Polystyrene nanoplastics target lysosomes interfering with lipid metabolism through the PPAR system and affecting macrophage functionalization†
Received
29th November 2022
, Accepted 13th June 2023
First published on 14th June 2023
Abstract
Nanoplastics (NPs) are currently a main concern for environmental, animal and human health due to their potential to accumulate in different environmental compartments and provoke effects in living organisms. Nevertheless, neither these effects nor the interaction of NPs with the cellular machinery are well characterized, and only scattered information is available. In the present work, we focused on the interaction between NPs and fish cells, both intestinal cells and macrophages, in order to understand which cell organelles are targeted by polystyrene (PS)-NPs and how this could impact cell function. PS-NPs can pass through phospholipid membranes, entering cells via endocytosis, phagocytosis or passive transport. Once internalized, we found that PS-NPs co-localize with lysosomes but not with mitochondria. Moreover, using two types of fluorescent probe (H2DCFDA and DHE) we demonstrated that NPs did not trigger the production of reactive oxygen species (ROS), which was corroborated by the fact that neither the oxidative consumption ratio (OCR) nor the extracellular acidification rate (ECAR) in mitochondrial respiration were altered. RNASeq data revealed clear interference by PS-NPs with lipid metabolism, peroxisomes and PPAR signaling. The M1/M2 balance critically determines tissue homeostasis when exposed to exogenous agents such as microorganisms or pollutants. Thus, the expression of different genes (il1β, tnfα, il6, il10, il12, cox2, mmp9, ppar a, b and g) was further assessed to characterize the macrophage phenotype M1 or M2, induced by PS-NPs. Overall, in this study we demonstrate that PS-NPs co-localize within lysosomes, both in macrophages and in intestinal cells of rainbow trout, but do not trigger ROS production nor alter mitochondrial respiration. In macrophages, PS-NPs modulate polarization towards the M2-like phenotype.
Environmental significance
Small-sized plastic particles have been reported around the planet in all kinds of environments. Nevertheless, studies of the effects of nanoplastics (NPs) on vertebrate species are still scarce, probably because nanoplastics are more difficult to detect and to quantify. Few studies evaluate the impact of nanoplastics in relevant cell types, such as intestinal cells that first encounter the nanoplastic in aquatic organisms or in macrophages relevant to mounting a proper immune response also at the mucosal surfaces. Here we dissect the interaction of PS-NPs with cell machinery, and we show how this accumulation, mainly in the lysosomal compartment, interferes with the lipidic metabolism of macrophages and may modify their functional phenotype to a specific M2-like functional phenotype. We propose that modifications on the tissue microenvironment by nanoplastic accumulation may modulate the functional response of macrophages, thus modifying the global immune response.
|
1. Introduction
Nanoplastics (NPs) are the particle size-fraction under 1000 nm of plastic litter,1 and could potentially be the most hazardous. They have been found in different environments such as oceans, rivers, soils, mountain glaciers and polar ice.2–4 Their nanoscale physicochemical properties allow them to cross biological barriers, including the intestinal wall,5 reaching and accumulating in different tissues such as liver, muscle or brain.6–8 Aquatic organisms are highly exposed to NPs, as the external environment directly bathes internal surfaces. In particular, fish share an intimate relationship between gills, intestine and their water surroundings, which represent an extremely wide surface interacting with the external milieu. For example, the trout gill surface is estimated to be 0.1–0.4 m2 kg−1 body weight, and the salmon digestive tube is estimated to be 0.675 m2 kg−1 body weight.9,10 This pinpoints the digestive tube as the largest organ surface exposed to external media in fish. In addition, there is a short distance between blood and water (e.g., 6 μm on average in the gill lamellae of rainbow trout)9 and between the lumen of the intestine and the lamina propria of the microvillus. This large area and short distance envisage the easy uptake and wide impact of NPs directly on exposed surfaces, as well as subsequent impact on internal tissues. In zebrafish larvae, NPs mainly accumulate in the gut and pancreas after acute exposure by immersion,11 highlighting the gastrointestinal system as one of the main portals of entry of NPs.
The intestinal epithelium consists of a single layer of epithelial cells; thus intestinal cell cultures are excellent models to understand the final fate of NPs inside the cell and to understand how the cell machinery copes with this emergent pollutant.12,13 At a functional level, and in addition to digesting and absorbing nutrients, the intestine is critical for water and electrolyte balance, endocrine regulation of digestion and metabolism, and immunity.14,15 Moreover, the digestive tube is a complex tissue intimately associated with different immune cell types such as intra-epithelial lymphocytes (IEL) and macrophages.16 Accordingly, there is a large population of resident macrophages within the lamina propria of the intestine, which could also suffer the impact of NPs, once these NPs cross the one-cell epithelial barrier. In addition to disturbing the local immune response, this could lead to NPs being transported from the intestine to immune-relevant organs (spleen and head kidney), altering the systemic immune response to pathogens. Previous studies on the effects of NPs on the mammalian immune system reported that NPs were detected in macrophages, indicating an attempt by the host's immune system to clear and remove the NPs.17 Macrophages are dispersed in tissues of both lymphoid and non-lymphoid origin and can adapt their functions in response to signals from their microenvironment.18–20 Relevantly, the immune functions of macrophages can be regulated by lipids, developing into different functional phenotypes depending on the input stimuli. Macrophages take up lipoproteins (e.g. LDL or oxLDL) and cholesterol from the extracellular environment via phagocytosis, macropinocytosis and scavenger receptor-mediated pathways,21 processing them into lysosomes and mitochondria.22 When the excess of lipids and cholesterol is not eliminated from macrophages, it leads to the formation of “foam cells”, the accumulation of which is a characteristic feature observed in some pathologies: for example, during the development of atherosclerotic plaque in humans.23 M1 and M2 are the best characterized macrophage phenotypes, described both in mammals and in fish,24 and associated with specific pro-inflammatory (M1) and anti-inflammatory (M2) states and metabolic profiles.25 M1 macrophages basically rely on glycolytic metabolism, while M2 fuel the immune response through lipid metabolism. It has been previously described that nanomaterials can reprogram macrophage phenotypes.26 Moreover, Merkley and coauthors found that PS-NPs can modify the macrophage polarization status towards a glycolytic metabolic profile.27
Among different manufactured plastic polymers, PS is one of the most commonly fabricated, and is predominantly used in packaging, contributing to a significant fraction of plastic pollution.28 Moreover, PS has been one of the polymers identified in the nano-fraction of the few real environmental samples evaluated to date.2,3 Several studies have reported effects of PS-NPs in fish and have described alterations in the oxidative status, endocrine disruption, dysregulation of energy metabolism, interference with the immune system, gut dysbiosis and behavioral alterations.29,30 However, knowledge of where NPs accumulate within the cell and how this accumulation impacts the cell function is scattered and sometimes controversial. Different studies have reported the impact of PS-NPs on oxidative status,31,32 mitochondrial respiration,27 glucose and lipid metabolism33,34 or apoptosis.32
Oxidative stress and ROS production have been suggested as one of the main mechanisms of NPs effects and are synthesized in large amounts in M1 macrophages to eliminate pathogens.35,36 ROS are byproducts of biological reactions that occur during the processes of respiration in organelles such as mitochondria and peroxisomes, two organelles that interact in a coordinated way in fatty acid and ROS metabolism.37 Fatty acid β-oxidation is a multistep process by which fatty acyl-CoA esters are shortened. However, mitochondria and peroxisomes contain a distinct set of enzymes for each β-oxidation reaction step. The peroxisomal β-oxidation products can only be fully oxidized to CO2 and H2O after they have been shuttled to mitochondria. Oxidative status imbalance has attracted the attention of researchers because excessive ROS production leads to oxidative damage and activates a variety of stress-sensitive signaling pathways in cells. Taking all the above into account, the hypothesis of the present work is that intestinal cells and macrophages attempt to clear PS-NPs using the cellular machinery for lipid uptake and lipid metabolism, and that this could alter normal cell function, potentially affecting macrophage polarization. In this study, we present exhaustive data on the subcellular fate of PS-NPs in two different and functionally distant cell types that are at the forefront of interaction with PS-NPs. We describe the main subcellular organelles targeted by PS-NPs and how the initial steps of an acute exposure to PS-NPs impact cell machinery and specific functions such as lipid metabolism. The resilience of biological systems to environmental perturbation is remarkable, but given that the presence of NPs is extensive in air, water and soil and that NPs can be incorporated in trophic webs, the long-term impact of this still enigmatic contaminant could be extremely important.
2. Materials and methods
2.1. Nanoplastic properties and characterization in exposure media
Two types of NPs were purchased from Bangs Laboratories (Fisher, IN, USA): fluorescently labelled PS-NPs (50 nm size – ref. FSDG001 Dragon Green) and non-labelled PS-NPs (44 nm size – ref. PS02002). The hydrodynamic size and zeta potential were assessed by dynamic light scattering (DLS) (Zetasizer Pro, Malvern) in ultrapure water and cell culture media (DMEM) at 0 and 24 h. A summary of the PS-NPs characterization is presented in ESI† Table S1. Full characterization information in different conditions and corresponding figures were previously published for both the fluorescent PS-NPs11 and the non-labelled PS-NPs.7
2.2. Cell culture
Rainbow trout head kidney macrophages (RT-HKM) were isolated from Oncorhynchus mykiss (109 ± 18 g body weight) following previously described procedures.38 Primary adherent cultures were established in DMEM + GlutaMAX, with 10% heat-inactivated FBS and 100 μg ml−1 Primocin (Invitrogen) at 17 °C and 5% CO2. Experiments for NP uptake, protein and gene expression were performed on day 5 when the macrophages were fully differentiated.
RTgutGC cells were obtained from Dr Carolina Tafalla's lab39 and cultured at 20 °C, without CO2 in L-15 medium with GlutaMAX and supplemented with 10% of FBS and 1% penicillin–streptomycin. Sub-confluent cultures (at an approximate density of 80%) were detached by adding TrypLE Express enzyme (Gibco, cat.12605010) and left to incubate for 5 minutes. Detached cells were split up into new flasks at a 1
:
2 seeding ratio. Experiments for NP uptake and gene expression were performed at 80% confluency.
ZFL cells were cultured as previously11 in DMEM + GlutaMAX, with 10% heat-inactivated FBS and 1% penicillin–streptomycin.
2.3. Cytometry and confocal microscopy
To evaluate the uptake of PS-NPs in cells, RT-HKM, RTgutGC or ZFL cells were incubated in their respective minimal media for 2 h, then a range of concentrations of PS-NPs (0, 0.05, 1, 5, 10, 25, 50 and 100 μg mL−1; see figure legend for specific concentrations) was added to the media and left for 16 h.
To assess whether PS-NP uptake affected the number of lysosomes or mitochondria, cells were treated as stated above and then washed with PBS or HBSS. Cells were then incubated with media containing Lysotracker/Red (75 nM) or Mitotracker Deep Red (25 nM), for 1 h and 45 minutes, respectively, as indicated in the manufacturer's instructions. After treatment and staining, RT-HKM or RTgutGC cells were washed in PBS or HBSS, respectively, and incubated under their appropriate conditions (Section 2.1) with 1 mg ml−1 trypsin (Gibco) for 15 min. This strong trypsinization step aimed to remove PS-NPs attached to the cell surface. Subsequently, two volumes of complete medium were added, and the cells were pelleted by centrifugation at 300×g for 5 min at RT. The pellets were resuspended in PBS or HBSS for flow cytometry (FACSCalibur BD), and 10
000 events were counted. The data was analyzed using FlowJo 10.4 (Leland Stanford University) and plotted with Prism 8.01 (GraphPad Prism).
To obtain spatial information and confirm that the fluorescent PS-NPs were inside the cells, we performed confocal microscopy (Zeiss LSM 700). RT-HKM or RTgutGC were seeded on Ibidi plates (μ-dish 35 mm) and incubated at 17 or 20 °C and 5% or 0% CO2, respectively. The next day, cells at approximately 50–60% confluence were placed in minimal media. PS-NPs at 25 μg ml−1 were added 2 h later and the cells were incubated for 16 h at 17 or 20 °C. Prior to observation in the microscope, the cells were incubated with media containing Lysotracker Red (75 nM) or MitoTracker Deep Red (25 nM), for 1 h and 45 min, respectively, as indicated in the manufacturer's instructions. After staining, the media was replaced by fresh, prewarmed media. The cells were then stained with Hoechst (nuclei) and CellMask Deep Red or WGA (membrane) (Life Technologies). The images were analyzed using Imaris software v8.2.1 (Bitplane) and ImageJ (National Institute of Health, USA).
2.4. Oxygen consumption measurements
Oxygen consumption rate (OCR) was measured using a Seahorse XFp Extracellular Flux Analyzer (Agilent Technologies, Inc), at 21 °C. RT-HKM or RTgutGC cells were plated at 1.5 × 105 cells/well or 4 × 104 cells/well, respectively, and grown for 6 h. Cells were placed in minimal media for 2 h and PS-NPs were then added, at 25 μg mL−1, and left to incubate for 16 h. The cells were assayed in Agilent Seahorse XF DMEM Medium (Agilent Technologies, Inc) supplemented with 25 mM glucose, 1 mM pyruvate and 4 mM glutamine. First, basal measurements of oxygen consumption rate were obtained. Next, oligomycin (2 mM) was injected, followed by sequential injections of FCCP (5 mM) and antimycin A/rotenone (1.25 mM and 1 mM, respectively) to disrupt mitochondrial respiration. Altogether, this injection series allowed for the determination of basal respiration, spare capacity, ATP-linked respiration and maximal respiration.
2.5. Detection of reactive oxygen species
To measure ROS, cells were incubated with 10 μM 2′,7′-dichlorofluorescein diacetate (DCFDAH2; ThermoFisher) or 10 μM dihydroethidium (DHE, Sigma) in the dark at 17 or 21 °C for 30 min in the presence of 25 or 50 μg mL−1 PS-NPs (1 h pre-treatment) and/or doxorubicin (10 μM) (45 min) as a positive control. Cells then were trypsinised as explained in Section 2.2 for cytometry analysis. A probe control was always included in the experimental design, and ROS production (GeoMean Fluorescence Intensity and fold change) was calculated using this control. ROS production was measured by cytometry using a CytoFLEX LX (Beckman Coulter). Viability was monitored by staining the cells in parallel with BD Via-Probe Red Nucleic Acid Stain (VPR) or Propidium Iodide (PI).
2.6. RNA extraction and Q-PCR
RT-HKM or RTgutGC cells were plated to 70% confluence, cultured in minimal media for 2–3 h and then exposed to 25 μg ml−1 PS-NPs (12 h) and subsequently to Poly I:C or LPS (10 μg ml−1, 12 h). Total RNA was extracted using TriReagent (Sigma) following the manufacturer's instructions and RNA yield and quality were determined on a Nanodrop ND-1000 (Thermo Fisher Scientific) and integrity was assessed on an Agilent 2100 Bioanalyzer using an RNA 6000 Nano Lab-Chip kit (Agilent Technologies). For RNASeq analysis, see Section 2.7.
For Q-PCR, RT-HKM and RTgutGC, cells were exposed to 25 or 50 μg ml−1 PS-NPs (16 h). cDNA was synthesized from 1 μg of high-quality total RNA using an iScript cDNA synthesis kit (Bio-Rad). Quantitative real-time PCR (Q-PCR) was performed at 60 °C annealing temperature in a CFX384 touch real-time PCR detection system (Bio-Rad) using an iTaq Universal SYBR Green Supermix kit (Bio-Rad). Each PCR mixture consisted of 5 μl of SYBR green supermix, 0.4 μM of specific primers, 2 μl of diluted cDNA and 2.6 μl of water (Sigma-Aldrich) in a final volume of 10 μl. The reference gene was ef1-α. The dilution factor for all the genes tested was 1
:
10 (ppara, pparb, pparg, tnfa, il1b, il6, il12, cox2, il10, mmp9, cathepsinD, ef1-a, gas7 or b-actin (ESI† Table S1)). Reference gene stability was calculated using NormFinder (Andersen et al., 200440) and the best reference gene (gas7) was selected. Amplification efficiencies for the primers were also calculated (ESI† Table S1). All the samples (N = 3 or 6 per treatment) were run in triplicate, and data were analyzed for individual replicates using the Livak method.41
2.7. RNA Seq
Total RNA was extracted from RT-HKM cells using TriReagent (Sigma-Aldrich), as indicated in Section 2.6, and RNA yield and quality were determined on a Nanodrop ND-1000 (Thermo Fisher Scientific). The samples were quantified with a Qubit® RNA BR Assay kit (Thermo Fisher Scientific) and the RNA integrity was estimated with an RNA 6000 Nano Bioanalyzer 2100 Assay (Agilent). For all samples RIN was between 9 and 10. The RNA-Seq libraries were prepared with KAPA Stranded mRNA-Seq Illumina Platforms Kit (Roche) following the manufacturer's recommendations. Briefly, 500 ng of total RNA was used for poly-A fraction enrichment with oligo-dT magnetic beads, following mRNA fragmentation. Strand specificity was achieved during the second strand synthesis performed in the presence of dUTP instead of dTTP. The blunt-ended double-stranded cDNA was 3′ adenylated and Illumina platform compatible adaptors with unique dual indexes and unique molecular identifiers (Integrated DNA Technologies) were ligated. The ligation product was enriched with 15 PCR cycles. The size and quality of the libraries were assessed in a High Sensitivity DNA Bioanalyzer assay (Agilent). The libraries were sequenced on NovaSeq 6000 (Illumina) in paired-end mode with a read length of 2 × 101 bp, following the manufacturer's protocol for dual indexing. Image analysis, base calling and quality scoring of the run were processed using the manufacturer's software Real Time Analysis (NovaSeq 6000 RTA 3.4.4). Illumina readings were mapped against the O. mykiss reference genome (Omyk_1.0) using aligner STAR version 2.7.8a42 with ENCODE parameters. Annotated genes were quantified using RSEM version 1.3.043 with default parameters, using release 104 of O. mykiss EN-SEMBL annotation. Differential expression analysis was performed with the limma v3.42.3 R package, using TMM normalization. The voom function44 was used to estimate the mean–variance relationship and to compute observation-level weights. The voom-transformed counts were used to fit the linear model and contrasts were extracted. Genes with an adjusted p-value < 0.05 and absolute fold change |FC| > 1.5 were considered to be differentially expressed (DEG). Those genes were used to perform a functional enrichment analysis with gprofiler2 v0.1.8.45 To use human databases as a reference, the gorth function from gprofiler2 was used to translate gene identifiers between organisms (orthologous gene mappings are based on the information retrieved from the Ensembl database). Additionally, gene set enrichment analysis (GSEA) was performed with a list of genes pre-ranked with a t-statistic and human Reactome database, using the R package fgsea v1.12.0.
2.8. Statistics
Results are expressed as mean ± standard deviation and statistical data analysis was undertaken using GraphPad Prism 7.0. The data was first checked for normality using the Shapiro–Wilk test. Afterwards, different treatments were compared using one-way ANOVA or a t-test depending on the experimental design.
3. Results and discussion
3.1. Uptake and accumulation of PS-NPs in macrophages and intestinal cells
In this study, we used an in vitro approach to study the interaction of PS-NPs with the cell machinery using two relevant cell types: primary macrophages isolated from head kidney, RT-HKM, and intestinal cells, RTgutGC. The internalization of PS-NPs was very efficient and dose dependent in both cell types (Fig. 1a–d). However, some differences were observed regarding the total fluorescent signal intensity (MFI). RTgutGC cells (Fig. 1c) were able to internalize more fluorescent PS-NPs than RT-HKM (Fig. 1a) at a given dose (e.g. at 25 μg ml−1 HKM they reach an MFI value of 1000 while RTgutGC reach 4000). The uptake of PS-NPs in zebrafish liver cells (ZFL) has been described in detail previously11 and, when compared to RT-HKM and RTgutGC cells, ZFL showed an intermediate capacity of internalization (at 25 μg ml−1 we observed an MFI of 3000; Fig. 1e). The positive fluorescent cell percentage reaches around 100% at 5 μg ml−1 in all cell types (Fig. 1b–f). Differences in uptake could be attributed to functional differences among cell types, as RTgutGC is a cell line derived from epithelial intestinal cells, which have the main function of incorporating substances present in the intestinal lumen. No associated toxicity was found at the assayed doses and time in any of the cell types (ESI† Fig. S1). PS-NP toxicity has been reported at higher doses in different cell lines: up to 75 μg ml−1 in ZFL,11 up to 200 μg ml−1 in Caco-246 and up to 500 μg ml−1 in THP-1.47
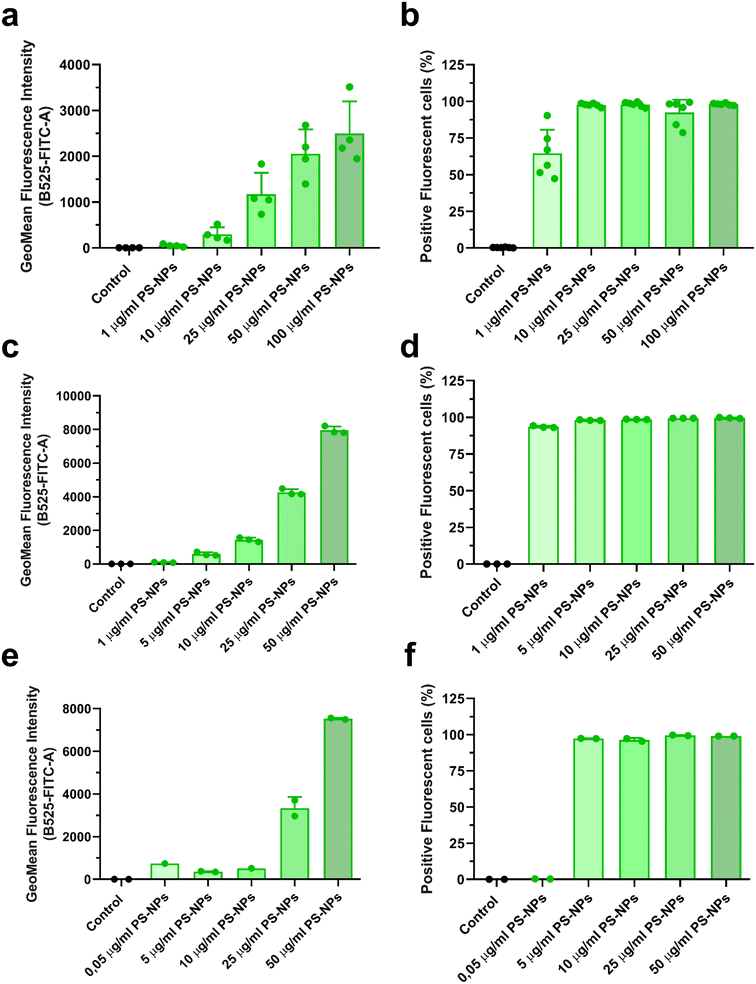 |
| Fig. 1 PS-NP uptake by HKM, RTgutGC and ZFL cells. Uptake of fluorescent PS-NPs by HKM (a and b), RTgutGC (c and d) and ZFL (e and f). The mean fluorescence intensity (MFI) of the dose–response (a, c and e) and the percentage of positive cells (b, d and f) are shown. Cells were incubated for 16 h with fluorescent PS-NPs at different concentrations (0.05, 1, 10, 25, 50 and 100 μg ml−1). Data show the mean ± standard deviation (SD). Each dot represents an independent experiment (n = 3 to 6). | |
3.2. PS-NPs localize within the lysosomes in intestinal cells and macrophages and modulate lysosomal abundance
We investigated the intracellular fate of PS-NPs in RTgutGC and RT-HKM by using flow cytometry to obtain quantitative information and confocal microscopy to gain morphological/dimensional information, and combining fluorescent dyes that specifically label lysosomes and mitochondria (see Section 2.2). As shown in Fig. 2, PS-NPs accumulated within the cytosol in both RT-HKM and RTgutGC cells, predominantly co-localizing with lysosomes (Fig. 2, right-hand panels, white arrows). Confocal images corroborated the differential ability of macrophages and intestinal cells to take up PS-NPs in a qualitative way, with RTgutGC cells showing higher PS-NP accumulation (Fig. 2). We used MitoTracker to investigate co-localization of PS-NPs in the mitochondria, as this organelle has been claimed to be one of the main targets of PS-NPs, where they would interfere with the mitochondrial function by inducing the production of ROS.48 However, we did not observe any trace of co-localization (Fig. 3, composites 1 and 2). To further characterize the interaction of PS-NPs with both lysosomes and mitochondria, we used cytometry to assess whether incubation with PS-NPs would modify the abundance of either of the two organelles. We detected a clear and significant increase in the fluorescent intensity due to LysoTracker and no modification of the fluorescent signal due to MitoTracker (Fig. 4a), indicating an increase in lysosomes after cellular exposure to PS-NPs, but no changes in mitochondria. Cytometry data was consistent with microscopy, where strong staining of mitochondria and more discrete and less abundant staining of lysosomes were observed in HKM (Fig. 2 and 3). The gating strategy and representative flow cytometry plots of HKM treated with fluorescent PS-NPs and stained with Mito- and LysoTracker are shown in Fig. 4b. Moreover, the confocal images shown in Fig. 3 also point to an increase in the number of lysosomes (LysoTracker) when the cells were treated with PS-NPs, compared with LysoTracker staining in control cells (Fig. 2).
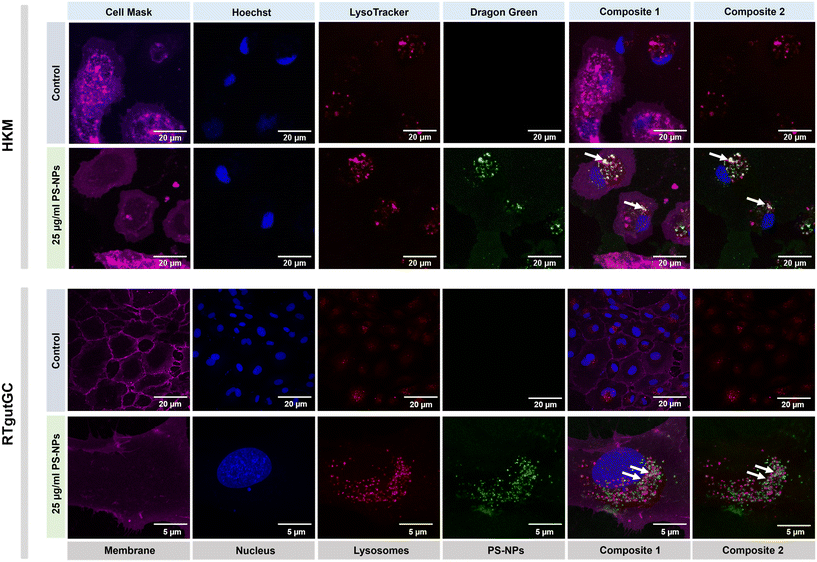 |
| Fig. 2 Confocal imaging of HKM (upper panel) and RTgutGC (lower panel) showing the internalization and co-localization of fluorescent PS-NPs, after 16 h of incubation at 25 μg ml−1 PS-NPs. Magenta corresponds to cell mask staining membranes; blue to Hoechst (nucleus); red to LysoTracker (lysosomes) and green to dragon green (PS-NPs). White arrows point to co-localization of PS-NPs and lysosomes. | |
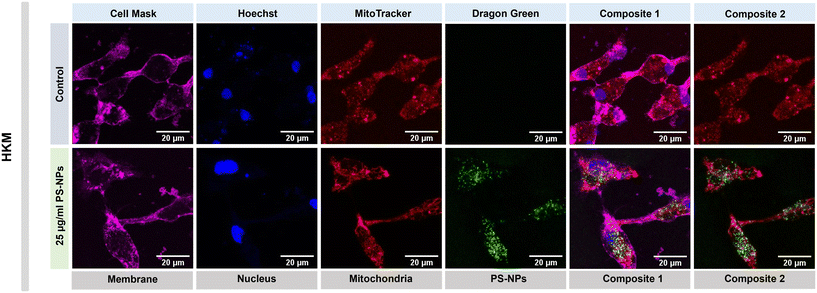 |
| Fig. 3 Confocal imaging of fluorescent PS-NP uptake by HKM and organelle staining. Representative confocal microscopy imaging of HKM showing the internalization and co-localization of fluorescent PS-NPs, after 16 h of incubation at 25 μg ml−1 PS-NPs. Magenta fluorescence corresponds to cell mask staining membranes; blue to Hoechst (nucleus); red to MitoTracker (mitochondria) and green to dragon green (PS-NPs). | |
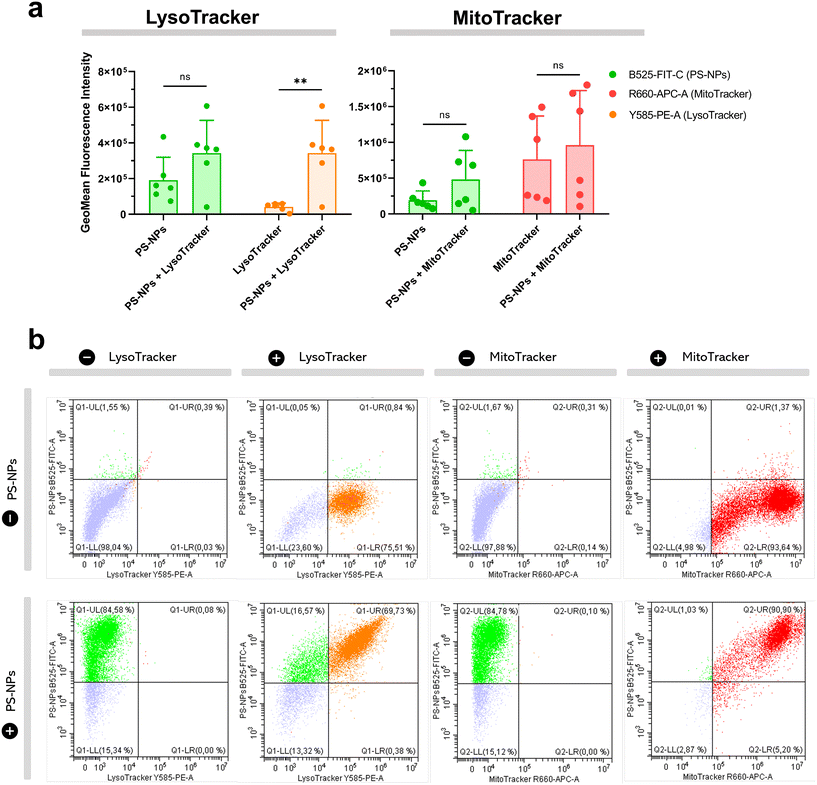 |
| Fig. 4 Uptake of fluorescent PS-NPs by HKM and organelle staining. HKM were stained with Lyso- and MitoTracker and exposed to 25 μg ml−1 PS-NPs for 16 h. (a) Differences in GeoMean fluorescence intensity between treatments (PS-NPs, PS-NPs + Lyso/MitoTracker). Data show the mean ± standard deviation and significant differences were assessed using a t-test with Welch's correction and indicated as: *p < 0.05, **p < 0.01. Lyso/MitoTracker and Lyso/MitoTracker + PS-NPs channels (FIT-C, APC-A, PE-A). (b) Representative dot plots of PS-NPs and Lyso/MitoTracker staining of HKM by cytometry. | |
3.3. Mitochondrial respiration rates and ROS production in intestinal cells and head kidney macrophages are not altered by PS-NPs
To explore the direct interaction of PS-NPs with mitochondria, we assessed mitochondrial respiration in RT-HKM and RTgutGC. The mitochondrial oxygen consumption rate (OCR) was assessed by using a Seahorse XFp extracellular flux analyzer (Fig. 5). OCR results revealed that respiration was similar for RT-HKM (Fig. 5a and b) and RTgutGC (Fig. 5c and d) cells treated or not with 25 μg mL−1 of PS-NPs. A more detailed analysis of mitochondrial respiration revealed that basal respiration, spare capacity, ATP-linked respiration and maximal respiration were unaffected by the presence of PS-NPs in both RT-HKM (Fig. 5b) and RTgutGC cells (Fig. 5d).
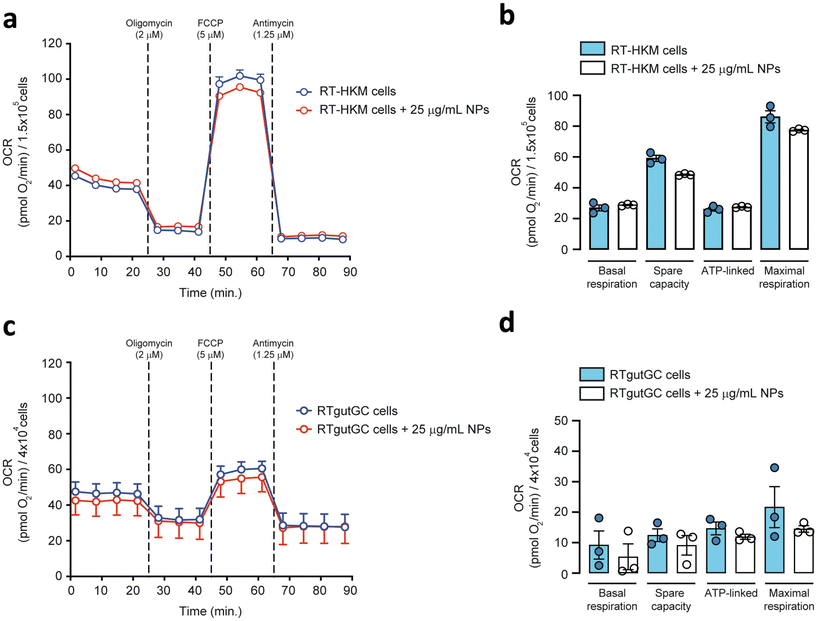 |
| Fig. 5 Mitochondrial respiration rates of HKM and RTgutGC cells. Representative pharmacological profile of oxygen consumption rate (OCR) from RT-HKM (a) and RTgutGC (c) cells. Respiratory capacities of RT-HKM (b) and RTgutGC (d) cells (calculated from Fig. 5a and c, respectively). Data are presented as the mean ± SEM with n = 3 in each group. | |
This result contrasts with previous studies that reported modifications in mitochondrial respiration in human liver and lung cells after exposure to PS-NPs, at similar doses and times to those used in this study.49,50 This could be explained by the fact that not every cell type may confront the same external factor in an identical way and may need different doses or times to reach the damage threshold. To further evaluate whether PS-NPs would alter cell oxidative status, even if not through direct interaction with mitochondria, we measured ROS production using a combination of fluorescent chemical probes and cytometry. This approach allowed us to evaluate ROS production and cell viability in parallel, to discard uncontrolled side effects. We assessed ROS levels using a DHE probe for HKM and a DCFDH2 probe for RTgutGC (see Section 2.4). As a positive control for ROS production, we used doxorubicin as a consistent ROS-inducing stimulus.51 It is worth mentioning that we also carried out these assays with TBH as a positive control for ROS production, but this compound did not consistently induce ROS in both intestinal cells and macrophages (data not shown). We set up a specific ROS assay for each cell line (DHE for HKM and DCFDH2 for RTgutGC) using cytometry, and viability was monitored in parallel, staining the cells with PI or VPR. We did not detect ROS production due to PS-NP treatment in HKM or in RTgutGC cells (Fig. 6a and b, respectively). These results demonstrated that PS-NPs did not trigger the production of ROS, under present conditions, which was corroborated by the fact that there were no alterations in the oxidative consumption ratio (OCR) or extracellular acidification rate (ECAR), either in HKM or in intestinal RTGut cells.
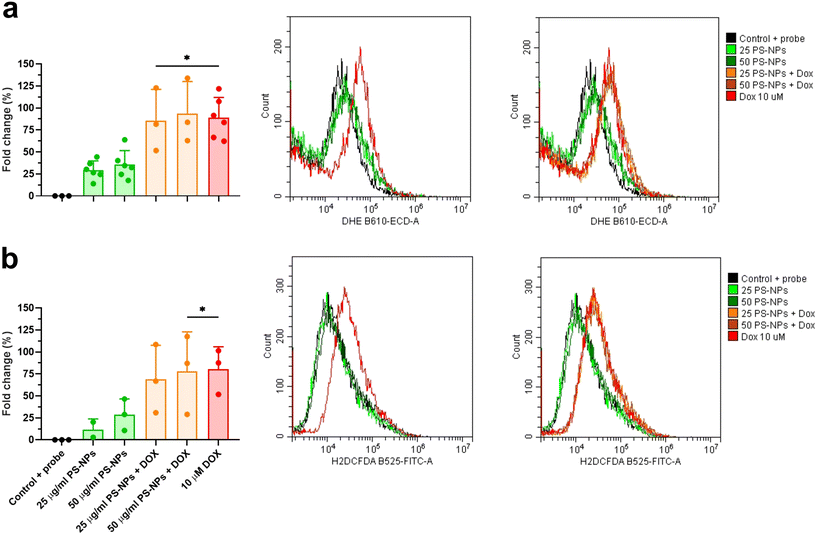 |
| Fig. 6 ROS production in RT-HKM and RTgutGC. DHE (RT-HKM) and H2DCFDA (RTgutGC). Fold change of mean fluorescence intensity (MFI) with respect to control plus probe in HKM (a) and RTgutGC (b). Histograms represent the GeoMean fluorescence intensity in each channel with only PS-NPs exposure (25 μg ml−1 or 50 μg ml−1) and PS-NPs plus 10 μM doxorubicin (DOX). Significant differences with the control were evaluated through one-way ANOVA and represented as: *p < 0.05. | |
3.4. PS-NPs modulate the lipid metabolism in macrophages through the PPAR system and promote an M2-like polarization state
Given that RTgutGC cells and HKM showed the same type of interaction with the cell machinery after PS-NP treatment, we decided to further investigate the functional response of macrophages to PS-NP exposure by using RNASeq. We planned an experimental design that would allow us to characterize both the macrophage response to PS-NPs and the response to PS-NPs combined with immune stimuli, by using bacterial (LPS) and viral (Poly(I:C)) mimics. Macrophages showed high transcriptional plasticity when they were treated with both bacterial and viral-like stimuli, presenting large changes in gene expression: 5354 altered genes in LPS-stimulated macrophages and 7365 genes in Poly(I:C)-treated macrophages (adjusted p = 0.05). However, the pre-exposure of macrophages to PS-NPs did not have any significant impact on the global gene expression response to subsequent LPS or Poly(I:C) stimulation, as similar percentages of up- and down-regulated genes and functional categories were observed (Fig. 8a). Moreover, when macrophages were treated with PS-NPs alone, we observed significant up- or down-regulation in only 93 genes, most of them coding for enzymes or transporters involved in lipid transport and catabolism (Fig. 7 and 8b). When we analyzed the functional enrichment in this gene list using either Reactome or KEGG databases, we observed functional categories related to lipid catabolism, peroxisome and PPAR signaling as the most significatively enriched (Fig. 8b). As PS is a neutral chemically inert polymer of lipophilic nature, it is possible that PS-NPs are detected by cells as lipid entities, therefore activating the cell machinery responsible for lipid catabolism. As previously stated, macrophages can be functionally divided into at least two different phenotypes, – a pro-inflammatory phenotype (M1) and an anti-inflammatory phenotype (M2), but this main characterization has begun to be updated with new subsets of functional phenotypes influenced by the microenvironment. For example, macrophages infiltrated in tumors have a specific functional profile related to this microenvironment.18 Moreover, it has been described that nanomaterials can also modify the macrophage polarization status.52 M1 macrophages are mainly characterized by a high expression of pro-inflammatory cytokines, glycolytic metabolism, ROS production and PPARα, while M2 macrophages are characterized by low expression of pro-inflammatory cytokines, high expression of anti-inflammatory cytokines, lipidic metabolism (FA oxidation), no ROS production and Arg2 and PPARβ expression (see the summary in ESI† Table S3). Our RNASeq data indicates a clear induction of FA oxidation associated also with changes in the expression of the PPAR signaling module (Fig. 8). As can be seen in Fig. 9, a Q-PCR profiling of trout macrophages after PS-NP exposure shows that pro-inflammatory cytokines (e.g. il1b, il12 or tnfa) were not up-regulated while the main anti-inflammatory cytokine, il-10, was up-regulated. The expression of pparβ was also up-regulated, while pparγ was down-regulated and no pparα expression was detected. Odegaard et al. showed that disruption of PPARγ in myeloid cells impaired M2 macrophage activation,53 supported by the data of Wu and coauthors, where a PPAR-γ antagonist favors the M1 phenotype.54 This modulation of PPAR-γ could influence macrophage polarization, as well as affecting lipid metabolism. The expression of cathepsinD and mmp9 was also up-regulated in macrophages after PS-NP treatment. The MMP9 protein is involved in the breakdown of the extracellular matrix in normal physiological processes, as well as in pathological processes and mmp9 expression is associated with M2 polarization.55 Cathepsin D is a ubiquitous lysosomal protease that degrades proteins in lysosomal compartments and inactivates different chemokines, such as macrophage inflammatory protein-1 alpha (Chemokine (C–C motif) ligand (CCL) 3), CCL4 and CCL21.56 The expression of cathepsinD has been associated with the M1 phenotype (ESI† Table S3). On the other hand, the RNASeq data showed up-regulation of ccl25b (Fig. 7), which has been related to the polarization of macrophages from M1 to M2 phenotype in a tumor microenvironment (TME).18 Moreover, the CCR9–CCL25 chemokine axis also strongly correlates with the up-regulation activity of MMP proteins,57 which matches the up-regulation of mmp9 gene expression found in the present study (ESI† Table S3). The anti-inflammatory profile, together with induced FA oxidation could suggest that trout macrophages exposed to PS-NPs polarized towards a M2-like phenotype, but we observe some important differences in this trend, such as down-regulation of pparγ and the increase in ccl25 and cathepsinD expression. Due to these significant differences, we propose that PS-NPs induce a specific polarization state associated with PS-NP exposure as a distinct microenvironment.
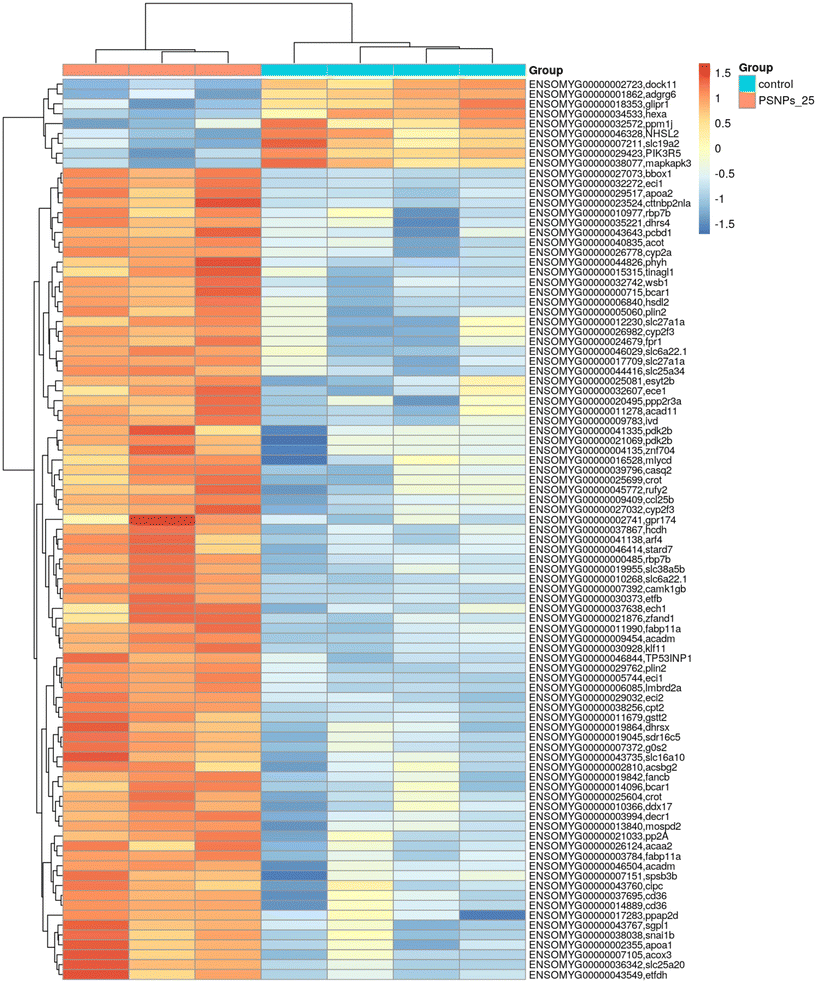 |
| Fig. 7 Transcriptome analysis showing the effects of PS-NPs (25 μg ml−1) on RT-HKM. The heatmap represents the transcript abundance of all DEGs. | |
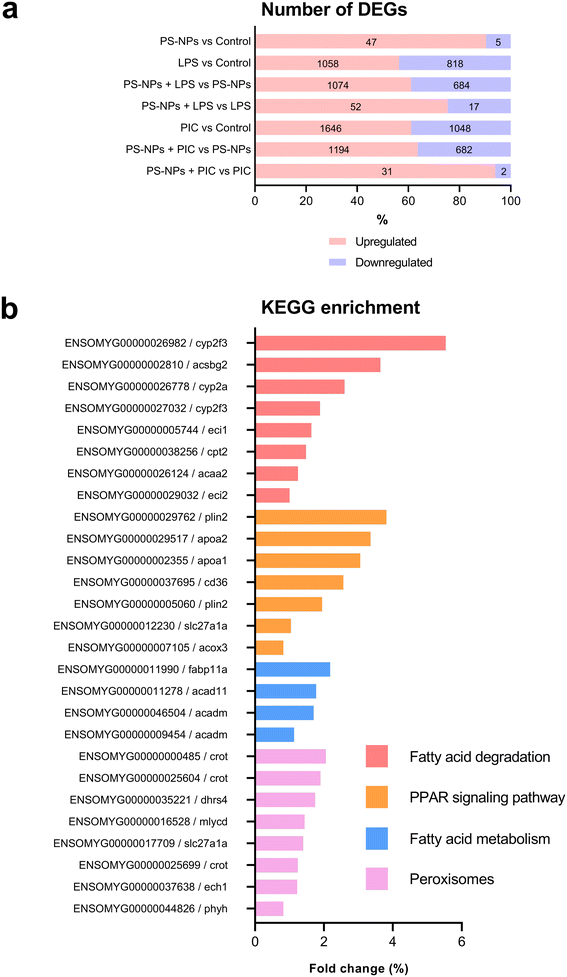 |
| Fig. 8 Transcriptome analysis of the effects of PS-NPs and PS-NPs combined with immune stimuli on HKM. (a) Bar chart showing the total amount of DEGs (up- or down-regulated) under different treatments with LPS and poly(I:C). (b) Main affected pathways and genes after PS-NP exposure. The Y-axis represents the genes and pathways, and the X-axis the fold change. | |
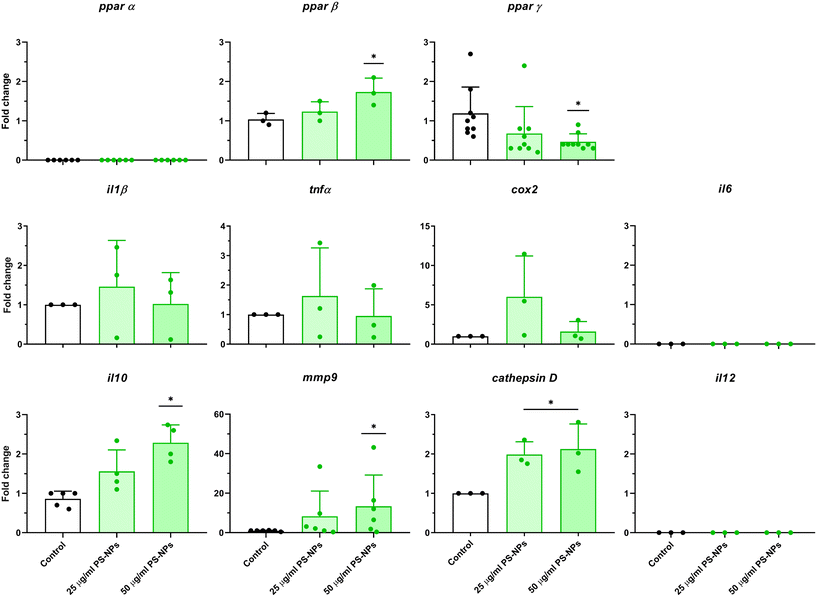 |
| Fig. 9 Analysis of gene expression in HKM after PS-NPs treatment. Cells were incubated with PS-NPs (25 μg ml−1 and 50 μg ml−1) for 16 h. gas7 was used as a reference gene for PPARs, il6, il10, il12 and mmp9. β-Actin was used as a reference gene for cox2, tnfa, il1b and cath d. Data show fold change mean ± standard deviation (SD) and significant differences with the control are represented as: *p < 0.05. | |
Concluding remarks
PS-NPs directly interact with lysosomes, as shown by their co-localization as well as the increase in lysosomes after exposure to PS-NPs. Lysosomes have a key lipid sorting function derived from their ability to process and sort exogenous and endogenous lipids, and the presence of PS-NPs in this organelle could impact lipid metabolism. Moreover, as shown by RNASeq data, PS-NPs significantly alter the expression of transcripts related to lipid metabolism, as well as to PPAR pathways. PPARs play a relevant role in intracellular lipid metabolism by up-regulating the expression of enzymes involved in the conversion of fatty acids into acyl-coA esters. Even though our data show no trace of the impact of PS-NPs in mitochondrial function, they could be affecting mitochondria in an indirect way, as suggested by changes in the expression of peroxisomal receptors. We might hypothesize that the lipophilic nature of PS-NPs could suggest that they are somehow partially confounded by lipid substances inside the cell, either by their own nature or by the biomolecules that might be bound to the NPs, and therefore activate the cellular machinery and pathway related to lipid metabolism.
Our data show a series of gene expression changes in macrophages after exposure to PS-NPs. However, they do not fully match the previously characterized M1 and M2 macrophage phenotypes, although key characteristics from both profiles are displayed. As the macrophagic phenotype is determined by their microenvironment, which is highly dependent on the lipidic substrates, we believe that a specific macrophage phenotype is attained after PS-NP exposure.
Author contributions
IB, RS, MG, AG and NR performed the experiments; NR designed the experiments and IB, MG, RS, AG, MT and NR analysed the data; RS, NR, BM and AC analysed the RNASeq data; AQ provided reagents and machinery for the Seahorse experiments; NR wrote the manuscript draft; NR conceived ideas and NR and MT oversaw the research. All authors were involved in discussions and contributed to the final writing of the manuscript.
Conflicts of interest
The authors declare that they have no known competing financial interests or personal relationships that could have appeared to influence the work reported in this paper.
Acknowledgements
This research was supported through Plan Nacional de Investigación: RTI2018-096957-B-C21 MINECO/FEDER and 2017-SGR-695 (AGAUR) to NR, and PID2020-114977RB-I00 to AQ and PT17/0009/0019 (ISCIII/MINECO and FEDER) to AEC. MT is supported by a Ramon y Cajal contract (ref. RYC2019-026841-I) and IB is supported by a PhD grant from Generalitat de Catalunya (2018FI_B_00711).
References
- N. B. Hartmann, T. Hüffer, R. C. Thompson, M. Hassellöv, A. Verschoor, A. E. Daugaard, S. Rist, T. Karlsson, N. Brennholt, M. Cole, M. P. Herrling, M. C. Hess, N. P. Ivleva, A. L. Lusher and M. Wagner, Environ. Sci. Technol., 2019, 53, 1039–1047 CrossRef CAS PubMed.
- A. T. Halle, L. Jeanneau, M. Martignac, E. Jardé, B. Pedrono, L. Brach and J. Gigault, Environ. Sci. Technol., 2017, 51, 13689–13697 CrossRef PubMed.
- M. Llorca, A. Vega-Herrera, G. Schirinzi, K. Savva, E. Abad and M. Farré, J. Hazard. Mater., 2021, 404, 124022 CrossRef CAS PubMed.
- D. Materić, R. Holzinger and H. Niemann, Sci. Total Environ., 2022, 846, 157371 CrossRef PubMed.
- P. Stapleton, AIMS Environ. Sci., 2019, 6, 367–378 CAS.
- X. Fan, X. Wei, H. Hu, B. Zhang, D. Yang, H. Du, R. Zhu, X. Sun, Y. Oh and N. Gu, Chemosphere, 2022, 288, 132607 CrossRef CAS PubMed.
- I. Brandts, M. Cánovas, A. Tvarijonaviciute, M. Llorca, A. Vega, M. Farré, J. Pastor, N. Roher and M. Teles, Environ. Res., 2022, 212, 113433 CrossRef CAS PubMed.
- M. Teng, X. Zhao, C. Wang, C. Wang, J. C. White, W. Zhao, L. Zhou, M. Duan and F. Wu, ACS Nano, 2022, 16, 8190–8204 CrossRef CAS PubMed.
-
E. O. Koppang, A. Kvellestad and U. Fischer, Fish mucosal immunity: gill, in Mucosal Health in Aquaculture, 2015, pp. 93–133, DOI:10.1016/B978-0-12-417186-2.00005-4.
- E. D. Stevens, G. N. Wagner and A. Sutterlin, J. Fish Biol., 1999, 55, 517–526 CrossRef CAS.
- I. Brandts, M. Garcia-Ordoñez, L. Tort, M. Teles and N. Roher, Environ. Sci.: Nano, 2020, 7, 2410–2422 RSC.
- M. Minghetti, C. Drieschner, N. Bramaz, H. Schug and K. Schirmer, Cell Biol. Toxicol., 2017, 33, 539–555 CrossRef CAS PubMed.
- M. Revel, C. Roman and A. Châtel, Ecotoxicology, 2021, 30, 421–430 CrossRef CAS PubMed.
- W. H. Karasov and A. E. Douglas, Compr. Physiol., 2013, 3, 741–783 Search PubMed.
- R. K. Buddington, A. Krogdahl and A. M. Bakke-Mckellep, Acta Physiol. Scand., Suppl., 1997, 638, 67–80 CAS.
- D. Parra, F. E. Reyes-Lopez and L. Tort, Front. Immunol., 2015, 6, 354 Search PubMed.
- K. Kenesei, K. Murali, Á. Czéh, J. Piella, V. Puntes and E. Madarász, J. Nanobiotechnol., 2016, 14, 55 CrossRef PubMed.
- C. Zhang, M. Yang and A. C. Ericsson, Front. Immunol., 2021, 12, 620510 CrossRef CAS PubMed.
- D. M. Mosser and J. P. Edwards, Nat. Rev. Immunol., 2008, 8, 958–969 CrossRef CAS PubMed.
- P. J. Murray and T. A. Wynn, Nat. Rev. Immunol., 2011, 11, 723–737 CrossRef CAS PubMed.
- I. Tabas and K. E. Bornfeldt, Circ. Res., 2016, 118, 653–667 CrossRef CAS PubMed.
- J. Yan and T. Horng, Trends Cell Biol., 2020, 30, 979–989 CrossRef CAS PubMed.
- A. Remmerie and C. L. Scott, Cell. Immunol., 2018, 330, 27–42 CrossRef CAS PubMed.
- M. Forlenza, I. R. Fink, G. Raes and G. F. Wiegertjes, Dev. Comp. Immunol., 2011, 35, 1246–1255 CrossRef CAS PubMed.
- A. S. Wentzel, J. J. E. Janssen, V. C. J. de Boer, W. G. van Veen, M. Forlenza and G. F. Wiegertjes, Front. Immunol., 2020, 11, 152 CrossRef CAS PubMed.
- X. Miao, X. Leng and Q. Zhang, Int. J. Mol. Sci., 2017, 18, 336 CrossRef PubMed.
- S. D. Merkley, H. C. Moss, S. M. Goodfellow, C. L. Ling, J. L. Meyer-Hagen, J. Weaver, M. J. Campen and E. F. Castillo, Cell Biol. Toxicol., 2022, 38, 31–41 CrossRef CAS PubMed.
- W. P. de Haan, A. Sanchez-Vidal, M. Canals and N. S. S. Party, Mar. Pollut. Bull., 2019, 140, 523–535 CrossRef CAS PubMed.
- C. Barría, I. Brandts, L. Tort, M. Oliveira and M. Teles, Mar. Pollut. Bull., 2020, 151, 110791 CrossRef PubMed.
- H. Jacob, M. Besson, P. W. Swarzenski, D. Lecchini and M. Metian, Environ. Sci. Technol., 2020, 54, 4733–4745 CrossRef CAS PubMed.
- Z. Liu, Y. Huang, Y. Jiao, Q. Chen, D. Wu, P. Yu, Y. Li, M. Cai and Y. Zhao, Aquat. Toxicol., 2020, 220, 105420 CrossRef CAS PubMed.
- H. Wu, J. Guo, Y. Yao and S. Xu, Fish Shellfish Immunol., 2022, 125, 1–8 CrossRef CAS PubMed.
- N. R. Brun, P. van Hage, E. R. Hunting, A.-P. G. Haramis, S. C. Vink, M. G. Vijver, M. J. M. Schaaf and C. Tudorache, Commun. Biol., 2019, 2, 382 CrossRef PubMed.
- W. Lai, D. Xu, J. Li, Z. Wang, Y. Ding, X. Wang, X. Li, N. Xu, K. Mai and Q. Ai, J. Hazard. Mater., 2021, 419, 126454 CrossRef CAS PubMed.
- A. Covarrubias, V. Byles and T. Horng, Cell Res., 2013, 23, 984–985 CrossRef CAS PubMed.
- Q. Hu, H. Wang, C. He, Y. Jin and Z. Fu, Environ. Pollut., 2021, 269, 116075 CrossRef CAS PubMed.
- M. Fransen, C. Lismont and P. Walton, Int. J. Mol. Sci., 2017, 18, 1126 CrossRef PubMed.
- N. Roher, A. Callol, J. V. Planas, F. W. Goetz and S. A. MacKenzie, Innate Immun., 2011, 17, 16–28 CrossRef CAS PubMed.
- A. Kawano, C. Haiduk, K. Schirmer, R. Hanner, L. E. J. Lee, B. Dixon and N. C. Bols, Aquacult. Nutr., 2011, 17, e241–e252 CrossRef.
- C. L. Andersen, J. L. Jensen and T. F. Ørntoft, Cancer Res., 2004, 64, 5245–5250 CrossRef CAS PubMed.
- K. J. Livak and T. D. Schmittgen, Methods, 2001, 25, 402–408 CrossRef CAS PubMed.
-
A. Dobin and T. R. Gingeras, Comment on “TopHat2: accurate alignment of transcriptomes in the presence of insertions, deletions and gene fusions” by Kim et al., DOI:10.1101/000851.
- B. Li and C. N. Dewey, BMC Bioinf., 2011, 12, 323 CrossRef CAS PubMed.
- C. W. Law, Y. Chen, W. Shi and G. K. Smyth, Genome Biol., 2014, 15, R29 CrossRef PubMed.
- L. Kolberg, U. Raudvere, I. Kuzmin, J. Vilo and H. Peterson, F1000Research, 2020, 9(ELIXIR), 709 Search PubMed.
- J. Domenech, M. de Britto, A. Velázquez, S. Pastor, A. Hernández, R. Marcos and C. Cortés, Biomolecules, 2021, 11, 1442 CrossRef CAS PubMed.
- I. Florance, N. Chandrasekaran, P. M. Gopinath and A. Mukherjee, Ecotoxicol. Environ. Saf., 2022, 238, 113612 CrossRef CAS PubMed.
- L. Rubio, I. Barguilla, J. Domenech, R. Marcos and A. Hernández, J. Hazard. Mater., 2020, 398, 122900 CrossRef CAS PubMed.
- S. Lin, H. Zhang, C. Wang, X.-L. Su, Y. Song, P. Wu, Z. Yang, M.-H. Wong, Z. Cai and C. Zheng, Environ. Sci. Technol., 2022, 56, 12483–12493 CrossRef CAS PubMed.
- S. E. Lee, Y. Yi, S. Moon, H. Yoon and Y. S. Park, Metabolites, 2022, 12, 897 CrossRef CAS PubMed.
- T. A. Jacinto, G. S. Meireles, A. T. Dias, R. Aires, M. L. Porto, A. L. Gava, E. C. Vasquez, T. M. C. Pereira, B. P. Campagnaro and S. S. Meyrelles, Biol. Res., 2018, 51, 33 CrossRef PubMed.
- D. Reichel, M. Tripathi and J. M. Perez, Nanotheranostics, 2019, 3, 66–88 CrossRef PubMed.
- J. I. Odegaard, R. R. Ricardo-Gonzalez, M. H. Goforth, C. R. Morel, V. Subramanian, L. Mukundan, A. R. Eagle, D. Vats, F. Brombacher, A. W. Ferrante and A. Chawla, Nature, 2007, 447, 1116–1120 CrossRef CAS PubMed.
- H. Wu, X. Ni, Q. Xu, Q. Wang, X. Li and J. Hua, J. Gastroenterol. Hepatol., 2020, 35, 1998–2008 CrossRef CAS PubMed.
- E. Zajac, B. Schweighofer, T. A. Kupriyanova, A. Juncker-Jensen, P. Minder, J. P. Quigley and E. I. Deryugina, Blood, 2013, 122, 4054–4067 CrossRef CAS PubMed.
- M. Wolf, I. Clark-Lewis, C. Buri, H. Langen, M. Lis and L. Mazzucchelli, Am. J. Pathol., 2003, 162, 1183–1190 CrossRef CAS PubMed.
-
H. Mir and S. Singh, CCL25 signalling in the tumor environment, in Tumor environment. The role of chemokines – Part B, 2021 Search PubMed.
|
This journal is © The Royal Society of Chemistry 2023 |