DOI:
10.1039/D3FO02111D
(Paper)
Food Funct., 2023,
14, 9792-9802
Consuming almonds with chocolate or lettuce influences oral processing behaviour, bolus properties and consequently predicted lipid release from almonds
Received
26th May 2023
, Accepted 7th October 2023
First published on 12th October 2023
Abstract
Lipids in almonds are naturally encapsulated by cell walls which may reduce the actual metabolizable energy content of almonds. Oral processing increases the accessibility of lipids to digestive enzymes by grinding the almond matrix. This study aimed to investigate the effect of adding accompanying foods (chocolate and iceberg lettuce) to almonds on oral processing behaviour, bolus properties and predicted lipid release. Natural chewing times of four almond model foods including one almond (1.3 g), four almonds (4.6 g), one almond with chocolate (4.3 g) and one almond with iceberg lettuce (4.3 g) were collected from n = 59 participants in duplicate. Expectorated boli at the moment of swallowing were characterized for number and mean area of almond bolus particles. Predicted lipid bioaccessibility was estimated using a previously validated model. At similar bite size (4.3–4.6 g), the addition of chocolate and iceberg lettuce to almonds significantly decreased (p < 0.05) chewing time and significantly increased (p < 0.05) eating rate compared to consumption of almonds alone. Almond bolus particle sizes were similar for almonds consumed alone (one and four almonds) and with chocolate, while consuming almonds with lettuce generated significantly fewer and larger almond bolus particles (p < 0.05). Predicted lipid bioaccessibility of almonds consumed with iceberg lettuce was significantly lower (p < 0.05) than for almonds consumed alone (one and four almonds) and almonds consumed with chocolate. Eating rate correlated significantly and positively with the mean area of bolus particles and significantly and negatively with predicted lipid release. In conclusion, combining almonds with other foods such as chocolate and lettuce influences oral processing behaviour and bolus properties and consequently predicted lipid bioaccessibility of almonds, highlighting the impact of food matrix and consumption context on these aspects.
1. Introduction
Almonds (Prunus dulcis) are nuts containing fibers, polyphenols and unsaturated fatty acids1,2 which are known for their beneficial effects on satiety and cardiovascular and gut health.3–9 The high lipid content of almonds is responsible for their high energy content when this is calculated using the general Atwater factors.8,10 However, the metabolizable energy of almonds is substantially lower than that calculated by the Atwater factors because of the cell walls hindering the accessibility of intracellular lipids to digestive enzymes and fluids in the human gastrointestinal tract.8,11–16 Therefore, it has been argued that the actual energy content of almonds is predominantly determined by the degree of breakdown of the almond matrix as the more cells walls are ruptured, the more lipids are released from the almond tissues and digested.17,18
Oral processing leads to the mechanical breakdown of the almond matrix generating smaller and more almond bolus particles with larger total bolus surface area which increases the release of lipids from almonds,7,8,11,13,19,20 Oral processing behaviour depends on food properties.21–25 Differences in oral processing behaviour contribute to differences in bioaccessibility of nutrients.8,14,18,24,26–28 Cassady et al.17 reported that chewing almonds 40 times per bite resulted in higher lipid digestibility than chewing almonds 10 times per bite.
Almonds are consumed in different contexts in real life. Almonds are frequently consumed alone or in combination with other foods like in chocolates or as part of meals like salads. However, the effect of combining almonds with other foods on oral processing behaviour and bolus properties has been underexplored and the potential effects of combining almonds with other foods on lipid digestibility has not been studied.
For instance, Hutchings et al.29 studied the oral processing behaviour and bolus properties of peanuts embedded in two food matrixes, chocolates and gelatin gels. The different matrixes led to differences in oral processing behaviour and peanut bolus particle size distributions. McArthur et al.7 examined bolus properties of different nuts (walnuts, almonds, pistachios) under different contexts including consumption with water, juice, and yoghurt. A higher proportion of larger nut bolus particles was found when nuts were consumed with water, juice and yogurt compared to consumption of nuts alone. Capuano et al.30 reported that incorporation of hazelnuts into breads modestly increased hazelnut bolus particle size.
The aim of the study was therefore to further investigate the effect of combining almonds with other foods on oral processing behaviour and bolus properties by expanding the type of accompanying foods previously investigated.29,30 Next to this, we aim at investigating how the changes in bolus properties would affect the amount of predicted lipid release which has never been assessed so far. We hypothesize that the addition of “softer” foods to almonds leads to shorter chewing time, fewer and larger almond bolus particles and lower predicted lipid bioaccessibility compared to consumption of almonds alone.
2. Materials and methods
2.1 Materials
Roasted, blanched and peeled almonds (Smits Specialiteiten, Rhenen, the Netherlands), milk chocolate (AH milk chocolate bar, Albert Heijn BV, the Netherlands) and precut, packed iceberg lettuce (Jumbo iceberg lettuce 200 g, Jumbo BV, the Netherlands) were bought at local supermarkets. Almonds and milk chocolate were stored at room temperature and iceberg lettuce in the refrigerator at 4 °C and used within 3 days.
2.2 Participants
A previous study by Grundy et al.14 measured predicted lipid release from masticated almond particles by using the theoretical model (8.5% for natural almonds and 11.3% for roasted almonds). Considering the low percentage of predicted lipid bioaccessibility, a power calculation was performed for this study with an effect size of 2.8, a Sigma of 5, a significance level of 0.05 and power of 0.8 (https://hedwig.mgh.harvard.edu/sample_size/js/js_crossover_quant.html) which yielded 53 participants. Considering potential withdrawal, 60 participants, were enrolled in our study. This is a larger cohort compared to similar studies previously published.14,29 Oral processing behaviour and bolus collection were studied with n = 60 participants (33 females, 27 males; 27 ± 4 years; 15 nationalities) in duplicate. Food intolerances, dental status (self-reported), chewing or swallowing status (self-reported) and smoking condition were collected from all participants using questionnaires and were used as selection criteria. Only participants with normal ability to chew and swallow, without history or undergoing treatment for chronic medical illness, no smoking, alcohol or drug use, and no self-reported food allergies or intolerances to the tested foods participated in the study.23,31 Participants had the ability to chew and swallow normally, without history or undergoing treatment for chronic medical illness, no smoking, alcohol or drug use, and no self-reported food allergies or intolerances to the tested foods. All participants (n = 60) completed the study but one participant was excluded from the dataset as data analysis could not be performed for that participant. The data of n = 59 participants (33 females, 26 males; 27 ± 4 years; 15 nationalities) in duplicate was analyzed for this study. All participants gave written informed consent prior to the study and received financial compensation for their participation. The study does not meet the requirements of the ‘Medical Research Involving Human Subjects Act’ of The Netherlands (WMO in Dutch) for assessment by the Medical Research Ethical Committee of The Netherlands. This study was conducted in agreement with the WMA Declaration of Helsinki about Ethical Principles for Medical Research Involving Human Subjects.
2.3 Sample preparation
An overview of the food samples is shown in Table 1. Single-component samples containing of one or four almonds (hereafter referred to as 1A and 4A) and multi-component samples containing of one almond and chocolate (hereafter referred to as 1A + C) or one almond and iceberg lettuce (hereafter referred to as 1A + I) were included in the study. All samples were prepared in duplicate freshly in the morning before the sessions. The total weight of the samples corresponds to the bite size and was approximately equivalent for 4A, 1A + C and 1A + I while 1A had a smaller bite size. The ratio between almond and chocolate of 1A + C was based on the ratio of commercially available milk chocolate bar with almonds (Rittersport Whole Almond chocolate bar which contains 25% almond, i.e., ratio of almond
:
chocolate of 1
:
3). The ratio between almond and iceberg lettuce of 1A + I was based on the average ratio between nuts and salad vegetables in a commercially available meal salad (AH Meal salad mango hazelnuts which contains 7.5% roasted hazelnuts and 28.8% curly lettuce and red cabbage, i.e., ratio of nuts
:
salad vegetables of 1
:
3.8). It was decided to use the same almond
:
food ratio for 1A + C and 1A + I to allow for better comparison between samples. The 1A sample (one almond) was intended to provide information about the effect of bite size of almonds on oral processing behaviour and bolus properties by comparison to the 4A sample (four almonds).
Table 1 Overview of the composition and weights of the four almond model food samples. Total weight of samples corresponds to bite size. Data are reported as mean ± SD (n = 59, duplicate)
Sample description |
One almond |
Four almonds |
One almond with chocolate |
One almond with iceberg lettuce |
Sample name |
1A |
4A |
1A + C |
1A + I |
Sample picture |
|
|
|
|
Almonds (pcs.) |
1 |
4 |
1 |
1 |
Weight almond (g) |
1.29 ± 0.16 |
4.62 ± 0.24 |
1.29 ± 0.16 |
1.29 ± 0.16 |
Weight additional food (g) |
N.A. |
N.A. |
3.03 ± 0.06 |
3.02 ± 0.05 |
Total weight sample (g) |
1.29 ± 0.16 |
4.62 ± 0.24 |
4.32 ± 0.22 |
4.31 ± 0.21 |
2.4 Characterization of oral processing behaviour of almond model food samples
The study consisted of one session with two parts. During the first part of the session of 15 min oral processing behaviour of participants (n = 59) were determined in duplicate for all samples. During the second part of the session of 15 min, expectorated bolus of all samples were collected from participants (n = 59) in duplicate. Chewing time of each sample (1A, 4A, 1A + C, 1A + I) was determined in duplicate, so eight randomized test samples were served to each participant during the first session. Additionally, there was one practice sample to facilitate the participants’ understanding of the tasks. Participants were offered one sample at a time and asked to take the whole sample in their mouth. Participants were instructed to chew naturally on the sample and to raise their hand to indicate the moments of beginning of chewing and swallowing. A stopwatch was used by the researcher to collect the chewing time of participants. The chewing time of the duplicate measurements was directly filled in into an excel spreadsheet and the average normal chewing time (s) for each sample calculated. Eating rate (g min−1) of each almond model food sample was calculated by dividing sample weight (g) by the average chewing time (min).
2.5 Almond bolus collection
Expectorated boli of almond model food samples at the moment of swallowing were collected from the n = 59 participants in duplicate after the determination of chewing time in the second part of the session. Eight randomized test samples including duplicates of 4 samples (1A, 4A, 1A + C, 1A + I) were served to each participant. An additional sample for the practice of expectorating bolus was provided at the beginning. Participants were instructed to chew the samples for the their own individual normal chewing time of each sample based on the results collected from the first part of the session. The effect of chewing frequency (chews per s) was excluded in the present study based on a previous pilot study that indicated interindividual differences in chewing frequency for these four samples were negligible (1.6 ± 0.1 chews per s, p > 0.05). After chewing, the almond bolus was expectorated into labelled containers (Greiner tube). Participants were instructed to spit out as much bolus as possible from their mouths, including any food particles that may have been trapped in the buccal pouches. Collected duplicate almond boli were divided into two parts. One part of the bolus was used for quantification of bolus particle number and size, and the other part of the bolus was used for the determinations of dry matter content and chewing loss.
2.6 Dry matter content
Dry matter content was determined for the individual foods before oral processing (almond, chocolate, and iceberg lettuce) and for the expectorated bolus of the four samples (Table 1) after oral processing. Dry matter content (%) was determined by drying a known amount of wet sample overnight in an oven at 105 °C to constant weight.
2.7 Chewing loss determination
Chewing loss is defined here as the amount of food bolus that is not expectorated during bolus collection as parts of the food bolus remained stuck in the oral cavity or were accidentally swallowed by the participant. Chewing loss was determined to standardize the number of almond particles in the bolus by weight to ensure this parameter is comparable among all samples. A preliminary trial (n = 3) showed considerable inter-individual differences regarding chewing loss whereas intra-individual differences were negligible (data not shown). We therefore assumed that chewing loss was similar in the two parts of the session. We assumed that the same relative proportion of almond and either chocolate or lettuce was lost (i.e., ratio of almond
:
additional food remains 1
:
3).
Chewing loss (%) was calculated with the following equation.
with:
mdry bolus, weight of dry matter in expectorated bolus (g);
mdry matrix, weight of dry matter in unchewed food sample (g).
2.8 Characterization of bolus properties of almond bolus particles
Number of almond bolus particles and mean area of almond bolus fragments were determined using image analysis as previously described24 with slight modification of the methodology. Maximally 3 g of expectorated almond bolus were spread on a Petri dish to ensure that bolus fragments did not overlap or agglomerate on the Petri dish. Expectorated bolus was placed in a large Petri dish (203 × 305 mm) and appropriate amount of water was added to separate the almond bolus fragments manually. Individual bolus fragments were gently separated by shaking and using a spatula. Petri dishes were placed on a flatbed scanner (Canon CanoScan 9000F mark II), and a 600 dpi colour picture was taken with a black background in duplicate.
The current study focused on the effect of adding foods (chocolate and iceberg lettuce) to almonds on bolus properties of the almonds. To quantify the bolus properties of the almond fragments only (number and size of almond bolus fragments), chocolate and iceberg lettuce bolus fragments were removed from the expectorated bolus before scanning. For the 1A + C sample, the bolus parts were separated and weighed into 50 mL Greiner tubes. To dissolve the chocolate, 50 mL hot water (approx. 60 °C) was added to the boli whereafter the Greiner tube was shaken and emptied in the large Petri dish. A pilot study was performed in which it was established that adding 300 mL hot water to 3 g food bolus (1A + C), was enough to dissolve the chocolate to the extent that chocolate did not interfere with the scanning. Then, the Greiner tube was rinsed with hot water for six times (approximately 40 mL per rinse) and merged in the Petri dish. To separate clustered almond bolus fragments, the dish was shaken and a spatula was used to gently separate the particles. For the 1A + I sample, iceberg lettuce fragments were removed manually from the expectorated bolus before scanning using a pincer.
The scans were imported into ImageJ (version 1.52a, National Institute of Health, USA) to conduct image analysis. A macro was used to obtain a binary image by applying a conversion to an 8-bit image, a brightness/contrast adjustment and a black and white threshold adjustment. Brightness/contrast was set to make sure that the translucent or green fragments from iceberg lettuce were excluded. Particles smaller than 0.07 mm2 or circularity smaller than 0.15 were discarded to prevent interference with the background. The projected area is calculated as the center segment of a sphere. For each image, the number of almond bolus particles and bolus mean area (mm2) were obtained and the number of almond bolus particles was standardized by bolus weight (no. per g).
2.9 Predicted lipid release
Lipid release from almonds was predicted using a theoretical model which was developed and previously validated for predicting lipid bioaccessibility of almonds12,14,26 with a modification of bolus particle area transformation. In the current study, the modified model calculated the predicted lipid bioaccessibility of almonds based on the mean area of almond bolus particles. In the original model,12 it was assumed that the almond bolus particles were cubes, but in the current study the almond bolus particles were assumed as spherical. Therefore, the current spherical almond bolus particle was transformed into a cubic particle with an identical surface area (4πr2 = 6 × side area). It is acknowledged that the volume of the transformed cube is smaller than the original sphere (Vcube ≈ 0.72 Vsphere) which could lead consistently to an overestimation of predicted lipid release. Predicted lipid release was calculated with the equation:
with, d = mean diameter of almond cells determined in the original study12 (36 μm); p = side length of the cubic particle which has equivalent surface area as the spherical almond bolus particle (μm).
2.10 Statistical data analysis
Data are expressed as mean ± standard deviation (SD) unless otherwise stated. A significance level of p < 0.05 was chosen. IBM SPSS Statistics (version 25.0) was used to perform all statistical analysis.
Descriptive statistics were applied to explore the characteristics of chewing time (s), eating rate (g min−1), bolus properties (number of particles (no. per g) and mean area (mm2)), predicted lipid release (%), and chewing loss (%) of the four almond model food samples. Normality was checked using Shapiro–Wilk tests and variances were checked using Levene's test.
Kruskal–Wallis H tests and post hoc Bonferroni tests were used to investigate the influence of sample on chewing time (s), eating rate (g min−1), and bolus properties (number of particles (no. per g) and mean area (mm2)) of almonds.
Bivariate Spearman correlation tests (two-tailed) were used to examine relationships between individual chewing time (s), and/or individual eating rate (g min−1), with bolus properties (number of particles (no. per g) and mean area (mm2)), and predicted lipid release (%) in almond for all samples merged by almond model food sample types.
3. Results
Fig. 1 shows chewing time (A) and eating rate (B) of four almond model food samples, i.e., one almond (1A), four almonds (4A), one almond with chocolate (1A + C) and one almond with iceberg lettuce (1A + I).
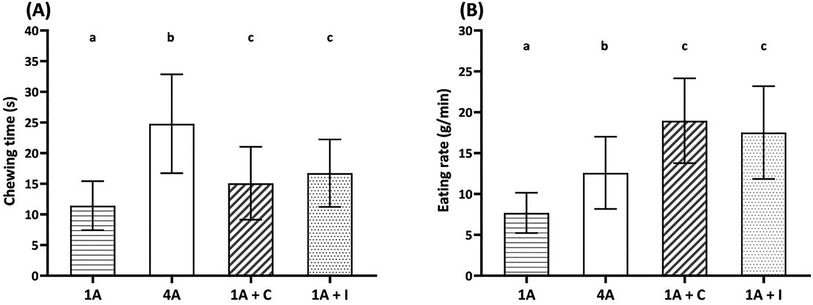 |
| Fig. 1 Chewing time (s) (A) and eating rate (g min−1) (B) of four almond model food samples. 1A: one almond (bite size 1.3 g); 4A: four almonds (bite size 4.6 g); 1A + C: one almond with chocolate (bite size 4.3 g); 1A + I: one almond with iceberg lettuce (bite size 4.3 g). Data are reported as mean ± SD (n = 59, duplicate). Different letters indicate significant differences among samples (p < 0.05). | |
Increasing the bite size resulted in significantly longer (Fig. 1(A), p < 0.05) chewing times and higher (Fig. 1(B), p < 0.001) eating rate by comparing 1A (bite size 1.3 g) and the other samples (4A, 1A + C, and 1A + I; bite size 4.3–4.6 g). Among the three similar bite size almond samples, 4A showed the longest chewing time (p < 0.05) and lowest (p < 0.001) eating rate. The addition of chocolate and iceberg lettuce reduced the chewing time and increased the eating rate compared to 4A. No significant differences in chewing time and eating rate were observed between the addition of chocolate and iceberg lettuce to one almond (1A + C and 1A + I).
Fig. 2 shows chewing loss (%) of four almond model food samples (1A, 4A, 1A + C, and 1A + I). All four food samples showed a considerable chewing loss during the study ranging from 23.4 ± 11.1% for 4A to 40.3 ± 17.0% for 1A + C. Significant and large differences among the almond food samples were observed and large interindividual variation between participants for each model food. Almond bolus properties were therefore standardized by the corresponding remaining weight of wet almond bolus considering the chewing loss (see section 2.7 and 2.8).
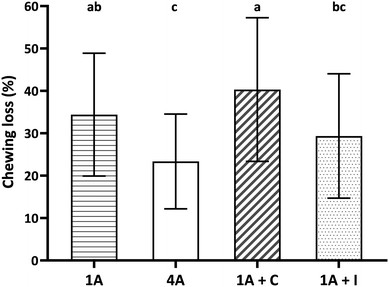 |
| Fig. 2 Chewing loss (%) of the four almond model food samples. 1A: one almond (bite size 1.3 g); 4A: four almonds (bite size 4.6 g); 1A + C: one almond with chocolate (bite size 4.3 g); 1A + I: one almond with iceberg lettuce (bite size 4.3 g). Data are reported as mean ± SD (n = 59, duplicate). Different letters indicate significant differences among samples (p < 0.05). | |
In Fig. 3, representative pictures of different almond model food samples before oral processing, expectorated boli and scans of separated almond boli particles of 1A, 4A, 1A + C, and 1A + I, as well as bolus properties (number and mean area of almond bolus particles) are shown. Although one almond (1A) and four almonds (4A) were significantly different in bite size (1.3 and 4.6 g) and chewing behaviour, they displayed very similar bolus properties in terms of almond bolus particle numbers and mean area (Fig. 3, p > 0.05). Combining one almond with an additional food like chocolate or iceberg lettuce (1A + C and 1A + I) had a significant effect on almond bolus numbers (Fig. 3, p < 0.05) compared to the samples with only almonds (1A and 4A). However, 1A + C showed significantly more and smaller (Fig. 3, p < 0.05) almond bolus particles, 1A + I showed significantly larger (Fig. 3, p < 0.05) mean area of almond bolus fragments compared to other samples.
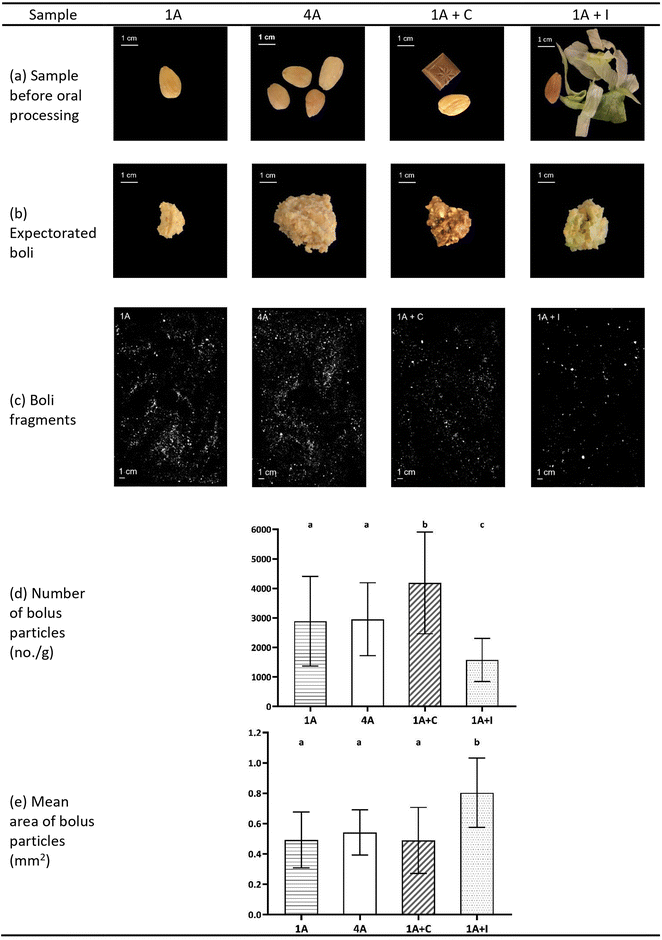 |
| Fig. 3 Bolus properties of the four almond model food samples. 1A: one almond (bite size 1.3 g); 4A: four almonds (bite size 4.6 g); 1A + C: one almond with chocolate (bite size 4.3 g); 1A + I: one almond with iceberg lettuce (bite size 4.3 g). (a) Representative pictures of almond samples before oral processing; (b) representative pictures of expectorated almond boli; (c) representative scans of almond bolus fragments; (d) number of almond bolus particles (no. per g); (e) mean area of almond bolus particles (mm2). Data for (d) and (e) are shown as mean ± SD (n = 59, duplicate). Different letters indicate significant differences among samples (p < 0.05). | |
Fig. 4 shows the predicted lipid release (%) from the four almond food samples (1A, 4A, 1A + C, and 1A + I). One almond, four almonds and one almond with chocolate showed similar predicted lipid release (Fig. 4, p > 0.05) as a consequence of the similarity in the almond bolus particle size. One almond with iceberg lettuce (1A + I) showed significantly lower predicted lipid release compared to the other samples (1A, 4A and 1A + C) (Fig. 4, p < 0.001). Adding iceberg lettuce significantly reduced the predicted lipid release by approximately 3–4% (in absolute terms) compared to the other three samples.
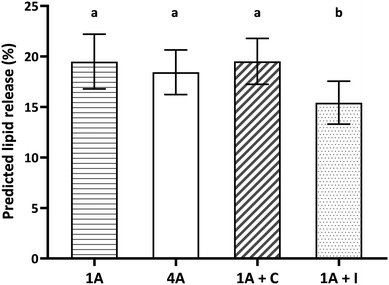 |
| Fig. 4 Predicted lipid release (%) from the four almond model food samples. 1A: one almond (bite size 1.3 g); 4A: four almonds (bite size 4.6 g); 1A + C: one almond with chocolate (bite size 4.3 g); 1A + I: one almond with iceberg lettuce (bite size 4.3 g). Data are reported as mean ± SD (n = 59, duplicate). Different letters indicate significant differences among samples (p < 0.001). | |
Furthermore, we investigated the correlations between chewing time (s) or eating rate (g min−1) with bolus properties (mean area, mm2), and predicted lipid release (%). A positive and significant correlation was found between eating rate (g min−1) and the mean area of bolus particles (mm2) (r = 0.324, p < 0.01). A negative and significant correlation between eating rate (g min−1) and predicted lipid release (%) (r = −0.322, p < 0.01) was found. There were no significant correlations between chewing time and mean area of almond bolus particles and between chewing time and predicted lipid release.
4. Discussion
In this study, we investigated the effects of almond bite size and the addition of two accompanying foods, chocolate and iceberg lettuce, to almonds on oral processing behaviour (chewing time, eating rate), almond bolus properties and predicted almond lipid release. Our results demonstrate that the differences in bite size and combinations of almond with an additional food led to alterations in oral processing behaviour (chewing time and eating rate), surface area of almond bolus, and predicted almond lipid release. For the almond consumed with iceberg lettuce, the predicted almond lipid release was significantly lower than for other samples due to the formation of larger almond bolus surface area during oral processing.
Bite size and the combination of almonds with chocolate and lettuce had significant effects on chewing time and eating rate. As expected, chewing time and eating rate were closely related with each other.32 The sample with only one single piece of almond (1A) showed the shortest chewing time while also the lowest eating rate. The effect of bite size on chewing time was very straightforward and was in line with previous findings that chewing time is prolonged by increasing bite size.33–35 Here we found that chewing time of one almond (1A) was 54% shorter and eating rate 39% slower (in relative terms) compared to four almonds per bite (4A). The effect of bite size on eating rate was also observed in previous studies.36 As one almond contained the smallest bite size (1.3 g) compared to the other 3 samples with almonds (4.3–4.6 g), it required less mastication effort, thereby resulting in a shorter chewing time and lower eating rate. The addition of chocolate and iceberg lettuce (1A + C and 1A + I) significantly reduced chewing time and increased eating rate of the single-component sample with similar bite size (4A) while there were no differences between 1A + C and 1A + I. Chewing time and eating rate could be influenced by multiple factors including food textural and mechanical properties of the food matrix such as hardness, or moisture content, etc.23,35,37–41It has been repeatedly proven that the harder a solid food matrix, the longer the chewing time,42,43 4A contained a much harder matrix (four almonds), than 1A + C and 1A + I (one almond with chocolate or iceberg lettuce) with chocolate and iceberg lettuce which could increase the chewing time and naturally lower the eating rate as these three samples were similar in bite size. Moreover, the moisture content of iceberg lettuce (97%) was much higher than almonds (3%), and previous findings reported that a high moisture content could help to lubricate almond bolus and accelerate the bolus formation.44,45 In addition, for 1A + C, the chocolate started to melt during mastication,29,45,46 which could also enhance the lubrication of the bolus shortening the chewing time and increasing eating rate compared to 4A.
The almond samples (1A and 4A) exhibited similar almond bolus sizes although they produced significantly different oral processing behaviours. This confirms that prolonged chewing time for 4A compared to 1A might have compensated for the difference in bite size so that a safe-to-swallow bolus with similar properties was formed independent of bite size,25,45,48 Moreover, our results showed that bolus properties of almonds were determined not only by oral processing behaviour but also by the mechanical properties of the additional foods. Addition of chocolate and lettuce affected the bolus properties of almonds, though the effect was product-specific. The almond with chocolate (1A + C) displayed similar almond bolus particle size to the 4A sample whereas the almond with iceberg lettuce sample (1A + I) showed significantly larger almond bolus particle size than other samples. This discrepancy in almond bolus properties suggests that the properties of the additional food may influence the breakage function and the selection function of almonds during the oral processing of the composite food.29,47 The main reason for the larger almond particle size of 1A + I was very straightforward as shorter chewing time reduced the extent of breakage of the almond matrix which generated less and larger almond bolus particles.7,25,44 However, 1A + C displayed a much smaller almond bolus particle size than 1A + I even though they were similar in chewing time and eating rate. Possible explanations for this result might be different mechanical properties of the chocolate compared to iceberg lettuce. The presence of fat in chocolate can affect lubrication in the mouth and might create a smoother texture by melting in the oral cavity and coating the almond particles which could increase the selection function of almonds, known as the probability that almond particles presenting to the occlusal plane.29,47 The mouth-coating effect of chocolate facilitated the formation of smaller, more consistent almond bolus particles. In contrast, water-rich iceberg lettuce would instead create a more crunchy texture and the presence of fibre in iceberg lettuce could possibly restrict the selection function of almonds so the molars were less easy to access to the almonds. Iceberg lettuce was less effective in binding almond pieces during chewing, leading to larger, less uniform bolus particles. These differences illustrate how texture properties of the food matrix influence oral processing behaviour and impact bolus particle formation.
Lipids in almonds are present in the form of lipid droplets within the intact almond cells.8 Although almonds are rich in lipids, the actual release of the intracellular lipids is relatively low due to the encapsulation of lipids in the cell walls.10 The human digestive system lacks the necessary enzymes to break down the cell walls and release the lipids for digestion so the particle size distribution ultimately determines the accessibility of lipids to lipases.8,13,14 In the current study, the predicted lipid release of almonds ranged from 15.4% to 19.5% based on the theoretical model.12 The predicted values are higher than the predicted value (8.5%) derived from the same model which was reported by Grassby et al.12 as we assumed a sphere instead of a cube for the shape of almond bolus particles, and hence our data is an overestimation. The relatively low predicted lipid release is in line with previous findings that the extent of the disintegration of intact almonds due to oral processing is quite limited.8,12–14,17 Prolonged chewing time could significantly help with the release of lipids from almonds by rupturing the cell walls but this is less effective than grinding the almond matrix directly into powder.13,14,26,27,49–51Our study showed there was no effect of bite size on predicted lipid release in samples consisting of almonds alone (1A and 4A) because oral processing behaviour of different almond bite size “adapt” to the bite size leading to almond bolus with similar bolus particle sizes.25,47,48 A significant effect of addition of iceberg lettuce on predicted lipid release in almonds was found, but addition of chocolate to almonds did not influence predicted lipid release. The predicted lipid release in almonds was calculated based on the corresponding mean area of almond bolus particle in the current study. This result demonstrates that the differences in almond bolus properties caused by the addition of iceberg lettuce to the almonds were sufficiently larger to cause changes in predicted lipid release. This result may indicate that different mechanical properties of the additional foods could change the consumption context of almonds which could subsequently influence on the oral processing behaviour, bolus properties and nutrients bioaccessibility.52 Capuano et al.30 reported that incorporation of whole hazelnuts into bread matrixes slightly increased the bolus particle size of hazelnuts. One study also reported that consuming almonds together with water, sweet yoghurt and plain yoghurt significantly reduced the chewing time, accelerated the eating rate and generated more and larger almond bolus particles.7 In our study, the addition of chocolate and iceberg lettuce might play a similar role of impacting on the almonds, though as discussed before, the different properties of the additional foods led to the different breakdown behaviour and potential bioaccessibility of the almond lipids.
The current study showed strong correlations between eating rate and chewing time, mean area of almond bolus particles as well as the predicted lipid release in almonds. This is in line with previous findings that a faster eating rate is associated with a shorter chewing time, larger bolus particle size, smaller surface area and less nutrient bioaccessibility.32,53,54 It is widely recognized that a lower eating rate contributes to energy intake within a meal, post-meal satiety/fullness, and relates to the metabolism of nutrients.53–55 In this study, since eating rate largely depends on the mechanical properties of the food matrix,23,53,55,56 the strong correlation of eating rate and predicted lipid release in almonds suggests the possibility to manipulate lipid digestibility in almonds by combining almonds with different foods.
One of the limitations of the present study is that lipid bioavailability has been estimated based on the assumption that this would be proportional to the lipid fraction that is immediately available to lipase, i.e., the fraction that is released, which is a relatively safe assumption for certain nuts like almonds. In addition, the theoretical model is an easy tool to investigate the research aim, and has been previously validated.12 We acknowledge that the use of the average particle size instead of the whole particle size distribution simplifies the complexity of the problem. Further work should determine lipid digestibility using an in vitro digestion model or explore whether the small differences observed in this study may have any physiological significance in vivo. Moreover, it is also worth noticing that undigested lipids encapsulated by almond intact cells could be further metabolized by the gut microbiota which is known to produce certain beneficial metabolites.8,11,50 Microbial metabolism of lipids in the gut mostly produces bioactive metabolites like conjugated linoleic acids (CLAs) or other linoleic acid (LA) metabolites like hydroxy fatty acids which may have physiological relevance in the large intestine,9,57,58 whereas production of short-chain fatty acid from lipids is uncertain and depending on of depletion other more easily available sources of energy.59
5. Conclusions
This study aimed to investigate the effect of adding foods (chocolate and iceberg lettuce) to almonds on oral processing behaviour, bolus properties and predicted lipid release. The study showed the addition of chocolate and iceberg lettuce had a significant effect on alteration of oral processing behaviour including reduction of chewing time and increase of eating rate compared to samples with only almonds at a constant bite size. Only the addition of iceberg lettuce showed a significant and modest decrease of predicted lipid release in almonds by 3–4% (in absolute terms) compared to other samples. We conclude that combining almonds with other foods such as chocolate and lettuce influences oral processing behaviour and bolus properties and consequently predicted lipid bioaccessibility of almonds. The study highlights that the food matrix and consumption context of plant foods like nuts impacts oral behaviour and consequently bolus properties and predicted lipid release. The findings of this study highlighted the importance of evaluating the influence of consumption context on oral processing behaviour and lipid bioaccessibility, since individual foods are commonly consumed in conjunction with other foods as meals. Further studies should explore how the presence of different foods in a meal effect in vivo lipid digestion and utilization by modulating oral processing behaviour.
Author contributions
C. Y., S. M., C. E. study design. C. Y., T. F., T. A. data collection. C. Y. data analysis. C.Y., S. M., C. E. Writing. C. Y., S. M., C. E. review and edit. C. Y. overall responsibility for the final manuscript.
Conflicts of interest
There are no conflicts to declare.
Acknowledgements
Yao Chen received a PhD scholarship from the China Scholarship Council (CSC No. 201807720020).
References
- J. A. Rothwell, J. Perez-jimenez and V. Neveu,
et al., Phenol-Explorer 3.0 : a major update of the Phenol-Explorer database to incorporate data on the effects of food processing on polyphenol content, Database, 2013, 2013, 1–8, DOI:10.1093/database/bat070.
- E. Ros, Nuts and CVD, Br. J. Nutr., 2015, 113, S111–S120, DOI:10.1017/S0007114514003924.
- D. J. A. Jenkins, C. W. C. Kendall and A. Marchie,
et al., Effect of almonds on insulin secretion and insulin resistance in nondiabetic hyperlipidemic subjects : a randomized controlled crossover trial, Metabolism, 2008, 57, 882–887, DOI:10.1016/j.metabol.2008.01.032.
- D. P. Richardson, A. Astrup, A. Cocaul and P. Ellis, The nutritional and health benefits of almonds: a healthy food choice, Food Sci. Tech. Bull. Funct. Foods, 2009, 6(4), 41–50, DOI:10.1616/1476-2137.15765.
- A. Kamil and C. O. Chen, Health Benefits of Almonds beyond Cholesterol Reduction, J. Agric. Food Chem., 2012, 60, 6694–6702 CrossRef CAS PubMed.
- Y. Lee, C. E. Berryman and S. G. West,
et al., Effects of dark chocolate and almonds on cardiovascular risk factors in overweight and obese individuals: A randomized controlled-feeding trial, J. Am. Heart Assoc., 2017, 6(12), 1–14, DOI:10.1161/JAHA.116.005162.
- B. M. McArthur, R. D. Mattes and R. V. Considine, Mastication of nuts under realistic eating conditions: Implications for energy balance, Nutrients, 2018, 10(6), 710, DOI:10.3390/nu10060710.
- E. Capuano, T. Oliviero, V. Fogliano and N. Pellegrini, Role of the food matrix and digestion on calculation of the actual energy content of food, Nutr. Rev., 2018, 76(4), 274–289, DOI:10.1093/NUTRIT/NUX072.
- F. Beppu, M. Hosokawa, L. Tanaka, H. Kohno, T. Tanaka and K. Miyashita, Potent inhibitory effect of trans 9, trans 11 isomer of conjugated linoleic acid on the growth of human colon cancer cells, J. Nutr. Biochem., 2006, 17, 830–836, DOI:10.1016/j.jnutbio.2006.01.007.
- J. A. Novotny, S. K. Gebauer and D. J. Baer, Discrepancy between the Atwater factor predicted and empirically measured energy values of almonds in human diets, Am. J. Clin. Nutr., 2012, 96(2), 296–301, DOI:10.3945/ajcn.112.035782.1.
- P. R. Ellis, C. W. C. Kendall and Y. Ren,
et al., Role of cell walls in the bioaccessibility of lipids in almond seeds, Am. J. Clin. Nutr., 2004, 80(3), 604–613, DOI:10.1093/ajcn/80.3.604.
- T. Grassby, D. R. Picout and G. Mandalari,
et al., Modelling of nutrient bioaccessibility in almond seeds based on the fracture properties of their cell walls, Food Funct., 2014, 5(12), 3096–3106, 10.1039/c4fo00659c.
- G. Mandalari, M. M. L. Grundy and T. Grassby,
et al., The effects of processing and mastication on almond lipid bioaccessibility using novel methods of in vitro digestion modelling and micro-structural analysis, Br. J. Nutr., 2014, 112(9), 1521–1529, DOI:10.1017/S0007114514002414.
- M. M. L. Grundy, T. Grassby and G. Mandalari,
et al., Effect of mastication on lipid bioaccessibility of almonds in a randomized human study and its implications for digestion kinetics, metabolizable energy, and postprandial lipemia, Am. J. Clin. Nutr., 2015, 101(1), 25–33, DOI:10.3945/ajcn.114.088328.
-
A. Alegría, G. Garcia-Llatas and A. Cilla, Static Digestion Models: General Introduction, Springer Cham, 2015 Search PubMed.
- D. Trombetta, A. Smeriglio and M. Denaro,
et al. Understanding the Fate of Almond (Prunus dulcis), Nutrients, 2020, 12(3397), 1–17 Search PubMed.
- B. A. Cassady, J. H. Hollis, A. D. Fulford, R. V. Considine and R. D. Mattes, Mastication of almonds: Effects of lipid bioaccessibility, appetite, and hormone response, Am. J. Clin. Nutr., 2009, 89(3), 794–800, DOI:10.3945/ajcn.2008.26669.
- S. Gallier, K. C. Gordon and H. Singh, Chemical and structural characterisation of almond oil bodies and bovine milk fat globules, Food Chem., 2012, 132(4), 1996–2006, DOI:10.1016/j.foodchem.2011.12.038.
- E. Capuano, The behavior of dietary fiber in the gastrointestinal tract determines its physiological effect, Crit. Rev. Food Sci. Nutr., 2017, 57(16), 3543–3564, DOI:10.1080/10408398.2016.1180501.
-
M. Golding, Exploring and exploiting the role of food structure in digestion, in Interdisciplinary Approaches to Food Digestion, Springer International Publishing, 2019, 81–128, DOI:10.1007/978-3-030-03901-1_5.
- J. Chen, Food oral processing-A review, Food Hydrocolloids, 2009, 23(1), 1–25, DOI:10.1016/j.foodhyd.2007.11.013.
- M. Devezeaux de Lavergne, J. A. M. Derks, E. C. Ketel, R. A. de Wijk and M. Stieger, Eating behaviour explains differences between individuals in dynamic texture perception of sausages, Food Qual. Prefer., 2015, 41, 189–200, DOI:10.1016/j.foodqual.2014.12.006.
- E. C. Ketel, M. G. Aguayo-Mendoza, R. A. de Wijk, C. de Graaf, B. Piqueras-Fiszman and M. Stieger, Age, gender, ethnicity and eating capability influence oral processing behaviour of liquid, semi-solid and solid foods differently, Food Res. Int., 2019, 119, 143–151, DOI:10.1016/j.foodres.2019.01.048.
- Y. Chen, M. Stieger and E. Capuano,
et al., Influence of oral processing behaviour and bolus properties of brown rice and chickpeas on in vitro starch digestion and postprandial glycaemic response, Eur. J. Nutr., 2022, 61(8), 3961–3974, DOI:10.1007/s00394-022-02935-7.
-
C.G Forde and M. Stieger, Metabolic Impacts of Food Oral Processing, in Food Chemistry, Function and Analysis: Oral Processing and Consumer Perception, ed. B. Wolf, S. Bakalis and J. Chen, Royal Society of Chemistry, 2022, 137–186, 10.1039/9781839160622-00137.
- G. Mandalari, M. L. Parker and M. M. L. Grundy,
et al. Understanding the effect of particle size and processing on almond lipid bioaccessibility through microstructural analysis: From mastication to faecal collection, Nutrients, 2018, 10(2), 213, DOI:10.3390/nu10020213.
- E. Capuano, N. Pellegrini, E. Ntone and C. V. Nikiforidis, In vitro lipid digestion in raw and roasted hazelnut particles and oil bodies, Food Funct., 2018, 9(4), 2508–2516, 10.1039/c8fo00389k.
- Y. Chen, E. Capuano and M. Stieger, Chew on it: Influence of oral processing behaviour on in vitro protein digestion of chicken and soy-based vegetarian chicken, Br. J. Nutr., 2020, 126(9), 1408–1419, DOI:10.1017/s0007114520005176.
- S. C. Hutchings, K. D. Foster, J. E. Bronlund, R. G. Lentle, J. R. Jones and M. P. Morgenstern, Mastication of heterogeneous foods: Peanuts inside two different food matrices, Food Qual. Prefer., 2011, 22(4), 332–339, DOI:10.1016/j.foodqual.2010.12.004.
- E. Capuano, N. Pellegrini, R. Van Bommel and M. Stieger, The effect of a bread matrix on mastication of hazelnuts, Food Res. Int., 2020, 137, 109692, DOI:10.1016/j.foodres.2020.109692.
- A. van Eck, V. Fogliano, V. Galindo-Cuspinera, E. Scholten and M. Stieger, Adding condiments to foods: How does static and dynamic sensory perception change when bread and carrots are consumed with mayonnaise?, Food Qual. Prefer., 2019, 73, 154–170, DOI:10.1016/j.foodqual.2018.11.013.
- E. Robinson, E. Almiron-Roig and F. Rutters,
et al., A systematic review and meta-analysis examining the effect of eating rate on energy intake and hunger, Am. J. Clin. Nutr., 2014, 100(1), 123–151, DOI:10.3945/ajcn.113.081745.
- M. B. D. Gavião, L. Engelen and A. Van Der Bilt, Chewing behavior and salivary secretion, Eur. J. Oral. Sci., 2004, 112(1), 19–24, DOI:10.1111/j.0909-8836.2004.00105.x.
- T. Goto, A. Nakamich, M. Watanabe, K. Nagao, M. Matsuyama and T. Ichikawa, Influence of food volume per mouthful on chewing and bolus properties, Physiol. Behav., 2015, 141, 58–62, DOI:10.1016/j.physbeh.2015.01.007.
- Q. Guo, Understanding the oral processing of solid foods : Insights from food structure, Compr. Rev. Food Sci. Food Saf., 2021, 20, 2941–2967, DOI:10.1111/1541-4337.12745.
- E. Van Kleef, C. Kavvouris, H. C. M. Van Trijp, E. Van Kleef, C. Kavvouris and H. C. M. Van Trijp, The unit size effect of indulgent food : How eating smaller sized items signals impulsivity and makes consumers eat less, Psychol. Health, 2014, 29(9), 1081–1103, DOI:10.1080/08870446.2014.909426.
- S. Watanabe and C. Dawes, A comparison of the effects of tasting and chewing foods on the flow rate of whole saliva in man, Arch. Oral. Biol., 1988, 33(10), 761–764, DOI:10.1016/0003-9969(88)90010-6.
- S. Wada, T. Goto and K. Fujimoto,
et al., Changes in food bolus texture during mastication, J. Texture Stud., 2017, 48(2), 171–177, DOI:10.1111/jtxs.12228.
- A. van der Bilt and J. H. Abbink, The influence of food consistency on chewing rate and muscular work, Arch. Oral. Biol., 2017, 83, 105–110, DOI:10.1016/j.archoralbio.2017.07.011.
- A. van Eck, C. Wijne, V. Fogliano, M. Stieger and E. Scholten, Shape up! How shape, size and addition of condiments influence eating behavior towards vegetables, Food Funct., 2019, 10(9), 5739–5751, 10.1039/c9fo01206k.
- N. Zijlstra, R. A. De Wijk, M. Mars, A. Stafleu and C. De Graaf, Effect of bite size and oral processing time of a semisolid food on satiation, Am. J. Clin. Nutr., 2009, 90(2), 269–275, DOI:10.3945/ajcn.2009.27694.
- J. Chen, N. Khandelwal, Z. Liu and T. Funami, Influences of food hardness on the particle size distribution of food boluses, Arch. Oral. Biol., 2013, 58(3), 293–298, DOI:10.1016/j.archoralbio.2012.10.014.
- M. L. Jalabert-Malbos, A. Mishellany-Dutour, A. Woda and M. A. Peyron, Particle size distribution in the food bolus after mastication of natural foods, Food Qual. Prefer., 2007, 18(5), 803–812, DOI:10.1016/j.foodqual.2007.01.010.
-
J. Chen, Bolus Formation and Swallowing, ed. J. Chen and L. Engelen, Blackwell Publishing Ltd, 2012, DOI:10.1002/9781444360943.ch7.
-
J. Chen and A. Rosenthal, Food Texture and Structure. Elsevier Ltd, 2015, vol 1, DOI:10.1016/B978-1-78242-333-1.00001-2.
- H. Singh, A. Ye and M. J. Ferrua, Aspects of food structures in the digestive tract, Curr. Opin. Food Sci., 2015, 3, 85–93, DOI:10.1016/j.cofs.2015.06.007.
- P.W Lucas, J.F Prinz, K.R Agrawal and I.C Bruce, Food physics and oral physiology, Food Qual. Prefer., 2002, 13, 203–213 CrossRef.
- J. M. Frecka, J. H. Hollis and R. D. Mattes, Effects of appetite, BMI, food form and flavor on mastication : almonds as a test food, Eur. J. Clin. Nutr., 2008, 62, 1231–1238, DOI:10.1038/sj.ejcn.1602838.
- G. Mandalari, R. M. Faulks and G. T. Rich,
et al., Release of protein, lipid, and vitamin E from almond seeds during digestion, J. Agric. Food Chem., 2008, 56(9), 3409–3416, DOI:10.1021/jf073393v.
- G. Mandalari, C. Nueno-Palop, G. Bisignano, M.SJ. Wickham and A. Narbad, Potential prebiotic properties of almond (Amygdalus communis L.) seeds, Appl. Environ. Microbiol., 2008, 74(14), 4264–4270, DOI:10.1128/AEM.00739-08.
- M. Zahir, V. Fogliano and E. Capuano, Food matrix and processing modulate: In vitro protein digestibility in soybeans, Food Funct., 2018, 9(12), 6326–6336, 10.1039/c8fo01385c.
- G. Mandalari, C. Bisignano and A. Filocamo,
et al., Bioaccessibility of pistachio polyphenols, xanthophylls, and tocopherols during simulated human digestion, Nutrition., 2013, 29(1), 338–344, DOI:10.1016/j.nut.2012.08.004.
- P. S. Hogenkamp and H. B. Schiöth, Effect of oral processing behaviour on food intake and satiety, Trends Food Sci. Technol., 2013, 34(1), 67–75, DOI:10.1016/j.tifs.2013.08.010.
- A. T. Goh, G. Chatonidi, M. Choy, S. Ponnalagu, M. Stieger and C. G. Forde, Impact of Individual Differences in Eating Rate on Oral Processing, Bolus Properties and Post-Meal Glucose Responses, Physiol. Behav., 2021, 238, 113495, DOI:10.1016/j.physbeh.2021.113495.
- A. T. Goh, J. Y. M. Choy and X. H. Chua,
et al., Increased oral processing and a slower eating rate increase glycaemic, insulin and satiety responses to a mixed meal tolerance test, Eur. J. Nutr., 2021, 60(5), 2719–2733, DOI:10.1007/s00394-020-02466-z.
- D. Bolhuis, C. Forde, Y. Cheng, H. Xu, N. Martin and C. De, Slow Food : Sustained Impact of Harder Foods on the Reduction in Energy Intake over the Course of the Day, PLoS One, 2014, 9(4), 1–7, DOI:10.1371/journal.pone.0093370.
- R Hontecillas, MJ Wannemeulher and DR Zimmerman,
et al., Nutritional Regulation of Porcine Bacterial-Induced Colitis by Conjugated Linoleic Acid, Nutr. Immunol., 2002, 132(7), 2019–2027, DOI:10.1093/jn/132.7.2019.
- H. e. Yang, Y. Li, A. Nishimura, H. f. Jheng and A. Yuliana, Synthesized enone fatty acids resembling metabolites from gut microbiota suppress macrophage-mediated inflammation in adipocytes, Mol. Nutr. Food Res., 2017, 61(10), 1–13, DOI:10.1002/mnfr.201700064.
- Z. Huyan, N. Pellegrini, W. Steegenga and E. Capuano, Insights into gut microbiota metabolism of dietary lipids: the case of linoleic acid, Food Funct., 2022, 13, 4513–4526, 10.1039/d1fo04254h.
|
This journal is © The Royal Society of Chemistry 2023 |