DOI:
10.1039/D3AN00080J
(Paper)
Analyst, 2023,
148, 3466-3475
Optimizing single cell proteomics using trapped ion mobility spectrometry for label-free experiments†
Received
14th January 2023
, Accepted 10th March 2023
First published on 3rd July 2023
Abstract
Although single cell RNA-seq has had a tremendous impact on biological research, a corresponding technology for unbiased mass spectrometric analysis of single cells has only recently become available. Significant technological breakthroughs including miniaturized sample handling have enabled proteome profiling of single cells. Furthermore, trapped ion mobility spectrometry (TIMS) in combination with parallel accumulation-serial fragmentation operated in data-dependent acquisition mode (DDA-PASEF) allowed improved proteome coverage from low-input samples. It has been demonstrated that modulating the ion flux in TIMS affects the overall performance of proteome profiling. However, the effect of TIMS settings on the analysis of low-input samples has been less investigated. Thus, we sought to optimize the conditions of TIMS with regard to ion accumulation/ramp times and ion mobility range for low-input samples. We observed that an ion accumulation time of 180 ms and monitoring a narrower ion mobility range from 0.7 to 1.3 V s cm−2 resulted in a substantial gain in the depth of proteome coverage and in detecting proteins with low abundance. We used these optimized conditions for proteome profiling of sorted human primary T cells, which yielded an average of 365, 804, 1116, and 1651 proteins from single, five, ten, and forty T cells, respectively. Notably, we demonstrated that the depth of proteome coverage from a low number of cells was sufficient to delineate several essential metabolic pathways and the T cell receptor signaling pathway. Finally, we showed the feasibility of detecting post-translational modifications including phosphorylation and acetylation from single cells. We believe that such an approach could be applied to label-free analysis of single cells obtained from clinically relevant samples.
Introduction
Single cell RNA-sequencing has become routine and is already being applied to solve a variety of biomedical research problems. However, a corresponding technology for unbiased proteome analysis of single cells has recently been introduced in the field of proteomics.1,2 Recently, several technological breakthroughs have been achieved to overcome the current limitations of analytical sensitivity and throughput of single cell proteomics.3,4 There have been significant improvements in sample preparation of low-input samples using miniaturized devices and microfluidic approaches to minimize the loss of analytes. The nanoPOTS (Nanodroplet Processing in One pot for Trace Samples) platform has enabled isolation, lysis and digestion of cells in nanoliter volumes of liquids to minimize sample losses and enable faster reaction kinetics.5 Microfluidic devices have been developed for processing samples in confined spaces such as oil,6,7 integrated proteomics chips (iProChip)8 and digital microfluidic devices.9,10 At the same time, there have been efforts to increase ion signals using carrier proteins in tandem mass tag (TMT) labeling approaches such as SCoPE-MS11,12 and BASIL strategies,13,14 which also provide increased throughput by multiplexing samples.
In addition, a substantial improvement in analyzing single cells has been achieved by coupling ion mobility spectrometry which modulates the ion flux introduced into the mass analyzer. For example, high field asymmetric ion mobility spectrometry (FAIMS) coupled with an orbitrap-based mass spectrometer improved signal-to-noise ratios of low-input samples through the removal of singly charged ions.15,16 Trapped ion mobility spectrometry (TIMS) coupled with Q-TOF mass spectroscopy showed improved sensitivity through synchronizing mobility separation with quadrupole mass selection using a novel data acquisition scheme termed as parallel accumulation-serial fragmentation (PASEF).17 More recently, a timsTOF SCP mass spectrometer has been introduced for the analysis of low-input samples detecting >550 proteins from ∼1 ng of HeLa lysate in data-dependent acquisition (DDA) mode.18 The authors further demonstrated that data-independent acquisition (DIA) mode on the timsTOF SCP instrument combined with low flow rate chromatography resulted in >3000 proteins from the same amount of sample. The improved sensitivity of this mass spectrometer was facilitated by a brighter ion source that could draw more ions into the analyzer. However, the effect of TIMS settings on the analysis of low-input samples has not been systematically evaluated.
Therefore, we carried out the optimization of TIMS parameters for DDA experiments. First, we varied the ramp time from 50 to 200 ms while keeping the duty cycle at 100% to use the same accumulation time. Primary T cells collected from blood were lysed and digested to prepare serially diluted peptide samples ranging from10 ng to 156 pg. We found that a ramp time of 180 ms was optimal for most sample input amounts, providing identification of low-abundance peptides. Next, based on the observation that there are more ions in certain regions of ion mobility space, we narrowed the ion mobility range to 0.7 to 1.3 V s cm−2, which resulted in 20% additional protein identification and an increase in protein sequence coverage compared to the conventional setting.
We next performed unbiased proteome profiling of single and a low number of primary T cells sorted using a picoliter dispensing device equipped with a piezoelectric dispensing capillary. One, five, ten and forty cells were collected in triplicate and analyzed under an optimized TIMS setting, which resulted in, on average, 365, 804, 1116, and 1651 proteins identified from single, five, ten, and forty T cells, respectively. Notably, the depth of proteome coverage in these experiments was sufficient to explain the essential metabolic pathways such as glycolysis. Additionally, several key molecules involved in the TCR signaling pathway were detected from low numbers of T cells. Importantly, identification of hundreds of phosphorylated, lysine acetylated and protein N-terminal acetylated peptides confirmed the feasibility to study post-translational modifications on single cells. To the best of our knowledge, this study is the first to deploy single cell proteomics for proteome profiling of primary T cells and shows the feasibility of adopting such an approach to understand the biology of T cells at single cell resolution.
Results and discussion
Optimization of ion ramp time in TIMS for low-input samples
A timsTOF instrument is a Q-TOF mass spectrometer equipped with a dual TIMS analyzer, which allows for parallel processing of ion accumulation and separation. Ions are collected in the first TIMS regions over a specific time period (accumulation time). In the second region, the ions are eluted by decreasing the voltage gradient over a time (ramp time). Ions with low mobility (larger collisional cross section, CCS) are eluted first followed by more mobile ions (smaller CCS). Accumulation and ramp times can be specified typically within 25 to 200 ms when analyzing peptide ions. Because accumulation and release of ions occur in spatially separated regions in parallel, it enables operation at a duty cycle that is ∼100% if the same accumulation and ramp times are used. It has been reported in a previous study using the first generation timsTOF mass spectrometer that times for accumulating and separating ions in TIMS are related to the number of features that are selected for fragmentation, which affects the sensitivity in peptide identification.17 We hypothesized that modulating the ion flux in TIMS largely affects the overall performance, especially for analyzing low-input samples. Therefore, we first evaluated the effect of ion accumulation and ramp time on identifying peptides and proteins of low-input samples. To accomplish this, we prepared peptide samples of primary T cells isolated from blood, and peptides were serially diluted from 10 ng to 156 pg. Each sample was analyzed through DDA-PASEF using different ramp times of 50, 100, 150, 180, or 200 ms while keeping the duty cycle as 100% by using the same accumulation times. As we used the 10 PASEF scan method, one cycle includes one MS1 scan and 10 PASEF MS2 scans. The cycle time is calculated based on the number of PASEF scans and ramp times. At 50, 100, 150, 180, and 200 ms ramp times, the cycle time is 0.62, 1.17, 1.72, 2.05 and 2.27 seconds, respectively (Fig. 1A). The raw mass spectrometry data were analyzed using MSFragger in the FragPipe suite for peptide and protein identification.19
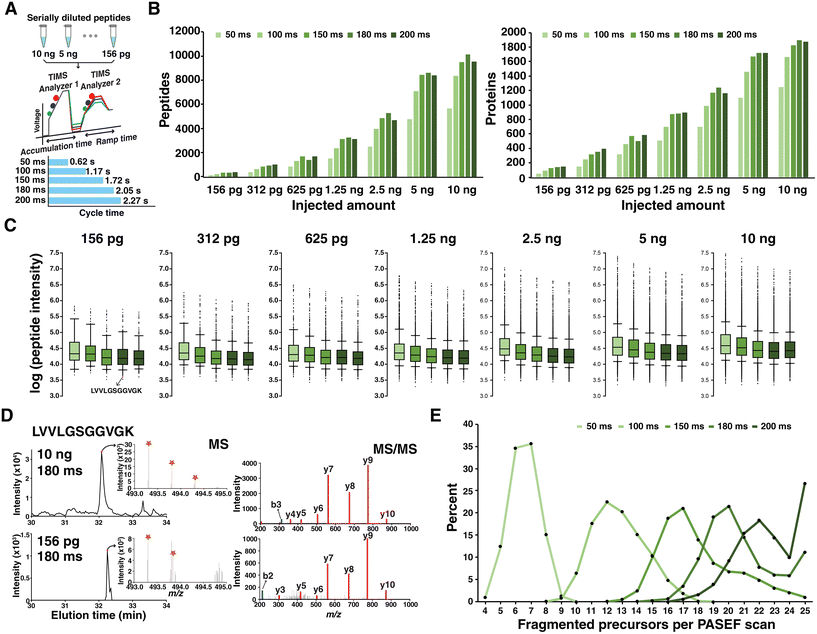 |
| Fig. 1 Optimization of the ramp time of TIMS for low-input samples. (A) Schematic of the experimental workflow using serially diluted peptides (i.e., 156 pg, 312 pg, 625 pg, 1.25 ng, 2.5 ng, 5 ng and 10 ng) from primary T cells. Peptides were analyzed using a timsTOF SCP mass spectrometer equipped with dual TIMS where ion accumulation and separation occur. Serially diluted peptides were injected at different ramp times (i.e., 50, 100, 150, 180, and 200 ms). Corresponding cycle times are depicted. (B) Bar charts of identified peptides and proteins at different ramp times. (C) Distributions of peptide intensities under different conditions. (D) A representative example of a peptide (LVVLGSGGVGK) with low abundance that was identified by applying a longer ramp time of 180 ms. The extracted ion chromatogram, MS1 spectrum and annotated MS/MS spectrum are shown for 10 ng and 156 pg samples. (E) Percent of selected precursors for fragmentation per PASEF scan at different TIMS ramp times. Data of the run injecting 2.5 ng peptides are shown. | |
The number of identified peptides and proteins at 100 ms was substantially lower than those under other conditions. The longer accumulation and ramp times more than 150 ms yielded similar numbers of peptides and proteins. The condition of 180 ms from several samples of loading 312 pg, 2.5 ng and 10 ng of peptides showed a slightly higher number of identifications than other conditions (Fig. 1B). When comparing the intensities of the identified peptides, conditions of 150, 180, and 200 ms slightly shifted to a lower value, which indicates that accumulating and separating for longer times provide a chance to sequence peptides of low abundance (Fig. 1C). One representative example is the peptide LVVLGSGGVGK which was identified in every run from 625 pg to 10 ng of loaded peptides. However, this peptide was sequenced only at ramp times of 180 and 200 ms when injecting 156 pg peptides. In the experiment where 312 pg of peptides were injected, again a short ramp time of 50 ms was not able to sequence this low abundance peptide. Although the intensity of the precursor ion of this peptide in 156 pg of sample was >30 times lower than the intensity in 10 ng of sample, the isotopic distribution was clearly interpretable. Annotated MS/MS spectra from 10 ng and 156 pg samples showing the same fragmentation pattern also strongly support the identification of this peptide (Fig. 1D).
We investigated the reason for additional identifications with longer accumulation and ramp times. The main contribution was the increased number of precursors selected for fragmentation per PASEF scan. A median of 20 precursor ions were selected for fragmentation per PASEF scan when using a ramp time of 180 ms (Fig. 1E). Although more precursor ions were subjected to fragmentation with a ramp time of 200 ms, a slightly lower number of proteins were identified compared to the condition of 180 ms. This is likely because of a longer cycle time (i.e., 2.3 s) when using 200 ms, which is not optimal under current chromatography conditions. Based on this, we decided to use 180 ms for subsequent studies described below. We should note that additional investigations could be performed by adjusting other parameters. For example, the maximum ion counts in TIMS, which is set as 2
000
000 in the current study, could be adjusted along with the changes in accumulation time to collect more ions in the TIMS analyzer. In addition, the maximum number of precursors that are fragmented per PASEF scan is locked at 25, which can also be further modified to acquire more MS/MS scans.
The effect of a narrower ion mobility range on the depth of proteome coverage
In TIMS, ion mobility is recorded as the inverse of reduced mobility (K0) which is the measured mobility at standard temperature and pressure. The ion mobility range is generally scanned from 0.6 to 1.6 V s cm−2 when analyzing peptide ions. This is the default setting for the DDA-PASEF method in the timsTOF mass spectrometer, and most experiments were performed using similar settings with minor modifications.17,20,21 Intriguingly, we observed that ion mobility was not fully exploited in a monitoring range of 0.6–1.6 V s cm−2. Most identified peptides (98%) were separated between 0.7 and 1.3 V s cm−2 in the samples for analyzing 2.5 ng of peptides with a ramp time of 180 ms (Fig. 2A). The same trends were observed for the other samples with a ramp time of 180 ms (Fig. S1†). This observation motivated us to adjust the ion mobility range to a narrower region of 0.7–1.3 V s cm−2 to fully utilize TIMS scanning of ion accumulation and separation on the populated region. The modified ion mobility range resulted in 6860 peptides corresponding to 1403 proteins from 2.5 ng of peptide sample. When compared to the result of default setting, 2505 peptides and 318 proteins were additionally identified (Fig. 2B). This included several proteins known to be elevated in T cells, such as actin-binding LIM protein 1 (ABLIM1), T cell surface glycoprotein CD4, chemokine (C–C motif) receptor 7 (CCR7), CD27 antigen and T cell receptor beta constant 2 (TRBC2). However, 217 proteins among 318 proteins were identified with a single peptide, and careful manual inspection of MS/MS spectra was performed. For example, proteins CCR7, CD27 and SATB1 were identified with one peptide whose annotated MS/MS spectra supported correct identification (Fig. S2†).
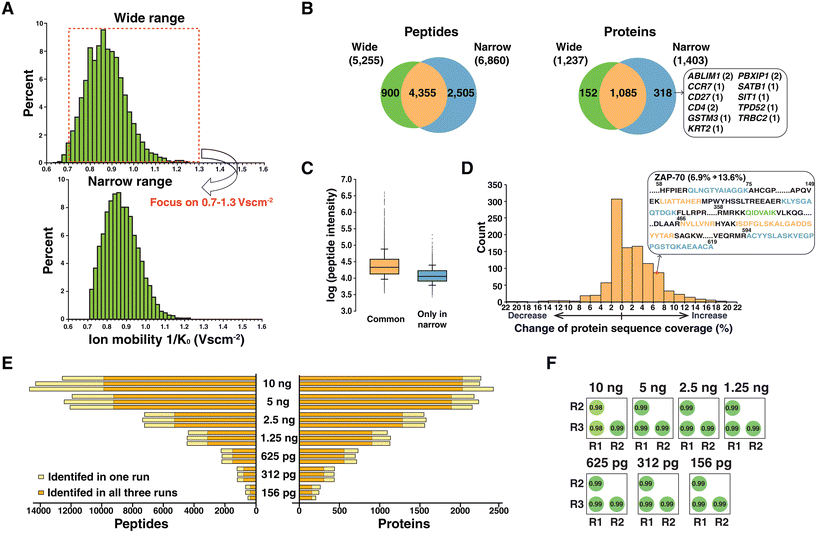 |
| Fig. 2 Effect of adjusting the ion mobility monitoring window on peptide identification to analyze low-input samples. (A) Ion mobility distributions of identified peptides from the run injecting 2.5 ng peptides with a ramp time of 180 ms. Plots with a default wide ion mobility range (0.6–1.6 V s cm−2) and a narrow ion mobility range (0.7–1.3 V s cm−2) are shown. (B) Venn diagrams showing comparison of the identified peptides and proteins from each approach. Several proteins detected only in the setting of the narrow ion mobility window are shown in a box along with the number of corresponding peptides in parentheses. (C) Box plots showing the intensities of peptides identified in both approaches (orange) and identified exclusively in the narrow ion mobility range (blue). (D) A histogram showing changes in protein sequence coverage in percent by applying a narrow ion mobility range. A representative example of protein ZAP-70 is described. Peptides shown in green, orange, and blue are peptides identified exclusively in a wide window, for both approaches, and exclusively in a narrow window, respectively. (E) Identified peptides and proteins from triplicate experiments by injecting 10 ng to 156 pg of peptides using an optimized ramp time and ion mobility range. Orange bars indicate the peptides or proteins identified in all three replicates while yellow bars indicate peptides or proteins identified only in a single run. (F) Correlation matrix showing the Pearson correlation coefficients of peptide intensities across triplicate experiments. | |
The median log-transformed intensities were 4.3 and 4.1 for peptides identified in both experiments and peptides exclusively identified in the narrower ion mobility range, respectively (Fig. 2C). This indicated that the gain through adapting a narrower ion mobility range specifically benefitted low-abundance peptide identification. We further assessed the benefit of using the narrower ion mobility range in improved protein sequence coverage for 1085 proteins identified in both approaches. Among them, 604 proteins had increased protein sequence coverage (Fig. 2D). A representative example is shown with protein zeta-chain-associated protein kinase-70 (ZAP-70), which is activated by engaging a T cell receptor with the antigen presented in the context of MHC. Following antigen engagement, ZAP-70 is phosphorylated by LCK, and subsequent binding of ZAP-70 to the CD3 zeta chain is the proximal event that initiates the TCR signalling cascade which results in T cell proliferation, activation and cytokine secretion.22 Because of these critical roles in T cell function, obtaining higher protein sequence coverage is important not only to increase the confidence of identification, but to cover important modification sites. With narrower ion mobility setting, 4 peptides were additionally identified, which increased the protein sequence coverage of ZAP-70 from 6.9 to 13.6%. In conclusion, our data imply that modulating the ion flux by changing the ion mobility range is beneficial in achieving increased sensitivity. Interestingly, a recent study using timsTOF Pro concluded that using a narrower ion mobility range outperformed a wide range,23 which is well correlated with our observation on low-input samples.
We analyzed diluted peptide samples again using the optimized settings of the ramp time and narrower ion mobility range. A substantial increase was observed both in the number of identified peptides and proteins with optimized settings. On average, 235, 432, 709, 1112, 1553, 2173 and 2332 proteins were identified from 156 pg, 312 pg, 625 pg, 1.25 ng, 2.5 ng, 5 ng and 10 ng, respectively (Fig. 2E). Among them, 87, 86, 82, 81, 78, 70, and 66% proteins were identified in all triplicate experiments. In addition, we observed robust quantification across triplicate experiments based on the correlation of the intensities of peptides (Fig. 2F). Compared to the initial results identifying only 140 proteins from 156 pg of sample (Fig. 1B), there was a significant improvement with a 67% increase in the number of protein identifications. To better understand the performance of the current mass spectrometry settings, we applied the optimal settings for proteome profiling of the HeLa protein digest standard which is widely used as a quality control sample. Serially diluted peptide samples from the HeLa protein digest standard (ranging from 10 ng to 156 pg) were analyzed, which resulted in 502 protein identifications from 156 pg HeLa lysate (Fig. S3†). We note that match-between-runs (MBR) which transfers identifications from the library generated using bulk samples or many cells was not applied in our current analysis. A primary aim of this study is to evaluate the benefit of optimal TIMS settings in sequenced peptides with MS/MS evidence. Further studies will be focused on deploying false discovery rate controlled MBR to increase the number of quantified features.24
Proteome profiling of small numbers of primary T cells
We sought to assess the depth of proteome coverage that we could achieve from single or low numbers of primary T cells using the optimized TIMS settings for low-input samples. To this end, primary T cells were isolated from human blood using density gradient separation followed by enrichment. T cells were suspended in PBS at a concentration of ∼200 cells per μl and subjected to the cellenONE platform which can isolate single cells and dispense picoliter liquids using a piezoelectric dispenser. Single cells were isolated based on the size and elongation filter to collect one, five, ten and forty cells in triplicate into individual wells of a 384-well plate (Fig. 3A). Lysis was performed using DDM (n-dodecyl-β-D-maltoside), which was demonstrated to benefit protein extraction for single cell proteomics.25 Peptides obtained from different numbers of cells after trypsin digestion were analyzed in triplicate under optimized mass spectrometry settings. An average of 2198, 4958, 6736, and 10
768 peptides corresponding to 365, 804, 1116, and 1651 proteins were identified from one, five, ten, and forty cells, respectively (Fig. 3B). Proteins identified from all three runs were 228, 503, 831, and 1308, respectively. As expected, most proteins detected from one, five and ten cells were covered from the identifications of forty cells (Fig. 3C). Overall, these results indicated reproducibility in the preparation of single cell samples and measurements using mass spectrometry, which will require further evaluation of many single cell samples.
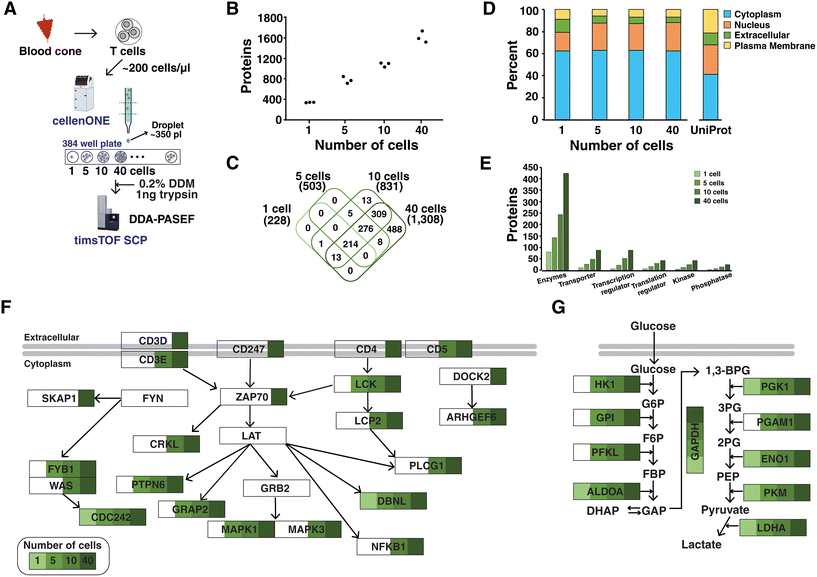 |
| Fig. 3 Proteome profiling of a low number of primary T cells. (A) Experimental workflow for cell isolation, lysis and digestion using the cellenONE platform. Peptide samples from 1, 5, 10, and 40 cells were analyzed in DDA-PASEF mode using a timsTOF SCP mass spectrometer. (B) The number of identified proteins from three runs of 1, 5, 10 and 40 cells. (C) A Venn diagram comparing proteins across different numbers of cells. Proteins identified in all three runs were used for comparison. (D) Bar charts displaying subcellular localization of proteins in each experiment. Data of UniProt denote the whole proteins in the UniProt protein database. (E) Bar charts showing the types of molecules. Coverage of proteins related to (F) the TCR signaling pathway and (G) glycolysis from different numbers of cells. | |
The proteins identified in all three runs were used for further analysis of subcellular localization through Ingenuity Pathway Analysis (IPA).26 A large proportion of proteins (60%) was from cytoplasm in every cell sample (Fig. 3D). When compared to the analysis using the whole proteins in the UniProt human database, it was found that a smaller portion of plasma membrane proteins were identified. Further investigations on protein lysis and digestion steps are required to improve the coverage of membrane proteins. In addition, functional annotation revealed that proteins identified from a low number of cells belonged to various classes of molecules such as enzymes, transporters, transcription/translation regulators, and kinase/phosphatase (Fig. 3E). Four kinases were identified (NAGK, PGK1, PKM, and NME2) in every three run of a single cell sample. In forty cells, 43 kinases were detected including important molecules related to the T cell receptor (TCR) signaling pathway such as LCK and ZAP-70. We further evaluated whether single or a low number of cells could achieve sufficient depth to describe the TCR signaling pathway (Fig. 3F). In addition, pathway enrichment analysis performed using WebGestalt27 on enzymes which were most frequently detected molecules in every sample revealed several metabolic pathways of glycolysis/gluconeogenesis, carbon metabolism and pyruvate metabolism (Fig. S4†). Notably, the depth of proteome coverage from a single cell was sufficient to explain the critical metabolic pathways including glycolysis/gluconeogenesis (Fig. 3G). However, we note that the study was performed on primary T cells with a size of ∼10 μm, which yielded ∼20 pg of proteins in the resting state.28 This could result in a lower number of identified proteins as compared to other studies using cultured cells. Further investigations will be required to increase the depth of proteome coverage even in primary cells. One promising strategy is to deploy narrow-bore packed columns operating at an ultralow flow rate of ∼20 nl min−1.29 The DIA approach using a timsTOF SCP mass spectrometer is another promising alternative strategy, which is expected to provide robust quantification across many single cell samples.
Feasibility of identifying post-translational modified peptides from single cell
Post-translational modifications expand the functional diversity of the proteome and orchestrate complex biological processes such as enzymatic activity, subcellular localization and interaction.30 Although materials from low numbers of cells are not sufficient to achieve in-depth coverage of PTMs, we assessed the feasibility of identifying PTMs from these low-input samples, focusing on phosphorylation and acetylation. To this end, phosphorylation on Ser/Thr/Tyr and acetylation on Lys and protein N-termini were considered during protein database search. In line with the results of unmodified peptides, a higher number of modified peptides were identified in a higher number of cells (Fig. 4A). In total, 27, 56, 82, and 105 phosphorylated peptides were identified from single, five, ten, and forty cells, respectively. Among them, 3, 8, 19, and 23 phosphorylated peptides (2, 7, 15, and 18 proteins) were detected in all triplicate experiments. Similarly, 16, 39, 48, and 66 lysine acetylated peptides were identified with 3, 8, 10, and 19 peptides detected in all three runs. We also detected 57, 330, 281, and 385 protein N-terminal acetylated peptides. Among them, 17, 62, 129, and 202 peptides were identified in every triplicate corresponding to 15, 45, 99, and 166 proteins.
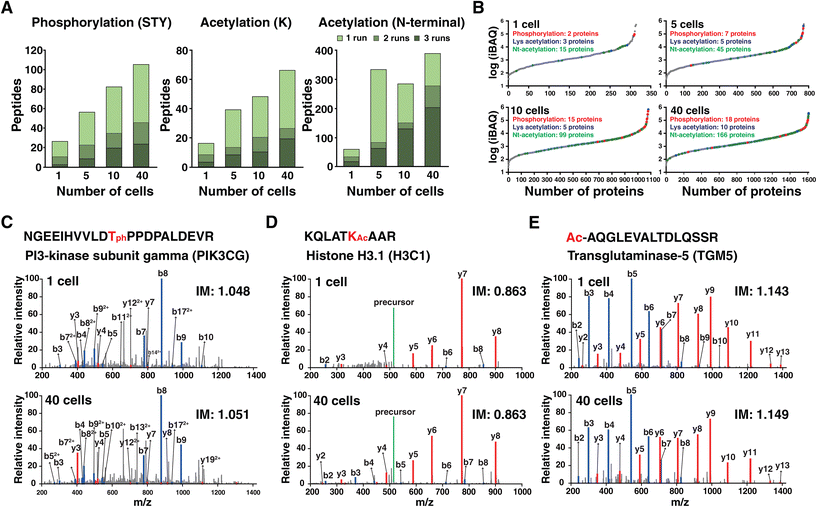 |
| Fig. 4 Identification of peptides with post-translational modifications. (A) Bar charts showing the number of peptides with PTMs (i.e., serine/threonine/tyrosine phosphorylation, lysine acetylation, and protein N-terminal acetylation) from experiments of different numbers of cells. (B) Water fall plots of protein iBAQ intensities. Proteins with phosphorylation, lysine acetylation and protein N-terminal acetylation are marked as red, blue and green dots, respectively. The representative examples of (C) phosphorylated, (D) lysine acetylated and (E) protein N-terminal acetylated peptides. Annotated MS/MS spectra are shown from one cell and forty cells. IM denotes the ion mobility value. | |
The chance of identifying PTMs is related to the natural abundance of proteins. Therefore, we estimated the abundance of proteins in cells by calculating intensity-based absolute quantification (iBAQ) values, which were obtained by dividing the measured protein intensities by the number of theoretically observable peptides for each protein (Fig. 4B).31 The iBAQ values spanned ∼4 orders of magnitude. As expected, phosphorylation and lysine acetylation were identified from the proteins of high abundance such as keratin and actin. Protein N-terminal acetylation was readily detected for the protein with lower abundance. This again indicated that the depth is not sufficient to cover PTMs with lower stoichiometry, especially phosphorylation in a single cell. Still, we could identify several interesting phosphorylation sites. A representative example is the phosphorylated peptide of phosphatidylinositol 4,5-biphosphate 3-kinase catalytic subunit gamma (PI3K-gamma), which is a leukocyte-specific lipid kinase.32 The annotated MS/MS spectrum of the phosphorylated peptides from one cell and from forty cells showed the same fragmentation pattern with the same ion mobility value, which indicates confident identification of this phosphorylated peptide from the single cell sample (Fig. 4C). The representative example of lysine acetylation from the single cell is K23 of histone H3 which is a previously reported site associated with disease such as cancer and memory impairment33 (Fig. 4D). One of the representative examples for protein N-terminal acetylation is protein TGM5 with peptide AQGLEVALTDLQSSR (Fig. 4E). Notably, most N-terminally acetylated peptides have been previously reported by our group.34
Overall, the results indicated that several PTMs of phosphorylation and acetylation from abundant proteins can be identified from single cells. However, phosphorylation of proteins other than cytoskeleton proteins became detectable when collecting 40 cells. Therefore, further studies are required to enhance the sensitivity to detect PTMs that exist with low stoichiometry. We anticipate that the chance of detecting modified peptides, especially phosphorylated peptides, will increase using the targeted approach of prm-PASEF mode.35 In addition, further studies are required to employ miniaturized PTM enrichment strategies on low-input samples.36 Finally, we envision that the chance of detecting abundant PTMs such as proline hydroxylation, arginine citrullination, lysine ubiquitylation and N-glycosylation should be evaluated on these data sets by using sophisticated search engines for analyzing PTMs.37,38
Conclusions
It is evident that injecting or accumulating more ions in a mass spectrometer increases the signal-to-noise ratio and detection sensitivity especially for low-input samples. Ion accumulation is mainly controlled by a parameter of ion injection time and automatic gain control (AGC) value in orbitrap-based instruments, which demonstrated that longer injection time for fragmentation improved the depth of proteome coverage for low-input samples.39,40 Similar to the optimization that has been performed on orbitrap-based mass spectrometers, we found optimal ion ramp times and ion mobility ranges to analyze low-input samples on a timsTOF SCP mass spectrometer. Although the primary goal of this study was to optimize TIMS settings for DDA experiments, the presented settings can also be beneficial for DIA-based studies by generating more comprehensive DDA-based spectral libraries. To our knowledge, this is the first study providing unbiased proteome profiling of single T cells. Future studies will be performed to understand the biology of T cells at a single cell level. Within a population of T cells, as many as ∼1% are specific for any given antigen, and only antigen specific T cells will respond, proliferate, activate and secrete cytokines in response to the antigen. Therefore, the ability to study changes in individual T cells that are present in low abundance is important for the study of natural or vaccine-induced immunity to a variety of pathogens such as SARS-CoV2, influenza and others.41 It is increasingly recognized that T cells are central mediators of protection against a variety of infectious diseases, immune surveillance against cancer, and as mediators of autoimmunity. We expect that single cell proteomics will allow the study of rare antigen specific T cells or rare populations of T cells at different stages of differentiation (e.g., Treg, Th17, Th1 and Th2) to understand their individual proteomes at single cell resolution.
Methods
Preparation of primary T cells from blood
Whole blood samples from healthy volunteers were collected under Mayo Clinic's Institutional Review Board approved protocol (IRB protocol #1039-03). Informed consent was obtained from all participants. Primary T cells were isolated from a human blood cone using antibody cocktail negative selection and density gradient separation. The blood cone was sprayed with 70% ethanol, the bottom pipe was cut, and the cone was placed on a 50 ml Falcon tube. After the top pipe was cut, the blood flowed from the cone. The blood was around 7 ml and then diluted with 1× complete PBS (1× PBS, 2% heat inactivated FBS, and 1 mM EDTA) to 25 ml. The RosetteSep™ Human T Cell Enrichment Cocktail (STEMCELL, 15061) was added to the diluted blood sample (25 μl cocktail per ml diluted blood sample) for isolating T cells by negative selection. Unwanted cells were targeted for removal with tetrameric antibody complexes recognizing non-T cells and glycophorin A on red blood cells. The blood–cocktail mixture was incubated at room temperature for 20 minutes and then layered gently onto 13 ml Ficoll (a density gradient reagent) in a 50 ml Falcon tube. The mixture was centrifuged at room temperature and 600g for 20 minutes using the lowest acceleration and deceleration rate without any interruption. The purified T cells are present as a highly enriched population at the interface between the plasma and the dense medium. The T-cell layer was carefully transferred to a new 50 ml tube and diluted to 25 ml using PBS with gentle mixing followed by centrifugation at 600g for 10 minutes. The cell pellet was resuspended in 25 ml of ACK lysis buffer and incubated for 5 minutes. Cells were pelleted down and washed with PBS two times and resuspended in RPMI media at a density of 1 × 10 6 cells per ml. IL2 was added to the cells at a concentration of 10 ng ml−1 which were then transferred to a new flask and incubated at 37 °C overnight. Purified T cells were counted and taken for the following experiments.
Sample preparation for diluted peptides
Urea lysis buffer (8 M urea, 50 mM triethylammonium bicarbonate buffer, pH 8.5 (TEAB), protease and phosphatase inhibitor cocktail) was added to the cells followed by sonication on ice for 2 minutes. The sample was centrifuged at 10
000g for 5 minutes and the supernatant containing proteins was transferred to another 1.5 ml tube. Proteins were reduced with 10 mM dithiothreitol for 30 minutes and then alkylated with 20 mM IAA for 20 minutes at room temperature in the dark. The concentration of urea was diluted to <1 M using 50 mM TEAB for efficient digestion. Trypsin (Thermo Fisher Scientific, 90057) in a ratio of 1
:
20 (enzyme
:
protein) was used to digest proteins overnight at 37 °C. The digested peptides were acidified with formic acid to quench the reaction and then dried under vacuum. Peptide samples were resuspended in 50 mM TEAB and peptide estimation was performed using Pierce quantitative colorimetric peptide assay (Thermo Fisher Scientific, 23275). Serially diluted peptides were prepared ranging from 10 ng to 156 pg. In addition, a lyophilized HeLa protein digest standard (Thermo Scientific, 88328) was used to prepare serially diluted peptide samples ranging from 10 ng to 156 pg.
Sample preparation of single primary T cells
A suspension of primary T cells in PBS was prepared with a concentration of ∼200
000 cells per ml. Cell sorting was performed using a cellenONE system (Cellenion, France) with a picoliter dispensing ability for single cell isolation. Primary T cells were isolated in each well of a 384-well plate using the isolation parameters where the cell diameter was set as 8–14 μm and the maximum cell elongation was set to 1.8. To lyse the cells, 350 nl of lysis buffer with protease (2% n-dodecyl-β-D-maltoside, 100 mM TEAB and 2 ng μl−1 trypsin) was dispensed into each well using the cellenONE system. The cells were incubated at 37 °C for 1 hour at a humidity of 75% inside the cellenONE platform. Peptide samples from each well were resuspended in 5 μl of 0.1% formic acid and transferred into sample vials for mass spectrometry analysis.
Mass spectrometry data acquisition
Peptide samples were directly injected and separated on an analytical column (25 cm × 50 μm, C18, 1.9 μm, Bruker Daltonics) using a nanoElute liquid chromatography system (Bruker Daltonics, Bremen, Germany). Solvent A (0.1% formic acid in water) and solvent B (0.1% formic acid in acetonitrile) were used to generate a linear gradient over 75 min; 2–27% sol B in 55 min, 27–40% sol B in 5 min, 40–80% sol B in 5 min, maintaining at 80% sol B for 5 min, and 2% sol B for 5 min. The flow rate was set as 200 nl min−1. The separated peptides were ionized using a captive spray source with a spray voltage of 1300 V and introduced into the timsTOF SCP mass spectrometer (Bruker Daltonics, Bremen, Germany). The mass range was set as 100–1700 m/z with DDA-PASEF mode. The ramp time was varied from 50 to 200 ms using a 100% duty cycle. Precursor repetitions were set at a 20
000 target intensity with 500 intensity thresholds. The collision energy was linearly increased from 20 (0.6 V s cm−2) to 59 eV (1.6 V s cm−2). All mass spectrometry data have been deposited to the ProteomeXchange Consortium via the PRIDE partner repository with the data set identifier PXD 039066.42
Data analysis
The raw mass spectrometry data were searched against the UniProt human protein database (20
430 entries) using MSFragger (version 3.4) embedded in the FragPipe suite (version 17.0). Two missed cleavages were allowed with a fully tryptic option. When analyzing samples of diluted peptides, carbamidomethylation of cysteine was considered as a fixed modification and oxidation of methionine and acetylation of the protein N-terminal were set as variable modifications. For sorted cells, oxidation of methionine, acetylation of protein N-terminal/lysine and phosphorylation of serine/threonine/tyrosine were considered as variable modifications. PSM and protein validation were performed using PeptideProphet and ProteinProphet, respectively, at a 1% false discovery rate (FDR).
Ethics approval and consent from participants
This study was approved by the Mayo Clinic's Institutional Review Board (IRB protocol #1039-03). Informed consent was obtained from all participants of the study.
Author contributions
D.-G. M. and A. P. designed the experiments. S. N., F. A. B. and H. D. prepared primary T cells. D.-G. M. and B. J. M. performed single cell sorting for proteomics. D.-G. M., F. A. B. and K. L. J. acquired mass spectrometry data. D.-G. M. and F. A. B. analyzed mass spectrometry data. D.-G. M., A. D. B., R. T. K. and A. P. wrote the manuscript with input from all authors.
Conflicts of interest
The authors declare that they have no competing interests.
Acknowledgements
This work was supported by grants from NCI to A. P. and R. T. K. (U01CA271410) and to A. P. (P30CA15083).
References
- N. Slavov, Science, 2020, 367, 512–513 CrossRef CAS PubMed.
- V. Marx, Nat. Methods, 2019, 16, 809–812 CrossRef CAS PubMed.
- L. F. Vistain and S. Tay, Trends Biochem. Sci., 2021, 46, 661–672 CrossRef CAS PubMed.
- R. T. Kelly, Mol. Cell. Proteomics, 2020, 19, 1739–1748 CrossRef CAS PubMed.
- Y. Zhu, P. D. Piehowski, R. Zhao, J. Chen, Y. Shen, R. J. Moore, A. K. Shukla, V. A. Petyuk, M. Campbell-Thompson, C. E. Mathews, R. D. Smith, W. J. Qian and R. T. Kelly, Nat. Commun., 2018, 9, 882 CrossRef PubMed.
- Z. Y. Li, M. Huang, X. K. Wang, Y. Zhu, J. S. Li, C. C. L. Wong and Q. Fang, Anal. Chem., 2018, 90, 5430–5438 CrossRef CAS PubMed.
- T. Masuda, Y. Inamori, A. Furukawa, M. Yamahiro, K. Momosaki, C. H. Chang, D. Kobayashi, H. Ohguchi, Y. Kawano, S. Ito, N. Araki, S. E. Ong and S. Ohtsuki, Anal. Chem., 2022, 94, 10329–10336 CrossRef CAS PubMed.
- S. T. Gebreyesus, A. A. Siyal, R. B. Kitata, E. S. Chen, B. Enkhbayar, T. Angata, K. I. Lin, Y. J. Chen and H. L. Tu, Nat. Commun., 2022, 13, 37 CrossRef CAS PubMed.
- J. Leipert and A. Tholey, Lab Chip, 2019, 19, 3490–3498 RSC.
- J. Lamanna, E. Y. Scott, H. S. Edwards, M. D. Chamberlain, M. D. M. Dryden, J. Peng, B. Mair, A. Lee, C. Chan, A. A. Sklavounos, A. Heffernan, F. Abbas, C. Lam, M. E. Olson, J. Moffat and A. R. Wheeler, Nat. Commun., 2020, 11, 5632 CrossRef CAS PubMed.
- B. Budnik, E. Levy, G. Harmange and N. Slavov, Genome Biol., 2018, 19, 161 CrossRef PubMed.
- H. Specht, E. Emmott, A. A. Petelski, R. G. Huffman, D. H. Perlman, M. Serra, P. Kharchenko, A. Koller and N. Slavov, Genome Biol., 2021, 22, 50 CrossRef CAS PubMed.
- L. Yi, C. F. Tsai, E. Dirice, A. C. Swensen, J. Chen, T. Shi, M. A. Gritsenko, R. K. Chu, P. D. Piehowski, R. D. Smith, K. D. Rodland, M. A. Atkinson, C. E. Mathews, R. N. Kulkarni, T. Liu and W. J. Qian, Anal. Chem., 2019, 91, 5794–5801 CrossRef CAS PubMed.
- C. F. Tsai, R. Zhao, S. M. Williams, R. J. Moore, K. Schultz, W. B. Chrisler, L. Pasa-Tolic, K. D. Rodland, R. D. Smith, T. Shi, Y. Zhu and T. Liu, Mol. Cell. Proteomics, 2020, 19, 828–838 CrossRef CAS PubMed.
- J. Woo, G. C. Clair, S. M. Williams, S. Feng, C. F. Tsai, R. J. Moore, W. B. Chrisler, R. D. Smith, R. T. Kelly, L. Pasa-Tolic, C. Ansong and Y. Zhu, Cell Syst., 2022, 13, 426–434 CrossRef CAS PubMed.
- Y. Cong, K. Motamedchaboki, S. A. Misal, Y. Liang, A. J. Guise, T. Truong, R. Huguet, E. D. Plowey, Y. Zhu, D. Lopez-Ferrer and R. T. Kelly, Chem. Sci., 2020, 12, 1001–1006 RSC.
- F. Meier, A. D. Brunner, S. Koch, H. Koch, M. Lubeck, M. Krause, N. Goedecke, J. Decker, T. Kosinski, M. A. Park, N. Bache, O. Hoerning, J. Cox, O. Rather and M. Mann, Mol. Cell. Proteomics, 2018, 17, 2534–2545 CrossRef CAS PubMed.
- A. D. Brunner, M. Thielert, C. Vasilopoulou, C. Ammar, F. Coscia, A. Mund, O. B. Hoerning, N. Bache, A. Apalategui, M. Lubeck, S. Richter, D. S. Fischer, O. Raether, M. A. Park, F. Meier, F. J. Theis and M. Mann, Mol. Syst. Biol., 2022, 18, e10798 CrossRef CAS PubMed.
- A. T. Kong, F. V. Leprevost, D. M. Avtonomov, D. Mellacheruvu and A. I. Nesvizhskii, Nat. Methods, 2017, 14, 513–520 CrossRef CAS PubMed.
- T. J. Aballo, D. S. Roberts, J. A. Melby, K. M. Buck, K. A. Brown and Y. Ge, J. Proteome Res., 2021, 20, 4203–4211 CrossRef CAS PubMed.
- D. G. Mun, P. M. Vanderboom, A. K. Madugundu, K. Garapati, S. Chavan, J. A. Peterson, M. Saraswat and A. Pandey, J. Proteome Res., 2021, 20, 4165–4175 CrossRef CAS PubMed.
- H. Wang, T. A. Kadlecek, B. B. Au-Yeung, H. E. Goodfellow, L. Y. Hsu, T. S. Freedman and A. Weiss, Cold Spring Harbor Perspect. Biol., 2010, 2, a002279 Search PubMed.
- J. Guergues, J. Wohlfahrt and S. M. Stevens, Jr., J. Proteome Res., 2022, 21, 2036–2044 CrossRef CAS PubMed.
- F. Yu, S. E. Haynes and A. I. Nesvizhskii, Mol. Cell. Proteomics, 2021, 20, 100077 CrossRef CAS PubMed.
- C. F. Tsai, P. Zhang, D. Scholten, K. Martin, Y. T. Wang, R. Zhao, W. B. Chrisler, D. B. Patel, M. Dou, Y. Jia, C. Reduzzi, X. Liu, R. J. Moore, K. E. Burnum-Johnson, M. H. Lin, C. C. Hsu, J. M. Jacobs, J. Kagan, S. Srivastava, K. D. Rodland, H. Steven Wiley, W. J. Qian, R. D. Smith, Y. Zhu, M. Cristofanilli, T. Liu, H. Liu and T. Shi, Commun. Biol., 2021, 4, 265 CrossRef CAS PubMed.
- A. Kramer, J. Green, J. Pollard, Jr. and S. Tugendreich, Bioinformatics, 2014, 30, 523–530 CrossRef PubMed.
- Y. Liao, J. Wang, E. J. Jaehnig, Z. Shi and B. Zhang, Nucleic Acids Res., 2019, 47, W199–W205 CrossRef CAS PubMed.
- I. Lefkovits, Comp. Funct. Genomics, 2003, 4, 531–536 CrossRef CAS PubMed.
- Y. Cong, Y. Liang, K. Motamedchaboki, R. Huguet, T. Truong, R. Zhao, Y. Shen, D. Lopez-Ferrer, Y. Zhu and R. T. Kelly, Anal. Chem., 2020, 92, 2665–2671 CrossRef CAS PubMed.
- C. T. Walsh, S. Garneau-Tsodikova and G. J. Gatto, Jr., Angew. Chem., Int. Ed., 2005, 44, 7342–7372 CrossRef CAS PubMed.
- B. Schwanhausser, D. Busse, N. Li, G. Dittmar, J. Schuchhardt, J. Wolf, W. Chen and M. Selbach, Nature, 2011, 473, 337–342 CrossRef PubMed.
- N. Ladygina, S. Gottipati, K. Ngo, G. Castro, J. Y. Ma, H. Banie, T. S. Rao and W. P. Fung-Leung, Eur. J. Immunol., 2013, 43, 3183–3196 CrossRef CAS PubMed.
- B. J. Klein, S. M. Jang, C. Lachance, W. Mi, J. Lyu, S. Sakuraba, K. Krajewski, W. W. Wang, S. Sidoli, J. Liu, Y. Zhang, X. Wang, B. M. Warfield, A. J. Kueh, A. K. Voss, T. Thomas, B. A. Garcia, W. R. Liu, B. D. Strahl, H. Kono, W. Li, X. Shi, J. Cote and T. G. Kutateladze, Nat. Commun., 2019, 10, 4724 CrossRef PubMed.
- C. H. Na, M. A. Barbhuiya, M. S. Kim, S. Verbruggen, S. M. Eacker, O. Pletnikova, J. C. Troncoso, M. K. Halushka, G. Menschaert, C. M. Overall and A. Pandey, Genome Res., 2018, 28, 25–36 CrossRef CAS PubMed.
- A. Lesur, P. O. Schmit, F. Bernardin, E. Letellier, S. Brehmer, J. Decker and G. Dittmar, Anal. Chem., 2021, 93, 1383–1392 CrossRef CAS PubMed.
- Q. Kong, Y. Weng, Z. Zheng, W. Chen, P. Li, Z. Cai and R. Tian, Anal. Chem., 2022, 94, 13728–13736 CrossRef CAS PubMed.
- A. Devabhaktuni, S. Lin, L. Zhang, K. Swaminathan, C. G. Gonzalez, N. Olsson, S. M. Pearlman, K. Rawson and J. E. Elias, Nat. Biotechnol., 2019, 37, 469–479 CrossRef CAS PubMed.
- A. Prakash, S. Ahmad, S. Majumder, C. Jenkins and B. Orsburn, J. Am. Soc. Mass Spectrom., 2019, 30, 2408–2418 CrossRef CAS PubMed.
- E. M. Schoof, B. Furtwangler, N. Uresin, N. Rapin, S. Savickas, C. Gentil, E. Lechman, U. A. D. Keller, J. E. Dick and B. T. Porse, Nat. Commun., 2021, 12, 3341 CrossRef CAS PubMed.
- B. Sun, J. R. Kovatch, A. Badiong and N. Merbouh, J. Proteome Res., 2017, 16, 3711–3721 CrossRef CAS PubMed.
- E. W. Newell and M. M. Davis, Nat. Biotechnol., 2014, 32, 149–157 CrossRef CAS PubMed.
- Y. Perez-Riverol, J. Bai, C. Bandla, D. Garcia-Seisdedos, S. Hewapathirana, S. Kamatchinathan, D. J. Kundu, A. Prakash, A. Frericks-Zipper, M. Eisenacher, M. Walzer, S. Wang, A. Brazma and J. A. Vizcaino, Nucleic Acids Res., 2022, 50, D543–D552 CrossRef CAS PubMed.
Footnote |
† Electronic supplementary information (ESI) available: Fig. S1: Distribution of the ion mobility of peptides identified from ramp times of 50, 100, 150, and 200 ms injecting 2.5 ng of peptides. Fig. S2: Annotated MS/MS spectra of a single hit peptide from proteins CCR7, CD27 and SATB1. Fig. S3: The number of identified peptides and proteins from 10 ng to 156 pg of HeLa peptides. Fig. S4: Pathway enrichment analysis using identified enzymes from one, five, ten and forty T cells. See DOI: https://doi.org/10.1039/d3an00080j |
|
This journal is © The Royal Society of Chemistry 2023 |
Click here to see how this site uses Cookies. View our privacy policy here.