Aircraft measurements of single particle size and composition reveal aerosol size and mixing state dictate their activation into cloud droplets†
Received
6th April 2023
, Accepted 2nd August 2023
First published on 14th August 2023
Abstract
Shallow convective clouds are common in many regions of the world. Currently, aerosol–cloud interactions parameterizations for convective clouds are a major source of uncertainty in global climate model predictions of radiative forcing. Size and composition of individual aerosol particles are the most important properties that determine aerosol activation into cloud droplets and the impacts of aerosol on aerosol–cloud-climate interactions. A challenge to accurately describe aerosol activation is often due to a lack of measurements of individual particle size and composition, making it necessary to rely on simplistic and, most often, unrealistic aerosol mixing state assumptions, which are known to lead to significant errors in predicted concentrations of cloud condensation nuclei (CCN). We present the aircraft-based single-particle measurements of the size and composition of individual below-cloud particles, interstitial aerosol particles, and cloud droplet residuals during two contrasting seasons. Measurements reveal enhanced contribution from larger and sulfate-rich particles in cloud droplet residuals and provide direct evidence for sulfate and isoprene-epoxydiol-derived secondary organic aerosol (IEPOX-SOA) formation in cloud droplets. We observe a strong dependence of the size and composition of below-cloud aerosol on their cloud droplet activation fraction during the spring campaign, when the observed dynamic range in aerosol properties was large. Furthermore, we report clear seasonal differences in the aerosol activation fraction (0.38 ± 0.21 for spring and 0.20 ± 0.08 for summer) over the Atmospheric Radiation Measurements (ARM), Southern Great Plains (SGP) atmospheric observatory in Oklahoma, consistent with high fluxes of biogenic volatile organic compounds that drive the formation and growth of less-hygroscopic organic components during summer but not during spring when the measured aerosol composition was more variable. A closure between measured cloud droplet number concentrations and predicted CCN (using κ-Köhler theory and measurement-constrained aerosol properties, including their mixing state) revealed that the effective supersaturations of shallow cumuli ranged between 0.06% and 0.24%. This study highlights the importance of measuring particle-by-particle variability in size and composition, and mixing state of aerosol population to accurately represent their activation into shallow cumuli cloud droplets, even at a background site like SGP.
Environmental significance
Fair weather, shallow convective clouds are ubiquitous over land, but their representation in models is poorly constrained. Size and composition of individual aerosol particles are the most important properties that determine aerosol activation into cloud droplets and the impacts of aerosol on aerosol–cloud-climate interactions. A challenge to accurately describe aerosol activation is often due to a lack of measurements of individual particle size and composition, making it necessary to rely on simplistic and, most often, unrealistic representations of aerosol mixing state. Here, we present the measurements of the size and composition of individual below-cloud particles and cloud droplet residuals (particles that remain after evaporation of cloud water) during two contrasting seasons. We demonstrate that below-cloud aerosol hygroscopicity and size strongly affect their activation during spring, when below-cloud aerosol properties were highly variable, as compared to summer, when the activated fraction was low and aerosol composition was dominated by oxygenated organic components, consistent with higher summertime surface emissions of biogenic volatile organic compounds.
|
1 Introduction
Shallow, fair-weather, convective clouds over land cover 10–30% of the surface,1 which is enough to affect Earth's radiation budget on global2 and regional3 scales. Shallow cumuli are linked to surface properties and fluxes,4–7 emphasizing the importance of understanding how shifts in natural and anthropogenic land use, surface properties, and emissions affect shallow cumuli properties. Aerosol number concentrations were shown to alter properties of shallow cumuli,3,8–12 although these effects are often obfuscated by large-scale meteorological forcing,13 making it difficult to draw robust conclusions regarding the role of aerosol properties on cloud droplet activation. Currently, aerosol–cloud interaction parameterizations in global climate models are a large source of radiative uncertainty.14
Furthermore, there are uncertainties regarding cloud coupling to the sub-cloud layer, including the sensitivity of cloud condensation nuclei (CCN) concentrations to variability in aerosol properties, including their size and chemical composition, within a population. This is especially true for continental shallow convective clouds4 due to complex below-cloud aerosol composition, including the formation of secondary organic aerosol (SOA) that can significantly alter particle hygroscopicity (e.g., Massoli et al.15) and CCN activity.
Ultimately, climate and cloud aerosol effects are controlled by the subset of particles that act as CCN. CCN activity depends on the properties of individual particles, which are often not measured. Individual ambient particles represent a complex mixture of multiple chemical components.16–22 Because of the inherent complexity of aerosol mixing state, resolving aerosol activation often relies on simplistic and unrealistic aerosol mixing state assumptions (e.g., complete internal or external mixture) or idealized treatment of their composition.23–28 These assumptions are known to lead to significant errors in predicted CCN concentrations.22,28–34 Validation of these assumptions comes from closure studies, where different mixing state and composition scenarios are tested to produce the closest agreement with measurements (Kulkarni, et al. and references therein).28 These simplistic assumptions have commonly been used with various degrees of success.29,35,36 Moreover, there is large variability in the reported sensitivity of aerosol composition and mixing state on their CCN activity from field observations. For instance, Padró et al.37 reported that CCN closure was highly sensitive (+20% to +240% overprediction) to the different mixing state and chemical composition scenarios in Atlanta, GA. Furutani et al.38 reported large errors (−30% to +70%) in predicted CCN number concentrations, when assuming constant composition for different air masses sampled along the southern coast of California. Ching et al.22 used transmission electron microscopy to analyze the size, composition, and morphology of ∼32
000 single particles collected from urban, mountain, and rural sites in Japan to demonstrate that all the samples exhibit a wide range of mixing states and that assumption of homogeneous chemical composition could lead to significant error (up to 90%) in the predicted CCN concentrations.22 In contrast, Dusek et al.39 and Rose et al.40 reported that use of average aerosol composition was sufficient to represent CCN measurements. Similarly, Jurányi et al.41 concluded that for reliable CCN predictions at the Jungfraujoch alpine site it is important to know the average chemical composition, but the temporal variability in aerosol chemical composition could be neglected.
In addition, multiple assumptions regarding aerosol composition and mixing state are often required to satisfactorily achieve CCN closure, but these assumptions often break down in different locations or during different times of the day, and may not reflect the true nature of ambient particles.42 Lance et al.43 found that treating the aerosol as externally mixed during morning rush hours in Mexico city, but not during other times of the day, achieved the best CCN closure. Cubison et al.44 found good CCN closure assuming size-dependent composition and treating fresh organic and soot particles as externally mixed over Riverside, CA. Wang et al.45 concluded that CCN concentrations can be derived with sufficient accuracy by assuming internal mixture and bulk composition during the daytime and tens of kilometers away from primary organic sources. Roberts et al.46 found that bulk chemical measurement predicted CCN concentrations reasonably well when sampling over the California Central Valley, but not when sampling the marine boundary layer, which was attributed to the presence of externally mixed aerosol.
Most recently, Kulkarni et al.28 performed a CCN closure study using airborne measurements of below-cloud non-refractory bulk aerosol chemical composition, aerosol size distributions, and CCN concentrations measured by CCN counter (CCNC) at two instrument supersaturations (0.24% and 0.46%) during the Holistic Interactions of Shallow Clouds, Aerosols and Land Ecosystems (HI-SCALE) field campaign in the spring and summer of 2016.28 They found that the best CCN closure was achieved assuming either that all particles are composed of pure organics, or that particles are externally mixed and composed of pure sulfates, nitrates, and organics, with hygroscopicity values for organic particles varying between 0.04 and 0.17. The assumption that particles are internally mixed led to systematic overprediction of CCN concentrations over the SGP site and the largest differences between the measured and predicted CCN concentrations. To improve agreement with ±20% with the measured CCN concentrations required varying additional parameters, e.g., density and the fraction of insoluble organics. Most importantly, all three assumptions of aerosol mixing state are not supported by the single-particle measurements conducted during the very same field campaign. Furthermore, the measurements of bulk aerosol composition are mass-based, while both CCN activation and single-particle measurements are number-based.
These reported differences between predicted and measured CCN concentrations are not surprising given that ambient particles exhibit various degrees of external mixture19,21,22,47 and demonstrate the importance of measuring the aerosol mixing state to improve CCN predictions. Recent improvements in aerosol representation32 can account for more realistic aerosol mixing states, but improving the representation of aerosol activation requires coincident field observations of size, composition, and mixing state of below-cloud aerosol and cloud droplet residuals, which are scarce, e.g.,.21,48,49 The high spatial variability50 and small horizontal extent (∼1 km)51,52 of shallow cumuli makes them particularly difficult to comprehensively sample. Highly sensitive instrumentation such as single-particle mass spectrometers have the potential to accurately represent the size, composition, and real-world mixing state of ambient particles, especially during aircraft sampling and thus detect a clear aerosol signature on the properties of shallow cumuli.
Here we present measurements collected during HI-SCALE field campaign4 over the Atmospheric Radiation Measurements (ARM), Southern Great Plains (SGP) atmospheric observatory in Oklahoma53 during two seasons, characterized by below-cloud aerosol with very different composition and investigate the role of single particle size and composition, and mixing state of ambient aerosol population on cloud activation fraction of continental shallow cumuli.
2 Experimental
2.1 Campaign overview and instrumentation
The Holistic Interactions of Shallow Clouds, Aerosols, and Land Ecosystems (HI-SCALE) field campaign occurred in the spring and summer of 2016 near the Department of Energy's ARM climate research facility at SGP. A detailed overview of HI-SCALE is presented in Fast et al.4 Briefly, 38 flights were conducted from Bartlesville, Oklahoma onboard the ARM Aerial Facility's Gulfstream 1 (G-1) aircraft platform54 to characterize the below-, in-, and above-cloud aerosol. 6.5 hours out of 57.8 flight hours (11%) and 1.1 hours out of 47.8 flight hours (2.3%) were spent sampling clouds during spring (Apr. 25 – May 20) and summer (Aug. 29 – Sep. 22), respectively.
Sampling alternated between an isokinetic inlet (for characterization of below-cloud, interstitial, and above-cloud aerosol) and a counterflow virtual impactor inlet (CVI, for characterization of cloud residuals).55 The CVI inlet transmitted only droplets with aerodynamic diameters larger than ∼13 μm, resulting in ∼20% (range = 0.2–64%) of droplets being transmitted through the CVI inlet. Because condensation growth alone leads to narrow droplet size spectrum, the width of droplet size distribution is often dominated by other processes, such as entrainment mixing (e.g.,56), and not the dry particle sizes. Therefore, the reported composition and size of droplet residuals are expected to be representative of the entire droplet population, since larger droplets are not necessarily associated with more hygroscopic and/or larger residual particles.57
Aerosol size distributions (mobility diameter, dm) in the size range from 10 nm to 0.4 μm were measured using a fast integrated mobility spectrometer (FIMS)58 and used to quantify total particle number concentrations and concentration of particles in accumulation mode (with diameters >0.1 μm). Cloud condensation nuclei (CCN) measurements were performed using a cloud condensation nuclei counter (CCNC, DMT),59 operating at two instrument supersaturations: 0.24% and 0.46%.
Ambient number size distributions were also measured using a wing-mounted passive cavity aerosol spectrometer probe (PCASP, PMS Inc., size range from 125 nm to 2.9 μm optical aerosol diameter) and an ultra-high-sensitivity aerosol spectrometer (UHSAS, DMT, size range from 73 nm to 683 nm optical aerosol diameter). PCASP measurements were used to quantify interstitial aerosol number concentrations, when the FIMS was used to sample cloud droplets residuals behind the CVI, and UHSAS measurements were used for flights when FIMS measurements were not available. Cloud droplet number size distributions in the size range from 0.75 μm to 50 μm diameter were measured using a wing mounted fast cloud droplet probe (FCDP).
Size (vacuum aerodynamic diameter, dva) and composition of individual aerosol particles and cloud droplet residuals in the size range from 50 nm to 2 μm were characterized using single particle mass spectrometer, miniSPLAT, described in detail elsewhere.21,47 miniSPLAT detects and characterizes ∼50% of 83 nm-diameter particles, and nearly 100% of spherical particles in the size range from 125 nm to 600 nm sampled by the instrument.47
2.2 Cloud periods and activation fraction
To reduce the influence of cloud edges we define cloud periods using a cloud flag, which marks periods with cloud droplet number concentration (Nd) larger than 3 cm−3. The activation fraction is defined as the ratio of Nd and the sum of Nd and interstitial (not activated) aerosol. The activation ratio has been extensively used to interpret laboratory (e.g., ref. 60 and 61) and field (e.g., ref. 25, 37, 38, 62–64) CCN measurements. Using the activation fraction eliminates the effects of variable particle concentrations. Because UHSAS and PCASP have a lower cutoff of ∼0.1 μm, in this study the activation fraction is representative of accumulation mode particles only. Considering only particles >0.1 μm diameter is not expected to significantly affect our conclusions since 83% (median value for flight averages) of cloud residuals were >0.1 μm mobility diameter, consistent with the low effective cloud supersaturations encountered during HI-SCALE.
2.3 Single particle characterization
During HI-SCALE, miniSPLAT sized over 140 million individual particles and acquired mass spectra of ∼700
000 of them, characterizing both refractory and non-refractory fractions of each particle. Measured single particles vacuum aerodynamic diameters were grouped to produce one-minute averaged dva size distributions. The dva distributions were then normalized to the total number of particles sized per minute.
The single particle mass spectra were classified into hundreds of classes, which are then merged into nodes by sequentially combining similar clusters, as detailed in Zelenyuk et al.65 For simplicity, similar aerosol classes have been subsequently combined into 10 distinct, physically meaningful, aerosol types, with the final particle classes including all the particles in the original dataset. These aerosol types include sulfate- and nitrate-rich particles internally mixed with organics, fresh and oxygenated/aged organics (Org1 and Org2, respectively, with latter characterized by dominant 44CO2+ peak), soot, biomass burning aerosol (BB, characterized by strong 39K+ peak, and signatures of organic and elemental carbon, polyaromatic hydrocarbons, and some inorganics (213K3SO4+)), BB SOA (which is correlated strongly with the BB and exhibit high intensity organic peaks at e.g., 43C2H3O/C3H7+, 59C3H7O+), dust, pyridine-containing particles (characterized by intense 80C5H5NH+ peak), and isoprene-epoxydiol-derived (IEPOX) SOA, with characteristic peaks identified in the laboratory studies (e.g., 101C5H9O2+, 105C4H9O3+, 87C4H7O2+, 83C5H7O+, 75C3H7O2+, 71C4H7O+).47,66 Soot particles were found to be internally mixed with small amounts of organics and hygroscopic components, as expected from fresh emissions.
3 Results and discussion
3.1. Timeseries
Fig. 1 shows, as an example, the data obtained during two HI-SCALE flights conducted on April 25 (a–d) and May 1, 2016 (e–h). The top panels present the altitude profiles showing that for both flights, measurements were conducted below-cloud, in-cloud (magenta datapoints), and above-cloud, with the shaded sections marked by the CVI flag = 1, indicating sampling droplet residuals through the CVI. Also shown are the normalized miniSPLAT-measured particle dva size distributions (b and f), particle compositions, plotted as number fractions of particles of different types (c and g), and the fraction of cloud activated particles (d and h). The measured concentrations of cloud droplets and interstitial particles, used to calculate the fraction of cloud activated particles, are shown in Fig. S1.†
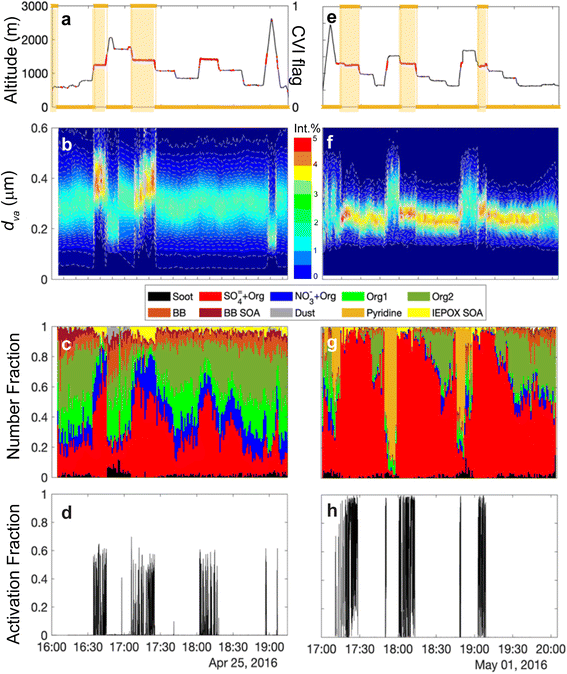 |
| Fig. 1 Timeseries for measurements conducted during April 25 and May 1 flights, left and right columns, respectively: (a and e) the altitude profiles with cloud periods marked in magenta, and the shaded section representing sampling through the CVI; (b and f) vacuum aerodynamic diameter size distributions normalized by sum; (c and g) number fractions of different aerosol types; and (d and h) droplet activation fractions. | |
The data clearly indicate large temporal and spatial variations in aerosol properties, including the significant differences in size and composition of particles within the boundary layer and in the free troposphere. Below-cloud particles sampled on April 25 were larger (dva = 0.33 ± 0.02 μm) as compared to particles sampled on May 1 (dva = 0.25 ± 0.02 μm). In contrast, particles sampled in the free troposphere on April 25 where significantly smaller (mobility diameter dm = 0.05 ± 0.01 μm with most particles below miniSPLAT detection), compared to May 1 (dva = 0.29 ± 0.01 μm). Changes in particle size were accompanied by changes in particle composition. On April 25 more than 50% of below-cloud particles were organics (Org1 and Org2), as compared to <30% for May 1 when sulfate-rich particles represented the dominant aerosol type. BB, BB SOA, and dust particles comprised 20 ± 8% of the particles sampled above the boundary layer as compared to 11 ± 5% below-cloud level on April 25. In contrast, on May 1 particles with mass spectra dominated by protonated pyridine ion (H+C5H5N) accounted for over 60% of above-cloud aerosol compared to <1% below-cloud. Although the origin of these particles has not yet been fully established,67–69 pyridine-containing particles are commonly observed in the free troposphere.69–71
The comparison of the size and compositions of below-cloud particles with those of cloud residuals shows that the fraction of more hygroscopic sulfate-rich particles increased from 18 ± 6% for below-cloud aerosol to 55 ± 9% for cloud residuals for April 25, and from 66 ± 19% to 91 ± 1% for May 1, indicating preferential activation of these particles and/or formation of sulfate in the cloud droplets.21 The data also show decreased relative contributions from less hygroscopic organic, BB, BB SOA, and soot particles, consistent with their lower CCN efficiencies.72 The larger contribution of sulfate-rich particles to residuals observed during May 1 compared to April 25 can explain the smaller size of cloud droplet residuals during May 1 (dva = 0.26 ± 0.01 μm) as compared to April 25 (dva = 0.35 ± 0.03 μm), consistent with the fact that more hygroscopic aerosols require smaller activation diameters. Similar sulfate enrichment in cloud residuals was observed for Arctic clouds and was attributed to preferential activation of more hygroscopic particles47 and observation of in-cloud droplet processing.21
The dva size distributions shown in Fig. 1b and f indicate that the vacuum aerodynamic diameters of cloud residuals are larger than those of below-cloud particles. Similarly, Fig. S2† shows that their mobility diameters are larger as well. As with the differences in composition, the increase in cloud droplet residual size could be caused by more efficient activation of larger particles and/or aqueous chemistry that adds hygroscopic components (e.g., sulfate and IEPOX-SOA) and increases the particle size.49,73
An example of in-cloud sulfate formation is evident in Fig. 1c, which shows an increase in the fraction of sulfate-rich particles in the interstitial particles (magenta points and CVI flag = 0, Fig. 1a) compared to below cloud particles. Number fractional contribution of sulfate-rich particles was 44 ± 9% during the interstitial leg as compared to 26 ± 7% for the two adjacent below-cloud legs. This most likely is the result of in-cloud sulfate formation followed by droplet recirculation, a process very common in these types of clouds. Similar to in-cloud sulfate formation, the data show that IEPOX-SOA was enhanced in cloud droplet residuals (7 ± 3%) compared to below-cloud aerosol (2 ± 1%) (Fig. 1c) for April 25, consistent with recent work74 that suggests that the higher volume of cloud droplets can be more conducive to IEPOX-SOA formation as compared to deliquesced aerosol particles. The larger fractional abundance of IEPOX-SOA in cloud droplet residuals for April 25 compared to May 1 despite a smaller contribution from acidic seeds can be explained by lower gas-phase concentrations of IEPOX during May 1 (4.6 ± 1.9 pptv) compared to April 25 (33.8 ± 13.6 pptv).
The data presented in Fig. 1d and h point to significant variability for the activation fraction within each flight and between the two flights. For instance, 25 ± 19% of the particles with diameters >0.1 μm activated during April 25 as compared to 68 ± 31% during May 1. The higher average activation fraction observed during May 1 cannot be explained by the in-cloud updraft velocities (Fig. S3a†) and is likely due to higher contribution from sulfate-rich particles and lower below-cloud aerosol concentrations: 918 ± 347 cm−3 (May 1) and 1676 ± 676 cm−3 (April 25). Entrainment mixing of drier air75 can also contribute to the observed variability in activation fraction on short timescales.
3.2. Composition of below-cloud aerosol and cloud droplet residuals
Here, we compare the composition of below-cloud aerosol and cloud droplet residuals on seasonal timescales. Only data from cloudy flights are included for a consistent comparison (total of 17 flights). The spring and summer campaigns were characterized by different meteorological conditions, biogenic volatile organic compound (VOC) emissions, and hence aerosol composition, size distributions, and number concentrations. The higher surface temperatures during summer (24.2 ± 1.8 °C) compared to spring (14.1 ± 4.5 °C, Fig. S4†) served as a strong driver for biogenic VOC emissions at SGP,76 resulting in ∼2 times higher isoprene and α-pinene concentrations during summer as compared to spring.77 Surface RH were similar for the two campaigns: 78.1 ± 10.3% (spring) and 73.3 ± 9.0% (summer). Below-cloud accumulation-mode particle number concentrations (N100) also increased from 535 ± 266 cm−3 (spring) to 841 ± 313 cm−3 (summer), although total below-cloud number concentrations were comparable for the two campaigns: 3382 ± 1786 cm−3 (spring) and 3601 ± 723 cm−3 (summer).
The miniSPLAT measurements of below-cloud aerosol composition indicate substantial seasonal differences (Fig. 2a and c). During spring, sulfate-rich particles represented the dominant aerosol type. In contrast, organic particles (Org1 + Org2) accounted for 64% of the below-cloud particles during summer, consistent with lower bulk aerosol densities estimated during summer.78 Oxygenated organic (Org2) particles represented a significantly larger number fraction of particles during summer (34.2% and 53% of organics) compared to spring (4.5% and 20% of organics), consistent with the enhanced formation of SOA components during summer from higher biogenic VOC emissions and solar radiation, and in line with previous observations at SGP.79 The larger contribution from IEPOX-SOA particles to below-cloud aerosol observed during spring (5.5%) compared to summer (2%), despite higher summer isoprene (and IEPOX) emissions, is likely due to higher contribution from sulfate-rich seed particles (44% during spring and only 8% during summer), which are essential for multiphase formation of IEPOX-SOA (e.g., Surratt et al.80).
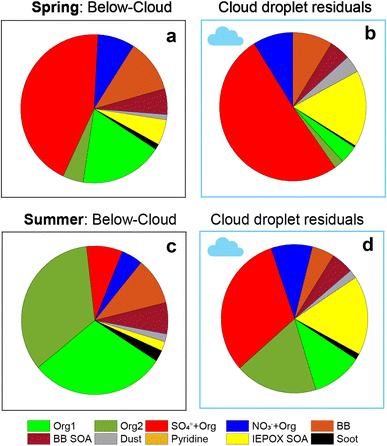 |
| Fig. 2 Composition of aerosol particles below-cloud (a and c) and cloud droplet residuals (b and d) sampled during spring (upper panels) and summer (lower panels) for 17 cloudy flights (8 in spring and 9 in summer) for which below-cloud aerosol and cloud droplet residuals were characterized. | |
The differences between below-cloud particles and cloud droplet residuals observed for individual flights (Fig. 1) also hold on seasonal scales. Cloud droplet residuals were enriched in sulfate-rich particles compared to below-cloud aerosol during spring and summer (Fig. 2). The campaign average contribution of sulfate-containing particles increased from 44% for below-cloud aerosol to 51% for cloud droplet residuals during spring, and from 8% for below-cloud aerosol to 31% for cloud droplet residuals during summer. The enhancement in sulfate-rich particles in cloud droplet residuals was mirrored by decreased contributions from organics and soot particles, especially during summer. Organic particles (Org1 + Org2) accounted for 23% of below-cloud particles but only 6% of cloud droplet residual number during spring. Similarly, organics accounted for 64% of below-cloud particle number but their contribution was 29% of cloud droplet residuals during summer. These trends are predicted by model simulations (e.g. Sanchez et al.81), and consistent with measurements reported for other locations (e.g., by Sellegri et al.82 at a mountainous site in France and Zelenyuk et al.21,47 in Arctic).
Fig. 2 also shows that oxygenated Org2 particles accounted for a higher fraction of organics in cloud droplet residuals (62% in summer and 35% in spring) as compared to below-cloud aerosol (53% in summer and 20% in spring), indicating their higher hygroscopicity as compared to fresh Org1 particles, consistent with previous observations.15,83
The differences in the fractional contribution from nitrate-rich particles between below-cloud and cloud droplet residuals were small during spring, but significant during summer, in line with previous studies (e.g., Hayden et al.84). The contribution of IEPOX-SOA particles was significantly larger in cloud droplet residuals as compared to below-cloud aerosol, suggesting that the larger size of cloud droplets facilitated the rapid uptake of IEPOX and hence formation of IEPOX-SOA, as proposed by Tsui et al.74
3.3. Factors driving the variability of the activation fraction
Here, we correlate the flight averaged activation fraction with flight averaged meteorological variables, including T, RH, updraft velocity, as well as aerosol properties, which include below-cloud accumulation mode number concentrations (N100), particle hygroscopicity (κ), and cloud droplet residual diameter. Below-cloud aerosol properties (e.g., hygroscopicity) were consistent during each flight (as illustrated by the small (<10%) relative one-standard deviation in measurements, Fig. 3) to justify the use of flight average. Particle hygroscopicity was assumed to be: 0 for soot and dust,85,86 0.1 for Org1, BB, and BB SOA, 0.2 for Org2 and IEPOX-SOA, and 0.4 for sulfate- and nitrate-rich particles (i.e., 50–50% mix of organics and sulfate/nitrate, estimated based on the measured particle density78). Although soot and dust particles were often mixed with small amounts of hygroscopic sulfate and nitrate components (as evidenced by the particle mass spectra), these particles contributed <5% of particle number concentrations. Consequently, omitting these compounds from the CCN analysis is unlikely to have a significant impact on our results.
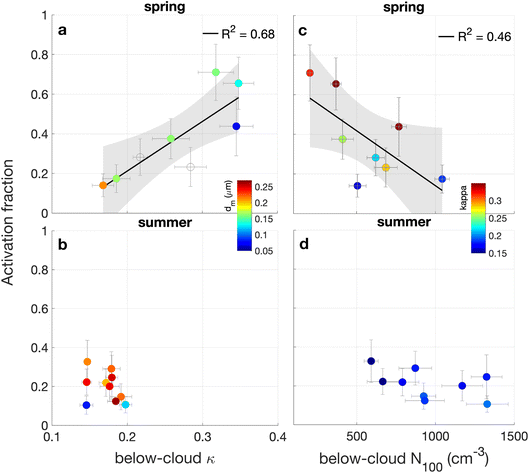 |
| Fig. 3 Scatter plot of 18 flight-averaged activated cloud droplet fraction versus the below-cloud aerosol hygroscopicity for spring (a) and summer (b) and versus below-cloud N100 for spring (c) and summer (d). Datapoints in (a) and (b) are colored by the average mobility diameter of cloud residuals and by the below-cloud aerosol hygroscopicity in (c) and (d). Vertical and horizontal “error” bars are the one-standard deviations from 1 Hz measurements except for hygroscopicity (0.02 Hz). Linear fits and their fit 98% confidence bands are shown. Open circles in (a) indicate flights for which particle size distribution measurements were not available. There were only 9 datapoints in (d) compared to 10 in (b) because we lacked below-cloud aerosol number concentrations information for a single flight during summer. | |
During the spring season, the activation fraction increases linearly (R2 = 0.68) from 0.1 to 0.6 as below-cloud aerosol κ increased from 0.15 (organic-rich aerosol) to 0.35 (mixture of organics and more hygroscopic components, e.g., sulfate), (Fig. 3a). The strong positive correlation shown in Fig. 3a indicates that a larger fraction of the below-cloud accumulation-mode particles activated when these particles were more hygroscopic. In contrast, we observed no correlation between the activation fraction and below-cloud hygroscopicity for summer due to a small dynamic range in below-cloud particle hygroscopicity during summer (κ = 0.17 ± 0.02, Fig. 3b) compared to spring (κ = 0.27 ± 0.07, Fig. 3a). Differences in below-cloud hygroscopicity for the two seasons are attributed to high contribution from organic-rich particles during summer (as shown in Fig. 2). The high biogenic fluxes of organic vapor precursors during summer resulted in more homogeneous aerosol composition. Therefore, assuming a seasonally average κ during summer will likely result in reasonable CCN predictions above SGP,28 however, this assumption clearly breaks down during spring, when we observe significant day-to-day variability in aerosol composition.
It is also clear from Fig. 3a and b that larger particle diameters were required to activate less hygroscopic particles; the average mobility diameter of cloud droplet residuals was dm = 0.15 ± 0.02 μm for all flights with a below-cloud aerosol κ > 0.3 compared to dm = 0.20 ± 0.04 μm for all flights with a below-cloud aerosol κ < 0.2, consistent with previous measurements.87–89
The activation fraction was negatively correlated with N100 during spring (R2 = 0.46, Fig. 3c) but not during summer (Fig. 3d), potentially due to a saturation effect from higher aerosol number concentrations and lower aerosol hygroscopicity measured during the summer campaign as compared to the spring one. The negative correlation shown in Fig. 3c indicates a non-linear relationship between cloud droplet number concentrations and N100,90,91 likely driven by a suppression of cloud supersaturations from increased competition for water vapor between the numerous particles.63,90,92,93 In fact, N100 and calculated effective cloud supersaturation (Section 3.4) were negatively correlated (Fig. S5†). Observed seasonal differences in the activation fraction (0.38 ± 0.21 for spring compared to 0.20 ± 0.08 for summer) cannot be attributed to differences in updraft velocities (Fig. S3b†).
The activation fraction was also negatively correlated with surface temperatures (R2 = 0.67, Fig. S4a†); however, this relationship is largely driven by the strong dependence of κ on temperature (R2 = 0.9, Fig. S4b†). In addition to the effects of below-cloud aerosol hygroscopicity and number concentrations on the activation fraction, entrainment mixing of drier air can completely evaporate cloud droplets near cloud edges94 and decrease the activation fraction.91,92 However, these non-adiabatic effects are beyond the scope of this study.
3.4. Shallow cumuli effective supersaturations
Supersaturation was shown to play a critical role in determining the cloud activation fraction (e.g., Gillani et al.,91 Jia et al.92,95). However, supersaturations are difficult to measure96 and they remain poorly constrained.97 Effective cloud supersaturations have been estimated using a closure between cloud droplet number and CCN measurements at various supersaturations,81,98,99 using a closure between cloud droplet number and CCN predicted from Köhler theory,100,101 or using remote sensing techniques (e.g., Yang et al.97).
Here, we estimate the effective cloud supersaturations using cloud droplet number – CCN closure, with CCN concentrations calculated using κ-Köhler theory and below-cloud aerosol number size distributions, hygroscopicity, and measured real-world mixtures for 12 (out of 17) cloudy flights for which concurrent measurements were available, following the methodology illustrated in Fig. S6.† First, flight averaged CCN concentrations between 0.01 and 0.5% supersaturations (CCN(SS)) are calculated using κ-Köhler theory, in 0.01% increments using the measured real-world mixtures of the different particle clusters identified (i.e., different κ values for the different identified aerosol types). For simplicity, the same below-cloud particle number size distributions were used for all particle types. To account for inherent variability in particle-to-particle composition within each particle class, we accounted for a 20% error in hygroscopicity values and propagated that error in our calculations. Then, the effective cloud supersaturation is estimated as the supersaturation value that minimizes |CCN(SS) − Nd| for each flight, where Nd are the measured flight averaged cloud droplet number concentrations.
Fig. 4 shows that the activation fraction correlated strongly (R2 = 0.9) with calculated effective supersaturations for all cloudy flights (expect one), consistent with previous observations.91,100,102 This relationship accounts for the effects of below-cloud particle κ, size distribution, and real-world mixing state because these quantities are used as inputs to the calculations of supersaturations. The strong correlation between activation fraction and effective cloud supersaturations indicates that individual measurements of particle properties (size and chemical composition) and their variability can be used to accurately describe in situ droplet activation for continental shallow cumuli.
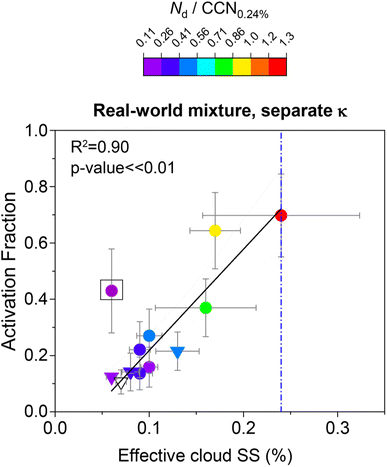 |
| Fig. 4 Flight averaged activation fraction versus calculated effective cloud supersaturation (SS) for real-world mixture of compositions with different κ values for the different aerosol types. Colors represent the ratio of cloud activation fraction to CCNC measured activation fraction at 0.24%, marked by the vertical line. Circle and triangle represent spring and summer, respectively. Linear fit (excluding the highlighted datapoint) is shown as solid black line. Horizontal bars are the sensitivity of retrieved SS to changes in below-cloud number size distributions and hygroscopicity. | |
The average calculated effective cloud supersaturation for the entire HI-SCALE campaign was 0.11 ± 0.05%. Seasonal average effective cloud supersaturation for continental shallow clouds was higher during spring (0.13 ± 0.06%) compared to summer (0.08 ± 0.03%), although the difference was not statistically significant at a 5% threshold on a two-sample t-test.
Most importantly, it is possible to examine the validity of the calculated effective supersaturations by using the CCNC-measured activation fraction at a fixed supersaturation of 0.24%. We expect the ratios of the in-cloud activation fraction to the below-cloud activation fraction measured by CCNC at 0.24% to converge to unity when the calculated effective supersaturation is close to 0.24% and be <1 when it is lower than 0.24%, which are indicated in Fig. 4 by the color of the datapoints. Using supersaturations calculated with measured below-cloud size, composition, and real-world mixture information, we find that all datapoints in Fig. 4 with calculated SS <0.24% have measured Nd/CCN0.24% ratios <1, and when the calculated effective maximum supersaturation was in the vicinity of 0.24%, that ratio was ∼1.2.
4 Conclusions
We present measurements taken during the spring and summer of 2016 as part of the HI-SCALE field campaign. The size and composition of individual particles sampled below-cloud and behind a CVI inlet (cloud droplet residuals) were characterized using the single particle mass spectrometer, miniSPLAT. Below-cloud aerosol information was used to investigate aerosol activation without invoking simplifying assumptions regarding aerosol composition and mixing state. Cloud droplet residuals of shallow cumuli were larger and more hygroscopic than below-cloud particles, consistent with Köhler activation theory and the aqueous chemistry in-cloud droplets (i.e., sulfate and IEPOX-SOA formation). For flight-averaged data, below-cloud aerosol composition, size, and number concentrations were correlated with shallow cumuli activation fraction. Furthermore, clear seasonal differences in the aerosol activation fraction (0.38 ± 0.21 for spring and 0.20 ± 0.08 for summer) over the SGP were observed, consistent with high biogenic surface flux emissions that drive the formation and growth of organic components during summer. The seasonal difference in activation fraction is the result of lower aerosol hygroscopicity (κ = 0.17 ± 0.02 for summer and 0.27 ± 0.07 for spring) and higher accumulation-mode particle concentrations (841 ± 313 cm−3 for summer and 535 ± 266 cm−3 for spring) measured during summer that lower effective cloud supersaturation. Using a closure between cloud droplet number concentrations and CCN predicted using κ-Kõhler and below-cloud measurement-constrained aerosol properties and their mixing state yielded effective supersaturations of shallow cumuli of 0.13 + 0.06% during spring and 0.08 + 0.03% during summer. The calculated supersaturations were validated by in situ CCNC-measurements at 0.24%. This study highlights the importance of individual particle measurements of size and composition, to accurately represent their activation into shallow cumuli cloud droplets, even at a background site like SGP.
Data availability
Data used in this manuscript are available from the ARM data archive (https://adc.arm.gov/discovery/).
Author contributions
DB, KS, AZ, GK, FM, MP, JW: data acquisition, analyses; GS, AZ: data analysis and writing original draft. All authors: writing – review and editing.
Conflicts of interest
There are no conflicts of interest to declare.
Acknowledgements
This work was supported by the U.S. Department of Energy (DOE), Office of Science, Biological, and Environmental Research's Atmospheric System Research (ASR) program. The HI-SCALE field campaign was supported by the Atmospheric Radiation Measurement (ARM) Climate Research Facility and the Environmental Molecular Science Laboratory (EMSL), both are U.S. Department of Energy (DOE) Office of Science User Facilities sponsored by the Office of Biological and Environmental Research. The Pacific Northwest National Laboratory is operated for DOE by Battelle Memorial Institute under contract DE-AC06-76RL01830. The authors thank the crews of G-1 aircrafts, and the entire HI-SCALE team for their help during the field campaign.
References
- B. A. Wielicki and R. M. Welch, Cumulus Cloud Properties Derived Using Landsat Satellite Data, J. Appl. Meteorol. Climatol., 1986, 25, 261–276 CrossRef.
- D. L. Hartmann, M. E. Ockert-Bell and M. L. Michelsen, The Effect of Cloud Type on Earth's Energy Balance: Global Analysis, J. Clim., 1992, 5, 1281–1304 CrossRef.
- L. K. Berg, C. M. Berkowitz, J. C. Barnard, G. Senum and S. R. Springston, Observations of the first aerosol indirect effect in shallow cumuli, Geophys. Res. Lett., 2011, 38, L03809 CrossRef.
- J. D. Fast, L. K. Berg, L. Alexander, D. Bell, E. D'Ambro, J. Hubbe, C. Kuang, J. Liu, C. Long, A. Matthews, F. Mei, R. Newsom, M. Pekour, T. Pinterich, B. Schmid, S. Schobesberger, J. Shilling, J. N. Smith, S. Springston, K. Suski, J. A. Thornton, J. Tomlinson, J. Wang, H. Xiao and A. Zelenyuk, Overview of the HI-SCALE Field Campaign: A New Perspective on Shallow Convective Clouds, Bull. Am. Meteorol. Soc., 2019, 100, 821–840 Search PubMed.
- M. A. LeMone and W. T. Pennell, The Relationship of Trade Wind Cumulus Distribution to Subcloud Layer Fluxes and Structure, Mon. Weather Rev., 1976, 104, 524–539 CrossRef.
- A. M. Vogelmann, G. M. McFarquhar, J. A. Ogren, D. D. Turner, J. M. Comstock, G. Feingold, C. N. Long, H. H. Jonsson, A. Bucholtz, D. R. Collins, G. S. Diskin, H. Gerber, R. P. Lawson, R. K. Woods, E. Andrews, H.-J. Yang, J. C. Chiu, D. Hartsock, J. M. Hubbe, C. Lo, A. Marshak, J. W. Monroe, S. A. McFarlane, B. Schmid, J. M. Tomlinson and T. Toto, RACORO Extended-Term Aircraft Observations of Boundary Layer Clouds, Bull. Am. Meteorol. Soc., 2012, 93, 861–878 CrossRef.
- C. P. Weaver and R. Avissar, Atmospheric Disturbances Caused by Human Modification of the Landscape, Bull. Am. Meteorol. Soc., 2001, 82, 269–282 CrossRef.
- G. Feingold, W. L. Eberhard, D. E. Veron and M. Previdi, First measurements of the Twomey indirect effect using ground-based remote sensors, Geophys. Res. Lett., 2003, 30(6), 1287 CrossRef.
- B.-G. Kim, S. E. Schwartz, M. A. Miller and Q. Min, Effective radius of cloud droplets by ground-based remote sensing: Relationship to aerosol, J. Geophys. Res.: Atmos., 2003, 108(D23), 4740 CrossRef.
- M.-L. Lu, G. Feingold, H. H. Jonsson, P. Y. Chuang, H. Gates, R. C. Flagan and J. H. Seinfeld, Aerosol-cloud relationships in continental shallow cumulus, J. Geophys. Res.: Atmos., 2008, 113, D15201 CrossRef.
- F. Werner, F. Ditas, H. Siebert, M. Simmel, B. Wehner, P. Pilewskie, T. Schmeissner, R. A. Shaw, S. Hartmann, H. Wex, G. C. Roberts and M. Wendisch, Twomey effect observed from collocated microphysical and remote sensing measurements over shallow cumulus, J. Geophys. Res.: Atmos., 2014, 119, 1534–1545 CrossRef.
- R. B. Seigel, Shallow Cumulus Mixing and Subcloud-Layer Responses to Variations in Aerosol Loading, J. Atmos. Sci., 2014, 71, 2581–2603 CrossRef.
- B. Stevens and G. Feingold, Untangling aerosol effects on clouds and precipitation in a buffered system, Nature, 2009, 461, 607–613 CrossRef CAS PubMed.
- J. H. Seinfeld, C. Bretherton, K. S. Carslaw, H. Coe, P. J. DeMott, E. J. Dunlea, G. Feingold, S. Ghan, A. B. Guenther, R. Kahn, I. Kraucunas, S. M. Kreidenweis, M. J. Molina, A. Nenes, J. E. Penner, K. A. Prather, V. Ramanathan, V. Ramaswamy, P. J. Rasch, A. R. Ravishankara, D. Rosenfeld, G. Stephens and R. Wood, Improving our fundamental understanding of the role of aerosol−cloud interactions in the climate system, Proc. Natl. Acad. Sci., 2016, 113, 5781–5790 CrossRef CAS PubMed.
- P. Massoli, A. T. Lambe, A. T. Ahern, L. R. Williams, M. Ehn, J. Mikkilä, M. R. Canagaratna, W. H. Brune, T. B. Onasch, J. T. Jayne, T. Petäjä, M. Kulmala, A. Laaksonen, C. E. Kolb, P. Davidovits and D. R. Worsnop, Relationship between aerosol oxidation level and hygroscopic properties of laboratory generated secondary organic aerosol (SOA) particles, Geophys. Res. Lett., 2010, 37, L24801 CrossRef.
- W. Li and L. Shao, Transmission electron microscopy study of aerosol particles from the brown hazes in northern China, J. Geophys. Res.: Atmos., 2009, 114, D09302 Search PubMed.
- A. Laskin, J. P. Cowin and M. J. Iedema, Analysis of individual environmental particles using modern methods of electron microscopy and X-ray microanalysis, J. Electron Spectrosc. Relat. Phenom., 2006, 150, 260–274 CrossRef CAS.
- R. M. Healy, J. Sciare, L. Poulain, M. Crippa, A. Wiedensohler, A. S. H. Prévôt, U. Baltensperger, R. Sarda-Estève, M. L. McGuire, C. H. Jeong, E. McGillicuddy, I. P. O'Connor, J. R. Sodeau, G. J. Evans and J. C. Wenger, Quantitative determination of carbonaceous particle mixing state in Paris using single-particle mass spectrometer and aerosol mass spectrometer measurements, Atmos. Chem. Phys., 2013, 13, 9479–9496 CrossRef.
- R. C. Moffet and K. A. Prather, In-situ measurements of the mixing state and optical properties of soot with implications for radiative forcing estimates, Proc. Natl. Acad. Sci., 2009, 106, 11872–11877 CrossRef CAS PubMed.
- S. Takahama, S. Gilardoni, L. M. Russell and A. L. D. Kilcoyne, Classification of multiple types of organic carbon composition in atmospheric particles by scanning transmission X-ray microscopy analysis, Atmos. Environ., 2007, 41, 9435–9451 CrossRef CAS.
- A. Zelenyuk, D. Imre, M. Earle, R. Easter, A. Korolev, R. Leaitch, P. Liu, A. M. Macdonald, M. Ovchinnikov and W. Strapp, In Situ Characterization of Cloud Condensation Nuclei, Interstitial, and Background Particles Using the Single Particle Mass Spectrometer, SPLAT II, Anal. Chem., 2010, 82, 7943–7951 CrossRef CAS PubMed.
- J. Ching, K. Adachi, Y. Zaizen, Y. Igarashi and M. Kajino, Aerosol mixing state revealed by transmission electron microscopy pertaining to cloud formation and human airway deposition, npj Clim. Atmos. Sci., 2019, 2, 22 CrossRef.
- R. H. Moore, R. Bahreini, C. A. Brock, K. D. Froyd, J. Cozic, J. S. Holloway, A. M. Middlebrook, D. M. Murphy and A. Nenes, Hygroscopicity and composition of Alaskan Arctic CCN during April 2008, Atmos. Chem. Phys., 2011, 11, 11807–11825 CrossRef CAS.
- G. Roberts, G. Mauger, O. Hadley and V. Ramanathan, North American and Asian aerosols over the eastern Pacific Ocean and their role in regulating cloud condensation nuclei, J. Geophys. Res.: Atmos., 2006, 111, D13205 CrossRef.
- T. M. VanReken, T. A. Rissman, G. C. Roberts, V. Varutbangkul, H. H. Jonsson, R. C. Flagan and J. H. Seinfeld, Toward aerosol/cloud condensation nuclei (CCN) closure during CRYSTAL-FACE, J. Geophys. Res.: Atmos., 2003, 108(D20), 4633 CrossRef.
- S. Lance, A. Nenes, C. Mazzoleni, M. K. Dubey, H. Gates, V. Varutbangkul, T. A. Rissman, S. M. Murphy, A. Sorooshian, R. C. Flagan, J. H. Seinfeld, G. Feingold and H. H. Jonsson, Cloud condensation nuclei activity, closure, and droplet growth kinetics of Houston aerosol during the Gulf of Mexico Atmospheric Composition and Climate Study (GoMACCS), J. Geophys. Res.: Atmos., 2009, 114, D00F15 CrossRef.
- A. Asa-Awuku, R. H. Moore, A. Nenes, R. Bahreini, J. S. Holloway, C. A. Brock, A. M. Middlebrook, T. B. Ryerson, J. L. Jimenez, P. F. DeCarlo, A. Hecobian, R. J. Weber, R. Stickel, D. J. Tanner and L. G. Huey, Airborne cloud condensation nuclei measurements during the 2006 Texas Air Quality Study, J. Geophys. Res.: Atmos., 2011, 116, D11201 CrossRef.
- G. Kulkarni, F. Mei, J. E. Shilling, J. Wang, R. P. Reveggino, C. Flynn, A. Zelenyuk and J. Fast, Cloud Condensation Nuclei Closure Study Using Airborne Measurements Over the Southern Great Plains, J. Geophys. Res.: Atmos., 2023, 128, e2022JD037964 CrossRef.
- N. Riemer, A. P. Ault, M. West, R. L. Craig and J. H. Curtis, Aerosol Mixing State: Measurements, Modeling, and Impacts, Rev. Geophys., 2019, 57, 187–249 CrossRef.
- F. Zhang, Y. Wang, J. Peng, J. Ren, D. Collins, R. Zhang, Y. Sun, X. Yang and Z. Li, Uncertainty in Predicting CCN Activity of Aged and Primary Aerosols, J. Geophys. Res.: Atmos., 2017, 122, 11723–11736 CrossRef.
- J. Ren, F. Zhang, Y. Wang, D. Collins, X. Fan, X. Jin, W. Xu, Y. Sun, M. Cribb and Z. Li, Using different assumptions of aerosol mixing state and chemical composition to predict CCN concentrations based on field measurements in urban Beijing, Atmos. Chem. Phys., 2018, 18, 6907–6921 CrossRef CAS.
- J. Ching, J. Fast, M. West and N. Riemer, Metrics to quantify the importance of mixing state for CCN activity, Atmos. Chem. Phys., 2017, 17, 7445–7458 CrossRef CAS.
- L. Fierce, N. Riemer and T. C. Bond, Toward Reduced Representation of Mixing State for Simulating Aerosol Effects on Climate, Bull. Am. Meteorol. Soc., 2017, 98, 971–980 Search PubMed.
- M. Mahish, A. Jefferson and D. R. Collins, Influence of Common Assumptions Regarding Aerosol Composition and Mixing State on Predicted CCN Concentration, Atmosphere, 2018, 9, 54 CrossRef.
- K. Broekhuizen, R. Y. W. Chang, W. R. Leaitch, S. M. Li and J. P. D. Abbatt, Closure between measured and modeled cloud condensation nuclei (CCN) using size-resolved aerosol compositions in downtown Toronto, Atmos. Chem. Phys., 2006, 6, 2513–2524 CrossRef CAS.
- G. Kulkarni, F. Mei, J. Shilling, J. Wang, R. P. Reveggino, C. Flynn, A. Zelenyuk and J. Fast, loud Condensation Nuclei Closure Study Using Airborne Measurements over the Southern Great Plains, J. Geophys. Res.: Atmos., 2023, e2022JD037964 CrossRef.
- L. T. Padró, R. H. Moore, X. Zhang, N. Rastogi, R. J. Weber and A. Nenes, Mixing state and compositional effects on CCN activity and droplet growth kinetics of size-resolved CCN in an urban environment, Atmos. Chem. Phys., 2012, 12, 10239–10255 CrossRef.
- H. Furutani, M. Dall’osto, G. C. Roberts and K. A. Prather, Assessment of the relative importance of atmospheric aging on CCN activity derived from field observations, Atmos. Environ., 2008, 42, 3130–3142 CrossRef CAS.
- U. Dusek, G. P. Frank, L. Hildebrandt, J. Curtius, J. Schneider, S. Walter, D. Chand, F. Drewnick, S. Hings, D. Jung, S. Borrmann and M. O. Andreae, Size Matters More Than Chemistry for Cloud-Nucleating Ability of Aerosol Particles, Science, 2006, 312, 1375–1378 CrossRef CAS PubMed.
- D. Rose, A. Nowak, P. Achtert, A. Wiedensohler, M. Hu, M. Shao, Y. Zhang, M. O. Andreae and U. Pöschl, Cloud condensation nuclei in polluted air and biomass burning smoke near the mega-city Guangzhou, China – Part 1: Size-resolved measurements and implications for the modeling of aerosol particle hygroscopicity and CCN activity, Atmos. Chem. Phys., 2010, 10, 3365–3383 CrossRef.
- Z. Jurányi, M. Gysel, E. Weingartner, P. F. DeCarlo, L. Kammermann and U. Baltensperger, Measured and modelled cloud condensation nuclei number concentration at the high alpine site Jungfraujoch, Atmos. Chem. Phys., 2010, 10, 7891–7906 CrossRef.
- B. Ervens, M. J. Cubison, E. Andrews, G. Feingold, J. A. Ogren, J. L. Jimenez, P. K. Quinn, T. S. Bates, J. Wang, Q. Zhang, H. Coe, M. Flynn and J. D. Allan, CCN predictions using simplified assumptions of organic aerosol composition and mixing state: a synthesis from six different locations, Atmos. Chem. Phys., 2010, 10, 4795–4807 CrossRef CAS.
- S. Lance, T. Raatikainen, T. B. Onasch, D. R. Worsnop, X. Y. Yu, M. L. Alexander, M. R. Stolzenburg, P. H. McMurry, J. N. Smith and A. Nenes, Aerosol mixing state, hygroscopic growth and cloud activation efficiency during MIRAGE 2006, Atmos. Chem. Phys., 2013, 13, 5049–5062 CrossRef CAS.
- M. J. Cubison, B. Ervens, G. Feingold, K. S. Docherty, I. M. Ulbrich, L. Shields, K. Prather, S. Hering and J. L. Jimenez, The influence of chemical composition and mixing state of Los Angeles urban aerosol on CCN number and cloud properties, Atmos. Chem. Phys., 2008, 8, 5649–5667 CrossRef CAS.
- J. Wang, M. J. Cubison, A. C. Aiken, J. L. Jimenez and D. R. Collins, The importance of aerosol mixing state and size-resolved composition on CCN concentration and the variation of the importance with atmospheric aging of aerosols, Atmos. Chem. Phys., 2010, 10, 7267–7283 CrossRef CAS.
- G. C. Roberts, D. A. Day, L. M. Russell, E. J. Dunlea, J. L. Jimenez, J. M. Tomlinson, D. R. Collins, Y. Shinozuka and A. D. Clarke, Characterization of particle cloud droplet activity and composition in the free troposphere and the boundary layer during INTEX-B, Atmos. Chem. Phys., 2010, 10, 6627–6644 CrossRef CAS.
- A. Zelenyuk, D. Imre, J. Wilson, Z. Zhang, J. Wang and K. Mueller, Airborne single particle mass spectrometers (SPLAT II & miniSPLAT) and new software for data visualization and analysis in a geo-spatial context, J. Am. Soc. Mass Spectrom., 2015, 26, 257–270 CrossRef CAS PubMed.
- C. H. Twohy and J. R. Anderson, Droplet nuclei in non-precipitating clouds: composition and size matter, Environ. Res. Lett., 2008, 3, 045002 CrossRef.
- A. Wonaschuetz, A. Sorooshian, B. Ervens, P. Y. Chuang, G. Feingold, S. M. Murphy, J. de Gouw, C. Warneke and H. H. Jonsson, Aerosol and gas re-distribution by shallow cumulus clouds: An investigation using airborne measurements, J. Geophys. Res.: Atmos., 2012, 117, D17202 CrossRef.
- A. Marshak, A. Davis, W. Wiscombe and R. Cahalan, Radiative effects of sub-mean free path liquid water variability observed in stratiform clouds, J. Geophys. Res.: Atmos., 1998, 103, 19557–19567 CrossRef.
- L. K. Berg and E. I. Kassianov, Temporal Variability of Fair-Weather Cumulus Statistics at the ACRF SGP Site, J. Clim., 2008, 21, 3344–3358 CrossRef.
- G. M. McFarquhar, S. Platnick, L. Di Girolamo, H. Wang, G. Wind and G. Zhao, Trade wind cumuli statistics in clean and polluted air over the Indian Ocean from in situ and remote sensing measurements, Geophys. Res. Lett., 2004, 31, L21105 CrossRef.
- D. L. Sisterson, R. A. Peppler, T. S. Cress, P. J. Lamb and D. D. Turner, The ARM Southern Great Plains (SGP) Site, Meteorol. Monogr., 2016, 57, 6.1–6.14 CrossRef.
- B. Schmid, J. M. Tomlinson, J. M. Hubbe, J. M. Comstock, F. Mei, D. Chand, M. S. Pekour, C. D. Kluzek, E. Andrews, S. C. Biraud and G. M. McFarquhar, The DOE ARM Aerial Facility, Bull. Am. Meteorol. Soc., 2014, 95, 723–742 CrossRef.
- K. J. Noone, J. A. Ogren, J. Heintzenberg, R. J. Charlson and D. S. Covert, Design and Calibration of a Counterflow Virtual Impactor for Sampling of Atmospheric Fog and Cloud Droplets, Aerosol Sci. Technol., 1988, 8, 235–244 CrossRef CAS.
- J. Wang, P. H. Daum, S. S. Yum, Y. Liu, G. I. Senum, M.-L. Lu, J. H. Seinfeld and H. Jonsson, Observations of marine stratocumulus microphysics and implications for processes controlling droplet spectra: Results from the Marine Stratus/Stratocumulus Experiment, J. Geophys. Res.: Atmos., 2009, 114, D18210 CrossRef.
- H. Akagawa and K. Okada, Sizes of cloud droplets and cloud droplet residues near stratus cloud base, Atmos. Res., 1993, 30, 37–49 CrossRef.
- J. Wang, M. Pikridas, S. R. Spielman and T. Pinterich, A fast integrated mobility spectrometer for rapid measurement of sub-micrometer aerosol size distribution, Part I: Design and model evaluation, J. Aerosol Sci., 2017, 108, 44–55 CrossRef CAS.
- G. C. Roberts and A. Nenes, A Continuous-Flow Streamwise Thermal-Gradient CCN Chamber for Atmospheric Measurements, Aerosol Sci. Technol., 2005, 39, 206–221 CrossRef CAS.
- C. D. Hatch, K. M. Gierlus, J. D. Schuttlefield and V. H. Grassian, Water adsorption and cloud condensation nuclei activity of calcite and calcite coated with model humic and fulvic acids, Atmos. Environ., 2008, 42, 5672–5684 CrossRef CAS.
- D. Vu, S. Gao, T. Berte, M. Kacarab, Q. Yao, K. Vafai and A. Asa-Awuku, External and internal cloud condensation nuclei (CCN) mixtures: controlled laboratory studies of varying mixing states, Atmos. Meas. Tech., 2019, 12, 4277–4289 CrossRef CAS.
- S. S. Gunthe, S. M. King, D. Rose, Q. Chen, P. Roldin, D. K. Farmer, J. L. Jimenez, P. Artaxo, M. O. Andreae, S. T. Martin and U. Pöschl, Cloud condensation nuclei in pristine tropical rainforest air of Amazonia: size-resolved measurements and modeling of atmospheric aerosol composition and CCN activity, Atmos. Chem. Phys., 2009, 9, 7551–7575 CrossRef CAS.
- F. Zhang, Y. Li, Z. Li, L. Sun, R. Li, C. Zhao, P. Wang, Y. Sun, X. Liu, J. Li, P. Li, G. Ren and T. Fan, Aerosol hygroscopicity and cloud condensation nuclei activity during the AC3Exp campaign: implications for cloud condensation nuclei parameterization, Atmos. Chem. Phys., 2014, 14, 13423–13437 CrossRef.
- J. Ren, L. Chen, T. Fan, J. Liu, S. Jiang and F. Zhang, The NPF Effect on CCN Number Concentrations: A Review and Re-Evaluation of Observations From 35 Sites Worldwide, Geophys. Res. Lett., 2021, 48, e2021GL095190 Search PubMed.
- A. Zelenyuk, D. Imre, Y. Cai, K. Mueller, Y. Han and P. Imrich, SpectraMiner, an interactive data mining and visualization software for single particle mass spectroscopy: A laboratory test case, Int. J. Mass Spectrom., 2006, 258, 58–73 CrossRef CAS.
- M. Riva, D. M. Bell, A.-M. K. Hansen, G. T. Drozd, Z. Zhang, A. Gold, D. Imre, J. D. Surratt, M. Glasius and A. Zelenyuk, Effect of Organic Coatings, Humidity and Aerosol Acidity on Multiphase Chemistry of Isoprene Epoxydiols, Environ. Sci. Technol., 2016, 50, 5580–5588 CrossRef CAS PubMed.
- D. S. Kosyakov, N. V. Ul’yanovskii, T. B. Latkin, S. A. Pokryshkin, V. R. Berzhonskis, O. V. Polyakova and A. T. Lebedev, Peat burning – An important source of pyridines in the earth atmosphere, Environ. Pollut., 2020, 266, 115109 CrossRef CAS PubMed.
- A. Laskin, J. S. Smith and J. Laskin, Molecular Characterization of Nitrogen-Containing Organic Compounds in Biomass Burning Aerosols Using High-Resolution Mass Spectrometry, Environ. Sci. Technol., 2009, 43, 3764–3771 CrossRef CAS PubMed.
- D. M. Murphy, D. J. Cziczo, K. D. Froyd, P. K. Hudson, B. M. Matthew, A. M. Middlebrook, R. E. Peltier, A. Sullivan, D. S. Thomson and R. J. Weber, Single-particle mass spectrometry of tropospheric aerosol particles, J. Geophys. Res.: Atmos., 2006, 111, D23S32 Search PubMed.
- C. Frege, F. Bianchi, U. Molteni, J. Tröstl, H. Junninen, S. Henne, M. Sipilä, E. Herrmann, M. J. Rossi, M. Kulmala, C. R. Hoyle, U. Baltensperger and J. Dommen, Chemical characterization of atmospheric ions at the high altitude research station Jungfraujoch (Switzerland), Atmos. Chem. Phys., 2017, 17, 2613–2629 CrossRef CAS.
- P. Schulte and F. Arnold, Pyridinium ions and pyridine in the free troposphere, Geophys. Res. Lett., 1990, 17, 1077–1080 CrossRef CAS.
- M. D. Petters and S. M. Kreidenweis, A single parameter representation of hygroscopic growth and cloud condensation nucleus activity, Atmos. Chem. Phys., 2007, 7, 1961–1971 CrossRef CAS.
- Y. B. Lim, Y. Tan, M. J. Perri, S. P. Seitzinger and B. J. Turpin, Aqueous chemistry and its role in secondary organic aerosol (SOA) formation, Atmos. Chem. Phys., 2010, 10, 10521–10539 CrossRef CAS.
- W. G. Tsui, J. L. Woo and V. F. McNeill, Impact of Aerosol-Cloud Cycling on Aqueous Secondary Organic Aerosol Formation, Atmosphere, 2019, 10, 666 CrossRef CAS.
- B.-G. Kim, M. A. Miller, S. E. Schwartz, Y. Liu and Q. Min, The role of adiabaticity in the aerosol first indirect effect, J. Geophys. Res.: Atmos., 2008, 113, D05210 CrossRef.
- B. N. Duncan, Y. Yoshida, M. R. Damon, A. R. Douglass and J. C. Witte, Temperature dependence of factors controlling isoprene emissions, Geophys. Res. Lett., 2009, 36, L05813 CrossRef.
- J. Liu, L. Alexander, J. D. Fast, R. Lindenmaier and J. E. Shilling, Aerosol characteristics at the Southern Great Plains site during the HI-SCALE campaign, Atmos. Chem. Phys., 2021, 21, 5101–5116 CrossRef CAS.
- T. D. Vaden, D. Imre, J. Beránek and A. Zelenyuk, Extending the Capabilities of Single Particle Mass Spectrometry: II. Measurements of Aerosol Particle Density without DMA, Aerosol Sci. Technol., 2011, 45, 125–135 CrossRef CAS.
- C. Parworth, J. Fast, F. Mei, T. Shippert, C. Sivaraman, A. Tilp, T. Watson and Q. Zhang, Long-term measurements of submicrometer aerosol chemistry at the Southern Great Plains (SGP) using an Aerosol Chemical Speciation Monitor (ACSM), Atmos. Environ., 2015, 106, 43–55 CrossRef CAS.
- J. D. Surratt, M. Lewandowski, J. H. Offenberg, M. Jaoui, T. E. Kleindienst, E. O. Edney and J. H. Seinfeld, Effect of Acidity on Secondary Organic Aerosol Formation from Isoprene, Environ. Sci. Technol., 2007, 41, 5363–5369 CrossRef CAS PubMed.
- K. J. Sanchez, L. M. Russell, R. L. Modini, A. A. Frossard, L. Ahlm, C. E. Corrigan, G. C. Roberts, L. N. Hawkins, J. C. Schroder, A. K. Bertram, R. Zhao, A. K. Y. Lee, J. J. Lin, A. Nenes, Z. Wang, A. Wonaschütz, A. Sorooshian, K. J. Noone, H. Jonsson, D. Toom, A. M. Macdonald, W. R. Leaitch and J. H. Seinfeld, Meteorological and aerosol effects on marine cloud microphysical properties, J. Geophys. Res.: Atmos., 2016, 121, 4142–4161 CrossRef.
- K. Sellegri, P. Laj, R. Dupuy, M. Legrand, S. Preunkert and J.-P. Putaud, Size-dependent scavenging efficiencies of multicomponent atmospheric aerosols in clouds, J. Geophys. Res.: Atmos., 2003, 108(D11), 4334 CrossRef.
- J. Duplissy, P. F. DeCarlo, J. Dommen, M. R. Alfarra, A. Metzger, I. Barmpadimos, A. S. H. Prevot, E. Weingartner, T. Tritscher, M. Gysel, A. C. Aiken, J. L. Jimenez, M. R. Canagaratna, D. R. Worsnop, D. R. Collins, J. Tomlinson and U. Baltensperger, Relating hygroscopicity and composition of organic aerosol particulate matter, Atmos. Chem. Phys., 2011, 11, 1155–1165 CrossRef CAS.
- K. L. Hayden, A. M. Macdonald, W. Gong, D. Toom-Sauntry, K. G. Anlauf, A. Leithead, S.-M. Li, W. R. Leaitch and K. Noone, Cloud processing of nitrate, J. Geophys. Res.: Atmos., 2008, 113, D18201 CrossRef.
- G. Saliba, C. L. Chen, S. Lewis, L. M. Russell, P. K. Quinn, T. S. Bates, T. G. Bell, M. J. Lawler, E. S. Saltzman, K. J. Sanchez, R. Moore, M. Shook, L. H. Rivellini, A. Lee, N. Baetge, C. A. Carlson and M. J. Behrenfeld, Seasonal Differences and Variability of Concentrations, Chemical Composition, and Cloud Condensation Nuclei of Marine Aerosol Over the North Atlantic, J. Geophys. Res.: Atmos., 2020, 125, e2020JD033145 CAS.
- Z. Shi, D. Zhang, M. Hayashi, H. Ogata, H. Ji and W. Fujiie, Influences of sulfate and nitrate on the hygroscopic behaviour of coarse dust particles, Atmos. Environ., 2008, 42, 822–827 CrossRef CAS.
- S. Mertes, K. Lehmann, A. Nowak, A. Massling and A. Wiedensohler, Link between aerosol hygroscopic growth and droplet activation observed for hill-capped clouds at connected flow conditions during FEBUKO, Atmos. Environ., 2005, 39, 4247–4256 CrossRef CAS.
- B. Svenningsson, H.-C. Hansson, B. Martinsson, A. Wiedensohler, E. Swietlicki, S.-I. Cederfelt, M. Wendisch, K. N. Bower, T. W. Choularton and R. N. Colvile, Cloud droplet nucleation scavenging in relation to the size and hygroscopic behaviour of aerosol particles, Atmos. Environ., 1997, 31, 2463–2475 CrossRef CAS.
- H. Lihavainen, V. M. Kerminen, M. Komppula, A. P. Hyvärinen, J. Laakia, S. Saarikoski, U. Makkonen, N. Kivekäs, R. Hillamo, M. Kulmala and Y. Viisanen, Measurements of the relation between aerosol properties and microphysics and chemistry of low level liquid water clouds in Northern Finland, Atmos. Chem. Phys., 2008, 8, 6925–6938 CrossRef CAS.
- W. R. Leaitch, J. W. Strapp, G. A. Isaac and J. G. Hudson, Cloud droplet nucleation and cloud scavenging of aerosol sulphate in polluted atmospheres, Tellus B, 1986, 38, 328–344 CrossRef.
- N. V. Gillani, S. E. Schwartz, W. R. Leaitch, J. W. Strapp and G. A. Isaac, Field observations in continental stratiform clouds: Partitioning of cloud particles between droplets
and unactivated interstitial aerosols, J. Geophys. Res.: Atmos., 1995, 100, 18687–18706 CrossRef.
- H. Jia, X. Ma and Y. Liu, Exploring aerosol–cloud interaction using VOCALS-REx aircraft measurements, Atmos. Chem. Phys., 2019, 19, 7955–7971 CrossRef CAS.
- Y. Liu and P. H. Daum, Indirect warming effect from dispersion forcing, Nature, 2002, 419, 580–581 CrossRef CAS PubMed.
- F. Burnet and J.-L. Brenguier, Observational Study of the Entrainment-Mixing Process in Warm Convective Clouds, J. Atmos. Sci., 2007, 64, 1995–2011 CrossRef.
- H. Jia, X. Ma, F. Yu, Y. Liu and Y. Yin, Distinct Impacts of Increased Aerosols on Cloud Droplet Number Concentration of Stratus/Stratocumulus and Cumulus, Geophys. Res. Lett., 2019, 46, 13517–13525 CrossRef.
- H. Siebert and R. A. Shaw, Supersaturation Fluctuations during the Early Stage of Cumulus Formation, J. Atmos. Sci., 2017, 74, 975–988 CrossRef.
- F. Yang, R. McGraw, E. P. Luke, D. Zhang, P. Kollias and A. M. Vogelmann, A new approach to estimate supersaturation fluctuations in stratocumulus cloud using ground-based remote-sensing measurements, Atmos. Meas. Tech., 2019, 12, 5817–5828 CrossRef.
- K. J. Sanchez, G. C. Roberts, G. Saliba, L. M. Russell, C. Twohy, J. M. Reeves, R. S. Humphries, M. D. Keywood, J. P. Ward and I. M. McRobert, Measurement report: Cloud processes and the transport of biological emissions affect southern ocean particle and cloud condensation nuclei concentrations, Atmos. Chem. Phys., 2021, 21, 3427–3446 CrossRef CAS.
- S. S. Yum, J. G. Hudson and Y. Xie, Comparisons of cloud microphysics with cloud condensation nuclei spectra over the summertime Southern Ocean, J. Geophys. Res.: Atmos., 1998, 103, 16625–16636 CrossRef CAS.
- F. Ditas, R. A. Shaw, H. Siebert, M. Simmel, B. Wehner and A. Wiedensohler, Aerosols-cloud microphysics-thermodynamics-turbulence: evaluating supersaturation in a marine stratocumulus cloud, Atmos. Chem. Phys., 2012, 12, 2459–2468 CrossRef CAS.
- C. Shen, C. Zhao, N. Ma, J. Tao, G. Zhao, Y. Yu and Y. Kuang, Method to Estimate Water Vapor Supersaturation in the Ambient Activation Process Using Aerosol and Droplet Measurement Data, J. Geophys. Res.: Atmos., 2018, 123, 10606–10619 Search PubMed.
- N. Moteki, T. Mori, H. Matsui and S. Ohata, Observational constraint of in-cloud supersaturation for simulations of aerosol rainout in atmospheric models, npj Clim. Atmos. Sci., 2019, 2, 6 CrossRef.
Footnotes |
† Electronic supplementary information (ESI) available. See DOI: https://doi.org/10.1039/d3ea00052d |
‡ Present address: California Air Resources Board, Sacramento, CA, USA. |
§ Present address: Paul Scherrer Institute, Villigen, Switzerland. |
¶ Present address: Juul Labs, San Francisco, CA, USA. |
|
This journal is © The Royal Society of Chemistry 2023 |