DOI:
10.1039/D3AN00353A
(Paper)
Analyst, 2023,
148, 2415-2424
Comparison of the biological effects of gadodiamide (Omniscan) and gadoteridol (ProHance) by means of multi-organ and plasma metabolomics†
Received
6th March 2023
, Accepted 19th April 2023
First published on 24th April 2023
Abstract
Gadolinium-based contrast agents (GBCAs) are massively employed in radiology to increase the diagnostic power of MRI. However, investigations aiming at detecting possible metabolic perturbations or adverse health effects due to gadolinium deposition are still lacking. In this work, aqueous organs extract and plasma samples were analyzed by GC-MS and 1H-NMR, respectively, to investigate the effects of multiple administrations of one linear (Omniscan) and one macrocyclic (ProHance) GBCA, on the main metabolic pathways in healthy mice. Multivariate analysis revealed that plasma metabolome was not differently perturbed by the two GBCAs, while, the multiorgan analysis displayed a clear separation of the Omniscan-treated from the control and the ProHance-treated groups. Interestingly, the most affected organs were the brain, cerebellum and liver. Thus, this work paves the way to both the safest use of the commercially available GBCAs and the development of new GBCAs characterized by lower general toxicity.
1. Introduction
Contrast agents have been used to enhance the contrast of images in magnetic resonance imaging (MRI) since the 1980s. Most of the contrast agents are based on strongly paramagnetic metal ions, such as iron, manganese, or gadolinium. These metal ions are able to alter the relaxation times of protons in their vicinity thus increasing the signal intensity of water protons in the tissues. Currently available agents are mainly gadolinium-based, and it is estimated that a total of about 10 million doses of gadolinium-based contrast agents (GBCAs) are administered annually worldwide.1 Free gadolinium (Gd) is known to be toxic to the human body and may cause spleen, liver, and bone damage.2 To circumvent the toxic effects of free Gd, and simultaneously maintain its excellent magnetic properties, the free ions are commonly chelated by polyaminocarboxylic acid compounds, which can be either macrocyclic or linear. A large body of research has demonstrated that macrocyclic GBCAs are more stable in vivo toward dissociation compared to the linear ones.3–5 GBCAs are rapidly cleared from the intravascular space through kidney excretion in patients with normal renal function (90% eliminated during the first 12 hours). Consequently, GBCAs should not distribute into the central nervous system unless the blood–brain barrier is impaired, and only small amounts can enter the brain intra-cellular fluid through the exchange with cerebrospinal fluid via the glymphatic system.6–8 Nevertheless, extensive evidence of gadolinium retention in the deep cerebellar nuclei (DCN) after repeated injections of linear, and, to a far less extent, macrocyclic GBCAs has been reported.9–14 Most of the studies have been conducted on postmortem samples or resections pieces and they have shown the presence of non-negligible quantities of gadolinium in brain tissue of patients. However, investigations aiming at detecting possible metabolic perturbations and/or potential adverse health effects due to long-term gadolinium deposition are still lacking. The only valuable attempt has been made by El Hamrani and collaborators in 2020.15 Their aim was to determine potential metabolism and histological modifications due to gadolinium retention within DCN after repeated injections of gadodiamide (Omniscan, linear GBCA) in rats. The results of their study confirmed the retention of gadolinium; however, they did not detect any changes in the levels of the few measured brain metabolic biomarkers nor they found histological modifications within the DCN. Nevertheless, in that study, the use of the relatively low sensitive in vivo1H-NMR technique hampered the detection of many of the possible modifications of cerebellar metabolism, thus a comprehensive assessment of the metabolic perturbation induced in different organs by repeated injection of a linear GBCA is still missing. The only way to obtain such a global picture of the metabolic changes that occur at different levels in an organism is to employ a holistic approach such as a metabolomics study. Metabolomics allows a large-scale study of small molecules, commonly known as metabolites, within cells, biofluids, and tissues. Being the end-products of cellular regulatory processes, metabolites levels can be regarded as the ultimate response of an organism to genetic or environmental changes (diseases, treatments, nutrition, etc.), thus reflecting the physiological state of such organism.16 Indeed, metabolomics represents a paradigm shift in medical research from approaches that focus on a limited number of enzymatic reactions or single pathways, to a global view of the complexity of metabolic networks and their alterations after external stimuli.17 Thus, we decided to employ a multi-organ and plasma metabolomics approach to investigate the effects of multiple administrations of the ‘riskiest’ linear GBCA, i.e., Omniscan (gadiodiamide), compared to ProHance (gadoteridol), a macrocyclic GBCA, known to give rise to very limited gadolinium retention in vivo. Here, by following a well-established preclinical experimental work-up to simulate the condition of patients receiving several life-span GBCAs administrations, we report a study where mice received twenty consecutive injections of each GBCA during a 5-week period, allowing us to obtain, 1 month later, a clear and complete picture of the global metabolic variations induced by each GBCA compared to the control group (Fig. 1). It has been found, in fact, that successive administrations of GBCA to rodents over periods of few weeks lead to the retention of Gd in the brain structures that mimic the pattern observed in patients.5,14,15
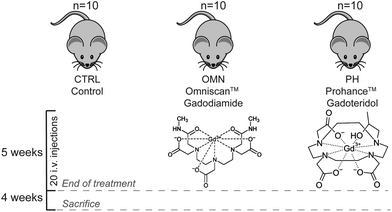 |
| Fig. 1 Overview of the study design. A total of thirty mice divided into three groups: Control, Omniscan, and ProHance-treated groups. Every group received 4 i.v. injections per week, for 5 weeks, accounting for a total of 20 injections. | |
2. Experimental
2.1 Chemicals
Analytical grade chloroform, methanol, trimethylsilyl cyanide (TMSCN) (99.8%) and C10–C40 all-even alkane mixture, heparin, and all other chemicals were purchased from Merck (Darmstadt, Germany). Water used throughout the study was purified using a Millipore Milli-Q lab water system (Merck Millipore, Darmstadt, Germany) equipped with a 0.22 μm filter membrane. Gd-based contrast agents employed in this study were (i) ProHance (Gd-HPDO3A, gadoteridol, 0.5 mol L−1; Bracco Imaging, Milan, Italy) and (ii) Omniscan (Gd-DTPA-BMA, gadodiamide, 0.5 mol L−1; GE Healthcare, Chalfont St Giles, England).
2.2 Animal study
A total of thirty male Balb/c mice (Envigo RMS S.r.l, Udine, Italy) were used for the in vivo injection of GBCAs (8–10-week-old, mean weight 20 ± 2 g). All mice were kept in standard housing with the same standard rodent chow and water available ad libitum, and a 12 h light/dark cycle. Experiments were performed according to the national laws on experimental animal (L.D. 26/2014; Directive 2010/63/EU) and were approved by the Italian Health Ministry (authorization number: 808/2017-PR in 19/10/2017, integration in 18/03/2020). Mice were randomly divided into three groups (n = 10 for each group) and treated as follows: (i) Control mice, (ii) i.v. injection of ProHance at the dose of 0.6 mmol kg−1 and (iii) i.v. injection of Omniscan at the dose of 0.6 mmol kg−1. GBCAs were administrated four times for week, for a total of 20 administrations. Mice were housed for one month after the treatments, then they were ethically sacrificed by cervical dislocation and organs excised for further analysis. In particular, blood and the following organs were collected: brain, cerebellum, kidneys, liver, spleen. A total of 0.7 mL of blood were collected from each test subject, added with heparin (final concentration of 40 IU mL−1) to avoid coagulation, and centrifuged at 1183 rcf, at 4 °C. Blood plasma was collected and used for analysis. Other organs were weighted, added of fresh PBS and mechanically homogenized (Homogenizer OV5;VELP Scientifica, Usmate Velate, Italy). Details about the weight of the fresh tissues and volumes of added buffer are reported in Table S1.† Then, homogenates were frozen at −80 °C and stored for further analysis.
2.3
1H-NMR-based plasma analysis
A total of thirty frozen plasma samples were thawed at room temperature and prepared by mixing 350 μL of plasma with 350 μL of sodium phosphate buffer (70 mM Na2HPO4; 20% v/v D2O, 6.1 mM NaN3; pH 7.4) as reported elsewhere.18 They were then briefly vortexed and 650 μl of the mixture were transferred into 5 mm NMR tubes for analysis. One-dimensional 1H-NMR spectra were acquired at 37 °C, by employing a 600 MHz Bruker Avance NEO 600 MHz spectrometer (Bruker Biospin Gmbh, Rheinstetten, Germany) equipped with a QCI cryo-probe set for 5 mm sample tubes and a cooled autosampler (SampleJet, at 300 K). The 1H-NMR spectra were acquired with Topspin 4.1 (Bruker Biospin GmbH, Rheinstetten, Germany), using both the standard noesygppr1d (1D NOESY) and the cpmgpr1d (CPMG) pulse sequences. The first allows for a quantitative evaluation even close to the water signal which was presaturated at 4.704 ppm. The latter reduces the broad resonances from high-molecular-weight compounds, allowing the observation of low-molecular-weight metabolites. All samples were automatically tuned, matched and shimmed. Prior to Fourier transformation, the free induction decays were multiplied by an exponential function equivalent to a 0.3 Hz line-broadening factor. Then, the transformed spectra were automatically corrected for phase and baseline distortions and calibrated using Topspin built-in processing tools. The NMR assignment was achieved by (i) analysis of literature data;19,20 (ii) comparison with the chemical shifts of the metabolites in the Human Metabolome Database (HMDB); (iii) peak fitting routine within the spectral database in Chenomx NMR Suite (version 8.6, Chenomx Inc., Edmonton, Alberta, Canada, https://www.chenomx.com, date of last access: 11 November 2022).
2.4 GC-MS analysis of aqueous extracts of mice brain, cerebellum, kidney, liver and spleen
A total of 150 homogenized organ samples (5 organs × 30 mice) were thawed at room temperature, centrifuged at 7000 rcf at 4 °C for 15 min and then the supernatants were submitted to a dual phase extraction procedure as reported elsewhere.21 Briefly, a mixture of water (already present in the homogenized sample), methanol and chloroform in the volume ratio of 0.9
:
1
:
1 was added to each sample. The samples were first briefly vortexed and then centrifuged at 21
000 rcf at 4 °C for 15 min. This procedure generated a two-phase extract: the aqueous upper phase containing hydrophilic metabolites, while non-polar metabolites, as lipid molecules, moved in the organic lower phase. Proteins and macromolecules were trapped, instead, in the thin skin-like layer between the two phases. For each organ, different amounts of the aqueous phases (see Table S1† for details) were then transferred into glass inserts and dried by using a SpeedVac Concentrator, for 4 hours. The glass inserts were then sealed with airtight magnetic lids into GC-MS vials and derivatized by addition of appropriate volumes of trimethylsilyl cyanide (TMSCN) (Table S2†) in a 1
:
1 ratio as described elsewhere.22 Derivatization and injection were fully automated using a PAL autosampler with RTC system (CTC Analytics, Zwingen, Switzerland) integrated to the GC-MS-TOF (Pegasus BT, LECO Corporation, Saint Joseph, MI, USA). The GC-MS consisted of an Agilent 7890B GC (Agilent Technologies, Santa Clara, California, USA) and a time-of-flight mass spectrometer (LECO Corporation, Saint Joseph, MI, USA). After the addition of the derivatization reagent, samples were transferred into the agitator of the autosampler and incubated at 40 °C for 40 min at 750 rpm. This procedure ensures precise derivatization time and reproducible sample injection. Immediately after derivatization, 1 μl of the derivatized sample was injected into the injection port, in splitless mode. The septum purge flow and purge flow were set to 25 and 15 mL min−1, respectively. The injection port temperature was set to 250 °C. GC separation was performed on a DB-5MS UI 5% Phenyl 95% Dimethylpolysiloxane column (30 m with I.D. 0.25 mm and film thickness 0.25 μm) (Agilent Technologies, Santa Clara, California, USA). The initial temperature of the GC oven was set to 40 °C and held for 2 min, followed by heating at 12 °C min−1 to 320 °C and kept for an additional 8 min, making the total run time 33.3 min. Mass spectra were recorded in the range of 45–600 m/z with an acquisition rate of 10 spectra per second, and MS detector and ion source were switched off during the first 6.4 min of solvent delay time. The transfer line and ion source temperature were set to 280 °C and 250 °C, respectively. Helium (grade 6.0) was used as carrier gas, at a constant flow rate of 1 mL min−1. The mass spectrometer was tuned according to manufacturer's recommendation using perfluorotributylamine (PFTBA). The autosampler and GC-MS were controlled using vendor software PAL Sample Control (CTC Analytics, Zwingen, Switzerland) and ChromaTOF (LECO Corporation, Saint Joseph, MI, USA), respectively. Two technical replicates were prepared for each sample, they were then randomized prior to derivatization and GC-MS analysis. In order to monitor the instrument performance, a blank sample containing only derivatization reagent, a control sample (pooled sample), and an alkane mixture standard sample (all even C10–C40 alkanes at 50 mg L−1 in hexane) were injected after every 10 real samples. The raw GC-TOF-MS data was processed by the ChromaTOF Sync software (Version v0.93.0.0-alpha) that compiles peak information through sets of samples by performing peak finding and deconvolution on the sample set, producing a composite sample set peak table; moreover, it performs peak identification using NIST11 library (NIST, Gaithersburg Maryland, USA). Deconvoluted peaks were aligned across all samples considering a retention time shift allowance of 10 s and a signal to noise ratio of 10 while the library search was set to return top 10 hits with EI-MS match of >800 using normal-forward search. In this way, final datasets consisting of 27 metabolites for the brain, 27 metabolites for the cerebellum, 30 metabolites for the kidney, 42 metabolites for the liver, and 27 metabolites for the spleen, were obtained. The level of identification for each metabolite is generally assigned according to the Metabolomics Standard Initiatives (MSI):23 (i) level 1 if the peaks are confirmed using authentic standards, (ii) level 2 when the peaks are identified based on their EI-MS ≥80 (%) and Retention Index (RI) match (±50) and (iii) level 3 when the peaks are identified based on their EI-MS ≥65 (%). In our case, no authentic standards were employed and only the metabolites characterized by EI-MS ≥80 (%) were considered for data analysis. The complete list of identified metabolites in the five organs is reported in Table S3.†
2.5 Univariate and multivariate data analysis
2.5.1
1H-NMR plasma data.
1H-NMR spectra (1D NOESY and CPMG) were imported into MATLAB (R2015b The Mathworks Inc., Natick, MA) where the spectral regions above 10 ppm and below 0 ppm were removed since no relevant signals were present. To correct for spectral misalignment, an interval-based alignment step was carried out using the icoshift algorithm24 and choosing the alanine doublet at 1.49 ppm as a reference signal. Then, to reduce the model complexity and filter out noise while improving the interpretation effort, the peak areas of the known signals from high- and low-molecular-weight metabolites were individually integrated using Multivariate Curve Resolution (MCR).25 This approach, already successfully applied by the authors26 allows to extract deconvoluted spectral profiles and their relative integrated areas, thus solving the common NMR issue concerning the integration of overlapping signals. In brief, each plasma NMR dataset (1D NOESY and CPMG) was first divided into small intervals (containing one or more individual and/or overlapped signals) which were then modelled by building, for each interval, four different MCR models of increasing complexity (from 2 to 5 components). The best performing model was then chosen, and the resolved signals were therefore identified and assigned, as reported in Fig. S1 and S2.† The results of the interval-based resolution process consisted of 11 resolved chemical components for the 1D NOESY dataset, and of 19 resolved components for the CPMG dataset, accounting for a total of 38 components. The final data matrix, consisting of 30 samples and 38 metabolites relative concentrations, was then submitted to Principal Component Analysis (PCA).27
2.5.2 GC-MS organs data.
Five different data matrices (one for each organ), generated by the ChromaTOF SYNC software, were investigated by employing both univariate and multivariate data analysis under MATLAB environment.
In order to assess if each metabolite's concentration significantly differed among the three studied groups, a one-way ANOVA (anova1 MATLAB function) test was performed. Then, the multcompare MATLAB function, using the Tukey–Kramer correction, was employed to perform a multiple comparison (post-hoc) test. The anova1 and multcompare functions were available through the “Statistics and Machine Learning Toolbox” of MATLAB. A p value below 0.05 was considered statistically significant. Details about GC-MS metabolite identification, ANOVA, and multiple comparison analysis outputs are reported in Table S3.†
Then, low-level data fusion28 was performed by concatenating the data matrices of the five different organs to generate an individual data matrix, made of 30 rows (samples) and 155 columns (metabolites). Prior to data fusion each data matrix was individually normalized using the Norm1 approach, which consists of dividing each variable by the sum of all variables within a sample.
Then the fused matrix was inspected by employing PCA. Before PCA, two pre-processing steps were applied: autoscaling and group scaling. The aim of the autoscaling preprocessing (also called “unit variance scaling”) is to let all variables have the same chance to affect the model, and it consists of mean center followed by scaling, the latter performed using the standard deviation of each variable.29 The step of group scaling (gscale MATLAB function) was carried out to allow each block to have the same impact on the final model, independently from the number of variables they contain. PCA was then computed by using the PLS_Toolbox (v8.6.2, Eigenvector Research, Manson, WA, USA) under MATLAB environment.
3. Results
Thirty mice were divided into three groups according the treatment they received (see Experimental section): control group (CTRL), Omniscan-treated group (OMN), and the ProHance-treated group (PH). Plasma, brain, cerebellum, kidney, liver, and spleen were recovered from each animal 1 month after the end of the treatments and submitted to a metabolomic study.
3.1
1H-NMR-based metabolomics analysis of plasma
Plasma samples from the three groups of mice (CTRL, OMN, and PH) were analyzed by 1H-NMR spectroscopy. 1D NOESY (Fig. S3†) and CPMG (Fig. 2) experiments were carried out to retrieve information about the high- and low-molecular-weight metabolites, respectively.
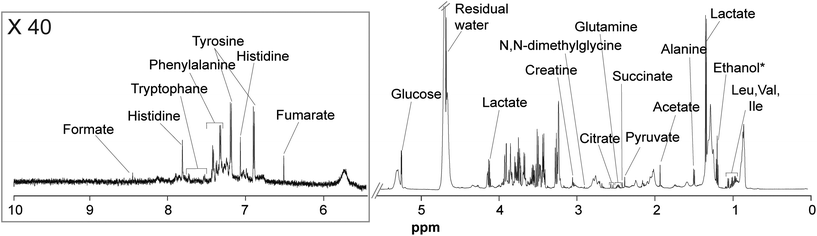 |
| Fig. 2
1H-NMR (CPMG) spectrum of a representative control sample along with the signal assignment. *Ethanol and water residual signals were not included in the analysis. | |
High-molecular-weight metabolites detected in 1D NOESY experiments of plasma included lipids, glycerophosphocholine, various phosphocholines, lipoproteins and cholesterol; whereas, low-molecular-weight metabolites detected in CPMG experiments included amino acids (tryptophan, phenylalanine, histidine, tyrosine, N,N-dimethylglycine, glutamine, alanine, valine, isoleucine, valine, and leucine), organic acids (formate, fumarate, lactate, citrate, succinate, pyruvate, and acetate) as well as glucose and creatine.
In order to recover reliable integrations of the signals from both NMR sets of experiments, the spectra were subjected to an advanced chemometric approach called Multivariate Curve Resolution (MCR). This method allowed us to reliably assign the nature and the relative concentration of 38 metabolites. Data were then submitted to Principal Component Analysis (PCA), a multivariate data analysis used to retrieve patterns and correlation between the samples and the measured metabolites, thus allowing to preliminary explore the data. Scores plots of the PCA models calculated including CTRL, OMN, and PH groups are reported in the ESI (Fig. S5 and S6†). To specifically investigate the differences between the OMN and PH groups, it was decided to perform a PCA only including those groups. Interestingly, it turned out that the plasma of the OMN group had lower levels of alanine, pyruvate and lactate compared to the plasma of the PH group (Fig. 3). Univariate statistics confirmed that pyruvate level was significantly different between the two groups (Table S4†).
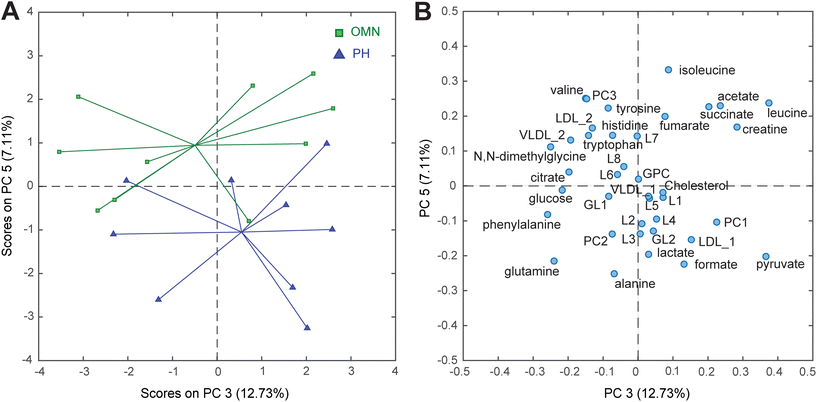 |
| Fig. 3 (A) PC3 vs. PC5 scores and (B) loadings plots of the PCA model calculated using the MCR integrated peaks of the 1D NOESY and CPMG spectra of plasma sample. Keys (score plot) Omniscan-treated mice (OMN, green) and ProHance-treated mice (PH, blue). Keys (loading plot) L1-8: lipid 1-8; GPC: glycerophosphocholine; GL1-2: glyceryl lipid 1-2; PC1-3: phosphatidylcholine 1-3; VLDL_1 and VLDL_2: very low-density lipoprotein; LDL_1 and LDL_2: low-density lipoprotein. | |
3.2 GC-MS-based metabolomics analysis of the organs
The aqueous extracts of brains, cerebella, kidneys, livers, and spleens retrieved from the CTRL, OMN and PH groups were analyzed by GC-MS (Fig. S4†). The deconvoluted data, consisting of 155 metabolites detected across the five investigated organs, were then submitted to PCA. By inspecting the PC1 vs. PC2 scores plot (Fig. 4), it is apparent that PC1 only shows the biological variability that characterizes this animal model, thus not allowing a clear distribution of the three groups of samples.
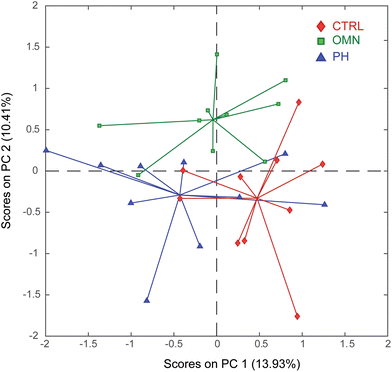 |
| Fig. 4 PC1 vs. PC2 scores plot of the PCA model calculated using all organ extracts analyzed by GC-MS. Keys: Control (CTRL, red), Omniscan-treated mice (OMN, green), ProHance-treated mice (PH, blue). | |
On the other hand, interestingly, PC2 displays a clear separation of the OMN group (lying in the upper part of the plot) from the PH and CTRL groups (bottom part of the plot), suggesting that treatment with Omniscan perturbs the physiological state of the mouse model. Furthermore, the proximity of the PH group to the CTRL one also suggests that the effect of the ProHance administration does not significantly affect the mice metabolome. To understand the reasons of this separation it is important to look at the loadings plot (Fig. 5).
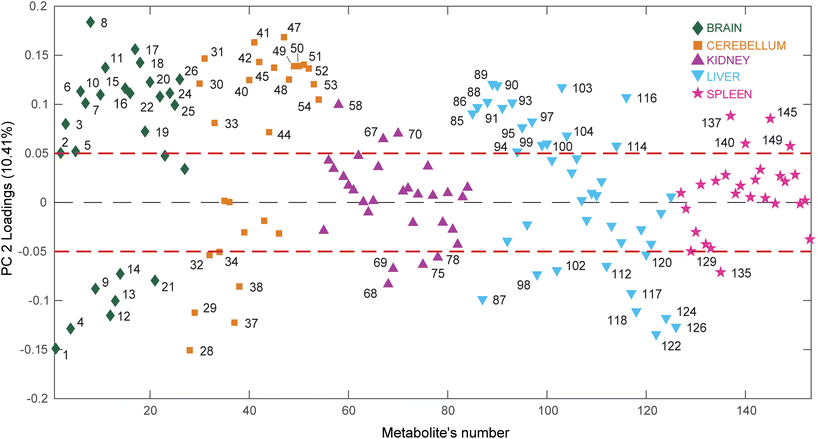 |
| Fig. 5 PC2 loadings plot of the PCA model calculated using all organ extracts analyzed by GC-MS. The dashed red lines represent an arbitrary threshold set at loading values of ±0.05 to easily identify the metabolites responsible for the sample's separations observed along PC2 in the scores plot. Keys: Brain (diamonds, green); Cerebellum (squares, orange); Kidney (triangles, purple); Liver (upside down triangles, light blue); Spleen (stars, pink). | |
This time the plot was built reporting the loading values (y axis) for each metabolite (x axis), where the metabolites belonging to different organs are depicted using different colors, to easily evaluate which organ has the most considerable number of metabolites having high (absolute value) PC2 loading values (loadings value threshold set at ±0.05). The list of the most relevant metabolites is reported in Table 1.
Table 1 List of the metabolites, and their relative identification number, characterized by PC2 loadings values higher than ±0.05. Gray and white cells contain metabolites whose levels are, respectively, increased and decreased in the OMN group compared to the CTRL and PH groups
Interestingly, the organs most affected by the Omniscan treatment are the brain, cerebellum and liver, since they contain a remarkable number of metabolites characterized by large loading values. In particular, when mice are treated with Omniscan, brain (green diamonds) turns out to be characterized by higher levels of amino acids (serine, threonine, aspartic acid, tyrosine, leucine, valine, glutamic acid, alanine, 4-aminobutanoic acid), sugar alcohols (scyllo-inositol, myo-inositol), small organic acids (3-hydroxybutyric, malic, glyceric) as well as uracil and urea. At the same time, the brain is characterized by lower levels of phenylalanine, glycine, ethanolamine and organic acids such as picolinic, succinic, oxalic and lactic acid. The cerebellum (orange square) shows a metabolomic impairment very similar to that of the brain having high levels of amino acids (serine, threonine, aspartic acid, tyrosine, glutamic acid, glycine, phenylalanine, alanine, 4-aminobutanoic acid), sugar alcohols (myo-inositol, mannitol), small organic acids (citric, pipecolic, malic) as well as uridine, urea and 2-pyrrolidinone; and low levels of organic acids such as picolinic, succinic, oxalic, lactic acid, and glycolic acids. The third organ most perturbed by the Omniscan administration is the liver (cyan triangles). Indeed, the hepatic metabolome of the OMN group shows higher levels of amino acids (glycine, alanine, 2-aminobutanoic acid, valine, proline, isoleucine), organic acids (glycolic, oxalic, benzoic, fumaric, malonic, 3-methylbutanoic), nucleobases (uracil and adenine), as well as ethanolamine and hypoxantine. The two least perturbed organs are the kidney and the spleen. In fact, for the kidney, only 7 metabolites out of 30 are characterized by loading values outside the ±0.05 limits, while for the spleen only 5 metabolites out of 27 exceed the thresholds. In particular, the renal metabolome of the OMN group is enriched with propanoic and glutamic acids, and erytrithol, while it presents lower levels of lactic and aspartic acids, as well as threonine and 5-oxoproline compared to the PH and CTRL groups. At the same time the splenic metabolome (magenta stars) turns out to be enriched of fumaric, ascorbic, and glutamic acids and urea while it was characterized by lower levels of glycine with respect to the other groups.
4. Discussion
According to a recent survey, a total of about 10 million doses of gadolinium-based contrast agents (GBCAs) are administered annually.1 Nevertheless, during the past decades, warnings about potential harmful effects from the use of linear GBCAs ascribable to the release of free gadolinium cations have been raised.30,31 In particular, there are findings proving that multiple administrations of linear GBCAs, are responsible for a far higher retention of gadolinium in the brain of healthy patients with respect to macrocyclic ones.32–34 Although the risk of adverse effects due to gadolinium deposition was not proven, in 2017 the European Medicines Agency's recommended the removal of linear GBCAs from the market,35 while, in the same year, the US Food and Drug Administration announced that they would not restrict the use of linear GBCAs and suggested that the type of GBCA used should be carefully selected in high-risk patients (pregnant women, children, and patients with inflammatory conditions).36 Probably, these different rules about the use of linear GBCAs in Europe and America are also due to the lack of clear information about the actual toxic effects related to Gd retention.37 Thus, the aim of our study was to add a piece to this puzzle by obtaining, for the very first time, a comprehensive in vivo assessment of the metabolic effects induced by the administration of both a linear (Omniscan, gadodiamide,) and a macrocyclic (ProHance, gadoteridol) GBCA compared to a control group (Fig. 1). In particular, by employing a multi-organ metabolomics approach and state-of-the-art data analysis we aimed at (i) evaluating the global alterations of the mice physiological state induced by the treatment with different GBCAs, and (ii) understand at which level (plasma, brain, cerebellum, kidney, liver, and spleen) they occur.
The difference in gadolinium retention extent upon the administration of Omniscan and ProHance, being much more pronounced (13–20 fold) for the less stable Omniscan, was taken as a state-of-the-art knowledge38–40 and the concentration of retained gadolinium in the investigated organs was not determined in the present study. This decision was made in order to preserve the entire organs for the metabolomics studies and to avoid the use of additional mice.
Moreover, all the studies reported so far dealing with the assessment of the chemical form of retained gadolinium clearly demonstrated that less stable linear GBCAs undergo dissociation in vivo while the macrocyclic complexes are recovered as intact complexes14,41–43, apart from bones, where, even in the case of macrocyclic complexes, retained gadolinium was mostly present in its dechelated form.44
The multivariate analysis of plasma data highlighted significant lower levels of pyruvate, lactate, formate and alanine in the OMN group compared to the PH one. This observation suggested the presence of a dysregulation in carbohydrate and energetic metabolism caused by the administration of the linear contrast agent (gadodiamide). However, it should be noted that plasma analysis alone cannot be considered exhaustive enough to understand the alteration of the physiological state of the mouse model. For this reason, we also carried out a multi-organ metabolomic study to obtain a global picture of the effects caused by the selected GBCAs.
The multivariate analysis of GC-MS data acquired from five selected organs (brain, cerebellum, kidney, liver, and spleen) revealed a clear difference in the global metabolome of the OMN group compared to the PH and to the control groups (Fig. 4). In particular, the administration of the linear contrast agent (Omniscan) perturbs the metabolic homeostasis of the mice, while the use of ProHance, seems to not cause any significant alteration of the metabolome. Interestingly, the organs most altered by the Omniscan treatment turned out to be the brain, cerebellum, and liver. These data are consistent with available data about gadolinium retention in the brain34 and with the hepatocytes alterations found in a mouse model treated with repeated doses of Omniscan.45
In general, the analysis of the metabolome of all organs suggests that the treatment with Omniscan causes an up-regulation of the energetic pathways (such as glycolysis, Kreb's cycle, fatty acids beta oxidation and gluconeogenesis), as well as a dysregulation of the amino acids and nucleotide metabolism in the brain, cerebellum and liver. These considerations were made by taking into account the concentration of the discriminating metabolites, thus they are based on quantitative results.
The up-regulation of glycolysis was detectable from the reduced hepatic glucose level and the increased levels of 3-hydroxubutyric acid (3-HB) in the brain. In fact, 3-HB is an early marker for impaired glucose regulation since it is produced in the liver in case of low levels of glucose and then it is transferred to the brain that employs it as energy source. A decrease of the glucose levels also up-regulates gluconeogenesis, which is able to synthesizes glucose from non-sugar precursors, such as lactate and pyruvate. This agrees with the low levels of these precursors found both in liver and kidney (organs able to perform gluconeogenesis) as well as in the plasma of the gadodiamide-treated group. Overall, these observations suggest that the administration of this contrast agent may induce an increase of the energy need and, thus, a lack of energy sources. This can be also deduced by the alteration of the main Kreb's cycle intermediates (citrate, succinate, fumarate, and malate) which is visible in brain, cerebellum, liver and spleen of the gadodiamide-treated group.
Upregulation of the energetic pathways have been associated to the insurgence of fibrotic conditions due to the extra-energy need of fibroblasts to fuel the enhanced synthesis, deposition and remodelling of ECM which characterize the fibrotic condition.46 Pathological cells in fibrosis (fibroblasts, epithelial cells, immune cells and others) are subjected to metabolic adaptations or reprogramming to enable their proliferative and synthetic activities. For example, aerobic glycolysis takes place in radiation-induced skin fibrosis,47 renal fibrosis,48 and pulmonary fibrosis.49
Amino acid dysregulation was also observed. In particular, an increase of the hepatic glycine, alanine, valine, proline, and isoleucine levels suggested an alteration of the protein degradation or synthesis. These changes in amino acids were accompanied by increases in adenine and uracil in liver suggesting also an increased nucleic acid catabolism. These findings are in line with a previous metabolomics-based study carried out by administering gadolinium chloride (GdCl3), and not GBCAs, to rats,50 which suggest that the effect induced by the Gadodiamide administration could probably be related to the fraction of free gadolinium released by the linear chelate. As for glucose metabolism, also studies linking amino acid metabolism and fibrosis have been reported. In particular, as proline and glycine are the main constituents of collagen, their dysregulated metabolism is gaining interest, particularly in the fields of pulmonary51 and liver52 fibrosis.
The very high content of urea in the brain (green diamond no. 8 in Fig. 5) of mice treated with gadodiamide is of particular interest. In recent years, diffused elevations in brain urea have been reported in certain types of age-related neurodegenerative diseases, i.e., Alzheimer's disease (AD),53 Huntington's disease (HD)54,55 and Parkinson disease (PD).56 Urea cycle activity localizes primarily in the liver, but systemic over-production of urea is not known in AD and HD. These findings have been correlated to impaired local urea regulation in brain, by up-regulation of synthesis and/or defective clearance. Interestingly, also in our animal model liver urea values are not distinguishing OMN group from PH and CTRL groups.
In this study, a multi-organ and plasma metabolomic approach was used for the first time to provide a comprehensive assessment of the metabolic effects induced by the administration of both a linear (Omniscan, gadodiamide) and a macrocyclic (ProHance, gadoteridol) GBCA compared to a control group. Multivariate data analysis revealed that plasma metabolome was not differently perturbed by the two investigated GBCAs. On the contrary, the multiorgan metabolomics analysis displayed a clear difference between the Omniscan and the ProHance groups, suggesting that treatment with Omniscan actually perturbs the physiological state of the mouse model. Interestingly, the biochemical alteration induced by Omniscan seems to be organ-related since the strongest perturbations were found mainly in brain, cerebellum and liver. We speculate that the observed biochemical alteration could be ascribed to the higher amount of released gadolinium from the Omniscan chelate.
Overall, the results of this study clarify, for the first time, the toxic effects related to the use of a linear GBCA vs. macrocyclic one, by identifying the organs most affected by the administration of the investigated chelates. Thus, this work paves the way both to a safest use of the commercially available GBCAs and to the development of new GBCAs characterized by lower general toxicity.
5. Conclusions
It has been estimated that about 40–50% of the current clinical MRI scans are contrast-enhanced. The high stability of the Gd-complexes used as MR-contrast agents should ensure their complete excretion without side effects for patients. However, if from one side, it has been shown that small amounts of Gd3+ are retained in tissues of patients which received during their life multiple administrations of GBCAs, studies aimed at detecting possible metabolic perturbations and/or potential adverse health effects due to long-term gadolinium deposition are still lacking. In this work, a metabolomic study was carried out to provide, for the first time, a comprehensive assessment of the metabolic effects induced by the multiple administration of one linear chelate (less stable, which releases higher amounts of Gd3+) and one macrocyclic chelate (more stable, which releases very low amounts of Gd3+) in comparison with a control group in a mice model, with a view to investigating possible alterations of metabolic cellular processes in the context of tissues Gadolinium retention.
Indeed, the multi-organ analysis revealed that while the effect of the macrocyclic Gd-chelate administration does not significantly affect the mice metabolome, treatment with the linear one perturbs the physiological state of the mouse model. Interestingly, the observed biochemical alterations seemed to be organ-related since the strongest perturbations were found mainly in brain, cerebellum and liver, where an up-regulation of the energetic pathways, as well as a dysregulation of the amino acids and nucleotide metabolism was observed. The results of this study shed light on the potential toxic effects related to Gd retention and suggest that the metabolomics approach can be considered as a valid additional tool for the evaluation of the toxicity of the investigated GBCAs.
Author contributions
Conceptualization, E. G., N. I.; methodology, N. I., E. G.; formal analysis, N. I., F. R., G. R., N. C., S. D. T., F. S.; investigation, E. D. G., F. R., G. R., C. F., A. D. P.; resources, A. R., E. G., and N. I.; data curation, N. I., F. R., G. R., N. C., and F. S.; writing—original draft preparation, F. R., G. R., E. D. G., N. I., N. C., E. G., and C. F.; writing—review and editing, A. R., S. D. T., E. G., N. I., A. D. P., and C. F.; supervision, E. G., N. I. All authors have read and agreed to the published version of the manuscript.
Conflicts of interest
There are no conflicts to declare.
References
- C. Do, J. DeAguero, A. Brearley, X. Trejo, T. Howard, G. P. Escobar and B. Wagner, Kidney, 2020, 1, 561–568 CrossRef PubMed.
- M. A. Sieber, H. Pietsch, J. Walter, W. Haider, T. Frenzel and H. J. Weinmann, Invest. Radiol., 2008, 43, 65–75 CrossRef PubMed.
- E. Tedeschi, F. Caranci, F. Giordano, V. Angelini, S. Cocozza and A. Brunetti, Radiol. Med., 2017, 122, 589–600 CrossRef PubMed.
- C. Robic, M. Port, O. Rousseaux, S. Louguet, N. Fretellier, S. Catoen, C. Factor, S. Le Greneur, C. Medina, P. Bourrinet, I. Raynal, J. M. Idée and C. Corot, Invest. Radiol., 2019, 54, 475–484 CrossRef CAS PubMed.
- E. Gianolio, E. Di Gregorio and S. Aime, Eur. J. Inorg. Chem., 2019, 2019, 137–151 CrossRef CAS.
- Y. Errante, V. Cirimele, C. A. Mallio, V. Di Lazzaro, B. B. Zobel and C. C. Quattrocchi, Invest. Radiol., 2014, 49, 685–690 CrossRef CAS PubMed.
- C. C. Quattrocchi, C. A. Mallio, Y. Errante, V. Cirimele, L. Carideo, A. Ax and B. B. Zobel, Invest. Radiol., 2015, 50, 470–472 CrossRef PubMed.
- G. Jost, T. Frenzel, J. Lohrke, D. C. Lenhard, S. Naganawa and H. Pietsch, Eur. Radiol., 2017, 27, 2877–2885 CrossRef PubMed.
- Y. Cao, D. Q. Huang, G. Shih and M. R. Prince, Am. J. Roentgenol., 2016, 206, 414–419 CrossRef PubMed.
- R.-E. Yoo, C.-H. Sohn, K. M. Kang, T. J. Yun, S. H. Choi, J. Kim and S.-W. Park, Invest. Radiol., 2018, 53, 20–25 CrossRef CAS PubMed.
- D. A. Stojanov, A. Aracki-Trenkic, S. Vojinovic, D. Benedeto-Stojanov and S. Ljubisavljevic, Eur. Radiol., 2016, 26, 807–815 CrossRef PubMed.
- A. Splendiani, M. Perri, C. Marsecano, V. Vellucci, G. Michelini, A. Barile and E. Di Cesare, Radiol. Med., 2018, 123, 125–134 CrossRef PubMed.
- P. Kelemen, J. Alaoui, D. Sieron, A. Chan, C. P. Kamm, M. R. Heldner, J. Gralla, R. Wiest and R. K. Verma, Sci. Rep., 2018, 8, 16844 CrossRef PubMed.
- I. Strzeminska, C. Factor, P. Robert, A. L. Grindel, P. O. Comby, J. Szpunar, C. Corot and R. Lobinski, Invest. Radiol., 2020, 55, 138–143 CrossRef CAS PubMed.
- D. El Hamrani, V. Vives, R. Buchholz, W. Même, C. Factor, S. Fingerhut, M. Sperling, U. Karst, P. Robert and S. Même, Invest. Radiol., 2020, 55, 120–128 CrossRef CAS PubMed.
- D. Ryan and K. Robards, Anal. Chem., 2006, 78, 7954–7958 CrossRef CAS PubMed.
- M. Deidda, C. Piras, P. P. Bassareo, C. Cadeddu Dessalvi and G. Mercuro, IJC Metab. Endocr., 2015, 9, 31–38 CrossRef.
- A. Vignoli, V. Ghini, G. Meoni, C. Licari, P. G. Takis, L. Tenori, P. Turano and C. Luchinat, Angew. Chem., Int. Ed., 2019, 58, 968–994 CrossRef CAS PubMed.
- Y. Li and X. Zhao, Anal. Methods, 2020, 12, 1995–2001 RSC.
- W. Qiao-feng, G. Ling-ling, Y. Shu-guang, Z. Qi, L. Sheng-feng, Z. Fang, Y. Hai-yan, T. Yong and Y. Xian-zhong, Exp. Gerontol., 2011, 46, 787–793 CrossRef PubMed.
- N. Iaccarino, J. Amato, B. Pagano, A. Di Porzio, M. Micucci, L. Bolelli, R. Aldini, E. Novellino, R. Budriesi and A. Randazzo, J. Enzyme Inhib. Med. Chem., 2019, 34, 1041–1050 CrossRef CAS PubMed.
- B. Khakimov, M. S. Motawia, S. Bak and S. B. Engelsen, Anal. Bioanal. Chem., 2013, 405, 9193–9205 CrossRef CAS PubMed.
- L. W. Sumner, A. Amberg, D. Barrett, M. H. Beale, R. Beger, C. A. Daykin, T. W.-M. Fan, O. Fiehn, R. Goodacre, J. L. Griffin, T. Hankemeier, N. Hardy, J. Harnly, R. Higashi, J. Kopka, A. N. Lane, J. C. Lindon, P. Marriott, A. W. Nicholls, M. D. Reily, J. J. Thaden and M. R. Viant, Metabolomics, 2007, 3, 211–221 CrossRef CAS PubMed.
- F. Savorani, G. Tomasi and S. B. Engelsen, J. Magn. Reson., 2010, 202, 190–202 CrossRef CAS PubMed.
- J. Jaumot, A. de Juan and R. Tauler, Chemom. Intell. Lab. Syst., 2015, 140, 1–12 CrossRef CAS.
- N. Cavallini, F. Savorani, R. Bro and M. Cocchi, Molecules, 2021, 26, 1472 CrossRef CAS PubMed.
- H. Hotelling, J. Educ. Psychol., 1933, 24, 417–441 Search PubMed.
-
A. K. Smilde and I. Van Mechelen, in Data Handling in Science and Technology, 2019, vol. 31, pp. 27–50 Search PubMed.
- R. A. van den Berg, H. C. J. Hoefsloot, J. A. Westerhuis, A. K. Smilde and M. J. van der Werf, BMC Genomics, 2006, 7, 142 CrossRef PubMed.
- K. A. Layne, P. I. Dargan, J. R. H. Archer and D. M. Wood, Br. J. Clin. Pharmacol., 2018, 84, 2522–2534 CrossRef CAS PubMed.
- L. Blomqvist, G. F. Nordberg, V. M. Nurchi and J. O. Aaseth, Biomolecules, 2022, 12, 742 CrossRef CAS PubMed.
- G. Jost, D. C. Lenhard, M. A. Sieber, J. Lohrke, T. Frenzel and H. Pietsch, Invest. Radiol., 2016, 51, 83–89 CrossRef CAS PubMed.
- P. Robert, S. Lehericy, S. Grand, X. Violas, N. Fretellier, J.-M. Idée, S. Ballet and C. Corot, Invest. Radiol., 2015, 50, 473–480 CrossRef CAS PubMed.
- T. Kanda, H. Oba, K. Toyoda, K. Kitajima and S. Furui, Jpn. J. Radiol., 2016, 34, 3–9 CrossRef CAS PubMed.
- PRAC concludes assessment of gadolinium agents used in body scans and recommends regulatory actions, including suspension for some marketing authorisations, https://www.ema.europa.eu/ema/index.jsp?curl=pages/news_and_events/news/2017/03/news_detail_002708.jsp&mid=WC0b01ac058004d5c1, (accessed 15 December 2022).
- FDA Drug Safety Communication: FDA warns that gadolinium-based contrast agents (GBCAs) are retained in the body; requires new class warnings, https://www.fda.gov/drugs/drug-safety-and-availability/fda-drug-safety-communication-fda-warns-gadolinium-based-contrast-agents-gbcas-are-retained-body, (accessed 1 December 2022).
- T. Kanda, Magn. Reson. Med. Sci., 2019, 18, 1–3 CrossRef CAS PubMed.
- J. Lohrke, A.-L. Frisk, T. Frenzel, L. Schöckel, M. Rosenbruch, G. Jost, D. C. Lenhard, M. A. Sieber, V. Nischwitz, A. Küppers and H. Pietsch, Invest. Radiol., 2017, 52, 324–333 CrossRef CAS PubMed.
- G. Jost, T. Frenzel, J. Boyken, J. Lohrke, V. Nischwitz and H. Pietsch, Radiology, 2019, 290, 340–348 CrossRef PubMed.
- E. Di Gregorio, R. Iani, G. Ferrauto, R. Nuzzi, S. Aime and E. Gianolio, J. Trace Elem. Med. Biol., 2018, 48, 239–245 CrossRef CAS PubMed.
- E. Gianolio, P. Bardini, F. Arena, R. Stefania, E. Di Gregorio, R. Iani and S. Aime, Radiology, 2017, 285, 839–849 CrossRef PubMed.
- P. Robert, S. Fingerhut, C. Factor, V. Vives, J. Letien, M. Sperling, M. Rasschaert, R. Santus, S. Ballet, J.-M. Idée, C. Corot and U. Karst, Radiology, 2018, 288, 424–433 CrossRef PubMed.
- P. Bücker, S. K. I. Funke, C. Factor, M. Rasschaert, P. Robert, M. Sperling and U. Karst, Metallomics, 2022, 14, mfac004 CrossRef PubMed.
- L. Schlatt, A. Köhrer, C. Factor, P. Robert, M. Rasschaert, M. Sperling and U. Karst, Anal. Chem., 2021, 93, 11398–11405 CrossRef CAS PubMed.
- T. Mercantepe, L. Tümkaya, F. B. Çeliker, Z. Topal Suzan, S. Çinar, K. Akyildiz, F. Mercantepe and A. Yilmaz, J. Magn. Reson. Imaging, 2018, 48, 1367–1374 CrossRef PubMed.
- C. Y. Ung, A. Onoufriadis, M. Parsons, J. A. McGrath and T. J. Shaw, Int. J. Biochem. Cell Biol., 2021, 139, 106073 CrossRef CAS PubMed.
- X. Zhao, P. Psarianos, L. S. Ghoraie, K. Yip, D. Goldstein, R. Gilbert, I. Witterick, H. Pang, A. Hussain, J. H. Lee, J. Williams, S. V. Bratman, L. Ailles, B. Haibe-Kains and F.-F. Liu, Nat. Metab., 2019, 1, 147–157 CrossRef CAS PubMed.
- X.-N. Yin, J. Wang, L.-F. Cui and W.-X. Fan, Eur. Rev. Med. Pharmacol. Sci., 2018, 22, 4243–4251 Search PubMed.
- N. Xie, Z. Tan, S. Banerjee, H. Cui, J. Ge, R.-M. Liu, K. Bernard, V. J. Thannickal and G. Liu, Am. J. Respir. Crit. Care Med., 2015, 192, 1462–1474 CrossRef CAS PubMed.
- P. Liao, L. Wei, H. Wu, W. Li, Y. Wu, X. Li, J. Ni and F. Pei, J. Rare Earths, 2009, 27, 280–287 CrossRef.
- M. T. Gaugg, A. Engler, L. Bregy, Y. Nussbaumer-Ochsner, L. Eiffert, T. Bruderer, R. Zenobi, P. Sinues and M. Kohler, Respirology, 2019, 24, 437–444 CrossRef PubMed.
- T. Hasegawa, C. Iino, T. Endo, K. Mikami, M. Kimura, N. Sawada, S. Nakaji and S. Fukuda, Nutrients, 2020, 12, 1450 CrossRef CAS PubMed.
- J. Xu, P. Begley, S. J. Church, S. Patassini, K. A. Hollywood, M. Jüllig, M. A. Curtis, H. J. Waldvogel, R. L. M. Faull, R. D. Unwin and G. J. S. Cooper, Biochim. Biophys. Acta, Mol. Basis Dis., 2016, 1862, 1084–1092 CrossRef CAS PubMed.
- S. Patassini, P. Begley, S. J. Reid, J. Xu, S. J. Church, M. Curtis, M. Dragunow, H. J. Waldvogel, R. D. Unwin, R. G. Snell, R. L. M. Faull and G. J. S. Cooper, Biochem. Biophys. Res. Commun., 2015, 468, 161–166 CrossRef CAS PubMed.
- R. R. Handley, S. J. Reid, R. Brauning, P. Maclean, E. R. Mears, I. Fourie, S. Patassini, G. J. S. Cooper, S. R. Rudiger, C. J. McLaughlan, P. J. Verma, J. F. Gusella, M. E. MacDonald, H. J. Waldvogel, C. S. Bawden, R. L. M. Faull and R. G. Snell, Proc. Natl. Acad. Sci., 2017, 114, E11293–E11302 CrossRef CAS PubMed.
- M. Scholefield, S. J. Church, J. Xu, S. Patassini, F. Roncaroli, N. M. Hooper, R. D. Unwin and G. J. S. Cooper, Front. Mol. Neurosci., 2021, 14 DOI:10.3389/fnmol.2021.711396.
|
This journal is © The Royal Society of Chemistry 2023 |
Click here to see how this site uses Cookies. View our privacy policy here.