DOI:
10.1039/D2LC00020B
(Perspective)
Lab Chip, 2023,
23, 1358-1375
Lab on a chip for a low-carbon future
Received
7th January 2022
, Accepted 27th December 2022
First published on 15th February 2023
Abstract
Transitioning our society to a sustainable future, with low or net-zero carbon emissions to the atmosphere, will require a wide-spread transformation of energy and environmental technologies. In this perspective article, we describe how lab-on-a-chip (LoC) systems can help address this challenge by providing insight into the fundamental physical and geochemical processes underlying new technologies critical to this transition, and developing the new processes and materials required. We focus on six areas: (I) subsurface carbon sequestration, (II) subsurface hydrogen storage, (III) geothermal energy extraction, (IV) bioenergy, (V) recovering critical materials, and (VI) water filtration and remediation. We hope to engage the LoC community in the many opportunities within the transition ahead, and highlight the potential of LoC approaches to the broader community of researchers, industry experts, and policy makers working toward a low-carbon future.
Introduction
The 6th report of the Intergovernmental Panel on Climate Change (IPCC)1 emphasizes that “the only path out [of climate change] demands intensive, immediate efforts to bring net-carbon emissions to zero, combined with unprecedented efforts to extract carbon from the atmosphere”. The International Energy Agency (IEA) Net Zero by 2050 report2 provides some clarity on how this transition may be accomplished by mid-century. As noted in the IEA report, it will require “a wide-spread transformation of the energy systems that underpin our economies… [because] the energy sector is the source of around three-quarters of greenhouse gas emissions today and holds the key to averting the worst effects of climate change, perhaps the greatest challenge humankind has faced”.
Lab-on-a-chip (LoC) systems can help address this challenge by levering the attributes of high spatiotemporal resolution and excellent environmental control, and do so in two distinct ways. First, LoCs can provide insight into the fundamental physical and geochemical processes underlying many of the solutions outlined in the IEA roadmap, ranging from carbon sequestration to alternative approaches to energy storage and extraction. Second, they can be used for exploration, development, and testing of new processes and materials required to develop such solutions. LoC techniques can provide critically important insights for the design and optimization of environmental/engineered approaches and—when combined with physical upscaling approaches to translate the results of idealized microscale studies to the field—aid in our transition to a low-carbon future.
In this perspective article, which brings together speakers from a recent symposium on Microfluidics & Energy, we outline some of the most important opportunities for LoC systems in this future. Our goal is not to present a comprehensive overview of all the literature in this field, but rather, to highlight some areas of research we consider to be particularly promising, and outline the challenges and opportunities ahead. Some of the application areas are relatively new to the LoC community, whereas in others there is a track record of LoC contributions. In the more established areas of application, we highlight more comprehensive review articles that describe important developments using LoC systems, and instead focus on assessing the benefits provided by LoC and point to the challenges and opportunities ahead.
We first describe the use of LoC systems applied to (I) subsurface carbon sequestration, considered a key pillar of decarbonization,2 and (II) subsurface hydrogen storage, as well as the related process of (III) geothermal energy extraction. We then describe applications of LoC systems for (IV) bioenergy, another key pillar in decarbonization plans. Transition to a low-carbon future will also require electrification of vehicular transport and industrial processes with a concomitant expansion of renewable electricity generation. The scaling of solar, wind, and battery production will demand incredible material resources and present another opportunity for LoC systems: to inform the development of sustainable approaches for extracting these (V) critical material resources. Finally, we note that progress toward a low-carbon future requires advances not just in the management of greenhouse gas emissions and in the adoption of alternative energy sources, but also requires water security. Thus, we end by describing ways in which LoC systems can address challenges associated with (VI) water filtration and remediation. Microscale transport phenomena, more generally, are integrated within a wide array of relevant energy systems including fuel cells3 and electrolyzers for CO2-reduction4 and hydrogen-generation;5 however, we limit the scope here to chip-based approaches native to the LoC community. Likewise, we note that there are areas of importance for which the microfluidic toolkit appears to be poorly suited: for example, renewable power generation by solar, wind, and nuclear energy—all areas relevant to our low carbon future, but largely out of reach for LoC methods. Our motivation for this article is to engage the LoC community in considering the many opportunities within the transition ahead. Likewise, we highlight the potential of LoC approaches to the broader community of researchers, industry experts, and policy makers supporting the energy transition.
I. Sequestering carbon
The IEA net-zero report2 notes that CO2 capture, utilization, and sequestration (CCUS) “is the only group of technologies that contributes both to reducing emissions in key sectors directly and to removing CO2 to balance emissions that cannot be avoided”, and is essential in the pursuit of a net-zero future. CCUS can take several forms, beyond capturing and storing emissions from natural gas and from coal-fired power plants, to include the production of low-carbon hydrogen via steam methane reforming combined with CCUS (termed “blue” hydrogen), and carbon dioxide removal from the atmosphere via bioenergy with CCS (BECCS) or direct air capture (DAC) and subsequent storage. The most established means of CO2 storage is geological, injecting CO2 into the subsurface (Fig. 1A).6–9 Here we outline ways in which LoC systems have provided, and continue to provide, scientific insights to inform effective approaches to geological carbon storage.
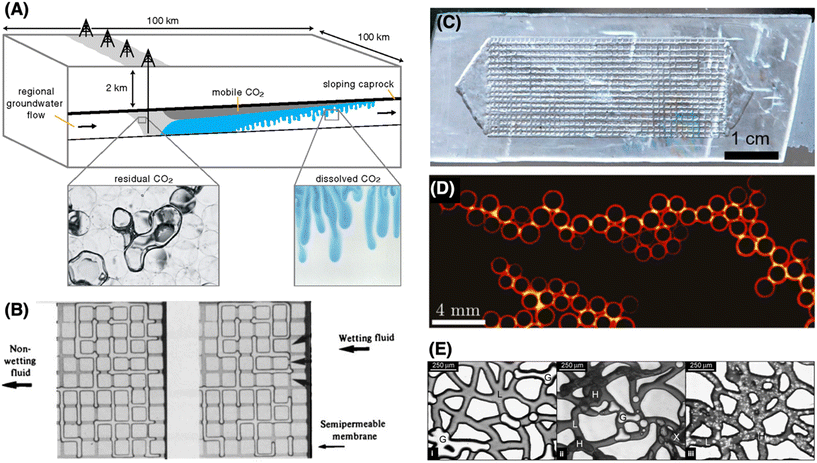 |
| Fig. 1 Lab-on-a-chip visualization of multiphase flow processes relevant to carbon sequestration. (A) Schematic of subsurface CO2 storage describing the two key trapping mechanisms that contribute to storage capacity, residual trapping (left inset) and solubility trapping (right).10 (B) Snap-off of wetting films leading to capillary trapping of gas during imbibition.11 (C) Geometrically and chemically representative real-rock micromodel for reservoir engineering studies.12 (D) Snapshot from strong imbibition illustrating invasion by corner flow: the invading fluid advances by coating the perimeters of the posts rather than by filling the pore bodies; pendular rings link the coated posts, forming chains.13 (E) Snapshots of methane hydrate formation in a micromodel.14 i: Bubbles of methane (G) and liquid (L) prior to hydrate formation. ii: Newly formed hydrates (H), including encapsulated gas bubbles (X), prior to complete conversion to clathrate. iii: Hydrate redistribution after 2 days. | |
Multiphase flow physics underlying subsurface CO2 storage.
Geological CO2 storage in the subsurface is controlled by the dynamics of multiphase flow through porous media.15–17 In particular, several of the trapping mechanisms like capillary trapping and solubility trapping depend strongly on the interplay between capillary forces, viscous forces and wettability characteristics of the porous rock. Given that most porous media (including all natural rocks) are disordered, rough and opaque, microfluidics has played a key role in advancing our understanding of multiphase flow at the pore level by enabling precise control of geometry and surface properties, and allowing direct visualization. This is perhaps best exemplified by early work employing glass-etched networks11,18,19 to uncover the pore-scale mechanisms responsible for fluid–fluid displacement and trapping (Fig. 1B), which allowed synthesizing the behavior of drainage (the displacement of a wetting fluid by a nonwetting fluid) in the form of a now classic phase diagram.20
In addition to fluid transport through networks, CO2 is highly reactive with minerals found naturally into the subsurface. CO2 injection into a brine-filled carbonate formation typically results in the formation of acid that dissolves carbonate minerals, forming wormholes and large channels that can affect the integrity of the rock. LoC technology has evolved and gained sufficient sophistication to provide progressively more realistic analogues of geologic media, including so-called 2.5D chips,21 transparent 3D packings of particles,22–24 and micromodels that replicate the pore space and chemistry in actual rocks25 and the pressure–temperature conditions in the subsurface12,26,27 (Fig. 1C). The reproducibility of such micromodels provides a unique opportunity to disentangle the effect of fluid mechanics and thermodynamics from rock/soil pore geometry and mineralogy, particularly in the context of unstable multiphase flows.28,29
Given the importance of the wettability characteristics of the rock in the process of CO2 migration and trapping,30–32 there has been an increased interest in extending our understanding of multiphase flow in porous media from drainage to imbibition (the displacement of a nonwetting fluid by a wetting fluid).33 Here, once again, LoCs have been a key enabling technology allowing for precise control over the wettability characteristics, including fabrication techniques based on polymers whose surface properties can be engineering through exposure to UV light.34,35 These and other microfluidic fabrication protocols have then been used to elucidate wetting transitions in imbibition13,36,37 (Fig. 1D), help benchmark pore-scale simulation models of multiphase flow,38 and guide the extension of Lenormand's diagram to all wettability characteristics.39
Microfluidics have provided new insights into CO2 behavior for subsurface storage, including invasion patterns of CO2 displacing the fluids in model porous media,40–43 characterizing the physical properties of CO2 in the supercritical state,44–46 and screening CO2 solubility in various solvents and brines for delivery.47,48 Although CO2 mineralization occurs on geological timescales, microfluidic devices have provided new insights into how CaCO3 precipitates along the transverse mixing zone, thus substantially reducing porosity and permeability, which can limit the formation of more stable carbonate phase, such as calcite.49–52
Reproducing the chemical properties of subsurface media, in addition to their structure, can be necessary to assess and predict system reactivity during carbon injection and storage. This need has led to the development of functionalized microfluidics devices whose surfaces are directly coated by geomaterials.53 More recent advancements have enabled researchers to directly embed real rock samples in microfluidic chips, enabling observations of chemical reaction progression and resulting alterations in pore space topology in real time,54 thereby helping to elucidate feedbacks between multiphase transport and chemical reactions.55–57
While microfluidics has already enabled a wealth of observations on the fluid-flow physics of carbon storage in the past two decades,58–60 several knowledge gaps remain. We expect that LoC systems will continue to offer a fantastic testbed to address those gaps, including in: (i) elucidating the influence of mixed wettability, both at the sub-pore level61,62 and at the level of heterogeneous patches involving many pores,63 on the flow behavior; (ii) upscaling dynamic macroscopic multiphase flow descriptors such as relative permeability and capillary pressure;64,65 (iii) examining the influence of partial dissolution66 and compositional effects on multiphase flows in multicomponent systems, which we briefly describe next; and (iv) studying chemical reactions (mineral dissolution and carbonate precipitation) coupled with permeability evolution in in situ CO2 mineralization.51 In each of these examples, LoC systems are particularly useful, given that they enable researchers to create repeatable experimental samples and change flow and thermodynamic conditions systematically—providing insights that could help practitioners select optimal operating conditions. Another emerging application of LoC systems is as sensors of CO2 leakage into water, providing a potentially fast, cheap, and reliable way to monitor reservoir integrity.67
CO2 foams.
CO2 foams are being applied for enhanced oil recovery and there is significant interest in using foams to assist with improved subsurface sequestration.68,69 The use of CO2 as foam in the subsurface can control the mobility of the gas and limit the extent of fingering and gravity override.29 Indeed, foams in microfluidic porous media have been shown to increase the volume of gas trapped in the swept zone and reduce the amount of gas entering thief zones.70,71 Foams are typically generated by mixing gas with aqueous chemical surfactants and their stability is highly dependent on gas-type and capillary pressure,72 which is influenced by rock geometry.73,74 Although strong foams can be generated, in practice, they typically collapse in the presence of organics, thus limiting their transport deep into the reservoir. However, microfluidic studies of foams in porous media have shown in situ foam generation mechanisms that are able to regenerate foam in porous media. Therefore, in recent years, studies of foam dynamics in micro-channels and porous-media chips have provided fundamental new insights into CO2 foam formation mechanism, oil and water displacements, and the effect of permeability on CO2 foam migration.35–38 Deep learning algorithms have also been employed to process images taken during the tests, allowing the determination of quantitative information from visual monitoring.39
An avenue to obtain a stable foam is the use of nanoparticles with or without conventional surfactant, as nanoparticles adsorb irreversibly at the gas–liquid interface.75 This extraordinary property of nanoparticles enables the formation of strong foams even for gas fractions as large as 97 vol% of CO2—a feature that has sparked the exploration of CO2 foams in applications like geologic CO2 storage, geothermal energy production, and enhanced oil recovery.76–79 Traditionally, identification of the optimal conditions for the foam-nanoparticle system requires costly and time-consuming experiments in conventional column-flood setups. The possibility of conducting chemical screening experiments using microfluidic systems is therefore an attractive alternative.
In gas-injection operations where the gas phase is delivered as a foam and it is supposed to react with the liquid and the rock, the knowledge of mass transfer kinetics from gas-bubble to the liquid is critical. This is the case of carbon sequestration in deep aquifers or fractured rocks where CO2 is injected as a foam, dissolves into the liquid phase, and in some cases it can react with the rock minerals. To study mass transfer kinetics at reservoir conditions,80 high-pressure microfluidic systems have been built. In the application by Martin Ho et al. (2021) the analysis of the evolution of single CO2 bubbles32 yielded the conclusion that CO2 mass transfer kinetics at reservoir conditions is controlled by the Reynolds number and is independent from the pressure, contrarily to CO2 mass transfer kinetics at atmospheric conditions.81
Given this tremendous potential, we expect that moving forward, LoC systems will provide useful insights with which to address key remaining challenges: (i) elucidating nanoparticle-foam dynamics, including quantitatively describing coalescence and trapping of the bubbles and nanoparticle interaction with the gas–liquid interface; (ii) exploring foam dynamics in porous media of varying and mixed wettabilities akin to natural systems; (iii) monitoring the injection process to ensure safety and validate models; and (iv) screening surface-active materials, such as surfactants, nanoparticles, or combinations of them, to select for optimal compositions and concentrations to guide larger-scale testing at reservoir conditions. Future research should focus on these next challenges and provide a deep understanding of nanoparticle-foam evolution and transport in porous media.
CO2 and methane hydrates.
A gas hydrate is an ice-like solid that forms from a gas–water mixture under sufficiently high pressure and low temperature. For CO2 and methane (CH4), the conditions for hydrate stability are reached in the ocean floor at depths of about 500 meters and deeper, or in permafrost regions. Methane, in particular, is a potent greenhouse gas, yet methane fluxes in both terrestrial and marine settings are poorly understood, raising fundamental questions about the role of methane hydrates in past and future climatic change.82
Experimental observations using microfluidics have provided important insights into the mode of hydrate growth in multiphase gas–water systems14,83,84 (Fig. 1E), including the role of wettability.85 These laboratory observations, along with many field observations, suggest that most hydrate systems are out of equilibrium,86 a feature that explains the co-existence of gas and hydrate in nature,87 and points to challenges in scaling up the tantalizing proposal of simultaneous CO2 storage and CH4 production from natural gas hydrate.88
LoC systems can help address these challenges moving forward—for example, in improving quantitative descriptions of the kinetics of hydrate formation and dissociation in porous media, including near wellbores, where the permeability reduction due to hydrate growth may impede fluid transport. Indeed, given the stochasticity inherent in hydrate formation, LoC systems may provide a useful way to obtain statistically significant data that may otherwise be challenging to obtain.89,90 However, it is important to note that in field conditions, ice and hydrates—which may be challenging to visually differentiate from each other—can coexist; thus, it may be necessary to couple LoC systems with non-invasive analytical techniques such as micro-Raman spectroscopy.91–93
II. Storing hydrogen
The transition from fossil fuels to renewable energy sources is one of the key measures to mitigate climate change and build a sustainable, reliable, and secure energy supply system.1 However, widespread application of renewable energy will require a means of storing electrical energy on an unprecedented scale, which will likely require chemical forms of energy storage. Hydrogen (H2) is an emission-free energy carrier that can be produced from surplus renewable electricity (“green” hydrogen), with specific energy (MJ kg−1) almost three times higher than natural gas. Hence, H2 can have a major role in the energy transition; indeed, as noted in the IEA net-zero roadmap,2 “The initial focus for hydrogen use in the NZE (Net Zero Emissions) plan is the conversion of existing uses of fossil energy to low-carbon hydrogen in ways that do not immediately require new transmission and distribution infrastructure. This includes hydrogen use in industry and in refineries and power plants, and the blending of hydrogen into natural gas for distribution to end-users”. Available storage capacity today is, however, insufficient to balance supply and local demand for future renewable energy source implementation, and H2 storage capacity must improve to accommodate widespread implementation. Similar to CO2 sequestration, subsurface, porous, geological formations like saline aquifers and depleted natural gas reservoirs represent widely available large-scale H2 storage capacity.9
Such subsurface porous formations offer large working gas capacities, worldwide distribution, and were therefore recently identified as the most cost-effective H2 subsurface storage option.94 However, storage in porous geological formations will cause a variety of effects that impact storage capacity including H2 migration, leakage, trapping and activation of indigenous microbial metabolic groups (Fig. 2A). Therefore, similar to the case of geological sequestration of CO2, understanding fluid migration patterns is crucial. Additionally, intermittent H2 withdrawal requires new fundamental understanding of coupled subsurface H2 flow/microbial processes. We describe here ways in which LoC systems provide scientific insights to inform effective approaches to geological H2 storage.
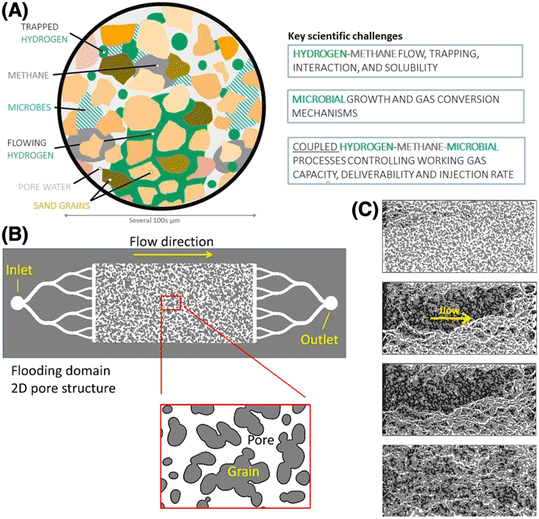 |
| Fig. 2 Lab-on-a-chip visualization of multiphase flow processes relevant to hydrogen storage. (A) Schematic of subsurface H2 storage describing the underlying pore-scale multi-phase interactions. (B) Example of a 2D microfluidic chip for studying the relationship between microbial growth and hydraulic properties at the pore scale.115 (C) Images from the chip in (B) showing the phase distribution in time steps during a nutrient flooding (NF) experiment in the total flooding domain; top to bottom after 16, 23, 29, and 40 h of NF, respectively. The images are segmented in three phases: white is the open pore space, light gray denotes the grains, and black is the biomass. | |
Multiphase flow physics underlying subsurface H2 storage.
With scarce literature, few research projects worldwide, limited industrial testing at scale, and lack of dedicated laboratory data there is an obvious need for focused studies to assess coupled processes for subsurface H2 storage in porous media. To our knowledge, only few laboratory studies assessing porous media H2 flow are available at the time of writing.95–97 Relevant publications mostly use numerical simulation98 to test new conceptual approaches towards e.g., feasible strategies and impact of geological heterogeneity. Overall, most simulations use scaled or extrapolated flow data not specifically measured for H2; this approach represents a significant uncertainty. A broader characterization of coupled parameters that determine working gas capacity, deliverability and injection rate is needed, including flow functions, bio-chemical reactions, and biotic gas consumption and conversion. An even more basic uncertainty that also needs to be addressed is whether H2 permeates through caprocks that are known to be effectively sealing to CO2 and CH4: are they sealing to H2 as well, and if not, what is the leakage rate? Despite the extensive knowledge developed on CO2 storage in the past decades, the unique physical, chemical, and molecular properties of H2 render its dynamics and interactions with geologic seals, the host rock, the brine and resident fluids largely unknown.
Flow functions and physically unrecoverable gas largely determines working gas capacity, deliverability and injection rates. Residual gas trapping, relevant for saline aquifer H2 storage and frequently discussed for CO2 sequestration,16,99 is not described in the context of H2 subsurface porous media storage. Residual trapping occurs when the injected gas breaks into discontinuous bubbles that becomes trapped, reducing working gas capacity as residually trapped H2 is not available for withdrawal. Disconnected bubbles may reconnect during subsequent H2 injection, described as hysteresis. This is particularly important when establishing a H2 storage site. Hysteresis is rarely discussed for H2 subsurface porous media, although described for nano-porous carbon tubes.100 Contact angle measurements improve understanding of wettability and capillary pressure and relative permeability hysteresis. However, hydrogen contact angles are often still not appropriately investigated, with lack of consistent and systematic approaches. To our knowledge, hydrogen contact angles were only recently derived for basaltic101 and measured for quartz102 and sandstone103 rocks.
Overall, pore-scale displacement and trapping mechanisms are widely investigated for CO2 storage,104–106 but remain largely unaddressed for hydrogen. To the best of our knowledge, the first systematic experimental study on a H2/water/sandstone rock system at the core scale (mm cm−1 scale) under shallow and deep aquifer pressure and temperature conditions was only performed recently,96 providing useful X-ray CT visualization of the flow behavior of hydrogen in initially water saturated rock for both drainage and imbibition. The authors also determined the wettability of the system and identified complex displacement patterns including gravity segregation and enhancement of spreading. These forms of direct pore scale observations of hydrogen displacement and trapping mechanisms at the sub-mm scale are crucial for understanding of hydrogen flow physics during underground storage. Storage in depleted gas reservoirs must account for H2 mixing and interaction with CH4, and dedicated H2 flow functions with increasing CH4 share are needed. Additionally, a fundamental understanding of the impact of injection–withdrawal cycles on trapping, mixing and reactions is necessary. As demonstrated in the context of the formation of chemical hotspots due to intermittent oxygen delivery in fractured rocks,107 temporal cycling may lead to subsurface biogeochemistry to be spatially and temporally dynamic. New modular designs of microfluidics allowing the creation of temporally varying inlet concentration signals108 could provide a way to elucidate the impact of H2 cycling on many important biogeophysicochemical processes.
Microbial effects.
Microbial activity determines H2 loss in the subsurface,109 and increased understanding of microbial processes (activity, growth rates and gas conversion) is crucially needed. Depleted gas reservoirs and saline aquifers are not sterile environments (microbial life occurs up to 120 °C without thresholds for pressure or brine salinity), and contain a variety of indigenous or anthropogenically introduced anaerobic microorganisms (both bacteria and archaea) that use H2 as electron donor. Methanogenic, acetogenic, and sulphate-reducing bacteria are the most important groups110 as they can convert H2 to CH4, generate hydrogen sulfide (H2S) that renders produced gases toxic and unusable, influence water chemistry, and reduce injection rates. Many microbial issues for hydrocarbon production, CO2 sequestration and geothermal storage are known,111–113 whereas bio-geochemical reactions relevant for subsurface H2 storage are poorly understood and often only inferred from shallow environments like lakes or soil. Hence, little is known about the extent of H2 loss in subsurface porous media for specific microbial groups, and how they are influenced by high H2 partial pressures. It is known that higher H2 partial pressure in shallow environments can strongly inhibit H2-related enzymes and growth;114 however, it is not known if microbial activity is suppressed during subsurface H2 storage. Hence, LoC approaches to studying microbial activity in porous media (such as that shown in Fig. 2B and C,115 as well as those further detailed in IV and other recent publications116–118) will be critical to addressing these uncertainties.
III. Enabling geothermal energy
Geothermal energy—the accessible thermal energy resource in the subsurface—holds the potential to provide 150 GWe of electrical energy by 2050, or ∼5% global electricity generation.119 It is renewable and flexible; geothermal resources can be found globally and run continuously, with the potential to provide for baseload power needs, or respond to demand. Current geothermal electricity generation installed capacity is roughly 10% of this 2050 goal (with 16 GWe). Geothermal has a particularly important niche among renewables: it is dispatchable, and can thus be responsive to swings in demand or other supplies. In contrast, solar and wind are intermittent. Other forms of low-carbon energy generation such as nuclear energy can provide for steady, baseload, but they cannot respond to demand spikes nor can they compensate for the intermittency of solar and wind. Geothermal energy is unique in this regard.
As noted by the US Department of Energy in the description of a recent grant opportunity,120 “Only a fraction of this potential has been realized due to technical and non-technical barriers that constrain industry growth”. Some of the key challenges to massively scaling geothermal energy by 2050 are developing means to extract more energy from conventional geothermal hot-spots, and the means to tap energy from the deep subsurface—a breakthrough that would enable both scaling and much broader application of renewable geothermal power production.
LoC systems are contributing to the expansion of geothermal energy recovery in two ways: micromodel-based study of fluid and heat transport in the subsurface, and thermal fluid testing and development. Here we describe these two approaches and the opportunities they present (Fig. 3).
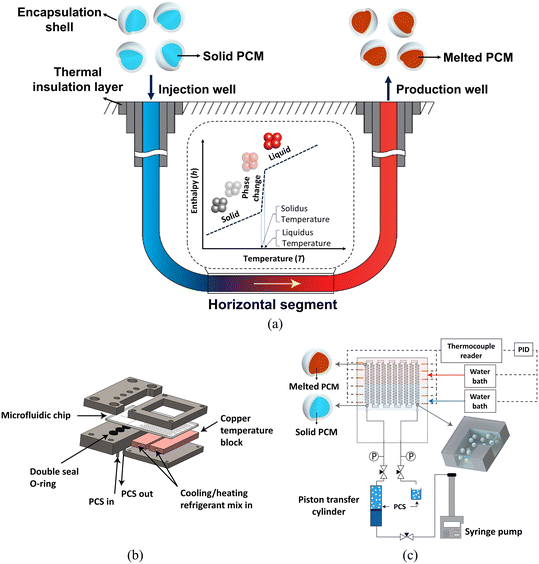 |
| Fig. 3 Lab-on-a-chip analysis of processes relevant to geothermal energy. A closed-loop geothermal system (a) is a closed system, and can benefit from specialized working fluids. The work highlighted here employs LoC methods (b and c) to assess the potential for a microencapsulated phase change slurry as a working fluid, with testing at temperatures, pressures and shear-rates relevant to large scale operations. Reprinted with permission from ref. 121 copyright 2021 American Chemical Society. | |
Micromodels of geothermal reservoirs.
Here the field can leverage the extensive toolkit of microfluidic methods developed for oil and gas technologies,122 and particularly those applied to higher temperatures and pressures, such as steam-on-chip.123 Many enhanced geothermal sources suffer from poor fluid distribution through the subsurface, limiting the heat transfer potential. Thus, a pressing current challenge is to develop ways to control flow of fluids in subsurface geothermal reservoirs to promote heat transfer. Micromodel-based studies of fluid and heat transport, particularly in structurally heterogeneous124 and fractured media, have yielded important insights in oil/gas recovery—and will therefore be particularly useful in addressing these conceptually similar problems in geothermal settings. For example, micromodels provide a unique opportunity to screen the utility of methods traditionally used for conformance control in oil/gas recovery (e.g., use of gels,125 polymers,126,127 foams122) in promoting fluid mixing, homogeneous transport, and heat transfer from hot rock to address this challenge in geothermal energy.
Thermal fluid testing and development.
Efforts to develop advanced thermal working fluids can benefit from the characteristic control and speed offered by LoC testing, but with a new emphasis—thermal properties. To date, LoC approaches have focused on chemical, biological and to a lesser extent, physical properties of fluids. To enable the next generation of thermal working fluids for geothermal and other applications, LoC approaches that can provide thermal properties with high accuracy and throughput are needed.121,128 This is a multifaceted fluid optimization challenge, with the full combination of thermal properties (such as heat capacity) and physical properties (such as density and viscosity) and other factors such as stability and cost influencing the ultimate applicability.121,128 LoC systems can provide critically important data with which to navigate this broad landscape of physical, chemical and thermal properties.
Looking ahead, there are additional opportunities to expand the pressure and temperature range of LoCs—both for microfluidic testing methods, but also for in-field operations. One of the challenges in predicting and optimizing the power output of a geothermal plant is to estimate the flow rate and temperature based on the injection rate, fluid composition, and the structure of the medium. Seismic data for each site provide some of the basis needed for computational modeling and assessment of the viability of an operation. However, this area could be greatly accelerated with machine learning, suitably combined with the high-throughput approaches afforded by LoC systems, as in other application areas.129–131 In particular, the application of machine learning here will require large training data sets under controlled experimental conditions, well beyond that available currently. The development and testing of machine learning algorithms are identified as major research challenges by the US Department of Energy.132,133 Here, there is a clear path for LoCs to contribute as a provider of large quantities of accurate measurements to serve as training data sets. Here the community can make use of fabrication techniques, and approaches to control wetting conditions and withstand high pressures, as developed previously for the oil and gas industry.134 There are additional opportunities for LoCs in assessing upscaling (physics-based) models.135 The LoC community has many relevant technologies, as well as new opportunities, to aid in the expansion of renewable geothermal energy harvesting.
IV. Enabling bioenergy
Decarbonization of transportation is a critical step in reducing global greenhouse gas emissions. Electrification of light-duty vehicles is already playing a role, but not all types of transportation can be readily electrified, e.g. shipping, aviation. Fuels derived from organic matter (biofuels) are expected to contribute to the equivalent of 40–50 Mboe per day,136,137 ∼8% of the world's primary energy demand by 2030. Assuming an average oil production carbon intensity of 10.3 gCO2 MJ−1,138 the annual volume of biofuels would represent an addition 1 GT CO2 equivalent of emissions were these barrels produced from fossil fuels. Biofuels represent a sustainable fuel option that could help close the carbon cycle: plants utilize CO2 to produce organic compounds that can be converted into hydrocarbons suitable for ethanol, biodiesel, and other fuels. If applied with carbon capture and sequestration, energy production from biofuels (bioenergy) has potential for net-negative emissions—but a great deal of technological innovation is needed to realize that potential (Fig. 4).
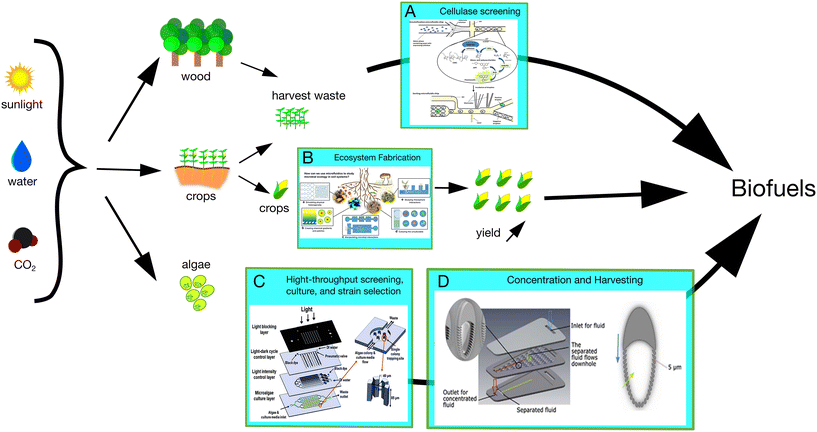 |
| Fig. 4 Lab-on-a-chip studies of processes relevant to bioenergy. A variety of feedstocks, each one having its own pathway, will contribute to the production increase to meet demand in biofuels. Lab-on-a-chip approaches can substantially help in optimizing industrial processes, for example by: (A) increasing high-throughput screening of cellulase activity,139 (B) understanding soil function and optimizing interactions,140 (C) easily screening multiple relevant conditions to increase microalgae yield,141 and (D) finding the physico-chemical conditions allowing to pre-concentrate and harvest microalgae.142 | |
One outstanding issue in first generation biofuels is that, in practice, crops use valuable land and water resources at large scale; ways to address this demand are therefore of critical importance, particularly because most of the crops used are food sources as well. Indeed, while widespread application of chemical fertilizers enabled crop yields to increase fast enough to meet growing demand over the past century, such yield increases are slowing and leveling off. One way of addressing this issue is through scientific research to develop new ways to increase crop yield. As noted in the IEA Net Zero 2050 report:2 “Most liquid biofuels produced today come from dedicated bioenergy crops such as sugarcane, corn or oil crops, often known as conventional biofuels. The expanded use of feedstocks and arable land to produce these biofuels can conflict with food production”. Thus, in the roadmap proposed by the IEA, there needs to be “a shift towards the use of sustainable, certified agricultural products and wood… also on advanced bioenergy feedstocks, including waste streams from other processes, short-rotation woody crops and feedstocks that do not require the use of arable land”. However, this promising approach of using second generation biofuels is not exempt from technological challenges that still need to be resolved through scientific research; as described below, LoC systems can be of great help due to the myriad of variables to be tested.
Biofuels from vegetables (crops and waste).
Growing understanding of the soil microbiome—the collection of microorganisms that inhabit and play key roles in the functioning of soil—has started to yield new insights into how to sustain healthy and productive soils. However, soil microbiome experiments have traditionally been laborious, time-consuming, and low-throughput. Recent developments in microfluidics have started to overcome these limitations.140,143 For example, Ecosystem Fabrication (EcoFAB) is an approach recently developed using traditional microfluidic technology to create controlled laboratory habitats for growing bioenergy plants on a chip and thereby enable mechanistic studies of plant–microbe interactions within specific environmental conditions.144 By enabling new investigation of how soil microbes interact with and help sustain plant roots, and potentially how the soil microbiome can be manipulated to improve plant growth, such approaches have potential to address current limitations in bioenergy. Moving forward, integrating such microfluidic approaches with developments in e.g. experimental tools to study processes in 3D “artificial soils”145–147 will potentially enable such lab-based studies to be even more representative of processes occurring in more complex natural media.
In second generation biofuels, a key step in converting biomass to useful fuels is the degradation of lignocellulosic plant cell walls, and the subsequent hydrolysis of cellulosic macromolecules into fermentable sugars. Selection of the right enzyme cocktail using a traditional approach is labor intensive and costly. The development and use of high-throughput screening microfluidic platforms have paved the way to overcome this hurdle,139,148 with 250-fold increases in throughput compared to automated systems reported in some cases. Further work is needed to screen for the right enzyme cocktails for different uses, particularly in a directed manner. Gaining insight on the hydrolysis mechanism will allow to direct enzyme screening studies. High resolution imaging techniques, such as synchrotron radiation, combined with microfluidics have already shown the potential to improve our understanding on this path.149 The existing data are limited, and more experiments combining microfluidics and analytics are needed in this area.
Biofuels from microalgae.
Algae is one alternative to conventional biofuel crops that does not require potable water or arable land; however, current production costs make algae biofuels uncompetitive in a market dominated by relatively cheap fossil fuels. Thus, further research—much of which can be done with LoC systems—is needed to develop ways to improve biofuel production from algae. Unlike crops, algae can produce large quantities of the lipids required for biofuel production without needing arable land and can be grown with non-potable water. Since algae are ∼20–50% lipid by weight, they have potential to produce ∼100 times more lipids per acre than traditional crop-based biofuel feedstocks. However, this potential still has not been realized due to the high energy cost of photobioreactors used to cultivate algae. Such reactors are designed to concentrate and pump CO2, manage oxygen accumulation (which is detrimental to algae growth), and maintain set temperatures so algae can optimally perform photosynthesis and maximize biomass production. However, identifying optimal photobioreactor conditions is still a challenge; therefore, microfluidic technologies have been used to do so in a high-throughput manner, as well as to screen different microalgae for optimal production.141,150–154
Most of the work performed thus far has focused on selecting strains and determining light and nutrient conditions to optimize growth rate and lipid production. The enormous number of conditions to be tested make this topic ideally suited for LoC systems to address, and certainly there is still work to be done along these lines. Nevertheless, algae production is only the first step of the overall process. The main bottleneck to make biofuel from microalgae economically viable is harvesting, which accounts for 60% of the costs.141 Microfluidic chip designs have already been tested to preconcentrate microalgae streams, but with limited success.142 A LoC approach allowing for parallelized high-throughput testing would be ideal to tackle this problem; it would allow screening of conditions leading to flocculation of the algae either by controlling physical–chemical conditions of by co-culture of different species that could induce flocculation via polymer-mediated depletion interactions, for example. Co-culture of different species could also have other benefits as rendering cultures more resilient, less prone to harmful contamination and widen the effective range of working conditions for the process.
V. Recovering materials for renewable energy
Transitioning toward a low-carbon future requires the large-scale adoption of renewable energy technologies. However, this adoption requires massive volumes of critical materials, such as arsenic, gallium, germanium, indium, tellurium, aluminum, rare earth elements, cobalt, lithium, graphite, and manganese.155 Between 2018 and 2019, global consumption of Li increased ∼18%, and by 2040, global demand for rare earth elements is expected to grow three to seven-fold its current (i.e., 2021) consumption.156,157 Current methods for production of these material resources rely on the extraction (e.g., surface mining, in situ mining) of ore and subsequent physicochemical processes (e.g., grinding, flotation, acid leaching, smelting, solvent extraction, electrolytic refining) to separate the elements of interest. Due to the mineral heterogeneity of the ore, minerals extraction and separation are energy intensive and involve massive volumes of environmental wastes. For example, the primary energy consumption required to produce titanium from ore is ∼263 MJ kg−1 due to its high melting point and bond strength.158 Greenhouse gas emissions from mineral and metals productions, as a result, totaled ∼3.6 × 1012 kg CO2e in 2018, or about 10% of total energy-related emissions that year.159
Thus, a set of opportunities arise for the LoC community to help produce the material resources required for low-carbon systems (Fig. 5). First, significant effort is required to optimize the current extraction and separation processes used to reduce energy consumed by the mining sector (∼1200 TBtu per year). Extracting metals from ore involves the reactive transport of reagents and metals of interest at sub-millimeter length scales that match those of microfluidics. Importantly, LoCs are well-suited both to understand physico-chemical processes and their coupling, such as reactivity and mixing,12,26 and to help develop and screen new reagent chemistries and reaction conditions to optimize the extraction process.134,160 For example, Yang et al. 2020 developed a microfluidic screening method to predict and remediate acid mine drainage, an environmental waste product of the mining industry.161 Even more recently, Le et al. (2022)162 applied microfluidics to investigate the performance of various solvent extraction methods for the recovery of critical minerals—minimizing the use of reactants and the time required for experiments. However, it is important to note that while LoC systems provide a fast and cost-effective way to screen multiple separation processes suitable for mineral extraction, larger-scale tests are essential for scaling up, given the limitations of LoC systems to mix fluids at small scales.
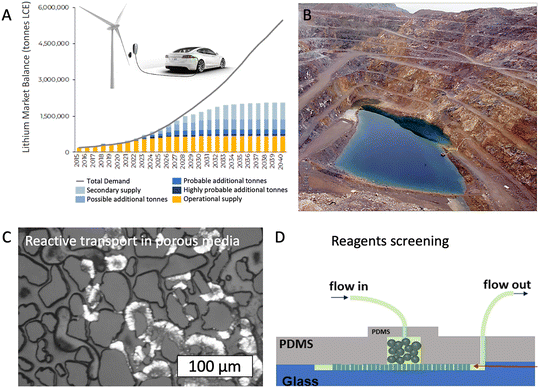 |
| Fig. 5 Opportunities for microfluidics in recovering critical mineral resources. (A) A significant demand gap is projected for mineral resources such as lithium over the next 20 years. (B) Geologic resources hosting the critical materials required. (C) Porous microfluidics (i.e., micromodels) present an opportunity to understand the reactive transport phenomena controlling minerals recovery from geologic and waste materials. (D) Microfluidic platforms provide an approach to screen reagents rapidly and economically to improve mineral recovery. Figures adapted from Benchmark Minerals Intelligence, New York Times, and ref. 112 and 161. | |
Second, new methods are needed to process and recycle existing end-of-life resources.163 Industrial wastes such as electronic devices and coal ash contain concentrated quantities of the metals that are mined. Currently, only ∼17% of total e-wastes are recycled, whereas a total of ∼54 Mt of e-wastes are generated annually. Circularity in the mineral economy will need improved methods for recovering metals out of electronic devices, many that are designed to be miniature in size. Here, microfluidics offers an opportunity to understand the reactive transport and reagent chemistries required to recover these metals in an environmentally benign manner. For example, geochemical microfluidics12,26,112,164–166 enable the visual probing of reactive transport interactions between reagents and metals in porous end-of-life materials to understand and design methods of recycling metals from industrial wastes.
VI. Ensuring water security
Water and energy are scarce and valuable resources that are closely linked:167 cool and clean water is used to produce energy (e.g., cooling or hydropower production for electric power, hydraulic fracturing for oil and gas), which generates hot, contaminated water that requires additional energy-intensive purification and treatment processes (e.g., wastewater treatment, desalination). Thus, moving toward a low-carbon future also requires advances in approaches to ensuring water security. Indeed, access to clean water is already an issue faced by billions of people globally today, and unfortunately, while technologies already exist to make use of subsurface and surface water sources (aquifers, lakes, rivers, the ocean), they often have high energy demands. Thus, development of ways to remediate dirty water sources is inherently part of reaching a low-carbon future. Toward this goal, the circular economy approach in Fig. 6 outlines how reuse (of waste) and repair (of contaminated resources) will become increasingly critical—necessitating improved design and optimization of the underlying processes. LoC approaches provide a way to do so, as we describe below by highlighting two key examples: remediation of contaminated sites using nanoparticles or beneficial microbes (Fig. 6E and F), and water desalination (Fig. 6B, G and I), in addition to traditional uses of LoC systems for contaminant detection, which continue to be critically important.168,169
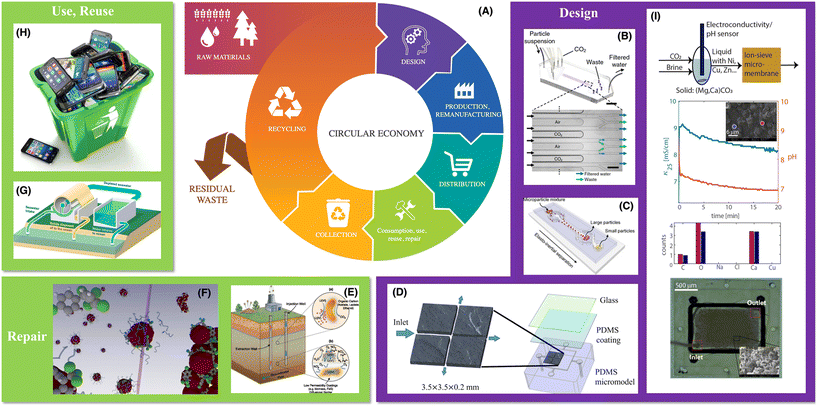 |
| Fig. 6 Opportunities for LoC approaches in ensuring water security. (A) A description of material use, reuse, repair, and design in a circular economy,170 along with the role of LoC. (B–D) Examples of microfluidic devices used to assess the efficacy of (B) membraneless water filtration using dissolved CO2;171 (C) membraneless water filtration using the elasto-inertial effect in a non-Newtonian fluid;172 (D) multiscale dissolution dynamics in natural rocks.54 (E) Schematic of bioremediation of contaminated water sources.173 (F) Schematic of nanoparticles used to remediate contaminated water.174 (G) Schematic of a seawater desalination process.175 (H) Photograph (from Bet_Noire/Getty Images/iStockphoto) showing materials to be recycled. (I) A microfluidic approach to studying brine desalination combined with CO2 mineralization; top to bottom show the reactor scheme, CaCO3 precipitation in the presence of copper (inset SEM image shows produced CaCO3), EDS analysis of the produced CaCO3, and 3D printed HMO-coated micro-membrane, respectively. | |
Groundwater remediation.
More than half of the world's drinking and agriculture water supply comes from porous groundwater aquifers. However, these sources are frequently susceptible to contamination from industrial processes. In particular, non-aqueous phase liquid (NAPL) and poorly-soluble contaminants, such as perchloroethylene and trichloroethylene, persist in groundwater aquifers years after their initial introduction—in many cases taking several decades to completely dissolve—leading to negative environmental, ecological, and health consequences. For example, the environmental and ecological consequences of contamination by organic compounds like these includes enhanced morbidity of fish and local plants, ecosystem dysfunction, and reduced biodiversity. Moreover, human use of contaminated water is directly linked with diseases such as hepatitis, dysentery, and cancer. Remediating contaminated groundwater aquifers is, therefore, critical to protecting water security. While “pump-and-treat” approaches have been used for decades, these are energy- and time-intensive, requiring continual removal of contaminated water from the source and costly annual upkeep. As a result, many groundwater sources remain contaminated for extended periods of time. Time and cost efficient in situ methods of water remediation are increasingly being sought to replace outdated pump-and-treat remedies, which are energy and time intensive, requiring complete removal of contaminated water from an aquifer and costly annual upkeep. LoC approaches provide a useful way to assess alternative approaches to groundwater remediation.
One such approach is using subsurface injection of chemically active nanoparticles, which have a large reactive surface area and are theorized to be able to travel long distances and pervade the small pores that are frequently occupied by contaminants within an aquifer. However, two challenges currently limit widespread adoption of catalytic nanomaterials for groundwater remediation. First, in many cases, aqueous suspensions of these particles aggregate quickly, reducing their overall chemical reactivity and ability to travel long enough distances to reach contaminants in an aquifer. Second, these particles do not specifically localize at trapped NAPL interfaces, reducing their remediation efficacy and requiring the addition of large amounts of nanoparticles and repeated injection at multiple sites, since contaminants can be difficult to locate. While core flood-type experiments have been instrumental in helping to shed light on these limitations, and provide a useful way to assess ways to overcome them,176 such approaches are often limited in their ability to probe pore-scale transport in opaque 3D porous media. Microfluidic technologies can play a useful role in shedding light. For example, micromodels can be used to develop a deeper understanding of the processes controlling how injected nanomaterials spread through a porous medium177 and how they interact with a trapped non-aqueous phase.178 Microfluidics with functionalized surfaces particularly can help to improve the accuracy of the testing by mimicking the mineralogy of natural soil.179 Future studies using such approaches to screen different classes of nanomaterials for in situ groundwater remediation will be useful.
Another way to improving the delivery of reactive nanoparticles to contaminated subsurface sites is using foam as a carrier. Wet foam (or simply foam) is a complex fluid consisting of gas bubbles dispersed in a liquid-phase. It is often referred to waterless fluid because the volume fraction of the gas-phase can be up to 97 vol%. Foam is injected into the subsurface to improve the gas mobility control as it increases the effective gas viscosity and allows to divert the gas towards low permeability zones.180 It has been successfully employed in gas-injection operations such as enhanced oil recovery (EOR)181–184 and suggested as an alternative option to continuous gas injection in geological CO2 sequestration78,185–190 (see I), hydraulic fracturing for geothermal energy,191 and shale-gas extraction.192–194 Furthermore, foam has been proposed for the remediation of contaminated sites as an effective way to: deliver amendments,195–199 mobilize non-aqueous phase liquid contaminants,200 and confine a source zone of contaminants in groundwater.201 In addition, since foam stability is significantly enhanced when surfactant and nanoparticles are added and nanoparticles adsorb irreversibly at gas–liquid interface, foam has been also considered as a carrier of nanoparticles towards selected targets in the subsurface.202–204
However, for the description and the design of a remediation technique based on foam and nanoparticle flooding a model is required. Current models consider only foam stabilized with surfactant focusing on the description of foam texture and water/gas saturation and yet missing the description of nanoparticle migration.205–212 To address this issue, it is necessary to gain an insight into physical and chemical processes such as surfactant and nanoparticle adsorption/desorption at the gas–liquid interface, nanoparticle interactions with a reactive porous medium in the presence of bubbles, and diversion of foam into low permeability zone in the presence of nanoparticles. To gain these insights, experiments where foam and nanoparticle transport dynamics can be observed at the pore scale should be performed. Microfluidic systems would provide valuable laboratory tools for direct observation of physical phenomena as well as more efficient and cost-effective execution than traditional column-flood tests. However, deep learning algorithms for image processing would be required to convert visual observations into quantitative information.213
Yet another way of remediating contaminated groundwater aquifers by manipulating chemical reactions in situ is by using bacteria that move through the pore space towards trapped contaminant and metabolize it. While such bioremediation approaches have shown promise in groundwater remediation, their efficacy and widespread use has been limited by a poor understanding of how to effectively target trapped contaminants. Addressing this challenge requires fundamental studies of bacterial transport in disordered porous media. It is well known that diverse strains of bacteria can direct their swimming motion towards and metabolize trapped partly-soluble contaminants in groundwater aquifers. This process of bioremediation has been explored for several decades due to its immense promise for rapid aquifer remediation.214 However, widespread application of bioremediation has been hindered by several gaps in knowledge. Specifically, it is unknown how the time taken for bacteria, either naturally present in an aquifer or exogenously introduced, to move toward and degrade trapped contaminant depends on (i) the geometry of the pore space, (ii) the amount and spatial distribution of contaminant, (iii) the amount and spatial distribution of bacteria, (iv) the ability of the bacteria to sense and metabolize the contaminant, and (v) the ambient fluid flow. As a result, bioremediation strategies often proceed on a trial-and-error basis and, in many cases, the kinetics of aquifer remediation are too slow to yield appreciable improvement in water quality. Just as with the other examples noted in previous subsections of how LoC approaches can help shed light on microbial processes (e.g., in influencing H2 storage, for bioenergy), micromodel studies of bacteria sensing and remediation of contaminants in situ147,215–217 will be important to overcome this issue.
Desalination and its products.
Due to the scarcity of potable water resources, water remediation and desalination are becoming more and more critical for the supply of clean water to the world population.218 In continental semiarid and arid regions, subsurface brine is an attractive alternative to surface water and groundwater not only for drinking water use as well as for applications to agricultural fields.219 However, subsurface brine requires complex treatments that often imply significant energy-intensive chemical processes.220 Therefore, microfluidic approaches play a critical role in assessing new low-carbon ways of desalinating water (e.g., Fig. 6I).171,221,222
Desalination processes conventionally employ membrane reactors because of their high selectively and separation performance without requiring chemicals and large reactor volume, unlike large-scale settling tanks and filtration columns. Thus, LoC systems are increasingly being used to study membrane processes;223 in this context, they are of growing interest because they provides a platform for testing new materials to reduce fouling on membrane surfaces and increase permeate flux across membranes.224
LoC approaches also yield a powerful way to examine how desalination products may be useful in sustainable processes. For example, the combination of water desalination with carbon storage through ex situ CO2 mineralization15 has been proposed to mitigate the impact of the technology and increase its sustainability.225–227 Indeed, the waste brine generated through desalination is a potential source for CO2 mineralization, because in brine the concentration of divalent cations (i.e., Ca2+ and Mg2+) necessary for binding CO2 into stable minerals, is almost twice that of ordinary seawater. For instance, the process proposed by Oh et al. (2018)225 can achieve up to 230 tonne CO2 reduction per year in addition to an economical benefit of water desalination. However, the precipitation kinetics of carbonate minerals changes with brine composition and an intense experimental activity is required to determine the rates. This type of investigation involves the use of comprehensive batch and continuous flow-through reactors and extensive time. Therefore, it would be envisaged to use microfluidic systems which require smaller amounts of chemicals and allow faster execution of tests. Monitoring tools including microscope and high-speed camera coupled with X-ray diffraction (XRD), Fourier transform infrared (FTIR) and Raman spectroscopy, which are generally employed in CO2 mineralization experiments off-line characterization, could be implemented for online monitoring.
Microfluidic systems have been used recently to study carbonate precipitation228,229 and they have shown a significant advantage over conventional experimental approaches by providing highly reproducible control over crystal polymorph, size, and shape. Therefore, future research should focus on developing frameworks for experimental design and data processing to determine the kinetics of CO2 mineralization using desalination brine of various chemical compositions; one such approach is shown in Fig. 6I. Indeed, conventional experimental approaches typically involve the use of large reactors and solution volumes, as well as costly monitoring tools for online and offline characterization. LoC could be uniquely helpful in miniaturizing such experiments, thereby reducing the amount of infrastructure and resources required and shorting amount of time needed to perform tests. Due to their versatility and ease of use, LoC systems could also be useful in investigating multiple systems (e.g., with different chemistries), especially when integrated with other spectroscopy, microscopy, and XRD tools.
Conclusion
Sustainability is at the forefront of decision making in industry and governments worldwide. Although the time and length scales of LoCs are typically much smaller than conventional processes, the application and adaptation of LoCs to enable a low carbon future presents a tremendous opportunity. In many of the examples described above, LoCs provide new understanding and insights in the fundamental transport, thermodynamic, and kinetics governing processes involving sustainable energy. Combined with controlled surface chemistry and the ability to conduct high-throughput screening, microfluidics offers a path to quickly evaluate new methods and processes.
While LoC systems provide tremendous opportunities, they also have limitations. Many energy systems involving geological media are intrinsically spatially heterogeneous and characterized by multiple physicochemical processes across a broad range of length scales. LoC systems cannot capture this full complexity. How can we improve our LoC systems to be more representative of the larger-scale systems they are intended to represent? How do we build quantitative correlations/maps between what we observe and understand at the sub-mm scale using LoC systems and what we predict at larger scales? What scale of heterogeneity should/could a microfluidic chip represent? Can we be more deliberate in designing experiments such that results can be more readily translated across scales—for example, using hierarchies of LoC systems at different scales?166 Addressing these questions is an important ongoing challenge for this field.
The many areas outlined above present a balance of precedence for LoC technologies, and new challenges. The transition period ahead, leading to 2050, corresponds to the professional lifetime of current graduate students and postdoctoral fellows. These decades present the opportunity to leverage the world's smallest fluid systems to address the world's largest fluids challenge and achieve a low-carbon future.
Conflicts of interest
There are no conflicts to declare.
Acknowledgements
SSD acknowledges funding in part from NSF grants CBET-1941716 and DMR-2011750, the Eric and Wendy Schmidt Transformative Technology Fund at Princeton, the Donors of the American Chemical Society Petroleum Research Fund for partial support of this research through grant PRF 59026-DNI9, and the New Jersey Water Resources Research Institute (NJWRRI) junior faculty grant program. This material is also based upon work by SSD supported by the U.S. Department of Energy's Office of Energy Efficiency and Renewable Energy (EERE) under the Geothermal Technologies Office (GTO) INNOVATIVE METHODS TO CONTROL HYDRAULIC PROPERTIES OF ENHANCED GEOTHERMAL SYSTEMS Award Number DE-EE0009790. IB acknowledges funding from the Center for Mechanistic Control of Water-Hydrocarbon-Rock Interactions in Unconventional and Tight Oil Formations (CMC-UF), an Energy Frontier Research Center funded by the U.S. Department of Energy, Office of Science under DOE (BES) Award DE-SC0019165. MF was funded in part by the Norwegian Research Council under project 325457 – HyPE. RJ acknowledges funding from the U.S. Department of Energy (Grant No. DE-SC0018357). SP acknowledges funding from the Donors of the American Chemical Society Petroleum Research Fund for partial support of this research through grant PRF 62566-DNI9. VP would like to thank the American Chemical Society Petroleum Research Fund (ACS-PRF) under the grant number PRF# 57739-DNI9. WS acknowledges funding in part from DOE grants EE0009440 and FE0031791, from the Donors of the American Chemical Society Petroleum Research Fund for partial support of this research through grant PRF 61218-DNI9, and the University of Texas at Austin Energy Institute. DS acknowledges support of the Natural Sciences and Engineering Research Council of Canada (NSERC), and the Canada Research Chairs (CRC) Program.
References
- Climate Change 2021, The Physical Science Basis; IPCC AR6 WGI, 2021.
- Net Zero by 2050 – Analysis – IEA, https://www.iea.org/reports/net-zero-by-2050, (accessed January 5, 2022).
- E. Kjeang, N. Djilali and D. Sinton, J. Power Sources, 2009, 186, 353–369 CrossRef CAS.
- C.-T. Dinh, T. Burdyny, M. G. Kibria, A. Seifitokaldani, C. M. Gabardo, F. P. G. de Arquer, A. Kiani, J. P. Edwards, P. D. Luna, O. S. Bushuyev, C. Zou, R. Quintero-Bermudez, Y. Pang, D. Sinton and E. H. Sargent, Science, 2018, 360, 783–787 CrossRef CAS PubMed.
- S. M. H. Hashemi, M. A. Modestino and D. Psaltis, Energy Environ. Sci., 2015, 8, 2003–2009 RSC.
- A. Majumdar and J. Deutch, Joule, 2018, 2, 805–809 CrossRef.
- L. Joppa, A. Luers, E. Willmott, S. J. Friedmann, S. P. Hamburg and R. Broze, Nature, 2021, 597, 629–632 CrossRef CAS.
- F. M. Orr Jr., SPE J., 2018, 23, 2444–2455 CrossRef.
- S. Krevor, H. de Coninck, S. Gasda, N. S. Ghaleigh, V. de Gooyert, H. Hajibeygi, R. Juanes, J. Neufeld, J. J. Roberts and F. Swennenhuis, Nat. Rev. Earth Environ., 2023 Search PubMed , accepted, in press.
- M. L. Szulczewski, C. W. MacMinn, H. J. Herzog and R. Juanes, Proc. Natl. Acad. Sci. U. S. A., 2012, 109, 5185–5189 CrossRef CAS PubMed.
- R. Lenormand, C. Zarcone and A. Sarr, J. Fluid Mech., 1983, 135, 337–353 CrossRef CAS.
- W. Song, T. W. De Haas, H. Fadaei and D. Sinton, Lab Chip, 2014, 14, 4382–4390 RSC.
- B. Zhao, C. W. MacMinn and R. Juanes, Proc. Natl. Acad. Sci. U. S. A., 2016, 113, 10251–10256 CrossRef CAS PubMed.
- B. Tohidi, R. Anderson, R. W. Burgass and A. B. Biderkab, Geology, 2001, 867 CrossRef CAS.
- Carbon Dioxide Capture and Storage — IPCC, https://www.ipcc.ch/report/carbon-dioxide-capture-and-storage/, (accessed January 6, 2022).
- R. Juanes, E. J. Spiteri, F. M. Orr and M. J. Blunt, Water Resour. Res., 2006, 42, 12418 CrossRef.
- H. E. Huppert and J. A. Neufeld, Annu. Rev. Fluid Mech., 2014, 46, 255–272 CrossRef.
- C. D. Tsakiroglou and A. C. Payatakes, Adv. Colloid Interface Sci., 1998, 75, 215–253 CrossRef CAS.
- M. I. J. van Dijke, K. S. Sorbie, M. Sohrabi, D. Tehrani and A. Danesh, All Days, 2002 DOI:10.2118/75192-MS.
- R. Lenormand, E. Touboul and C. Zarcone, J. Fluid Mech., 1988, 189, 165–187 CrossRef CAS.
- K. Xu, T. Liang, P. Zhu, P. Qi, J. Lu, C. Huh and M. Balhoff, Lab Chip, 2017, 17, 640–646 RSC.
- A. Anbari, H.-T. Chien, S. S. Datta, W. Deng, D. A. Weitz, J. Fan, A. Anbari, H. Chien, J. Fan, S. S. Datta, W. Deng and D. A. Weitz, Small, 2018, 14, 1703575 CrossRef PubMed.
- M. Stöhr, K. Roth and B. Jähne, Exp. Fluids, 2003, 35, 159–166 CrossRef.
- P. Sharma, P. Aswathi, A. Sane, S. Ghosh and S. Bhattacharya, Rev. Sci. Instrum., 2011, 82, 113704 CrossRef PubMed.
- R. F. Neumann, M. Barsi-Andreeta, E. Lucas-Oliveira, H. Barbalho, W. A. Trevizan, T. J. Bonagamba and M. B. Steiner, Sci. Rep., 2021, 11, 11370 CrossRef CAS PubMed.
- M. L. Porter, J. Jiménez-Martínez, R. Martinez, Q. McCulloch, J. W. Carey and H. S. Viswanathan, Lab Chip, 2015, 15, 4044–4053 RSC.
- Y. Q. Zhang, A. Sanati-Nezhad and S. H. Hejazi, Lab Chip, 2018, 18, 285–295 RSC.
- A. Ferrari, J. Jimenez-Martinez, T. L. Borgne, Y. Méheust and I. Lunati, Water Resour. Res., 2015, 51, 1381–1400 CrossRef.
- B. Ling, J. Bao, M. Oostrom, I. Battiato and A. M. Tartakovsky, Adv. Water Resour., 2017, 105, 29–38 CrossRef CAS.
- D. N. Espinoza and J. C. Santamarina, Water Resour. Res., 2010, 46, 7537 CrossRef.
- S. Iglauer, Acc. Chem. Res., 2017, 50, 1134–1142 CrossRef CAS PubMed.
- T.-H. M. Ho, J. Yang and P. A. Tsai, Lab Chip, 2021, 21, 3942–3951 RSC.
- M. Rücker, W.-B. Bartels, K. Singh, N. Brussee, A. Coorn, H. A. van der Linde, A. Bonnin, H. Ott, S. M. Hassanizadeh, M. J. Blunt, H. Mahani, A. Georgiadis and S. Berg, Geophys. Res. Lett., 2019, 46, 3225–3234 CrossRef.
- D. Bartolo, G. Degré, P. Nghe and V. Studer, Lab Chip, 2008, 8, 274–279 RSC.
- B. Levaché, A. Azioune, M. Bourrel, V. Studer and D. Bartolo, Lab Chip, 2012, 12, 3028–3031 RSC.
- C. Odier, B. Levaché, E. Santanach-Carreras and D. Bartolo, Phys. Rev. Lett., 2017, 119, 208005 CrossRef PubMed.
- T. Lan, R. Hu, Z. Yang, D.-S. Wu and Y.-F. Chen, Geophys. Res. Lett., 2020, 47, e2020GL089682 CrossRef.
- B. Zhao, C. W. MacMinn, B. K. Primkulov, Y. Chen, A. J. Valocchi, J. Zhao, Q. Kang, K. Bruning, J. E. McClure, C. T. Miller, A. Fakhari, D. Bolster, T. Hiller, M. Brinkmann, L. Cueto-Felgueroso, D. A. Cogswell, R. Verma, M. Prodanović, J. Maes, S. Geiger, M. Vassvik, A. Hansen, E. Segre, R. Holtzman, Z. Yang, C. Yuan, B. Chareyre and R. Juanes, Proc. Natl. Acad. Sci. U. S. A., 2019, 116, 13799–13806 CrossRef CAS PubMed.
- B. K. Primkulov, A. A. Pahlavan, X. Fu, B. Zhao, C. W. MacMinn and R. Juanes, J. Fluid Mech., 2021, 923, 34 CrossRef.
- C. Zhang, M. Oostrom, J. W. Grate, T. W. Wietsma and M. G. Warner, Environ. Sci. Technol., 2011, 45, 7581–7588 CrossRef CAS PubMed.
- S. Morais, N. Liu, A. Diouf, D. Bernard, C. Lecoutre, Y. Garrabos and S. Marre, Lab Chip, 2016, 16, 3493–3502 RSC.
- F. Kazemifar, G. Blois, D. C. Kyritsis and K. T. Christensen, Adv. Water Resour., 2016, 95, 352–368 CrossRef CAS.
- L. Zuo, C. Zhang, R. W. Falta and S. M. Benson, Adv. Water Resour., 2013, 53, 188–197 CrossRef CAS.
- C. Chang, Q. Zhou, T. J. Kneafsey, M. Oostrom, T. W. Wietsma and Q. Yu, Adv. Water Resour., 2016, 92, 142–158 CrossRef CAS.
- B. Pinho, S. Girardon, F. Bazer-Bachi, G. Bergeot, S. Marre and C. Aymonier, Lab Chip, 2014, 14, 3843–3849 RSC.
- H. Yoon, K. N. Chojnicki and M. J. Martinez, Environ. Sci. Technol., 2019, 53, 14233–14242 CrossRef CAS PubMed.
- P. N. Perera, H. Deng, P. J. Schuck and B. Gilbert, J. Phys. Chem. B, 2018, 122, 4566–4572 CrossRef CAS PubMed.
- J. Xu and M. T. Balhoff, Adv. Water Resour., 2022, 164, 104200 CrossRef CAS.
- C. Zhang, K. Dehoff, N. Hess, M. Oostrom, T. W. Wietsma, A. J. Valocchi, B. W. Fouke and C. J. Werth, Environ. Sci. Technol., 2010, 44, 7833–7838 CrossRef CAS PubMed.
- H. Yoon, A. J. Valocchi, C. J. Werth and T. Dewers, Water Resour. Res., 2012, 48(2) DOI:10.1029/2011WR011192.
- M. Nooraiepour, H. Fazeli, R. Miri and H. Hellevang, Environ. Sci. Technol., 2018, 52, 6050–6060 CrossRef CAS PubMed.
- H. Fazeli, M. Nooraiepour and H. Hellevang, Ind. Eng. Chem. Res., 2020, 59, 450–457 CrossRef CAS.
- Y. Zhang, G. Yesiloz, H. J. Sharahi, H. Khorshidian, S. Kim, A. Sanati-Nezhad and S. H. Hejazi, Adv. Mater. Interfaces, 2019, 6, 1900995 CrossRef CAS.
- B. Ling, M. Sodwatana, A. Kohli, C. M. Ross, A. Jew, A. R. Kovscek and I. Battiato, Proc. Natl. Acad. Sci. U. S. A., 2022, 119, e2122520119 CrossRef CAS PubMed.
- J. Jiménez-Martínez, J. D. Hyman, Y. Chen, J. W. Carey, M. L. Porter, Q. Kang, G. Guthrie Jr. and H. S. Viswanathan, Geophys. Res. Lett., 2020, 47, e2020GL087163 CrossRef.
- C. Soulaine, S. Roman, A. Kovscek and H. A. Tchelepi, J. Fluid Mech., 2017, 827, 457–483 CrossRef CAS.
- C. Soulaine, S. Roman, A. Kovscek and H. A. Tchelepi, J. Fluid Mech., 2018, 855, 616–645 CrossRef CAS.
- N. K. Karadimitriou and S. M. Hassanizadeh, Vadose Zone J., 2012, 11(3) DOI:10.2136/vzj2011.0072.
- N. S. K. Gunda, B. Bera, N. K. Karadimitriou, S. K. Mitra and S. M. Hassanizadeh, Lab Chip, 2011, 11, 3785–3792 RSC.
- A. Gerami, Y. Alzahid, P. Mostaghimi, N. Kashaninejad, F. Kazemifar, T. Amirian, N. Mosavat, M. Ebrahimi Warkiani and R. T. Armstrong, Transp. Porous Media, 2019, 130, 277–304 CrossRef.
- A. R. Kovscek, H. Wong and C. J. Radke, AIChE J., 1993, 39, 1072–1085 CrossRef CAS.
- T. Hiller, J. Ardevol-Murison, A. Muggeridge, M. Schröter and M. Brinkmann, SPE J., 2018, 24, 200–214 CrossRef.
- A. Irannezhad, B. K. Primkulov, R. Juanes and B. Zhao, Phys. Rev. Fluids, 2023, 8, L012301 CrossRef.
- J. W. Barker and S. Thibeau, SPE Reservoir Eng., 1997, 12, 138–143 CrossRef CAS.
-
S. E. Gasda and M. A. Celia, in Developments in Water Science, ed. C. T. Miller, M. W. Farthing, W. G. Gray and G. F. Pinder, Elsevier, 2004, vol. 55, pp. 793–804 Search PubMed.
- N. Liu, C. Aymonier, C. Lecoutre, Y. Garrabos and S. Marre, Chem. Phys. Lett., 2012, 551, 139–143 CrossRef CAS.
- A. Schaap, D. Koopmans, M. Holtappels, M. Dewar, M. Arundell, S. Papadimitriou, R. Hanz, S. Monk, M. Mowlem and S. Loucaides, Int. J. Greenhouse Gas Control, 2021, 110, 103427 CrossRef CAS.
- G. Jian, C. A. Fernandez, M. Puerto, R. Sarathi, A. Bonneville and S. L. Biswal, J. Pet. Sci. Eng., 2021, 202, 108447 CrossRef CAS.
-
W. R. Rossen, R. Farajzadeh, G. J. Hirasaki and M. Amirmoshiri, OnePetro, 2022 Search PubMed.
- K. Ma, R. Liontas, C. A. Conn, G. J. Hirasaki and S. L. Biswal, Soft Matter, 2012, 8, 10669–10675 RSC.
- S. Xiao, Y. Zeng, E. D. Vavra, P. He, M. Puerto, G. J. Hirasaki and S. L. Biswal, Langmuir, 2018, 34, 739–749 CrossRef CAS PubMed.
- Y. Zeng, R. Farajzadeh, A. A. Eftekhari, S. Vincent-Bonnieu, A. Muthuswamy, W. R. Rossen, G. J. Hirasaki and S. L. Biswal, Langmuir, 2016, 32, 6239–6245 CrossRef CAS PubMed.
- R. Liontas, K. Ma, G. J. Hirasaki and S. L. Biswal, Soft Matter, 2013, 9, 10971–10984 RSC.
- E. Vavra, M. Puerto, C. Bai, K. Ma, K. Mateen, L. Biswal and G. Hirasaki, J. Colloid Interface Sci., 2022, 621, 321–330 CrossRef CAS PubMed.
- N. Yekeen, M. A. Manan, A. K. Idris, E. Padmanabhan, R. Junin, A. M. Samin, A. O. Gbadamosi and I. Oguamah, J. Pet. Sci. Eng., 2018, 164, 43–74 CrossRef CAS.
- V. Prigiobbe, A. J. Worthen, K. P. Johnston, C. Huh and S. L. Bryant, Transp. Porous Media, 2016, 1, 265–285 CrossRef.
- A. J. Worthen, H. G. Bagaria, Y. Chen, S. L. Bryant, C. Huh and K. P. Johnston, J. Colloid Interface Sci., 2013, 391, 142–151 CrossRef CAS PubMed.
- A. U. Rognmo, S. Heldal and M. A. Fernø, Fuel, 2018, 216, 621–626 CrossRef CAS.
- H. Hosseini, J. S. Tsau, K. Shafer-Peltier, C. Marshall, Q. Ye and R. B. Ghahfarokhi, Ind. Eng. Chem. Res., 2019, 58, 9431–9449 CrossRef CAS.
- T. Gothsch, C. Schilcher, C. Richter, S. Beinert, A. Dietzel, S. Büttgenbach and A. Kwade, Microfluid. Nanofluid., 2015, 18, 121–130 CrossRef CAS.
- M. Abolhasani, M. Singh, E. Kumacheva and A. Günther, Lab Chip, 2012, 12, 1611–1618 RSC.
- C. D. Ruppel and J. D. Kessler, Rev. Geophys., 2017, 55, 126–168 CrossRef.
- S. Almenningen, J. Flatlandsmo, A. R. Kovscek, G. Ersland and M. A. Fernø, Lab Chip, 2017, 17, 4070–4076 RSC.
- L. P. Hauge, J. Gauteplass, M. D. Høyland, G. Ersland, A. Kovscek and M. A. Fernø, Int. J. Greenhouse Gas Control, 2016, 53, 178–186 CrossRef CAS.
- A. Touil, D. Broseta and A. Desmedt, Langmuir, 2019, 35, 12569–12581 CrossRef CAS PubMed.
- X. Fu, L. Cueto-Felgueroso and R. Juanes, Phys. Rev. Lett., 2018, 120, 144501 CrossRef CAS PubMed.
- X. Fu, J. Jimenez-Martinez, T. P. Nguyen, J. William Carey, H. Viswanathan, L. Cueto-Felgueroso and R. Juanes, Proc. Natl. Acad. Sci. U. S. A., 2020, 117, 31660–31664 CrossRef CAS PubMed.
- B. Kvamme, Can. J. Chem., 2015, 93, 897–905 CrossRef CAS.
- M. L. Martinez de Baños, O. Carrier, P. Bouriat and D. Broseta, Chem. Eng. Sci., 2015, 123, 564–572 CrossRef.
- D. Atig, A. Touil, M. Ildefonso, L. Marlin, P. Bouriat and D. Broseta, Chem. Eng. Sci., 2018, 192, 1189–1197 CrossRef CAS.
- W. Chen, B. Pinho and R. L. Hartman, Lab Chip, 2017, 17, 3051–3060 RSC.
- W. Chen and R. L. Hartman, Energy Fuels, 2018, 32, 11761–11771 CrossRef CAS.
- J. D. Wells, W. Chen, R. L. Hartman and C. A. Koh, J. Chem. Phys., 2021, 154, 114710 CrossRef CAS PubMed.
- R. Tarkowski, Renewable Sustainable Energy Rev., 2019, 105, 86–94 CrossRef CAS.
- A. E. Yekta, J. C. Manceau, S. Gaboreau, M. Pichavant and P. Audigane, Transp. Porous Media, 2018, 122, 333–356 CrossRef CAS.
- M. Boon and H. Hajibeygi, Sci. Rep., 2022, 12, 14604 CrossRef CAS PubMed.
- M. Lysyy, G. Ersland and M. Fernø, Adv. Water Resour., 2022, 163, 104167 CrossRef CAS.
- P. Gabrielli, A. Poluzzi, G. J. Kramer, C. Spiers, M. Mazzotti and M. Gazzani, Renewable Sustainable Energy Rev., 2020, 121, 109629 CrossRef CAS.
- M. A. Hesse, F. M. Orr and H. A. Tchelepi, J. Fluid Mech., 2008, 611, 35–60 CrossRef CAS.
- S. H. Barghi, T. T. Tsotsis and M. Sahimi, Int. J. Hydrogen Energy, 2014, 39, 1390–1397 CrossRef CAS.
- A. Al-Yaseri and N. K. Jha, J. Pet. Sci. Eng., 2021, 200, 108387 CrossRef CAS.
- S. Iglauer, M. Ali and A. Keshavarz, Geophys. Res. Lett., 2021, 48, e2020GL090814 CAS.
- L. Hashemi, W. Glerum, R. Farajzadeh and H. Hajibeygi, Adv. Water Resour., 2021, 154, 103964 CrossRef CAS.
- M. Buchgraber, A. R. Kovscek and L. M. Castanier, Transp. Porous Media, 2012, 95, 647–668 CrossRef CAS.
- S. C. Cao, S. Dai and J. Jung, Int. J. Greenhouse Gas Control, 2016, 44, 104–114 CrossRef CAS.
- C. Chang, Q. Zhou, M. Oostrom, T. J. Kneafsey and H. Mehta, Adv. Water Resour., 2017, 100, 14–25 CrossRef CAS.
- O. Bochet, L. Bethencourt, A. Dufresne, J. Farasin, M. Pédrot, T. Labasque, E. Chatton, N. Lavenant, C. Petton, B. W. Abbott, L. Aquilina and T. Le Borgne, Nat. Geosci., 2020, 13, 149–155 CrossRef CAS.
- B. Ling and I. Battiato, Micromachines, 2022, 13, 774 CrossRef PubMed.
- V. Reitenbach, L. Ganzer, D. Albrecht and B. Hagemann, Environ. Earth Sci., 2015, 73, 6927–6937 CrossRef CAS.
- S. P. Gregory, M. J. Barnett, L. P. Field and A. E. Milodowski, Microorganisms, 2019, 7, 53 CrossRef CAS PubMed.
- L. Pellizzari, T. Lienen, M. Kasina and H. Würdemann, Environ. Earth Sci., 2017, 76, 1–19 CrossRef CAS.
- W. Song, F. Ogunbanwo, M. Steinsbø, M. A. Fernø and A. R. Kovscek, Lab Chip, 2018, 18, 3881–3891 RSC.
- R. L. Tyne, P. H. Barry, M. Lawson, D. J. Byrne, O. Warr, H. Xie, D. J. Hillegonds, M. Formolo, Z. M. Summers, B. Skinner, J. M. Eiler and C. J. Ballentine, Nature, 2021, 600, 670–674 CrossRef CAS PubMed.
- E. W. J. Van Niel, P. A. M. Claassen and A. J. M. Stams, Biotechnol. Bioeng., 2003, 81, 255–262 CrossRef CAS PubMed.
- N. Hassannayebi, B. Jammernegg, J. Schritter, P. Arnold, F. Enzmann, M. Kersten, A. P. Loibner, M. Fernø and H. Ott, Transp. Porous Media, 2021, 139, 579–593 CrossRef.
- Combining Fluidic Devices With Microscopy And Flow Cytometry To Study Microbial Transport In Porous Media Across Spatial Scales – Video, https://www.jove.com/t/60701/combining-fluidic-devices-with-microscopy-flow-cytometry-to-study, (accessed December 22, 2022).
- P. de Anna, A. A. Pahlavan, Y. Yawata, R. Stocker and R. Juanes, Nat. Phys., 2021, 17, 68–73 Search PubMed.
- G. Ceriotti, S. M. Borisov, J. S. Berg and P. de Anna, Environ. Sci. Technol., 2022, 56, 17471–17480 CrossRef CAS PubMed.
- E. Jolie, S. Scott, J. Faulds, I. Chambefort, G. Axelsson, L. C. Gutiérrez-Negrín, S. Regenspurg, M. Ziegler, B. Ayling, A. Richter and M. T. Zemedkun, Nat. Rev. Earth Environ., 2021, 2, 324–339 CrossRef.
- Department of Energy (DOE) Office of Energy Efficiency and Renewable Energy (EERE) Innovative Methods to Control Hydraulic Properties of Enhanced Geothermal Systems Funding Opportunity Announcement (FOA) Number: DE-FOA-0002498, https://eere-exchange.energy.gov/FileContent.aspx?FileID=3062faca-bd37-4795-a1d0-7a287d10406c, (accessed January 6, 2022).
- V. Soni, S. Saber, H. McPhee, J. Riordon, M. Zargartalebi, M. Holmes, M. Toews and D. Sinton, Energy Fuels, 2021, 35, 10293–10302 CrossRef CAS.
- C. A. Conn, K. Ma, G. J. Hirasaki and S. L. Biswal, Lab Chip, 2014, 14, 3968–3977 RSC.
- T. W. De Haas, H. Fadaei, U. Guerrero and D. Sinton, Lab Chip, 2013, 13, 3832–3839 RSC.
- Y. Zhang, C. Zhou, C. Qu, M. Wei, X. He and B. Bai, Lab Chip, 2019, 19, 4071–4082 RSC.
- B. Bai, Y. Liu, J.-P. Coste and L. Li, SPE Reservoir Eval. Eng., 10(2), 176–184 CrossRef CAS.
- C. A. Browne and S. S. Datta, Sci. Adv., 2021, 7, 2619 CrossRef PubMed.
- C. A. Browne, A. Shih and S. S. Datta, Small, 2020, 16, 1903944 CrossRef CAS PubMed.
- J. Zhong, V. Soni, S. Saber, J. Riordon, B. Schwarz, M. Toews and D. Sinton, Energy Fuels, 2020, 34, 11219–11226 CrossRef CAS.
- K. Lee, S. E. Kim, J. Doh, K. Kim and W. K. Chung, Lab Chip, 2021, 21, 1798–1810 RSC.
- H. Cai, Z. Ao, Z. Wu, S. Song, K. Mackie and F. Guo, Lab Chip, 2021, 21, 2194–2205 RSC.
- K. M. Graczyk and M. Matyka, Sci. Rep., 2020, 10, 1–11 CrossRef PubMed.
- Using Machine Learning to Predict Future Temperature Outputs in Geothermal Systems (Conference)|OSTI.GOV, https://www.osti.gov/biblio/1710149, (accessed January 6, 2022).
- Energy Department Awards $5.5 Million to Apply Machine Learning to Geothermal Exploration|Department of Energy, https://www.energy.gov/eere/articles/energy-department-awards-55-million-apply-machine-learning-geothermal-exploration, (accessed January 6, 2022).
- V. A. Lifton, Lab Chip, 2016, 16, 1777–1796 RSC.
- B. Ling, M. Oostrom, A. M. Tartakovsky and I. Battiato, Phys. Fluids, 2018, 30, 076601 CrossRef.
- Total Energy Outlook 2020.
- Bp, Energy Outlook 2020 edition.
- M. S. Masnadi, H. M. El-Houjeiri, D. Schunack, Y. Li, J. G. Englander, A. Badahdah, J. C. Monfort, J. E. Anderson, T. J. Wallington, J. A. Bergerson, D. Gordon, J. Koomey, S. Przesmitzki, I. L. Azevedo, X. T. Bi, J. E. Duffy, G. A. Heath, G. A. Keoleian, C. McGlade, D. Nathan Meehan, S. Yeh, F. You, M. Wang and A. R. Brandt, Science, 2018, 361, 851–853 CrossRef CAS PubMed.
- R. Ostafe, R. Prodanovic, W. L. Ung, D. A. Weitz and R. Fischer, Biomicrofluidics, 2014, 8, 041102 CrossRef PubMed.
- K. Aleklett, E. T. Kiers, P. Ohlsson, T. S. Shimizu, V. E. Caldas and E. C. Hammer, ISME J., 2018, 12, 312–319 CrossRef PubMed.
- H. S. Kim, T. L. Weiss, H. R. Thapa, T. P. Devarenne and A. Han, Lab Chip, 2014, 14, 1415–1425 RSC.
- B. K. Hønsvall, D. Altin and L. J. Robertson, Bioresour. Technol., 2016, 200, 360–365 CrossRef PubMed.
- C. E. Stanley and M. G. A. van der Heijden, Trends Microbiol., 2017, 25, 610–613 CrossRef CAS PubMed.
- J. Gao, J. Sasse, K. M. Lewald, K. Zhalnina, L. T. Cornmesser, T. A. Duncombe, Y. Yoshikuni, J. P. Vogel, M. K. Firestone and T. R. Northen, J. Visualized Exp., 2018, 2018, 57170 Search PubMed.
- L. Ma, Y. Shi, O. Siemianowski, B. Yuan, T. K. Egner, S. V. Mirnezami, K. R. Lind, B. Ganapathysubramanian, V. Venditti and L. Cademartiri, Proc. Natl. Acad. Sci. U. S. A., 2019, 166, 11063–11068 CrossRef PubMed.
- T. Bhattacharjee and S. S. Datta, Nat. Commun., 2019, 10, 1–9 CrossRef CAS PubMed.
- T. Bhattacharjee, D. B. Amchin, J. A. Ott, F. Kratz and S. S. Datta, Biophys. J., 2021, 120, 3483–3497 CrossRef CAS PubMed.
- M. Najah, R. Calbrix, I. P. Mahendra-Wijaya, T. Beneyton, A. D. Griffiths and A. Drevelle, Chem. Biol., 2014, 21, 1722–1732 CrossRef CAS PubMed.
- M. F. Devaux, F. Jamme, W. André, B. Bouchet, C. Alvarado, S. Durand, P. Robert, L. Saulnier, E. Bonnin and F. Guillon, Front. Plant Sci., 2018, 9, 200 CrossRef PubMed.
- P. J. Graham, J. Riordon and D. Sinton, Lab Chip, 2015, 15, 3116–3124 RSC.
- H. S. Kim, S. C. Hsu, S. I. Han, H. R. Thapa, A. R. Guzman, D. R. Browne, M. Tatli, T. P. Devarenne, D. B. Stern and A. Han, Plant Direct, 2017, 1, e00011 CrossRef PubMed.
- B. Ozdalgic, M. Ustun, S. R. Dabbagh, B. Z. Haznedaroglu, A. Kiraz and S. Tasoglu, Biotechnol. Bioeng., 2021, 118, 1716–1734 CrossRef PubMed.
- S. Mishra, Y. J. Liu, C. S. Chen and D. J. Yao, Energies, 2021, 14, 1817 CrossRef CAS.
- E. E. Jung, M. Kalontarov, D. F. R. Doud, M. D. Ooms, L. T. Angenent, D. Sinton and D. Erickson, Lab Chip, 2012, 12, 3740–3745 RSC.
- E. Alonso, A. M. Sherman, T. J. Wallington, M. P. Everson, F. R. Field, R. Roth and R. E. Kirchain, Environ. Sci. Technol., 2012, 46, 3406–3414 CrossRef CAS PubMed.
- Mineral Commodity Summaries 2020|U.S. Government Bookstore, https://bookstore.gpo.gov/products/mineral-commodity-summaries-2020, (accessed January 6, 2022).
- The Role of Critical Minerals in Clean Energy Transitions – Analysis – IEA, https://www.iea.org/reports/the-role-of-critical-minerals-in-clean-energy-transitions, (accessed January 6, 2022).
- U. Department of Energy.
- M. Azadi, S. A. Northey, S. H. Ali and M. Edraki, Nat. Geosci., 2020, 13, 100–104 CrossRef CAS.
- T. W. de Haas, B. Bao, H. Acosta Ramirez, A. Abedini and D. Sinton, Energy Fuels, 2021, 35, 7866–7873 CrossRef CAS.
- D. Yang, R. Fan, C. Greet and C. Priest, Environ. Sci. Technol., 2020, 54, 14000–14006 CrossRef CAS PubMed.
- T. N. Q. Le, Q. D. Tran, N. N. Tran, C. Priest, W. Skinner, M. Goodsite, C. Spandler, N. J. Cook and V. Hessel, Green Chem., 2022, 24, 8879–8898 RSC.
-
V. Forti, C. P. Balde, R. Kuehr and G. Bel, United Nations University (UNU)/United Nations Institute for Training and Research (UNITAR) – co-hosted SCYCLE Programme, International Telecommunication Union (ITU) & International Solid Waste Association (ISWA), Bonn/Geneva/Rotterdam, 2020, pp. 1–119.
- A. Gerami, P. Mostaghimi, R. T. Armstrong, A. Zamani and M. E. Warkiani, Int. J. Coal Geol., 2016, 159, 183–193 CrossRef CAS.
- A. L. Harrison, G. M. Dipple, W. Song, I. M. Power, K. U. Mayer, A. Beinlich and D. Sinton, Chem. Geol., 2017, 463, 1–11 CrossRef CAS.
- B. Ling, H. J. Khan, J. L. Druhan and I. Battiato, Energies, 2021, 14, 21 CrossRef.
-
E. Metzger, P. Reig, W. H. Wen, R. S. Young and B. Owens, Water-Energy Nexus: Business Risks and Rewards, 2016 Search PubMed.
- P. K. Rai, M. Islam and A. Gupta, Sens. Actuators, A, 2022, 347, 113926 CrossRef CAS.
- J. Saez, R. Catalan-Carrio, R. M. Owens, L. Basabe-Desmonts and F. Benito-Lopez, Anal. Chim. Acta, 2021, 1186, 338392 CrossRef CAS PubMed.
- Circular economy: the importance of re-using products and materials|News|European Parliament, https://www.europarl.europa.eu/news/en/headlines/economy/20150701STO72956/circular-economy-the-importance-of-re-using-products-and-materials, (accessed January 6, 2022).
- S. Shin, O. Shardt, P. B. Warren and H. A. Stone, Nat. Commun., 2017, 8, 1–6 CrossRef CAS PubMed.
- J. Nam, H. Lim, D. Kim, H. Jung and S. Shin, Lab Chip, 2012, 12, 1347–1354 RSC.
- K. H. Williams, J. R. Bargar, J. R. Lloyd and D. R. Lovley, Curr. Opin. Biotechnol., 2013, 24, 489–497 CrossRef CAS PubMed.
- New study shows how nanoparticles can clean up environmental pollutants|MIT News|Massachusetts Institute of Technology, https://news.mit.edu/2015/nanoparticles-clean-environmental-pollutants-0721, (accessed January 6, 2022).
- E. C. La Plante, D. A. Simonetti, J. Wang, A. Al-Turki, X. Chen, D. Jassby and G. N. Sant, ACS Sustainable Chem. Eng., 2021, 9, 1073–1089 CrossRef CAS.
- T. Pak, L. F. De Lima Luz, T. Tosco, G. S. R. Costa, P. R. R. Rosa and N. L. Archilha, Proc. Natl. Acad. Sci. U. S. A., 2020, 117, 13366–13373 CrossRef CAS PubMed.
- N. Bizmark, J. Schneider, R. D. Priestley and S. S. Datta, Sci. Adv., 2020, 6, 2530–2543 CrossRef PubMed.
- J. Schneider, R. D. Priestley and S. S. Datta, Phys. Rev. Fluids, 2021, 6, 014001 CrossRef.
- S. M. Seyedpour, M. Janmaleki, C. Henning, A. Sanati-Nezhad and T. Ricken, Sci. Total Environ., 2019, 686, 1272–1281 CrossRef CAS PubMed.
- R. F. Li, W. Yan, S. Liu, G. J. Hirasaki and C. A. Miller, SPE J., 2010, 15, 928–942 CrossRef.
- W. R. Rossen, Foams, 2017, 413–464 Search PubMed.
- T. C. Ransohoff and C. J. Radke, SPE Reservoir Eng., 1988, 3, 573–585 CrossRef CAS.
- G. J. Hirasaki, C. A. Miller, R. Szafranski, D. Tanzil, J. B. Lawson, H. Meinardus, M. Jin, J. T. Londergan, R. E. Jackson, G. A. Pope and W. H. Wade, SPE Annu. Tech. Conf. Exhib., 1997, SPE-39292-MS Search PubMed.
- R. Farajzadeh, A. Andrianov, R. Krastev, G. J. Hirasaki and W. R. Rossen, Adv. Colloid Interface Sci., 2012, 183–184, 1–13 CrossRef CAS PubMed.
- A. J. Worthen, P. S. Parikh, Y. Chen, S. L. Bryant, C. Huh and K. P. Johnston, Energy Procedia, 2014, 63, 7929–7938 CrossRef CAS.
- M. Lotfollahi, I. Kim, M. R. Beygi, A. J. Worthen, C. Huh, K. P. Johnston, M. F. Wheeler and D. A. Dicarlo, Transp. Porous Media, 2017, 116, 687–703 CrossRef CAS.
- F. Guo, S. A. Aryana, Y. Wang, J. F. McLaughlin and K. Coddington, Int. J. Greenhouse Gas Control, 2019, 87, 134–141 CrossRef CAS.
- T. Føyen, B. Brattekås, M. A. Fernø, A. Barrabino and T. Holt, Int. J. Greenhouse Gas Control, 2020, 96, 103016 CrossRef.
- Ø. Eide, M. Fernø, S. Bryant, A. Kovscek and J. Gauteplass, J. Nat. Gas Sci. Eng., 2020, 80, 103378 CrossRef.
- K. R. Chaturvedi and T. Sharma, Chem. Eng. Sci., 2021, 235, 116484 CrossRef CAS.
- W. A. Nugroho, S. Hermawan, B. H. Lazuardi and R. Mirza, SPE Asia Pac. Oil Gas Conf. Exhib., 2017, SPE-186922-MS Search PubMed.
- Q. Lv, Z. Li, B. Li, S. Li and Q. Sun, Ind. Eng. Chem. Res., 2015, 54, 9456–9477 CrossRef.
- W. A. M. Wanniarachchi, P. G. Ranjith, M. S. A. Perera, A. Lashin, N. Al Arifi and J. C. Li, Geomechanics and Geophysics for Geo-Energy and Geo-Resources, 2015, 1, 121–134 CrossRef.
- C. Xiao, S. N. Balasubramanian and L. W. Clapp, Ind. Eng. Chem. Res., 2017, 56, 8340–8348 CrossRef CAS.
- C. N. Mulligan and S. Wang, Eng. Geol., 2006, 85, 75–81 CrossRef.
- L. Zhong, N. P. Qafoku, J. E. Szecsody, P. E. Dresel and Z. F. Zhang, Vadose Zone J., 2009, 8, 976–985 CrossRef CAS.
- L. Zhong, J. E. Szecsody, F. Zhang and S. V. Mattigod, Vadose Zone J., 2010, 9, 757–767 CrossRef.
- J. Maire, A. Coyer and N. Fatin-Rouge, J. Hazard. Mater., 2015, 299, 630–638 CrossRef CAS PubMed.
- J. Maire, E. Brunol and N. Fatin-Rouge, Chemosphere, 2018, 197, 661–669 CrossRef CAS PubMed.
- S. Liao, Z. Saleeba, J. D. Bryant, L. M. Abriola and K. D. Pennell, Water Res., 2021, 195, 116975 CrossRef CAS PubMed.
- C. Portois, C. S. Boeije, H. J. Bertin and O. Atteia, Transp. Porous Media, 2018, 124, 787–801 CrossRef.
- J. Quinn, C. Geiger, C. Clausen, K. Brooks, C. Coon, S. O'Hara, T. Krug, D. Major, W. S. Yoon, A. Gavaskar and T. Holdsworth, Environ. Sci. Technol., 2005, 39, 1309–1318 CrossRef CAS PubMed.
- X. Shen, L. Zhao, Y. Ding, B. Liu, H. Zeng, L. Zhong and X. Li, J. Hazard. Mater., 2011, 186, 1773–1780 CrossRef CAS PubMed.
- Y. Ding, B. Liu, X. Shen, L. Zhong and X. Li, J. Environ. Eng., 2013, 139, 1206–1212 CrossRef CAS.
- Q. Li and V. Prigiobbe, Transp. Porous Media, 2020, 131, 269–288 CrossRef CAS.
- W. R. Rossen, J. Colloid
Interface Sci., 1990, 136, 1–16 CrossRef CAS.
- S. S. Mohammadi, D. A. Coombe and V. M. Stevenson, J. Can. Pet. Technol., 1993, 32, 49–54 CrossRef CAS.
- A. R. Kovscek, T. W. Patzek and C. J. Radke, Chem. Eng. Sci., 1995, 50, 3783–3799 CrossRef CAS.
- S. I. Kam and W. R. Rossen, SPE Annu. Tech. Conf. Exhib., 2002, 3137–3150 Search PubMed.
- M. Kun, G. Ren, K. Mateen, D. Morel and P. Cordelier, Proc. - SPE/DOE Symp. Improved Oil Recovery, 2014, 2, 1041–1065 Search PubMed.
- M. Lotfollahi, R. Farajzadeh, M. Delshad, A. Varavei and W. R. Rossen, J. Nat. Gas Sci. Eng., 2016, 31, 184–197 CrossRef CAS.
- Z. H. Zhou and W. R. Rossen, SPE Advanced Technology Series, 1995, 3, 154–162 CrossRef.
- Q. Li and V. Prigiobbe, Chem. Eng. Sci., 2020, 215, 115427 CrossRef CAS.
- R. B. Marx and M. D. Aitken, Environ. Sci. Technol., 2000, 34, 3379–3383 CrossRef CAS.
- X. Wang, T. Long and R. M. Ford, Biotechnol. Bioeng., 2012, 109, 1622–1628 CrossRef CAS PubMed.
- X. Wang, J. Atencia and R. M. Ford, Biotechnol. Bioeng., 2015, 112, 896–904 CrossRef CAS PubMed.
- X. Wang, L. M. Lanning and R. M. Ford, Environ. Sci. Technol., 2016, 50, 165–172 CrossRef CAS PubMed.
- P. Greve, T. Kahil, J. Mochizuki, T. Schinko, Y. Satoh, P. Burek, G. Fischer, S. Tramberend, R. Burtscher, S. Langan and Y. Wada, Nat. Sustain., 2018, 1, 486–494 CrossRef.
- E. Jones, M. Qadir, M. T. H. van Vliet, V. Smakhtin and S. M. Kang, Sci. Total Environ., 2019, 657, 1343–1356 CrossRef CAS PubMed.
- D. Xevgenos, K. Moustakas, D. Malamis and M. Loizidou, Desalin. Water Treat., 2014, 57, 2304–2314 CrossRef.
- B. D. MacDonald, M. M. Gong, P. Zhang and D. Sinton, Lab Chip, 2014, 14, 681–685 RSC.
- S. H. Roelofs, A. Van Den Berg and M. Odijk, Lab Chip, 2015, 15, 3428–3438 RSC.
- J. de Jong, R. G. H. Lammertink and M. Wessling, Lab Chip, 2006, 6, 1125–1139 RSC.
- H. S. Santana, J. L. Silva, B. Aghel and J. Ortega-Casanova, SN Appl. Sci., 2020, 2, 395 CrossRef.
- J. Oh, D. Jung, S. H. Oh, K. Roh, J. Chung, J. I. Han and J. H. Lee, IFAC-PapersOnLine, 2018, 51, 85–90 CrossRef.
- J. H. Bang, Y. Yoo, S. W. Lee, K. Song and S. Chae, Minerals, 2017, 7, 207 CrossRef.
- J. H. Bang, S. C. Chae, S. W. Lee, J. W. Kim, K. Song, J. Kim and W. Kim, J. CO2 Util., 2019, 33, 427–433 CrossRef CAS.
- A. Yashina, F. Meldrum and A. DeMello, Biomicrofluidics, 2012, 6, 022001 CrossRef PubMed.
- K. Y. Ko and I. H. Kim, Biotechnol. Bioprocess Eng., 2016, 21, 453–462 CrossRef CAS.
|
This journal is © The Royal Society of Chemistry 2023 |