High-resolution maps of carbon dioxide and moisture fluxes over an urban neighborhood†
Received
17th August 2022
, Accepted 3rd June 2023
First published on 5th June 2023
Abstract
Based on the Monin–Obukhov similarity theory and challenging the Reynolds analogy, the aerodynamic resistance approach to estimate the transfer of sensible heat (QH) can be used as a proxy to investigate the surface–atmosphere exchange of any passive scalar, such as carbon dioxide (CO2) and moisture (H2O). As a proof of concept, a bicycle was instrumented to measure georeferenced mixing ratios of CO2 and H2O every second along a defined route across a residential neighborhood of Singapore during ∼1.5 hours periods. These measurements are used in conjunction with readings of incoming and outgoing longwave radiation, and fluxes of QH collected from a tall eddy covariance flux tower located within the study area, to derive exchange rates of CO2 and H2O along the transect route considering the bulk aerodynamic resistance to QH as equivalent to the bulk aerodynamic resistance for both trace gases. An interpolation subroutine based on the original Delaunay triangulation is then applied to interpolate the observed fluxes across the neighborhood for 20 × 20 m grid cells. This approach is able to identify hotspots of CO2 emissions, and spots of evapotranspiration or anthropogenic moisture directly associated with the energy channeled into latent heat. No other method based on field observations can deliver information on the exchange of CO2 and H2O with such fine spatial resolution. For the particular neighborhood assessed in this study, larger emission rates of CO2 are observed at traffic intersections along major roads, as well as at an urban block with multiple eateries and coffee shops. With respect to fluxes of H2O, high evapotranspiration rates are observed along a drainage channel at one end of the neighborhood and along blocks with narrow and densely tree-lined streets. The sum of the interpolated fluxes from all grid cells for each individual set of measurements agrees within one standard deviation with the long-term fluxes of CO2 and H2O measured by eddy covariance over the entire neighborhood.
Environmental significance
Smart and effective measures to mitigate emissions of greenhouse gases and urban warming must be based on robust and useful information to identify sources and sinks at scales that are directly related to the human activities that generate the emissions or modify the respective components of the energy balance (i.e., heat fluxes). Only then can they be meaningful for practical policy. Stakeholders need to know the locations of the emissions and factors that alter the urban microclimate at the scale of neighborhoods, blocks, buildings, roads, and traffic intersections to take informed action. Maps at fine spatial resolution of fluxes of carbon dioxide and moisture can help in this regard, especially if they are built from direct field observations to reduce uncertainties.
|
1. Introduction
Accurate and updated emission inventories of greenhouse gases (GHG) at different spatial and temporal scales are needed to develop effective strategies to mitigate climate change. Similarly, detailed maps of moisture (H2O) giving rise to latent heat (QE) are needed to improve the thermal environment. At city scale, emission inventories and moisture maps need to account for all emission sources and sinks, and climatological processes occurring within the urban landscape. Integrated information systems based on different methodologies, including bottom-up approaches using emission factors and activity data, direct observations, and systems of data assimilation into transport models and urban schemes are required for such efforts.1 The methodology to choose depends on the particular conditions of each city and the desired outcomes.
Among the observational methods, eddy covariance (EC) flux towers are the only alternative to directly measure net exchange of carbon dioxide (CO2) and H2O, and energy fluxes of QE and sensible heat (QH) between the urban surface and overlaying atmosphere at neighborhood scale.2–4 They have many advantages over other methods. They directly measure the vertical exchange of any scalar entity through turbulent motions (eddies) within the mean air flow. These flux measurements include contributions from all major and minor natural and anthropogenic sources and sinks; they are in situ, non-intrusive, quasi-continuous and with proper selection of the footprint can represent a large upwind extent similar to the size of a complete urban neighborhood.
The EC method provides valuable flux data to investigate the anthropogenic and biogeochemical processes driving the release and uptake of CO2 in urban ecosystems, as well as to validate the accuracy of emission estimates based on prediction models.5–11 Similarly, the method untangles the energy balance partitioning by measuring the heat related with H2O, i.e., QE, and the energy diverted with temperature, QH, and the respective observations provide information to evaluate the performance of land surface models used in climatic simulations.12–15
While one EC flux tower cannot assess the total CO2 emissions from a whole city, its measurements are representative of emissions at the same scale as the resolution of typical gridded emission inventories (250 × 250 m to 1 × 1 km), hence they provide unique information to test the performance of bottom-up aggregation processes used for their construction.16–18 These towers also provide insight on the effectiveness of mitigation policies (e.g., vehicular traffic limitations and house heating strategies), as well as on the impact of rapid changes in social mobility on local emissions, like those experienced in 2020 due to the COVID-19 pandemic.19–21 In terms of urban warming, flux towers provide information of the energy components at the same spatial resolution as weather forecast models coupled with urban canopy schemes used to investigate the urban climate at city scale.22–24
Eddy covariance flux towers cannot assess the carbon or heat exchange at fine spatial resolutions such as those of individual buildings or blocks, nor can they identify individual hotspots of CO2 emissions, or specific point-sources of moisture. In terms of CO2 emissions, only a few state-of-the-art emission products based on building energy models and bottom-up methods have addressed fossil fuel emissions at such fine scales.25–27 However, their accuracy relies on data availability and data quality of the economic sectors associated with the emissions, and of course on the representativeness of the respective emission factors. The situation is made worse considering that most cities do not have such information.
With respect to heat fluxes, approaches to estimate the contribution of anthropogenic sources such as from building energy consumption, car exhausts, air conditioning or human metabolism have been developed for use in combination with meteorological models coupled with urban canopy schemes.28–30 More recently, these approaches have also started to include contributions from urban vegetation.31–33 Although these models can estimate heat emissions from each component of the urban ecosystem, they cannot assess the resulting energy exchange of all components together at the scale of buildings to blocks. To the best of our knowledge, only Smith et al. have been able to estimate QE fluxes related to urban vegetation at such a fine scale using a deterministic model, but they still do not consider fluxes from anthropogenic sources.34 Current models average the characteristics of the urban landscape across a single grid, and seldom include contributions of anthropogenic QE. Only a few modeling studies have addressed the impact of moisture ejected from air conditioning and other anthropogenic sources.35–39 However, advances in data-driven modeling already make it possible to combine machine learning with satellite imagery to map QE fluxes in cities, considering both natural and anthropogenic sources at high spatial and temporal resolution. Vulova et al. very recently followed this approach to map hourly fluxes of H2O in 10 × 10 m grid-cells across Berlin using as reference flux data from two EC flux towers.40
Mobile measurements have been used to investigate the spatial variability of GHG in terms of concentrations at high spatial resolution within the urban landscape,41–43 but not in terms of emissions. Similarly, mobile platforms have been instrumented for evaluating the spatial variability of temperature, humidity and other microclimatic variables at street level, but not for measuring fluxes of heat or moisture.44–46 Only Lee et al. have derived CO2 emissions at fine spatial resolution based on field measurements.47 In a pioneering work they applied an aerodynamic resistance method to calculate emissions from mixing ratios of CO2 measured at street level in conjunction with mixing ratios and fluxes collected from a tall EC flux tower located within the study area. They used five mobile units to simultaneously measure CO2 mixing ratios across five neighborhoods and one urban park in Vancouver, Canada, and derived hourly emissions in 100 × 100 m grid-cells.
For this study we instrumented a bicycle to collect CO2 mixing ratios and humidity data along streets and alleys in a residential neighborhood of Singapore where an EC flux tower was already installed, to calculate and map fluxes of CO2 and H2O at high spatial resolution. Similar to Lee et al., we applied the aerodynamic resistance method for QH transfer as a proxy to derive fluxes of CO2 and H2O.47 The latter is directly associated with the energy channeled into QE. Because of the heterogenous urban landscape of Singapore characterized by districts with distinct land covers and building morphologies (i.e., Local Climate Zones, LCZ), and thus varying aerodynamic resistances, we limited the study to the neighborhood within the footprint of the flux tower. A small study area allowed to be ambitious in terms of spatial resolution, and map fluxes in 20 × 20 m grid-cells and reach the scale of ‘buildings to blocks’.
The aim of this study is to evaluate the ability of a relatively simple approach to map urban fluxes of CO2 and H2O at fine spatial resolution in neighborhoods equipped with EC flux towers. We argue that mapping fluxes of CO2 at high granular scale can assist in developing effective climate change mitigation policies by identifying individual hotspots of emissions, including emission sources hidden within the urban fabric, such as kitchens and construction machinery for the particular case of the neighborhood assessed here. Likewise, we propose that the method can be used to detect hotspots of CO2 emissions, such as traffic intersection. Educating local residents about the main emission sources within their own neighborhood could encourage greater involvement on their part in mitigation efforts.
In terms of QE (i.e., H2O fluxes), these maps can show the influence of green areas and trees on the local microclimate. Evapotranspiration is an important mechanism of atmospheric cooling in urban environments at the local scale,48 although in specific conditions urban vegetation may cause higher temperatures.49 These maps also show moisture emissions from anthropogenic sources, such as air conditioning systems and vehicle exhaust.50
2. Methods
We applied a bulk aerodynamic resistance method based on Monin–Obukhov similarity theory and Reynolds analogy to evaluate the transfer of QH as a proxy of the surface–atmosphere exchange of CO2 and H2O. The fluxes of CO2 (FCO2, μg m−2 s−1) and H2O (FH2O, mg m−2 s−1) were calculated as the difference of the mixing ratios of CO2 (CCO2, mg m−3) and absolute humidity (CH2O, g m−3) at a height above the roughness sublayer (CCO2_tower and CH2O_tower) and screen level at 1.7 m above ground (CCO2_mobile and CH2O_mobile) divided by the aerodynamic resistance to CO2 and H2O of the urban fabric (rCO2, rH2O, s m−1), respectively: | 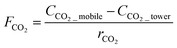 | (1) |
| 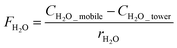 | (2) |
The mixing ratios of CO2 and H2O were obtained from the flux tower and bicycle measurements, while the bulk aerodynamic resistance to QH (rH), or thermal resistance in short, was considered equivalent to rCO2 and rH2O. According to Reynolds analogy, the resistance to transfer heat, moisture, trace gases, or any other passive scalar from homogeneous surfaces to the inertial sublayer can be considered equal.51,52 Urban surfaces are not homogeneous, but we assume that they are in order to apply Reynolds analogy. The thermal resistance relies on the energy balance partitioning and the surface aerodynamic temperature (T0, K), which in turn responds to the composition and geometry of the urban components (e.g., buildings, roads, etc.), and heterogeneous thermal properties of their materials.53 Parameterizations of different complexity have been proposed to derive rH.54 In this study we used the empirical relationship based on QH and ambient temperature measured by the flux tower (Ttower, K) as described by Kanda et al.:55
| 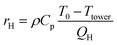 | (3) |
where
Cp is the heat capacity of the air (J kg
−1 K
−1), and
ρ is the air density (kg m
−3). The surface aerodynamic temperature was calculated as:
| 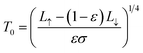 | (4) |
where
L↑ and
L↓ are the outgoing and incoming longwave radiation (W m
−2) measured by a net radiometer placed at the top of the flux tower,
σ is the Stefan–Boltzmann constant (5.6 × 10
−8 W m
−2 K
−4), and
ε is the surface emissivity, assuming a constant value of 0.931 obtained from mean emissivity values according to the type and fraction of surfaces present in the studied neighborhood (
εroofs = 0.914,
εroads = 0.965,
εimpervios surfaces = 0.914,
εtrees = 0.990,
εgrass = 0.978).
56
The method is only valid under the assumption that the mixing ratios of CO2 and H2O measured at the top of the flux tower are representative of the atmospheric layer above the neighborhood, and no CO2 or moisture accumulates within the urban fabric (i.e., no storage flux). Therefore, the application of the method is limited to daytime under vigorous conditions of vertical mixing. To ensure the flux at the height of the tower is directly related to the flux at the surface, averaging periods must represent conditions of atmospheric instability (u* > 0.1 m s−1) and meet the eddy covariance's criteria of stationarity.57 Similarly, to reduce the uncertainties of the method, the difference between T0 and Ttower must be larger than 0.1 K, and QH must be positive, i.e., away from the surface.54 Periods with rainfall are excluded.
Details of the residential neighborhood assessed in this study, and of the EC flux and mobile measurements are provided below. Fig. 1 shows both measurement platforms and main instrumentation. The procedure for interpolating the observed fluxes along streets and alleys to the entire neighborhood area is also described.
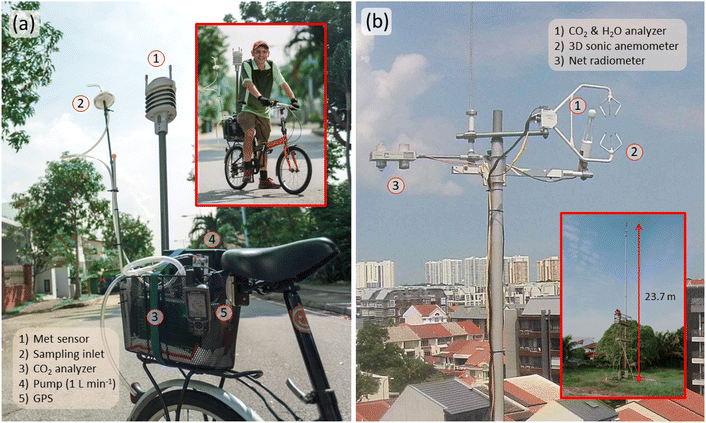 |
| Fig. 1 (a) Instrumented bicycle and (b) eddy covariance flux tower used to map the distribution of turbulent fluxes of CO2 and H2O at fine spatial resolution across the residential neighborhood of Telok Kurau, Singapore, using an aerodynamic resistance method for the transfer of QH. | |
2.1. Site description
The study was conducted in the low-rise residential neighborhood of Telok Kurau, Singapore, where an EC flux tower measured fluxes of energy and CO2 almost continuously between 2007 and 2017.5,58 This neighborhood is located on the east side of island city-state (1°18′51.46′′ N, 103°54′40.31′′ E; 5 m above sea level), and consists of 2 to 3 story residential buildings dissected by a dense network of street. The neighborhood is densely populated (7500 inhabitants per km2) and is home to 3550 woody trees and 1990 palm trees per square kilometer. Thirty nine percent of the area is covered by buildings; roads and other impervious surfaces account for 12% and 34%, respectively, while vegetation covers the remaining 15% (11% tree crowns and 4% grass). The area corresponds to ‘compact low-rise’ according to the LCZ classification used for urban microclimate assessments.59
Singapore's climate is characterized by high temperatures, relative humidity and rainfall, and low average wind speed all year round. Ambient temperature ranges from 25 °C in the early morning to 35 °C in the afternoon. Relative humidity is 85–90% in the early morning and remains above 60% during the rest of the day, and rarely falls below 50% (https://www.weather.gov.sg/). The mobile measurements at street level were carried out in October–November 2016 during the fall inter-monsoon season, when winds of variable direction are experienced.
2.2. Eddy covariance flux tower measurements
The instruments and operational details of the EC flux tower are fully described in Velasco et al. and Roth et al.5,58 Briefly, the tower was equipped with an open-path gas analyzer (Li-7500; LICOR, Lincoln, Nebraska, USA) and a 3D sonic anemometer (CSAT3; Campbell Scientific Inc., Logan, Utah USA) for measuring fluctuations of CO2, water vapor, vertical wind speed, and temperature at 10 Hz to calculate fluxes of CO2, H2O, QH, and QE over 30 min averaging periods using the EC method. The usual quality control and correction procedures were applied.2 Additional sensors included a net radiometer (CNR1; Kipp and Zonen, Delft, The Netherlands) for measuring the individual components of the incoming and outgoing shortwave and longwave radiation, and a meteorological probe (HMP45; Vaisala, Helsinki, Finland) for measuring air temperature and relative humidity (RH). All sensors were mounted at a height of 23.7 m within the inertial sublayer, well above the height of roughness obstacles (i.e., buildings and trees). An analysis of the flux source area demonstrated that the measured fluxes were representative of the entire neighborhood; the footprint extent ranged from 300 to 1000 m during day and night, respectively (see Fig. 2c). There is little directional variation in the morphological properties and land cover, demonstrating the homogeneity of the studied neighborhood.
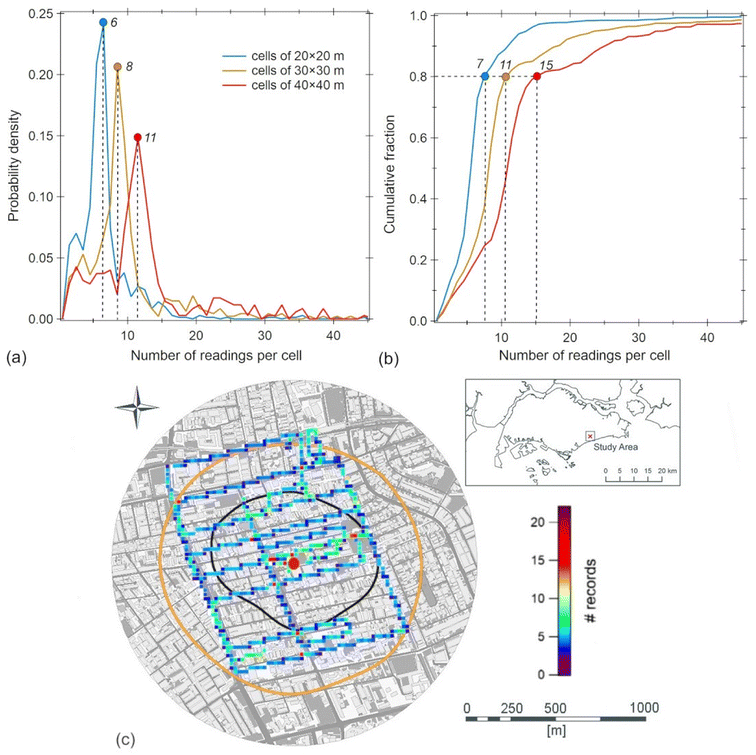 |
| Fig. 2 (a) Probability density and (b) cumulative fraction of the number of readings collected in each grid cell during the evening rush hour sampling period on 31 October 2016, for grid sizes of 20 × 20 m, 30 × 30 m, and 40 × 40 m. The numbers next to the colored circles are the respective median values in (a), and the least number of readings collected in 80% of the cells in (b). (c) Bicycle route during the sampling period plotted on a map of the study neighborhood. The color gradient indicates the number of readings collected in each 20 × 20 m grid cell. The red dot in the middle of the map indicates the location of the eddy covariance flux tower. The black and orange contours depict the typical day- and nighttime ensemble footprint areas based on long-term measurements of the EC sensors on top of the flux tower, respectively. The inset map shows the location of the Telok Kurau study area within the island city-state of Singapore. | |
2.3. Mobile measurements
A bicycle was instrumented with an infrared gas analyzer (Li-840; LICOR, Lincoln, Nebraska, USA), a meteorological probe (WXT520, Vaisala, Helsinki, Finland) and a global positioning system (GPS-60CSx; Garmin International, Olathe, Kansas, USA) to collect georeferenced mixing ratios of CO2, and temperature and humidity at 1 Hz along a defined route across the study neighborhood. The sampling inlet was placed at a height of 1.7 m above the ground, and fitted with an Acro 50 vent filter (pore size 0.2 μm) to protect the optical path of the Li-840 analyzer from particle contamination. The sampling flow was set at 1 L m−1, and a lag time of 6 s in the readings was determined after accounting for the length of the tube and instrument response. The CO2 monitors mounted on the tower and bicycle were calibrated at the start and end of the study period with two standard gas mixtures (Scott-Martin Inc. 337 and 531 ppmv, National Institute of Standards and Technology). The relative humidity and temperature data collected by the WXT520 meteorological sensor, and the saturation pressure equation for water vapor were used to calculate absolute humidity: | 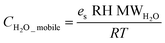 | (5) |
| 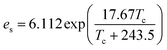 | (6) |
where es is the saturated vapor pressure of water (N m−2) calculated using Bolton's empirical formula (eqn (6)),60 which returns es in mbar; RH is relative humidity, and T and Tc temperature in K and °C, respectively, R is the universal gas constant (8314.3 J kmol−1 K−1), and MWH2O is the molecular weight of water (18 kg kmol−1).
The sampling route was designed to cover the entire area of the neighborhood (1290 × 1290 m) in 60–80 min. The relatively long sampling period is because the variations in T and RH in Singapore, as a tropical city, are small. At locations where variations are larger, sampling periods should be limited to the length of averaging periods used to calculate turbulent fluxes by eddy covariance (usually 30 min).
A cycling speed of 12 ± 6 m s−1 allowed to cover 13–18 km during every run, which was enough to sample almost every street and alley within the studied area at least once. Measurements were conducted on weekdays during the morning and evening rush hours and during midday, starting at 7
:
00, 11
:
30, and 18
:
30 h, capturing periods when CO2 emissions peak as a result of heavy vehicular traffic, or photosynthesis of the abundant vegetation offsets the anthropogenic emissions.5 Six repetitions were carried out for each period, generating a total of 17 datasets since one evening trial was cancelled due to rain.
The georeferenced readings collected during each transverse were analyzed using grid resolutions of 20 × 20 m, 30 × 30 m and 40 × 40 m. The finest resolution permitted the calculation of a median value consisting of six readings per grid cell crossed by the route, with 8 and 11 readings for the second and third grid size, respectively. Fig. 2 shows the probability density and cumulative fraction of the number of readings collected in each cell along a particular round with the number of readings collected per cell considering a 20 × 20 m grid scale.
2.4. Flux mapping
Eqn (3) and (4) were used to calculate rH, assumed to be equivalent to rCO2 and rH2O, using the mean values of L↑, L↓, QH, and Ttower recorded during the two or three 30 min averaging periods corresponding to each round of the mobile measurements. The average mixing ratios of CO2 and absolute humidity were then calculated for each grid cell along the sampled route, considering three different grid sizes. Finally, using the average values of the same variables measured at the top of the EC flux tower, FCO2 and FH2O were obtained for each grid cell using eqn (1) and (2).
The spatial distribution of FCO2 and FH2O across the study area was obtained by applying an interpolation subroutine based on the original Delaunay triangulation (Igor v.7, WaveMetrics, Inc.) using the fluxes calculated for each grid cell along the sampled route. Delaunay triangulation is often used to interpolate geometrically irregularly distributed data, like on weather or topographic maps, which is the expected spatial distribution of urban fluxes of CO2 and moisture at fine spatial resolution as evaluated here.61,62Fig. 3 shows examples of FCO2 and FH2O maps obtained from the sampling route used in Fig. 2.
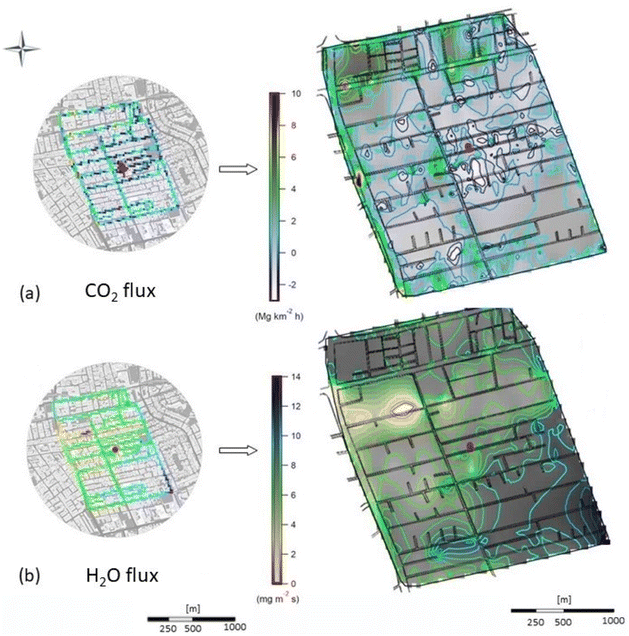 |
| Fig. 3 Spatial distribution of (a) CO2 and (b) H2O fluxes during the evening rush hour on 31 October 2016. The maps on the left show the fluxes measured along the sampled route using a 20 × 20 m grid cell resolution, while the maps on the right show the interpolated fluxes across the entire neighborhood. The white oval on the H2O flux map indicates no flux as a consequence of an issue with the interpolation procedure for this particular trial. The gradient in shades of gray shows the magnitude of the interpolated flux, while the isolines highlight changes in the flux distribution as indicated by the vertical scale on each map. The street network is overlayed on the maps, and the red dot in the middle of the maps indicates the location of the eddy covariance flux tower. | |
3. Results
The sum of the interpolated fluxes of CO2 across the entire neighborhood obtained by the aerodynamic resistance approach agreed within one standard deviation of the long-term flux measured by eddy covariance in thirteen occasions (Fig. 4a). Three rounds of measurements significantly exceeded this threshold and one only slightly. The correlation between the fluxes resulting from both methods for each individual round of measurements showed a moderate coefficient of determination (r2) of 0.66. With respect to H2O, operational problems with the meteorological probe mounted on the bicycle limited the study to eleven rounds, of which only one exceeded the one standard deviation threshold (Fig. 4b). The correlation between both methods for H2O fluxes was higher (r2 = 0.88). In the case of CO2 fluxes, 75% of the values determined using the aerodynamic resistance approach yielded representative results, and 90% in the case of H2O fluxes.
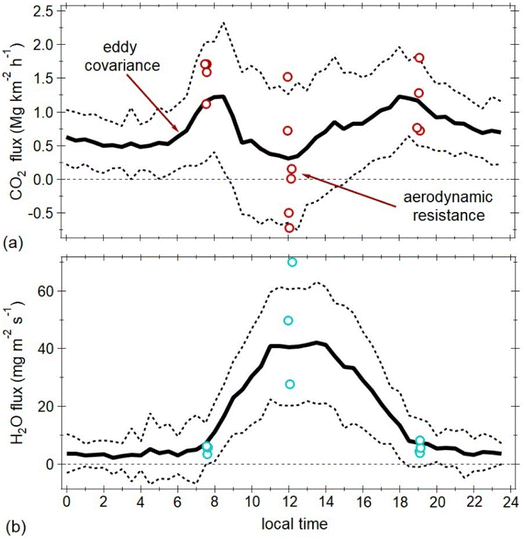 |
| Fig. 4 Neighborhood-wide mean fluxes using the aerodynamic resistance approach (symbols) and diurnal variability of fluxes measured by eddy covariance (solid line) for (a) CO2 and (b) H2O on weekdays during the two-month period (October–November 2016) when bicycle measurements were made. The dashed lines represent ±1 standard deviation from the average flux and give an indication of the day-to-day variability. | |
Table S1† in the ESI shows the statistical metrics of the fluxes obtained by the aerodynamic resistance approach and the mean fluxes measured by EC. The parameters necessary to apply the former method, such as QH, T0 and rH are included in the same table, together with reasons why the method failed in particular cases (not shown in Fig. 4). For CO2, two rounds of measurements whose resulting fluxes turned out to be suspicious were affected by light rain, in another one the EC stationarity criterion was not met, and in one more case the difference between T0 and Ttower was < 0.1 K. For H2O, the sampling period in which the flux obtained by the aerodynamic resistance approach exceeded the range predicted by the flux tower did not meet the EC stationarity criterion.
Fluxes obtained using the aerodynamic resistance approach in general captured the diurnal pattern observed by the EC flux tower (Fig. 4). CO2 fluxes tended to be higher during the morning and evening sampling rounds, and lower during the rounds at midday. In contrast, H2O fluxes were slightly lower during the morning and evening rounds, but higher during midday.
Vehicular traffic is the main contributor of CO2 to the atmosphere in this neighborhood, with increased emissions during the morning and evening rush hours.5 During daytime the extensive greenery partially offsets the anthropogenic contributions, sometimes even generating a negative net flux (i.e., the urban surface acts as a carbon sink). Three midday sampling periods yielded negative fluxes, contrary to the flux measured by EC (see Table S1†). This discrepancy can be explained by differences in the respective footprint area observed by the two methods during such periods. The EC method measures only flux contributions aligned with the mean airflow, while the aerodynamic resistance approach includes contributions from all sectors covered by the sampling route, irrespective if they originate from upwind or downwind locations.
Evapotranspiration is the main source of H2O to the atmosphere in tropical urban ecosystems with extensive vegetation such as the one assessed here. Photosynthetic activity and stomatal behavior control the H2O exchange between plants and the air. Plants release moisture from their leaves when they open their stomas to assimilate CO2. This process responds in part to light intensity and air temperature, which explains why the exchange of H2O occurs mainly during daytime. However, when the vapor pressure deficit is high (i.e., water stress conditions), leaves experience patchy stomatal closure as a mechanism to prevent excess water loss, so the evapotranspiration rate tends to plateau shortly before noon, when ambient conditions become harsher, as observed in our case (see Fig. 4b). The evaporation rate from impervious surfaces is highly variable since it depends on the amount of rain during previous hours, which evaporates fast thanks to the hot weather in Singapore. There is no anthropogenic water input from irrigation since almost daily rainfalls keep the soil moist. In addition, moisture emissions from air conditioning, vehicle exhaust, and human metabolism are only present to a small extent.32,49
3.1. Sources of uncertainty
The aerodynamic resistance approach is sensitive to the variables that are used to derive the thermal resistance term, rH, in addition, that it must be assumed that the urban surface behaves as a homogeneous surface in order to apply the Reynolds analogy.51,52 We assumed that L↑ and L↓,T0, Ttower, and QH were constant during the 60–80 min period of each sampling round. Some traverses were affected by changes in cloud cover. In a tropical island like Singapore, the sky can overcast in a few minutes, and modify the components of the radiative budget and energy balance, and thus the degree to which atmospheric turbulence facilitates the transfer of entities like momentum, heat, moisture, and trace gases between the urban surface and overlying atmosphere.63
According to the prevailing weather conditions and atmospheric stability, rH tends to decrease as the day progresses. Under warmer and drier conditions, atmospheric instability and QH increases, while rH decreases as described by eqn (1). L↑ was about 40 W m−2 higher than L↓ during the morning and evening measurement periods, and slightly above 50 W m−2 higher during midday. These differences resulted in ΔT (T0 − Ttower) being 1.20, 2.00, and 6.50 °C lower in the morning, evening, and midday sampling periods, respectively. Sensible heat was as expected highest during midday (>65 W m−2, except on a very cloudy day), and lowest but always positive during the morning and evening periods (4–10 W m−2) (see Table S1†). Lower QH and smaller ΔT during the morning and evening sampling periods worked in favor of rH. Taking as a reference the geometric mean (25th–75th percentile) of rH obtained from all rounds for each sampling period, the lowest values were observed during midday, 36.9 (24.2–51.0) m s−1, followed by those during the morning rush hours, 107.6 (72.9–195.5) m s−1, and being highest during the afternoon rush hours, 207.9 (97.3–380.0) m s−1. Midday rH values agree with those reported in the literature for other urban areas, but values observed in the morning and evening rush hours were higher.47,53 Values obtained during these latter periods may be somewhat biased due to uncertainties in QH caused by a less vigorous vertical mixing and because the assumptions necessary to apply the Monin–Obukhov similarity theory begin to break down.13
The empirical relation used to calculate T0 depends on the representativeness of ε across the entire urban surface and on the radiometer view bias. The source area (>300 m) observed by the EC system usually exceeds that observed by the narrow field-of-view of the downfacing radiation sensors (200 m), hence it is important that the surface characteristics near the tower are similar to those throughout the neighborhood. Also, the lack of atmospheric correction in eqn (2) to capture the diurnal variability of the roughness length for heat (zH, height at which T0 = Ttower) and variations in atmospheric conditions (friction velocity, u*, and Obukhov length, L) are another sources of uncertainty.53
In addition to the sources of uncertainty faced to calculate T0, the relation to derive rH (eqn (3)) is affected by uncertainties in QH caused by the lack of energy balance closure typically experienced by EC flux systems,64,65 and the influence of anthropogenic heat (QF). A fraction of QF (∼2/3) is expressed as QH when this it is measured by EC.66 The anthropogenic heat in this particular neighborhood does not exceeds 13 W m−2 during daytime,67 thus a bias of <9 W m−2 is expected in QH. This can represent a large fraction during the morning and evening measurements (i.e., >50%), but will only be a minor fraction during midday (<5%).58
The aerodynamic resistance approach is affected by various sources of uncertainty. While an error propagation cannot be quantified, a sensitivity analysis of random effects can help to quantify the net uncertainty. When errors are only random (i.e., neglecting systematic errors), errors in the computed means decrease with increasing number of samples according to N−1/2, where N is the number of samples. Thus, random errors can be estimated by examining the convergence of the individual rounds of measurements on the net flux. Following the approach proposed by Montcrieff et al. to evaluate random errors in EC flux systems,68 we applied a percentage random error (pr = 20%) to each mean flux obtained in each sampling round, using the average flux measured by EC during the two months of the study period (October–November 2016) as the reference. Errors were 27%, 47% and 22% for CO2 fluxes measured during the morning, midday, and evening periods, respectively. The respective net errors for H2O fluxes were 14%, 25% and 10%.
Based on this error evaluation, we can determine how many sampling rounds are necessary to reduce the uncertainties to a certain threshold. Considering a random error of 20% and again using the mean fluxes measured by EC during the study period as reference, we found that 25, 70, and 20 repetitions would be needed to reduce the net error to 5% during the morning, midday, and evening periods for the calculation of CO2 fluxes, and 5, 20, and 10 repetitions for H2O fluxes. Considering unavoidable measurement periods with inadequate atmospheric conditions as well as human errors setting up instruments, one month of measurements for each sampling period is recommended to obtain fluxes with <5% uncertainty, except for midday CO2 fluxes, which would produce an uncertainty of 9%.
3.2. CO2 and H2O flux maps
Although the aerodynamic resistance approach yielded realistic and consistent results, the net fluxes obtained during each sampling round at the neighborhood scale were somewhat variable when compared to each other (Fig. 4). We have already seen that to obtain mean fluxes similar to those measured by EC, 30 repetitions of measurement would be needed for each sampling period. It is a feasible task, but quite demanding. Fortunately, and as expected, no significant variation was observed in the spatial distribution among the interpolated fluxes for each sampling round within the same period. This allows merging the estimated fluxes for each cell in each of the measurement rounds that were carried out for each sampling period. This was done by normalizing the estimated fluxes for each grid-cell with respect to the median over the entire area evaluated during each repetition. Fig. 5 shows the resulting ensemble maps using a 20 × 20 m grid-cell resolution with relative fluxes of CO2 and H2O across the entire neighborhood during each of the three sampling periods.
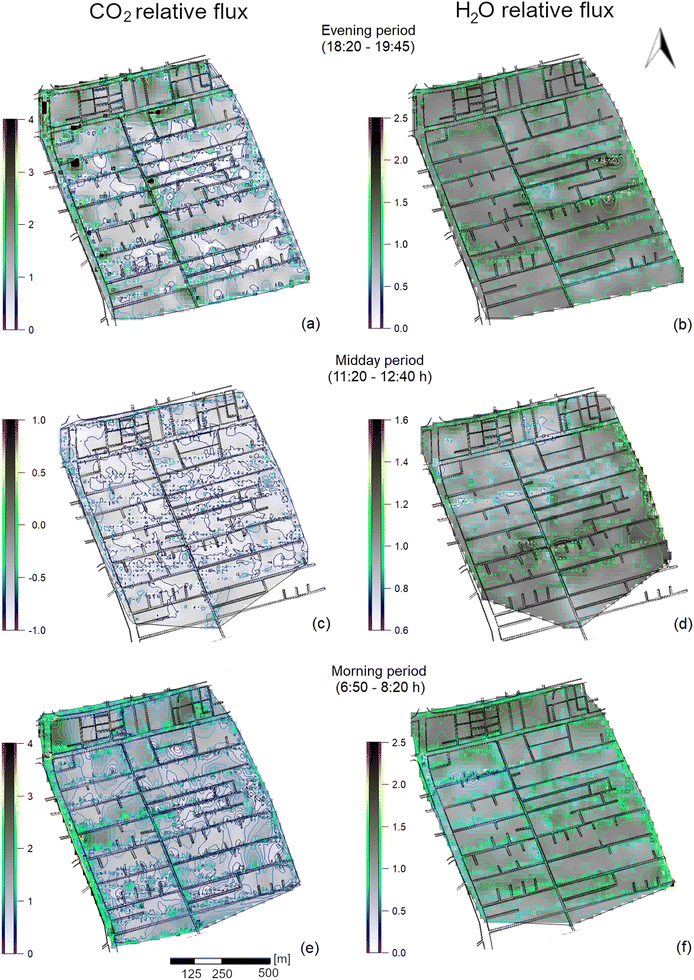 |
| Fig. 5 Ensemble spatial distribution of normalized fluxes of CO2 (a, c, e) and H2O (b, d, f) interpolated at 20 × 20 m grid cell resolution across the complete neighborhood during the three sampling periods evaluated in this study. The normalized ensembles were obtained by interpolating the relative fluxes computed for each trial with respect to their corresponding neighborhood median value. For example, the normalized fluxes of CO2 during the evening period (panel a) ranged from close to zero to four times the median flux obtained from the four sampling rounds conducted for this period and averaged across the complete neighborhood. The gradient in shades of gray shows the magnitude of the interpolated flux, while the isolines highlight changes in the flux distribution as indicated by the vertical scale on each map. The street network is overlayed on the maps. | |
Despite the relative homogeneity in terms of urban morphology and emission sources throughout the neighborhood, distinctive spatial patterns in CO2 and H2O fluxes are observed for each sampling period. The small variation as a function of wind direction in the magnitude of fluxes measured by EC also demonstrates such homogeneity.5
For CO2, traffic intersections and main roads acted as emission hotspots, evident in both morning and evening rush hour periods (Fig. 5 a and e). A block with multiple eateries and coffee shops located in the northeast corner of the studied neighborhood was also identified as a major emission source during these two periods. A more homogenous distribution of CO2 fluxes was observed during midday when anthropogenic emissions are a bit less intense and photosynthetic activity peaks (Fig. 5c). Individual grid-cells showed fluxes of up to 4 times higher than the median flux during the morning an evening rush hour periods, no negative fluxes were observed. During midday the range of fluxes was smaller, but many cells showed negative fluxes. Relative fluxes ranged from −1.0 to zero times the observed median flux.
With respect to H2O, the spatial variability was lower, however blocks with narrow and densely tree-lined streets showed consistently larger fluxes, which is most obvious during the midday sampling periods (Fig. 5d). However, the largest range of fluxes was observed during the morning and evening rush hour periods, due to a few-grid cells with contrasting fluxes. Fluxes varied from zero to 2.5 times the median flux during these two periods, and from 0.6 to 1.6 times at midday. The contributions from a drainage channel located at the east side of the neighborhood was clearly observed during all three sampling periods. Major roads saw fluxes up to 1.5 times the median flux as a consequence of vehicular traffic and road-side greenery. No point sources associated with moisture ejection from air conditioners were observed. During the day, household air conditioning usage is low as residents commute to work or school.
4. In closing
The aerodynamic resistance approach can be used to map fluxes of trace gases and latent heat at fine spatial resolution at the scale of buildings to blocks, as well as to identify point emission sources and sinks. In the present case, variability from one day to another in the fluxes of CO2 and H2O are largely caused by anthropogenic and meteorological factors. Fluxes obtained from individual measurement transects should therefore be viewed as snapshots. One snapshot alone cannot be used to determine the net flux across the neighborhood, but it does provide useful insight into the spatial distribution of fluxes. Thirty snapshoots are necessary to obtain a representative mean value of the net flux at the neighborhood scale for each sampling period. The method works best under conditions of atmospheric instability, so its application should be limited to daytime.
The method can be theoretically extended to measure fluxes of any other non-reactive trace gas, and can be used to assess the accuracy of gridded emission inventories built for urban air quality modeling.69 Challenging Reynolds analogy, the thermal resistance term calculated for QH, rH, can be used to investigate the emissions of any pollutant or GHG species that is emitted directly into the atmosphere (i.e., primary pollutant) within the urban fabric. For example, for the case of methane the aerodynamic resistance approach would help to identify and quantify emissions related to leaks of natural gas in distribution systems.70,71 A pair of accurate analytical sensors with a temporal resolution of 1 second or less would be required. Preferably, the sensors should be battery-powered and of a practical size to be mounted on a bicycle and on top of a flux tower. Larger instruments could also be used, but different arrangements would be needed on both platforms, the flux tower and the mobile unit that would replace the bicycle. Similarly, a second or third mobile unit would reduce the time needed to cover the area to be surveyed and the sampling time would approach the 30 min averaging periods typically used for eddy covariance flux observations. Alternatively, it would allow a larger route to be covered and more data to be collected within the study area and, in turn, improve the interpolation procedure. A third alternative would be to increase the size of the footprint to be evaluated, taking into account the requirements of relative homogeneity in terms of urban morphology and distribution of emission sources and sinks. However, adding more mobile units would increase the cost of the measurements.
In principle, a network of flux towers installed in representative sites along with a fleet of mobile units could be used to map emissions across a city at a much finer scale than is possible with current atmospheric observational methods (e.g., ambient observations and mass balance, dense networks of sensors, data assimilation systems, atmospheric measurements and inverse modelling, tracer release experiments, remote sensing). For the case of the city-state of Singapore, ten flux towers would be needed to cover the about ten different Local Climate Zones (i.e., different rH values) that make up the majority of the urban sprawl,72 assuming that a flux tower can be installed in each LCZ, and the variability in ambient concentrations of the trace gas of interest among them is not significant. About 150 coordinated mobile units would be also required, assuming that each could cover two of the 330 neighborhoods of the city during 1 hour sampling periods. This effort does not sound realistic. However, composite maps could be created from mobile measurements during different days under similar conditions and at the same time, thereby reducing the number of mobile units. Data-driven models in combination with databases of urban parameters (e.g., land use, urban morphology, population density, vehicular traffic, etc.) could also be used to determine clusters of neighborhoods with similar characteristics and identify the most representative ones to reduce the total area to survey.73
Finally, the application of the aerodynamic resistance approach to improve the quantification and identification of CO2 emissions and spots of moisture that enhance QE in urban areas at scales that are directly related to human activities that generate the emissions or modify the energy balance, is feasible. The approach must be seen as a useful tool that provides information to design effective and smart mitigation measures at the scale of households, buildings, roads, and traffic intersections that are meaningful to practical policy aiming to build resilient green cities. It can be used to verify emission estimates based on bottom-up methodologies, as well as to increase the spatial resolution of emission observations obtained from top-down measurements. By visualizing the location where GHG emissions occur and learning what factors alter humidity at scales from buildings to blocks, the public might be more willing to engage in mitigation efforts.
Conflicts of interest
There are no conflicts to declare.
Acknowledgements
The field measurements were supported by Singapore's National Research Foundation through the Singapore MIT Alliance for Research and Technology (SMART). The operation of the eddy covariance flux tower was jointly supported by SMART and the National University of Singapore (grant R-109-000-091-112). Data analysis and manuscript preparation were self-financed by the authors.
References
-
World Meteorological Organization (WMO), IG3IS Urban greenhouse gas emission observation and monitoring best practices. WMO GAW IG3IS Report, 2022 Search PubMed.
- E. Velasco and M. Roth, Cities as net sources of CO2: review of atmospheric CO2 exchange in urban environments measured by eddy covariance technique, Geogr. Compass, 2010, 4(9), 1238–1259 CrossRef.
-
C. Feigenwinter, R. Vogt and A. Christen, Eddy covariance measurements over urban areas, in Eddy covariance: a practical guide to measurement and data analysis, ed. M. Aubinet, T. Vesala and D. Papale, Springer, ISBN 978-94-007-2350-4, 2012, pp. 377–397 Search PubMed.
- A. Christen, Atmospheric measurement techniques to quantify greenhouse gas emissions from cities, Urban Clim., 2014, 10, 241–260 CrossRef.
- E. Velasco, M. Roth, S. H. Tan, M. Quak, S. Nabarro and L. Norford, The role of vegetation in the CO2 flux from a tropical urban neighbourhood, Atmos. Chem. Phys., 2013, 13, 10185–10202 CrossRef.
- H. C. Ward, J. G. Evans and C. S. B. Grimmond, Multi-season eddy covariance observations of energy, water, and carbon fluxes over a suburban area in Swindon, UK, Atmos. Chem. Phys., 2013, 13, 4645–4666 CrossRef CAS.
- A. Björkegren and C. S. B. Grimmond, Net carbon dioxide emissions from central London, Urban Clim., 2018, 23, 31–158 CrossRef.
- M. Goret, V. Masson, R. Schoetter and M. P. Moine, Inclusion of CO2 flux modelling in an urban canopy layer model and an evaluation over an old European city centre, Atmos. Environ.: X, 2019, 3, 100042 CAS.
- S. Stagakis, N. Chrysoulakis, N. Spyridakis, C. Feigenwinter and R. Vogt, Eddy Covariance measurements and source partitioning of CO2 emissions in an urban environment: application for Heraklion, Greece, Atmos. Environ., 2019, 201, 278–292 CrossRef CAS.
- P. Li and Z. H. Wang, Modeling carbon dioxide exchange in a single-layer urban canopy model, Built Environ., 2020, 184, 107243 CrossRef.
- K. Wu, K. J. Davis, N. L. Miles, S. J. Richardson, T. Lauvaux, D. P. Sarmiento, N. V. Balashov, K. Keller, J. Turnbull, K. R. Gurney, J. Liang and G. Roest, Source decomposition of eddy-covariance CO2 flux measurements for evaluating a high-resolution urban CO2 emissions inventory, Environ. Res. Lett., 2022, 17, 074035 CrossRef.
- C. S. B. Grimmond, M. Blackett, M. J. Best, J. J. Baik, S. E. Belcher, J. Beringer, S. I. Bohnenstengel, I. Calmet, F. Chen, A. Coutts, A. Dandou, K. Fortuniak, M. L. Gouvea, R. Hamdi, M. Hendry, M. Kanda, T. Kawai, Y. Kawamato, H. Kondo, E. S. Krayenhoff, S. H. Lee, T. Loridan, A. Martilli, V. Masson, S. Miao, K. Leson, R. Ooka, G. Pigeon, A. Porson, Y. H. Ryu, F. Salamanca, F. Steenevels, M. Tombrou, J. A. Voogt, D. T. Young and N. Zhang, Initial results from Phase 2 of the international urban energy balance model comparison, Int. J. Biometeorol., 2011, 31(2), 244–272 Search PubMed.
- M. J. Best and C. S. B. Grimmond, Key conclusions of the first international urban land surface model comparison project, Bull. Am. Meteorol. Soc., 2015, 96(5), 805–819 CrossRef.
- P. Karsisto, C. Fortelius, M. Demuzere, C. S. B. Grimmond, K. W. Oleson, R. Kouznetsov, V. Masson and L. Järvi, Seasonal surface urban energy balance and wintertime stability simulated using three land-surface models in the high-latitude city Helsinki, Q. J. R. Meteorol. Soc., 2015, 142(694), 401–417 CrossRef.
- M. Demuzere, S. Harshan, L. Järvi, M. Roth, C. S. B. Grimmond, V. Masson, K. W. Oleson, E. Velasco and H. Wouters, Impact of urban canopy models and external parameters on the modelled urban energy balance in a tropical city, Q. J. R. Meteorol. Soc., 2017, 143(704), 1581–1596 CrossRef.
- E. Velasco, S. Pressley, R. Grivicke, E. Allwine, T. Coons, W. Foster, T. Jobson, H. Westberg, R. Ramos, F. Hernández, L. T. Molina and B. Lamb, Eddy covariance flux measurements of pollutant gases in urban Mexico City, Atmos. Chem. Phys., 2009, 9, 7325–7342 CrossRef CAS.
- E. Velasco, R. Perrusquia, E. Jiménez, F. Hernández, P. Camacho, S. Rodríguez, A. Retama and L. T. Molina, Sources and sinks of carbon dioxide in a neighborhood of Mexico City, Atmos. Environ., 2014, 97, 226–238 CrossRef CAS.
- L. Järvi, M. Havu, H. C. Ward, V. Bellucco, J. P. McFadden, T. Toivonen, V. Heikinheimo, P. Kolari, A. Riikonen and C. S. B. Grimmond, Spatial modeling of local-scale biogenic and anthropogenic carbon dioxide emissions in Helsinki, J. Geophys. Res.: Atmos., 2019, 124(15), 8363–8384 CrossRef.
- C. Lamprecht, M. Graus, M. Striednig, M. Stichaner and T. Karl, Decoupling of urban CO2 and air pollutant emission reductions during the European SARS-CoV-2 lockdown, Atmos. Chem. Phys., 2021, 21(4), 3091–3102 CrossRef CAS.
- H. Sugawara, S. Ishidoya, Y. Terao, Y. Takane, Y. Kikegawa and K. Nakajima, Anthropogenic CO2 emissions changes in an urban area of Tokyo, Japan, due to the COVID-19 pandemic: a case study during the state of emergency in April–May 2020, Geophys. Res. Lett., 2021, 48, e2021GL092600 CrossRef CAS.
- G. Nicolini, G. Antoniella, F. Carotenuto, A. Christen, P. Ciais, C. Feigenwinter, B. Gioli, S. Stagakis, E. Velasco, R. Vogt, H. C. Ward, J. Barlow, N. Chrysoulakis, T. Karl, M. Graus, C. Helfter, B. Heusinkveld, L. Järvi, S. Marras, V. Masson, B. Matthews, F. Meier, E. Nemitz, S. Sabbatini, D. Scherer, H. Schume, C. Sirca, D. Spano, G. J. Steeneveld, C. Vagnoli, Y. Wang, A. Zaldei, B. Zheng and D. Papale, Direct observations of CO2 emission reductions due to COVID-19 lockdown across European urban districts, Sci. Total Environ., 2022, 830, 154662 CrossRef CAS PubMed.
- D. Meyer, R. Schoetter, M. Riechert, A. Verrelle, M. Tewari, J. Dudhia, V. Masson, M. Van Reeuwijk and C. S. B. Grimmond, WRF-TEB: Implementation and evaluation of the coupled Weather Research and Forecasting (WRF) and Town Energy Balance (TEB) model, J. Adv. Model. Earth Syst., 2020, 12(8), 2019MS001961 Search PubMed.
- A. Simón-Moral, A. Dipankar, M. Roth, C. Sánchez, E. Velasco and X. Y. Huang, Application of MORUSES single-layer urban canopy model in a tropical city: results from Singapore, Q. J. R. Meteorol. Soc., 2020, 146(727), 576–597 CrossRef.
- B. Sánchez, M. Roth, A. Simón-Moral, A. Martilli and E. Velasco, Assessment of a meteorological mesoscale model's capability to simulate intra-urban thermal variability in a tropical city, Urban Clim., 2021, 40, 101006 CrossRef.
- K. R. Gurney, I. Razlivanov, Y. Song, Y. Zhou, B. Benes and M. Abdul-Massih, Quantification of fossil fuel CO2 emissions on the building/street scale for a large US city, Environ. Sci. Technol., 2012, 46(21), 12194–12202 CrossRef CAS PubMed.
- S. Dai, S. Zuo and Y. Ren, A spatial database of CO2 emissions, urban form fragmentation and city-scale effect related impact factors for the low carbon urban system in Jinjiang city, China, Data Brief, 2020, 29, 105274 CrossRef PubMed.
- H. Liu, F. Yan and H. A. Tian, A vector map of carbon emission based on point-line-area carbon emission classified allocation method, Sustainability, 2020, 12, 10058 CrossRef CAS.
- D. J. Sailor and L. Lu, A top-down methodology for developing diurnal and seasonal anthropogenic heating profiles for urban areas, Atmos. Environ., 2004, 38(17), 2737–2748 CrossRef CAS.
- F. Salamanca, A. Krpo, A. Martilli and A. Clappier, A new building energy model coupled with an urban canopy parameterization for urban climate simulations—part I. formulation, verification, and sensitivity analysis of the model, Theor. Appl. Climatol., 2010, 99, 331–344 CrossRef.
- W. Y. L. Chow, F. Salamanca, M. Georgescu, A. Mahalov, J. M. Milne and B. L. Ruddell, A multi-method and multi-scale approach for estimating city-wade anthropogenic heat fluxes, Atmos. Environ., 2014, 99, 64–76 CrossRef CAS.
- E. S. Krayenhoff, T. Jiang, A. Christen, A. Martilli, T. R. Oke, B. N. Bailey, N. Nazarian, J. A. Voogt, M. G. Giometto, A. Stastny and B. R. Crawford, A multi-layer urban canopy meteorological model with trees (BEP-Tree): street tree impacts on pedestrian-level climate, Urban Clim., 2020, 32, 100590 CrossRef.
- N. Meili, G. Manoli, P. Burlando, E. Bou-Zeid, W. T. Chow, A. M. Coutts, E. Daly, K. A. Nice, M. Roth, N. J. Tapper, E. Velasco, E. Vivoni and S. Fatichi, An urban ecohydrological model to quantify the effect of vegetation on urban climate and hydrology (UT&C v1. 0), Geosci. Model Dev., 2020, 13, 335–362 CrossRef CAS.
- G. Mussetti, D. Brunner, S. Henne, J. Allegrini, E. S. Krayenhoff, S. Schubert, C. Feigenwinter, R. Vogt, A. Wicki and J. Carmeliet, COSMO-BEP-Tree v1. 0: a coupled urban climate model with explicit representation of street trees, Geosci. Model Dev., 2020, 13(3), 1685–1710 CrossRef.
- I. A. Smith, J. B. Winbourne, F. K. Tieskens, T. S. Jones, F. L. Bromley, D. Li and L. R. Hutyra, A satellite-based model for estimating latent heat flux from urban vegetation, Front. Ecol. Evol., 2021, 30, 695995 CrossRef.
- R. Moriwaki, M. Kanda, H. Senoo, A. Hagishima and T. Kinouchi, Anthropogenic water vapor emissions in Tokyo, Water Resour. Res., 2008, 44, W11424 CrossRef.
- C. de Munck, G. Pigeon, V. Masson, F. Meunier, P. Bousquet, B. Tréméac, M. Merchat, P. Poeuf and C. Marchadier, How much can air conditioning increase air temperatures for a city like Paris, France?, Int. J. Biometeorol., 2013, 33, 210–227 Search PubMed.
- E. Gutiérrez, J. E. González, A. Martilli and R. Bornstein, On the anthropogenic heat fluxes using an air conditioning evaporative cooling parameterization for mesoscale urban canopy models, J. Sol. Energy Eng., 2015, 137(5), 051005 CrossRef.
- Y. Wang, Y. Li, S. Di Sabatino, A. Martilli and P. W. Chan, Effects of anthropogenic heat due to air-conditioning systems on an extreme high temperature event in Hong Kong, Environ. Res. Lett., 2018, 13, 034015 CrossRef.
- X. Wang, S. Miao, H. Liu, J. Sun, N. Zhang and J. Zou, Assessing the impact of urban hydrological processes on the summertime urban climate in Nanjing using the WRF model, J.
Geophys. Res.: Atmos., 2019, 124(23), 12683–12707 CrossRef.
- S. Vulova, A. D. Rocha, F. Meier, H. Nouri, C. Schulz, C. Soulsby, D. Tetzlaff and B. Kleinschmit, City-wide, high-resolution mapping of evapotranspiration to guide climate-resilient planning, Remote Sens Environ., 2023, 287, 113487 CrossRef.
- S. Henninger and W. Kuttler, Methodology for mobile measurements of carbon dioxide within the urban canopy layer, Clim. Res., 2007, 34, 161–167 CrossRef.
- B. Crawford and A. Christen, Spatial variability of carbon dioxide in the urban canopy layer and implications for flux measurements, Atmos. Environ., 2014, 98, 308–322 CrossRef CAS.
- L. E. Mitchell, E. T. Crosman, A. A. Jacques, B. Fasoli, L. Leclair-Marzolf, J. Horel, D. R. Bowling, J. R. Ehleringer and J. C. Lin, Monitoring of greenhouse gases and pollutants across an urban area using a light-rail public transit platform, Atmos. Environ., 2018, 187, 9–23 CrossRef CAS.
- B. G. Heusinkveld, G. J. Steeneveld, L. W. A. van Hove, C. M. J. Jacobs and A. A. M. Holtslag, Spatial variability of the Rotterdam urban heat island as influenced by urban land use, J. Geophys. Res.: Atmos., 2014, 119(2), 677–692 CrossRef.
- C. Jacobs, T. Singh, G. Gorti, U. Iftikhar, S. Saeed, A. Syed, F. Abbas, B. Ahmad, S. Bhadwal and C. Siderius, Patterns of outdoor exposure to heat in three South Asian cities, Sci. Total Environ., 2019, 674(15), 264–278 CrossRef CAS PubMed.
- I. Kousis, I. Pigliautile and A. L. Pisello, Intra-urban microclimate investigation in urban heat island through a novel mobile monitoring system, Sci. Rep., 2021, 11, 9732 CrossRef CAS PubMed.
- J. K. Lee, A. Christen, R. Ketler and Z. Nesic, A mobile sensor network to map carbon dioxide emissions in urban environments, Atmos. Meas. Tech., 2017, 10, 645–665 CrossRef CAS.
- D. E. Pataki, M. Alberti, M. L. Cadenasso, A. J. Felson, M. J. McDonnell, S. Pincetl, R. V. Pouyat, H. Setälä and T. H. Whitlow, The benefits and limits of urban tree planting for environmental and human health, Front. Ecol. Evol., 2021, 9, 603757 CrossRef.
- N. Meili, G. Manoli, P. Burlando, J. Carmeliet, W. T. Chow, A. M. Coutts, M. Roth, E. Velasco, E. R. Vivoni and S. Fatichi, Tree effects on urban microclimate: diurnal, seasonal, and climatic temperature differences explained by separating radiation, evapotranspiration, and roughness effects, Urban For Urban Green, 2021, 58, 126970 CrossRef.
- D. J. Sailor, A review of methods for estimating anthropogenic heat and moisture emissions in the urban environment, Int. J. Climatol., 2011, 31(2), 189–199 CrossRef.
- G. Katul, S. M. Goltz, C. I. Hsieh, Y. Cheng, F. Mowry and J. Sigmon, Estimation of surface heat and momentum fluxes using the flux-variance method above uniform and non-uniform terrain, Bound.-Layer Meteorol., 1995, 74, 237–260 CrossRef.
- M. Roth and T. R. Oke, Relative efficiencies of turbulent transfer of heat, mass, and momentum over a patchy urban surface, J. Atmos. Sci., 1995, 52(11), 1863–1874 CrossRef.
- B. Crawford, C. S. B. Grimmond, A. Gabey, M. Marconcini, H. C. Ward and C. W. Kent, Variability of urban surface temperatures and implications for aerodynamic energy exchange in unstable conditions, Q. J. R. Metorol. Soc., 2018, 144, 1719–1741 CrossRef.
- S. Liu, L. Lu, D. Mao and L. Jia, Evaluating parameterizations of aerodynamic resistance to heat transfer using field measurements, Hydrol. Earth Syst. Sci., 2007, 11, 769–783 CrossRef.
- M. Kanda, M. Kanega, T. Kawai, R. Moriwaki and H. Sugawara, Roughness lengths for momentum and heat derived from outdoor urban scale models, J. Appl. Meteorol. Climatol., 2007, 46, 1067–1079 CrossRef.
- J. A. Sobrino, R. Oltra-Carrió, J. C. Jiménez-Muñoz, Y. Julien, G. Soria, B. Franch and C. Mattar, Emissivity mapping over urban areas using a classification-based approach: Application to the Dual-use European Security IR Experiment (DESIREX), Int. J. Appl. Earth Obs. Geoinf., 2012, 18, 141–147 Search PubMed.
- T. Foken and B. Wichura, Tools for quality assessment of surface-based flux measurements, Agric. Meteorol., 1996, 78, 83–105 CrossRef.
- M. Roth, C. Jansson and E. Velasco, Multi-year energy balance and carbon dioxide fluxes over a residential neighborhood in a tropical city, Int. J. Climatol., 2017, 37, 2679–2698 CrossRef.
- I. D. Stewart and T. R. Oke, Local climate zones for urban temperature studies, Bull. Am. Meteorol. Soc., 2012, 93, 1879–1900 CrossRef.
- D. Bolton, The computation of equivalent potential temperature, Mon. Weather Rev., 1980, 108(7), 1046–1053 CrossRef.
- D. T. Lee and B. J. Schachter, Two algorithms for constructing a Delaunay triangulation, Int. J. Comp Inf. Sci., 1980, 9, 219–242 CrossRef.
- D. F. Watson and G. M. Philip, Triangle based interpolation, Math. Geol., 1984, 16, 779–795 CrossRef.
-
T. R. Oke, G. Mills, A. Christen and J. A. Voogt, Urban Climates, Cambridges University Press, USA, 2017, DOI:10.1017/9781139016476.
- A. Nordbo, L. Järvi and T. Vesala, Revised eddy covariance flux calculation methodologies – effect on urban energy balance, Tellus B, 2012, 64(1), 18184 CrossRef.
- S. Kim, Y. H. Lee, K. R. Kim and Y. S. Park, Analysis of surface energy balance closure over heterogeneous surfaces, Asia Pac. J. Atmos. Sci., 2014, 50, 553–565 CrossRef.
- S. I. Bohnenstengel, I. Hamilton, M. Davies and S. E. Belcher, Impact of anthropogenic heat emissions on London's temperatures, Q. J. R. Meteorol. Soc., 2014, 140(679), 687–698 CrossRef.
- A. K. Quah and M. Roth, Diurnal and weekly variation of anthropogenic heat emissions in a tropical city, Singapore, Atmos. Environ., 2012, 46, 92–103 CrossRef CAS.
- J. B. Moncrieff, Y. Malhi and R. Leuning, The propagation of errors in long-term measurements of land-atmosphere fluxes of carbon and water, Global Change Biol., 1996, 2(3), 231–240 CrossRef.
- M. Guevara, C. Tena, M. Porquet, O. Jorba and C. Pérez García-Pando, HERMESv3, a stand-alone multi-scale atmospheric emission modelling framework–Part 2: The bottom–up module, Geosci. Model Dev., 2020, 13, 873–903 CrossRef.
- Z. D. Weller, J. R. Roscioli, W. C. Daube, B. K. Lamb, T. W. Ferrara, P. E. Brewer and J. C. von Fischer, Vehicle-based methane surveys for finding natural gas leaks and estimating their size: Validation and uncertainty, Environ. Sci. Technol., 2018, 52(20), 11922–11930 CAS.
- H. Maazallahi, J. M. Fernandez, M. Menoud, D. Zavala-Araiza, Z. D. Weller, S. Schwietzke, J. C. von Fischer, H. Denier Van Der Gon and T. Röckmann, Methane mapping, emission quantification, and attribution in two European cities: Utrecht (NL) and Hamburg (DE), Atmos. Chem. Phys., 2020, 20, 14717–14740 CrossRef CAS.
- M. Roth, B. Sánchez, R. Li and E. Velasco, Spatial and temporal characteristics of near-surface air temperature across local climate zones in a tropical city, Int. J. Climatol., 2022, 9730–9752 CrossRef.
- V. Moosavi, G. Aschwanden and E. Velasco, Finding candidate locations for aerosol pollution monitoring at street level using a data-driven methodology, Atmos. Meas. Tech., 2015, 8(9), 3563–3575 CrossRef.
|
This journal is © The Royal Society of Chemistry 2023 |