Variations of air pollutant response to COVID-19 lockdown in cities of the Tibetan Plateau†
Received
1st December 2022
, Accepted 16th February 2023
First published on 17th February 2023
Abstract
Coronavirus Disease 2019 (COVID-19) accidentally appeared in Tibet on August 7, 2022, and broke the 920 consecutive epidemic-free days. The cities in Tibet completely kept lockdown to restrict the public to homes. It provided a valuable opportunity for understanding how variations of urban air pollutants responded to the COVID-19 lockdown in the special highland cities of the third polar. Compared with the global COVID-19 restrictions in 2020, a slightly negative O3 anomaly and greater PM2.5 and NO2 reductions were found. The study showed PM10 (−65%) < NO2 (−53%) < PM2.5 (−52%) < O3 (−15%) < CO (−11%) < SO2 (10%) in the Tibetan Lockdown 2022. The total gaseous oxidant (Ox = NO2 + O3) decreased 18% in the Tibetan Lockdown 2022, but showed a limited change in the Lockdown 2020. Moreover, the diurnal profiles of NO2 and PM10 disappeared and the diurnal profiles of Ox, PM2.5, and PM2.5/CO became weakened. Via the random forest model-based weather normalization technique, we obtained the decoupled meteorological effects 1.3 ± 0.6 times higher than the net emission reduction due to the Tibetan Lockdown 2022. Meanwhile, we found that the deweathered PM2.5 was mainly from residential combustion emissions due to the weak industrialization in Tibet. Here we roughly estimated that vehicle-related sources can contribute about 28% and 29% to PM2.5 and NO2 in urban cities of Tibet respectively. For eco-vulnerability protection and sustainable development in Tibet, more attention should be paid to reducing the more intensive local pollutant emissions from vehicles and residential combustion of urban cities.
Environmental significance
It is generally assumed that the main sources of atmospheric and glacial pollutants in the Third Pole region are transported from external locations. The main local emissions distribute in the Tibetan cities. Coronavirus Disease 2019 (COVID-19) accidently appeared in Tibet on August 7, 2022, and broke the 920 consecutive epidemic-free days. It provided a valuable opportunity for understanding how variations of urban air pollutants responded to the COVID-19 lockdown in the special highland cities of the third polar. Global research showed limited change in the total gaseous oxidant (Ox = NO2 + O3) due to Lockdown 2020, as gains in O3 compensated for drops in NO2, including Tibetan urban cities. A slightly negative O3 anomaly and greater PM2.5 and NO2 reductions were found in the Lockdown 2022. The decrease of atmospheric oxidation smoothed the diurnal profiles of NO2 and PM10 and weakened those of O3, PM2.5, and PM2.5/CO. The random forest model-based weather normalization technique suggested that vehicle-related sources can contribute about 28% and 29% to PM2.5 and NO2, respectively. Others were mainly from residential combustion emissions due to the weak industrialization in Tibet cities. This is the first study to understand the variations of air pollutant response to the large COVID-19 lockdown in Tibetan cities. The findings provide a reference for future air pollution management and urbanization in the Tibet.
|
1 Introduction
Millions of people die every year from diseases caused by exposure to outdoor air pollution,1,2 especially particulate matter with an aerodynamic diameter < 2.5 μm (PM2.5) and ozone (O3).3,4 Surface ozone (O3) pollution can absorb ultraviolet rays and also impede plant growth and biomass accumulation, thereby further increasing global warming.5 The Tibetan Plateau (TP), containing the most extensive glacial area outside of the Polar Regions, is known as the Asian Water Tower.6 It is generally assumed that the main sources of atmospheric and glacial pollutants in the Third Pole region are transported from external locations (e.g., South Asia and Middle East) in regional areas.7–9 Moreover, local pollutant emissions from residential burning activities and vehicles cannot be ignored with the economic development and urbanization on the TP.10–12
Many studies have applied various statistical models, remote sensing, and numerical models to decouple the nonlinear effects of the meteorological conditions and emissions on the air quality. These studies were the basis of the policies on eco-vulnerability protection and regional sustainable development for Tibet.13–15 Short-term interventions on real-world air quality provide a unique opportunity to check and constrain these decoupling models. Stringent emission controls have proven effective for temporary improvement of air quality, such as the 2014 Asia-Pacific Economic Cooperation (APEC) meeting, the 2015 China Victory Day Parade and the 2022 Beijing Winter Olympics,16–18 when control strategies were planned in advance for the main emission sectors.19 However, Coronavirus Disease 2019 (COVID-19) appeared as a sudden intervention event and has caused disastrous health and socioeconomic crises around the world.20 Meanwhile, diverse policies have been adopted by governments across the world, such as limiting public transportation, encouraging or mandating working from home, and enforcing closure of public services.21,22 Global researchers focused on the air quality change due to lockdown from February to May in 2020, and mostly in the urban agglomerates:23–25 The total gaseous oxidant (Ox = NO2 + O3) showed limited change as gains in O3 compensated for drops in NO2. Decreasing trends of PM2.5 were observed in most cities, and short-term and unexpected fluctuations were accounted for the atmospheric oxidation enhancement due to reduced titration.26–28 To the best of our knowledge, negative ozone anomalies due to COVID-19 lockdown were rare and only reported at two UK sites and a high mountain site in northern Italy.24,25
Atmospheric pressure that decreases with altitude affects lung physiology, so COVID-19 is likely more harmful to the public in Tibet (ave. 4000 m a.s.l.) and needs special consideration.29 Fortunately, lockdown policies throughout China effectively contained the transmission of the COVID-19 epidemic (“Lockdown 2020”), resulting in only one confirmed case in Tibet. An emission assessment report suggested that the Lockdown 2020 has less impact on the local daily lives and industrial production (e.g. energy, raw material processing and manufacturing) in Tibet.30 A telephone interview also reported that the transportation frequency in Tibet was also less affected.31 Studies on two remote high mountains on the TP supported the opinion that the decrease in air pollutant was exclusively associated with the emission reduction in the surrounding areas, such as South Asia.32,33 However, the COVID epidemic suddenly appeared in Tibet on August 7, 2022, and powerful control policies were implemented (“Tibetan Lockdown 2022”). We systematically analyzed and quantified the role of emission reductions and meteorological contribution for the air pollutants, i.e. NO2, O3, sulfur dioxide (SO2), carbon monoxide (CO), PM2.5, and PM10 in Tibetan Lockdown 2022. This provides one chance to understand how air quality is influenced by the local emissions in Tibetan urban cities. Compared with Lockdown 2020, a slight negative ozone anomaly and greater PM2.5 and NO2 reductions were found in Tibetan urban areas due to the current lockdown. The findings provide a reference for future air pollution management and urbanization in Tibet.
2 Materials and methods
2.1 Data sources and preprocessing
Tibet has a weak industrial base, but is rich in hydropower resources.34 The Tibetan Statistical Department reported that the third industry accounted for 56% of the gross domestic product, much higher than the industrial production (9%) and construction (27%) in 2021. There were 1.34 million urban residents and 41.53 million tourists in 2021. Mining, the major industrial production sector, was located in the counties (i.e. Dazi, Mozhu Gongka, and Zhongba) instead of in the Tibetan urban areas. Water supply, medicine, and cement were mainly for the urban residential consumption (http://www.xizang.gov.cn/zwgk/zfsj/ndtjgb/). About 5–25% of residential energy consumption was coal, while firewood and dung cake still accounted for 50–60% in Tibet.35 Through literature reviews, vehicle emissions and residential combustion emissions were the main sources for air pollutants in Tibetan urban cities.12,35,36
Fig. S1† shows the locations of seven major cities in Tibet, i.e. Lhasa, Xigaze, Nyingchi, Qamdo, Nagqu, Ngari, and Lhoka. The concentrations of six criteria air pollutants (i.e., NO2, O3, SO2, CO, PM2.5, and PM10) were obtained from the China National Environmental Monitoring Center (CNEMC) (https://air.cnemc.cn:18007/). Only 11 selected sites contained long-term observation data in Tibet. Six of them were further merged with the nearest meteorological observation site in the Integrated Surface Database (ISD) of NOAA (National Oceanic and Atmospheric Administration). These sites were located in the government departments in the urban center. The time resolution of ISD was 3 hours, which was obtained via the “worldmet” R package, including wind speed, wind direction, atmospheric temperature, visibility, dew point, relative humidity, and atmospheric pressure (represented by the sea level pressure). 72 hour back trajectories were calculated for each hour using the Hybrid Single-Particle Lagrangian Integrated Trajectory (HYSPLIT) model, with the NCEP/NCAR reanalysis data as input. The starting height was set as 100 m and then clustered into 6 clusters via the Euclidian distance by “openair” R package.26,37
2.2 Tibetan Lockdown 2022
China Central Television (CCTV) and Tibet Satellite TV reported that there was only one confirmed case in Tibet for 920 consecutive days, since the first appearance of COVID-19 in Wuhan. However, COVID-19 accidentally appeared in Tibet on August 7, 2022, from which the confirmed cases increased exponentially. Meanwhile, the migration index decreased sharply, suggesting that the peak of tourism in Tibet came to an abrupt end (Fig. 1) (Baidu Company, https://qianxi.baidu.com/#/). All major cities implemented lockdown policies before August 14, including the highest epidemic emergency response, community lockdown, and traffic restrictions. The Tibetan government successively cut off all COVID-19 transmission chains in communities from August 26 to 31 in different cities. Via effective actions, Tibetan urban cities successively announced success in achieving “dynamic zero-COVID” conditions, and the growth rate of confirmed cases slowed down after September 8. Therefore, the period from July 1 to August 1 was defined as the “pre-lockdown” period and the period from August 14 to September 8 was the “lockdown” period (Fig. 1).
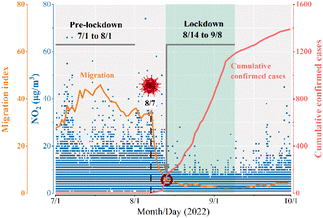 |
| Fig. 1 Temporal variations in the mass concentration of NO2 at observation sites, cumulative confirmed cases of COVID-19 (data from: National Health Commission of China) and migration index (100 times Baidu's migration scale index, reflecting the population moving into Tibet, https://qianxi.baidu.com/#/). | |
2.3 Random forest model and weather normalization
This study assessed the percentage change (P2022) in the observed air pollution concentrations using eqn (1). | 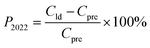 | (1) |
where Cpre and Cld are the average concentrations in the pre-/lockdown period, respectively.
Due to the influence of the Indian monsoon, the usual seasonal variation of aerosols in summer on the Tibetan Plateau cannot be ignored.7,8 The percentage change P2019–2021 was used to build “business-as-usual” conditions (BAU) according to the same periods in 2019–2021. So the net percentage change P* (i.e., the change in air pollutant concentration arising from lockdown effects alone) was given as eqn (2).24,26
| P* = P2022 − P2019–2021 | (2) |
The “rmweather” R package is a random forest model-based weather normalization technique (RF-deweather). Many studies applied it to investigate the variations of air pollutant response to the COVID-19 lockdown.26,38,39 It is nonparametric with fewer limits from assumptions, including sample normality, independence, homoscedasticity, and adherence to other strict parametric assumptions.23,37,40 80% of original data were randomly selected to build the model and the other was used to evaluate the accuracy of the model. Through literature reviews, input factors for the model included time variables (i.e., Unix time, Julian day, day of the week, and hour of the day), meteorological data and trajectory clusters in “Data Sources and Preprocessing”.26,41,42 The initial parameters were as follows: 300 decision trees (n_trees = 300) and 1000 times to sample the database (n_samples = 1000), and others were default in the “rmweather” R package. The statistics of the deweathered result and original data were based on the “openair” R package.43,44 The average correlation coefficients between predicted values and observation values was 0.91. Details are in Table S1.†
Via RF-deweather, the percentage change, P, could be divided into two parts, meteorological effect (Pmet) and anthropogenic effect (Pant) as eqn (3).45
| 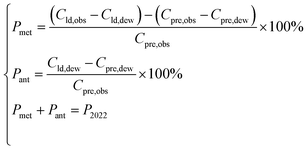 | (3) |
where the subscripts “
obs” and “
dew” were further used to distinguish the results between observation and the deweathered. Meteorological conditions affect the air quality, positively or negatively, and the
Pmet is the net difference of (
Cobs −
Cdew) between lockdown and pre-lockdown periods. The anthropogenic effect was acknowledged as the net effects of the local anthropogenic emission change.
45,46 According to the emission scenarios in Lockdown 2020, vehicle-related emissions and industry emissions were controlled most.
19 The sources of vehicle-related emissions included on-road vehicles and some off-road engines,
i.e. agricultural or industrial equipment, locomotives and commercial generators.
47 SO
2 emitted mainly from coal burning.
22,48 Construction activities and mechanical grinding could emit coarse man-made fugitive dust.
49 Especially for NO
2, O
3, PM
2.5, and CO, the change of the deweathered concentrations roughly represented the contribution of the vehicle-related emissions (
Pveh) in Tibetan urban cities as
eqn (4).
41 | 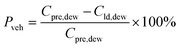 | (4) |
3 Results and discussion
3.1 Overview of air pollutants and meteorological conditions
From July 1 to October 1, 2022, the daily average mass concentrations of NO2, O3, PM2.5, PM10, SO2, and CO were 6.1, 106.9, 4.2, 9.7, 7.3 and 349 μg m−3, respectively, and all met the Ambient Air Quality Standards I in China (GB 3095—2012). 8 hour daily maximum O3 (MDA8 O3) was 100.5, from 34 to 167 μg m−3, up to the WHO Air Quality Guideline (AQG, 100 μg m−3). So ozone is a non-negligible long term health risk substance to the public in Tibet.
Pre-lockdown 2022 and July to September in 2019, 2020, and 2021 could represent the usual summer conditions in Tibetan urban cities. Fig. 2 shows the diurnal variations of six air pollutants for an average of 11 sites. Similar results are summarized in Fig. S2† for the same periods in 2019, 2020 and 2021. Generally, the hourly concentrations of NO2, CO, PM2.5, and PM10 displayed a bimodal distribution in the usual summer, with two peaks from 9:00 to 10:00 and 21:00 to 22:00 (Beijing time), and minima from 5:00 to 7:00 and 14:00 to 17:00, respectively. SO2 only had a peak at 10:00. The diurnal variance of O3 was opposite and unimodal with a peak at 17:00. The result was similar to the typical diurnal cycle mode of six criteria air pollutants in Chinese plain cities. It was mainly ascribed to elevated traffic emissions in rush hours, and the combined effect from a raised boundary layer height and photochemical oxidation reactions.15,50,51 The first valley point of NO2 and the peak point of O3 were at about 6:00 and 20:00, respectively, when sun usually rose and set in summer. During the study periods, the variations of meteorological factors were limited, and the water vapor content in the air tended to slightly increase: RH and dew point increased, and air temperature slightly decreased during the lockdown (Fig. S3†).
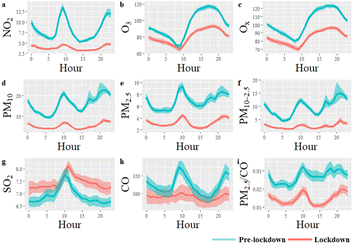 |
| Fig. 2 Diurnal variations in air pollutants during the pre-lockdown and lockdown in 2022. Units: μg m−3 for NO2 (a), O3 (b), total gaseous oxidant (Ox = NO2 + O3) (c), PM10 (d), PM2.5 (e), coarse particles (PM10–2.5 = PM10–PM2.5) (f), SO2 (g) and CO (h); dimensionless for the rate of PM2.5/CO (i). Error bars represent the 95% confidence interval of the hourly mean via “openair” R-package. | |
3.2 Air quality change during the lockdown
According to the BAU conditions, P2022 was equivalent to P* for all sites, suggesting that general changes of air pollutants cannot be fully attributable to the seasonal variation of meteorology (Fig. S4†). Fig. 3 shows that the mean change rates (P2022) were PM10 (−65%) < NO2 (−53%) < PM2.5 (−52%) < O3 (−15%) < CO (−11%) < SO2 (10%) at Tibetan urban cities. Particulate matter and NO2 both displayed the largest reduction due to the lockdown. Fig. 3 shows the meteorological effect (Pmet) and anthropogenic effect (Pant), and Table 1 lists the dewearthered concentrations and estimated Pveh.
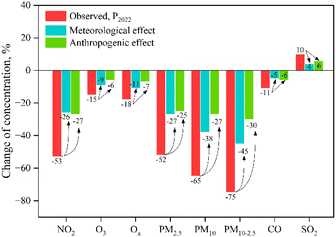 |
| Fig. 3 Change rate of concentrations due to the Tibetan Lockdown 2022, and results of the random forest model-based weather normalization, including assigned anthropogenic effects and meteorological effects. | |
Table 1 Dewearthered concentration and contribution of vehicle-related emission (Pveh) via random forest model-based weather normalization
Concentration, μg m−3 |
CO |
NO2 |
O3 |
Ox |
PM2.5 |
Pre-lockdown |
Mean |
380 |
6.8 |
84 |
90 |
6.2 |
Std. |
150 |
2.7 |
16 |
18 |
1.2 |
Lockdown |
Mean |
360 |
4.8 |
78 |
83 |
4.5 |
Std. |
150 |
1.8 |
15 |
17 |
0.9 |
Average Pveh, % |
6 |
29 |
7 |
8 |
28 |
|
3.2.1 SO2 and CO.
Our study shows that SO2 slightly increased from 6.3 to 6.9 μg m−3, mainly in the afternoon (Fig. 2g), and two peaks of CO almost disappeared and its diurnal profile had a 95% confidence interval (Fig. 2h). The average changes were small, about −11% and 10% for CO and SO2, respectively (Fig. 3). The variations were consistent with those (about ±20%) in other cities in East China during the COVID lockdown periods.52 The reason for SO2 increase was usually attributed to the power plants and raw material industry, as the main contributors to SO2 emissions. They must operate for the electricity consumption of households and have uninterrupted industrial processes. Moreover, traffic reduction induced the disappearance of CO peaks from 370 to 300 μg m−3 in rush hours and decreased CO from 320 to 290 μg m−3 in non-rush hours in Tibetan urban areas (Fig. 2h). It was reported that household stoves in Tibet also emitted CO when burning firewood and dung cake, which could contribute 0.4–0.5 Mt, five times the transportation emission (∼77 Kt) by the latest assessment.35,47 After the RF-deweather process, we roughly estimated CO sources accounting for ∼6% from vehicle-related emissions (Pveh), suggesting that residential combustion emissions dominated for CO in Tibetan cities (Table 1).
3.2.2 NO2.
The vehicle-related emission was the major source of NO2 in cities.42,53 According to the Multi-resolution Emission Inventory for China (MEIC), the vehicle-related emission accounted for 85% of total nitrogen oxide (NOx = NO + NO2) emission in Tibet, twice higher than the national average level at 36.5 ± 12.2%.47 During the Tibetan Lockdown 2022, an approximately 53% decrease rate of NO2 in Tibet was observed. The result was higher than 42% during the Lockdown 2020 (Fig. 3 and 4). The transportation frequency in Tibet was less affected in Lockdown 2020.31 The range of the NO2 decrease rate around the world was only up to −50% in some severely affected cities (e.g., Wuhan and Milan) due to the lockdown.23Fig. 2a shows that the diurnal fluctuation of NO2 basically disappears and smoothly ranged from 3 to 5 μg m−3. The diurnal profile was similar to the observation at the background site in the Tibetan Plateau (4.4 ± 2.7 μg m−3).54Table 1 shows that NO2 sources accounted for ∼29% from vehicle-related emissions (Pveh), via RF-deweather. These parts of emissions almost disappeared due to the lockdown and led to a −27% decrease in the observed concentration. The others were attributed to the conducive meteorological conditions, and Pmet was −26% (Fig. 3). We noticed [1 − Pveh] up to ∼71% and deweathered concentrations deceasing from 6.8 to 4.8 μg m−3 due to the lockdown. The result reflected that the residential combustion emissions remained the high contribution in Tibetan cities during the lockdown.
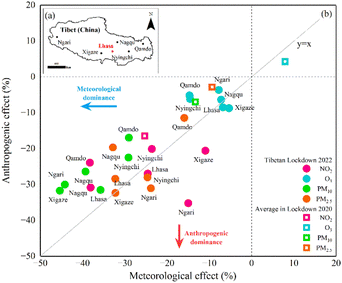 |
| Fig. 4 Anthropogenic effects and meteorological effects on changes of air pollutants in Tibetan Lockdown 2022 for six cities. (a) Locations of the studied Tibetan cities. Lhoka was not included, because meteorological data were not accessible. (b) Open squares were the average changes for the Lockdown 2020, via the random forest model-based weather normalization. | |
3.2.3 Surface O3.
Surface O3 is a secondary pollutant produced by photochemical oxidation of volatile organic compounds (VOCs) and carbon monoxide (CO) in the presence of NOx. In the presence of sunlight, O3 is chemically produced from the oxidation of VOCs in the presence of NOx. On the other hand, in the process of NOx titration, freshly emitted NO rapidly reacts with O3 to form NO2 (titration reaction). It was acknowledged that the surface O3 in Tibet could be affected more by natural sources (including biogenic sources, lightning and soil), stratospheric intrusion, and long-range transport.13,14,55 For example, Yin et al. (2017) proposed that stratospheric intrusion contributed 18.2 ± 2.6% of the ozone variance at a Tibetan remote site, 21.10% in spring but only 0.41% in Autumn.56 Tibetan Lockdown 2022 is an opportunity to generally evaluate the role of local anthropogenic pollutants in surface O3. Fig. 3 shows the weak negative ozone anomalies (about −15%) in Tibet due to the lockdown. Via RF-deweather, anthropogenic contributions accounted for ∼7% from vehicle-related emissions (Pveh) in Table 1.
The diurnal profile did show decreasing trends of O3 and Ox (Fig. 2b and c). Before the lockdown, the pattern of daytime O3 was similar to the typical parabola pattern and had a higher range from the peak to the valley point. It was similar to the condition driven by the atmospheric photochemistry in polluted plain areas,15,51 and different from the smooth O3 profiles at the remote sites on the TP.54,56,57 NOx from local vehicle-related emission was likely more sufficient than VOCs from 6:00 to 10:00 in the usual summer morning, making a strong titration reaction, and Ox remained at about 80 μg m−3 (Fig. 2c). During the lockdown, the O3 and Ox concentrations both decreased significantly, and the maximum declines were from 11:00 to 20:00, when NOx was no longer sufficient, resulting in a NOx-limited regime for surface O3. In addition, a broader platform-like diurnal profile of surface O3 was observed after sunrise, followed by a shorter climb (Fig. 3b and c). These were different from the same periods in 2019–2021 (Fig. S2†) and similar to observations in TP remote sites.56,57 Therefore, we can conclude that O3 in urban areas on the TP is strongly related to NOx anthropogenic emission and regional O3 is mainly contributed by stratospheric intrusion and long-range transport.
3.2.4 PM10 and PM2.5.
The local epidemic in Tibet had a great impact on atmospheric particles. PM10 and PM2.5 both decreased up to −50%, due to the lockdown. Their decrease rates in Lockdown 2020 averaged −20% in China.27,28,58 PM10–2.5 was mainly dominated by man-made fugitive dust from construction activities, mechanical grinding and roads, natural dust from the ground, and biogenic sources.49Fig. 3 shows that observed PM10–2.5 decreased 75%, much higher than −52% in PM2.5. The machine learning method further calculated meteorological effects accounting for 45%, 38% and 27% of the decreases of PM10–2.5, PM10 and PM2.5, respectively. The anthropogenic effects were similar, 25–30% (Fig. 3). PM2.5/CO is a good tool to show the formation rate of secondary aerosols.26,27 We noticed that the diurnal variation almost disappeared for PM10 and primary PM10–2.5 during the lockdown, and the diurnal patterns of PM2.5 and PM2.5/CO just weakened but remained bimodal (Fig. 2). It suggested that the reduction of atmospheric oxidation capacity (Ox) weakened the formation processes of secondary aerosol in Tibetan urban cities due to the Tibetan Lockdown 2022. In Tibet, 38% of the primary PM2.5 emission was set as the vehicle-related contribution in the MEIC.47 Zhao et al. (2022) reported that vehicle-related organic aerosol accounted for ∼14% in fine particles in Lhasa.36 In Table 1, we roughly estimated that 28% of PM2.5 emissions were assigned to vehicle-related emissions. Our studies supplied a reference benchmark scene for the emission inventory update and revision: the emissions from residential combustion emissions (∼72%) could still dominate the primary PM2.5 in Tibetan urban cities, and deweathered PM2.5 concentrations varied from 6.2 to 4.5 μg m−3 in the studied cities with negligible traffic flux due to the lockdown.
3.3 Comparison between lockdowns 2022 and 2020
During the Tibetan Lockdown 2022, meteorological conditions were conducive to the removal of air pollutants, i.e. PM10 (−38%) < PM2.5 (−27%) < NO2 (−26%) < O3 (−9%) (Fig. 3). Different Tibetan cities had similar trends, and meteorological effects were slightly larger (1.3 ± 0.6) than anthropogenic effects (Fig. 4). Only PM2.5 and NO2 in a few cities were dominated by anthropogenic effects, such as NO2 in Ngari and Xigaze cities. The result is likely related to the type and total number of local motor vehicles in different cities.
Tibet had a lockdown from January 26 to February 19 in 2020 and a pre-lockdown from January 1 to 20 in 2020.32,33 We found that anthropogenic effects were more important for the NO2 decrease in 2022 than Lockdown 2020 (Fig. 4). PM2.5 and PM10 both decreased more in Tibetan Lockdown 2022, under more stringent traffic control and more conducive weather (Fig. 4). The changes of O3 in 2022 and 2020 were in the opposite phase as shown in Fig. 4. Compared with 2022, the diurnal profiles of six criteria air pollutants and indices (Ox, PM2.5/CO, and PM10–2.5) only weakened slightly in 2020, and smooth diurnal trends were not found (Fig. S5†). The result suggested that the pollutant reductions were much larger and then weakened the atmospheric oxidizing capacity during the Tibetan Lockdown 2022. Importantly, O3 compensated for the drops in NO2 (about 10 μg m−3), and hourly Ox rarely changed in 2020 (Fig. S5†). It suggested that decreases of NOx were not enough and NOx was still sufficient relative to VOCs in the Lockdown 2020. The titration reaction of NO and O3 was probably depressed, and thus promoted the elevation of the O3 concentration, especially in the night. The net effects on the total oxidant (Ox = NO2 + O3) were limited. Other researchers reported similar results in Tibet during the Lockdown 2020 and estimated that O3 increased about 20%.39,52 Global studies also reported that the gains in O3 compensated for drops in NO2 due to the Lockdown 2020.23,52 The negative ozone anomalies due to the Lockdown 2020 were rarely reported and only in Aberdeen city, UK (−36 ± 8.6%), and a mountain site near urban areas in Italy (−16%).24,25 They proposed that great NO2 reductions in urban areas could decrease surface O3 under a NOx-limited regime and even affect nearby mountains.24,25 In Tibetan urban cities, the greater NO2 reductions (−53%) also led to a negative ozone anomaly (−15%) (Fig. 3), as the COVID lockdown policy was more stringent in 2022 than those in Lockdown 2020. Considering the max hourly concentration of O3, MDA8 O3 decreased up to −20%, from 116 to 93 μg m−3, and it met the AQG of WHO (Fig. S4†). Therefore, vehicle-related emission control was a potential way to reduce the public health risk from O3 in Tibetan urban areas.
4 Conclusions
The sudden appearance of COVID on August 7, 2022, broke the 920-consecutive-day safe condition in Tibet. The rapid decline of NO2 possibly led to a NOx-limited regime for surface O3, in Tibetan urban areas, and a net negative anomaly of surface O3 was for the first time found followed by larger decreases of PM10 and PM2.5. This was different from the air quality change in Lockdown 2020, when air pollutant declines were not large enough to offset the O3 enhancement by the weakened titration reaction in Tibet. Our study conducted diurnal variation analysis using “business-as-usual” analysis and random forest model-based weather normalization analysis during the lockdown 2022 in Tibet. Differences between lockdowns 2020 and 2022 did provide clear evidence that local anthropogenic emissions influenced the atmospheric environment in Tibetan cities, especially NO2, O3, PM2.5, and PM10.
In summer, we proposed that the surface O3 concentrations become smaller than 100 μg m−3 (the AQG of WHO) by reducing the vehicle-related emission and controlling NO2 at 2.5–5 μg m−3. For local anthropogenic emissions, the contributions of vehicle-related emissions were estimated via RF-deweather: NO2 (29%) > PM2.5 (28%) > O3 (7%) > CO (6%). The other emissions were mainly assigned to residential combustion emissions due to weak industrialization in Tibetan cities. For observation concentrations, meteorological effects were more conducive to the changes of air pollutants, and were 1.3 ± 0.6 times higher than the net effects from emission changes during the Tibetan Lockdown 2022. This study serves as a general reference for future air pollution control in Tibetan cities. We should point that the monitoring sites are fewer in Tibet than those in Chinese plain cities, which might lead to certain uncertainty in this study. In the future, the construction of monitoring networks and detailed source apportionments could provide more accurate references for air pollution management and urbanization in Tibet. Meanwhile, the advances of public transport, railway transport, and new energy vehicle plan will significantly improve urban air quality through a series of great projects in the future. More attention should also be paid to reducing the more intensive local pollutant emissions from residential combustion emissions and vehicles in Tibetan urban cities.
Author contributions
Xiyao Chen: writing – original draft, methodology, and visualization. Fan Zhang: methodology and writing – review & editing. Dianguo Zhang: resources and funding acquisition. Liang Xu: data curation and resources. Rui Liu: data curation and resources. Xiaomi Teng: data curation and resources. Xin Zhang: methodology and writing – review & editing. Shuo Wang: data curation and resources. Weijun Li: conceptualization, supervision, and funding acquisition.
Conflicts of interest
There are no conflicts to declare.
Acknowledgements
This work was supported by the National Natural Science Foundation of China (42277080), Innovative Development Special Project of China Meteorological Administration (CXFZ2022J034), and Fundamental Research Funds for the Central Universities (2022FZZX01-05).
Notes and references
- Q. Zhang, X. Jiang, D. Tong, S. J. Davis, H. Zhao, G. Geng, T. Feng, B. Zheng, Z. Lu, D. G. Streets, R. Ni, M. Brauer, A. van Donkelaar, R. V. Martin, H. Huo, Z. Liu, D. Pan, H. Kan, Y. Yan, J. Lin, K. He and D. Guan, Transboundary health impacts of transported global air pollution and international trade, Nature, 2017, 543, 705–709 CrossRef CAS.
- A. Jbaily, X. Zhou, J. Liu, T. H. Lee, L. Kamareddine, S. Verguet and F. Dominici, Air pollution exposure disparities across US population and income groups, Nature, 2022, 601, 228–233 CrossRef CAS PubMed.
- P. Yin, M. Brauer, A. J. Cohen, H. Wang, J. Li, R. T. Burnett, J. D. Stanaway, K. Causey, S. Larson, W. Godwin, J. Frostad, A. Marks, L. Wang, M. Zhou and C. J. L. Murray, The effect of air pollution on deaths, disease burden, and life expectancy across China and its provinces, 1990-2017: an analysis for the Global Burden of Disease Study 2017, Lancet Planet. Health, 2020, 4, E386–E398 CrossRef.
- X. Chen, F. Li, J. Zhang, W. Zhou, X. Wang and H. Fu, Spatiotemporal mapping and multiple driving forces identifying of PM2.5 variation and its joint management strategies across China, J. Cleaner Prod., 2020, 250, 119534 CrossRef CAS.
- N. Unger, Y. Zheng, X. Yue and K. L. Harper, Mitigation of ozone damage to the world's land ecosystems by source sector, Nat. Clim. Change, 2020, 10, 134–137 CrossRef CAS.
- T. Yao, T. Bolch, D. Chen, J. Gao, W. Immerzeel, S. Piao, F. Su, L. Thompson, Y. Wada, L. Wang, T. Wang, G. Wu, B. Xu, W. Yang, G. Zhang and P. Zhao, The imbalance of the Asian water tower, Nat. Rev. Earth Environ., 2022, 3, 618–632 CrossRef.
- S. Kang, Q. Zhang, Y. Qian, Z. Ji, C. Li, Z. Cong, Y. Zhang, J. Guo, W. Du, J. Huang, Q. You, A. K. Panday, M. Rupakheti, D. Chen, O. Gustafsson, M. H. Thiemens and D. Qin, Linking atmospheric pollution to cryospheric change in the Third Pole region: current progress and future prospects, Natl. Sci. Rev., 2019, 6, 796–809 CrossRef CAS.
- C. Li, F. Yan, S. Kang, C. Yan, Z. Hu, P. Chen, S. Gao, C. Zhang, C. He, S. Kaspari and A. Stubbins, Carbonaceous matter in the atmosphere and glaciers of the Himalayas and the Tibetan plateau: an investigative review, Environ. Int., 2021, 146, 106281 CrossRef CAS PubMed.
- Q. Yuan, J. Xu, L. Liu, A. Zhang, Y. Liu, J. Zhang, X. Wan, M. Li, K. Qin, Z. Cong, Y. Wang, S. Kang, Z. Shi, M. Pósfai and W. Li, Evidence for Large Amounts of Brown Carbonaceous Tarballs in the Himalayan Atmosphere, Environ. Sci. Technol. Lett., 2020, 8, 16–23 CrossRef.
- C. Li, S. Kang and F. Yan, Importance of Local Black Carbon Emissions to the Fate of Glaciers of the Third Pole, Environ. Sci. Technol., 2018, 52, 14027–14028 CrossRef CAS PubMed.
- X. Ma, Z. Yang, J. Wang and F. Han, Mapping population on Tibetan Plateau by fusing VIIRS data and nighttime Tencent location-based services data, Ecol. Indic., 2022, 139, 108893 CrossRef.
- L. Ran, W. L. Lin, Y. Z. Deji, B. La, P. M. Tsering, X. B. Xu and W. Wang, Surface gas pollutants in Lhasa, a highland city of Tibet – current levels and pollution implications, Atmos. Chem. Phys., 2014, 14, 10721–10730 CrossRef.
- J. Yang, K. Wang, M. Lin, X. Yin and S. Kang, Not biomass burning but stratospheric intrusion dominating tropospheric ozone over the Tibetan Plateau, Proc. Natl. Acad. Sci. U. S. A., 2022, 119, e2211002119 CrossRef CAS PubMed.
- M. Li, J. Mao, S. Chen, J. Bian, Z. Bai, X. Wang, W. Chen and P. Yu, Significant contribution of lightning NOx to summertime surface O3 on the Tibetan Plateau, Sci. Total Environ., 2022, 829, 154639 CrossRef CAS PubMed.
- M. Kuerban, Y. Waili, F. Fan, Y. Liu, W. Qin, A. J. Dore, J. Peng, W. Xu and F. Zhang, Spatio-temporal patterns of air pollution in China from 2015 to 2018 and implications for health risks, Environ. Pollut., 2020, 258, 113659 CrossRef CAS PubMed.
- Y. Liu, X. Xu, X. Yang, J. He, D. Ji and Y. Wang, Significant Reduction in Fine Particulate Matter in Beijing during 2022 Beijing Winter Olympics, Environ. Sci. Technol. Lett., 2022, 9, 822–828 CrossRef CAS.
- M. Gao, Y. Yang, H. Liao, B. Zhu, Y. Zhang, Z. Liu, X. Lu, C. Wang, Q. Zhou, Y. Wang, Q. Zhang, G. R. Carmichael and J. Hu, Reduced light absorption of black carbon (BC) and its influence on BC-boundary-layer interactions during “APEC Blue”, Atmos. Chem. Phys., 2021, 21, 11405–11421 CrossRef CAS.
- H. Ren, W. Hu, L. Wei, S. Yue, J. Zhao, L. Li, L. Wu, W. Zhao, L. Ren, M. Kang, Q. Xie, S. Su, X. Pan, Z. Wang, Y. Sun, K. Kawamura and P. Fu, Measurement report: vertical distribution of biogenic and anthropogenic secondary organic aerosols in the urban boundary layer over Beijing during late summer, Atmos. Chem. Phys., 2021, 21, 12949–12963 CrossRef CAS.
- P. Wang, K. Chen, S. Zhu, P. Wang and H. Zhang, Severe air pollution events not avoided by reduced anthropogenic activities during COVID-19 outbreak, Resour., Conserv. Recycl., 2020, 158, 104814 CrossRef PubMed.
- A. Josephson, T. Kilic and J. D. Michler, Socioeconomic impacts of COVID-19 in low-income countries, Nature Human Behaviour, 2021, 5, 557–565 CrossRef PubMed.
- J. Zhang, Y.-H. Lim, Z. J. Andersen, G. Napolitano, S. M. Taghavi Shahri, R. So, M. Plucker, M. Danesh-Yazdi, T. Cole-Hunter, J. Therming Jørgensen, S. Liu, M. Bergmann, A. Jayant Mehta, L. H. Mortensen, W. Requia, T. Lange, S. Loft, N. Kuenzli, J. Schwartz and H. Amini, Stringency of COVID-19 Containment Response Policies and Air Quality Changes: A Global Analysis across 1851 Cities, Environ. Sci. Technol., 2022, 56, 12086–12096 CrossRef CAS PubMed.
- L. Liu, J. Zhang, R. Du, X. Teng, R. Hu, Q. Yuan, S. Tang, C. Ren, X. Huang, L. Xu, Y. Zhang, X. Zhang, C. Song, B. Liu, G. Lu, Z. Shi and W. Li, Chemistry of Atmospheric Fine Particles during the COVID-19 Pandemic in a Megacity of Eastern China, Geophys. Res. Lett., 2021, 48, 2020GL091611 CAS.
- C. A. Keller, M. J. Evans, K. E. Knowland, C. A. Hasenkopf, S. Modekurty, R. A. Lucchesi, T. Oda, B. B. Franca, F. C. Mandarino, M. V. Díaz Suárez, R. G. Ryan, L. H. Fakes and S. Pawson, Global impact of COVID-19 restrictions on the surface concentrations of nitrogen dioxide and ozone, Atmos. Chem. Phys., 2021, 21, 3555–3592 CrossRef CAS.
- J. D. Lee, W. S. Drysdale, D. P. Finch, S. E. Wilde and P. I. Palmer, UK surface NO2 levels dropped by 42% during the COVID-19 lockdown: impact on surface O3, Atmos. Chem. Phys., 2020, 20, 15743–15759 CrossRef CAS.
- P. Cristofanelli, J. Arduni, F. Serva, F. Calzolari, P. Bonasoni, M. Busetto, M. Maione, M. Sprenger, P. Trisolino and D. Putero, Negative ozone anomalies at a high mountain site in northern Italy during 2020: a possible role of COVID-19 lockdowns?, Environ. Res. Lett., 2021, 16, 074029 CrossRef CAS.
- Z. Shi, C. Song, B. Liu, G. Lu, J. Xu, T. V. Vu, R. J. R. Elliott, W. Li, W. J. Bloss and R. M. Harrison, Abrupt but smaller than expected changes in surface air quality attributable to COVID-19 lockdowns, Sci. Adv., 2021, 7, eabd6696 CrossRef CAS PubMed.
- X. Huang, A. Ding, J. Gao, B. Zheng, D. Zhou, X. Qi, R. Tang, J. Wang, C. Ren, W. Nie, X. Chi, Z. Xu, L. Chen, Y. Li, F. Che, N. Pang, H. Wang, D. Tong, W. Qin, W. Cheng, W. Liu, Q. Fu, B. Liu, F. Chai, S. J. Davis, Q. Zhang and K. He, Enhanced secondary pollution offset reduction of primary emissions during COVID-19 lockdown in China, Natl. Sci. Rev., 2021, 8, nwaa137 CrossRef CAS PubMed.
- T. Le, Y. Wang, L. Liu, J. Yang, Y. L. Yung, G. Li and J. H. Seinfeld, Unexpected air pollution with marked emission reductions during the COVID-19 outbreak in China, Science, 2020, 369, 702–706 CrossRef CAS PubMed.
- A. Breevoort, G. A. Carosso and M. A. Mostajo-Radji, High-altitude populations need special considerations for COVID-19, Nat. Commun., 2020, 11, 3280 CrossRef CAS PubMed.
- Z. Liu, D. Cui, Z. Deng, Y. Wang, H. Zhong, X. Yue, N. Zhang, B. Chen, X. Ren, W. Wei, Y. Lü, K. Jiang, X. Dou, B. Zhu, R. Guo, T. Sun, P. Ke, D. Guan and P. Gong, Impact on China's CO2 emissions from COVID-19 pandemic, Chin. Sci. Bull., 2020, 66, 1912–1922 CrossRef.
- N. Jiang, S. Li, C. Suzhen, J. Wei, B. Wang, N. Qin and X. Duan, Transportation Activity Patterns of Chinese Population during the COVID-19 Epidemic, Res. Environ. Sci., 2020, 33, 1675–1682 Search PubMed.
- F. Xie, Y. C. Lin, L. Ren, C. Gul, J. Q. Wang, F. Cao, Y. X. Zhang, T. Xie, J. Y. Wu and Y. L. Zhang, Decrease of atmospheric black carbon and CO2 concentrations due to COVID-19 lockdown at the Mt. Waliguan WMO/GAW baseline station in China, Environ. Res., 2022, 211, 112984 CrossRef CAS PubMed.
- Y. Liu, Y. Wang, Y. Cao, X. Yang, T. Zhang, M. Luan, D. Lyu, A. D. A. Hansen, B. Liu and M. Zheng, Impacts of COVID-19 on Black Carbon in Two Representative Regions in China: Insights Based on Online Measurement in Beijing and Tibet, Geophys. Res. Lett., 2021, 48, e2021GL092770 CAS.
- W. Shen, W. Han, T. J. Wallington and S. L. Winkler, China Electricity Generation Greenhouse Gas Emission Intensity in 2030: Implications for Electric Vehicles, Environ. Sci. Technol., 2019, 53, 6063–6072 CrossRef CAS PubMed.
- K. Liu, Q. Wu, Y. Ren and S. Wang, Air Pollutant Emissions from Residential Solid Fuel Combustion in the Pan-Third Pole Region, Environ. Sci. Technol., 2022, 56, 15347–15355 CrossRef CAS PubMed.
- W. Zhao, X. Zhang, L. Zhai, X. Shen and J. Xu, Chemical characterization and sources of submicron aerosols in Lhasa on the Qinghai-Tibet Plateau: insights from high-resolution mass spectrometry, Sci. Total Environ., 2022, 815, 152866 CrossRef CAS PubMed.
- S. K. Grange, D. C. Carslaw, A. C. Lewis, E. Boleti and C. Hueglin, Random forest meteorological normalisation models for Swiss PM10 trend analysis, Atmos. Chem. Phys., 2018, 18, 6223–6239 CrossRef CAS.
- S. K. Grange, J. D. Lee, W. S. Drysdale, A. C. Lewis, C. Hueglin, L. Emmenegger and D. C. Carslaw, COVID-19 lockdowns highlight a risk of increasing ozone pollution in European urban areas, Atmos. Chem. Phys., 2021, 21, 4169–4185 CrossRef CAS.
- Q. Dai, L. Hou, B. Liu, Y. Zhang, C. Song, Z. Shi, P. K. Hopke and Y. Feng, Spring Festival and COVID-19 Lockdown: Disentangling PM Sources in Major Chinese Cities, Geophys. Res. Lett., 2021, 48, e2021GL093403 CAS.
- G. He, Y. Pan and T. Tanaka, The short-term impacts of COVID-19 lockdown on urban air pollution in China, Nature Sustainability, 2020, 3, 1005–1011 CrossRef.
- S. K. Grange and D. C. Carslaw, Using meteorological normalisation to detect interventions in air quality time series, Sci. Total Environ., 2019, 653, 578–588 CrossRef CAS PubMed.
- H. Zheng, S. Kong, N. Chen, Y. Yan, D. Liu, B. Zhu, K. Xu, W. Cao, Q. Ding, B. Lan, Z. Zhang, M. Zheng, Z. Fan, Y. Cheng, S. Zheng, L. Yao, Y. Bai, T. Zhao and S. Qi, Significant changes in the chemical compositions and sources of PM2.5 in Wuhan since the city lockdown as COVID-19, Sci. Total Environ., 2020, 739, 140000 CrossRef CAS.
- L. Xu, J. Zhang, X. Sun, S. Xu, M. Shan, Q. Yuan, L. Liu, Z. Du, D. Liu, D. Xu, C. Song, B. Liu, G. Lu, Z. Shi and W. Li, Variation in Concentration and Sources of Black Carbon in a Megacity of China during the COVID-19 Pandemic, Geophys. Res. Lett., 2020, 47, e2020GL090444 CAS.
- C. Ou, F. Li, J. Zhang, Y. Hu, X. Chen, S. Kong, J. Guo and Y. Zhou, Multiple driving factors and hierarchical management of PM2.5: evidence from Chinese central urban agglomerations using machine learning model and GTWR, Urban Climate, 2022, 46, 101327 CrossRef.
- L. Qu, S. Liu, L. Ma, Z. Zhang, J. Du, Y. Zhou and F. Meng, Evaluating the meteorological normalized PM2.5 trend (2014-2019) in the “2+26” region of China using an ensemble learning technique, Environ. Pollut., 2020, 266, 115346 CrossRef CAS.
- H. Yin, Y. Sun, J. Notholt, M. Palm, C. Ye and C. Liu, Quantifying the drivers of surface ozone anomalies in the urban areas over the Qinghai-Tibet Plateau, Atmos. Chem. Phys., 2022, 22, 14401–14419 CrossRef CAS.
- M. Li, H. Liu, G. Geng, C. Hong, F. Liu, Y. Song, D. Tong, B. Zheng, H. Cui, H. Man, Q. Zhang and K. He, Anthropogenic emission inventories in China: a review, Natl. Sci. Rev., 2017, 4, 834–866 CrossRef CAS.
- R. J. Huang, Y. Zhang, C. Bozzetti, K. F. Ho, J. J. Cao, Y. Han, K. R. Daellenbach, J. G. Slowik, S. M. Platt, F. Canonaco, P. Zotter, R. Wolf, S. M. Pieber, E. A. Bruns, M. Crippa, G. Ciarelli, A. Piazzalunga, M. Schwikowski, G. Abbaszade, J. Schnelle-Kreis, R. Zimmermann, Z. An, S. Szidat, U. Baltensperger, I. El Haddad and A. S. Prevot, High secondary aerosol contribution to particulate pollution during haze events in China, Nature, 2014, 514, 218–222 CrossRef CAS PubMed.
- H. Qiu, L. Wang, L. Zhou and J. Pan, Coarse particles (PM2.5-10) and cause-specific hospitalizations in southwestern China: association, attributable risk and economic costs, Environ. Res., 2020, 190, 110004 CrossRef CAS.
- S. Zhao, Y. Yu, D. Yin, J. He, N. Liu, J. Qu and J. Xiao, Annual and diurnal variations of gaseous and particulate pollutants in 31 provincial capital cities based on in situ air quality monitoring data from China National Environmental Monitoring Center, Environ. Int., 2016, 86, 92–106 CrossRef CAS PubMed.
- R. Li, Z. Wang, L. Cui, H. Fu, L. Zhang, L. Kong, W. Chen and J. Chen, Air pollution characteristics in China during 2015-2016: spatiotemporal variations and key meteorological factors, Sci. Total Environ., 2019, 648, 902–915 CrossRef CAS PubMed.
- Y. Zhao, K. Zhang, X. Xu, H. Shen, X. Zhu, Y. Zhang, Y. Hu and G. Shen, Substantial Changes in Nitrogen Dioxide and Ozone after Excluding Meteorological Impacts during the COVID-19 Outbreak in Mainland China, Environ. Sci. Technol. Lett., 2020, 7, 402–408 CrossRef CAS.
- Q. Yuan, B. Qi, D. Hu, J. Wang, J. Zhang, H. Yang, S. Zhang, L. Liu, L. Xu and W. Li, Spatiotemporal variations and reduction of air pollutants during the COVID-19 pandemic in a megacity of Yangtze River Delta in China, Sci. Total Environ., 2021, 751, 141820 CrossRef CAS PubMed.
- B. Han, W. Yang, J. Wang, X. Zhao, B. Yin, X. Wang, C. Geng, X. Dou, X. Xu and Z. Bai, Characterizations and Potential Formation Pathways of Atmospheric Inorganic Ions at a National Background Site in the Northeastern Qinghai-Tibet Plateau during Autumn Season, J. Geophys. Res.: Atmos., 2020, 125, e2020JD032819 Search PubMed.
- R. Dang, H. Liao and Y. Fu, Quantifying the anthropogenic and meteorological influences on summertime surface ozone in China over 2012-2017, Sci. Total Environ., 2021, 754, 142394 CrossRef CAS PubMed.
- X. Yin, S. Kang, B. de Foy, Z. Cong, J. Luo, L. Zhang, Y. Ma, G. Zhang, D. Rupakheti and Q. Zhang, Surface ozone at Nam Co in the inland Tibetan Plateau: variation, synthesis comparison and regional representativeness, Atmos. Chem. Phys., 2017, 17, 11293–11311 CrossRef CAS.
- W. Lin, X. Xu, X. Zheng, J. Dawa, C. Baima and J. Ma, Two-year
measurements of surface ozone at Dangxiong, a remote highland site in the Tibetan Plateau, J. Environ. Sci., 2015, 31, 133–145 CrossRef CAS PubMed.
- B. Chu, S. Zhang, J. Liu, Q. Ma and H. He, Significant concurrent decrease in PM2.5 and NO2 concentrations in China during COVID-19 epidemic, J. Environ. Sci., 2021, 99, 346–353 CrossRef CAS PubMed.
|
This journal is © The Royal Society of Chemistry 2023 |