DOI:
10.1039/D1TA09878K
(Paper)
J. Mater. Chem. A, 2022,
10, 6679-6689
Machine learning assisted high-throughput screening of transition metal single atom based superb hydrogen evolution electrocatalysts†
Received
17th November 2021
, Accepted 31st January 2022
First published on 2nd February 2022
Abstract
Carbon-based transition metal (TM) single-atom catalysts (SACs) have shown great potential toward electrochemical water splitting and H2 production. Given that two-dimensional (2D) materials are widely exploited for sustainable energy conversion and storage applications, the optimization of SACs with respect to diverse 2D materials is of importance. Herein, using density functional theory (DFT) and machine learning (ML) approaches, we highlight a new perspective for the rational design of TM-SACs. We have tuned the electronic properties of ∼364 rationally designed catalysts by embedding 3d/4d/5d TM single atoms in diverse substrates including g-C3N4, π-conjugated polymer, pyridinic graphene, and hexagonal boron nitride with single and double vacancy defects each with a mono- or dual-type non-metal (B, N, and P) doped configuration. In ML analysis, we use various types of electronic, geometric and thermodynamic descriptors and demonstrate that our model identifies stable and high-performance HER electrocatalysts. From the DFT results, we found 20 highly promising candidates which exhibit excellent HER activities (|ΔGH*| ≤ 0.1 eV). Remarkably, Pd@B4, Ru@N2C2, Pt@B2N2, Fe@N3, Fe@P3, Mn@P4 and Fe@P4 show practically near thermo-neutral binding energies (|ΔGH*| = 0.01–0.02 eV). This work provides a fundamental understanding of the rational design of efficient TM-SACs for H2 production through water-splitting.
1. Introduction
To reduce fossil fuel consumption and mitigate global warming, tremendous attention has been paid to hydrogen as a renewable fuel.1,2 Water electrolysis is regarded as an ideal approach for high-purity hydrogen generation, which is of great significance for global clean energy deployment and ecological environmental protection.3–5 The key aspect to promote this technology is the development of inexpensive, stable and high-performance electrocatalysts.6,7 Platinum (Pt) based catalysts have been most practical and efficient for the hydrogen evolution reaction (HER), however, the practical implementations for electrochemical hydrogen production have been thwarted by high cost and natural scarcity of Pt. Recently, for cost-effective and highly active electrocatalysts it has been suggested to utilize ultra-low loading of precious metals such as Pt, Ru, Rh, Pd and Ir as SACs which show very small overpotentials for the HER.8–13 Meanwhile, considerable efforts are being made to search for new cost-effective, stable and active non-noble metal electrocatalysts to replace these precious noble metals.14–18 Recently, a vast number of different classes of materials have emerged in the field of electrocatalysis, such as metal carbides,19,20 nitrides,21–23 phosphides,24 and two dimensional (2D) material families including metal oxides,25–27 layered double hydroxides (LDHs),28,29 graphene,30,31 MXenes,32–34 phosphorene,35 graphitic carbon nitride (g-C3N4)36,37 and TM dichalcogenides (TMDs).38,39 These diverse types of materials have been widely exploited for various electrocatalytic applications, and indeed have shown impressive electrocatalytic activities towards the HER,40,41 oxygen evolution/reduction reaction (OER/ORR),22,30,42–44 nitrogen reduction reaction (NRR),45–48 carbon dioxide reduction reaction (CO2RR)49 ammonia oxidation reaction (AOR),50etc. Among these heterogeneous material classes, graphene-supported TM-SACs have gained considerable attention due to their well-defined atomically dispersed active sites and tunable electrocatalytic properties. The electronic properties and durability of TM-SACs can be controlled by the modification of the coordination environment and the formation of particular types of vacancies on the surface.51 In principle, by tuning these two parameters, the HER performance of these materials can be substantially improved. Extensive work has been done on series of different non-metal (B, N, P, and S) doped TM-SACs with various metal-nonmetal configurations, which have shown great potential towards multifunctional electrocatalytic applications.52–54 Also, significant efforts have been devoted to identifying 2D materials beyond graphene that offer a greater degree of tunability and excellent conductivity. For example, Galeotti et al. successfully fabricated a two-dimensional π-conjugated polymer (2DCP) with kagome lattices in the mesoscale order.55 The 2DCP exhibited excellent stability with a tunable bandgap and high carrier mobility. Given these advantages, we also sought to consider 2DCP. Theoretical exploration of key insights is advantageous for promoting experimental development of new high-performance HER catalysts.40,56–58 Recently, the ML approach has shown immense potential to accelerate the discovery of energy materials59,60 and electrocatalysts. Various geometric and electronic descriptors have been proposed to guide the design and screening of catalyst properties, such as Coulomb matrix,61 bag of bonds (BOB),62 smooth overlap of atomic positions (SOAP),63 adsorption free energy d-band center,64etc. Recently, Jäger et al. predicted the hydrogen adsorption free energies on various nanoclusters by using SOAP, many-body tensor representation (MBTR), and atom-centered symmetry function (ACSF) descriptors.65 To this end, substantial interest has been devoted to developing such descriptors which can be directly employed to design and screen catalysts without performing extensive DFT calculations.
Herein, we adopted a stepwise screening strategy and investigated the stability and activity of several experimentally feasible 2D structures for H2 production. By DFT calculations we screened over 364 catalysts, and out of these we found that 20 catalysts exhibit excellent stabilities and near-zero overpotentials. Based on the thermodynamic stability energy (Estab) and dissolution potential (Udiss), we further classified the catalysts into four different groups and assessed their HER activities. For the ML study, we initially considered basic physicochemical properties as possible features to train various ML models. In order to recognize the intrinsic factors that govern the stability and activities, we further employed the subsequent equation learning method using the sure independence screening and sparsifying operator (SISSO) approach (see ESI Note 2†).66 The top-ranked SISSO generated descriptors were further passed to the feature selection step. By using the step forward feature selection method, we carefully evaluated the feature importance and reserved only the most relevant features in the database for the catalytic performance prediction. Our identified descriptors and systematic screening strategy provide a stepping stone toward designing durable and high-performance electrocatalysts.
2. Computational methods
DFT calculations were carried out by using the Vienna ab initio simulation package (VASP)67 with the projector augmented wave (PAW) method.68 We set a kinetic energy cutoff of 500 eV for plane-wave expansion of the electronic wave function. The exchange–correlation interaction was treated by the Perdew–Burke–Ernzerhof (PBE) functional within the generalized gradient approximation (GGA).69,70 For geometry optimization we use a 3 × 3 × 1 Monkhorst–Pack k-point grid, however, a denser 7 × 7 × 1 k-point grid was chosen for electronic structure calculations. A vacuum of 15 Å along the z-axis was set throughout all the calculations to avoid interactions between repeated images. The energy and force convergence criteria were set to 10−5 eV and 0.01 eV Å−1, respectively. Given that the HER reaction intermediate adsorption/desorption is a surface phenomenon, the effect of the van der Waals (vdW) interaction was accounted for by applying the Tkatchenko–Sheffler (TS) dispersion correction method.71 The solvation effect was taken into account by using the pure implicit solvent model as implemented in VASPsol.72 In order to evaluate the HER activities we utilized different active sites and calculated the differential hydrogen adsorption Gibbs free energies by using the computational hydrogen electrode (CHE) model developed by Nørskov et al.:73 | ΔGH* = ΔEnH* + ΔEnH*(ZPE) − TΔS + eU + pH × kBT × ln(10) | (1) |
where ΔEH* represents the electronic energy difference between the catalytic surfaces with and without adsorbates, and ΔEH*(ZPE) and TΔS are the changes in the zero-point energy (ZPE) and the entropic energy, respectively, of the reaction at room temperature. U is the electrode potential, which is referenced to be 0 V in the CHE model such that the protons and electrons are in equilibrium with the gas-phase of H2 under standard conditions (temperature T = 298.15 K, pressure P = 1 bar, and pH = 0). The last term is the free energy correction at a finite pH. The H-adsorption energy was calculated by using the following equation: | 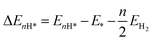 | (2) |
where EnH* and E* are the total energies of the catalyst with and without adsorbed nH atoms (n = 1 except for n = 2 for Kubo's form), respectively, and EH2 is the total energy of an isolated H2 gas molecule. ΔE(ZPE) is calculated by using the following equation: |  | (3) |
where EnH*(ZPE) and E*(ZPE) are ZPEs of the adsorbed nH atoms and the pristine surface, respectively, and the ZPE of an isolated H2 molecule is represented as EH2(ZPE).
The ΔS is obtained from
|  | (4) |
The theoretical overpotential ηHER is given by
|  | (5) |
To compare the HER performance of promising candidates, we calculated the exchange current densities (io) by utilizing free energy values at pH = 0.
| 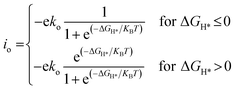 | (6) |
Here,
ko and
KB represent the rate constant and Boltzmann constant, respectively. We set
ko as unity for calculating exchange current values.
74
Furthermore, in our ML analysis, we employed various ML classification and regression models to classify the structural stabilities and to predict HER activities. To estimate the model performance, we employed the Monte-Carlo cross-validation method.75 The data samples were randomly split 100 times into different training and test sets with 8
:
2 split ratios and the weight-averaged prediction errors were presented for each ML model.
3. Results and discussion
3.1 Structural stability
In spite of exhibiting excellent electrocatalytic activities, the practical applications rely strongly on the long-lasting stability/durability of catalysts. Using DFT calculations, we first investigated the thermodynamic and electrochemical stabilities of ∼364 rationally designed catalysts by using a combination of 28 (3d/4d/5d) transition metal (TM) atoms with 13 kinds of experimentally available substrates (C4, N4, B4, N2C2, B2C2, B2N2, 2DCP, g-C3N4, h-B2N2, h-BN, C3, N3 and B3). Fig. 1 and Fig. S3 and S4† present all the geometrical structures we used in this study. In order to obtain the TM binding strength, we calculated the embedding energies (Eemb) by using the following equation: | Eemb = ETM@surf − Esurf − ETM | (7) |
where ETM@surf and Esurf are the TM embedded surface and the defected (single or double vacancy) surface, respectively, and ETM is the energy of an isolated TM single atom. The DFT optimized geometries revealed that embedding TM may form an in- or out-plane configuration depending on the atomic radius of TM and the coordinating non-metal atoms. For example, most of the phosphorous doped optimized geometries revealed that the embedded TM and surface non-metal atoms are protruded from the planar surface, forming a distorted final geometry (Fig. S4†), and so we ruled out such unstable systems in our further studies. However, among 3d, 4d and 5d TMs, the elements of Ti, Cr, Mn, Fe, Co, Ni, Ru, Rh, Pd, Ir and Pt form the planar geometric configurations in double vacancy structures. Moreover, in all the single vacancy structures the TM atom is protruded out of the planar geometry. Compared with double vacancy systems the weak Eemb values in single vacancy structures indicate that these are more liable to form aggregates. Overall, Fe, Co, Ni, Rh, Pd, Ir and Pt are relatively more stabilized in single and double vacancy structures. Moreover, their negative embedding energy values indicate that the TM atom is bound onto the surface strongly enough to avoid the metal diffusion, and hence it could suppress the metal aggregation or nanoparticle (NP) formation. We further calculated the thermodynamic stability energies (Estab) by considering the metal bulk cohesive energies. The Estab is defined as the difference between Eemb and metal bulk cohesive energies (E−coh ≡ −Ecoh), opted from the previous study42 (Estab = Eemb − E−coh). The calculated Eemb and Estab values are presented in Tables S1 and S2.† The Estab is considered to be a good descriptor to govern the thermodynamic stabilities of catalysts. The negative Estab values indicate that TM embedding is preferred over metal clustering/aggregation. The electrochemical stabilities are investigated in terms of the dissolution potential (Udiss),76,77 which is defined as |  | (8) |
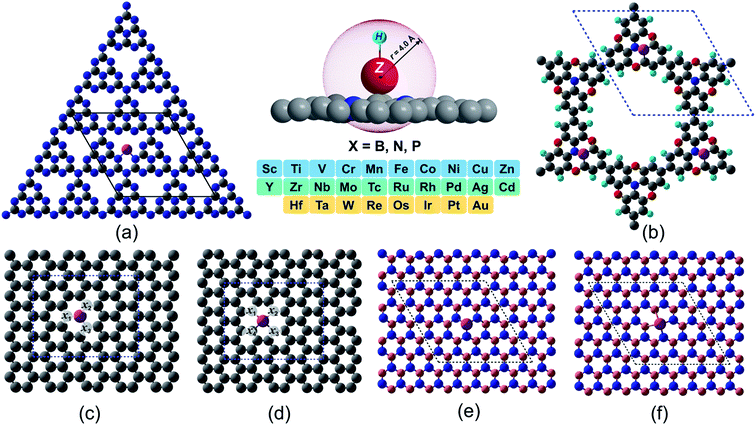 |
| Fig. 1 Proposed 2D materials with TM embedded at various defect sites. (a) g-C3N4 (b) 2DCP (c) graphene with one C defect site (d) graphene with two C defects site (e) h-BN with one B defect site (f) h-B2N2 with one B and one N defects site. The labeled sites (x1–x4) in light gray color in (c) and (d) represent the non-metal (B, N, P) doping sites in single and dual type doping fashion. Color code: metal, magenta; B, light pink; N, blue; C, gray; O, red; H, cyan). | |
The
and n represent the standard dissolution potential of the bulk TM and the number of electrons involved in dissolution, respectively. The materials with Udiss > 0 vs. the standard hydrogen electrode (SHE) are regarded to be electrochemically stable. The exact Udiss values are listed in Table S3.† A catalyst with Estab < 0 and Udiss > 0 is considered to be thermodynamically and electrochemically stable. According to our defined stability criteria, we grouped all the catalysts into four classes (G1: unaggregatable and indissoluble, G2: aggregatable and indissoluble, G3: unaggregatable and dissoluble, G4: aggregatable and dissoluble) depicted in Fig. 2 and Table S4.† It is worth noting that most of the experimentally synthesized catalysts are falling into our stability evaluation criteria, which suggests the reliability and feasibility of our approach. The computed Estab and Udiss values reveal that the substrates such as C4, N4, N2C2, 2DCP, h-BN, h-B2N2 and C3 are good supports to form single atom moieties. Generally, the early TM-SACs such as Sc, Ti, Cr, V, Y, Zr, Nb, Hf and Ta are more likely to be stabilized thermodynamically (Eemb < 0), however, their negative Udiss values indicate that these catalysts are electrochemically unstable under acidic conditions. We found that, particularly, the 3d-TMs including Mn, Fe, Co, Ni and Cu in double vacancy sites have planar structures with strong metal-support interactions and exhibit excellent stabilities. Among 4d- and 5d-TMs, the elements of Ru, Rh, Pd, Ir and Pt display superior thermodynamic and electrochemical stabilities on all the surfaces studied here. To further check the dynamic stability of single and double vacancy systems, we performed the DFT-based ab initio molecular dynamics (AIMD) simulations at 500 K by using the Nose–Hoover thermostat method78 and also sparse Gaussian process regression (SGPR)79,80 machine-learning DFT potential/force based AIMD simulations which reproduced the DFT-based AIMD simulation results in a much faster manner which can be readily extended to other metal cases using universal potential generation. Fig. S5† illustrates that during the AIMD simulations the energy and temperature remain within a small range, indicating the stability of these structures. Our identified mono- and dual-type non-metal doped TM-SACs hold outstanding promise for synthesis.
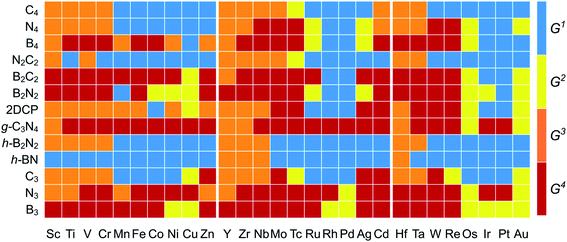 |
| Fig. 2 Stability classification of proposed 2D surfaces based on TM embedding over metal bulk cohesive energies (Estab) and electrochemical dissolution potential (Udiss) values (Tables S2 and S3†). Four different classes (G1/G2/G3/G4) of stabilities are represented with (blue/yellow/orange/brown color) [G1: unaggregatable and indissoluble, G2: aggregatable and indissoluble, G3: unaggregatable and dissoluble, G4: aggregatable and dissoluble]. | |
3.2 HER activity and the reaction mechanism
Under standard conditions, the HER may go through two possible mechanistic pathways involving the hydrogen adsorption in the first step through the Volmer reaction (H+ + e− → H* where the asterisk denotes an adsorbed state), and in the second step the H2 desorption takes place through either the Heyrovsky reaction (H* + H+ + e− → H2) or the Tafel reaction pathway (2H* → H2) (Fig. 3a). For an ideal HER electrocatalyst, the Sabatier principle suggests a thermoneutral binding condition i.e. |ΔGH*| = 0 of hydrogen adsorption,81 since too strong or too weak H-adsorption would decrease the overall reaction rate. In order to establish a definite structure–activity relationship, we utilized different active sites and estimated the H-binding strength (ΔEH*) over a wide range of 2D materials (Fig. S3 and S4†). We found that in most of the double vacancy systems H atoms preferentially get adsorbed at the bond edge site (i.e. M–X, X = C, B, N). In the cases of Co/Ni/Cu/Pd/Ag/Pt/Au at C4, Cr/Fe/Co/Ni at B4, Ni/Rh/Pd/Pt at N2C2, Mn/Co/Ni/Cu/Pd/Pt at B2C2, Co/Ni/Cu/Pd/Pt at B2N2 and Mn/Fe/Co/Ni/Cu/Zn/Rh/Pd/Ag/Ir/Pt/Au at h-B2N2 templates, the active site is shared by metal and surface non-metal atoms. In contrast, hydrogen tends to bind at a metal site in all the single vacancy systems. For better understanding of structural properties, we calculated the metal to hydrogen bond distance (dM–H) and the angle (φH–M–Y) between adsorbed hydrogen (H) on a TM atom (M) and H on its first coordinating nearest neighbor non-metal atom (Y) of the 2D support (Tables S6, S7 and Fig. S6, S7†). Compared with the metal top site, we found that H-adsorption at the bond edge site exhibits a shorter bond distance (dM–H) with a smaller bond angle (φH–M–Y), which is also obvious from their optimal ΔEH* values (Table S8†).
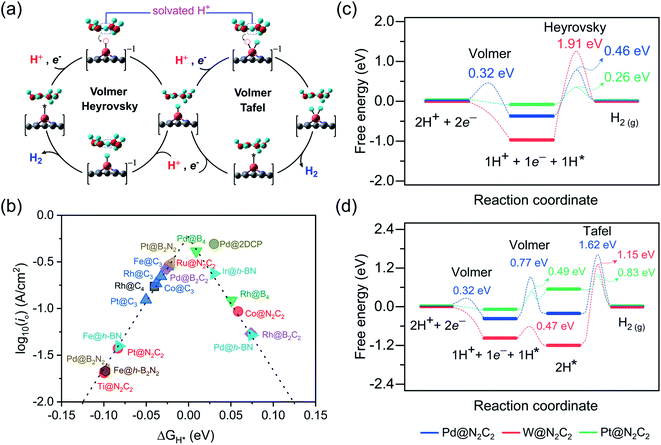 |
| Fig. 3 (a) Schematic representation for the mechanisms and reaction pathways of electrocatalytic reaction cycles over different proposed 2D surfaces. (b) HER volcano plot for the promising electrocatalysts. The exchange current density plotted versus the calculated free energy of H-adsorption. Free energy diagram for the (c) Volmer–Heyrovsky and (d) Volmer–Tafel route on Pd@N2C2 (blue color), W@N2C2 (red color) and Pt@N2C2 (green color) surfaces. | |
In order to determine the HER activities, we calculated hydrogen adsorption Gibbs free energies and HER overpotentials (ηHER) for each system and considered the condition of |ΔGH*| ≤ 0.1 eV as a descriptor. Relative to an ideal HER electrocatalyst such as Pt, we compared the HER free energy values of undoped systems with those of their mono- and dual-type non-metal doped templates. The computed ΔGH* values are listed in Table S9† and their relative HER activities are presented in HER free energy diagrams shown in Fig. S8–S12.† To find the high-performance catalysts we plot the volcano curve for the exchange current density (io) as a function of ΔGH* values (Fig. 3b). We have found promising durable catalysts with excellent HER activities (i.e. |ΔGH*| ≤ 0.1 eV) (Table S10†), such as Rh(−0.04) at C4, Rh(0.05)/Pd(0.01) at B4, Ti(−0.10)/Co(0.06)/Ru(−0.02)/Pt(−0.08) at N2C2, Rh(0.07)/Pd(−0.03) at B2C2, Pd(−0.10)/Pt(−0.02) at B2N2, Fe(−0.02)/Co(−0.04)/Rh(−0.03)/Pt(−0.05) at C3, Fe(−0.08)/Pd(0.07)/Ir(0.03) at h-BN and Pd(0.03) at 2DCP. In particular, Pd@B4, Pt@B2N2, Pd@2DCP and Ru@N2C2 have almost thermoneutral H-adsorption Gibbs free energies and are located around the top of the volcano plot.
For getting deep insight into the kinetic aspects of the reaction mechanism, we investigated various TM-SACs and calculated the energy barriers for three elementary reaction steps (Volmer step, Heyrovsky step, and Tafel step) of the HER. By using the climbing-image nudged elastic band (CI-NEB) method, we interpolated seven images between the initial and final states. Herein, we presented the HER mechanism over Pd@N2C2, W@N2C2 and Pt@N2C2 surfaces. Fig. 3a demonstrates the mechanism for each reaction step involved in the HER. Initially, in the Volmer step, we considered a layer of three water molecules over these surfaces along with one hydronium ion (H3O+). The solvated proton82 from the water layer is transferred to the surface and is favorably adsorbed at the Pd–C bond edge site with an activation barrier of 0.32 eV, while W@N2C2 having metal top as the most active site for the HER shows a barrierless first Volmer reaction step. Similarly, Pt@N2C2 having the Pt–C bond edge as the most active site for H adsorption exhibits a barrierless first Volmer reaction step.
In the second step, the hydrogen evolution takes place either by the Heyrovsky or Tafel reaction mechanism. In the Heyrovsky reaction pathway, the solvated proton from the water layer reacts with the adsorbed H atom to form molecular H2, while in the Tafel reaction pathway the two adsorbed H-atoms next to each other react to form a H2 molecule. Fig. 3c and d present the activation barriers and free energy values of Volmer–Heyrovsky and Volmer–Tafel reaction steps for H2 evolution. The calculated energy barriers indicate that after adsorption of the first H-atom, both the second Volmer step and the Heyrovsky reaction step compete with each other to accomplish the HER process. A precise probing of the local atomistic structure revealed that the adsorbed hydrogen may form a Kubas42 type stable dihydrogen complex83 which may change the overall kinetics of the reaction. We found that on Pd@N2C2 and Pt@N2C2 surfaces the second H-atom is adsorbed at the Pd–C and Pt–C bond edge sites in the trans-form. Their first and second adsorbed H-atoms are separated by 3.21 Å and 2.13 Å, which exhibit large activation barriers of 1.62 eV and 0.83 eV, respectively, indicating that the H2 formation through the Tafel reaction pathway is not favorable. In contrast, the W@N2C2 catalyst favors the Volmer–Tafel reaction pathway with an activation barrier of 1.15 eV due to the favored direct migration of adsorbed H-atoms to form molecular H2. These results indicate that the accelerated HER kinetics is tightly associated with the coordination geometry of the active site and the spatial alignment of reaction intermediates, which intimately govern the overall HER performance of catalysts.
3.3 Machine learning modelling
ML is becoming more popular for the semi-automated and quantitative discovery of data correlations in chemistry and materials science.45,61,62,65,84 Although many data-driven strategies are being successfully utilized in heterogeneous catalysis,79,85 ML still remains at an early stage due to numerous underexplored material data for water electrocatalysis. We adopted a stepwise multistage screening strategy for discovering promising electrocatalysts with enhanced HER activities. We employed different ML classification and regression models for the prediction of Estab, Udiss and HER differential Gibbs free energies. Fig. S2† demonstrates our stepwise feature selection strategy and ML protocol for training, testing and validation of different ML models used in this work.
3.3.1 Intrinsic descriptors.
A core challenge in ML is to effectively encode the material structure into unique and useful representations which can be learned by the ML model for the prediction of the desired target. In order to identify the correct structure–activity relationship, we constructed various descriptors which provide unique fingerprints for predicting stabilities and activities of catalysts. Inspired by previous studies,45,62,65 we consider four classes of feature sets (Table S12†) including (a) twelve elemental features from periodic table properties, (b) eight features based on the coordination type and surface composition, (c) five top-ranked SISSO generated features (see ESI Note 2 and Table S11†), and (d) four DFT derived features. Additionally, we use a Coulomb matrix as a descriptor which requires a set of nuclear charges (Zi, and Zj) and the corresponding Cartesian coordinates (ri, and rj) as inputs for representing geometric structures, given by the following eqn (9): | 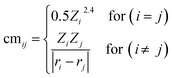 | (9) |
Being a local descriptor, cmij facilitates the comparison of chemical environments around adsorption sites within a certain cutoff radius (rcut). To be constrained specifically on the metal site and its neighboring non-metal active sites we calculated cmij at three cutoff radii (3, 4 and 5 Å). As the Coulomb matrix is symmetric with off-diagonal elements corresponding to the Coulomb repulsion between atoms i and j, while diagonal elements correspond to a polynomial fit of atomic self-energies to nuclear charges, we considered nine Coulomb matrix elements from the upper triangular part of the total-sorted elements within a 4 Å (optimum) rcut. For training our ML models we adopted a stepwise feature selection strategy by taking into account feature–feature correlation and feature importance (Fig. S13†) which are calculated by using the Pearson correlation coefficient and mutual information method, respectively (Fig. 4a and b). Low-ranked features with high feature–feature correlation values (>0.6) are removed from our feature set, and after preprocessing, the final features were imported for training different ML models.
 |
| Fig. 4 ML analysis for prediction of durable high performing electrocatalysts. (a) The heatmap of Pearson correlation coefficient matrix among the selected 21 features/descriptors (see Tables S11 and S12†). The intensity of colors represents the direct correlation (E: H-adsorption/free energy). (b) The most important features ranking predicted by mutual information (MI) method. (c) Receiver operating characteristic (ROC) curve for ERT-classifier and the corresponding area under the curve (AUC) for classification of Estab and Udiss. The mean ROC is calculated among curves for 100 random splits into the training and validation sets for predicting the thermodynamic and electrochemical stabilities. (d) Comparison of DFT computed ΔGH* values with those of predicted by CatBoost regression model, while the inset of histograms denotes the values calculated by our DFT workflow. | |
3.3.2 ML prediction of catalyst stabilities and HER activities.
We performed ML classification prior to regression, and for training our ML classification model we took the Coulomb matrix element (given in eqn (9)) with labeled site representation as discussed in our previous work (for detail see ESI Note 1†).42 By learning the structure–stability relationship of initially computed ∼364 mono- and dual type non-metal doped catalysts, we constructed a ML classification model that successfully classified the catalysts based on Estab and Udiss values. Out of twelve different ML classification models, the ERT-Extremely Randomized Trees classifier showed the best ROC-AUC scores of 0.87 and 0.93 for the prediction of Estab and Udiss, respectively, showing good capability of classification (Fig. 4c and S14†). After successfully training and validating our classification model, we generated ∼140 novel structures with different non-metal doped configurations and predicted their stabilities through our trained ML model (see Fig. S15†). Out of 140 systems, only 41 candidates passed the stability criteria and we found that our model predicted most of the mono- and dual-type phosphorous doped P2C2, P2B2, P2N2 and B2Nc2 structures into an unstable class. We further performed DFT calculations for a few cases and found distorted geometries (Fig. S16†) with stability values out of the bound space (i.e. Estab < 0 and Udiss > 0) and hence we excluded such systems from our dataset before proceeding to the next screening step. The next section describes how we constructed the ML regression model and performed the prediction analysis for HER activities of these 41 stable candidates.
After classifying all the catalysts into stable and unstable classes, in the second step we constructed a ML regression model for the prediction of HER activities. It is worth noting that our input properties for training the ML regression model are ideally available and require only one step calculation of H-adsorbed geometry optimization. For learning the structure–activity relationship we characterized the local atomic and electronic environment of active sites by using various electronic and structural descriptors in combination with the elemental feature set from periodic table properties. Unlike the classification problem, here we used the Coulomb matrix (cmij) descriptor based on DFT-optimized geometries. For training our ML regression model we used a DFT-fitted model with a set of total 21 features, including 6 elemental features (Z: atomic number, rcov: covalent radius, χ: Pauling electronegativity, mp: melting point, rv: radius of the last occupied valence orbital, and θd: unpaired d-electrons), 4 DFT-derived features (
: average distance from TM to four nearest coordinating atoms, φ: angle between adsorbed hydrogen on TM and the sorted first nearest surface non-metal atom, εho: highest-occupied Kohn–Sham eigenvalue and εlu: lowest-unoccupied Kohn–Sham eigenvalue), 2 SISSO generated features (s2 and & s3) and 9 Coulomb matrix elements (cm1 through cm9). The features presented in Fig. 4a and b are the ones that we finally imported to train different ML regression models. The root-mean-square error (RMSE) and the coefficient of determination values (R2) for each model are demonstrated in Fig. S17.† Among the nine different ML regression models which we tested for prediction of HER activities, the CatBoost regression model exhibited the highest accuracy with 0.18 eV RMSE and 0.88 R2 score. Fig. 4d demonstrates an obvious linear relationship in the whole data set, indicating that the predicted results of the CatBoost regression model are in good agreement with DFT-calculated data. In order to examine the accuracy of our model, we conducted more predictions on 41 stable candidates (see Fig. S15 and S18†). The model predicted that Sc@P3, Fe@P3, Zr@P3, Au@P3, Cr@P4, Mn@P4, Fe@P4, Nb@P4, Mo@P4, Ru@P4 and Ir@P4 are high performance HER electrocatalysts. We further performed DFT calculations for these candidates and compared the results with the ML predicted values (see Table S14†). The small mean square error (0.012 eV) demonstrates the great reliability of our model. To encode the significance of the ML model and interpret the contribution of each feature/descriptor, we adopt the analysis framework of SHAP (Shapley Additive exPlanation).86 The SHAP values provide a deep insight into the prediction results. We computed the SHAP values and mean absolute SHAP values for each feature/descriptor, which are presented in Fig. S19.† It is worth noting that the features εlu, cm2, s2 and φ have the highest mean absolute SHAP values and these features are considered to be more critical in the model and have great impact on the prediction results. The feature values of εlu, cm2 and s2 show a negative correlation with the SHAP values, while φ shows a positive correlation with the SHAP values. The mean absolute SHAP values for cm7, cm8, cm9 and Z demonstrate that these features have the lowest impact on the prediction performance of the model. However, we observed that removal of any single feature from the set of 21 selected features decreases the overall prediction performance. Therefore, it is important to keep all these features for the future prediction analysis of high performance HER catalysts.
3.3.3 Deciphering the structure–activity relationship.
Given that the structural stability and catalytic activities are mainly correlated with the surface electronic structure properties and d-band level of TMs, we calculated the local charge distribution and density of states (DOS) near the Fermi level to probe the surface–adsorbate interaction. Fig. 5a illustrates the schematics for H-adsorption on the catalytic surface. Generally, during the HER reaction, the proton from the electrolyte solution interacts with the active site on the catalyst surface and their hybridized states split into bonding (σ) and anti-bonding states (σ*) across the Fermi level. The fully occupied bonding states and empty or partially filled anti-bonding states usually represent the optimal structural stability, however, the excellent catalytic activity may arise from free electrons near the Fermi level. From our DFT calculated results, we found that most of the structures with a low spin state demonstrated good stabilities, and so we compared the computed spin state values for each converged configuration before and after H-adsorption, which are listed in Table S5.† In order to quantitatively estimate the charge transfer between adsorbed H and the catalyst surface, we carried out the Bader charge analysis. Herein, as an example, we considered Ti/Co/Rh and Pd embedded in the N2C2 moiety, each structure exhibiting a different kind of H-adsorbed optimized configuration, i.e. [Ti: slightly pop-up structure with H at the metal top, Co: planar structure with H at the metal top, Rh: slightly pop-up structure with H at the Rh–C bond edge site and Pd: planar structure with H at the Pd–C bond edge site]. The Bader charge profile revealed that an intermediate value of charge transfer from surface → H (0.52 e−) in the Ti@N2C2 system resulted in an optimal H-adsorption strength with an exothermic adsorption free energy value (ΔGH* = −0.10 eV), while the Co@N2C2 system showed a very little charge transfer (0.04 e−) from surface → H and exhibited a weak H-adsorption strength with an endothermic adsorption free energy value (ΔGH* = 0.06 eV). Similarly, Rh@N2C2 also exhibited a very small charge transfer (0.06 e−) from surface → H with an exothermic adsorption free energy value (ΔGH* = −0.15 eV). Remarkably, Pd@N2C2 showed H → surface charge transfer (0.10 e−) and exhibited a relatively poor HER activity (ΔGH* = −0.37 eV). Fig. 5b and S20† display the isosurfaces of differential charge density distribution (Δρ = ρH* − ρ* − ρH), where the ρ* and ρH* represent the charge densities of pure and H-adsorbed surfaces, respectively, while ρH indicates the charge density of adsorbed hydrogen atoms. To gain deep insight into the catalytic activity we calculated the d-band center (εd) and PDOS before and after H adsorption, which are presented in Fig. 5c, d and e, respectively. As indicated in Fig. 5c, the d-band center (εd) of Ti@N2C2 is located at 0.44 eV relative to the Fermi level, while downshifts of −0.51, −1.70 and −1.58 eV from the Fermi level are observed for Co@N2C2, Rh@N2C2 and Pd@N2C2 systems, respectively. As can be seen from Fig. 5c–e, the d-states are more localized near the Fermi level for Ti@N2C2 and Co@N2C2 systems, implying the strong hybridization between the H-s orbital and Ti/Co dz2 orbitals, and these structures showed the metal top as an optimal binding site for H-adsorption. However, for the cases of Rh@N2C2 and Pd@N2C2 surfaces the d-band center (εd) is located far away from the Fermi level. Hence, the major orbital hybridization in these systems occurs between the H-s orbital and C-p orbitals, rendering the M–C bond edge site more active for H adsorption on Rh@N2C2 and Pd@N2C2 structures, which is also obvious in Fig. 5b that the H is adsorbed at the M–C bond edge site with an oblique angle of ∠H–Rh–C 61.4° and ∠H–Pd–C 30.5° at Rh@N2C2 and Pd@N2C2 structures, respectively. These findings suggest that the distinctive charge transfer behavior facilitates the HER activity.
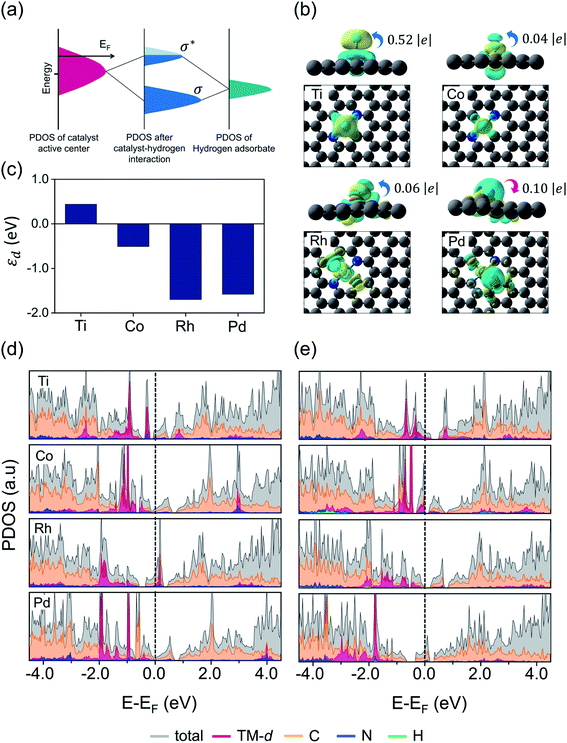 |
| Fig. 5 Correlation between electronic structure and electrochemical properties. (a) Schematic diagram of orbital hybridization of catalyst and HER reaction intermediates. EF is the Fermi level of the substrate, σ and σ* indicate bonding and anti-bonding states, respectively. (b) Isosurfaces of differential charge density distribution for chemisorbed H atom on (Ti/Co/Rh/Pd) N2C2 systems. The charge depletion and accumulation are depicted as cyan and yellow colors, respectively. The isosurface value is 0.003 e Å−3. (c) d-Band center, total and projected density of states (PDOS) for (d) pristine and (e) H-adsorbed (Ti/Co/Rh/Pd) N2C2 systems. | |
4. Conclusion
In summary, by means of the DFT combined ML framework, we demonstrated that mono- or dual-type non-metal (B, N & P) doping in a proper choice of support (g-C3N4, 2DCP, graphene and hexagonal boron nitride) can substantially enhance the HER activity and stability. In order to unveil the structure–activity relationship, we constructed various kinds of electronic and geometric descriptors which work universally for these systems. Our descriptors for prediction of stabilities are easily accessible and exclusively based on elemental properties, however for the prediction of HER activities we used the H-adsorbed converged geometry for creating Coulomb matrix elements. Besides this we also employed a SISSO method to create more feature space. Based on our ML analysis we found that ERT-Extremely Randomized Trees classifier showed the best ROC-AUC scores of 0.87 and 0.93 for the prediction of Estab, and Udiss, respectively, while the CatBoost regression model predicted the HER activities with a minimum test RMSE of 0.18 eV and 0.88 R2 score. Moreover, through our DFT analysis we elucidated the potential dependence of the coordination environment and charge transfer behavior on activation energy values of different reaction mechanisms of the HER. Out of ∼364 catalysts, we found 20 most promising catalysts which exhibited excellent stabilities and superior activities toward the HER. Particularly, Pd@B4, Ru@N2C2, Pd@B2C2, Pt@B2N2, Ir@h-BN, Fe@C3, Rh@C3, and Pd@2DCP and ML-recommended systems Fe@P3, Mn@P4 and Fe@P4 exhibited an ultra-small magnitude of the HER overpotential (ηHER ≅ −0.01 to −0.03 V), much better than that of commercial Pt based catalysts. We believe that our established DFT based ML framework should be equally applicable to other 2D systems and spur both theoretical and experimental research in a wider effort to explore ideal HER catalysts.
Author contributions
M. U. performed the DFT calculations and ML analysis. S. U. helped in ML analysis. M. Z., M. H., A. R. A. H. and A. A. helped in analysis. M. U. drafted the manuscript and K. S. K. revised it. G. L. and K. S. K. supervised the project.
Conflicts of interest
There are no conflicts to declare.
Acknowledgements
This work was supported by Basic Science Research Program (2021R1I1A1A01050280, 2021R1I1A1A01050085, 2021R1A2C1006039 and 2019R1A4A1029237) through National Research Foundation of Korea (NRF) funded by the Ministry of Education. It was also supported by the A.I. Incubation Project Fund (1.210091.01) of UNIST (Ulsan National Institute of Science & Technology). The supercomputing resources including technical support are from the National Supercomputing Center KISTI (KSC-2021-CRE-0193, and KSC-2020-CRE-0146).
References
- S. Chu and A. Majumdar, Nature, 2012, 488, 294–303 CrossRef CAS PubMed.
- G. Glenk and S. Reichelstein, Nat. Energy, 2019, 4, 216–222 CrossRef CAS.
- Y. Yang, Y. Qian, H. Li, Z. Zhang, Y. Mu, D. Do, B. Zhou, J. Dong, W. Yan and Y. Qin, Sci. Adv., 2020, 6, eaba6586 CrossRef CAS PubMed.
- J. A. Turner, Science, 2004, 305, 972–974 CrossRef CAS PubMed.
- J. N. Tiwari, N. K. Dang, S. Sultan, P. Thangavel, H. Y. Jeong and K. S. Kim, Nat. Sustain., 2020, 3, 556–563 CrossRef.
- S. Cobo, J. Heidkamp, P.-A. Jacques, J. Fize, V. Fourmond, L. Guetaz, B. Jousselme, V. Ivanova, H. Dau and S. Palacin, Nat. Mater., 2012, 11, 802–807 CrossRef CAS PubMed.
- A. Le Goff, V. Artero, B. Jousselme, P. D. Tran, N. Guillet, R. Métayé, A. Fihri, S. Palacin and M. Fontecave, Science, 2009, 326, 1384–1387 CrossRef CAS PubMed.
- M. Li, Q. Ma, W. Zi, X. Liu, X. Zhu and S. F. Liu, Sci. Adv., 2015, 1, e1400268 CrossRef PubMed.
- N. K. Dang, M. Umer, P. Thangavel, S. Sultan, J. N. Tiwari, J. H. Lee, M. G. Kim and K. S. Kim, J. Mater. Chem. A, 2021, 9, 16898–16905 RSC.
- A. M. Harzandi, S. Shadman, M. Ha, C. W. Myung, D. Y. Kim, H. J. Park, S. Sultan, W.-S. Noh, W. Lee and P. Thangavel, Appl. Catal., B, 2020, 270, 118896 CrossRef CAS.
- H. Jin, S. Sultan, M. Ha, J. N. Tiwari, M. G. Kim and K. S. Kim, Adv. Funct. Mater., 2020, 30, 2000531 CrossRef CAS.
- S. Sultan, M. H. Diorizky, M. Ha, J. N. Tiwari, H. Choi, N. K. Dang, P. Thangavel, J. H. Lee, H. Y. Jeong and H. S. Shin, J. Mater. Chem. A, 2021, 9, 10326–10334 RSC.
- J. N. Tiwari, N. K. Dang, H. J. Park, S. Sultan, M. G. Kim, J. Haiyan, Z. Lee and K. S. Kim, Nano Energy, 2020, 78, 105166 CrossRef CAS.
- L. A. King, M. A. Hubert, C. Capuano, J. Manco, N. Danilovic, E. Valle, T. R. Hellstern, K. Ayers and T. F. Jaramillo, Nat. Nanotechnol., 2019, 14, 1071–1074 CrossRef CAS PubMed.
- A. Bruix, J. T. Margraf, M. Andersen and K. Reuter, Nat. Catal., 2019, 2, 659–670 CrossRef CAS.
- S. Anantharaj, S. R. Ede, K. Sakthikumar, K. Karthick, S. Mishra and S. Kundu, ACS Catal., 2016, 6, 8069–8097 CrossRef CAS.
- S. Sultan, J. N. Tiwari, A. N. Singh, S. Zhumagali, M. Ha, C. W. Myung, P. Thangavel and K. S. Kim, Adv. Energy Mater., 2019, 9, 1900624 CrossRef.
- J. N. Tiwari, A. N. Singh, S. Sultan and K. S. Kim, Adv. Energy Mater., 2020, 10, 2000280 CrossRef CAS.
- S. Li, B. Chen, Y. Wang, M.-Y. Ye, P. A. van Aken, C. Cheng and A. Thomas, Nat. Mater., 2021, 1–8 Search PubMed.
- C. Eames and M. S. Islam, J. Am. Chem. Soc., 2014, 136, 16270–16276 CrossRef CAS PubMed.
- A. Fischer, J. O. Müller, M. Antonietti and A. Thomas, ACS Nano, 2008, 2, 2489–2496 CrossRef CAS PubMed.
- J. N. Tiwari, A. M. Harzandi, M. Ha, S. Sultan, C. W. Myung, H. J. Park, D. Y. Kim, P. Thangavel, A. N. Singh and P. Sharma, Adv. Energy Mater., 2019, 9, 1900931 CrossRef.
- M. B. Stevens, M. E. Kreider, A. M. Patel, Z. Wang, Y. Liu, B. M. Gibbons, M. J. Statt, A. V. Ievlev, R. Sinclair, A. Mehta, R. C. Davis, J. K. Nørskov, A. Gallo, L. A. King and T. F. Jaramillo, ACS Appl. Energy Mater., 2020, 3, 12433–12446 CrossRef CAS.
- F. Yu, H. Zhou, Y. Huang, J. Sun, F. Qin, J. Bao, W. A. Goddard, S. Chen and Z. Ren, Nat. Commun., 2018, 9, 1–9 CrossRef PubMed.
- W. Cheng, H. Zhang, D. Luan and X. W. D. Lou, Sci. Adv., 2021, 7, eabg2580 CrossRef CAS PubMed.
- C. Wang and L. Qi, Angew. Chem., Int. Ed., 2020, 59, 17219–17224 CrossRef CAS PubMed.
- J. Rossmeisl, Z.-W. Qu, H. Zhu, G.-J. Kroes and J. K. Nørskov, J. Electroanal. Chem., 2007, 607, 83–89 CrossRef CAS.
- A. N. Singh, M. H. Kim, A. Meena, T. U. Wi, H. W. Lee and K. S. Kim, Small, 2021, 17, 2005605 CrossRef CAS PubMed.
- P. Zhai, M. Xia, Y. Wu, G. Zhang, J. Gao, B. Zhang, S. Cao, Y. Zhang, Z. Li and Z. Fan, Nat. Commun., 2021, 12, 1–11 CrossRef PubMed.
- H.-Y. Zhuo, X. Zhang, J.-X. Liang, Q. Yu, H. Xiao and J. Li, Chem. Rev., 2020, 120, 12315–12341 CrossRef CAS PubMed.
- H. Xu, D. Cheng, D. Cao and X. C. Zeng, Nat. Catal., 2018, 1, 339–348 CrossRef CAS.
- S. Bai, M. Yang, J. Jiang, X. He, J. Zou, Z. Xiong, G. Liao and S. Liu, npj 2D Mater. Appl., 2021, 5, 1–15 CrossRef.
- A. Bhat, S. Anwer, K. S. Bhat, M. I. H. Mohideen, K. Liao and A. Qurashi, npj 2D Mater. Appl., 2021, 5, 1–21 CrossRef.
- R. Anand, A. S. Nissimagoudar, M. Umer, M. Ha, M. Zafari, S. Umer, G. Lee and K. S. Kim, Adv. Energy Mater., 2021, 2102388 CrossRef CAS.
- M. Wu and X. C. Zeng, Nano Lett., 2016, 16, 3236–3241 CrossRef CAS PubMed.
- Z. Zhuang, Y. Li, Z. Li, F. Lv, Z. Lang, K. Zhao, L. Zhou, L. Moskaleva, S. Guo and L. Mai, Angew. Chem., 2018, 130, 505–509 CrossRef.
- F. K. Kessler, Y. Zheng, D. Schwarz, C. Merschjann, W. Schnick, X. Wang and M. J. Bojdys, Nat. Rev. Mater., 2017, 2, 1–17 Search PubMed.
- X. Chia and M. Pumera, Nat. Catal., 2018, 1, 909–921 CrossRef CAS.
- D. Y. Kim, M. Ha and K. S. Kim, J. Mater. Chem. A, 2021, 9, 3511–3519 RSC.
- J. Greeley, T. F. Jaramillo, J. Bonde, I. Chorkendorff and J. K. Nørskov, Nat. Mater., 2006, 5, 909–913 CrossRef CAS PubMed.
- C. Zhou, J. Y. Zhao, P. F. Liu, J. Chen, S. Dai, H. G. Yang, P. Hu and H. Wang, Chem. Sci., 2021, 12, 10634–10642 RSC.
- M. Ha, D. Y. Kim, M. Umer, V. Gladkikh, C. W. Myung and K. S. Kim, Energy Environ. Sci., 2021, 14, 3455–3468 RSC.
- M. D. Hossain, Z. Liu, M. Zhuang, X. Yan, G. L. Xu, C. A. Gadre, A. Tyagi, I. H. Abidi, C. J. Sun and H. Wong, Adv. Energy Mater., 2019, 9, 1803689 CrossRef.
- X. Guo, S. Lin, J. Gu, S. Zhang, Z. Chen and S. Huang, ACS Catal., 2019, 9, 11042–11054 CrossRef CAS.
- M. Zafari, D. Kumar, M. Umer and K. S. Kim, J. Mater. Chem. A, 2020, 8, 5209–5216 RSC.
- C. Liu, Q. Li, C. Wu, J. Zhang, Y. Jin, D. R. MacFarlane and C. Sun, J. Am. Chem. Soc., 2019, 141, 2884–2888 CrossRef CAS PubMed.
- M. Zafari, A. S. Nissimagoudar, M. Umer, G. Lee and K. S. Kim, J. Mater. Chem. A, 2021, 9, 9203–9213 RSC.
- C. Ling, Y. Zhang, Q. Li, X. Bai, L. Shi and J. Wang, J. Am. Chem. Soc., 2019, 141, 18264–18270 CrossRef CAS PubMed.
- V. V. Voronin, M. S. Ledovskaya, A. S. Bogachenkov, K. S. Rodygin and V. P. Ananikov, Molecules, 2018, 23, 2442 CrossRef PubMed.
- D. Chakraborty, C. D. Damsgaard, H. Silva, C. Conradsen, J. L. Olsen, H. W. Carvalho, B. Mutz, T. Bligaard, M. J. Hoffmann and J. D. Grunwaldt, Angew. Chem., 2017, 129, 8837–8841 CrossRef.
- Y. Jiao, Y. Zheng, K. Davey and S.-Z. Qiao, Nat. Energy, 2016, 1, 1–9 Search PubMed.
- J.-Q. Chi, X.-Y. Zhang, X. Ma, B. Dong, J.-Q. Zhang, B.-Y. Guo, M. Yang, L. Wang, Y.-M. Chai and C. Liu, ACS Sustainable Chem. Eng., 2019, 7, 17714–17722 CrossRef CAS.
- J. Wan, Z. Zhao, H. Shang, B. Peng, W. Chen, J. Pei, L. Zheng, J. Dong, R. Cao and R. Sarangi, J. Am. Chem. Soc., 2020, 142, 8431–8439 CrossRef CAS PubMed.
- X. Guo, J. Gu, X. Hu, S. Zhang, Z. Chen and S. Huang, Catal. Today, 2020, 350, 91–99 CrossRef CAS.
- G. Galeotti, F. De Marchi, E. Hamzehpoor, O. MacLean, M. R. Rao, Y. Chen, L. Besteiro, D. Dettmann, L. Ferrari and F. Frezza, Nat. Mater., 2020, 19, 874–880 CrossRef CAS PubMed.
- A. Wang, J. Li and T. Zhang, Nat. Rev. Chem., 2018, 2, 65–81 CrossRef CAS.
- Z. W. Seh, J. Kibsgaard, C. F. Dickens, I. Chorkendorff, J. K. Nørskov and T. F. Jaramillo, Science, 2017, 355, eaad4998 CrossRef PubMed.
- J. K. Nørskov, T. Bligaard, J. Rossmeisl and C. H. Christensen, Nat. Chem., 2009, 1, 37–46 CrossRef PubMed.
- M. Grewer and R. Birringer, Phys. Rev. B: Condens. Matter Mater. Phys., 2014, 89, 184108 CrossRef.
- Q. Zhou, S. Lu, Y. Wu and J. Wang, J. Phys. Chem. Lett., 2020, 11, 3920–3927 CrossRef CAS PubMed.
- M. Rupp, A. Tkatchenko, K.-R. Müller and O. A. Von Lilienfeld, Phys. Rev. Lett., 2012, 108, 058301 CrossRef PubMed.
- K. Hansen, F. Biegler, R. Ramakrishnan, W. Pronobis, O. A. Von Lilienfeld, K.-R. Müller and A. Tkatchenko, J. Phys. Chem. Lett., 2015, 6, 2326–2331 CrossRef CAS PubMed.
- A. P. Bartók, R. Kondor and G. Csányi, Phys. Rev. B: Condens. Matter Mater. Phys., 2013, 87, 184115 CrossRef.
- G. Zheng, Y. Li, X. Qian, G. Yao, Z. Tian, X. Zhang and L. Chen, ACS Appl. Mater. Interfaces, 2021, 13, 16336–16344 CrossRef CAS PubMed.
- M. O. Jäger, E. V. Morooka, F. F. Canova, L. Himanen and A. S. Foster, npj Comput. Mater., 2018, 4, 1–8 CrossRef.
- R. Ouyang, S. Curtarolo, E. Ahmetcik, M. Scheffler and L. M. Ghiringhelli, Phys. Rev. Mater., 2018, 2, 083802 CrossRef CAS.
- G. Kresse and J. Furthmüller, Phys. Rev. B: Condens. Matter Mater. Phys., 1996, 54, 11169 CrossRef CAS PubMed.
- G. Kresse and D. Joubert, Phys. Rev. B: Condens. Matter Mater. Phys., 1999, 59, 1758 CrossRef CAS.
- J. P. Perdew, K. Burke and M. Ernzerhof, Phys. Rev. Lett., 1996, 77, 3865 CrossRef CAS PubMed.
- P. E. Blöchl, Phys. Rev. B: Condens. Matter Mater. Phys., 1994, 50, 17953 CrossRef PubMed.
- A. Tkatchenko and M. Scheffler, Phys. Rev. Lett., 2009, 102, 073005 CrossRef PubMed.
- K. Mathew, R. Sundararaman, K. Letchworth-Weaver, T. Arias and R. G. Hennig, J. Chem. Phys., 2014, 140, 084106 CrossRef PubMed.
- J. K. Nørskov, J. Rossmeisl, A. Logadottir, L. Lindqvist, J. R. Kitchin, T. Bligaard and H. Jonsson, J. Phys. Chem. B, 2004, 108, 17886–17892 CrossRef.
- J. K. Nørskov, T. Bligaard, A. Logadottir, J. Kitchin, J. G. Chen, S. Pandelov and U. Stimming, J. Electrochem. Soc., 2005, 152, J23 CrossRef.
- M. Dwass, Ann. Math. Stat., 1957, 181–187 CrossRef.
- J. Greeley and J. K. Nørskov, Electrochim. Acta, 2007, 52, 5829–5836 CrossRef CAS.
- X. Guo, J. Gu, S. Lin, S. Zhang, Z. Chen and S. Huang, J. Am. Chem. Soc., 2020, 142, 5709–5721 CrossRef CAS PubMed.
- G. J. Martyna, M. L. Klein and M. Tuckerman, J. Chem. Phys., 1992, 97, 2635–2643 CrossRef.
- A. Hajibabaei and K. S. Kim, J. Phys. Chem. Lett., 2021, 12, 8115–8120 CrossRef CAS PubMed.
- A. Hajibabaei, C. W. Myung and K. S. Kim, Phys. Rev. B, 2021, 103, 214102 CrossRef CAS.
- P. Sabatier, Chem. Ber., 1911, 44, 1984–2001 CrossRef CAS.
- M. Park, I. Shin, N. J. Singh and K. S. Kim, J. Phys. Chem., 2007, 111, 10692–10702 CrossRef CAS PubMed.
- G. Di Liberto, L. A. Cipriano and G. Pacchioni, J. Am. Chem. Soc., 2021, 143(48), 20431–20441 CrossRef CAS PubMed.
- X. Wang, S. Ye, W. Hu, E. Sharman, R. Liu, Y. Liu, Y. Luo and J. Jiang, J. Am. Chem. Soc., 2020, 142, 7737–7743 CrossRef CAS PubMed.
- A. Abbas, L. Boithias, Y. Pachepsky, K. Kim, J. A. Chun and K. H. Cho, Geosci. Model Dev. Discuss., 2021, 1–29 Search PubMed.
- S. M. Lundberg, G. Erion, H. Chen, A. DeGrave, J. M. Prutkin, B. Nair, R. Katz, J. Himmelfarb, N. Bansal and S.-I. Lee, Nature Machine Intelligence, 2020, 2, 56–67 CrossRef PubMed.
Footnote |
† Electronic supplementary information (ESI) available. See DOI: 10.1039/d1ta09878k |
|
This journal is © The Royal Society of Chemistry 2022 |