Teaching old presumptive tests new digital tricks with computer vision for forensic applications†
Received
13th April 2023
, Accepted 26th June 2023
First published on 28th June 2023
Abstract
Presumptive (or ‘spot’) tests have served forensic scientists, law enforcement, and legal practitioners for over a hundred years. Yet, the intended design of such tests, enabling quick identification of drugs by-eye, also hides their full potential. Here, we report the development and application of time-resolved imaging methods of reactions attending spot tests for amphetamines, barbiturates, and benzodiazepines. Analysis of the reaction videos helps distinguish drugs within the same structural class that, by-eye, are judged to give the same qualitative spot test result. It is envisaged that application of these results will bridge the existing suite of field and lab-based confirmatory forensic tests, and support a broader range of colorimetric sensing technologies.
1 Introduction
Chemical presumptive (or ‘spot’) tests for the rapid detection of drugs have been developed and in use for over a century.1–3 Based on the principle of triggering colour changes on reaction with function groups specific to a particular drug class, a wide range of such tests, each providing different characteristic colour change on reaction with different drugs, are now widely available.1 By design (and name), the primary purpose of such tests is to enable rapid, by-eye identification of suspected illicit substances, helping law enforcement make quick decisions on the next course of action. Presumptive tests can also be considered the first in a line of increasingly specific analytical measurements made on an unknown batch of seized substance, en route to a full suite of confirmatory analysis that can be used as evidence for legal purposes. Indeed, the use of presumptive tests on their own is oftentimes not considered as a viable piece of evidence. The situation differs around the world.
Contradictory to their main purpose, the analytical challenge of spot tests comes when more than one substance gives the same suspected colour change (positive result) when exposed to the same reaction. In certain regions, such false positives can result in serious, criminal allegations being lodged at an innocent party.4
In this paper, we investigate what happens when these ‘shake and bake’ tests, normally analysed by-eye, are instead recorded on camera.
1.1 Drug legislation and relevant trends
Taking our base in the U.K. as an example, the legal classification and handling of drug-related activity is governed under several acts of parliament. Namely these include the Misuse of Drugs Act 1971, the Misuse of Drugs Regulation 2001, and the Psychoactive Substances Act 2016, attending the classification, supply, and control of different drug classes in circulation (legally or otherwise).
1.2 Presumptive tests
Named spot tests, partly delineated in Table 1, include the Mandelin,5 Dille–Koppanyi,6 Zimmermann,3 Mecke and Marquis tests.1 Our investigation has focused on digital variants of the first three of these tests. To aid with the subjectivity of assessing such tests by-eye, the Munsel or RAL colour charts are typically employed,7 with the latter being used to aid our investigation.
Table 1 Exemplar presumptive (or ‘spot’) tests and their primary targeted drug class
Test name |
Primary use |
Colour change |
Mandelin |
Amphetamines |
Yellow → Black |
Dille–Koppanyi |
Barbiturates |
Colourless → Purple |
Zimmermann |
Benzodiazepines |
Colourless → Purple/Red |
Mecke |
Opioids |
Yellow → Green/Black |
Marquis |
Opioids |
Colourless → Red |
1.3 Computer vision in analytical chemistry (CVAC)
1.3.1 Colour theory.
Computer vision encompasses the camera hardware and software used to digitally quantify colours and objects recorded in images and videos. Fig. 1 depicts some of the most commonly employed colour spaces. These mathematical frameworks are used to quantify the colours captured on camera pixels. Starting from RGB (red, green, blue) as the core quantification of colour from a digital camera, these raw data can be transformed into other colour spaces, including HSV (hue, saturation, value) and CIE–L*a*b* (lightness, green-red, and blue-yellow).8 It is with these colorimetric frameworks that, within chemistry alone, computer vision has blossomed as an independent analytical approach for non-contact determination of many phenomena.8–11
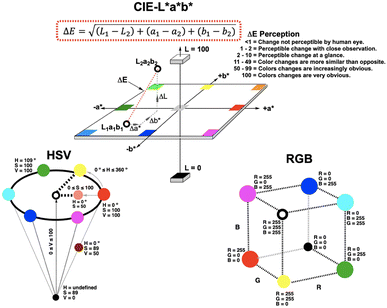 |
| Fig. 1 Simplified depiction of the RGB, HSV, and CIE–L*a*b* colour spaces. While ΔE from the CIE–L*a*b* space is the main tool exemplified herein, time-resolved data for all components of all colour spaces are accessible via Kineticolor computer vision analysis. | |
1.3.2 CVAC in forensics.
The use of digital imaging in forensic applications is well established across a range of use cases. Applications span handwriting recognition,12 face detection,13 security screening,13 blood stain characterisation,14–16 identification of chemical warfare agents,17 quantifying explosives,18 and ballistics.19
More specifically within presumptive testing, smart phone-enabled single imaging approaches have been applied to, for example, recreational drug detection in drinks,20 risk-mitigating false positives in cocaine detection,21 bodily fluid identification,15 digitalising amphetamine spot tests,22 and micro-device fabrication for more objective spot testing.23
1.4 Aims
Despite the above-mentioned advances in computer vision for forensic spot tests, few developments on time-resolved imaging approaches in the field have emerged to date. In 2019, Jornet-Martínez et al. showed that RGB analysis of single images, plotted versus time and deriving RGB numbers from available apps, could distinguish drug responses to polydimethylsiloxane (PDMS)-stabilised Marquis tests.24 Saito's team video recorded luminol chemiluminescence phenomena and used ImageJ in order to distinguish blood from ferrate salts.16
Based on our team's development of video analysis software platform Kineticolor for a range of chemical kinetics problems,25,26 we hypothesised that more highly (or at least, more flexibly) time-resolved digital spot testing could reveal characteristic kinetic differences between spot test results that, by-eye, might otherwise be deemed equivalent (Fig. 2). Indeed, our own efforts with Kineticolor represents an approach that is complementary to those of O'Brien,27 Hein,28 Ley,10 Aspuru-Guzik,29 and Kapur30 whose respective teams have also contributed valuable advances in the field of dynamic computer vision for other applications.
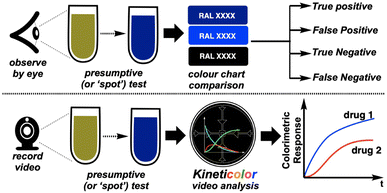 |
| Fig. 2 Hypothesis that introduction of time-resolved imaging could distinguish spot tests that, by-eye, can generate false positive and false negative results. | |
The conceptual workflow of this computer vision centred kinetic analysis is depicted in Fig. 3.
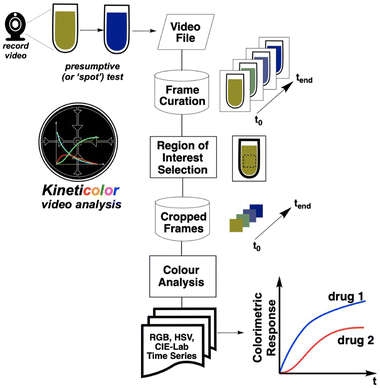 |
| Fig. 3 Conceptual workflow for deriving kinetic insights from video footage. | |
2 Results and discussion
2.1 Psychological assessment of spot test subjectivity
We began our study with a psychological experiment to demonstrate the aforementioned subjectivity of spot tests judged by-eye. To this end, 43 anonymous participants were asked to answer a short series of open-ended questions regarding comparisons of two colours. To avoid bias, participants were invited by third-party email and provided with no context (within the bounds of ethical approval) behind the colours in the photographs they were asked to look at.31 Participants were requested to describe, in plain english, the colour of a solution (which they were unaware was from a forensic spot test). Follow-up questions then prompted participants to compare solution colours from images of pairs of spot test results. All images presented to participants were cropped with the white ceramic tile still visible, enabling participants to subconsciously calibrate the contrast between the background and the coloured solution they were asked to judge.
In sum, the survey results showed that: (i) no single colour yielded a consensus on what the colour actually was (Fig. 4, left), and (ii) two structurally related drugs from different legal classes can yield by-eye results that are the same when they are supposed to differ in RAL chart colour (Fig. 4, right). Anonymised survey data are available in the ESI.†
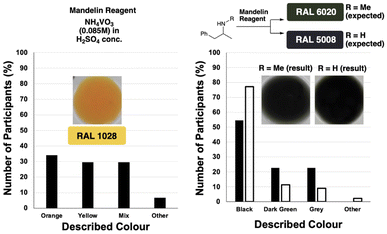 |
| Fig. 4 Survey data demonstrating the subjective challenges with presumptive spot tests whose results were determined by-eye. (Left) When asked to describe the colour of a single chemical solution, several answers were given. (Right) Two different amphetamine derivatives resulted in by-eye observations reported as a range of answers (filled bars = methamphetamine; unfilled bars = amphetamine). RAL colour chart numbers provided are those expected. | |
2.2 Proof-of-concept
To investigate the potential value of our Kineticolor video analysis suite in turning subjective spot tests into more a more objective evidence base, we began by recording video footage of tests like those in Fig. 4. The positive Mandelin test for methamphetamine was expected to produce colour changes of a bright yellow solution (RAL 1028) to a dark green solution (RAL 6020). This is thought to be driven by the reduction of V(V) to V(IV) and concurrent oxidation of the primary or secondary amine in the amphetamine drug.1 From Kineticolor analysis of the video recording, the colour contrast change, ΔE, over time was extracted, evidencing an obvious change in perceived colour (ΔE = 50) in the time of the experiment (Fig. 5).
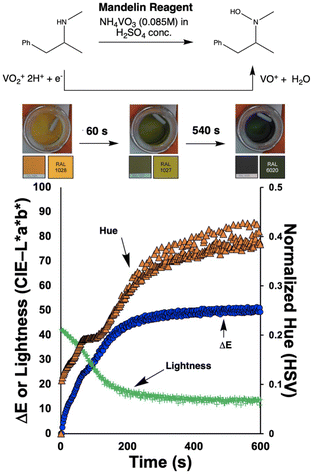 |
| Fig. 5 Proof-of-concept showing that a video recording of a positive test for methamphetamine using the Mandelin reagent holds valuable kinetic information. Primary y-axis: profiles of ΔE (blue circles) and lightness (green crosses). ΔE is a colour-agnostic measure of contrast, referenced against the colour observed at t = 0 s (frame 1) derived from the CIE-L*a*b* colour space. Secondary y-axis: normalized Hue (orange triangles) from the alternative HSV colour space, showing a trend from a yellow to a green colour over time. Images of the RAL code from the RAL K7 classic colour chart were taken under the same light source as the reactions. | |
While ΔE has tended to be used as the default exploratory metric,25,26 it was important at this early stage to sense-check this colour-agnostic metric versus the more colour-specific metrics output from Kineticolor analysis. In Fig. 4, Lightness (L*), as one of three components used to derive ΔE from the CIE–L*a*b* space, decreases with time, consistent with the observed darkening of the solution during the reaction of the methamphetamine solution with the Mandelin reagent. Furthermore, tracking the Hue (from HSV) shows a sensible evolution from yellow to green over the timescale of the video recording. These results are consistent with the more subjective RAL colour codes expected for such a presumptive test.
2.3 Comparative characterisation of spot tests with computer vision
The proof-of-concept justified looking at how kinetic colour profiles of drugs (within the same class and exposed to the same spot test reagent) might enable more quantitative comparison and characterisation of said drugs otherwise giving the same by-eye spot test result.
2.3.1 Amphetamines.
First, extending the proof-of-concept exercise to include analysis of amphetamine and MDMA as well as methamphetamine revealed three distinct ΔE profiles (Fig. 6). For the same mass quantity of each pure drug, the Mandelin tests evidenced rates of colour change in the order MDMA (fastest) > amphetamine > methamphetamine (slowest); a result consistent over triplicate runs of each spot test. This qualitative ordering of spot test rates of colour change was made more quantitative by fitting (by regression) the ΔE profiles to the base-2 exponential function shown in eqn (1): | 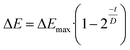 | (1) |
where ΔEmax is the maximum contrast plateau achieved in the recorded time, t is the reaction time, and D relates to the time at which ΔE equals
. As ΔEmax increases, the observable colour change after versus before the spot test was triggered becomes more stark. As D increases, recorded rate of colour change increases, and thus the time to reach ΔEmax decreases. These reaction parameters (Table 2) are consistent with the hypothesis that, while the observed spot test results by-eye may be similar, the rate of change may provide clearer identification. This point is manifest in the larger differences in D values than between ΔEmax values extracted from model fitting experimental data to eqn (1).
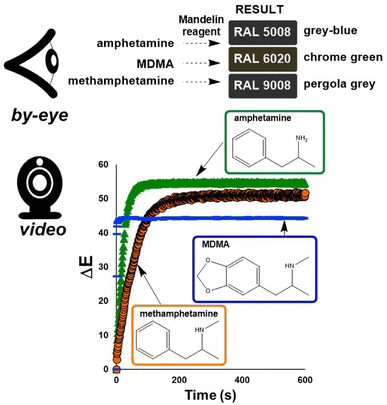 |
| Fig. 6 (Top) RAL colour chart results, as judged by-eye, for the Mandelin tests for amphetamine, MDMA, and methamphetamine.32 Bottom: ΔE profiles derived from video-recorded Mandelin tests for methamphetamine (orange circles), amphetamine (green triangles), and MDMA (blue lines). | |
Table 2 Extracted reaction parameters when modelling Mandelin test ΔE parameters from Fig. 5 using eqn (1). Model fittings for all experiments are available in the ESI
Drug |
ΔEmax |
D (s) |
RMSD |
Methamphetamine |
51.1 |
36.5 |
0.6 |
Amphetamine |
54.6 |
13.8 |
0.5 |
MDMA |
43.8 |
0.7 |
4.4 |
Considering the primary forensic application of our investigation, it was important to analyse impure ‘street’ samples via computer vision, in addition to pure drugs.33 To this end, we were able to demonstrate that the ΔE profile for the positive Mandelin test for methamphetamine was distinct from the profiles generated for the same test run on a 1
:
1 (w/w) mixture of methamphetamine cut with either glucose or caffeine (Fig. 7). The characteristic difference in each ΔE profile for the pure versus cut drugs is shown numerically for parameters extracted from the best fit of each profile to eqn (1) (Table 3). While such a capability might arguably have minimum value versus a by-eye spot test in cases like pure methamphetamine versus glucose-cut methamphetamine (these produce distinct colour results), the digitalisation of spot tests helps draw out more subtle by-eye colour differences for the likes of the glucose blank versus the glucose-cut methamphetamine.
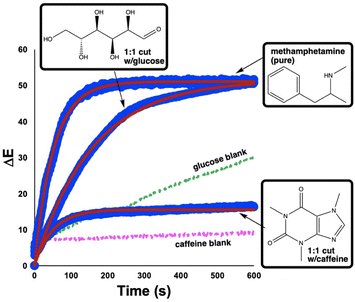 |
| Fig. 7 Comparison of ΔE profiles generated for Mandelin tests of pure methamphetamine (top), a 1 : 1 (w/w) cut of the drug with glucose (middle), and a similar cut with caffeine (bottom). Large blue circles indicate experimental data. Small red circles through each blue dataset represent best fit to eqn (1). Dashed green and pink lines denote blank Mandelin tests of pure glucose and caffeine, respectively. | |
Table 3 Extracted reaction parameters when modelling Mandelin test ΔE parameters from Fig. 6 using eqn (1) for pure methamphetamine versus the cut samples with either glucose or caffeine
Drug |
ΔEmax |
D (s) |
RMSD |
Methamphetamine (pure) |
51.1 |
36.5 |
0.6 |
Glucose cut |
51.6 |
109.4 |
4.7 |
Caffeine cut |
15.5 |
35.1 |
0.8 |
2.3.2 Benzodiazepines.
By extending our initial study of amphetamine derivatives to benzodiazepines, we aimed to explore a different structural drug class, and one that is of contemporary concern (at the time of writing) as a major drug of abuse, particularly in Scotland.34
Benzodiazepines are most commonly tested for using the two-component Zimmermann reagent.35 First, the suspected benzodiaepine sample is exposed to a solution of potassium hydroxide, deprotonating the methylene C–H group on the azepine ring. On exposure to the second component of the reagent, a positive colorimetric test results from the intermediate enolate generating a presumed Meisenheimer complex, turning solutions from colourless to dark red/purple.
As part of this study, we exposed a range of drugs in the benzodiazepine family to the standard Zimmermann test conditions, capturing video for all the reactions. Of the 6 drugs tested, initial assessment of results by-eye revealed that 5 gave detectable colour changes. The remaining drug (chlordiazepoxide) stayed colourless under test conditions (Table 4). The ΔE profiles for the visibly colour changing subset of benzodiazepines exposed to the Zimmermann test, each generated a characteristic profile (Fig. 8). Most informatively for the investigatory hypothesis of objectifying by-eye spot tests with computer vision, the ΔE profiles of flurazepam and flunitrazepam helped distinguish two positive Zimmermann tests that were otherwise identical by-eye (Table 4).
Table 4 Survey of Zimmermann test results for various benzodiazepines, by-eye, using the Zimmermann reagent
Drug |
Colour change |
RAL (start) |
RAL (end) |
Diazepam |
Clear → Brown |
9010 |
3007 |
Nitrazepam |
Clear → Yellow |
9010 |
1021 |
Flurazepam |
Clear → Brown |
9010 |
8016 |
Ketazolam |
Clear → Pink |
9010 |
3012 |
Flunitrazepam |
Clear → Brown |
9010 |
3007 |
Chlordiazepoxide |
None |
9010 |
9010 |
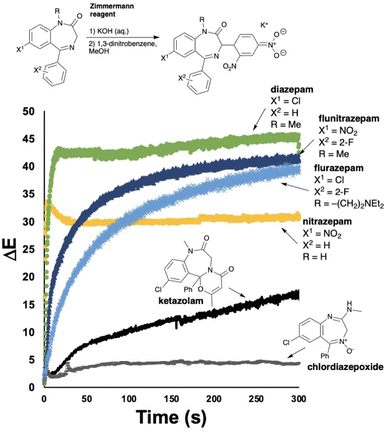 |
| Fig. 8 (Top) Simplified proposed mechanism responsible for the colour changes exploited by the Zimmermann test for benzodiazepines. (Bottom) Comparison of ΔE profiles generated for Zimmermann tests of pure benzodiazepine drugs. | |
2.4 Detection of unknown samples
To explore the potential use of characteristic ΔE traces as part of a confirmatory suite of forensic tests of unknown seized substances, the computer vision-enabled Mandelin and Zimmermann spot tests were repeated for two random blind samples, supplied to our lab without any identifying labels.
It was hypothesized that the shape more than the magnitude of ΔE traces over a fixed test time would serve to characterise one spot test result versus another. From the distinct colours apparent by-eye, it became clear that unknown A was likely to be an amphetamine derivative while unknown B was more likely to be a member of the benzodiazepine family. From this elimination process, we co-plotted normalized ΔE traces for amphetamine, methamphetamine, MDMA, and unknown A; nitrazepam and flurazepam were co-plotted with unknown B (Fig. 9). Consistent with visual inspection, quantitative comparison of the normalized ΔE profile of unknown sample A versus each of the known amphetamine derivatives revealed lower normalized root mean square deviation (RMSD, %) between unknown A and amphetamine than for MDMA or methamphetamine (Table 5). Similar experiments and RMSD scoring of ΔE profile differences across a set of recorded Zimmermann tests were used to tentatively identify unknown B as nitrazepam over flurazepam.
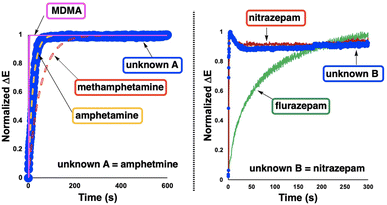 |
| Fig. 9 Using ΔE profiles to help identify unknown drugs from known spot test profiles. (Left) Application of normalized ΔE traces to identify an unknown amphetamine derivative from three candidates. The unknown trace is compared against models of the known candidates based on eqn (1). (Right) Application of normalized ΔE traces to identify unknown B (a benzodiazepine derivative) from two candidates. In both cases, the unknown sample is plotted as large blue circles. See Table 5 for supporting RMSD data. | |
Table 5 RMSD data used to quantify the differing levels of overlap between ΔE traces of drugs versus unknown samples A and Ba
Drug |
% RMSD (w/A) |
% RMSD (w/B) |
The asterisk is used to denote which drug each unknown was identified as. The line separating MDMA and flurazepam denotes tests performed with the Mandelin reagent (above the line) and Zimmermann reagent (below the line). The by-eye colour changes observed helped filter the necessary ΔE RMSD comparisons.
|
Amphetamine |
0.8* |
— |
Methamphetamine |
11.8 |
— |
MDMA |
12.4 |
— |
Flurazepam |
— |
24.6 |
Nitrazepam |
— |
3.9* |
2.5 Barbiturates
In a final extension of our study focused on developing a computer vision-enabled Dille–Koppanyi test for barbiturates,1,6 the opportunity was two-fold. First, as per the bulk of this study, we aimed to digitalise known by-eye tests as video recordings analysed with computer vision technology, for a range of barbiturates. As shown in Fig. 10 and Table 6, the recorded ΔE profiles for all barbiturates evidenced characteristic trends, many of which helped differentiate otherwise identical by-eye results. As first demonstrated in Fig. 5, it is once again important to remember that the dominant use of the colour agnostic contrast metric ΔE is merely the most convenient parameter extracted from the video footage. And, from these data, characteristic parameters of each trend were extracted using regression models fitted with eqn (1) (Table 7). Each digitalised spot test carries with it a wealth of additional information about the evolution of colour-specific parameters over time.
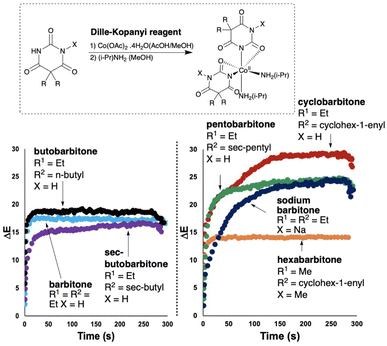 |
| Fig. 10 Comparison of ΔE profiles generated for Dille–Koppanyi tests of barbiturates. (Left) three differentiated profiles that give the same resulting violet colour by-eye. (Right) Further ΔE profiles of positive Dille–Koppanyi barbiturate tests, showing differentiation of pentobarbitone and sodium barbitone (both purple by-eye), as well as cyclobarbitone and hexabarbitone (red and pink by-eye, respectively). | |
Table 6 Survey of spot test results for various barbiturates, by-eye, using the Dille–Koppanyi reagent
Drug |
Colour change |
RAL (start) |
RAL (end) |
Barbitone |
Clear → Violet |
9003 |
4008 |
Butobarbitone |
Clear → Violet |
9003 |
4008 |
sec-Butobarbitone |
Clear → Violet |
9003 |
4008 |
Cyclobarbitone |
Clear → Red |
9003 |
4013 |
Hexabarbitone |
Clear → Pink |
9003 |
4003 |
Pentobarbitone |
Clear → Purple |
9003 |
4006 |
Sodium barbitone |
Clear → Purple |
9003 |
4006 |
Table 7 Extracted reaction parameters when modelling Dille–Koppanyi test ΔE parameters from Fig. 9 using eqn (1)
Drug |
ΔEmax |
D (s) |
RMSD |
Barbitone |
17.4 |
1.5 |
0.4 |
Butobarbitone |
18.5 |
0.7 |
0.7 |
sec-Butobarbitone |
15.8 |
4.4 |
0.7 |
Cyclobarbitone |
27.8 |
11.6 |
2.4 |
Hexabarbitone |
14.1 |
1.5 |
0.3 |
Pentobarbitone |
23.7 |
5.6 |
1.0 |
Sodium barbitone |
23.6 |
22.6 |
1.2 |
The second insight from the barbiturate case study centred on the coordination complex nature of the reagent, and its potentially tunable amine ligands. Indeed, when the original Dille–Koppanyi formulation, employing iso-propylamine, was modified to instead use the less encumbered n-propylamine, we recorded an increase of the rate of change of normalised ΔE versus the standard formulation (Fig. 11). These data are consistent with calculated steric maps from Nolan and Cavallo's Percent Buried Volume (% Vbur) model36 and related steric map visualisations,37,38 where the original Dille–Koppanyi formulation leads to more encumbered intermediates from which it is more difficult to bind the second of two anionic barbitone ligands (Fig. 12). This hypothesis and the steric maps are both consistent with Boys–Bernardi counterpoise calculations of binding energies for addition of the second barbitone ligand to the key, proposed Co(II) intermediate complex.39,40 The binding energy calculated was higher for the original reagent formulation than its less encumbered modification (Table 8). While consistent, these latter calculations, of course, assume that the thermodynamic parameter (final binding energy) directly correlates with the kinetic barrier to ligand coordination. The resulting DFT model complexes are pseudo-octahedral, consistent with literature reports of Co(II) barbitone tetrahydrate complexes.41
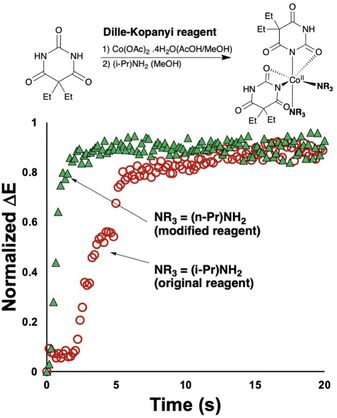 |
| Fig. 11 Different recorded reaction rates for original (NR3 = i-PrNH2; open red circles) and modified (NR3 = n-PrNH2; green triangles) versions of the Dille–Koppanyi test for barbitone, as measured by ΔE. | |
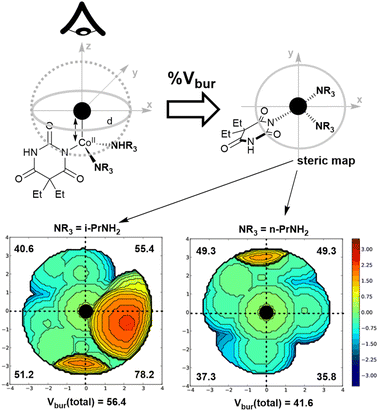 |
| Fig. 12 Steric maps to visualise the steric impact of switching the ancillary amine ligand used in the Dille–Koppanyi reagent. (Left) Original reagent. (Right) modified (less encumbered) reagent. Calculations are based on DFT-optimised geometries calculated on open-shell doublets at the M06-2X/Def2TZVP/LANL2DZ ECP (Co) level of theory. | |
Table 8 Counterpoise method for estimating relative binding energies of the ligation event [Co(barb)(NR3)2] + barb → [Co(barb)2(NR3)2]. All energies are in kcal mol−1
NR3 |
E
dist
|
E
int
|
E
bind
|
i-PrNH2 (original) |
−152.67 |
−260.92 |
−413.59 |
n-PrNH2 (modified) |
−154.65 |
−368.17 |
−522.82 |
While the links between camera-captured data and molecular specifics remains unclear, the ability to use macroscopic computer vision data of reaction bulk to inform microscopic cheminformatic modelling remains rare. We recently reported on this combined DFT and computer vision methodology for applications in peptide synthesis.42
3 Conclusions
In this study, we have shown how computer vision analysis holds potential to enhance the subjective nature of forensic spot tests towards a more objective, time-resolved, and specific analytical tool for the identification of seized substances. The time-resolved nature of the analysis enables more specific characterisation of drugs across structural and legal classes. The reported advance helps differentiate test results that, by-eye and by RAL colour chart reference, yield the same result. These remarks held true for studies spanning amphetamines, benzodiazepines, and barbiturates investigated with Mandelin, Zimmermann, and Dille–Koppanyi reagents, respectively.
The kinetic profiles extracted from the video-recorded spot tests enabled statistical comparison of their profile shapes. Such comparisons were applied to determining the most likely identity of an unknown sample whose colorimetric kinetic profile was compared to known samples. Such statistical comparisons were also valuable in analysing differences between pure and cut (‘street’) samples tested under the same conditions. Further, as exemplified in the study of the Dille–Koppanyi test for barbiturates and modifications therein, relative rates of colour change were shown to be consistent with cheminformatic and quantum chemical (DFT) calculations of steric encumbrance and ligand binding events, and with the proposed mechanism underpinning of this cobalt-centred test.
Attending the limitations and future developments of reaction monitoring method, we are not claiming that it stands to replace traditional spot tests, especially in cases where rapid, by-eye tests in the field are still valuable. Similarly, we are not claiming that the quantified test comparison enabled by regression modelling with eqn (1) is globally applicable. Indeed, a full understanding of the impact of drug adulterants and lighting changes in a range of field-relevant environments remain key challenges to be addressed in further research. It will, in relation, also be valuable to consider the broader impact of experiment illumination, beyond the measures taken herein and laid out in the ESI.† In turn, the impact of lighting changes on more complex drug formulation (i.e. material) will likely provide further insight into how to evolve this fundamental computer vision approach towards application. The focus in this preliminary study has been to demonstrate that, with ready accessible data-dense colour profiles that can be extracted from video footage, that such curve-fitting methods could be further developed for a range of spot test/drug class combinations. With all these limitations acknowledged, we envisage that the digitalisation of forensic spot tests could enable risk-mitigation of false positives, and add to the suite of confirmatory testing required by lab analysis and curators of evidence for use in legal matters.
Data availability
The following information is reproduced from Section 7 of the ESI PDF,† uploaded as part of this submission.
Excel files, Python scripts, and PDF outputs for all data discussed in the manuscript is available in an accompanying zipped folder, as part of this ESI† package:
Folder |
Subfolder |
Contents |
Figure 3 |
N/A |
Figure 3.xlsx |
Figure 4 |
N/A |
Figure 4.xlsx |
Figure 5_Table 2 |
N/A |
Figure 5.xlsx |
Table 2.xlsx |
Figure 6_Table 3 |
N/A |
Figure 6_Table 3.xlsx |
Figure 7 |
N/A |
Figure 7.xlsx |
Figure 8_Table 5 |
N/A |
Figure 8_amphetamine data.xlsx |
|
|
Figure 8_benzodiazepine data.xlsx |
Figure 8_Table 5 |
Table 5_RMSE code and input csv files |
amphetamine.csv |
|
|
flurazepam.csv |
|
|
mdma.csv |
|
|
methamphetamine.csv |
|
|
nitrazepam.csv |
|
|
RMSE.py |
|
|
unknown_A.csv |
|
|
unknown_B.csv |
Figure 9 |
N/A |
Figure 9.xlsx |
Figure 10 |
N/A |
Figure 10.xlsx |
Figure 10 |
FT-IR |
barbitone_2022-03-08T12-16-11.pdf |
|
|
barbitone and dille-koppanyi reagent crystals_2022-03-08T12-45-25.pdf |
|
|
barbitone and modified dille-koppanyi reagent crystals_2022-03-08T12-54-51.pdf |
|
|
cobalt(II) acetate tetrahydrate_2022-03-08T12-28-24.pdf |
Figure 11 |
Geometry Optimizations and Thermal Calcs |
i-Pr_UNSATURATED.log |
|
|
n-Pr_UNSATURATED.log |
Figure 11 |
Steric Map inputs and outputs |
i-Pr_UNSATURATED.xyz |
|
|
n-Pr_UNSATURATED.xyz |
|
|
SambVca-Result_i-Pr_UNSATURATED.pdf |
|
|
SambVca-Result_n-Pr_UNSATURATED.pdf |
Table 7 |
N/A |
Table 7.xlsx |
Table 8 |
N/A |
Barbitonate.log |
|
|
Table 8.xlsx |
|
|
i-Pr_COUNTERPOISE.log |
|
|
i-Pr_FULL_opt-freq.log |
|
|
n-Pr_COUNTERPOISE.log |
|
|
n-Pr_FULL_opt-freq.log |
The code for the proprietary Kineticolor software is not shared. This software is available under licence through the University of Strathclyde, contacting the corresponding author (E-mail: marc.reid.100@strath.ac.uk; ) and the intellectual property office (iprmanager@strath.ac.uk).
In due course, the software will be licensable through a standalone company, not yet incorporated at the time of this publication.
Author contributions
N. B.: methodology; formal analysis; data curation. C. O.: methodology; formal analysis; data curation. N. M.: methodology; formal analysis; data curation. J. M.: validation; formal analysis. C. Y.: validation; formal analysis. F. C.-D.: resources, methodology, supervision, project administration; writing (review & editing). M. R.: conceptualisation; methodology; software; formal analysis; resources; data curation; writing (original draft); writing (review & editing); visualisation; supervision; project administration; funding acquisition.
Conflicts of interest
M. R. is leading the commercialisation of the Kineticolor software.
Acknowledgements
The authors thank colleagues Alex Clunie, Lynn Curran, and Margaret Robinson, in the Centre for Forensic Science at the University of Strathclyde, for their support in acquiring and safe managing of the analytical samples of the drugs required for this study. MR thanks UK Research & Innovation for Future Leaders Fellowship funding (MR/T043458/1).
References
- M. Philp and S. Fu, Drug Test. Anal., 2018, 10, 95–108 CrossRef CAS PubMed.
- R. Singh, Crit. Rev. Anal. Chem., 2020, 1–18 Search PubMed.
- K. Artur Kovar and M. Laudszun, Can. Inst. Food Sci. Technol. J., 1978, 11, A108–A109 CrossRef.
-
R. Gabrielson, Since We Reported on Flawed Roadside Drug Tests, Five More Convictions Have Been Overturned, ProPublica, 2020 Search PubMed.
- C. F. Poe and D. W. O'Day, J. Am. Pharm. Assoc., 1930, 19, 1292–1299 CAS.
- J. M. Dille and T. Koppanyi, J. Am. Pharm. Assoc., 1934, 23, 1079–1084 CAS.
- L. D. Öztürk, Color Res. Appl., 2005, 30, 130–134 CrossRef.
- L. F. Capitán-Vallvey, N. López-Ruiz, A. Martínez-Olmos, M. M. Erenas and A. J. Palma, Anal. Chim. Acta, 2015, 899, 23–56 CrossRef PubMed.
- V. V. Apyari, M. V. Gorbunova, A. I. Isachenko, S. G. Dmitrienko and Y. A. Zolotov, J. Anal. Chem., 2017, 72, 1127–1137 CrossRef CAS.
- S. V. Ley, R. J. Ingham, M. O'Brien and D. L. Browne, Beilstein J. Org. Chem., 2013, 9, 1051–1072 CrossRef CAS PubMed.
- Y.-J. Shin and J.-B. Lee, Rev. Sci. Instrum., 2010, 81, 014302 CrossRef PubMed.
- A. R. Mamatsis, E. Mamatsi, C. Chalatsis, D. Arabadjis, P. Kampouri and C. Papaodysseus, Heritage Sci., 2023, 11, 38 CrossRef.
- S. Mo, P. Lu and X. Liu, Sensors, 2022, 22, 8228 CrossRef PubMed.
-
M. H. F. Butt, H. Ayaz, M. Ahmad, J. P. Li and R. Kuleev, 2022 IEEE Congress on Evolutionary Computation, CEC, 2022, pp. 1–8 Search PubMed.
- T. Layne, K. Jackson, A. Scott, N. A. Tanner, A. Piland, D. M. Haverstick and J. P. Landers, J. Forensic Sci., 2021, 66, 1033–1041 CrossRef CAS PubMed.
- K. Saito, M. Kogure, A. Sonoda and R. Ito, Forensic Chem., 2020, 21, 100278 CrossRef CAS.
- M. J. Kangas, R. M. Burks, J. Atwater, R. M. Lukowicz, P. Williams and A. E. Holmes, Crit. Rev. Anal. Chem., 2017, 47, 138–153 CrossRef CAS PubMed.
- A. Choodum, P. Kanatharana, W. Wongniramaikul and N. NicDaeid, Forensic Sci. Int., 2012, 222, 340–345 CrossRef CAS PubMed.
- S. N. Jaluddin, Z. M. Zain, M. I. A. Halim, M. F. Safian, M. A. A. Rani and M. S. M. Subri, Indones. J. Chem., 2021, 21, 1550–1559 CrossRef CAS.
- A. Procida and K. C. Honeychurch, J. Forensic Sci., 2022, 67, 1697–1703 CrossRef CAS PubMed.
- M. C. A. Marcelo, K. C. Mariotti, R. S. Ortiz, M. F. Ferrão and M. J. Anzanello, Microchem. J., 2016, 127, 87–93 CrossRef CAS.
- A. Choodum and N. N. Daeid, Drug Test. Anal., 2011, 3, 277–282 CrossRef CAS PubMed.
- S. T. Krauss, T. P. Remcho, S. M. Lipes, R. I. Aranda, H. P. I. Maynard, N. Shukla, J. Li, R. E. J. Tontarski and J. P. Landers, Anal. Chem., 2016, 88, 8689–8697 CrossRef CAS PubMed.
- N. Jornet-Martínez, R. Herráez-Hernández and P. Campíns-Falcó, Anal. Bioanal. Chem., 2019, 411, 2141–2148 CrossRef PubMed.
- H. Barrington, A. Dickinson, J. McGuire, C. Yan and M. Reid, Org. Process Res. Dev., 2022, 26, 3073–3088 CrossRef CAS PubMed.
- C. Yan, M. Cowie, C. Howcutt, K. M. P. Wheelhouse, N. S. Hodnett, M. Kollie, M. Gildea, M. H. Goodfellow and M. Reid, Chem. Sci., 2023, 14, 5323–5331 RSC.
- M. O'Brien and R. Moraru, ChemPlusChem, 2023, 88, e202200167 Search PubMed.
- P. Shiri, V. Lai, T. Zepel, D. Griffin, J. Reifman, S. Clark, S. Grunert, L. P. Yunker, S. Steiner, H. Situ, F. Yang, P. L. Prieto and J. E. Hein, iScience, 2021, 24, 102176 CrossRef CAS PubMed.
- S. Vargas, S. Zamirpour, S. Menon, A. Rothman, F. Häse, T. Tamayo-Mendoza, J. Romero, S. Sim, T. Menke and A. Aspuru-Guzik, J. Chem. Educ., 2020, 97, 689–694 CrossRef CAS.
- J. Daglish, A. J. Blacker, G. de Boer, A. Crampton, D. R. J. Hose, A. R. Parsons and N. Kapur, Org. Process Res. Dev., 2023, 27, 627–639 CrossRef CAS PubMed.
- I. E. Dror, Anal. Chem., 2020, 92, 7998–8004 CrossRef CAS PubMed.
- E. Cuypers, A. J. Bonneure and J. Tytgat, Drug Test. Anal., 2016, 8, 137–141 CAS.
- Y. Peck, A. R. Clough, P. N. Culshaw and M. J. Liddell, Drug Alcohol Depend., 2019, 201, 49–57 CrossRef CAS PubMed.
- J. v. Amsterdam, W. v. d. Brink and M. Pierce, Eur. Addict. Res., 2021, 27, 399–412 CrossRef PubMed.
-
K. Kovar and M. Laudszun, SCITEC/6, United Nations, Vienna, 1989, https://www.unodc.org/pdf/scientific/SCITEC6.pdf, accessed 5th July, 2023 Search PubMed.
- A. Gómez-Suárez, D. J. Nelson and S. P. Nolan, Chem. Commun., 2017, 53, 2650–2660 RSC.
- L. Falivene, R. Credendino, A. Poater, A. Petta, L. Serra, R. Oliva, V. Scarano and L. Cavallo, Organometallics, 2016, 35, 2286–2293 CrossRef CAS.
- L. Falivene, Z. Cao, A. Petta, L. Serra, A. Poater, R. Oliva, V. Scarano and L. Cavallo, Nat. Chem., 2019, 11, 872–879 CrossRef CAS PubMed.
- S. F. Boys and F. Bernardi, Mol. Phys., 1970, 19, 553–566 CrossRef CAS.
- K. Kirschner, J. Chem. Educ., 2007, 84, 1225–1229 CrossRef.
- N. N. Golovnev, L. A. Solovyov, M. K. Lesnikov, S. N. Vereshchagin and V. V. Atuchin, Inorg. Chim. Acta, 2017, 467, 39–45 CrossRef CAS.
-
C. Yan, C. Fyfe, C. Jamieson and M. Reid, Computer Vision as a New Paradigm for Monitoring of Solution and Solid Phase Peptide Synthesis, 2023, https://chemrxiv.org/engage/chemrxiv/article-details/64070568cc600523a3cb7904 Search PubMed.
|
This journal is © The Royal Society of Chemistry 2023 |