Development of a quantitative structure–activity relationship model for predicting quantum yield of hydroxyl radical generation from organic compounds†
Received
29th September 2022
, Accepted 23rd November 2022
First published on 24th November 2022
Abstract
Organic compounds are capable of generating hydroxyl radicals (˙OH) through their excited triplet states in natural water. It is of significance to reveal the underlying mechanism of the generation and obtain the generation quantum yield of ˙OH from organic compounds for better understanding of its involvement in indirect photochemical processes in the environment. In this study, the ˙OH quantum yields (Φ˙OH) of 20 organic compounds were determined by photochemical experiments. The calculated Φ˙OH values for the selected organic compounds vary from (1.2 ± 0.39) × 10−5 to (7.2 ± 0.16) × 10−4. A quantitative structure–activity relationship (QSAR) model for log
Φ˙OH was developed and the established model was proven to have a proper goodness of fit, robustness, and predictive ability. The QSAR model was successfully used to predict the Φ˙OH value of organic pollutants. Mechanistic interpretation showed that the electron distribution and the electronegativity of organic compounds are the most important factors that determine the generation of ˙OH. The results are helpful for understanding the generation mechanism of ˙OH from organic compounds and also provide insights into the generation of ˙OH from dissolved organic matter in natural water.
Environmental significance
Chromophoric organic pollutants can produce ˙OH under sunlight irradiation in natural fresh water and marine water. But it is difficult to experimentally determine the quantum yield of ˙OH produced by a wide variety of organic pollutants. In the study, the Φ˙OH values for organic compounds with different structures were determined and a QSAR model was constructed for predicting the Φ˙OH values and for mechanism interpretation of the generation of ˙OH in sunlit natural water. The results of this study are important for comprehending the generation of ˙OH from organic pollutants and understanding its environmental chemical processes in natural water.
|
1. Introduction
The hydroxyl radical (˙OH) is a powerful oxidant with a redox potential of 2.8 eV.1 It has an unselective character and ability to react with recalcitrant contaminants, and it plays a major role in the degradation of organic matter in natural waters and in sewage treatment facilities.2 The steady-state concentration of ˙OH ([˙OH]ss) in natural waters is very low due to its high reactivity, and the ˙OH is difficult to be quantified directly by conventional monitoring techniques. It is therefore necessary to use the reaction of probes with ˙OH to determine the concentration of ˙OH in natural waters. Commonly used chemical probes include benzene, terephthalate, benzoic acid, and so on.3–5 By using these probes, the researchers estimated that the production rate and [˙OH]ss in lakes ranged from 10−12 to 10−10 M s−1 and 10−17 to 10−15 M, respectively.6,7
In natural fresh water and marine water, dissolved organic matter (DOM) consists of many organic molecules from natural sources, in which molecular structures are still poorly characterized due to their structural complexity.8 Besides DOM, chromophoric organic pollutants can also produce ˙OH under sunlight irradiation, such as triclosan9 and fluoroquinolone antibiotics;10 the information on the quantum yield of the photo-generation of ˙OH from organic small molecules and DOM is the basis for estimating its environmental concentration, and the generation of ˙OH is determined by the molecular structures of the organic pollutants and the organic molecules in DOM. However, on one hand, it is difficult to experimentally determine the quantum yield of ˙OH produced by a wide variety of organic pollutants; and on the other hand, the structure of natural DOM is complex and it differs when from different sources.5,6,11
A quantitative structure–activity relationship (QSAR) model is extensively applied to predict a specific endpoint, such as the physicochemical properties, environmental behavior parameters, and toxicological parameters of compounds based on their molecular structure information.12,13 Zhao et al. (ref. 14) established a QSAR model which can predict the quantum yield of generation of 1O2 from DOM-analogs. Li et al. (ref. 1) established a QSAR model predicting the rate constants for the reaction of the hydroxyl radical with 18 organophosphate esters (OPEs) in the UV/H2O2 process. Thus, it is possible to establish a QSAR model to predict the quantum yield and reveal the generation mechanism of ˙OH from organic compounds.
In this study, a multiple linear regression-QSAR (MLR-QSAR) model was established to predict the quantum yield of the generation of ˙OH from organic compounds. Seventeen organic compounds with different molecular structures were selected as the training set of the QSAR model and a validation set with 3 organic compounds was used to check the predictive competence of the constructed QSAR model. These small molecular analogs have different structures, such as aromatic ketones and quinones, which could represent the chromophore groups in DOM as it has been proved that DOM contains chromophores such as benzene rings, carbon groups and carboxyl groups.15 The mechanism of the generation of ˙OH from organic compounds was also revealed. The results of the study are important for comprehending the generation of ˙OH from DOM and organic pollutants in natural water.
2. Materials and methods
2.1 Chemical substances
Naphthalene (99%), acetophenone (98%), 2-chlorohydroquinone (85%), gallic acid (99%), coumarin (98%), 1,4-benzoquinone (99%), benzophenone (99%), 3-methoxyacetophenone (99%), 4-chloro-4′-hydroxybenzophenone (98%), trans-cinnamic acid (98%), 2-acetonaphthone (99%), 1,4-naphthoquinone (99%), dibenzoyl (98%), 7-hydroxycoumarin (98%), fenofibric acid (99%), riboflavin (98%), and 2,4-dihydroxybenzophenone (99%) were purchased from J&K Scientific Ltd. (Beijing, China). Benzene (98%) and duroquinone (97%) were obtained from Sigma-Aldrich (St. Louis, MO, U.S.A.). Biacetyl (99%) and 2-hydroxy-4-methylbenzaldehyde (98%) were purchased from Tokyo Chemical Industry (Tokyo, Japan). The others are shown in Text S1 in the ESI.‡
2.2 Light irradiation experiments
The experiments were carried out by using an XPA-7 merry-go-round photochemical reactor (Xujiang Technology Co, Nanjing) equipped with quartz tubes, and the temperature of the reaction system was kept at 25 ± 1.0 °C. The solutions were irradiated by using a 500 W medium-pressure mercury lamp. The light intensity was determined by using a TriOS-RAMSES spectroradiometer (TriOS, GmbH, Germany), and is shown in Fig. S1.† Irradiation below 290 nm was cut off with a filter to simulate sunlight. Benzene was used as a probe for detecting the production rate of ˙OH. The original concentrations of benzene and targeted pollutants were 3 mM and 10 mg L−1, respectively. The irradiation time of targeted pollutants is 50 minutes for 2,4-dihydroxybenzophenone, 150 minutes for 7-hydroxycoumarin, and 100 minutes for all the other pollutants. Three samples were irradiated in parallel. One mL of each sample was taken from each of the quartz tubes at a selected time interval and injected into a brown liquid vial. Then the concentration of the target compounds was quantified by high performance liquid chromatography (HPLC), as detailed below.
2.3 Analytical methods
Table 1 shows the structures of organic compounds. The ultraviolet-visible (UV-vis) absorption spectra of the samples were obtained by using a UV-vis spectrophotometer (U-2900, Shimadzu Scientific Instruments, Japan). The UV-vis absorption spectra of all small organic molecules are shown in Fig. S2.† A Shimadzu LC-20A HPLC system (Shimadzu, Kyoto, Japan) equipped with a UV-vis detector and an Ultimate™ AQ-C18 column (250 mm × 4.6 mm, 5 μm, Welch Materials, Maryland, USA) was used to monitor and quantify the concentrations of phenol. Phenol was detected at a wavelength of 210 nm at 30 °C. The mobile phase was methanol and water with a ratio of 35
:
65 and the flow rate was 0.7 mL min−1.
Table 1 Chemical structures of the organic compounds
Name |
Structure |
Name |
Structure |
Represents the chemicals of the training set.
Represents the chemicals of the validation set.
|
Naphthalenea |
|
4-Chloro-4′-hydroxybenzophenonea |
|
Acetophenonea |
|
Trans-cinnamic acida |
|
2-Chlorohydroquinonea |
|
2-Acetonaphthonea |
|
Gallic acida |
|
2-Hydroxy-4-methylbenzaldehydea |
|
7-Hydroxycoumarina |
|
Dibenzoyla |
|
1,4-Benzoquinonea |
|
Biacetyla |
|
Benzophenonea |
|
1,4-Naphthoquinonea |
|
3-Methoxyacetophenonea |
|
Coumarina |
|
Duroquinonea |
|
2,4-Dihydroxybenzophenoneb |
|
Riboflavinb |
|
Fenofibric acidb |
|
2.4 Calculation of the quantum yield of the generation of ˙OH
The efficiency of photon utilization is expressed by using the quantum yield (Φ).16 The quantum yield of hydroxyl radical generation was calculated as follows: | 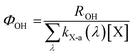 | (1) |
where kX-a (λ) (einstein M−1 s−1 nm−1) is the specific rate of light absorption of the photosensitizer X; [X] (mol L−1) is the concentration of the photosensitizer; R˙OH (mol L−1 s−1) represents the production rate of ˙OH; kX-a (λ) is defined as follows:17,18 | 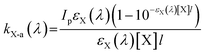 | (2) |
where Ip (einstein·s−1 cm−2) represents the intensity of incident light; εX (λ) (M−1 cm−1) represents the molar absorption coefficient of the photosensitizer; l (cm) represents the optical path length, and the method of measurement is described in Text S2.† Only the initial conversion rate of the probe molecule was considered in the calculation of the ˙OH quantum yield, resulting in the initial formation rate of the transient species. Therefore, in order to maintain the consistency of the data, only the initial absorbance of the organic compounds was considered in the calculation process.19
Benzene can react with ˙OH to produce phenol, with a yield of 0.70.20,21 Therefore, the amount of ˙OH and the production rate were determined by using a chemical probe (benzene). The production rate of ˙OH can be calculated with the following equation:
|  | (3) |
where
k˙OH is the second-order rate constant of benzene with ˙OH (7.8 × 10
9 M
−1 s
−1);
7R˙OH is the production rate of ˙OH (M s
−1); [˙OH] and [Benzene] are the concentrations of ˙OH and benzene in the system (mol L
−1). The initial concentration of benzene was 3 mM.
The [˙OH]ss is computed by using the following equation:
| 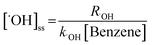 | (4) |
2.5 Establishment and evaluation of the QSAR model
The training set consisting of seventeen organic compounds was used to develop the QSAR model, which is forecasting the quantum yield of ˙OH production. To obtain reasonable geometric structures of these compounds, the molecular configuration was optimized using the Gaussian 16 program suite22 at the B3LYP/6-31+G (d, p) level.23,24 The analysis of the water solvent effect was carried out with the integral equation formalism of the polarizable continuum model (IEFPCM).1,25 DRAGON descriptors were extracted using DRAGON software (verion 7.0), in which the constant values were eliminated after acquiring the DRAGON descriptors. In addition, quantum chemical descriptors that characterize molecular interactions (e.g. electrostatic interactions, hydrogen bonds, and van der Waals' forces) were also included in the descriptor pool.12
MLR was adopted to obtain the appropriate molecular structure descriptors by SPSS software (SPSS 19.0) for establishing a QSAR model. Before building the model, the Φ˙OH value was log-transformed in order to obtain a normal-distributed dataset. The best model needs to satisfy the following criteria:26 (1) least number of molecular descriptors, with the number of compounds in the training set/the number of descriptors exceeding 5; (2) a high value of the coefficient of determination R2, generally greater than 0.7; (3) a variable inflation factor (VIF) less than 10. The VIF represents the multi-collinearity of the predictor variables.
The established QSAR model was validated for its internal and external predictive performance according to the guidance of the Organization for Economic Co-operation and Development (OECD).12,26 In order to estimate the fitness, robustness, and predictive capacity for the developed model, the coefficient of determination (R2), the root mean square error (RMSE), the leave one out cross-validated correlation coefficient (QLOO2) as well as the bootstrap method (QBOOT2) for model evaluation were calculated. The applicability domain (AD) of the model was characterized based on the Euclidean distance method with AMBIT Discovery (version 0.04) software.
3. Results and discussion
3.1 Quantum yield of the generation of ˙OH from small organic molecules
The formation of phenol was detected using HPLC in the solutions containing target compounds and benzene, which indicated that ˙OH was produced during the irradiation of these compounds. The relationship between the phenol concentrations generated by each organic compounds and time is shown in Fig. S3 in the ESI.† The Φ˙OH, R˙OH, and [˙OH]ss were calculated by using eqn (1), (3), and (4), respectively, and the results of Φ˙OH are shown in Table 2, and R˙OH and [˙OH]ss are shown in Table S1.† The values of Φ˙OH, R˙OH, and [˙OH]ss ranged from (1.2 ± 0.39) × 10−5 to (7.2 ± 0.16) × 10−4, (0.61 ± 0.14) × 10−10 M s−1 to (1.8 ± 0.03) × 10−9 M s−1, and (0.26 ± 0.09) × 10−17 M to (0.79 ± 0.05) × 10−16 M, respectively.
Table 2
Φ
˙OH, predicted log
Φ˙OH and experimental log
Φ˙OH of organic compounds
Class |
Chemical name |
Φ
˙OH
× 10−5 |
Predicted log Φ˙OHb |
Experimental log Φ˙OH |
Mean value with a 95% confidence interval with three replicates.
Predicted values of log Φ˙OH as calculated by using eqn (5).
|
I |
Naphthalene |
72 ± 1.6 |
−3.17 |
−3.14 |
Acetophenone |
56 ± 1.4 |
−3.01 |
−3.25 |
2-Chlorohydroquinone |
19 ± 1.2 |
−3.60 |
−3.71 |
II |
Gallic acid |
7.6 ± 0.70 |
−4.22 |
−4.12 |
Coumarin |
7.2 ± 0.53 |
−4.17 |
−4.14 |
1,4-Benzoquinone |
6.8 ± 0.44 |
−4.03 |
−4.17 |
Benzophenone |
5.9 ± 0.38 |
−4.03 |
−4.23 |
3-Methoxyacetophenone |
5.6 ± 0.30 |
−3.86 |
−4.25 |
4-Chloro-4′-hydroxybenzophenone |
4.7 ± 0.14 |
−4.10 |
−4.32 |
2-Hydroxy-4-methylbenzaldehyde |
4.4 ± 0.41 |
−3.96 |
−4.36 |
Trans-cinnamic acid |
3.8 ± 0.34 |
−4.38 |
−4.42 |
2-Acetonaphthone |
3.6 ± 0.32 |
−4.16 |
−4.44 |
Duroquinone |
3.0 ± 0.32 |
−4.38 |
−4.52 |
Dibenzoyl |
2.9 ± 0.18 |
−4.13 |
−4.54 |
Biacetyl |
2.5 ± 0.26 |
−4.82 |
−4.59 |
1,4-Naphthoquinone |
1.7 ± 0.21 |
−4.51 |
−4.77 |
7-Hydroxycoumarin |
1.2 ± 0.39 |
−4.47 |
−4.92 |
|
2,4-Dihydroxybenzophenone |
5.6 ± 0.49 |
−4.53 |
−4.25 |
Fenofibric acid |
2.7 ± 0.16 |
−4.21 |
−4.57 |
Riboflavin |
3.5 ± 0.14 |
−4.37 |
−4.45 |
Among these organic compounds, naphthalene, acetophenone, and 2-chlorohydroquinone (class I in Table 2) have Φ˙OH values of (7.2 ± 0.16) × 10−4, (5.6 ± 0.14) × 10−4, and (1.9 ± 0.12) × 10−4, respectively, which is much higher than that of other compounds. These three compounds have a high value of Φ˙OH because they efficiently react with ground state oxygen to generate the superoxide anion free radical.14 This radical subsequently undergoes a disproportionation reaction to generate H2O2, H2O2 then produces ˙OH by photolysis.27 The electron-withdrawing ability of a chlorine substituent is in general stronger than the electron-withdrawing ability of carbonyl and hydroxyl substituents. The high value of Φ˙OH of naphthalene is dominated by its increased ability for electron donation. Acetophenone contains carbonyl groups, which leads to the competition between electron gain and electron donation. This results in its slightly lower Φ˙OH value than naphthalene.
The other 14 organic compounds are with Φ˙OH values lower than (7.6 ± 0.70) × 10−5 (class II in Table 2). These compounds contain a variety of functional groups of aromatic compounds, including ketone, quinone, phenol, aldehyde, and carboxylic acid functionalities. Similar to class I, the compounds in class II with high electron donating ability are with relatively high values. It is worth mentioning that if a hydroxyl substituent is attached to a benzene ring, it is going to have a conjugated effect of donating electrons. This explains the higher quantum yield for the generation of ˙OH of gallic acid and 2-chlorohydroquinone. The methoxy moiety of 3-methoxyacetophenone has a strong electron-withdrawing ability. This is the reason for its lower Φ˙OH compared with that of acetophenone. In this data, quinones show low quantum yield values of ˙OH, because the hydroxylating species produced by quinone photolysis is not ˙OH, but an ˙OH-like species.28,29 Overall, these findings suggest that the structure of the organic compounds has an enormous influence on the efficiency of generation of ˙OH.
Three organic compounds, including 2,4-dihydroxybenzophenone, fenofibric acid, and riboflavin were selected as the compounds in the validation set. Among which, riboflavin is a commonly used photosensitizer,30 and 2,4-dihydroxybenzophenone and fenofibrate acid are frequently detected organic pollutants31,32 having similar structures to that of the compounds in the training set. The determined Φ˙OH values for the organic compounds in the validation set, i.e. 2,4-dihydroxybenzophenone, fenofibric acid, and riboflavin were (5.6 ± 0.49) × 10−5, (2.7 ± 0.16) × 10−5, and (3.5 ± 0.14) × 10−5, respectively.
3.2 QSAR modelling of the quantum yield of generation of ˙OH
According to the experimental Φ˙OH values and the molecular structure descriptors of the training set, the following QSAR model was constructed (eqn (5)): | log Φ˙OH = –6.551 + 40.882 × X4Av + 35.324 × Psi_i_t − 0.265 × Mor32s, nt = 17, R2 = 0.894, Radj2 = 0.870, RMSEt = 0.255, F = 30.299, QLOO2 = 0.809, p < 0.0001 | (5) |
where F is the test of variance, p is the significance level of F and nt is the quantity of the training set.
The constructed QSAR model has three molecular structure descriptors: X4Av (average valence connectivity index of order 4) represents the Kier–Hall molecular connectivity indices; Psi_i_t (intrinsic state pseudoconnectivity index-type T) represents the electrical topological index; Mor32s (signal 32/weighted by I-state 3D-MoRSE descriptors) represents the 3D-Morse descriptor.
The specific values of these molecular structure descriptors can be clearly seen in Table S2 in the ESI.† The value of Radj2 (adjusted square of the determining coefficient) was 0.870, and the value of the RMSEt was 0.255, which indicated that the developed model had a high fitness. The high QLOO2 value illustrates the good robustness of the developed model. The distinction between Radj2 and QLOO2 is less than 0.3, which means that overfitting does not happen in this model.33 It can be seen from Table 3 that the VIF (variable inflation factor) of each descriptor is small, indicating that there is no multicollinearity problem in this model. Table 2 and Fig. 1 show that the predicted values of log
Φ˙OH of the selected organic compounds, which were calculated by using eqn (5), have a good fit with the experimental values of log
Φ˙OH. These results demonstrate the good predictive ability of the developed QSAR model.
Table 3 Descriptors of VIF, t and p values in the QSAR model
Descriptors |
VIF |
t
|
p
|
Psi_i_t
|
1.502 |
9.460 |
<0.000001 |
X4Av
|
1.452 |
8.693 |
<0.000001 |
Mor32s
|
1.402 |
−5.504 |
<0.0001 |
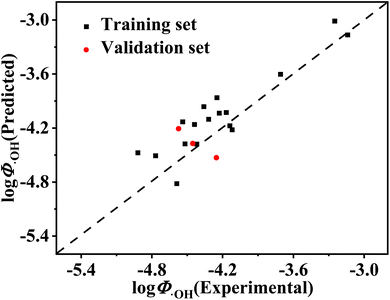 |
| Fig. 1 Plot of predicted values versus experimental values of log Φ˙OH for organic compounds in the training set and validation set. | |
3.3 Mechanistic interpretation
The related information about the descriptors selected for modeling is listed in Table 3, which consists of VIF, t (t-test statistic), and p (corresponding significance level). Psi_i_t is the major descriptor governing the generation of ˙OH compared with Mor32s and X4Av because it has lowest p value in the t-test.14Psi_i_t mainly describes the intrinsic state, valence electron distribution, and energy density within a molecule.34 It is defined as the intrinsic state pseudo-connectivity index. It is positively interrelated to the log
Φ˙OH of the selected organic compounds. Considering the specific chemical structure of the organic compounds, the energy distribution can affect the energy transfer reaction between the excited triplet state and dissolved O2, which dominates the generation of ˙OH. Excited triplet states of aromatic ketones and quinones have higher energy. This is beneficial for the subsequent formation of ˙OH.35
X4Av represents the average valence connectivity index. This index also presents a positive correlation with log
Φ˙OH. The connectivity index is a method to encode molecular structure characteristics such as molecular weight, unsaturated sites, the heteroatomic content, and periodicity according to the number of chemical bonds and branches of the molecule.36 This descriptor usually gives information about the size and molecular weight of a molecule,37 while the organic compounds selected are small molecules. On the one hand, the valence electronegativity of the Kier–Hall molecular connectivity indices is the basis of the electrical topological index. Electronegativity is essentially related to the number of π electrons and lone pair electrons, which contribute significantly to HOMO formation, and electronegativity is more substantial to the ability of gaining and losing electrons. The compounds with a strong electronegativity generate an excited triplet state when exposed to light. The excited triplet state extracts hydrogen from a surrounding water molecule to produce ˙OH. On the other hand, organic compounds with less electronegativity may generate ˙OH through H2O2 production or by the Fenton reaction, and those with electron-rich groups are more likely to form intramolecular interactions to quench the steady-state concentration of the excited triplet state.38
Mor32s is one of the 3D-MORSE descriptors. Mor32s generally describes the importance of atomic properties for ˙OH generation by using a radial basis function. Radial basis functions are often seen as simple neural networks. In this paper, their mathematical equation is shown in formula (6):
| 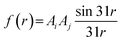 | (6) |
where
Ai and
Aj are the intrinsic states of the corresponding atoms applied for weights,
r represents the distance between atoms, and the intrinsic state value
Ai of the
ith atom can be calculated from the hydrogen-suppressed graph. This descriptor mainly represents electronic properties and contains molecular 3D structure information, which is weighted by the intrinsic state of electron ionization.
Mor32s has an important correlation with the main quantum number, the valence electron number, and the electron distribution characteristics.
All three descriptors present in the QSAR model constructed in this study point to the significance of electronic characteristics of organic compounds. This indicates that the electronic distribution and the electronegativity of the organic molecules plays key roles in the formation of ˙OH from organic compounds. The quantum yields of organic compounds are therefore higher when an electron loss reaction dominates in the system. This is consistent with the pathways for the generation of ˙OH.
Although the mechanism of photo-generation of ˙OH by organic compounds is still controversial, revealing the internal mechanism of generation of ˙OH from the organic compounds is of good importance for comprehending the process of photo-generation of ˙OH in natural waters and for further exploring this mechanism.
3.4 Applicability domain and QSAR model validation
3.4.1 Applicability domain.
As can be seen in Fig. 2, the all organic compounds fall in the applicability domain. The compounds used to construct the training set of the MLR-QSAR model contain various functional groups, for instance, hydroxyl, chlorine substituent, carboxyl, and carbonyl. As shown in Fig. 3, the standard residues of the 20 organic compounds were all fewer than |3|, and the leverage value (h) was lower than its warning leverage value (h*). Neither the compounds in the training set nor the compounds in the validation set of the model have outliers. Therefore, it is considered that the constructed model would be used for forecasting the Φ˙OH value of organic compounds. To our knowledge, no other QSAR model was reported for the prediction of Φ˙OH for organic compounds.
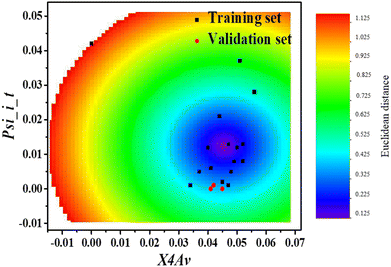 |
| Fig. 2 Molecular descriptor space of the constructed QSAR model, represented by the Euclidean distance-based approach. X4Av: Kier–Hall molecular connectivity indices; Psi_i_t: the intrinsic state pseudo-connectivity index. | |
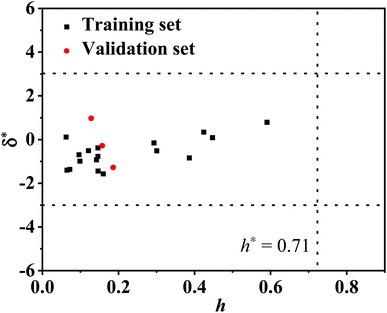 |
| Fig. 3 Williams plots of standardized residuals (δ*) versus leverage values (h) (h* is the warning leverage value). | |
3.4.2 Validation of the developed QSAR model.
As can be seen from Fig. 2 and 3, the organic molecules of the validation set are within the scope of the applicability domain of the constructed QSAR model. As shown in Table 2, the predicted log
Φ˙OH values of 2,4-dihydroxybenzophenone (−4.53), fenofibric acid (−4.21), and riboflavin (−4.37) are comparable to their experimental values. The predicted log
Φ˙OH of the validation set have a good fit with the experimental values of log
Φ˙OH (Fig. 1). This indicates that the constructed model has a reasonable predictive ability. Therefore, it can be used to predict the quantum yield of ˙OH generation from the organic molecules in DOM and also from the organic pollutants in natural water.
4. Conclusions
In this study, the experimental Φ˙OH values for organic compounds with all kinds of functional groups were determined. The results demonstrated that the selected compounds can effectively generate ˙OH as sensitizers, and the production of ˙OH by these compounds is dependent on their structures. The developed QSAR model showed an excellent fitness, robustness, and perfect predictability, and it is very helpful to explain the generation mechanism of ˙OH from DOM and organic pollutants in natural water. The electronegativity of a molecule determines the generation ability of ˙OH from organic compounds, and when there is an electronic competition between the gain and loss of electrons, the value of Φ˙OH is usually small. The constructed QSAR model can be potentially used to predict the generation quantum yield of ˙OH from organic compounds in natural water.
Author contribution
Liu Yue: conceptualization, methodology, software, data curation, writing – original draft preparation. Chen Xiaobing: conceptualization, software, visualization, investigation. Zhang Jianchen: data curation. Jin Wenjie: supervision. Zhang Kun: software. Zhang Ya-nan: conceptualization, supervision, writing – reviewing and editing, funding acquisition. Qu Jiao: supervision, project administration, funding acquisition. Chen Guangchao: writing – reviewing and editing. Willie J. G. M. Peijnenburg: writing – reviewing and editing.
Conflicts of interest
There are no conflicts of interest to declare.
Acknowledgements
This work was supported by the National Natural Science Foundation of China (No. 22176030, 42130705, 41877364, and 21976027), the Fundamental Research Funds for the Central Universities (No. 2412020FZ015 and 2412019FZ019), and the Jilin Province Science and Technology Development Projects (No. 20190303068SF and 20200301012RQ).
References
- C. Li, G. Wei, J. Chen, Y. Zhao, Y. Zhang, L. Su and W. Qin, Aqueous OH Radical Reaction Rate Constants for Organophosphorus Flame Retardants and Plasticizers: Experimental and Modeling Studies, Environ. Sci. Technol., 2018, 52(5), 2790–2799 CrossRef CAS PubMed.
- S. Gligorovski, R. Strekowski, S. Barbati and D. Vione, Environmental Implications of Hydroxyl Radicals (˙OH), Chem. Rev., 2015, 115(24), 13051–13092 CrossRef CAS PubMed.
- R. G. Zepp, J. Hoigne and H. Bader, Nitrate-induced photooxidation of trace organic chemicals in water, Environ. Sci. Technol., 1987, 21(5), 443–450 CrossRef CAS PubMed.
- S. Page, W. Arnold and K. McNeill, Terephthalate as a probe for photochemically generated hydroxyl radical, J. Environ. Monit., 2010, 12, 1658–1665 RSC.
- L. Carena, M. Minella, F. Barsotti, M. Brigante, M. Milan, A. Ferrero, S. Berto, C. Minero and D. Vione, Phototransformation of the herbicide propanil in paddy field water, Environ. Sci. Technol., 2017, 51, 2695–2704 CrossRef CAS PubMed.
- K. Mopper and X. L. Zhou, Hydroxyl radical photoproduction in the sea and its potential impact on marine processes, Science, 1990, 250(4981), 661–664 CrossRef CAS PubMed.
- F. Al Housari, D. Vione, S. Chiron and S. Barbati, Reactive photo induced species in estuarine waters. Characterization of hydroxyl radical, singlet oxygen and dissolved organic matter triplet state in natural oxidation processes, Environ. Sci.: Processes Impacts, 2010, 9(1), 78–86 CAS.
- J. P. Hassett, Dissolved natural organic matter as a microreactor, Science, 2006, 311(5768), 1723–1724 CrossRef CAS PubMed.
- Y.-N. Zhang, Q. Xie, J. Chen, Y. Li and Z. Fu, Insights into the photochemical transformation pathways of triclosan and 2′-HO-BDE-28, J. Hazard. Mater., 2015, 300, 354–358 CrossRef CAS PubMed.
- L. Ge, J. Chen, X. Wei, S. Zhang, X. Qiao, X. Cai and Q. Xie, Aquatic Photochemistry of Fluoroquinolone Antibiotics: Kinetics, Pathways, and Multivariate Effects of Main Water Constituents, Environ. Sci. Technol., 2010, 44, 2400–2405 CrossRef CAS PubMed.
- H. Xu, Y. Li, L. Zhao, H. Du and H. Jiang, Molecular weight-dependent heterogeneities in photochemical formation of hydroxyl radical from dissolved organic matters with different sources, Sci. Total Environ., 2020, 725, 138402 CrossRef CAS PubMed.
- Y. Wang, J. Chen, X. Yang, F. Lyakurwa, X. Li and X. Qiao, In silico model for predicting soil organic carbon normalized sorption coefficient (K-oc) of organic chemicals, Chemosphere, 2015, 119, 438–444 CrossRef CAS PubMed.
- C. Li, S. Zheng, T. Li, J. Chen, J. Zhou, L. Su, Y.-N. Zhang, J. C. Crittenden, S. Zhu and Y. Zhao, Quantitative structure–activity relationship models for predicting reaction rate constants of organic contaminants with hydrated electrons and their mechanistic pathways, Water Res., 2019, 151, 468–477 CrossRef CAS PubMed.
- J. Zhao, Y. Zhou, C. Li, Q. Xie, J. Chen, G. Chen, W. J. G. M. Peijnenburg, Y.-N. Zhang and J. Qu, Development of a quantitative structure–activity relationship model for mechanistic interpretation and quantum yield prediction of singlet oxygen generation from dissolved organic matter, Environ. Sci. Technol., 2020, 712, 136450 CAS.
- W. Wang, C. He, Y. Gao, Y. Zhang and Q. Shi, Isolation and characterization of hydrophilic dissolved organic matter in waters by ion exchange solid phase extraction followed by high resolution mass spectrometry, Environ. Chem. Lett., 2019, 17, 1857–1866 CrossRef CAS.
- M. Kishino, N. Okami, M. Takahashi and S.-E. Ichimura, Light utilization efficiency
and quantum yield of phytoplankton in a thermally stratified sea, Limnol. Oceanogr., 1986, 31(3), 557–566 CrossRef.
- Y.-N. Zhang, J. Wang, J. Chen, C. Zhou and Q. Xie, Phototransformation of 2,3-dibromopropyl-2,4,6-tribromophenyl ether (DPTE) in natural waters: important roles of dissolved organic matter and chloride ion, Environ. Sci. Technol., 2018, 52(18), 10490–10499 CrossRef CAS PubMed.
- C. Zhou, J. Chen, H. Xie, Y.-N. Zhang, Y. Li, Y. Wang, Q. Xie and S. Zhang, Modeling photodegradation kinetics of organic micropollutants in water bodies: a case of the Yellow River estuary, J. Hazard. Mater., 2018, 349, 60–67 CrossRef CAS PubMed.
- E. De Laurentiis, S. Buoso, V. Maurino, C. Minero and D. Vione, Optical and photochemical characterization of chromophoric dissolved organic matter from lakes in terra nova bay, antarctica. Evidence of considerable photoreactivity in an extreme environment, Environ. Sci. Technol., 2013, 47, 14089–14098 CrossRef CAS PubMed.
- L. Carena, M. Minella, F. Barsotti, M. Brigante, M. Milan, A. Ferrero, S. Berto, C. Minero and D. Vione, Phototransformation of the Herbicide Propanil in Paddy Field Water, Environ. Sci. Technol., 2017, 51(5), 2695–2740 CrossRef CAS PubMed.
- H. Xu, Y. Li, J. Liu, H. Du, Y. Du, Y. Su and H. Jiang, Photogeneration and steady-state concentration of hydroxyl radical in river and lake waters along middle-lower Yangtze region, China, Water Resour., 2020, 176, 115774 CAS.
-
M. J. Frisch, G. W. Trucks, H. B. Schlegel, G. E. Scuseria, M. A. Robb, J. R. Cheeseman, G. Scalmani, V. Barone, G. A. Petersson, H. Nakatsuji, X. Li, M. Caricato, A. V. Marenich, J. Bloino, B. G. Janesko, R. Gomperts, B. Mennucci, H. P. Hratchian, J. V. Ortiz, A. F. Izmaylov, J. L. Sonnenberg, D. Williams-Young, F. Ding, F. Lipparini, F. Egidi, J. Goings, B. Peng, A. Petrone, T. Henderson, D. Ranasinghe, V. G. Zakrzewski, J. Gao, N. Rega, G. Zheng, W. Liang, M. Hada, M. Ehara, K. Toyota, R. Fukuda, J. Hasegawa, M. Ishida, T. Nakajima, Y. Honda, O. Kitao, H. Nakai, T. Vreven, K. Throssell, J. A. Montgomery Jr, J. E. Peralta, F. Ogliaro, M. J. Bearpark, J. J. Heyd, E. N. Brothers, K. N. Kudin, V. N. Staroverov, T. A. Keith, R. Kobayashi, J. Normand, K. Raghavachari, A. P. Rendell, J. C. Burant, S. S. Iyengar, J. Tomasi, M. Cossi, J. M. Millam, M. Klene, C. Adamo, R. Cammi, J. W. Ochterski, R. L. Martin, K. Morokuma, O. Farkas, J. B. Foresman and D. J. Fox, Gaussian 16, Revision b.01, Gaussian, inc., Wallingford ct, 2016 Search PubMed.
- C. Lee, W. Yang and R. G. Parr, Development of the Colle-Salvetti correlation-energy formula into a functional of the electron density, Phys. Rev. B Condens. Matter, 1988, 37(2), 785–789 CrossRef CAS PubMed.
- A. D. Becke, Density-functional thermochemistry III. The role of exact exchange, J. Chem. Phys., 1993, 98(7), 5648–5652 CrossRef CAS.
- E. Cances, B. Mennucci and J. Tomasi, A new integral equation formalism for the polarizable continuum model: theoretical background and applications to isotropic and anisotropic dielectrics, J. Chem. Phys., 1997, 107(8), 3032–3041 CrossRef CAS.
- R. Xiao, T. Ye, Z. Wei, S. Luo, Z. Yang and R. Spinney, Quantitative Structure–Activity Relationship (QSAR) for the oxidation of trace organic contaminants by sulfate radical, Environ. Sci. Technol., 2015, 49(22), 13394–13402 CrossRef CAS PubMed.
- P. P. Vaughan and N. V. Blough, Photochemical formation of hydroxyl radical by constituents of natural waters, Environ. Sci. Technol., 1998, 32, 2947–2953 CrossRef.
- A. Bedini, E. De Laurentiis, B. Sur, V. Maurino, C. Minero, M. Brigante, G. Mailhot and D. Vione, Phototransformation of anthraquinone-2-sulphonate in aqueous solution, Photochem. Photobiol. Sci., 2012, 11(9), 1445–1453 CrossRef CAS PubMed.
- K. Couch, F. Leresche, C. Farmer, G. McKay and F. L. Rosario-Ortiz, Assessing the source of the photochemical formation of hydroxylating species from dissolved organic matter using model sensitizers, Environ. Sci.: Processes Impacts, 2022, 24, 102–115 RSC.
- M. Schmitt, K. J. Moor, P. R. Erickson and K. McNeill, Sorbic acid as a triplet probe: reactivity of oxidizing triplets in dissolved organic matter by direct observation of aromatic amine oxidation, Environ. Sci. Technol., 2019, 53(14), 8087–8096 CrossRef CAS PubMed.
- M. J. M. Bueno, M. J. Gomez, S. Herrera, M. D. Hernando, A. Agüera and A. R. Fernández-Alba, Occurrence and persistence of organic emerging contaminants and priority pollutants in five sewage treatment plants of Spain: two years pilot survey monitoring, Environ. Pollut., 2012, 164, 267–273 CrossRef CAS PubMed.
- M. T. Zou, Y. M. Qi, R. Qu, G. Al-Basher, X. X. Pan, Z. Y. Wang, Z. L. Huo and F. Zhu, Effective degradation of 2,4-dihydroxybenzophenone by zero-valent iron powder (Fe0)-activated persulfate in aqueous solution: kinetic study, product identification and theoretical calculations, Sci. Total Environ., 2021, 771, 144743 CrossRef CAS PubMed.
- A. Golbraikh and A. Tropsha, Beware of q2!, J. Mol. Graph. Model., 2002, 20(4), 269–276 CrossRef CAS PubMed.
- W. Liu, H. Lu, C. Cao, Y. Jiao and G. Chen, An improved quantitative structure property relationship model for predicting thermal conductivity of liquid aliphatic alcohols, J. Chem. Eng. Data, 2018, 63(12), 4735–4740 CrossRef CAS.
- K. Mcneill and S. Canonica, Triplet state dissolved organic matter in aquatic photochemistry: reaction mechanisms, substrate scope, and photophysical properties, Environ. Sci.: Processes Impacts, 2016, 18(11), 1381–1399 RSC.
- B. Y. Ren, Application of novel atom-type Al topological indices to QSPR studies of alkanes, Comput. Chem., 2002, 26(4), 357–369 CrossRef CAS PubMed.
- M. Shahlaei, A. Madadkar-Sobhani, L. Saghaie and A. Fassihi, Application of an expert system based on Genetic Algorithm-Adaptive Neuro-Fuzzy Inference System (GA-ANFIS) in QSAR of cathepsin K inhibitors, Expert. Syst. Appl., 2012, 39(6), 6182–6191 CrossRef.
- G. Mckay, W. Huang, C. Romera-Castillo, J. E. Crouch, F. L. Rosario-Ortiz and R. Jaffe, Predicting reactive intermediate quantum yields from dissolved organic matter photolysis using optical properties and antioxidant capacity, Environ. Sci. Technol., 2017, 51(10), 5404–5413 CrossRef CAS PubMed.
|
This journal is © The Royal Society of Chemistry 2023 |