DOI:
10.1039/D3FO01913F
(Paper)
Food Funct., 2023,
14, 8893-8902
Identification and quantification of (poly)phenol and methylxanthine metabolites in human cerebrospinal fluid: evidence of their ability to cross the BBB†
Received
11th May 2023
, Accepted 31st August 2023
First published on 5th September 2023
Abstract
Increasing evidence suggests that dietary (poly)phenols and methylxanthines have neuroprotective effects; however, little is known about whether they can cross the blood–brain barrier (BBB) and exert direct effects on the brain. We investigated the presence of (poly)phenol and methylxanthine metabolites in plasma and cerebrospinal fluid (CSF) from 90 individuals at risk of dementia using liquid chromatography-mass spectrometry and predicted their mechanism of transport across the BBB using in silico modelling techniques. A total of 123 and 127 metabolites were detected in CSF and plasma, respectively. In silico analysis suggests that 5 of the 20 metabolites quantified in CSF can cross the BBB by passive diffusion, while at least 9 metabolites require the aid of cell transporters to cross the BBB. Our results showed that (poly)phenols and methylxanthines are bioavailable, can cross the BBB via passive diffusion or transport carriers, and can reach brain tissues to exert neuroprotective effects.
1. Introduction
(Poly)phenols (PPs) are secondary plant metabolites containing one or more phenolic rings in their structure and have been widely investigated due to their potential health benefits, including neuroprotection.1–5 Another class of compounds largely present in foods such as tea, coffee and chocolate are methylxanthines. Amongst these are caffeine, theophylline and theobromine. Several epidemiological studies have related caffeine consumption with the prevention of neurodegenerative diseases, in particular with Alzheimer's disease and Parkinson's disease.6–9
One of the hypotheses on the effects of (poly)phenols on brain function is that their metabolites need to reach the brain to exert beneficial effects at an effective concentration.10 The same is expected for methylxanthines as the mechanism of action of these compounds in the central nervous system includes antagonism of adenosine receptors.11
The blood–brain barrier (BBB) is a dynamic interface which plays a key role in regulating the entrance of xenobiotics into the brain and implies several transport systems. On top of being dependent on the stereochemistry of the metabolites, the interactions with the BBB can involve efflux transporters such as P-glycoprotein or multidrug resistance-associated proteins.12,13In vitro and animal studies on rodents showed the potential of phenolic and methylxanthine compounds to penetrate the brain and potentially exert neuroprotective benefits.13–16 However, cell and animal studies present some limitations as many of the available in vitro studies do not take into consideration the use of physiological and dietary-achievable doses in their design, and the comparability of animal models with human physiology in terms of metabolic transformations tends to be lacking in animal studies.17 In addition, little is known about the preferable route used (simple diffusion or carrier-mediated transport) and the brain bioavailability of (poly)phenols and methylxanthines.
To overcome these limitations, we intended to perform a multi-dimensional approach using a human study and in silico prediction models, to investigate whether a large number of (poly)phenols and methylxanthine metabolites can cross the BBB. To do so, we analysed and quantified metabolites in the cerebrospinal fluid (CSF) and plasma of 90 volunteers, alongside in silico modelling to predict which compounds are most likely to cross the BBB by passive diffusion and to assess the parameters influencing their entrance into the brain structure.
2. Materials and methods
2.1. Study population and design
In this study, biological samples collected from individuals participating in the AnthoCyanins In Dementia (ACID) study (ClinicalTrial.gov identifier NCT03419039) were used. The trial's design has been described in a previous study.18 Briefly, the ACID study was a 24-week randomized, double-blind, controlled, parallel group, multi-center, single country study investigating the effect of purified anthocyanin extract supplementation (Medox® capsules, 320 mg day−1) on memory and cognition. The population consisted of males and females aged 60–80 years with no or stable CNS medication and either with mild cognitive impairment (MCI) and/or cognitively healthy having two or more cardiometabolic disorders (CMD) such as diabetes, hypertension, etc. The study was conducted in accordance with the Declaration of Helsinki and approved by the Norwegian and UK Regional Ethics Committees (2017/374). All volunteers provided written consent prior to being enrolled in the study. The present analysis included a sub-sample of 90 individuals (both intervention and control participants) that provided both plasma and CSF samples at the baseline (ESI Fig. 1†). The baseline characteristics of the 90 subjects are presented in Table 1.
Table 1 Baseline characteristics of the 90 subjects included in the present investigation
|
All (n = 90) |
BMI, body mass index; CERAD, Consortium to Establish a Registry for Alzheimer's Disease neuropsychological battery; GDS, geriatric depression scale; MMSE, mini mental state examination. Data are presented as mean ± SD or frequency (percentage), depending on the nature of the variable. |
Sex (n males, %) |
43 (47.8) |
Age (y) |
69.2 ± 5.4 |
Height (cm) |
171 ± 8.5 |
Weight (kg) |
80.6 ± 15.2 |
BMI (kg m−2) |
27.4 ± 4.4 |
Systolic blood pressure (mmHg) |
150 ± 19.2 |
Diastolic blood pressure (mmHg) |
82.8 ± 10.2 |
Pulse (bpm) |
65.5 ± 10.8 |
Nationality |
Norwegian (n, %) |
87 (96.7) |
Other (n, %) |
3 (3.3) |
Working (% of active subjects) |
27 (30) |
Years of education (y) |
14.3 ± 3.2 |
Family history of dementia (n, %) |
61 (67.8) |
Smoking status (% of smokers) |
6 (6.7) |
Alcohol consumption (units per week) |
4.2 ± 4.6 |
Caffeine intake (cups of coffee per day) |
3.2 ± 1.8 |
Hypercholesterolemia (n, %) |
55 (61.1) |
Hypertension (n, %) |
55 (61.1) |
Diabetes type 1 (n, %) |
3 (3.3) |
Diabetes type 2 (n, %) |
13 (14.4) |
Mild cognitive impairment (n, %) |
29 (32.2) |
ApoE status |
|
Non-carriers (ε3/3) (n, %) |
52 (57.8) |
ApoE ε3/4 heterozygote (n, %) |
31 (34.4) |
ApoE ε4/4 homozygote (n, %) |
4 (4.4) |
Ambiguous (ε2/4 or ε1/ε3) (n, %) |
3 (3.3) |
MMSE (average score) |
28.8 ± 1.5 |
GDS (average score) |
1.1 ± 1.3 |
CERAD (average score) |
19.0 ± 1.8 |
2.2. Sample collection, storage, and liquid chromatography-mass spectrometry (LC-MS) analysis
Baseline plasma and cerebrospinal fluid (CSF) samples were collected between 2018 and 2020 at three different centers in Norway (Stavanger University Hospital, Betanien Hospital in Bergen and Akershus University Hospital in Oslo) in fasted participants by trained research physicians in the morning. All samples were immediately kept on ice and processed within an hour of collection. Each participant's blood was collected into EDTA tubes and centrifuged at 1800g for 15 minutes at 4 °C. Plasma was then aliquoted and spiked with 2% formic acid before being stored at −75 °C. During the lumbar puncture, CSF was drained into vials and inspected for the presence of blood and discarded if any suspicion of contamination existed. Samples were centrifuged at 1800g for 15 minutes at 4 °C before being spiked with 2% formic acid and stored at −75 °C until analysis.
For LC-MS analysis, plasma and CSF samples were defrosted and immediately processed. (Poly)phenol metabolites were extracted from CSF and plasma samples using micro-elution solid phase extraction (μ-SPE) and measured by UPLC-Q-q-Q MS using a validated method.19 Briefly, undiluted CSF and plasma samples were acidified with 4% phosphoric acid (v
:
v 1
:
1). The mixture (600 μL) was loaded onto Oasis 96-well reversed-phase HLB (hydrophilic–lipophilic balanced) sorbent μ-SPE plates (Waters, Eschborn, Germany) and eluded with 90 μL of methanol after washing. Identification and quantification of (poly)phenol metabolites were performed on a SHIMADZU triple quadrupole mass spectrometer (LCMS-8060, SHIMADZU, Kyoto, Japan). Eluded samples (5 μL) were injected through a Raptor Biphenyl column (2.1 × 50 mm, 1.8 μm; Restek, Bellefonte, USA) with a compatible Raptor Biphenyl guard cartridge (5 × 2.1 mm; Restek, Bellefonte, USA) in the UPLC system. The two mobile phases were composed of HPLC grade water (solvent A, Sigma-Aldrich, Steinheim, Germany) and HPLC grade acetonitrile (Sigma-Aldrich, Steinheim, Germany) with 0.1% formic acid (solvent B). A 14-minute gradient followed by a 2-minute equilibration was applied to the run at a flow rate of 0.5 mL min−1 at 30 °C. The gradient was initially set at 1% solvent B, held for 1 minute, then increased to 12% at 4 minutes and maintained for a further 4 minutes. After 0.1 minutes, the percentage of B rose to 15%, remained steady for 2.9 minutes, then increased to 30% at 11.5 minutes and 99% at 12 minutes, and remained constant for another 2 minutes. Finally, a 2 min equilibration was applied to revert the proportion of B to 1%. The metabolites in samples were identified by comparing retention times with standards in the corresponding multiple reaction monitoring (MRM) transitions and quantified by calibration curves made from standard mixes using SHIMADZU LabSolutions™ LCMS software. The list of the 127 metabolites analyzed in CSF and plasma can be found in ESI Table S1.†
2.3. Structural in silico analysis
A database containing the structures of the 20 molecules quantified in CSF, including (poly)phenols and methylxanthines, was used. The 3D structures were created using Chem3D Pro (v.20.0, PerkinElmer, Waltham, MA, USA). Initially, these structures were imported as mol2 files into the Maestro software package (version 2022-1, Schrödinger, New York, NY, USA). The structures were then treated with LigPrep (version 2022-1, Schrödinger) using the OPLS4 force field. We defined pH 7.4 ± 2.0 as a biologically relevant target pH using Epik (version 2022-1, Schrödinger). For each molecule, 44 molecular descriptors were calculated (ESI Table S2†) using QikProp in normal mode (version 2022-1, Schrödinger). These molecular descriptors are relevant for predicting the chemical features and inferring the capability of molecules to cross the BBB by passive diffusion.
2.4. Statistical analysis
Plasma and CSF concentrations are expressed as mean ± standard deviation (SD), median and interquartile range (IQR) and percentage (%) of samples analyzed with concentrations above the limit of quantification. Correlations were tested using the Pearson correlation for plasma and CSF concentrations or their logarithm values. The Pearson correlation coefficient was calculated using GraphPad Prism (version 9.3, GraphPad, CA, USA). Compound saturation in the CSF was denoted for each individual compound when the coefficient of variation of CSF concentrations did not change more than 10% upon increasing micromolar concentrations in the plasma of different volunteers.
3. Results
3.1. Identification and quantification of (poly)phenol and methylxanthine metabolites in plasma
All the 127 (poly)phenol and methylxanthine metabolites analysed in our targeted metabolomics method were detected, of which 123 were above the limit of quantification (LOQ) and therefore quantified (ESI Table S1† and Fig. 1A). The 4 compounds below the LOQ were 2,3-dihydroxybenzoic acid, 4-hydroxybenzoic acid-3-glucuronide, α-hydroxyhippuric acid and daidzein.
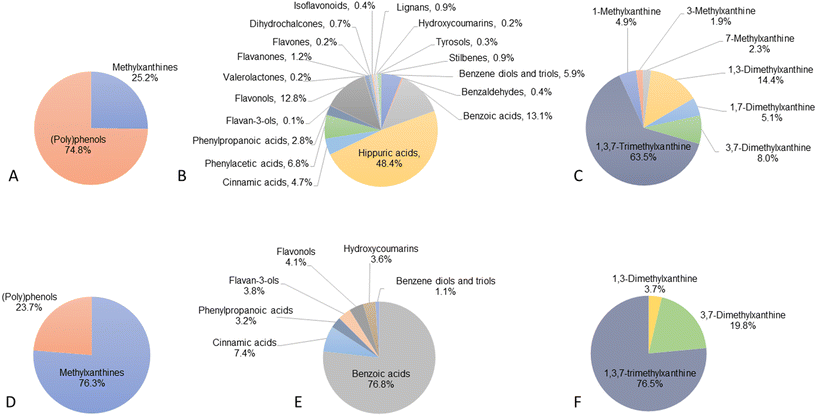 |
| Fig. 1 Percentage (%) of (poly)phenol and methylxanthine alkaloid metabolites detected and quantified in the plasma and CSF of volunteers (n = 90). (A) total (poly)phenol and methylxanthine metabolites found in plasma; (B) subclasses of (poly)phenol metabolites found in plasma; (C) subclasses of methylxanthine metabolites found in plasma; (D) total (poly)phenol and methylxanthine metabolites found in CSF; (E) subclasses of (poly)phenol metabolites found in CSF; and (F) subclasses of methylxanthine metabolites found in CSF. | |
Plasma (poly)phenol metabolite concentrations ranged from 5.3 ± 4.1 nM for 3,4,5-trihydroxybenzene ethyl ester to 387 ± 243 μM for hippuric acid (ESI Table S1†). As shown in Fig. 1B, the most abundant groups were hippuric acids (48.4%), followed by benzoic acids (13.1%), flavonols (12.8%), phenylacetic acids (6.8%), benzene diols and triols (5.9%), cinnamic acids (4.7%), phenylpropanoic acids (2.8%) and flavanones (1.2%). The rest of the groups represented less than 1% of the total (poly)phenol metabolites. Among the 116 (poly)phenol compounds quantified, 63 were detected and quantified in all volunteers, while the remaining 53 compounds were detected in only some participants, including 40 compounds detected in less than 50% of the subjects and 13 compounds in more than half the volunteers.
Among the 7 methylxanthine alkaloids analysed, all 7 were identified and detected in 100% of participants. Methylxanthine concentrations ranged from 5.3 ± 3.8 μM for 3-methylxanthine to 179 ± 118 μM for 1,3,7-trimethylxanthine (caffeine). The most abundant group, representing 63.5% of the total methylxanthines, was caffeine, followed by 1,3-dimethylxanthine (theophylline, 14.3%), 3,7-dimethylxanthine (theobromine, 8.0%) and 1,7-dimethylxanthine (paraxanthine, 5.1%). The remaining methylxanthines represented less than 5% of the total methylxanthines quantified (Fig. 1C).
3.2. Identification and quantification of (poly)phenol and methylxanthine metabolites in CSF
A total of 124 out of 127 compounds were detected in CSF, including 117 (poly)phenol metabolites and 7 methylxanthine alkaloids (ESI Table S1† and Fig. 1D). (Poly)phenol metabolites detected included derivatives from the families of cinnamic acids (n = 21), benzoic acids (n = 21), phenylpropanoic acids (n = 14), flavonols (n = 8), benzene diols and triols (n = 7), stilbenes (n = 6), hippuric acids (n = 5), flavanones (n = 5), lignans (n = 5), tyrosols (n = 5), phenylacetic acids (n = 4), isoflavonoids (n = 4), hydroxycoumarins (n = 4), benzaldehydes (n = 3), flavan-3-ols (n = 2), phenyl-γ-valerolactones (n = 1), flavones (n = 1) and dihydrochalcones (n = 1). These 124 compounds included 29 sulfate and 22 glucuronide metabolites (ESI Table S1†).
Among the detected metabolites in CSF, 20 compounds were present in levels above the LOQ and thus quantified, including 3 methylxanthine and 17 (poly)phenol metabolites from 7 subclasses (benzene diols and triols, n = 2; benzoic acids, n = 3; cinnamic acids, n = 5; phenylpropanoic acids, n = 2; flavan-3-ols, n = 2; flavonols, n = 2; hydroxycoumarins, n = 1). Among the (poly)phenol metabolites, the most abundant were benzoic acid derivatives (76.8% of the total (poly)phenol quantified), followed by cinnamic acids (7.4%) and flavonols (4.1%) (Fig. 1E and F).
(Poly)phenol metabolite concentrations in quantified samples ranged from 1.7 nM for 3-(3′-methoxyphenyl)propanoic acid-4′-glucuronide (dihydroferulic acid-4′-glucuronide) to 367 ± 292 nM for 3-hydroxybenzoic acid, while methylxanthine levels ranged from 65.2 ± 63 nM for 1,3-dimethylxanthine (theophylline) to 1348 ± 612 nM for 1,3,7-trimethylxanthine (caffeine). Among these 20 compounds, 13 were quantified in all volunteers, while 7 were quantified only in some volunteers, with caffeine and 3,7-dimethylxanthine quantified in 88 and 87 participants out of 90, respectively (Table 2). The 5 other compounds were all quantified in less than half the volunteers, from 1,3-dimethylxanthine being quantified in 43.3% of subjects to 3-(3′-methoxyphenyl)propanoic acid-4′-glucuronide being quantified in only 1 out of 90 individuals.
Table 2 CSF concentrations of the 20 compounds detected and/or quantified. Values shown are the descriptive statistics of the samples detected and quantified only
Compound name |
LOQ |
Mean |
SD |
Median |
IQR |
% samples quantified (>LOQ) |
Units in nM. IQR, interquartile range; LOQ, limit of quantification; SD, standard deviation. |
Benzene diols and triols
|
2,3-Dihydroxybenzene-1-sulfate |
1.58 |
3.8 |
0.15 |
3.8 |
0.2 |
100
|
2,6-Dihydroxybenzene-1-sulfate |
1.58 |
2.2 |
0.27 |
2.1 |
0.1 |
100
|
Benzoic acids
|
3-Hydroxybenzoic acid |
16.7 |
367 |
292 |
304 |
532 |
10.0
|
2,5-Dihydroxybenzoic acid |
16.7 |
35.3 |
0.08 |
35.3 |
0.1 |
100
|
4-Methoxybenzoic acid-3-sulfate |
8.43 |
18.8 |
0.44 |
18.8 |
0.4 |
100
|
Cinnamic acids
|
3′,4′-Dihydroxycinnamic acid |
1.76 |
4.7 |
3.19 |
3.8 |
5.0 |
20.0
|
3′-Methoxycinnamic acid-4′-glucuronide |
17.2 |
18.1 |
12.7 |
13.2 |
10.6 |
4.40
|
4′-Methoxycinnamic acid-3′-sulfate |
8.06 |
12.9 |
0.04 |
12.8 |
0.0 |
100
|
5-O-Caffeoylquinic acid |
1.67 |
3.2 |
0.06 |
3.2 |
0.0 |
100
|
4-O-Feruloylquinic acid |
1.65 |
1.8 |
0.05 |
1.8 |
0.1 |
100
|
Phenylpropanoic acids
|
3-(2′,3′-Dihydroxyphenyl)propanoic acid |
7.48 |
15.9 |
0.37 |
15.8 |
0.5 |
100
|
3-(3′-Methoxyphenyl)propanoic acid-4′-glucuronide |
1.56 |
1.7 |
— |
1.7 |
0.0 |
1.10
|
Flavan-3-ols
|
(−)-Epicatechin |
8.34 |
8.5 |
0.38 |
8.5 |
0.4 |
100
|
(−)-Epicatechin-3′-sulfate |
8.13 |
12.1 |
0.46 |
11.9 |
0.3 |
100
|
Flavonols
|
Quercetin-3′-sulfate |
8.36 |
18.1 |
0.01 |
18.1 |
0.0 |
100
|
Quercetin-3′-glucuronide |
1.73 |
4.5 |
0.05 |
4.5 |
0.0 |
100
|
Hydroxycoumarins
|
7,8-Dihydroxycoumarin |
8.43 |
19.6 |
0.03 |
19.6 |
0.0 |
100
|
Methylxanthine alkaloids
|
1,3-Dimethylxanthine |
17.0 |
65.2 |
63.0 |
43.9 |
57.5 |
43.3
|
3,7-Dimethylxanthine |
8.30 |
348 |
197 |
333 |
262 |
96.7
|
1,3,7-Trimethylxanthine |
17.4 |
1348 |
612 |
1313 |
661 |
97.8
|
Three compounds out of the 127 detected in plasma were not detected in CSF. This is the case for 3,4,5-trihydroxybenzene ethyl ester, 4′-hydroxy-3′,5′-dimethoxycinnamic acid, and ellagic acid. Furthermore, 4 metabolites were detected yet not quantified in both plasma and CSF, including 2,3-dihydroxybenzoic acid, 4-hydroxybenzoic acid-3-glucuronide (protocatechuic acid-3-glucuronide), α-hydroxyhippuric acid and daidzein (ESI Table S1†).
3.3. Comparison between plasma and CSF metabolites
Among the 127 molecules detected in plasma, 124 were detected in the CSF, while 20 were detected and quantified in both CSF and plasma. Across the 20 molecules quantified, all presented significantly lower concentrations in CSF in comparison with plasma. Notwithstanding, some differences were observed across the 20 molecules quantified. While some molecules such as 3′,4′-dihydroxycinnamic acid (caffeic acid, 1569
:
1) and 1,3-dimethylxanthine (theophylline, 620
:
1) presented a higher plasma
:
CSF ratio, others such as (−)-epicatechin (24
:
1), quercetin-3-glucuronide (23
:
1) and 7,8-dihydroxycoumarin (5
:
1) showed a lower plasma
:
CSF ratio. Overall, we observed a correlation among mean plasma and CSF concentrations for the 20 molecules quantified in both body fluids (Pearson correlation coefficient = 0.63, p = 0.003, 95%CI = 0.265–0.840, ESI Fig. S2†). When analysing each compound individually, no significant correlation was found. Interestingly, for some molecules, saturation of the concentration in CSF was detected based on the increasing plasma concentrations of different volunteers (Fig. 2). For these molecules, an increase in micromolar concentrations in the plasma of different volunteers did not significantly change the nanomolar concentrations in CSF, as shown by a coefficient of variation of up to 10% (Fig. 2). This was observed for 2,3-dihydroxybenzene-1-sulfate, 2,6-dihydroxybenzene-1-sulfate, 2,5-dihydroxybenzoic acid, 4-methoxybenzoic acid-3-sulfate, 5-O-caffeoylquinic, (−)-epicatechin-3-sulfate, 3-(2′,3′-dihydroxyphenyl)propanoic acid, 4′-methoxycinnamic acid-3-sulfate and quercetin-3′-sulfate (Fig. 2). The remaining molecules detected in CSF, namely quercetin-glucuronide, 4-O-feruoylquinic acid, 7,8-dihydrocoumarin and (−)-epicatechin also showed a coefficient of variation of up to 10% for CSF concentration (ESI Fig. S5†). However, for these four molecules, the concentrations detected in plasma were very low (below 1 μM) and thus further studies are required to confirm saturation in the CSF (ESI Fig. S4†). However, a large variability was observed for the three methylxanthines identified in CSF samples, together with 3-hydroxybenzoic acid and 3′,4′-dihydroxycinnamic acid (ESI Fig. S3†). Moreover, two molecules, namely 3-(3′-methoxyphenyl)propanoic acid-4′-glucuronide and 3′-methoxycinnamic acid-4′-glucuronide were detected in a low number of volunteers.
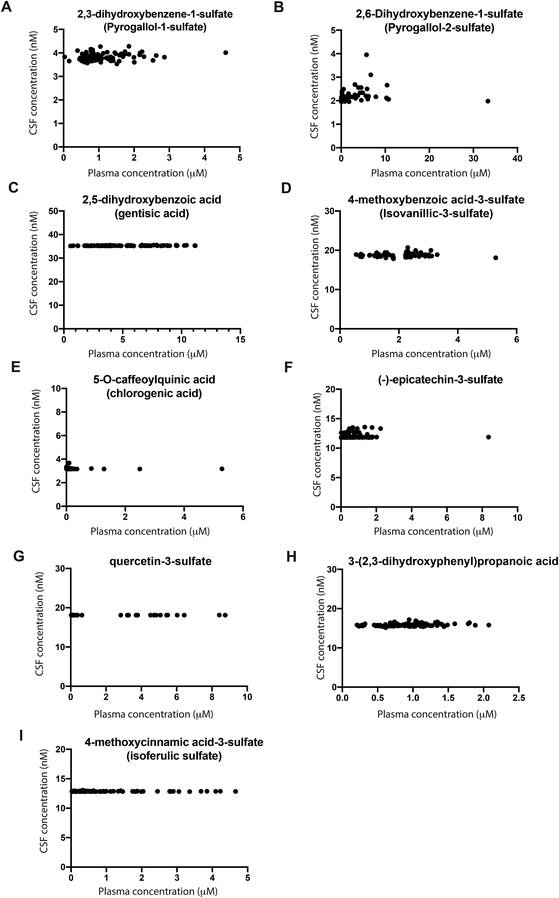 |
| Fig. 2 Increasing plasma concentrations detected in different volunteers (in the micromolar range) suggest saturation in CSF levels for (A) 2,3-dihydroxybenzene-1-sulfate; (B) 2,6-dihydroxybenzoic acid-1-sulfate; (C) 2,5-dihydroxybenzoic acid; (D) 4-methoxybenzoic acid-3-sulfate; (E) 5-O-caffeoylquinic acid; (F) (−)-epicatechin-3-sulfate; (G) quercetin-3-sulfate; (H) 3-(2′,3′-dihydroxyphenyl)propanoic acid; and (I) 4′-methoxycinnamic acid-3′-sulfate. | |
3.4. Structural analysis: in silico predicted blood–brain permeability
To explore whether these differences could be related to the molecular mechanism of transport across the BBB, we used an in silico model to predict which molecules could cross the BBB via passive diffusion. The partition coefficient between octanol and water has been extensively used as a simple yet effective model to explore whether a molecule can cross the BBB. All 20 evaluated molecules showed predicted values within the acceptable range for passive diffusion (Fig. 3A). QikProp also calculated the predicted coefficient between the brain and blood (Fig. 3B). Using this parameter, 3′-methoxycinnamic acid-4′-glucuronide (ferulic acid-glucuronide), 5-O-caffeoylquinic acid, 3-(3′-methoxyphenyl)propanoic acid-4′-glucuronide (dihydroferulic acid-glucuronide), quercetin-3′-sulfate, and quercetin-3′-glucuronide were found to be outside the expected range for passive diffusion. Unexpectedly, these molecules outside the range corresponded to more polar molecules, mainly glucoside and glucuronide conjugates, detected in the CSF. However, several sulfates were within the defined range for passive permeability.
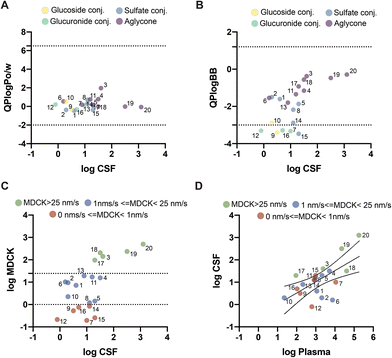 |
| Fig. 3 Insights into the transport mechanism of (poly)phenol and methylxanthine metabolites across the BBB. Several blood–brain barrier passive diffusion parameters were predicted using QikProp and compared with the CSF concentrations: (A) QikProp predicted logarithm partition octanol/water (QPlogPo/w); (B) QikProp predicted logarithm blood–brain partition coefficient (QPlogBB) and (C) logarithm predicted Madin–Darby canine kidney transport (logMDCK) in nm s−1. (D) Pearson's correlation of the molecules quantified in both plasma and CSF (Pearson's coefficient = 0.63, p = 0.003, 95%CI = 0.265–0.840) with the colour indication of QikProp predicted logMDCK. Molecules with high logMDCK, CSF and blood values suggest that these molecules reach the CSF by passive diffusion. Meanwhile, several molecules with low logMDCK values were detected in significant concentrations in both body fluids suggesting other mechanisms of transport across the BBB not related to passive diffusion. (1) 2,3-dihydroxybenzene-1-sulfate; (2) 2,6-dihydroxybenzene-1-sulfate; (3) 3-hydroxybenzoic acid; (4) 2,5-dihydroxybenzoic acid; (5) 4-methoxybenzoic acid-3-sulfate; (6) 3′,4′-dihydroxycinnamic acid; (7) 3′-methoxycinnamic acid-4′-glucuronide; (8) 4′-methoxycinnamic acid-3′-sulfate; (9) 5-O-caffeoylquinic acid; (10) 4-O-feruloylquinic acid; (11) 3-(2′,3′-dihydroxyphenyl)propanoic acid; (12) 3-(3′-methoxyphenyl)propanoic acid-4′-glucuronide; (13) (−)-epicatechin; (14) (−)-epicatechin-3′-sulfate; (15) quercetin-3′-sulfate; (16) quercetin-3′-glucuronide; (17) 7,8-dihydroxycoumarin; (18) 1,3-dimethylxanthine; (19) 3,7-dimethylxanthine; and (20) 1,3,7-trimethylxanthine. | |
Predicted permeability across MDCK (Madin–Darby canine kidney) cells has also been used as a good indicator of passive diffusion across the BBB and is predicted using QikProp based on the chemical structure of each compound. This parameter offers a more quantitative prediction of passive diffusion (in nm s−1). According to QikProp, molecules with a value above 25 nm s−1 cross the BBB by passive diffusion, whereas molecules with a value below 25 nm s−1 have poor to no permeability across the BBB. According to our results, five molecules had predicted values above 25 nm s−1: 3-hydroxybenzoic acid, 1,3-dimethylxanthine, 3,7-dimethylxanthine, 1,3,7-trimethylxanthine, and 7,8-dihydroxycoumarin (Fig. 3C). Six molecules had logMDCK values below 1 nm s−1, indicating almost no permeability across the BBB by passive diffusion: 3′-methoxycinnamic acid-4′-glucuronide, 5-O-caffeoylquinic acid, 3-(3′-methoxyphenyl)propanoic acid-4′-glucuronide, (−)-epicatechin-3′-sulfate, quercetin-3′-sulfate and quercetin-3′-glucuronide. The remaining molecules had values between 1 and 25 nm s−1 (Fig. 3C).
When comparing the results obtained from the predicted MDCK values with the concentrations present in the blood and CSF (Fig. 3D), it was observed that molecules with higher concentrations in plasma also had the highest average concentration in CSF and higher predicted MDCK values. Meanwhile, molecules with predicted MDCK levels below 1 nm s−1, such as 5-O-caffeoylquinic acid and 3-(3′-methoxyphenyl)propanoic acid-4′-glucuronide, showed low concentrations in the CSF. In contrast, molecules such as (−)-epicatechin-3′-sulfate, quercetin-3′-sulfate and quercetin-3′-glucuronide that had small MDCK values of 0.84 nm s−1, 0.25 nm s−1 and 0.75 nm s−1, respectively, showed concentrations in CSF on par with molecules with higher MDCK values, indicating that these molecules might cross the BBB and reach the CSF through the action of other mechanisms besides passive transport, like the action of specific cell transporters. In fact, some molecules with predicted MDCK values below 25 nm s−1 displayed some degree of saturation in the CSF upon increasing plasma concentrations in different volunteers, also suggesting the involvement of cell transporters. These molecules were 2,3-dihydroxybenzene-1-sulfate, 2,6-dihydroxybenzene-1-sulfate, (−)-epicatechin-3′-sulfate, quercetin-3′-sulfate, quercetin-3′-glucuronide, 2,5-dihydroxybenzoic acid, 4-methoxybenzoic acid-3-sulfate, 4′-methoxycinnamic acid-3′-sulfate, 5-O-caffeoylquinic acid, 4-O-feruloylquinic acid and quercetin-3′-glucuronide.
4. Discussion
In this study, a total of 124 metabolites were detected in the CSF of 90 older individuals with MCI and/or cardiometabolic diseases, including 117 (poly)phenol and 7 methylxanthine metabolites. Among those, the presence of 93 (poly)phenol and 3 methylxanthine metabolites in CSF is reported here for the first time, including 29 sulfate and 22 glucuronide metabolites of benzene diols and triols, benzoic acids, cinnamic acids, phenylpropanoic acids, phenylacetic acids, flavan-3-ols, flavanones, flavonols, isoflavonoids, lignans, tyrosols and stilbenes. Some of these compounds can only come from food sources such as naringenin and hesperetin (abundant in citrus fruits), flavan-3-ols (abundant in tea, cocoa, apples, and berries), lignans (seeds and wholegrain foods), stilbenes (red grapes and berries), isoflavonoids (soy products), and tyrosols (olive oil and oats).1 Other compounds can come from different dietary sources including commonly used food additives such as benzoic acid, but also from endogenous metabolism such as hippuric and phenylacetic acids.20–23
As expected, the levels of these metabolites in CSF were low, and only 17 (poly)phenol metabolites out of 117 were above the limit of quantification of our method. Among them, 6 sulfate and 3 glucuronide metabolites were quantified. The most abundant metabolite in CSF was 3-hydroxybenzoic acid, present at a level of 367 ± 292 nM, which is above the concentration reported by a previous study (58.5 nM).24 To our knowledge, we detected and quantified for the first time chlorogenic acid (5-O-caffeoylquinic acid) and gallic acid in CSF, which were not detected in previous work.24,25
Regarding the methylxanthine metabolites, we detected all 7 metabolites analysed including caffeine, theobromine, paraxanthine and theophylline in the plasma and CSF of all volunteers. However, only theophylline, theobromine and caffeine were quantified in CSF in 43%, 97% and 98% of the subjects, respectively. The caffeine concentration found in CSF in the present study (1348 ± 612 nM) was higher than those reported in previous work by Lin et al. (1.90 nM) and Sachse et al. (42.9 nM),26,27 and the same trend is observed for the concentrations of theophylline and theobromine. This could be due to a higher consumption of caffeine-rich foods such as coffee in our study population (average intake of 3.32 cups per day) or a higher permeability of the BBB in our older population at risk of vascular dementia.28,29
To date, the mechanisms of transport of (poly)phenol and methylxanthine metabolites across the BBB are largely unknown. This has been due to the lack of human studies nvestigating the levels of (poly)phenol and methylxanthine metabolites in brain and CSF samples. In our study, we found large amounts of 3-hydroxybenzoic acid in CSF. This is in accordance with a previous study in rats that found that 3-hydroxybenzoic acid accumulates in the brain after ingestion of dietary achievable amounts of (poly)phenol-rich grape seed extract.30 According to our in silico data, 3-hydroxybenzoic acid has a high probability of crossing the BBB by passive diffusion, with a predicted MDCK value of 140 nm s−1. Nonetheless, this molecule was detected in a relatively small number of individuals. Meanwhile, (−)-epicatechin-3′-sulfate, quercetin-3′-sulfate, and quercetin-3′-glucuronide were all detected in the CSF despite having low predicted MDCK values, suggesting that other types of transport mechanisms, besides passive diffusion, may be involved in the transport of these molecules. In fact, the mechanism of transport by quercetin-3′-sulfate is believed to be mediated by OATP4C1 in liver cells, while quercetin-glucuronides have been shown to be aided by the transporter MRP2.31,32 This is in accordance with our results suggesting some degree of CSF saturation for these compounds.
Gentisic acid (2,5-dihydroxybenzoic acid), a (poly)phenol metabolite also derived from phenylalanine and tyrosine metabolism, was detected in blood and CSF. This metabolite had a predicted MDCK value of 15.5 nm s−1, suggesting low permeability across the BBB by passive diffusion. This low transport has been observed in previous studies, together with non-saturable transport, suggesting that this metabolite crosses the membranes by passive diffusion.33 In contrast, in our study, we observed a saturation of the concentrations of this molecule in the CSF despite increasing concentrations in the plasma of various volunteers. Meanwhile, 3′,4′-dihydroxycinnamic acid (caffeic acid) has been detected in previous trials.25 In agreement with such studies, we have found low concentrations of caffeic acid in CSF and only in a small number of individuals, despite detecting significantly high concentrations in plasma. This is in line with the predicted low MDCK value of 10 nm s−1, which suggests low permeability by passive diffusion.
Interestingly, 3′-methoxycinnamic acid-4′-glucuronide (ferulic acid-glucuronide) and 3-(3′-methoxyphenyl)propanoic acid-4′-glucuronide (dihydroferulic acid-glucuronide), two molecules with similar structures, were found in CSF despite both having low predicted MDCK values of 0.19 nm s−1 and 0.2 nm s−1, respectively. We hypothesized that these two molecules might be transported into the CSF by specific cell transporters. However, in our study, these two metabolites were found in a very small number of individuals, so further tests are required to evaluate this hypothesis.
The limited evidence existing for methylxanthine metabolites suggests that they might share the same transport mechanisms as caffeine and be transported across the BBB mainly through passive diffusion, although some transporters might also be involved, mainly those involved in adenosine transport.34 Nevertheless, our results did not detect any obvious saturation in CSF upon increasing plasma concentrations in different volunteers, for any of the methylxanthines quantified in CSF.
Based on the observation that volunteers with higher plasma metabolite levels did not reach higher CSF levels, our findings indicate a phenomenon of saturation kinetics for some (poly)phenol metabolites regarding brain uptake. This saturation can occur due to the limited transporters or receptors available to facilitate brain entry. Saturation kinetics of BBB transport of multiple water-soluble metabolic substrates has been previously described.35–37
However, for (poly)phenols, little is known about their transport across the BBB. In fact, it is unknown which transporters are responsible for the presence of (poly)phenol metabolites in the brain. Our results also showed that all volunteers presented the same saturation concentration. This could be due to the existence of a maximum achievable concentration for CSF so that it cannot increase any further across all individuals; however, further work is needed to confirm this.
Our findings are limited to the study population investigated here, consisting of older subjects with two or more cardiometabolic disorders. As the integrity of the BBB has been shown to be disrupted during the normal aging process,38 as well as in patients with diabetes39 or hypertension,40 the findings described in this article cannot be extrapolated to a younger population or to healthy older individuals without these medical conditions.
Another limitation of this study is that we did not collect information about the dietary habits of the study population, which would have been useful in investigating correlations between (poly)phenol and methylxanthine consumption and the presence of metabolites in CSF and plasma.
In conclusion, the novel identification of a wide range of phenolic metabolites in CSF in the present study shows the possibility for molecules derived from diet to reach the BBB, have a direct action on the brain and thus be of importance in the interaction between brain homeostasis and health.
PubMed indexing
Le Sayec M, Carregosa D, Khalifa K, de Lucia C, Aarsland D, Santos CN, and Rodriguez-Mateos A.
Author contributions
ARM, DA, and CNS designed the research; MLS, DC, KK and CdL conducted the research; MLS and DC analyzed the data and performed statistical analysis; MLS, DC, ARM, CNS and DA wrote the paper. All authors have read and approved the final manuscript.
Conflicts of interest
The authors declare no conflict of interest.
Acknowledgements
The authors thank Anthony Sullivan, Chris Titman, and Neil Loftus from Shimadzu UK Ltd for their support on the LC-MS/MS instrument. The authors also want to acknowledge and thank the participants for their kind effort during the study and all the research staff members at the three sites for their assistance and contribution to the conduction of the trial. Sources of support for the work: This work was funded by a grant from Nasjonalforeningen and Evonik Operations GmbH to D. A. and A. R. M. iNOVA4Health Research Unit (LISBOA-01-0145-FEDER-007344), which is cofunded by Fundação para a Ciência e Tecnologia (FCT)/Ministério da Ciência e do Ensino Superior, through national funds, and by FEDER under the PT2020 Partnership Agreement and European Research Council (ERC) under the European Union's Horizon 2020 research and innovation programme under grant agreement no. 804229, is acknowledged.
References
- D. Del Rio, A. Rodriguez-Mateos, J. P. Spencer, M. Tognolini, G. Borges and A. Crozier, Dietary (poly)phenolics in human health: structures, bioavailability, and evidence of protective effects against chronic diseases, Antioxid. Redox Signal., 2013, 18, 1818–1892 CrossRef CAS PubMed.
- C. G. Fraga, K. D. Croft, D. O. Kennedy and F. A. Tomás-Barberán, The effects of polyphenols and other bioactives on human health, Food Funct., 2019, 10, 514–528 RSC.
- D. Carregosa, R. Carecho, I. Figueira and C. N. Santos, Low-Molecular Weight Metabolites from Polyphenols as Effectors for Attenuating Neuroinflammation, J. Agric. Food Chem., 2020, 68, 1790–1807 CrossRef CAS PubMed.
- P. Hepsomali, A. Greyling, A. Scholey and D. Vauzour, Acute Effects of Polyphenols on Human Attentional Processes: A Systematic Review and Meta-Analysis, Front. Neurosci., 2021, 15, 678769 CrossRef PubMed.
- N. Cheng, L. Bell, D. J. Lamport and C. M. Williams, Dietary Flavonoids and Human Cognition: A Meta-Analysis, Mol. Nutr. Food Res., 2022, 66, 2100976 CrossRef CAS PubMed.
- A. Nehlig, Is caffeine a cognitive enhancer?, J. Alzheimer's Dis., 2010, 20(Suppl. 1), S85–S94 CAS.
- M. Kolahdouzan and M. J. Hamadeh, The neuroprotective effects of caffeine in neurodegenerative diseases, CNS Neurosci. Ther., 2017, 23, 272–290 CrossRef PubMed.
- L. Maia and A. de Mendonça, Does caffeine intake protect from Alzheimer's disease?, Eur. J. Neurol., 2002, 9, 377–382 CrossRef CAS PubMed.
- J. Costa, N. Lunet, C. Santos, J. Santos and A. Vaz-Carneiro, Caffeine exposure and the risk of Parkinson's disease: a systematic review and meta-analysis of observational studies, J. Alzheimer's Dis., 2010, 20(Suppl. 1)), S221–S238 CAS.
- E. Balland, P. Lafenetre and D. Vauzour, Editorial: Polyphenols’ action on the brain, Front. Neurosci., 2022, 16, 947761 CrossRef PubMed.
- R. Franco, A. Oñatibia-Astibia and E. Martínez-Pinilla, Health benefits of methylxanthines in cacao and chocolate, Nutrients, 2013, 5, 4159–4173 CrossRef CAS PubMed.
- S. Kitagawa, Inhibitory effects of polyphenols on p-glycoprotein-mediated transport, Biol. Pharm. Bull., 2006, 29, 1–6 CrossRef CAS PubMed.
- P. Campos-Bedolla, F. R. Walter, S. Veszelka and M. A. Deli, Role of the Blood–Brain Barrier in the Nutrition of the Central Nervous System, Arch. Med. Res., 2014, 45, 610–638 CrossRef CAS PubMed.
- M. Domżalska, W. Wiczkowski, A. Szczepkowska, S. Chojnowska, T. Misztal, F. R. Walter, M. A. Deli, H. Ishikawa, H. Schroten, C. Schwerk and J. Skipor, Effect of Lipopolysaccharide-Induced Inflammatory Challenge on β-Glucuronidase Activity and the Concentration of Quercetin and Its Metabolites in the Choroid Plexus, Blood Plasma and Cerebrospinal Fluid, Int. J. Mol. Sci., 2021, 22(13), 7122 CrossRef PubMed.
- A. Scheepens, K. Tan and J. W. Paxton, Improving the oral bioavailability of beneficial polyphenols through designed synergies, Genes Nutr., 2010, 5, 75–87 CrossRef CAS PubMed.
- I. Figueira, G. Garcia, R. C. Pimpão, A. P. Terrasso, I. Costa, A. F. Almeida, L. Tavares, T. F. Pais, P. Pinto, M. R. Ventura, A. Filipe, G. J. McDougall, D. Stewart, K. S. Kim, I. Palmela, D. Brites, M. A. Brito, C. Brito and C. N. Santos, Polyphenols journey through blood-brain barrier towards neuronal protection, Sci. Rep., 2017, 7, 11456 CrossRef CAS PubMed.
- P. Mena and D. Del Rio, Gold Standards for Realistic (Poly)phenol Research, J. Agric. Food Chem., 2018, 66, 8221–8223 CrossRef CAS PubMed.
- K. Khalifa, A. K. Bergland, H. Soennesyn, K. Oppedal, R. Oesterhus, I. Dalen, A. I. Larsen, T. Fladby, H. Brooker, K. A. Wesnes, C. Ballard and D. Aarsland, Effects of Purified Anthocyanins in People at Risk for Dementia: Study Protocol for a Phase II Randomized Controlled Trial, Front. Neurol., 2020, 11, 916 CrossRef PubMed.
- M. Domínguez-Fernández, Y. Xu, P. Young Tie Yang, W. Alotaibi, R. Gibson, W. L. Hall, L. Barron, I. A. Ludwig, C. Cid and A. Rodriguez-Mateos, Quantitative Assessment of Dietary (Poly)phenol Intake: A High-Throughput Targeted Metabolomics Method for Blood and Urine Samples, J. Agric. Food Chem., 2021, 69(1), 537–554 CrossRef PubMed.
- T. Pallister, M. A. Jackson, T. C. Martin, J. Zierer, A. Jennings, R. P. Mohney, A. MacGregor, C. J. Steves, A. Cassidy, T. D. Spector and C. Menni, Hippurate as a metabolomic marker of gut microbiome diversity: Modulation by diet and relationship to metabolic syndrome, Sci. Rep., 2017, 7, 13670 CrossRef PubMed.
- M. S. Swallah, H. Fu, H. Sun, R. Affoh and H. Yu, The Impact of Polyphenol on General Nutrient Metabolism in the Monogastric Gastrointestinal Tract, J. Food Qual., 2020, 2020, 5952834 CrossRef.
- F. Brial, A. Le Lay, M.-E. Dumas and D. Gauguier, Implication of gut microbiota metabolites in cardiovascular and metabolic diseases, Cell. Mol. Life Sci., 2018, 75, 3977–3990 CrossRef CAS PubMed.
- V. Zabela, C. Sampath, M. Oufir, V. Butterweck and M. Hamburger, Single dose pharmacokinetics of intravenous 3,4-dihydroxyphenylacetic acid and 3-hydroxyphenylacetic acid in rats, Fitoterapia, 2020, 142, 104526 CrossRef CAS PubMed.
- M. E. Obrenovich, C. J. Donskey, I. T. Schiefer, R. Bongiovanni, L. Li and G. E. Jaskiw, Quantification of phenolic acid metabolites in humans by LC-MS: a structural and targeted metabolomics approach, Bioanalysis, 2018, 10, 1591–1608 CrossRef CAS PubMed.
- I. Grabska-Kobylecka, J. Kaczmarek-Bak, M. Figlus, A. Prymont-Przyminska, A. Zwolinska, A. Sarniak, A. Wlodarczyk, A. Glabinski and D. Nowak, The Presence of Caffeic Acid in Cerebrospinal Fluid: Evidence That Dietary Polyphenols Can Cross the Blood-Brain Barrier in Humans, Nutrients, 2020, 12, 1531 CrossRef CAS PubMed.
- K. T. Sachse, E. K. Jackson, S. R. Wisniewski, D. G. Gillespie, A. M. Puccio, R. S. Clark, C. E. Dixon and P. M. Kochanek, Increases in cerebrospinal fluid caffeine concentration are associated with favorable outcome after severe traumatic brain injury in humans, J. Cereb. Blood Flow Metab., 2008, 28, 395–401 CrossRef CAS PubMed.
- X. Lin, X. X. Li, R. Dong, B. Wang and Y. L. Bi, Habitual tea consumption and postoperative delirium after total hip/knee arthroplasty in elderly patients: The PNDABLE study, Brain Behav., 2022, 12, e2612 CrossRef CAS PubMed.
- S. A. Villeda, J. Luo, K. I. Mosher, B. Zou, M. Britschgi, G. Bieri, T. M. Stan, N. Fainberg, Z. Ding, A. Eggel, K. M. Lucin, E. Czirr, J. S. Park, S. Couillard-Després, L. Aigner, G. Li, E. R. Peskind, J. A. Kaye, J. F. Quinn, D. R. Galasko, X. S. Xie, T. A. Rando and T. Wyss-Coray, The ageing systemic milieu negatively regulates neurogenesis and cognitive function, Nature, 2011, 477, 90–94 CrossRef CAS PubMed.
- T. Tarumi and R. Zhang, Cerebral blood flow in normal aging adults: cardiovascular determinants, clinical implications, and aerobic fitness, J. Neurochem., 2018, 144, 595–608 CrossRef CAS PubMed.
- D. Wang, L. Ho, J. Faith, K. Ono, E. M. Janle, P. J. Lachcik, B. R. Cooper, A. H. Jannasch, B. R. D'Arcy, B. A. Williams, M. G. Ferruzzi, S. Levine, W. Zhao, L. Dubner and G. M. Pasinetti, Role of intestinal microbiota in the generation of polyphenol-derived phenolic acid mediated attenuation of Alzheimer's disease β-amyloid oligomerization, Mol. Nutr. Food Res., 2015, 59, 1025–1040 CrossRef CAS PubMed.
- G. Williamson, I. Aeberli, L. Miguet, Z. Zhang, M. B. Sanchez, V. Crespy, D. Barron, P. Needs, P. A. Kroon, H. Glavinas, P. Krajcsi and M. Grigorov, Interaction of Positional Isomers of Quercetin Glucuronides with the Transporter ABCC2 (cMOAT, MRP2), Drug Metab. Dispos., 2007, 35, 1262 CrossRef CAS.
- C. C. Wong, Y. Akiyama, T. Abe, J. D. Lippiat, C. Orfila and G. Williamson, Carrier-mediated transport of quercetin conjugates: Involvement of organic anion transporters and organic anion transporting polypeptides, Biochem. Pharmacol., 2012, 84, 564–570 CrossRef CAS PubMed.
- M. Koljonen, K. Rousu, J. Cierny, A. M. Kaukonen and J. Hirvonen, Transport evaluation of salicylic acid and structurally related compounds across Caco-2 cell monolayers and artificial PAMPA membranes, Eur. J. Pharm. Biopharm., 2008, 70, 531–538 CrossRef CAS PubMed.
- O. Rojo-Poveda, L. Barbosa-Pereira, C. El Khattabi, E. N. H. Youl, M. Bertolino, C. Delporte, S. Pochet and C. Stévigny, Polyphenolic and Methylxanthine Bioaccessibility of Cocoa Bean Shell Functional Biscuits: Metabolomics Approach and Intestinal Permeability through Caco-2 Cell Models, Antioxidants, 2020, 9, 1164 CrossRef CAS PubMed.
- H. Asaba, K.-i. Hosoya, H. Takanaga, S. Ohtsuki, E. Tamura, T. Takizawa and T. Terasaki, Blood—Brain Barrier Is Involved in the Efflux Transport of a Neuroactive Steroid, Dehydroepiandrosterone Sulfate, via Organic Anion Transporting Polypeptide 2, J. Neurochem., 2000, 75, 1907–1916 CrossRef CAS PubMed.
- Q. R. Smith, Carrier-mediated transport to enhance drug delivery to brain, Int. Congr. Ser., 2005, 1277, 63–74 CrossRef CAS.
- W. M. Pardridge, Drug transport across the blood-brain barrier, J. Cereb. Blood Flow Metab., 2012, 32, 1959–1972 CrossRef CAS PubMed.
- I. C. M. Verheggen, J. J. A. de Jong, M. P. J. van Boxtel, E. H. B. M. Gronenschild, W. M. Palm, A. A. Postma, J. F. A. Jansen, F. R. J. Verhey and W. H. Backes, Increase in blood–brain barrier leakage in healthy, older adults, GeroScience, 2020, 42, 1183–1193 CrossRef CAS PubMed.
- M. Bogush, N. A. Heldt and Y. Persidsky, Blood Brain Barrier Injury in Diabetes: Unrecognized Effects on Brain and Cognition, J. Neuroimmune Pharmacol., 2017, 12, 593–601 CrossRef PubMed.
- A. Setiadi, W. S. Korim, K. Elsaafien and S. T. Yao, The role of the blood–brain barrier in hypertension, Exp. Physiol., 2018, 103, 337–342 CrossRef CAS PubMed.
|
This journal is © The Royal Society of Chemistry 2023 |
Click here to see how this site uses Cookies. View our privacy policy here.