DOI:
10.1039/D2FO03060H
(Paper)
Food Funct., 2023,
14, 2260-2269
Associations between oral processing, saliva, and bolus properties on daily glucose excursions amongst people at risk of type-2 diabetes†
Received
11th October 2022
, Accepted 5th February 2023
First published on 6th February 2023
Abstract
Background: A greater time spent with glucose above the normal range (TAR) has been associated with poorer glycaemic control amongst pre-diabetic individuals. Individual differences in oral processing behaviours and saliva amylase activity have been shown to influence glucose responses. Objective: The current study is a preliminary exploration of the associations of oral processing behaviours, bolus characteristics, and salivary amylase activity with the variability in daily glucose excursions within a free-living setting in populations with an elevated risk of type-2 diabetes. Method: Participant oral processing behaviour was derived from video recordings while they consumed a test meal. Post-meal bolus characteristics and saliva properties were measured. Participants were fitted with a continuous glucose monitor (CGM) which monitored blood glucose fluctuation over 7 consecutive free-living days. Dietary intake was recorded through a smartphone application and physical activity was monitored using a wrist worn accelerometer. Results: Participants varied in daily time spent with glucose above the normal range (>7.8 mmol l−1) from 0% to 15%. Greater saliva uptake in the bolus was associated with a higher time spent above the normal range for glucose (β = 0.067 [95% CI = 0.015, 0.120]; p < 0.05), which remained significant after adjustment for dietary carbohydrate intake and BMI. Salivary amylase and saliva flow rate were not significantly associated with the time spent above the normal range. Conclusion: In addition to conventional dietary factors, more research is needed to understand how eating behaviours such as oro-sensory exposure, bolus surface area, and saliva uptake contribute to daily variations in postprandial glucose excursions among populations with a higher risk of developing type-2 diabetes.
1. Introduction
Individuals vary considerably in their blood glucose responses and prolonged periods spent above the normal range of glucose is associated with an increased risk of Type-2 diabetes (T2D).1 The normal glucose range in healthy populations varies between 3.9 mmol l−1 and 7.8 mmol l−1 throughout a day,2 while consistently spending larger proportions of time outside of this glucose range has previously been linked to greater oxidative stress.3–5 In recent years continuous glucose monitor (CGM) devices are growing in popularity as they allow people to measure their glucose continuously over time.6 CGM devices measure changes in interstitial glucose level, which is the glucose found in the fluid between the cells and this provides an approximation of blood glucose. Over the course of a given day, users can track the proportions of glucose variability via Time In Range (TIR), Time Below Range (TBR) and Time Above Range (TAR).7 These metrics give an indication of the quality of glycaemic control which can be used to personalize approaches to managing glucose excursions through changes in diet and physical activity.2
The oral phase of digestion occurs during mastication where the original food structure is broken down, lubricated and softened into a food bolus.8 Saliva plays a key role during oral processing where salivary amylase initiates the breakdown of starch digestion within the food structure, which releases glucose and stimulates initial insulin release.9,10 Salivary amylase levels are known to differ widely between individuals, with between 3–17 copy number variants of the AMY 1 gene.11 To date, the role of salivary amylase on glucose responses in healthy and pre-diabetic individuals have yielded equivocal findings.9,11 Recent findings suggest that a greater salivary amylase activity but not AMY 1 gene was associated with reduced risk of diabetes12 while Higuchi and colleagues showed that AMY 1 gene and high salivary amylase activity were correlated with lowered HbA1C.13 This suggests that variation in salivary amylase activity may interact with oral processing behaviours during the oral phase of digestion14 such as chews per bite and time a food spends in-mouth (i.e. oro-sensory exposure time), to influence postprandial glucose. This is further supported by a recent study from our group which also failed to show a significant role for salivary amylase activity in explaining the observed variance in glucose responses.10
Individual differences in oral processing behaviour are known to influence metabolic responses to the food ingested15 and when combined with variations in saliva secretion during mastication can influence postprandial glucose responses after meals. Recent studies have demonstrated that longer mastication duration led to higher bolus saliva uptake, and this was associated with a higher postprandial glucose and insulin response.10,16 Extending the degree to which a bolus is chewed has been shown to impact postprandial glucose release17–19 and is associated with higher early insulin secretion, which influenced temporal changes in glucose but did not attenuate the blood glucose responses.10,20 The sizes of formed bolus particles have also been shown to influence postprandial response, whereby smaller food bolus particles led to a higher blood glucose response among healthy individuals.21,22
Less is known about whether oral processing behaviours, bolus and saliva properties are associated with habitual daily glucose excursions among individuals at a higher risk of T2D. The impact of oral processing on glucose release over time has been studied acutely in healthy and diabetic populations using a CGM following an acute fixed carbohydrate test meal.23,24 Saito and colleagues monitored blood-glucose variations across 3 meal sessions in healthy participants and showed that eating at a faster pace led to higher glucose release throughout the day.23 The impact of macronutrients on glucose excursion has shown that addition of protein or fat to carbohydrate meal25 and consumption of vegetables before a carbohydrate meal can delay glucose spikes in individuals with diabetes when monitored over CGM.26 It remains unclear how oral processing behaviour, and saliva secretion interact to influence food bolus properties and subsequent glucose changes among individuals who are at risk of T2D.
The current study presents a preliminary exploration of the associations between differences in individual oral processing behaviours, bolus characteristics at swallow, and salivary amylase activity to the variability in daily glucose excursion above normal range in a free-living population with a higher risk of type-2 diabetes over a 7-day period.
2. Materials and methods
2.1. Subjects
Participants (N = 33) were enrolled from the Singapore Multi-Ethnic Cohort.27 All participants were aged between 21–60 years and were classified as being at risk for T2D based on either having elevated HbA1c levels (42–47 mmol mol−1; prediabetes), a BMI in the overweight range (25–30 kg m−2) or an immediate family history of diabetes. Additional recruitment criteria included individuals with healthy dentition and normal mastication abilities. Exclusion criteria included having a known sensitivity to medical-grade adhesives, allergies to ingredients of the study meal, pregnancy or lactation, long-term medication use, and self-reported medical conditions (bleeding disorders, diabetes mellitus of any type, hypertension, thyroid disease, myocardial infarction, cancer, psychosocial impairment). All participants provided informed consent and the study was approved by the National Healthcare Group Domain Specific Review Board (NHG DSRB, Ref: 2018/01220), Singapore. This trial is registered at clinicaltrial.gov (NCT04522063).
2.2. Study procedure
A detailed description of participants and measures are described elsewhere.10 In brief, participants were invited to attend two laboratory visits separated by 10 days. On the first visit (day 1), anthropometric measurements, blood pressure, and blood were collected. This was followed by a mixed meal tolerance test where participants consumed a fixed carbohydrate meal following a standardized protocol.28 Each participant's meal consumption was video recorded to facilitate the analysis of oral processing behaviours. Separately, participants chewed a fixed portion of the carbohydrate meal to the point of swallow and expectorated for bolus analysis. CGM device was fitted to each participant's upper arm to measure glucose levels intermittently and a smartphone application was also provided for participants to record food and beverage consumption for the subsequent 10-days. During a second visit (day 11 onwards) participants had their CGM removed and completed a series of oral processing, bolus, and saliva measures.10 Full details of the methods used for saliva and bolus analysis are reported elsewhere.10
2.3. Test meal and bolus collection
The fixed carbohydrate meal (Egg Rice, Tesco brand, NTUC FairPrice, Singapore) consisting of 50 g available carbohydrates, 6.1 g total fat and 6.8 g protein was served to the participants. The amount of test meal served was 169.5 g (1.73 kcal g−1) and drinking water (250 ml) was given to the participants after meal completion.
For bolus collection, an additional test meal sample (5 g) was provided to participants to ‘chew and spit’ along with a fixed amount of water (25 g) to ensure complete recovery of the sample. The measurement was done in duplicate. Each participant's bolus sample was measured Fiji image analysis29 for number of particles, average particle Feret size (mm2) and total bolus surface area (mm2) at swallow. Total bolus surface area represents the sum of the surface area of all bolus particles at expectoration (mm2). Bolus saliva uptake was estimated at the point of swallow.
2.4. Saliva collection
Using passive drool method, participants were asked to mimic gum chewing with a piece of parafilm (0.29 g, Parafilm M PM996) for 5-minutes. The measurement was conducted in duplicate with a 2-minute rest time in between. Saliva flow rate (g min−1) was calculated from average saliva volume collected. Stimulated salivary amylase activity (U ml−1) were obtained through colorimetric assay (Salimetrics Assay #1-1902, Salimetrics, LLC.) and cell imaging reader (Cytation 5, Winooski, VT, USA).
2.5. Behavioural coding of meal oral processing
A webcam (Logitech HD c310) was used to record oral processing behaviours during the test meal on day 1, and post-hoc behavioural coding analysis was used to derive objective quantitative measures of oral processing behaviours, using a standardized behavioural coding approach described previously.30 The frequencies of ‘bite’, ‘chew’, ‘swallow’ and duration of ‘time where food is spent in mouth’ were coded by a trained coder using an annotation software (ELAN 5.8, Max Planck Institute for Psycholinguistics, The Language Archive, Nijmegen, Netherlands). The coded behavioural data was used to derive a series of oral processing measures including average bite size (g per bite), average eating rate (g min−1), time where food spends in the mouth (seconds). A second trained coder validated the coding data by re-coding a random sample of 10% of the recordings, where coding was deemed acceptable when ≥80% agreement was achieved between coders.
2.6. Continuous glucose monitoring
Participant glucose level was repeatedly measured at 15-minute intervals (i.e., 96 measurements per day per person) using a CGM device (Freestyle Libre; Abbott Diabetes Care, Witney, Oxon, UK) between the two laboratory visits. Although the CGM sensor can store up to 8 hours of glucose measurements, participants were instructed to scan the CGM sensor every 6 hours to transfer the data using a paired reader device to avoid data loss. To avoid behavioural modification from reading the CGM values, the reader device was masked with an opaque sticker. As the glucose measurement from the CGM device is most accurate after the first 3 days of wear,31 it was determined a priori only free-living CGM measurements from day 4 to day 10 (i.e., 7 days) would be used for analysis. In addition, only days with CGM measurements for at least 16 hours were considered as valid and analysed. Glucose values between 3.0 mmol l−1 and 7.8 mmol l−1 were considered as in the normal range. For each participant on each day, their daily percentage of time above range (TAR, >7.8 mmol L−1) and daily average glucose level were calculated using the CGM measurements from the corresponding day.
2.7. Dietary and physical activity assessments
An event-contingent Ecological Momentary Assessment (EMA) was used for dietary recording via a smartphone application (“the app”) developed for this study (Jana Care Inc., Boston, MA, USA). Participants were guided to log all foods and beverages consumed during day 4 to day 10. A text reminder was sent on day 3. Participants logged meal types (i.e., breakfast, lunch, dinner, or snack), food and beverage names, and portion sizes corresponding to what they had eaten.32,33 The food composition database from Health Promotion Board Singapore and a comprehensive database of US foods was used to derive participants’ energy and nutrient intake on each day.32,33 Mean daily energy and carbohydrate intakes were calculated for each participant. Days with reported energy intakes below 500 kcal or greater than 5000 kcal were excluded. Carbohydrate was expressed as a percentage of total energy intake.
Each participant wore an accelerometer (Actigraph – Model wGT3x + BT) on the non-dominant wrist between the two laboratory visits. An open-source R-package ‘GGIR’ (version 1.11-0)34 was used to derive daily time spent in moderate-to-vigorous physical activity (MVPA). Days with less than 16 hours of wear time were excluded in the analysis. Full details of the analyses for dietary and physical activity assessments are reported elsewhere.35
2.8. Statistical analysis
Participants’ baseline characteristics are presented with descriptive statistics (mean ± SD). Participant's 24-hour glucose measurements were summarized and plotted based on participants’ average daily TAR levels (0, 0 to <5%, or 5 to <15%). The selected glucose target range is determined a-priori before conducting the analysis, which is consistent with other analysis of this study.35 Generalised additive model was applied to smooth the time series curves. The Generalized Estimating Equation (GEE) models were used to examine the association of participants’ glucose TAR on each day (the dependent variable) with the oral processing, saliva, and bolus characteristics (fixed factors). TAR levels are taken as continuous variable. An independent within-cluster correlation structure was used, where participants served as clusters (random factor). To avoid compromising the statistical reliability due to the modest sample size, the main analysis examined one fixed factor (univariate) in each GEE models, instead of multiple fixed factors simultaneously. Sensitivity analyses were performed, which included one additional fixed factor as a covariate at a time (i.e., BMI, daily carbohydrate and sugar intakes (% energy), and total daily and daily 10 minutes moderate-to-vigorous physical activity). Covariates such as age, gender, and other demographic variables were not significantly associated with daily glucose percentage above range, hence no further adjustments were made to these variables. To compare the relative association effect sizes by different factors, regression coefficient was also standardized using a published approach36 by adjusting to the standard deviation of the independent and dependent variables. Statistical significance was set at 5% (p < 0.05). Analysis was performed using SPSS (IBM SPSS statistics, version 26) and R statistical software (version 3.6.1). R package ‘geepack’ (version 1.3-2) was used for GEE models.
3. Results
3.1. Participants’ characteristics
A total of 33 individuals, but seven were excluded from the analyses: one did not fulfil the inclusion criteria, three withdrew because of sensor discomfort, and one had surgery before study commencement and two others were removed due to test meal video playback error. The remaining 26 participants provided valid data on CGM (165 days), food intake, oral processing, saliva, and bolus properties, and were thus included in the final analysis.
Baseline characteristics of participants are shown in Table 1. These participants (N = 26, 9 females) had a mean (±SD) age of 45.3 (9.8) years, a BMI of 27.4 (1.8) kg m−2, baseline fasting glucose of 4.8 (0.3) mmol L−1 and an HbA1c level of 5.4 (0.4) %. The average measurement hours per day during the valid days were 23.8 (0.7) hours for the accelerometer and 21.8 (1.8) hours for the glucose monitoring. During the 7-day study period, there were 179 participant-days with valid CGM data, of which 145 participant-days had valid accelerometer data and 142 participant-days had valid dietary data. 118 participant-days had all three types of valid data available simultaneously. On average, the participants provided 5.7 (0.9) days of valid accelerometer data, 6.4 (1.3) days of valid glucose monitoring data, and 6.1 (1.3) days of valid dietary data per person during the 7-day study period for the analysis. The average stimulated saliva flow rate 1.3 (1.0) g min−1 and stimulated α-amylase activity 143.2 (79.3) U mL−1 were within the normal range of previously reported values.37 Participants oral processing behaviours for the test meal were similar to those reported in previously published literature10 for a similar meal and participants for (mean ± SEM) bite size 5.6 (1.3) g per bite, eating rate 35.0 (13.9) kcal min−1, bolus saliva uptake 43.0 (27.1) g, bolus saliva incorporation rate 5.5 (3.6) g min−1, bolus surface area 2105.8 (429.6) mm2, and bolus particle size 1.3 (0.6) mm2.
Table 1 Participants’ characteristics
|
N = 26 (male = 17) |
TAR 0% (n = 7, male = 5) |
TAR 0 to <5% (n = 15, male = 10) |
TAR 5 to <15% (n = 4, male = 2) |
p-Value |
Missing data: 1 participant did not complete saliva measures (n = 25).
Missing data: due to incomplete recovery of bolus samples in 2 participants (n = 24).
|
Age (years) |
45.3 (9.8) |
46.1 (11.8) |
44.1 (9.4) |
48.0 (9.4) |
0.727 |
BMI (kg m−2) |
27.4 (1.8) |
26.5 (1.5) |
27.8 (2.0) |
27.5 (0.6) |
0.304 |
|
HbA1c (%) |
5.4 (0.4) |
5.4 (0.2) |
5.3 (0.4) |
5.8 (0.5) |
0.216 |
Mean glucose/day (mmol L−1) |
4.8 (0.6) |
4.2 (0.5) |
4.9 (0.3) |
5.6 (0.2) |
<0.001* |
TAR/day (%) |
2.4 (3.6) |
0.0 (0.0) |
1.5 (1.1) |
9.8 (3.7) |
<0.001* |
TBR/day (%) |
2.5 (6.7) |
6.5 (11.8) |
1.2 (3.0) |
0.8 (1.1) |
0.183 |
TIR/day (%) |
95.1 (7.2) |
93.5 (11.8) |
97.3 (3.7) |
89.4 (4.3) |
0.043* |
|
Saliva properties
|
Stimulated α-amylase (U mL−1) |
143.2 (79.3) |
107.1 (75.6) |
149.1 (71.6) |
175.2 (112.6) |
0.511 |
Saliva flow rate (g min−1) |
1.3 (1.0) |
1.1 (0.7) |
1.3 (1.1) |
1.7 (0.6) |
0.338 |
|
Bolus characteristics
|
Average particle size (mm2) |
1.3 (0.6) |
1.3 (0.4) |
1.4 (0.7) |
1.1 (0.1) |
0.849 |
Total surface area (mm2) |
2105.8 (429.6) |
2292.6 (431.3) |
2020.7 (371.9) |
2098.2 (634.9) |
0.426 |
Saliva incorporation rate (g min−1) |
5.5 (3.6) |
5.0 (3.3) |
5.0 (4.0) |
7.8 (1.7) |
0.117 |
|
Oral processing behaviours
|
Bite size (g per bite) |
5.6 (1.3) |
5.3 (1.1) |
5.6 (1.4) |
6.1 (1.2) |
0.653 |
Time in mouth (seconds) |
591.0 (266.4) |
717.7 (367.6) |
497.5 (175.5) |
719.8 (272.7) |
0.074 |
Eating rate (kcal min−1) |
35.0 (13.9) |
29.8 (13.5) |
39.7 (14.1) |
26.6 (7.7) |
0.074 |
|
Combined bolus and saliva
|
Saliva uptake (g)b |
43.0 (27.1) |
35.8 (20.2) |
37.6 (27.6) |
71.6 (18.6) |
0.076 |
Based on the inclusion criteria, 25 participants recruited had a BMI of >25 (TAR 0%: n = 6; TAR 0 to <5%: n = 15; TAR 5 to 15%: n = 4). Amongst the 4 participants with elevated BMI of >25, 1 had HbA1c of >6% (or 42 mmol mol−1). Significant differences between the 3 TAR groups were observed for mean glucose (p < 0.01), TAR/day (p < 0.01) and TIR day (p = 0.043). Participants who spent 5 to 15% in TAR had highest mean glucose level of 5.6 (0.2) mmol L−1, lowest TBR of 0.8 (1.1)% and TIR 89.4 (4.3)%.
3.2. Continuous glucose monitoring (CGM) profiles
Fig. 1 summarizes the 24-hour mean glucose level of participants from 7 days CGM data into 3 groups based on the time spent above the normal range (3.0–7.8 mmol l−1) for blood glucose. Most participants (n = 15) spent between 0 and 5% of time per day with glucose above the normal range, while 7 participants had all glucose levels within the normal range. Four participants experienced more frequent fluctuations with glucose level above the normal range for 5 to <15% of time per day.
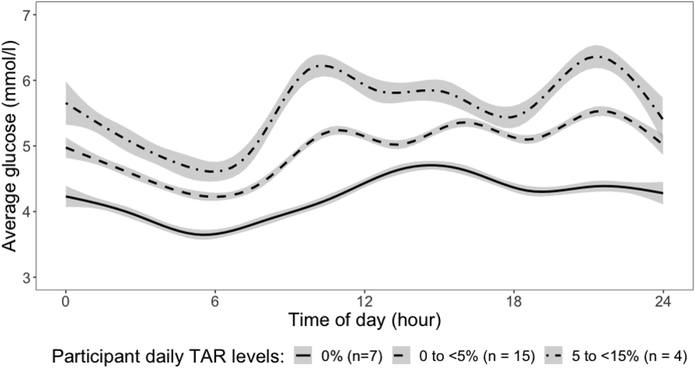 |
| Fig. 1 Average blood glucose levels over 7 days of 24-hour CGM for participants (n = 26; n participant-days = 165) grouped into three levels according to % time per day spent with glucose level above 7.8 mmol l−1: 0%; 0 to <5%; 5 to <15%. TAR: Time-Above-Range (%). The shaded area around each line represents the mean (±SE). | |
3.3. Contributors to time spent above normal range for daily glucose excursion
Table 2 and Fig. 2 show the univariate associations between saliva, bolus, and oral processing characteristics and TAR, the daily percentage of time spent above normal glucose range of 7.8 mmol l−1. The analysis included daily carbohydrate and sugar intakes as covariate as these have direct impact on glucose excursion. Neither movement (physical activity) nor dietary intakes were associated with mean glucose levels. No association was found between stimulated salivary amylase and saliva flow rate with TAR. There was a significant relationship between bolus saliva uptake and TAR, where greater saliva uptake in the bolus is associated with a higher TAR (β = 0.067 [0.015, 0.120]; p = 0.012). No associations were found between saliva, bolus, and oral processing characteristics and TBR (data not shown). TIR was associated with lower salivary amylase stimulation (β = −0.025 [−0.046, −0.004]; p = 0.017) and smaller bite size (β = −1.982 [−3.640, −0.323]; p = 0.019) (data not shown). Bolus saliva uptake remained significantly associated with TAR with consistent effect sizes after controlling for BMI, carbohydrate intake, sugar intake or physical activity (see ESI Tables 1 and 2† for model performance).
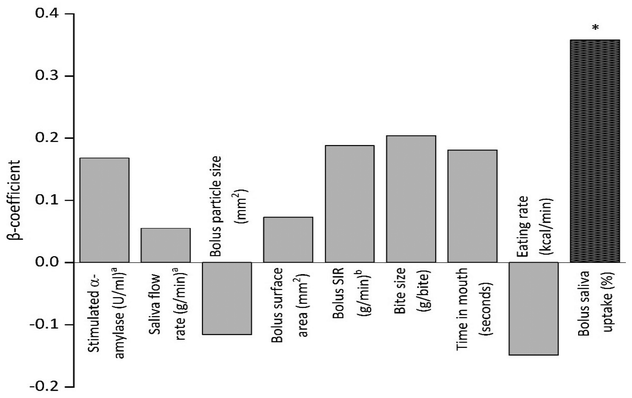 |
| Fig. 2 Standardized associations between saliva, bolus, and oral processing characteristics and daily % Time-Above-Range (glucose level >7.8 mmol l−1) over a 7-day period (N = 26). Positive β-coefficient values represent predictors associated with an increase TAR. *p value < 0.05. aMissing data: 1 participant did not complete saliva measures (n = 25). bMissing data: 2 participants due to incomplete recovery of bolus samples (n = 24). | |
Table 2 Associations between saliva, bolus, and oral processing characteristics with percentage daily Time-Above-Range (TAR) for glucose over a 7-day period
N = 26 |
Variables |
Unstandardized β (95% CI) |
p-Value |
Unstandardized β refers to regression coefficient from the GEE model. *p value <0.05. Positive β-coefficient values represent predictors associated with an increase in TAR. Missing data: 1 participant did not complete saliva measures (n = 25). Missing data: due to incomplete recovery of bolus samples in 2 participants (n = 24). |
Saliva propertiesa |
Stimulated salivary amylase activity (U ml−1) |
0.010 (−0.004, 0.025) |
0.158 |
Saliva flow rate (g min−1) |
0.271 (−0.604, 1.145) |
0.544 |
Bolus characteristicsb |
Average particle size (mm2) |
−0.975 (−2.662, 0.712) |
0.257 |
Total surface area (mm2) |
0.001 (−0.004, 0.006) |
0.770 |
Saliva incorporation rate SIR (g min−1) |
0.280 (−0.067, 0.627) |
0.114 |
Oral processing behaviours (OPB) |
Bite size (g per bite) |
0.761 (−0.373, 1.895) |
0.189 |
Time in mouth (seconds) |
0.003 (−0.005, 0.012) |
0.473 |
Eating rate (kcal min−1) |
−0.050 (−0.171, 0.072) |
0.426 |
Combined bolus and saliva |
Bolus saliva uptake (%)b |
0.067 (0.015, 0.120) |
0.012* |
Results from sensitivity analysis showed that the adjusted associations for bolus saliva uptake with TAR remained significant (β, effect size) when controlled for various covariates: BMI, 0.069 (95% CI: 0.016–0.122, p = 0.011); daily carbohydrate intake, 0.073 (95% CI: 0.017–0.128, p = 0.011); sugar intake, 0.073 (95% CI: 0.020–0.126, p = 0.007); daily 10-minute bout of moderate-to-vigorous physical activity 0.068 (95% CI: 0.008–0.126, p = 0.024); and total daily moderate-to-vigorous physical activity, 0.067 (95% CI: 0.012–0.122, p = 0.017).
4. Discussion
The current preliminary study sought to explore whether there is a relationship between oral processing parameters and time spent above the normal range for blood glucose. We showed that participant bolus saliva uptake was significantly associated with a longer time spent above the normal range for blood glucose, and that this relationship remained significant after controlling for BMI and dietary carbohydrate intake. Other factors such as saliva flow rate and α-amylase activity were not significantly associated with 24-hour glucose excursions.
Bolus saliva uptake was a significant predictor of the percentage of time participants spent ‘above’ the target range for blood glucose. This association remained significant after adjustment for carbohydrate intake, sugar intake, physical activity and BMI. Our study suggests that it is the combined effect of both oral processing behaviours and saliva that links to the differences in glucose variation above normal range. Bolus saliva uptake is a product of a tripartite of factors involved in the oral phase of digestions that includes the rate of eating and time a foods spends in mouth, chewing efficiency to change the surface area of food particles to encourage greater saliva uptake, and the rate of saliva production and incorporation to assist bolus formation and agglomeration before swallow.15 Extended mastication increases the duration a food spends in-mouth (i.e. oro-sensory exposure time), allows more time for saliva uptake and action on the available starch for digestion. In addition, longer chewing duration leads to an increase in the number of food particles that are smaller in size and a larger bolus surface area, which stimulates more saliva production and greater saliva uptake by bolus particles.8,22 Previous studies have reported that starch digestion increases when food particle size reduces and bolus surface area increases,10,38 which stimulates greater amylase activity and early glucose release. Experiments on mixed meal tolerance test demonstrated that increasing mastication supports early glucose release and stimulates insulin secretion,10,16 which suggests that greater bolus saliva uptake could change the kinetics of glucose release and may aid insulin release 8. Although insulin was not included in this study, it is worth noting recent studies have found the association between glucose fluctuation above normal range and insulin resistance35,39 in healthy and at risk population. These preliminary findings merit further experimentation to better understand the contribution of oral processing behaviours and bolus saliva uptake to glucose variations above normal range in pre-diabetic populations.
People vary widely in their habitual eating behaviours and individual differences in oral processing behaviour when consuming a meal can influence both the oro-exposure time and saliva uptake, which in turn could impact insulin releases. In combination, these factors could affect long-term maintenance of blood-glucose levels. It is worth noting that although our finding demonstrated slower eating rate and a higher saliva uptake were significantly associated with higher insulin release but also a longer time spent above the normal range for blood glucose. Previous research has shown that faster eating rate and taking fewer chews per bite has been linked with higher food intake18,40 and a lower satiety response.10,20 The current findings highlight that food textures can directly influence an individual's eating behaviour during a meal, and previous research has shown that texture changes to a meal can influence both eating rate and energy intake.41–43 Chewing for longer will slow eating rate, reduce energy intake and enhance insulin release and post-meal satiety per kcal consumed, but may stimulate a higher glucose trajectory. Further research is needed to better understand how food texture could be used as a natural approach to eating speed and influence food–saliva interactions and glucose response. In addition to food texture, it may also be possible to alter the material properties of foods to change their fracture properties and slow the available surface bolus surface area to alter saliva uptake kinetics and to better support glucose metabolism.44 A recent experiment by R. Janani and colleagues demonstrated how combinations of specific food texture modifications can be used to alter micro-structural patterns of oral processing and eating speed.45 A closer understanding of these changes will create new opportunities to moderate eating behaviours and metabolic response in the future.
Variations in salivary amylase activity were not associated with time spent above normal glucose range, which is in line with findings from previous research.46,47 Salivary amylase levels were within what can be considered a ‘normal’ range within our participant pool, and it is possible that salivary amylase only begins to influence postprandial glucose excursions at the extremes of the distribution, at either high or low levels of activity. Our preliminary findings highlight for the first time the impact of increased bolus saliva uptake on glucose variations, suggesting the importance of considering variations not only in saliva composition but also in bolus surface area, and oral processing behaviours such as oro-sensory exposure time.
Among participants, 15% spent between 5–15% of their time above 7.8 mmol l−1 in their daily glucose excursions. Extended periods of daily glucose excursion above the normal range is indicative of a higher risk of T2D. Earlier studies have reported that fluctuations of blood glucose above the normal range can triggers reactive oxygen species and oxidative stress, leading to endothelial dysfunction and the progression of T2D.3–5,48 In pre-diabetic population, early detection of glucose fluctuations above normal range may serve as a prevention strategy to the development of full-on diabetes. A personalised approach to guiding eating behaviours (e.g. eating pace and saliva secretion during food intake) as part of lifestyle and nutritional strategies could be applied for individuals at a higher risk of T2D, to support efforts to maintain a normal blood glucose levels within the desired range. In the present study we observed associations between natural variations in blood glucose and habitual eating behaviours in a free-living population with a higher risk of T2D. This is significant as much previous research has been conducted under laboratory conditions where participants follow a prescribed oral processing regime, such as being directed to fix the number of chews per bite or eating at a specific speed. Our findings highlight that natural variations in oral processing behaviour can have an impact on glucose control.
A strength of our preliminary study was that participants were free to follow their usual diet, meal patterns, and oral processing behaviour. In addition, we focused on a pre-diabetic population and performed detailed analysis of their oral processing behaviours and bolus and saliva characteristics. The extended duration of CGM profiling at 15-minutes intervals was a strength and this provided an opportunity to assess variations over a prolonged duration of 7-days. The generalizability of our study findings is limited by the loss of data and reduced sample size during the experimental duration, and the use of self-reported diet recall where we applied ecological momentary assessment (EMA) to capture dietary patterns. Self-reported food intake measures are often associated with under reporting and where participants were able to record their food intakes via an online diet application away from the test centre.49 We did not ask participants to record their mealtimes and hence was unable to investigate the associations between circadian changes in glucose and meal timing and carbohydrate loads during meals. We collected data from participants for 10 days and discarded the first 3 days such that only 7 consecutive days were included in the final analysis. Whereas we believe this provided a representative impression of typical variations in blood glucose among a pre-diabetic populations, we recommend that future studies track eating behaviour and glucose variations for an extended period, to observe how these metabolic variations impact metabolic markers of glucose metabolism such as haemoglobin A-1C (HbA1c50).
5. Conclusion
We showed that bolus saliva uptake was associated with daily variations in glucose excursions above the normal range among participants at a higher risk of Type 2 diabetes, under free-living conditions. Our findings suggest the importance of considering eating behaviours in conjunction with dietary factors in the understanding of differences in individual glucose excursions. These findings require further research, and we recommend future intervention studies that evaluate the impact of manipulating oral processing behaviours on postprandial glucose excursions over time.
Abbreviations
BMI | Body mass index |
CGM | Continuous glucose monitor |
EMA | Ecological momentary assessment |
GEE | Generalized estimating equation |
T2D | Type 2 diabetes |
TAR | Time-above-range |
Author contributions
CW, RMVD and CGF study design. ATG and XHC data collection. JY, RMVD, ATG, CGF data analysis and interpretation. ATG and CGF writing. XHC, JY, CW, RMVD, CGF review and edit.
Ethical statement
This study was approved by the National Healthcare Group Domain Specific Review Board (NHG DSRB, Ref: 2018/01220), Singapore.
Clinical trial registration
This trial is registered at clinicaltrial.gov (NCT04522063).
Consent to participate
All participants gave written informed consent and were compensated for their time.
Consent for publication
All authors have seen and approved the final version of the manuscript. The article is the authors’ original work and has not received prior publication and is not under consideration for publication elsewhere.
Availability of data and material
The datasets used and/or analysed during the current study are available from the corresponding author on reasonable request.
Code availability
Software application are available from the corresponding author on reasonable request.
Conflicts of interest
The authors declare that they have no known competing financial interests or personal relationships that could have appeared to influence the work reported in this paper.
Acknowledgements
The authors would like to thank Michelle Choy for her support during the data collection. The study was supported by the Singapore Ministry of Health's National Medical Research Council under its Centre Grant Programme (NMRC/CG/M009/2017_NUH/NUHS). A. T. G. and C. G. F. were supported by the Singapore Biomedical Research Council Food Structure Engineering for Nutrition and Health (Sub-grant Grant No. H18/01/a0/E11, Awarded to PI: Forde, C. G.).
References
- H. Du, D. L. Van Der A and E. J. M. Feskens, Dietary glycaemic index: A review of the physiological mechanisms and observed health impacts, Acta Cardiol., 2006, 61, 393–397 CrossRef.
- A. Advani, Positioning time in range in diabetes management, Diabetologia, 2020, 63, 242–252 CrossRef.
- S. E. Siegelaar, F. Holleman, J. B. Hoekstra and J. H. DeVries, Glucose variability; does it matter?, Endocr. Rev., 2010, 31, 171–182 CrossRef CAS.
- M. Ohara, H. Nagaike, S. Goto, A. Fukase, Y. Tanabe, M. Tomoyasu, T. Yamamoto, T. Hayashi, T. Fukui and T. Hirano, Improvements of ambient hyperglycemia and glycemic variability are associated with reduction in oxidative stress for patients with type 2 diabetes, Diabetes Res. Clin. Pract., 2018, 139, 253–261 CrossRef CAS PubMed.
- Y. Saisho, Glycemic variability and oxidative stress: a link between diabetes and cardiovascular disease?, Int. J. Mol. Sci., 2014, 15, 18381–18406 CrossRef CAS PubMed.
- R. S. Mazze, E. Strock, D. Wesley, S. Borgman, B. Morgan, R. Bergenstal and R. Cuddihy, Characterizing glucose exposure for individuals with normal glucose tolerance using continuous glucose monitoring and ambulatory glucose profile analysis, Diabetes Technol. Ther., 2008, 10, 149–159 CrossRef CAS.
- M. I. Maiorino, S. Signoriello, A. Maio, P. Chiodini, G. Bellastella, L. Scappaticcio, M. Longo, D. Giugliano and K. Esposito, Effects of Continuous Glucose Monitoring on Metrics of Glycemic Control in Diabetes: A Systematic Review With Meta-analysis of Randomized Controlled Trials, Diabetes Care, 2020, 43, 1146–1156 CrossRef CAS.
- M.-F. Hoebler, A. Devaux, C. Karinthi, J.-L. Belleville and C. Barry, Particle size of solid food after human mastication and in vitro simulation of oral breakdown, Int. J. Food Sci. Nutr., 2000, 51, 353–366 CrossRef PubMed.
- A. L. Mandel and P. A. S. Breslin, High endogenous salivary amylase activity is associated with improved glycemic homeostasis following starch ingestion in adults, J. Nutr., 2012, 142, 853–858 CrossRef CAS PubMed.
- A. T. Goh, J. Y. M. Choy, X. H. Chua, S. Ponnalagu, C. M. Khoo, C. Whitton, R. M. van Dam and C. G. Forde, Increased oral processing and a slower eating rate increase glycaemic, insulin and satiety responses to a mixed meal tolerance test, Eur. J. Nutr., 2021, 60(5), 2719–2733 CrossRef CAS PubMed.
- P. Pérez-Ros, E. Navarro-Flores, I. Julián-Rochina, F. M. Martínez-Arnau and O. Cauli, Changes in Salivary Amylase and Glucose in Diabetes: A Scoping Review, Diagnostics, 2021, 11, 453 CrossRef PubMed.
- N. S. Al-Akl, R. I. Thompson and A. Arredouani, Reduced odds of diabetes associated with high plasma salivary α-amylase activity in Qatari women: a cross-sectional study, Sci. Rep., 2021, 11, 11495 CrossRef CAS.
- R. Higuchi, T. Iwane, A. Iida and K. Nakajima, Copy number variation of the salivary amylase gene and glucose metabolism in healthy young Japanese women, J. Clin. Med. Res., 2020, 12, 184 CrossRef PubMed.
- Y. Zhang, Y. Chen and J. Chen, The starch hydrolysis and aroma retention caused by salivary α-amylase during oral processing of food, Curr. Opin. Food Sci., 2022, 43, 237–245 CrossRef CAS.
-
C. G. Forde and M. Stieger, in Oral Processing and Consumer Perception, 2022, pp. 137–186 Search PubMed.
- Y. Zhu and J. H. Hollis, Increasing the Number of Chews before Swallowing Reduces Meal Size in Normal-Weight, Overweight, and Obese Adults, J. Acad. Nutr. Diet., 2014, 114, 926–931 CrossRef PubMed.
- B. A. Cassady, J. H. Hollis, A. D. Fulford, R. V. Considine and R. D. Mattes, Mastication of almonds: effects of lipid bioaccessibility, appetite, and hormone response, Am. J. Clin. Nutr., 2009, 89, 794–800 CrossRef CAS PubMed.
- M. Lasschuijt, M. Mars, C. de Graaf and P. A. M. Smeets, How oro-sensory exposure and eating rate affect satiation and associated endocrine responses—a randomized trial, Am. J. Clin. Nutr., 2020, 111, 1137–1149 CrossRef PubMed.
- X. Jin, S. Lin, J. Gao, E. H.-J. Kim, M. P. Morgenstern, A. J. Wilson, D. Agarwal, Y. Wadamori, Y. Wang and J. Ying, Ethnicity impact on oral processing behaviour and glycemic response to noodles: Chinese (Asian) vs. New Zealander (Caucasian), Food Funct., 2022, 13, 3840–3852 RSC.
- Y. Zhu, W. H. Hsu and J. H. Hollis, Increasing the number of masticatory cycles is associated with reduced appetite and altered postprandial plasma concentrations of gut hormones, insulin and glucose, Br. J. Nutr., 2013, 110, 384–390 CrossRef CAS PubMed.
- V. Ranawana, C. J. Henry and M. Pratt, Degree of habitual mastication seems to contribute to interindividual variations in the glycemic response to rice but not to spaghetti, Nutr. Res., 2010, 30, 382–391 CrossRef CAS PubMed.
- S. Vanhatalo, M. Dall'Asta, M. Cossu, L. Chiavaroli, V. Francinelli, G. D. Pede, R. Dodi, J. Närväinen, M. Antonini and M. Goldoni, Pasta structure affects mastication, bolus properties, and postprandial glucose and insulin metabolism in healthy adults, J. Nutr., 2022, 152, 994–1005 CrossRef PubMed.
- Y. Saito, S. Kajiyama, A. Nitta, T. Miyawaki, S. Matsumoto, N. Ozasa, S. Kajiyama, Y. Hashimoto, M. Fukui and S. Imai, Eating Fast Has a Significant Impact on Glycemic Excursion in Healthy Women: Randomized Controlled Cross-Over Trial, Nutrients, 2020, 12(9), 2767 CrossRef PubMed.
- J. B. Nielsen, C. B. Abild, A. M. Pedersen, S. B. Pedersen and B. Richelsen, Continuous glucose monitoring after gastric bypass to evaluate the glucose variability after a low-carbohydrate diet and to determine hypoglycemia, Obes. Surg., 2016, 26, 2111–2118 CrossRef PubMed.
- K. J. Bell, C. E. Smart, G. M. Steil, J. C. Brand-Miller, B. King and H. A. Wolpert, Impact of fat, protein, and glycemic index on postprandial glucose control in type 1 diabetes: implications for intensive diabetes management in the continuous glucose monitoring era, Diabetes Care, 2015, 38, 1008–1015 CrossRef CAS PubMed.
- S. Imai, M. Fukui, N. Ozasa, T. Ozeki, M. Kurokawa, T. Komatsu and S. Kajiyama, Eating vegetables before carbohydrates improves postprandial glucose excursions, Diabetic Med., 2013, 30, 370–372 CrossRef CAS PubMed.
- K. H. X. Tan, L. W. L. Tan, X. Sim, E. S. Tai, J. J.-M. Lee, K. S. Chia and R. M. van Dam, Cohort Profile: The Singapore Multi-Ethnic Cohort (MEC) study, Int. J. Epidemiol., 2018, 47, 699–699j CrossRef PubMed.
- C. Dalla Man, M. Campioni, K. S. Polonsky, R. Basu, R. A. Rizza, G. Toffolo and C. Cobelli, Two-hour seven-sample oral glucose tolerance test and meal protocol: minimal model assessment of beta-cell responsivity and insulin sensitivity in nondiabetic individuals, Diabetes, 2005, 54, 3265–3273 CrossRef CAS PubMed.
- J. Schindelin, I. Arganda-Carreras, E. Frise, V. Kaynig, M. Longair, T. Pietzsch, S. Preibisch, C. Rueden, S. Saalfeld, B. Schmid, J.-Y. Tinevez, D. J. White, V. Hartenstein, K. Eliceiri, P. Tomancak and A. Cardona, Fiji: an open-source platform for biological-image analysis, Nat. Methods, 2012, 9, 676–682 CrossRef CAS PubMed.
- C. G. Forde, N. van Kuijk, T. Thaler, C. de Graaf and N. Martin, Oral processing characteristics of solid savoury meal components, and relationship with food composition, sensory attributes and expected satiation, Appetite, 2013, 60, 208–219 CrossRef CAS PubMed.
- T. Bailey, B. W. Bode, M. P. Christiansen, L. J. Klaff and S. Alva, The performance and usability of a factory-calibrated flash glucose monitoring system, Diabetes Technol. Ther., 2015, 17, 787–794 CrossRef CAS PubMed.
- University of Minnesota Nutrition Coordinating Center, Food and Nutrient Database, 2016.
- Health Promotion Board Singapore, Food Composition System Singapore, 2011.
- J. H. Migueles, A. V. Rowlands, F. Huber, S. Sabia and V. T. van Hees, GGIR: a research community–driven open source R package for generating physical activity and sleep outcomes from multi-day raw accelerometer data, J. Meas. Phys. Behav., 2019, 2, 188–196 Search PubMed.
- S. H. Park, J. Yao, X. H. Chua, S. R. Chandran, D. S. Gardner, C. M. Khoo, F. Müller-Riemenschneider, C. Whitton and R. M. van Dam, Diet and Physical Activity as Determinants of Continuously Measured Glucose Levels in Persons at High Risk of Type 2 Diabetes, Nutrients, 2022, 14, 366 CrossRef CAS PubMed.
- J. Lorah, Effect size measures for multilevel models: Definition, interpretation, and TIMSS example, Large-Scale Assess. Educ., 2018, 6, 1–11 CrossRef.
- L. Engelen, A. Fontijn-Tekamp and A. v. d. Bilt, The influence of product and oral characteristics on swallowing, Arch. Oral Biol., 2005, 50, 739–746 CrossRef PubMed.
- V. Ranawana, J. A. Monro, S. Mishra and C. J. Henry, Degree of particle size breakdown during mastication may be a possible cause of interindividual glycemic variability, Nutr. Res., 2010, 30, 246–254 CrossRef CAS PubMed.
- R. Dimova, N. Chakarova, G. Grozeva, G. Kirilov and T. Tankova, The relationship between glucose variability and insulin sensitivity and oxidative stress in subjects with prediabetes, Diabetes Res. Clin. Pract., 2019, 158, 107911 CrossRef CAS PubMed.
- P. S. Teo, R. M. van Dam and C. G. Forde, Combined impact of a faster self-reported eating rate and higher dietary energy intake rate on energy intake and adiposity, Nutrients, 2020, 12, 3264 CrossRef PubMed.
- K. McCrickerd, C. M. Lim, C. Leong, E. M. Chia and C. G. Forde, Texture-Based Differences in Eating Rate Reduce the Impact of Increased Energy Density and Large Portions on Meal Size in Adults, J. Nutr., 2017, 147, 1208–1217 CrossRef CAS PubMed.
- C. G. Forde, N. van Kuijk, T. Thaler, C. de Graaf and N. Martin, Texture and savoury taste influences on food intake in a realistic hot lunch time meal, Appetite, 2013, 60, 180–186 CrossRef CAS PubMed.
- P. S. Teo, A. J. Lim, A. T. Goh, J. Y. M. Choy, K. McCrickerd and C. G. Forde, Texture-based differences in eating rate influence energy intake for minimally processed and ultra-processed meals, Am. J. Clin. Nutr., 2022, 116(1), 244–254 CrossRef PubMed.
- D. P. Bolhuis and C. G. Forde, Application of food texture to moderate oral processing behaviors and energy intake, Trends Food Sci. Technol., 2020, 106, 445–456 CrossRef CAS.
- R. Janani, V. W. K. Tan, A. T. Goh, M. J. Y. Choy, A. J. Lim, P. S. Teo, M. Stieger and C. G. Forde, Independent and combined impact of texture manipulation on oral processing behaviours among faster and slower eaters, Food Funct., 2022, 13, 9340–9354 RSC.
- G. Alberti, J. Parada, L. Rodrigo Cataldo, J. Vega, C. M. Aguilera, A. I. Alvarez-Mercado, M. I. Hodgson, A. López, I. Angellotti, A. Gil and J. L. Santos, Glycemic Response after Starch Consumption in Relation to Salivary Amylase Activity and Copy-number Variation of AMY1 Gene, J. Food Nutr. Res., 2015, 3, 558–563 CAS.
- V. M. H. Tan, D. S. Q. Ooi, J. Kapur, T. Wu, Y. H. Chan, C. J. Henry and Y. S. Lee, The role of digestive factors in determining glycemic response in a multiethnic Asian population, Eur. J. Nutr., 2016, 55, 1573–1581 CrossRef CAS PubMed.
- L. Monnier, E. Mas, C. Ginet, F. Michel, L. Villon, J.-P. Cristol and C. Colette, Activation of oxidative stress by acute glucose fluctuations compared with sustained chronic hyperglycemia in patients with type 2 diabetes, J. Am. Med. Assoc., 2006, 295, 1681–1687 CrossRef CAS PubMed.
- J. Macdiarmid and J. Blundell, Assessing dietary intake: who, what and why of under-reporting, Nutr. Res. Rev., 1998, 11, 231–253 CrossRef CAS PubMed.
- E. Feskens, L. Brennan, P. Dussort, M. Flourakis, L. M. E. Lindner, D. Mela, N. Rabbani, W. Rathmann, F. Respondek, C. Stehouwer, S. Theis, P. Thornalley and S. Vinoy, Potential Markers of Dietary Glycemic Exposures for Sustained Dietary Interventions in Populations without Diabetes, Adv. Nutr., 2020, 11(5), 1221–1236 CrossRef PubMed.
Footnotes |
† Electronic supplementary information (ESI) available. See DOI: https://doi.org/10.1039/d2fo03060h |
‡ Current address: Curtin School of Population Health, Curtin University, Kent Street, GPO Box U1987, Perth 6845, WA, Australia. |
§ Current address: The George Washington University, The Milken Institute School of Public Health, 950 New Hampshire Ave NW #2, Washington, DC 20052, United States. |
¶ Current address: Sensory Science and Eating Behaviour, Division of Human Nutrition and Health, Wageningen University and Research, P.O. Box 17, 6700 AA Wageningen, The Netherlands. E-mail: ciaran.forde@wur.nl |
|
This journal is © The Royal Society of Chemistry 2023 |
Click here to see how this site uses Cookies. View our privacy policy here.