Impact of COVID-19 lockdown on particulate matter oxidative potential at urban background versus traffic sites†
Received
23rd January 2023
, Accepted 27th March 2023
First published on 11th April 2023
Abstract
In Europe, COVID-19 lockdown restrictions were first implemented in March 2020 to control the spread of the disease from the SARS-CoV-2 virus. Many studies have focused on the influence of the applied measures on pollution levels during this period, but very limited information on the oxidative potential (OP), an emerging metric of particulate matter (PM) exposure. Furthermore, most previous studies also commonly used comparative methods with historical datasets, which may not be estimating the real pollution levels without the lockdown restrictions in place. In this study, the OP of PM collected at urban background (Grenoble, France) and traffic (Bern, Switzerland) sites was assessed using dithiothreitol (DTT) and ascorbic acid (AA) assays. These measurements were also compared with PM and black carbon (BC) mass concentrations, including the wood burning and fossil fuel fractions of BC. To obtain a more realistic pollution level, assuming there were no lockdown restrictions in place, a machine learning technique called the Random Forest (RF) regression model was applied to predict a business-as-usual (BAU) level for OP, PM, and BC in both sites. This model provided a good estimate of the BAU levels, allowing a more realistic assessment of the pollution changes during the lockdown period. The results indicate a clear decrease in OP found in the traffic site, while a more modest change in OP was found at the urban background site, likely due to sustained contributions from wood burning sources for residential heating. Overall, this study confirms the major roles of both of these combustion sources in the OP levels in ambient air.
Environmental significance
COVID-19 lockdown restrictions have provided a rare opportunity to assess air quality during a window with significantly reduced human-led activities. Compared to other pollutants, very limited work has been done on the oxidative potential (OP) of particulate matter (PM), an emerging metric of PM exposure. Most studies used comparative methods with historical datasets, which may be insufficient to estimate real pollution levels without the lockdown restrictions in place. A machine learning technique called the Random Forest (RF) regression model was applied to predict a more realistic business-as-usual (BAU) level for OP, PM, and BC at urban background and traffic sites. While there is a clear decrease in OP found in the traffic site, more modest changes in OP were found in the urban site, likely due to sustained contributions from wood burning sources for residential heating. Overall, this study confirms the major roles of both combustion sources in the OP levels in ambient air during the lockdown period.
|
1 Introduction
The COVID-19 (coronavirus disease) pandemic has affected millions of people globally. Many countries have implemented lockdown restrictions to control the spread of the disease. These policies have provided a rare opportunity to assess air quality during a window with significantly reduced human-led activities of up to 90%.1 Most of the previous studies have focused on the impact of these lockdown measures on ambient air concentrations of key pollutants, such as particulate matter (PM), black carbon (BC), nitrogen dioxide (NO2), and ozone (O3) levels.2–7 In the meantime, there has been very limited information on the oxidative potential (OP) of PM, an emerging health-based exposure metric, during these lockdown periods.8
Atmospheric PM can originate from anthropogenic and natural sources. Anthropogenic sources include vehicular, industrial, residential combustion, and agricultural emissions, while natural sources include sea spray, wildfires, mineral dust, and biogenic sources.9 A bigger percentage of particulate pollution is driven by anthropogenic emissions, especially traffic and residential burning, in urban areas10 and even in background sites.11,12 During the lockdown periods in China and amidst strict restrictions on anthropogenic activities, there were still occurrences of severe PM pollution events, attributed to stagnant meteorological conditions.13–17 Reduction in NOx pollution levels was found in the United States during the lockdown period,18,19 Europe,20,21 and Canada;22 however, this was not reflected in PM levels, as exemplified in France.5 These findings have offered thought-provoking insights about the effect of lockdown restrictions on air quality and urge the need of a more comprehensive assessment that includes a health-based measure of PM exposure.
The OP of PM is commonly defined as the capacity of PM to generate reactive oxygen species (ROS) in biological media23 and is currently gaining attention as an emerging health-based metric of PM exposure.24–27 Acellular (or cell-free) assays are widely used to conveniently measure OP.25,28 Frequently used assays include dithiothreitol (DTT) and ascorbic acid (AA). The DTT assay measures OP based on the ability of PM to transfer electrons from DTT to oxygen, thereby producing ROS in the form of superoxides,29 while the AA assay measures OP based on PM-induced depletion of cellular AA antioxidants.30 Each OP assay appears to be sensitive to different components of PM and has varying levels of association with different health endpoints;25 hence, the use of multiple assays is frequently recommended.26,31
So far, only a few studies have considered OP measurements during the lockdown period. Wang et al.32 (2022) reported a decrease of OP simultaneously observed with decreasing biomass burning emissions in a typical coal-combustion city in China. Paraskevopoulou et al.8 (2022) found that there was no measurable change in the OP of PM2.5, even with the significant decrease in traffic during the COVID-19 lockdown in an urban background site in Athens, Greece. At an urban background site in the metropolitan area of Milan, Italy, the OP of PM2.5 (particularly OP assessed by DTT assay) was only slightly reduced during the lockdown period with much lower change than the other air quality parameters (e.g., chemical species in PM2.5, NO2, and BC).33,34 Overall, the lockdown periods opened a unique opportunity to evaluate OP of PM as a health exposure-relevant metric, especially in areas where no significant reduction in PM levels took place during the lockdown periods.
Due to multiple interplays between several factors (i.e., meteorology, seasonality, natural and anthropogenic emission sources) affecting PM pollution, it is crucial to take all important factors into account when predicting pollution levels. The efforts to reduce PM pollution through implemented air quality policies could also lead to decreasing trend of some emissions from sources, especially from traffic.11 Hence, to obtain a forecast of a business-as-usual (BAU) scenario, the use of simple and robust machine learning models can be useful apart from traditional interpolation using historical data. In fact, the use of stochastic data-driven models for forecasting has been proven useful for various applications in air pollution studies.35–37 One of the less computationally expensive machine learning techniques is called Random Forest (RF).38 This model uses a supervised learning algorithm that uses an ensemble learning method by combining predictions from multiple machine learning algorithms to make a more accurate prediction.
The present study takes advantage of the unique scenario brought about by the COVID-19 pandemic lockdown restrictions, enabling the estimation of the impact of significantly reduced anthropogenic emissions on the OP of atmospheric PM. The BAU levels were estimated using RF regression to more realistically assess the impact of the lockdown restrictions on PM pollution. This study aimed to elucidate the reduction in PM mass concentration and OP, as well as various combustion-related components (total equivalent black carbon (BCtot), wood burning BC (BCwb), and fossil fuel BC (BCff)) in an urban background site (Grenoble, France) and a traffic site (Bern, Switzerland). The findings of this study could provide a baseline impact of the COVID-19 lockdown restrictions on PM redox activity in an urban and traffic setting in Western Europe.
2 Materials and methods
2.1 Study areas and sampling parameters
The two sites included in this study are located in France and Switzerland, respectively. The GRE site (urban background) is located in Grenoble, France (45.2°N, 5.7°E), commonly regarded as the capital of the French Alps (altitude between 204 and 600 meters above sea level or masl) and has a population of approximately 440
000 inhabitants. This Alpine city is surrounded by several mountain ranges that favours the development of temperature inversions in the atmosphere,39 typical in a valley. The BERN site (traffic) is located in the city centre of Bern, Switzerland, a city with a population of about 145
000 inhabitants. This traffic site is situated within a busy canyon street and close to main train stations (47.0°N, 7.4°E).
The total PM10 mass concentration was measured in the GRE site using tapered element oscillating microbalances equipped with filter dynamics measurement systems (TEOM-FDMS),40 while an optical particle counter (Palas Fidas 200) was used in the BERN site. For both sites, equivalent black carbon (eBC) was measured using an aethalometer (AE33, Magee Scientific, USA).41 For the latter measurements, no further correction was applied to the concentration estimate calculated and provided by the AE33 software. The total equivalent BC concentration obtained in this way (BCtot) was apportioned to its wood burning (BCwb) and fossil fuel (BCff) fractions following the methodologies proposed in previous studies.42–44 Briefly, the contributions from liquid/fossil fuels (e.g., traffic) and solid fuels (typically wood burning) to BCtot were apportioned using the different absorption coefficients at 470 nm and 950 nm by the aethalometer. The absorption Ångström exponent (α) of 0.9 and 1.68 for the BERN site,43 while 1.0 and 2.0 for the GRE site45 were used for BCff and BCwb, respectively. The carbonaceous components, specifically organic carbon (OC) and elemental carbon (EC), were also analysed using the thermo-optical method on a Sunset Lab analyser,46 using the EUSAAR2 temperature protocol.
All of these measurements were conducted following the related EN standards (i.e., EN 12341, EN 14902, EN 16909, and EN 16913) and inter-laboratory comparison exercises for OC and EC within ACTRIS (Aerosols, Clouds and Trace Gases Research InfraStructure Network). Thanks to long-term observations at these given sites, as well as the presence of complementary monitoring stations in both areas, GRE10,47,48 and BERN49 are well characterized in terms of ambient levels and sources of PM pollution.
2.2 Oxidative potential (OP) analysis
The OP analysis was performed on PM extracted from collected filter samples on both sites using a simulated lung fluid (SLF) solution at 25 μg ml−1 iso-mass concentration,28 similar to the techniques applied in other studies by our group.11,47,50 Two assays were used to assess OP activity, namely (1) dithiothreitol (DTT) and (2) ascorbic acid (AA) assays. The OP activity or OPv is based on the OP consumption (nmol min−1) of the particles normalized by the sampled air volume (m3), representing the OP exposure in each sample. All samples were analysed in triplicate. The coefficient of variation (CV) between these triplicates was between 0 and 10% for each assay.
In the DTT assay, DTT is used as a chemical proxy that mimics the in vivo interaction of PM with biological reducing agents. This interaction represents the consumption of DTT in the assay or the capacity of PM to generate ROS.28 The leftover DTT in the initial reaction with PM is further reacted with 5,5′-dithiobis-(2-nitrobenzoic acid) (DTNB) which produces 5-mercapto-2-nitrobenzoic acid or TNB. The TNB is measured in terms of absorbance at 412 nm wavelength using a plate reader (TECAN spectrophotometer Infinite M200 Pro) and a 96-well multiwall plate (Greiner-Bio). To allow for the consumption of DTT, the absorbance is measured every 10 minutes for a total of 30 minutes of analysis time.
In the AA assay, the AA antioxidant is used in a respiratory tract lining fluid (RTFL)30 which represents the role of antioxidants in preventing the oxidation of lipids and proteins in the lung.51 The PM extract and AA mixture are analysed in terms of absorbance at 265 nm wavelength using a plate reader (TECAN spectrophotometer Infinite M200 Pro) and 96-well UV-transparent multiwall plate (Greiner-Bio) every 4 minutes for a total of 32 minutes of analysis time.
A positive control test is performed in every experiment, specifically a 1,4-naphthoquinone (1,4-NQ) solution for both the DTT (40 μl of 24.7 μM stock solution) and the AA (80 μl of 24.7 μM 1,4-NQ solution) assays. There is a < 3% CV in the positive control tests for both assays.
2.3 Random forest regression for estimating a business-as-usual (BAU) scenario during the lockdown period in 2020
The Random Forest (RF) regression model is an ensemble learning algorithm that fits multiple decision trees on extracted subsets from the dataset and uses averaging to improve the model predictive accuracy and control over-fitting.52–54 The RF model was used to estimate the BAU levels of all variables during the year 2020 based on historical data, assuming there were no lockdown restrictions in place. The features considered in each RF model were: meteorological variables temperature (°C), relative humidity (%), a variable representing a one-year lag of the target variable, and some temporal variables, such as Unix time (seconds) and Julian day of the year (1 to 365). Additionally, OC and EC mass concentrations were also used as a feature in the GRE site, while BCtot mass concentrations in other Swiss cities (Zurich and Basel) were used in the BERN site. These cities are located about 100 km from the BERN site with the same climatic, meteorological, and topographic characteristics. We supposed that in the local level, the BC mass concentration had similar tendencies and the BC levels in Zurich and Basel could be used for the prediction in Bern. The target variables considered were the daily (24-hour) levels of PM, BC, or OPv (OPDTT and OPAA) for both sites. Both the target variables and the features in the model were numeric. The RF model was implemented in Python 3.8 making use of the RandomForestRegressor in the Scikit-learn module.55
To improve the numerical stability of the model, both the target variable and the features were scaled using a standard scaling function. Afterwards, the dataset was split into training and testing sets by randomly assigning 70% of the input data as the training set and the rest of the 30% as testing. For the RF models for both OPDTT and OPAA in the BERN site, the test size had to be increased to 0.4 (0.6 for the training set) due to the number of data points (n = 177 and n = 273 for PM2.5 and PM10, respectively). Briefly, the training set is the dataset used to build and fit predictive models, while the test set is a subset of the dataset to assess the likely future performance of a model. A hyper-parameter tuning step was performed using a randomized search using a cross-validation technique based on a fixed number of parameter settings to determine an optimal architecture. The parameters considered and implemented were: the generated random numbers for the random forest, the number of trees in the forest, the minimum number of data points before the sample is split, the minimum number of leaf nodes that are required to be sampled, the maximum depth in a tree, applying a bootstrapping method when building decision trees, and applying a generalization score using out-of-bag samples. Typically, the hyper-parameter tuning step did not change the model performance by a large amount. Finally, the model performance was evaluated by calculating the root mean square error (RMSE) and R-squared value (r2) for both the training and testing sets.36 The optimal models were chosen based on their RMSE (as low as possible) and r2 (as high as possible) on the testing set. The modelling workflow from data collection, data processing, and the machine learning model optimization is presented in Fig. 1.
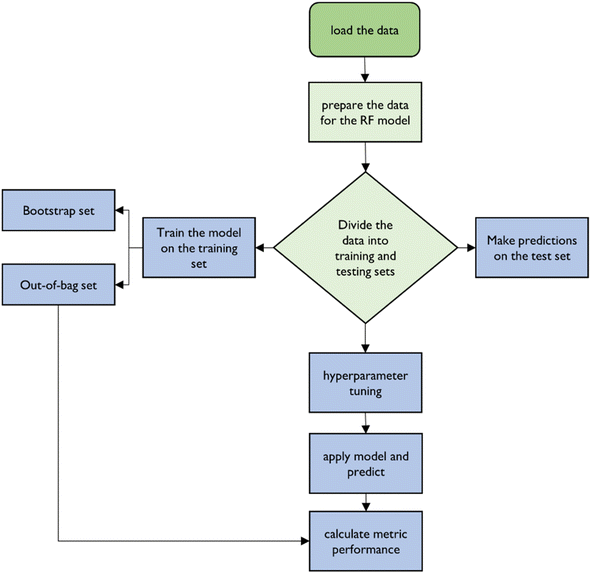 |
| Fig. 1 Scheme of the modelling workflow of the Random Forest (RF) model for predicting business-as-usual (BAU) levels for the COVID-19 lockdown period in 2020. | |
2.4 Decrease in pollution levels during the lockdown period in 2020
The first COVID-19 lockdown period occurred from 17 March to 11 May 2020 for both France and Switzerland. The strictest restrictions were implemented during this period such as reduced economic activity, travel restraints, and isolation, which have heavily affected mobility in both countries. These restrictions were lifted until 1 November 2020, when the second lockdown took place until 15 December 2020. The observed pollution levels during the first lockdown period in 2020 were compared with the historical average and the predicted BAU levels. To visualize the reduction of pollution levels, an empirical cumulative distribution function (ECDF) was performed and the results were compared between the observed and the historical and/or predicted BAU levels.
The percentage median change (%) based on mass concentrations (units: μg m−3 for PM, BCtot, BCwb, and BCff) or OP activity (units: nmol min−1 m−3 for OPDTT and OPAA) by period was calculated using eqn (1):
| 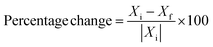 | (1) |
where
Xi is the historical median for all years prior to 2020 (or the RF-predicted levels for the year 2020) and
Xf is the observed median for the year 2020. A negative% change means there was a decrease in the observed levels during the year 2020 and
vice versa. A Mann–Whitney
U test was also performed to determine if there was a significant difference (
p-value ≤0.05) between the medians during the lockdown period and the historical or predicted BAU level. An overview of the available historical data is provided in the ESI (Table S1
†). All analyses were performed in Python 3.8 making use of the
Seaborn, a Python data visualization library based on
matplotlib.
56
3 Results and discussion
3.1 Overview of the pollution levels before and during the lockdown period
In an attempt to tackle the COVID-19 pandemic, strict lockdown restrictions were put in place, resulting in reduced human activities in many places. The daily PM, total BC (including BCwb and BCff) mass concentration, and OP activity (OPDTT and OPAA) at GRE and BERN sites are shown in Fig. 2 during the lockdown period in the year 2020 and the corresponding average (± standard deviation) of all years prior to 2020 on the same dates (17 March to 11 May).
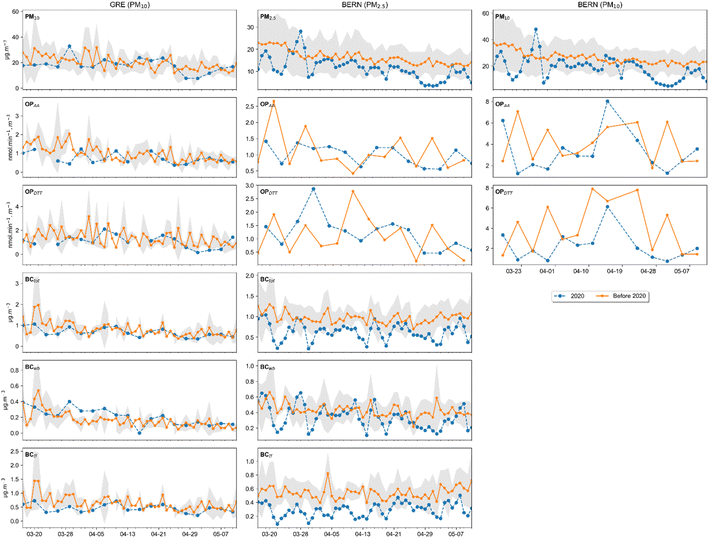 |
| Fig. 2 Comparison of daily PM mass concentration, equivalent black carbon (BCtot, BCwb, and BCff), and OP activity (OPDTT and OPAA) during the lockdown period (17 March to 11 May) in the year 2020 and the corresponding estimated levels based on historical data for the GRE (PM10 only) and BERN (PM10 and PM2.5) sites. Note: grey shades represent the standard deviation of each data point for the estimated concentrations. | |
Generally, it can be inferred that the average PM levels have not varied greatly even with the lockdown restrictions in place in the GRE site. Conversely, there was a noticeable decrease in the PM levels in the BERN site for both PM size fractions (PM10 and PM2.5), except for specific days implicated by a Saharan dust event from March 26 to 30, 2020.57 A comparison of BC mass concentration levels for both sites is also presented in Fig. 2. On the other hand, there was no large reduction in the total BC mass concentrations in the GRE site possibly due to the slight increase in BCwb. On the other hand, there seems to be a noticeable decrease in total BC in the BERN site due to the reduced BC from the fossil fuel fraction (BCff). Interestingly as well, for the OP activities, no drastic decrease is observed at each of the sites investigated in the present study.
In the GRE site, it has been consistently reported that biomass burning is a major contributor to PM10 mass during cold periods.10,58,59 Similar to other sites in Western Europe, increased residential/domestic heating emissions during lockdown due to people being at home likely affected the PM mass concentration, resulting in a less pronounced decrease in total PM levels.60 In fact, an increase in PM pollution was found in Tehran,61 China,62 and Malaysia63 potentially due to local burning activities as well. In Brazil, there is an increase in ozone levels due to the sharp decrease in nitrogen oxides resulting from decreased vehicular emissions.64 In Morocco, the decrease in local emissions was exceeded by regional and/or transboundary pollution resulting in an increase in pollution levels during the lockdown period.65 Overall, the decrease in vehicular emissions and mobility (i.e., travel restrictions) was not enough to offset other pollution sources and, potentially, the impact of secondary aerosol formation could be a more important driver of the increased pollution levels during the lockdown period.6,66
In a reference background site in France, an existing long-term decreasing trend in PM has been observed over the years.11 This has been attributed to the decreasing traffic emissions, likely due to the improvement of regulations restricting vehicular emissions, especially in bigger cities. Hence, it can be challenging to differentiate the potential reduction due to the impact of lockdown restrictions on pollution levels from an already existing decreasing trend. This has motivated an estimation of the concentrations for all variables under the hypothesis of BAU emissions to allow a more realistic assessment of pollution levels during the lockdown period in 2020.
3.2 Estimating the business-as-usual (BAU) levels using a random forest (RF) regression model
To achieve more realistic BAU pollution levels in the year 2020 (i.e., assuming no lockdown restrictions were in place), the RF model was used to estimate the levels of target variables such as PM, BC (BCtot, BCwb, and BCff), and OP (OPDTT and OPAA) in both the GRE and BERN sites. The features (i.e. predictors) considered in the RF model are meteorological, temporal variables, and a 1-year lag of the target variable. The selection of features is one of the crucial steps in machine learning applications as these have a direct impact on the model performance. The feature importance scores are presented in Fig. S1–S3† for the GRE and BERN sites. The highest importance score was commonly seen in OC and EC (or both) for most target variables in the GRE site, while BCtot mass concentration presents the higher rank for the BERN site. As unnecessary features are known to decrease the general performance of the test set,67 the extra features that were considered but showed low importance scores were excluded in the final RF models.
The associations between the observed and predicted values for both the training and test sets are presented in Fig. 3 for OPAA in the GRE site. The same comparisons are provided for the other variables in the ESI (Fig. S4–S17†). The model uncertainties based on the RMSE between the observed and RF predictions are also provided in the same figures.
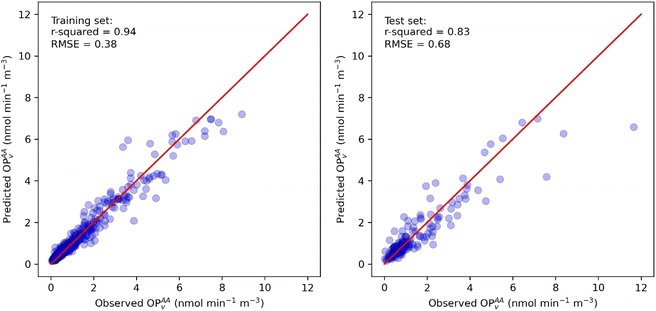 |
| Fig. 3 Comparison between the observed and RF-predicted OPAA (nmol min−1 m−3) of PM10 for the training (x samples) and testing (y samples) sets in the GRE site. Note: red line represents the 1 : 1 line. | |
In general, the model performed well for most variables for both sets for the GRE site (r2 ranging from 0.90 to 0.94 for training and 0.71 to 0.89 for test sets), but some variables were harder to predict in the BERN site (r2 ranging from 0.73 to 0.92 for training and 0.40 to 0.92 for test sets). Better predictions were found in the GRE site possibly due to the higher number of data points available for the model input (Table S1†). It is also important to note that the RF models were built differently, based on the available data in each site. In the GRE site, the available long-term data of OC (a species contributing to about 23 to 28% of total PM10 in the city68) showed high importance score in the RF model, especially for PM10, BCwb, and OPAA predictions. For the BERN site, the RF model relied more on the long-term BC data from other cities (Basel and Zurich) than any of the other considered features. Even the meteorological variables in the BERN site did not significantly increase the performance of the RF model for this site.
Very few studies have estimated BAU levels in terms of OP during the COVID-19 lockdown period.57,69 In the Ciarelli et al.69 (2021) study, a regional air quality model (Comprehensive Air Quality Model with extensions, version 6.50) was used, which relies on emission inventories to model NO2, O3, and PM2.5 in Italy (Milan) and Switzerland (Zurich). The model performance for PM2.5 is associated with r2 equal to 0.41 and 0.73, with a corresponding RMSE of 9.83 and 4.08 for the sites in Italy and Switzerland, respectively. In Lovrić et al.57 (2021), a RF regression model was used to predict “true pollution” levels in multiple traffic-loaded (r2 ranging from 0.42 to 0.61) and less traffic-loaded (r2 ranging from 0.66 to 0.71) sites in Graz, Austria. Overall, the RF models in our study provided a proper generalization based on available long-term data and performed better than the models of the two other studies, allowing a realistic estimation of a BAU scenario of the pollution levels during the COVID-19 lockdown period.
3.3 Impact of the lockdown restrictions
The distribution of the daily average of PM and BC mass as well as OPv during the days of the lockdown period is presented in this section based on the observed, historical, and RF-predicted datasets as presented in Fig. 4. The ECDF estimates a monotonically increasing curve representing each data point by its specific value. The corresponding probability density estimate is also provided in the ESI (Fig. S18†). The median percentage change (%) during the observed pollution levels during the lockdown period and the historical and RF-predicted levels is also summarized in Table 1. The presumption was that the observed pollution levels in the year 2020 would be lower than the average of all data prior to the year 2020 (“historical”) and the RF-predicted business-as-usual (“BAU”) levels, due to the lockdown restrictions implemented.
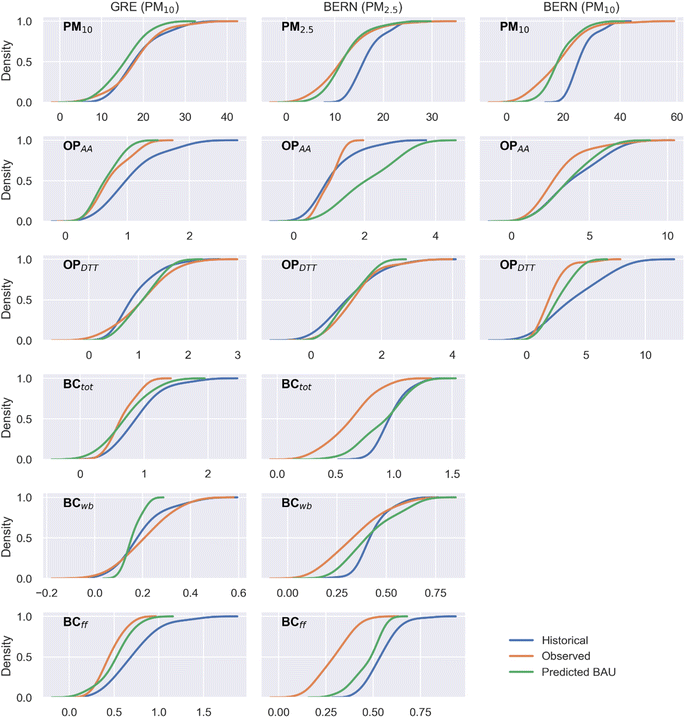 |
| Fig. 4 Distribution of the daily average of the target variables (PM10, BCtot, BCWB, BCFF, OPAA, and OPDTT) in each site during the COVID-19 lockdown period using an empirical cumulative distribution function (ECDF). Blue curves represent historical levels prior to the year 2020, the orange curves represent the observed levels during 2020, and green curves represent the RF-predicted business-as-usual (BAU) levels during 2020. Note: each x-axis depicts the unit of each target variable: μg m−3 for PM10, BCtot, BCWB, and BCFF, while nmol min−1 m−3 for OPAA and OPDTT. | |
Table 1 Median percentage change (% change) during the observed pollution levels during the COVID-19 lockdown period and the historical and RF-predicted levels in the GRE and BERN sitesa
Variables |
Percentage change (%) between observed vs. historical |
Percentage change (%) between observed vs. predicted |
GRE |
BERN |
GRE |
BERN |
PM10 |
PM10 |
PM2.5 |
PM10 |
PM10 |
PM2.5 |
The asterisks depict the Mann–Whitney U-test of statistically significant difference between observed and historical/predicted observations at p ≤ 0.05 (*) and p ≤ 0.01 (**). Note: a negative% change represents a decrease in median levels during the lockdown period, while a positive% change presents an increase.
|
PM |
0.6 |
−24.2** |
−27.1** |
8.4 |
5.8 |
0.3 |
OPAA |
−35.2* |
−17.5 |
30.8 |
12.7 |
−24.9 |
−39.2** |
OPDTT |
49.5 |
−41.6* |
48.3 |
3.3 |
−18.7 |
21.8 |
BCtot |
−31.3* |
|
−31.0** |
−14.2 |
|
−32.5** |
BCwb |
24.0 |
|
−17.8** |
47.1 |
|
−16.1** |
BCff |
−37.7** |
|
−44.4** |
−18.5 |
|
−40.1** |
Indeed, this is confirmed for the PM mass concentration in the BERN site with a clearer shift in levels when comparing the observed and historical levels. This shift pertains to a change in median levels of about −24% and −27% for PM10 and PM2.5, respectively, as shown in Table 1, implying decreased levels during the lockdown period. The predicted BAU levels were generally consistently lower than the historical levels of PM mass concentration as a result of an existing decreasing trend. Hence, there were minimal changes between the observed and the predicted BAU levels, implying a marginal effect of the lockdown restrictions on the PM mass concentration in the BERN site. In the GRE site, there was a negligible change in the observed compared to the historical level (<1%), while a slight increase when compared to the predicted BAU level (8%).
In terms of BC mass concentration, a much clearer decrease in levels (both in the BCwb and BCff fractions) was found in the BERN site. A stronger decrease was found in the BCff fraction, as expected, due to a sharp decrease in vehicular emissions during the lockdown period in this traffic site. This refers to a median% change in the observed level of −44% and −40% compared to historical and RF-predicted levels, respectively. In the GRE site, a decrease was also found in the BCff fraction (−38% and −19% for the historical and RF-predicted levels, respectively), while an increase was found in the BCwb fraction. This tends to imply a sustained contribution from wood burning emissions likely due to residential heating emissions during the lockdown period, especially in an urban area such as the GRE site. In fact, the median percentage change for BCwb in the GRE site has increased by 24 and 47% when the observed levels were compared with the historical average and the RF-predicted levels, respectively.
For OPv (both OPAA and OPDTT), the findings were less straight forward. Generally, the distributions of the observed OP were lower than the historical and BAU levels in the PM10 fraction in the BERN site. This suggests that OPv was as much influenced by the decrease in PM mass concentration in the area. This decrease in OP pertains to a median% change of −18% for OPAA and −42% for OPDTT. A similar shift was also found comparing the observed with the predicted BAU levels with −25% for OPAA and −19% for OPDTT. For the OPv of the PM2.5 fraction in the BERN site, only OPAA showed a decrease in the median% change (−39%) after comparing the observed with the predicted BAU level. In other cases, the observed was higher than the historical or the predicted BAU (OPDTT only). For the GRE site, the observed OPDTT was higher than the historical level (50%), but was about the same as the BAU levels. The observed OPAA decreased compared to the historical (−35%), but slightly increased compared to the BAU levels (13%).
The sensitivity of the assays could also play a role as BCff mass concentrations indeed have stronger associations with OPAA (Spearman correlation coefficient, rs of 0.7 and 0.3 for the GRE and BERN sites, respectively) (see Fig. S19–S21 in the ESI†). This is much weaker on OPDTT with rs of 0.3 and 0.2 for the GRE and BERN sites, respectively. The BCwb mass concentrations also showed slightly stronger correlations with OPDTT with rs of 0.5 and 0.6 for the GRE and BERN sites, respectively, than OPAA. Overall, these results present the difference in the impact of lockdown restrictions on OP between two different typologies (traffic vs. urban background sites).
3.4 Discussions
The findings of this study have highlighted discrepancies in the impact of lockdown restrictions based on the type of site and main drivers of PM pollution in the area. In an urban background site, there was no drastic reduction in PM, possibly due to the sustained contributions of BCwb, a variable closely related to biomass burning. The biomass burning source contributes 22% to total PM10 on the yearly average in the GRE site.10 This source is also one of the major contributors to OP of PM10 in the same site.47 Hence, it is not surprising that although there could be a reduction in vehicular emissions (as seen in the decrease in the BCff levels), the contributions of BCwb have dominated during the lockdown period. This led to a more modest decrease in PM mass concentration and a varied effect on OP in the urban site (decrease in OPAA and increase in OPDTT).
On the other hand, in the BERN site, the reductions in total BC (both in wood burning and fossil fuel BC fractions) were significant, resulting in a clearer impact of the lockdown restrictions on the levels of PM and OP. This site is also close to a train station, which suffered a drastic reduction in traffic during the lockdown. In a traffic area, it is anticipated that road dust resuspension could be a major source of PM10 pollution.26 Road dust is a common source of metals containing highly reactive species leading to increased OP activity in particulates.29 With the decrease in mobility during the lockdown period, this could be the primary cause of that reduction in OP in the traffic site.
Indeed, these findings are consistent with other studies that have reported greater reductions in pollution levels in areas directly impacted by anthropogenic emissions (i.e., bigger cities, traffic areas, and industrial sites).57,66
In an urban background site in Zagreb, Croatia, it was found that a decrease in mobility (e.g., transport to/from work, grocery shopping, etc.) during the lockdown period did not have a significant influence in PM mass concentration in the area.70 A similar case was found in an urban background site in Athens, Greece, revealing that the decrease in traffic did not result in any measurable change on OP (assessed by DTT assay) of PM2.5.8 In fact, this last study suggests unchanged contributions from the biomass burning and secondary aerosols. Interestingly, this is also consistent with the findings in an urban background site in Milan, Italy highlighting that OP (also assessed by DTT assay) has been mostly impacted by biomass burning resulting in weaker reductions than other pollutants during the lockdown period.33 Meanwhile, in a typical coal-combustion city in Linfen, China, a significant decrease in OP was observed attributed to the decrease of several subgroups of primary organic aerosols (OA).32 These authors also found an enhanced formation of secondary OA resulting from high oxidation capacity (i.e., high O3 levels) in the atmosphere during the lockdown period.
Fig. 5 presents the joint distribution of PM and OPAA during the lockdown period in both the GRE and BERN sites. A similar figure is presented for OPDTT in the ESI (Fig. S22†). These figures show a difference in the distributions during the lockdown period compared to the past few years and even on a predicted BAU scenario, particularly the shift towards a lower OPAA even when the PM mass concentrations were relatively similar for both sites. This indicates two important takeaways: first, the lockdown restrictions have potentially resulted in reduced redox activity of PM in the atmosphere and second, the added value of OP as a PM exposure metric. The apportioned BC in both sites has provided some indication of the changes in the contribution of combustion-related sources during the lockdown period. However, a complete chemistry would provide a more comprehensive view of the specific impacts of reduced source emissions to PM-induced oxidative stress.
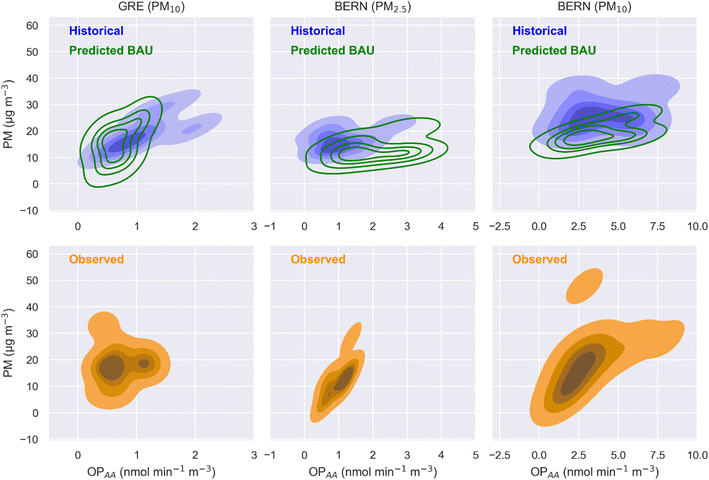 |
| Fig. 5 Bivariate distribution between PM and OPAA in each site during the COVID-19 lockdown period using a kernel density function (KDF). Blue plots represent historical levels prior to the year 2020, the orange plots represent the observed levels during 2020, and green plots represent the RF-predicted business-as-usual (BAU) levels during 2020. Note: the default number of contours was set to 5 levels. These contours were drawn at iso-proportions of the density plot representing the distributions of both variables. For aesthetic purposes, the shading was turned off for the RF-predicted BAU plots. | |
4 Summary
This study aimed to assess the impact of the COVID-19 lockdown restrictions on OP of PM in an urban background and traffic site in France and Switzerland, respectively. The levels of PM mass concentration and BCtot, as well as its apportioned fractions for wood burning and fossil fuels, were also studied. A Random Forest model was applied to achieve a business-as-usual scenario depicting the pollution levels during the lockdown period in the year 2020, assuming no restrictions were in place. The main findings of this study are:
• The impact of the lockdown restrictions on OP varies based on site types and pollution profiles in the area. In an urban background site, there were more modest changes in the PM mass concentration levels, and a decrease in OP (assessed by AA assay) was found. This decrease was not found in the OPDTT likely due to the sustained contributions from wood burning sources in the urban background site during the lockdown period.
• A clearer decrease in OP was found in the traffic site, highlighting the impact of lockdown restrictions on sites with direct anthropogenic emissions in terms of minimizing PM pollution and the potential health effects upon PM exposure.
• The RF modelling technique provided a good estimate of a BAU scenario, allowing for a more representative assessment of the changes of pollution levels during the lockdown period.
Overall, this study elucidated the differences in the impacts of the COVID-19 lockdown restrictions on OP of PM in two sites in Western Europe, further highlighting the importance of considering the redox activity of PM. This was done by using a more traditional approach through a comparison with historical data and, also, a more innovative approach using a machine learning technique that allows a comparison with a more representative BAU level.
Code availability
The software code could be made available upon request by contacting the corresponding author.
Data availability
The datasets could be made available upon request by contacting the corresponding author.
Author contributions
LJB and VTN performed the data analysis and the development of the Random Forest regression methodology. GU, JLJ, OF, and CH were involved in the funding and resource acquisition. GU, JLJ, SG, and CH helped with mentoring, supervision and validation of the methodology, techniques, and results. LJB and VTN were responsible for the data processing. LJB wrote the original draft. All authors reviewed and edited the manuscript.
Conflicts of interest
The authors declare that they have no conflict of interest.
Acknowledgements
This work was supported by the French National Research Agency in the framework of the “Investissements d'avenir” program (ANR-15-IDEX-02), for the Mobil’Air program. It also received support from the program QAMECS funded by ADEME (convention 1662C0029), and from LCSQA and the French Ministry of Environment for part of the analyses for the Les Frenes site within the CARA program. Chemical analysis on the Air-O-Sol facility at IGE was made possible with the funding of some of the equipment by Labex OSUG@2020 (ANR10 LABX56). The postdoc of Lucille Joanna Borlaza was funded by the Predict'air project (grant Foundation UGA-UGA 2022-16 and grant PR-PRE-2021 FUGA-Fondation Air Liquide). The work on oxidative potential measurements was also supported by the French National Research Agency in the framework of the Get OP Stand OP (ANR-19-CE34-0002-01). The long-term monitoring program at GRE was partly financed by the French Ministry of Environment, as part of the CARA program since 2008. Finally, this work was also supported by the European Union's Horizon 2020 research and innovation program under grant agreement 101036245 (RI-URBANS). The authors would like to kindly thank the dedicated efforts of many people from Atmo-AuRA at the sampling sites and in the lab at IGE (Anthony Vella, Claire Vérin, Céline Voiron, Rhabira El Azzouzi, and Armelle Crouzet) for collecting and analysing the samples, respectively. The authors would like to thank Meteomatics (https://www.meteomatics.com/) for additional meteorological data in the GRE site. The authors would also like to thank the Fondation Université Grenoble Alpes (FUGA) for supporting this research.
References
- S. Muhammad, X. Long and M. Salman, COVID-19 pandemic and environmental pollution: A blessing in disguise?, Sci. Total Environ., 2020, 728, 138820 CrossRef CAS PubMed.
- Z. S. Venter, K. Aunan, S. Chowdhury and J. Lelieveld, COVID-19 lockdowns cause global air pollution declines, Proc. Natl. Acad. Sci. U. S. A., 2020, 117(32), 18984–18990 CrossRef CAS.
- V. Sreekanth, M. Kushwaha, P. Kulkarni, A. R. Upadhya, B. Spandana and V. Prabhu, Impact of COVID-19 lockdown on the fine particulate matter concentration levels: Results from Bengaluru megacity, India, Adv. Space Res., 2021, 67(7), 2140–2150 CrossRef CAS PubMed.
- M. S. Hammer, A. van Donkelaar, R. V. Martin, E. E. McDuffie, A. Lyapustin and A. M. Sayer,
et al., Effects of COVID-19 lockdowns on fine particulate matter concentrations, Sci. Adv., 2021, 7(26), eabg7670 CrossRef CAS.
- J. E. Petit, J. C. Dupont, O. Favez, V. Gros, Y. Zhang and J. Sciare,
et al., Response of atmospheric composition to COVID-19 lockdown measures during spring in the Paris region (France), Atmos. Chem. Phys., 2021, 21(22), 17167–17183 CrossRef CAS.
- M. A. Hassan, T. Mehmood, E. Lodhi, M. Bilal, A. A. Dar and J. Liu, Lockdown Amid COVID-19 Ascendancy over Ambient Particulate Matter Pollution Anomaly, Int. J. Environ. Res. Public Health, 2022, 19(20), 13540 CrossRef CAS.
- M. Conte, A. Dinoi, F. M. Grasso, E. Merico, M. R. Guascito and D. Contini, Concentration and size distribution of atmospheric particles in southern Italy during COVID-19 lockdown period, Atmos. Environ., 2023, 295, 119559 CrossRef CAS PubMed.
- D. Paraskevopoulou, A. Bougiatioti, P. Zarmpas, M. Tsagkaraki, A. Nenes and N. Mihalopoulos, Impact of COVID-19 Lockdown on Oxidative Potential of Particulate Matter: Case of Athens (Greece), Toxics, 2022, 10(6), 280 CrossRef CAS PubMed.
-
J. H. Seinfeld and S. N. Pandis, Atmospheric Chemistry and Physics: from Air Pollution to Climate Change, 3rd edition, Wiley, Hoboken, New Jersey, 2016, p. 1120 Search PubMed.
- L. J. S. Borlaza, S. Weber, G. Uzu, V. Jacob, T. Cañete and S. Micallef,
et al., Disparities in particulate matter (PM10) origins and oxidative potential at a city scale (Grenoble, France) – Part 1: Source apportionment at three neighbouring sites, Atmos. Chem. Phys., 2021, 21(7), 5415–5437 CrossRef CAS.
- L. J. Borlaza, S. Weber, A. Marsal, G. Uzu, V. Jacob and J. L. Besombes,
et al., Nine-year trends of PM 10 sources and oxidative potential in a rural background site in France, Atmos. Chem. Phys., 2022, 22(13), 8701–8723 CrossRef CAS.
- V. Mardoñez, M. Pandolfi and L. J. S. Borlaza,
et al., Source apportionment study on particulate air pollution in two highaltitude Bolivian cities: La Paz and El Alto, Atmospheric Chemistry and Physics Discussions, 2022, 2022, 1–41 Search PubMed.
- T. Le, Y. Wang, L. Liu, J. Yang, Y. L. Yung and G. Li,
et al., Unexpected air pollution with marked emission reductions during the COVID-19 outbreak in China, Science, 2020, 369(6504), 702–706 CrossRef CAS PubMed.
- Y. Chang, R. Huang, X. Ge, X. Huang, J. Hu and Y. Duan,
et al., Puzzling Haze Events in China During the Coronavirus (COVID-19) Shutdown, Geophys. Res. Lett., 2020, e2020GL088533 CAS.
- X. Huang, A. Ding, J. Gao, B. Zheng, D. Zhou and X. Qi,
et al., Enhanced secondary pollution offset reduction of primary emissions during COVID-19 lockdown in China, Natl. Sci. Rev., 2021, 8(2), nwaa137 CrossRef CAS PubMed.
- M. Li, T. Wang, M. Xie, S. Li, B. Zhuang and Q. Fu,
et al., Drivers for the poor air quality conditions in North China Plain during the COVID-19 outbreak, Atmos. Environ., 2021, 246, 118103 CrossRef CAS PubMed.
- P. Wang, K. Chen, S. Zhu, P. Wang and H. Zhang, Severe air pollution events not avoided by reduced anthropogenic activities during COVID-19 outbreak, Resour., Conserv. Recycl., 2020, 158, 104814 CrossRef.
- L. W. A. Chen, L. C. Chien, Y. Li and G. Lin, Nonuniform impacts of COVID-19 lockdown on air quality over the United States, Sci. Total Environ., 2020, 745, 141105 CrossRef CAS.
- R. Tanzer-Gruener, J. Li, S. R. Eilenberg, A. L. Robinson and A. A. Presto, Impacts of Modifiable Factors on Ambient Air Pollution: A Case Study of COVID-19 Shutdowns, Environ. Sci. Technol. Lett., 2020, 7(8), 554–559 CrossRef CAS.
- G. Donzelli, L. Cioni, M. Cancellieri, A. Llopis Morales and M. Morales Suárez-Varela, The Effect of the Covid-19 Lockdown on Air Quality in Three Italian Medium-Sized Cities, Atmosphere, 2020, 11(10), 1118 CrossRef CAS.
- J. M. Baldasano, COVID-19 lockdown effects on air quality by NO2 in the cities of Barcelona and Madrid (Spain), Sci. Total Environ., 2020, 741, 140353 CrossRef CAS.
- M. D. Adams, Air pollution in Ontario, Canada during the COVID-19 State of Emergency, Sci. Total Environ., 2020, 742, 140516 CrossRef CAS PubMed.
- A. Nel, ATMOSPHERE: Enhanced: Air Pollution-Related Illness: Effects of Particles, Science, 2005, 308(5723), 804–806 CrossRef CAS PubMed.
- A. Marsal, R. Slama, S. Lyon-Caen, L. J. S. Borlaza, J. L. Jaffrezo and A. Boudier,
et al., Prenatal exposure to PM2.5 oxidative potential and lung function in infants and preschool age children: a prospective study, Environ. Health Perspect., 2022, 017004 Search PubMed.
- J. T. Bates, T. Fang, V. Verma, L. Zeng, R. J. Weber and P. E. Tolbert,
et al., Review of Acellular Assays of Ambient Particulate Matter Oxidative Potential: Methods and Relationships with Composition, Sources, and Health Effects, Environ. Sci. Technol., 2019, 53(8), 4003–4019 CrossRef CAS PubMed.
- K. R. Daellenbach, G. Uzu, J. Jiang, L. E. Cassagnes, Z. Leni and A. Vlachou,
et al., Sources of particulate-matter air pollution and its oxidative potential in Europe, Nature, 2020, 587(7834), 414–419 CrossRef CAS PubMed.
- M. R. Guascito, M. G. Lionetto, F. Mazzotta, M. Conte, M. E. Giordano and R. Caricato,
et al., Characterisation of the correlations between oxidative potential and in vitro biological effects of PM10 at three sites in the central Mediterranean, J. Hazard. Mater., 2023, 448, 130872 CrossRef CAS.
- A. Calas, G. Uzu, F. J. Kelly, S. Houdier, J. M. F. Martins and F. Thomas,
et al., Comparison between five acellular oxidative potential measurement assays performed with detailed chemistry on PM10 samples from the city of Chamonix (France), Atmos. Chem. Phys., 2018, 18(11), 7863–7875 CrossRef CAS.
- J. G. Charrier and C. Anastasio, On dithiothreitol (DTT) as a measure of oxidative potential for ambient particles: evidence for the importance of soluble transition metals, Atmos. Chem. Phys., 2012, 12(19), 9321–9333 CrossRef CAS.
- F. J. Kelly and I. S. Mudway, Protein oxidation at the air-lung interface, Amino Acids, 2003, 25(3–4), 375–396 CrossRef CAS.
- L. J. S. Borlaza, E. M. R. Cosep, S. Kim, K. Lee, H. Joo and M. Park,
et al., Oxidative potential of fine ambient particles in various environments, Environ. Pollut., 2018, 243, 1679–1688 CrossRef CAS.
- W. Wang, Y. Zhang, G. Cao, Y. Song, J. Zhang and R. Li,
et al., Influence of COVID-19 lockdown on the variation of organic aerosols: Insight into its molecular composition and oxidative potential, Environ. Res., 2022, 206, 112597 CrossRef CAS.
- M. C. Pietrogrande, C. Colombi, E. Cuccia, U. Dal Santo and L. Romanato, The Impact of COVID-19 Lockdown Strategies on Oxidative Properties of Ambient PM10 in the
Metropolitan Area of Milan, Italy, Environments, 2022, 9(11), 145 CrossRef.
- A. Altuwayjiri, E. Soleimanian, S. Moroni, P. Palomba, A. Borgini and C. De Marco,
et al., The impact of stay-home policies during Coronavirus-19 pandemic on the chemical and toxicological characteristics of ambient PM2.5 in the metropolitan area of Milan, Italy, Sci. Total Environ., 2021, 758, 143582 CrossRef CAS PubMed.
- W. Ding and X. Qie, Prediction of Air Pollutant Concentrations via RANDOM Forest Regressor Coupled with Uncertainty Analysis—A Case Study in Ningxia, Atmosphere, 2022, 13(6), 960 CrossRef CAS.
- S. M. Cabaneros and B. Hughes, Methods used for handling and quantifying model uncertainty of artificial neural network models for air pollution forecasting, Environ. Model. Softw., 2022, 158, 105529 CrossRef.
- S. Abu El-Magd, G. Soliman, M. Morsy and S. Kharbish, Environmental hazard assessment and monitoring for air pollution using machine learning and remote sensing, Int. J. Environ. Sci. Technol., 2022 DOI:10.1007/s13762-022-04367-6.
- M. Zamani Joharestani, C. Cao, X. Ni, B. Bashir and S. Talebiesfandarani, PM2.5 Prediction Based on Random Forest, XGBoost, and Deep Learning Using Multisource Remote Sensing Data, Atmosphere, 2019, 10(7), 373 CrossRef.
- B. Bessagnet, L. Menut, R. Lapere, F. Couvidat, J. L. Jaffrezo and S. Mailler,
et al., High Resolution Chemistry Transport Modeling with the On-Line CHIMERE-WRF Model over the French Alps—Analysis of a Feedback of Surface Particulate Matter Concentrations on Mountain Meteorology, Atmosphere, 2020, 11(6), 565 CrossRef CAS.
- B. D. Grover, Measurement of total PM 2.5 mass (nonvolatile plus semivolatile) with the Filter Dynamic Measurement System tapered element oscillating microbalance monitor, J. Geophys. Res., 2005, 110(D7), D07S03 CrossRef.
- S. K. Grange, H. Lötscher, A. Fischer, L. Emmenegger and C. Hueglin, Evaluation of equivalent black carbon source apportionment using observations from Switzerland between 2008 and 2018, Atmos. Meas. Tech., 2020, 13(4), 1867–1885 CrossRef CAS.
- J. Sandradewi, A. S. H. Prévôt, E. Weingartner, R. Schmidhauser, M. Gysel and U. Baltensperger, A study of wood burning and traffic aerosols in an Alpine valley using a multi-wavelength Aethalometer, Atmos. Environ., 2008, 42(1), 101–112 CrossRef CAS.
- P. Zotter, H. Herich, M. Gysel, I. El-Haddad, Y. Zhang and G. Močnik,
et al., Evaluation of the absorption Ångström exponents for traffic and wood burning in the Aethalometer-based source apportionment using radiocarbon measurements of ambient aerosol, Atmos. Chem. Phys., 2017, 17(6), 4229–4249 CrossRef CAS.
- R. M. Harrison, D. C. S. Beddows, L. Hu and J. Yin, Comparison of methods for evaluation of wood smoke and estimation of UK ambient concentrations, Atmos. Chem. Phys., 2012, 12(17), 8271–8283 CrossRef CAS.
- O. Favez, I. El Haddad, C. Piot, A. Boréave, E. Abidi and N. Marchand,
et al., Inter-comparison of source apportionment models for the estimation of wood burning aerosols during wintertime in an Alpine city (Grenoble, France), Atmos. Chem. Phys., 2010, 10(12), 5295–5314 CrossRef CAS.
- M. E. Birch and R. A. Cary, Elemental Carbon-Based Method for Monitoring Occupational Exposures to Particulate Diesel Exhaust, Aerosol Sci. Technol., 1996, 25(3), 221–241 CrossRef CAS.
- L. J. S. Borlaza, S. Weber, J. L. Jaffrezo, S. Houdier, R. Slama and C. Rieux,
et al., Disparities in particulate matter (PM10) origins and oxidative potential at a city scale (Grenoble, France) – Part 2: Sources of PM10 oxidative potential using multiple linear regression analysis and the predictive applicability of multilayer perceptron neural network analysis, Atmos. Chem. Phys., 2021, 21(12), 9719–9739 CrossRef CAS.
- O. Favez, S. Weber, J. E. Petit, L. Y. Alleman, A. Albinet and V. Riffault,
et al., Overview of the French Operational Network for In Situ Observation of PM Chemical Composition and Sources in Urban Environments (CARA Program), Atmosphere, 2021, 12(2), 207 CrossRef CAS.
- S. K. Grange, A. Fischer, C. Zellweger, A. Alastuey, X. Querol and J. L. Jaffrezo,
et al., Switzerland's PM10 and PM2.5 environmental increments show the importance of non-exhaust emissions, Atmos. Environ.: X, 2021, 12, 100145 CAS.
- L. J. S. Borlaza, G. Uzu, M. Ouidir, S. Lyon-Caen, A. Marsal and S. Weber,
et al., Personal exposure to PM2.5 oxidative potential and its association to birth outcomes, J. Exposure Sci. Environ. Epidemiol., 2022 DOI:10.1038/s41370-022-00487-w.
- M. Valko, H. Morris and M. Cronin, Metals, Toxicity and Oxidative Stress, Curr. Med. Chem., 2005, 12(10), 1161–1208 CrossRef CAS.
- S. K. Grange and D. C. Carslaw, Using meteorological normalisation to detect interventions in air quality time series, Sci. Total Environ., 2019, 653, 578–588 CrossRef CAS.
- L. Breiman, Random Forests, Mach. Learn., 2001, 45(1), 5–32 CrossRef.
- W. Tong, H. Hong, H. Fang, Q. Xie and R. Perkins, Decision Forest: Combining the Predictions of Multiple Independent Decision Tree Models, J. Chem. Inf. Comput. Sci., 2003, 43(2), 525–531 CrossRef CAS PubMed.
- F. Pedregosa, G. Varoquaux, A. Gramfort, V. Michel, B. Thirion and O. Grisel,
et al., Scikit-learn: Machine Learning in Python, J. Mach. Learn. Res., 2011, 12(85), 2825–2830 Search PubMed.
-
M. Waskom, O. Botvinnik, D. O'Kane, P. Hobson, S. Lukauskas, D. C. Gemperline, et al., Mwaskom/Seaborn: V0.8.1, available from: https://zenodo.org/record/883859 Search PubMed.
- M. Lovrić, K. Pavlović, M. Vuković, S. K. Grange, M. Haberl and R. Kern, Understanding the true effects of the COVID-19 lockdown on air pollution by means of machine learning, Environ. Pollut., 2021, 274, 115900 CrossRef PubMed.
- S. Weber, G. Uzu, O. Favez, L. J. S. Borlaza, A. Calas and D. Salameh,
et al., Source apportionment of atmospheric PM10 oxidative potential: synthesis of 15 year-round urban datasets in France, Atmos. Chem. Phys., 2021, 21(14), 11353–11378 CrossRef CAS.
- D. Srivastava, S. Tomaz, O. Favez, G. M. Lanzafame, B. Golly and J. L. Besombes,
et al., Speciation of organic fraction does matter for source apportionment. Part 1: A one-year campaign in Grenoble (France), Sci. Total Environ., 2018, 624, 1598–1611 CrossRef CAS PubMed.
- L. Menut, B. Bessagnet, G. Siour, S. Mailler, R. Pennel and A. Cholakian, Impact of lockdown measures to combat Covid-19 on air quality over western Europe, Sci. Total Environ., 2020, 741, 140426 CrossRef CAS PubMed.
- S. Faridi, F. Yousefian, S. Niazi, M. R. Ghalhari, M. S. Hassanvand and K. Naddafi, Impact of SARS-CoV-2 on Ambient Air Particulate Matter in Tehran, Aerosol Air Qual. Res., 2020, 20(8), 1805–1811 CrossRef CAS.
- J. E. Nichol, M. Bilal, MdA. Ali and Z. Qiu, Air Pollution Scenario over China during COVID-19, Remote Sens., 2020, 12(13), 2100 CrossRef.
- M. S. Mohd Nadzir, M. C. G. Ooi, K. M. Alhasa, M. A. A. Bakar, A. A. A. Mohtar and M. F. F. M. Nor,
et al., The Impact of Movement Control Order (MCO) during Pandemic COVID-19 on Local Air Quality in an Urban Area of Klang Valley, Malaysia, Aerosol Air Qual. Res., 2020, 20(6), 1237–1248 CrossRef.
- G. Dantas, B. Siciliano, B. B. França, C. M. da Silva and G. Arbilla, The impact of COVID-19 partial lockdown on the air quality of the city of Rio de Janeiro, Brazil, Sci. Total Environ., 2020, 729, 139085 CrossRef CAS PubMed.
- A. Otmani, A. Benchrif, M. Tahri, M. Bounakhla, E. M. Chakir and M. El Bouch,
et al., Impact of Covid-19 lockdown on PM10, SO2 and NO2 concentrations in Salé City (Morocco), Sci. Total Environ., 2020, 735, 139541 CrossRef CAS.
- H. Wang, Q. Miao, L. Shen, Q. Yang, Y. Wu and H. Wei,
et al., Characterization of the aerosol chemical composition during the COVID-19 lockdown period in Suzhou in the Yangtze River Delta, China, J. Environ. Sci., 2021, 102, 110–122 CrossRef CAS PubMed.
- T. T. Nguyen, J. Z. Huang and T. T. Nguyen, Unbiased Feature Selection in Learning Random Forests for High-Dimensional Data, Sci. World J., 2015, 2015, 1–18 CrossRef PubMed.
- L. J. S. Borlaza, S. Weber, G. Uzu, V. Jacob, T. Cañete and S. Micallef,
et al., Disparities in particulate matter (PM10) origins and oxidative potential at a city scale (Grenoble, France) – Part 1: Source apportionment at three neighbouring sites, Atmos. Chem. Phys., 2021, 21(7), 5415–5437 CrossRef CAS.
- G. Ciarelli, J. Jiang, I. El Haddad, A. Bigi, S. Aksoyoglu and A. S. H. Prévôt,
et al., Modeling the effect of reduced traffic due to COVID-19 measures on air quality using a chemical transport model: impacts on the Po Valley and the Swiss Plateau regions, Environ. Sci.: Atmos., 2021, 1(5), 228–240 CAS.
- M. Lovrić, M. Antunović, I. Šunić, M. Vuković, S. Kecorius and M. Kröll,
et al., Machine Learning and Meteorological Normalization for Assessment of Particulate Matter Changes during the COVID-19 Lockdown in Zagreb, Croatia, Int. J. Environ. Res. Public Health, 2022, 19(11), 6937 CrossRef PubMed.
|
This journal is © The Royal Society of Chemistry 2023 |
Click here to see how this site uses Cookies. View our privacy policy here.