V. Doyle, W. J. Allard, L. W. M. M. Terstappen and D. F. Hayes, N. Engl. J. Med., 2004, 351, 781–791 CrossRef CAS PubMed.
- T. Holien, O. A. Gederaas, S. R. Darvekar, E. Christensen and Q. Peng, Lasers Surg. Med., 2018, 50, 469–475 CrossRef PubMed.
- P. Przygodzka, I. Papiewska-Pająk, H. Bogusz-Koziarska, E. Sochacka, J. Boncela and M. A. Kowalska, Sci. Rep., 2019, 9, 2165 CrossRef PubMed.
- K. Espeland, A. Kleinauskas, P. Juzenas, A. Brech, S. Darvekar, V. Vasovic, T. Warloe, E. Christensen, J. Jahnsen and Q. Peng, Biomedicines, 2022, 10, 232 CrossRef CAS PubMed.
- J.-Y. Pierga, F.-C. Bidard, C. Mathiot, E. Brain, S. Delaloge, S. Giachetti, P. de Cremoux, R. Salmon, A. Vincent-Salomon and M. Marty, Clin. Cancer Res., 2008, 14, 7004–7010 CrossRef CAS PubMed.
- W. J. Janni, B. Rack, L. W. M. M. Terstappen, J.-Y. Pierga, F.-A. Taran, T. Fehm, C. Hall, M. R. de Groot, F.-C. Bidard, T. W. P. Friedl, P. A. Fasching, S. Y. Brucker, K. Pantel and A. Lucci, Clin. Cancer Res., 2016, 22, 2583–2593 CrossRef CAS PubMed.
- M. Karhade, C. Hall, P. Mishra, A. Anderson, H. Kuerer, I. Bedrosian, S. Krishnamurthy and A. Lucci, Breast Cancer Res. Treat., 2014, 147, 325–333 CrossRef CAS PubMed.
-
H. Bittersohl and W. Steimer, in Personalized Immunosuppression in Transplantation, ed. M. Oellerich and A. Dasgupta, Elsevier, 2016, pp. 199–226 Search PubMed.
- M. Kräter, S. Abuhattum, D. Soteriou, A. Jacobi, T. Krüger, J. Guck and M. Herbig, Adv. Sci., 2021, 8, 2003743 CrossRef PubMed.
- Z. Du, Y. Li, B. Chen, L. Wang, Y. Hu, X. Wang, W. Zhang and X. Yang, Lab Chip, 2022, 22, 3390–3401 RSC.
- P. Eulenberg, N. Köhler, T. Blasi, A. Filby, A. E. Carpenter, F. J. Theis, F. A. Wolf and P. Rees, Nat. Commun., 2017, 8, 463 CrossRef PubMed.
- H. Kobayashi, C. Lei, Y. Wu, C. J. Huang, A. Yasumoto, M. Jona, W. Li, Y. Wu, Y. Yalikun, Y. Jiang, B. Guo, C. W. Sun, Y. Tanaka, M. Yamada, Y. Yatomi and K. Goda, Lab Chip, 2019, 19, 2688–2698 RSC.
- E. J. Gualda, H. Pereira, G. G. Martins, R. Gardner and N. Moreno, Cytometry, Part A, 2017, 91, 144–151 CrossRef PubMed.
- J. Wu, J. Li and R. K. Y. Chan, Opt. Express, 2013, 21, 14474 CrossRef CAS PubMed.
- N. Nitta, T. Sugimura, A. Isozaki, H. Mikami, K. Hiraki, S. Sakuma, T. Iino, F. Arai, T. Endo, Y. Fujiwaki, H. Fukuzawa, M. Hase, T. Hayakawa, K. Hiramatsu, Y. Hoshino, M. Inaba, T. Ito, H. Karakawa, Y. Kasai, K. Koizumi, S. Lee, C. Lei, M. Li, T. Maeno, S. Matsusaka, D. Murakami, A. Nakagawa, Y. Oguchi, M. Oikawa, T. Ota, K. Shiba, H. Shintaku, Y. Shirasaki, K. Suga, Y. Suzuki, N. Suzuki, Y. Tanaka, H. Tezuka, C. Toyokawa, Y. Yalikun, M. Yamada, M. Yamagishi, T. Yamano, A. Yasumoto, Y. Yatomi, M. Yazawa, D. Di Carlo, Y. Hosokawa, S. Uemura, Y. Ozeki and K. Goda, Cell, 2018, 175, 266–276 CrossRef CAS PubMed.
- A. Isozaki, H. Mikami, H. Tezuka, H. Matsumura, K. Huang, M. Akamine, K. Hiramatsu, T. Iino, T. Ito, H. Karakawa, Y. Kasai, Y. Li, Y. Nakagawa, S. Ohnuki, T. Ota, Y. Qian, S. Sakuma, T. Sekiya, Y. Shirasaki, N. Suzuki, E. Tayyabi, T. Wakamiya, M. Xu, M. Yamagishi, H. Yan, Q. Yu, S. Yan, D. Yuan, W. Zhang, Y. Zhao, F. Arai, R. E. Campbell, C. Danelon, D. Di Carlo, K. Hiraki, Y. Hoshino, Y. Hosokawa, M. Inaba, A. Nakagawa, Y. Ohya, M. Oikawa, S. Uemura, Y. Ozeki, T. Sugimura, N. Nitta and K. Goda, Lab Chip, 2020, 20, 2263–2273 RSC.
- A. Isozaki, H. Mikami, K. Hiramatsu, S. Sakuma, Y. Kasai, T. Iino, T. Yamano, A. Yasumoto, Y. Oguchi, N. Suzuki, Y. Shirasaki, T. Endo, T. Ito, K. Hiraki and M. Yamada, Nat. Protoc., 2019, 14, 2370–2415 CrossRef CAS PubMed.
- A. A. Nawaz, M. Urbanska, M. Herbig, M. Nötzel, M. Kräter, P. Rosendahl, C. Herold, N. Toepfner, M. Kubánková, R. Goswami, S. Abuhattum, F. Reichel, P. Müller, A. Taubenberger, S. Girardo, A. Jacobi and J. Guck, Nat. Methods, 2020, 17, 595–599 CrossRef CAS PubMed.
- X. Chen, Y. Gu, J. Chen, C. H. Lee, I. Gagne, R. Tang, L. Waller, Z. Zhang, A. C. Zhang, Y. Han, W. Wang, I. Y. Lian, S. H. Cho and Y. H. Lo, APL Photonics, 2020, 5, 040801 CrossRef CAS.
- D. Schraivogel, T. M. Kuhn, B. Rauscher, M. Rodríguez-Martínez, M. Paulsen, K. Owsley, A. Middlebrook, C. Tischer, B. Ramasz, D. Ordoñez-Rueda, M. Dees, S. Cuylen-Haering, E. Diebold and L. M. Steinmetz, Science, 2022, 375, 315–320 CrossRef CAS PubMed.
- M. Yu, D. T. Ting, S. L. Stott, B. S. Wittner, F. Ozsolak, S. Paul, J. C. Ciciliano, M. E. Smas, D. Winokur, A. J. Gilman, M. J. Ulman, K. Xega, G. Contino, B. Alagesan, B. W. Brannigan, P. M. Milos, D. P. Ryan, L. V. Sequist, N. Bardeesy, S. Ramaswamy, M. Toner, S. Maheswaran and D. A. Haber, Nature, 2012, 487, 510–513 CrossRef CAS PubMed.
- L. Zhao, X. Wu, T. Li, J. Luo and D. Dong, Database, 2020, 2020, baaa020 CrossRef CAS PubMed.
- J. E. Lang, A. Ring, T. Porras, P. Kaur, V. A. Forte, N. Mineyev, D. Tripathy, M. F. Press and D. Campo, Ann. Surg. Oncol., 2018, 25, 2261–2270 CrossRef PubMed.
- N. Aceto, A. Bardia, D. T. Miyamoto, M. C. Donaldson, B. S. Wittner, J. A. Spencer, M. Yu, A. Pely, A. Engstrom, H. Zhu, B. W. Brannigan, R. Kapur, S. L. Stott, T. Shioda, S. Ramaswamy, D. T. Ting, C. P. Lin, M. Toner, D. A. Haber and S. Maheswaran, Cell, 2014, 158, 1110–1122 CrossRef CAS PubMed.
- W. T. Yan, X. Cui, Q. Chen, Y. F. Li, Y. H. Cui, Y. Wang and J. Jiang, Sci. Rep., 2017, 7, 43464 CrossRef CAS PubMed.
- W. Zheng, Y. Zhang, L. Guo, S. Wang, M. Fang, W. Mao and J. Lou, Cancer Manage. Res., 2019, 11, 5857–5869 CrossRef CAS PubMed.
- H. Pei, M. Yu, D. Dong, Y. Wang, Q. Li, L. Li and B. Tang, Chem. Sci., 2020, 11, 8895–8900 RSC.
- S. Maheswaran, L. V. Sequist, S. Nagrath, L. Ulkus, B. Brannigan, C. V. Collura, E. Inserra, S. Diederichs, A. J. Iafrate, D. W. Bell, S. Digumarthy, A. Muzikansky, D. Irimia, J. Settleman, R. G. Tompkins, T. J. Lynch, M. Toner and D. A. Haber, N. Engl. J. Med., 2008, 359, 366–377 CrossRef CAS PubMed.
|
This journal is © The Royal Society of Chemistry 2023 |
Click here to see how this site uses Cookies. View our privacy policy here.