Motivation to learn chemistry: a thorough analysis of the CMQ-II within the Brazilian context
Received
18th April 2022
, Accepted 30th May 2022
First published on 30th May 2022
Abstract
Studies on students’ motivation to learn Chemistry in developed countries are largely common in the literature. However, very few studies have been carried out with a view to investigating students' motivation to learn Chemistry in places like Latin America, and specifically in Brazil. The present study sought to translate the Chemistry Motivation Questionnaire II (CMQ-II) with a view to evaluating the motivation to learn Chemistry among Brazilian high school students. The study was conducted using responses obtained from 510 high school students who completed the CMQ-II during the COVID-19 pandemic. Exploratory factor analysis, confirmatory factor analysis and analysis of model invariance between genders were carried out in order to compare the results obtained with those of the other studies conducted using the CMQ-II. The results obtained from this investigation showed that the CMQ-II had a five-factor structure with factor loadings ≥0.52 and Cronbach's α ≥ 0.81. Multivariate analysis was also carried out with respect to gender differences, and the results obtained showed that Brazilian boys had higher confidence when it comes to learning Chemistry, while the girls mostly studied Chemistry to obtain a good grade or because of the importance of the discipline in their imagined future career. The findings show that the Brazilian version of CMQ-II applied in this study seems to provide a consistent scale of analysis compared to that reported in the literature.
Introduction
Conducting an investigation on students’ motivation to learn is somewhat tantamount to asking the following questions: “why do students do what they do? And what are the forces behind their behaviour?” In Science Education, this sort of investigation implies questioning what drives the students’ interest to learn a science-related subject. Students’ motivation to study science has been an important topic of international and national concern. This is especially relevant in light of some findings from previous studies in science education which show that students’ motivation to learn may be in decline as they progress from one grade to another, from primary school to high school (Vedder-Weiss and Fortus, 2011; Fortus and Vedder-Weiss, 2013), and this can greatly affect their persistence to learn (Lavigne et al., 2007) and amplify gender differences in science-related courses (Patall et al., 2018). Also, as the decision to pursue a science-related career can be made during the student's initial experience with science at school (Tai et al., 2006), identifying students’ reasons to pursue science-related disciplines may provide teaching practitioners/educators with some indication and useful insights into how to improve the learning experience and stimulate students’ desire and aspirations to study science and pursue a science-related career (DeWitt and Archer, 2015). In addition, previous studies reported in the literature have shown that students’ aspirations to learn science and chemistry are positively related to both extrinsic and intrinsic forms of motivation (Mujtaba et al., 2018). Although very few studies on motivation to learn have devoted their attention to chemistry-related subjects, researchers have successfully identified some existing differences in students’ motivation to learn in each of the sub-disciplines in science (Simpkins et al., 2015; Salta and Koulougliotis, 2020). In this context, investigating the reasons behind students’ desire to learn in Chemistry-related classes is conceivably relevant.
In Latin America, because of the students’ relatively low performance in international tests like the Programme for International Student Assessment (PISA), Brazil can be considered a particularly pertinent case when it comes to investigating students’ motivation to study science/chemistry. For instance, the analysis of the PISA results shows that while Brazilian students tend to enjoy learning science and perceive it as useful for their future (OECD, 2016), they also still perform lower than average in the subject in comparison to students from other countries (OECD, 2016). As motivation can be directly related to achievement (Ferrell et al., 2016), investigating and gaining a holistic understanding regarding the forces that drive students’ engagement and participation in science/chemistry can clearly help improve the students’ motivation to learn. Unfortunately, Brazilian researchers lack the needed quantitative instruments that would allow them to conduct a comprehensive investigation on students’ motivation to learn Chemistry at any level of education in Brazil; as a result, unlike their counterparts in developed countries, it is undoubtedly difficult for Brazilian researchers to clearly identify the reasons behind students' engagement or disengagement in Chemistry-related subjects and to find out why some high school students choose to study Chemistry at the university/higher education level while other students do not. Furthermore, the lack of adequate quantitative instruments in Brazil and in other countries in Latin America poses considerable limitations for cross-cultural research targeted at gaining a more nuanced understanding of students’ motivation to learn beyond some specific contexts (Wang et al., 2020). Taking the above considerations into account and the impossibility of generalizing some research findings across countries (Artelt, 2005), the present study sought to translate and study the applicability and functioning of a Brazilian version of the Chemistry Motivation Questionnaire II (CMQ-II), which is intended to investigate the motivation to learn Chemistry among high school students in Brazil.
Theoretical framework: the social-cognitive theory
The social-cognitive theory (SCT), proposed by Bandura (1986, 1989), studies how people can be cognitive and maintain certain patterns of behaviour; in essence, the theory seeks to understand how social people are or their behaviour in social settings. According to the SCT, depending on their living circumstances, individuals are self-organized, proactive, self-regulating and self-reflexive beings who are capable of contributing to life situations and not just being products of the circumstances of life (Bandura, 2005). These individuals acquire knowledge through direct observation, social interactions and experiences, as well as through external influences. Stakeholders in a given social setting are proactively involved in their own development and can determine the results of their actions. For Bandura (2002), the functioning of an individual is the result of their personal interactions, external influences and behaviour. Based on the SCT, learning can take place through the direct action of the individual or through observation of other people's behaviour. Bandura emphasizes the active role played by the individual as an agent in their social interactions, where they exercise a voluntary control of their actions with a view to their self-development, adaptation and change. Learning does not take place in the presence of external influences, such as corporal punishment, experiences, and other reinforcement tools, but rather through direct and indirect behavioural observation. In this context, human flexibility allows them to learn concepts, attitudes, skills and behaviours, and human actions are the result of the interaction involving three dependent variables: the environment, human behaviour and personal factors (cognitive and affective dimensions) – referred to as the triadic reciprocity (Bandura, 2002; Pajares and Olaz, 2008).
Individuals' perceptions of their competence to act in a given domain are formed through their interactions with the environment, as in the self-efficacy construct in the SCT. According to Bandura (1986), self-efficacy beliefs are judgments about one's capacity to perform an action and to achieve a set of goals as a reflection of one's choice and persistence during a task, and the efforts in acquiring abilities and knowledge. Self-efficacy beliefs can be developed in the following ways: (i) through direct experiences during the development of a task; (ii) through vicarious experiences as in the observation of social models in which self-efficacy perceptions are influenced by the similarities between one's self and the behaviour of the role models; (iii) in social persuasion with reinforcement or weakening of self-efficacy beliefs; and (iv) through emotional and physical states (Bandura, 2002; Pajares and Olaz, 2008).
Motivation is an important element in this theory; this element can be understood as an internal experience, be it a need, a cognition or an emotion, which energizes and directs an individual's behaviour (Ryan and Deci, 2000; Broussard and Garrison, 2004; Reeve, 2009). Generally, motivation is divided into two categories: intrinsic motivation and extrinsic motivation. Extrinsic motivation depends on external variables, while intrinsic motivation depends on internal variables of the individual. Motivation to learn chemistry, career motivation and grade motivation can be considered as extrinsic motivations, as individuals expect something in exchange for their engagement or efforts. On the other hand, self-efficacy and self-determination are elements of an intrinsic motivation. Self-determination can be seen as a relevant factor in learning Chemistry for personal goals and for the responsibility one takes as they trail this path.
While it is true that understanding students’ motivation to learn is essentially fundamental when it comes to planning and decision making in the school environment, studies in the field of educational psychology have pointed out the intrinsic complexities surrounding this issue and the importance of the importance of investigating the validity and reliability of the data collected by instruments used in new contexts for the proper assessment of motivation. In science and chemistry education, some of the instruments used for evaluating students’ motivation include the Science Motivation Questionnaire (SMQ) and its chemistry-specific version.
The science motivation questionnaire (SMQ)
Motivation is conceptualized in cognitive social theory as a multidimensional construct. Several attempts have been made in developing suitable instruments for measuring motivation. Glynn and Koballa (2006) developed the Science Motivation Questionnaire (SMQ), which consisted of six components and 30 items used for measuring the motivation of university students to learn science. In a study carried out to assess the psychometric properties of the SMQ, Glynn et al. (2009) gave support to the validation of the data collected by this analytical tool and obtained some relevant indicators that can help improve the scales for assessing the dimensions of motivation.
More recently, Glynn et al. (2011) presented the second version of the Science Motivation Questionnaire (SMQ-II), which consisted of the following five components: intrinsic motivation, self-determination, self-efficacy, career motivation, and grade motivation. Intrinsic motivation is related to a student's innate interest or desire to learn science (Deci and Ryan, 1985). Self-determination is linked to the control that students believe they have when they learn science (Black and Deci, 2000). Self-efficacy is used to describe students' confidence in displaying a good performance when it comes to science learning (Lawson et al., 2007). Unlike the three subscales described above, grade motivation and career motivation are extrinsic motivational constructs. For each of the items in the questionnaire, the participants/subjects of the research must decide the frequency of occurrence of the situations on a 5-point Likert scale, where 1 = “never”, 2 = “rarely”, 3 = “sometimes”, 4 = “normally” and 5 = “always”.
According to the authors of the SMQ-II, the questionnaire can be adapted and used for assessing students’ motivation in other specific disciplines, such as Biology – Biology Motivation Questionnaire II (BMQ-II), Chemistry – Chemistry Motivation Questionnaire II (CMQ-II), and Physics – Physics Motivation Questionnaire II (PMQ-II) (Glynn et al., 2011). As can be noted, for adaptation purposes, the word science is replaced by biology, chemistry and physics.
With a total of 25 items, the evidence shows the SMQ-II is suitable for the assessment of students’ motivation to learn science and the effectiveness of teaching strategies and materials designed to improve students' motivation. The questionnaire has been translated into different languages, and has been adapted and its functioning investigated for other science disciplines and different levels of education.
It is worth noting that the SMQ-II was initially developed for university students; however, other authors have successfully been able to extend its application to high school students (Salta and Koulougliotis, 2015; Schumm and Bogner, 2016; Ardura and Pérez-Bitrián, 2018; Covert et al., 2019). Ardura and Pérez-Bitrián (2018) and Salta and Koulougliotis (2015) used the instrument to assess high school students' motivation to learn chemistry. The psychometric properties of the instrument have also been investigated and translated to the Chinese (Dong et al., 2020), Indonesian (Wardhany et al., 2018), Spanish (Ardura and Pérez-Bitrián, 2018), German (Schumm and Bogner, 2016), and Greek (Salta and Koulougliotis, 2015) contexts.
Salta and Koulougliotis (2015) translated and adapted the SMQ-II to the Greek context where they applied the instrument for the analysis of students’ motivation to learn chemistry through comparisons in terms of gender and age. The findings of the study conducted by Salta and Koulougliotis (2015) showed that boys had lower self-determination relative to girls of the respective age group. Interestingly, similar findings were reported by Glynn et al. (2011), Schumm and Bogner (2016), and Ardura and Pérez-Bitrián (2018). With regard to age-based comparison, Salta and Koulougliotis (2015) showed that upper secondary school students exhibited lower grade motivation compared to lower secondary school students.
Komperda et al. (2020) carried out a statistical analysis of a modified version of the SMQ-II in general biology and chemistry courses with the aim of finding out whether the instrument could still provide consistent structural evidence when some items were changed, especially considering some inconsistencies in the instrument throughout the literature. The authors also compared the structure of the questionnaire with the words “science”, “biology” and “chemistry” (Komperda et al., 2020). After removing and modifying some problematic items, the results obtained showed acceptable data model fit for the five-factor structural model in most of the conditions investigated by the authors (considering wordings and courses), particularly when using the robust diagonally weighted least squares (WLSMV) estimator, compared to the use of the robust maximum likelihood which showed more inconsistent results (Komperda et al., 2020).
It is worth noting that the validation of the data collected by an instrument in other countries are required to undergo statistical analysis so as to verify its appropriateness for application within the national context of interest. To evaluate the effects of differences between gender on motivation to learn chemistry, the Brazilian version of CMQ-II was tested as a suitable instrument for assessing motivation among Brazilian high school students. The Brazilian version of the instrument was also analyzed in order to study the variation in outcomes. It is also part of this study intention to contribute to the cross-cultural literature which aim to provide robust evidence in support of the CMQ-II use for evaluating students’ motivation to learn chemistry. In this paper, we intend to answer the following research questions:
1. Does data from the Brazilian version of the CMQ-II show evidence for the structural validity of this instrument to measure the motivation to learn Chemistry among high school students in Brazil?
2. How can the motivation of high school students be characterized in terms of gender?
Research design
Context: chemistry education in Brazil
Brazil is a large continental country with considerable regional and educational differences. Education in Brazil is provided by private educational institutions (self-funded) or by public educational institutions (publicly funded). The public funding of education in Brazil occurs through different levels of funding and is paid for by different governmental organizations, including municipal, state and federal funding institutions. Brazilian census data from 2021 indicates that 32.2% of all students were enrolled in state funding schools, 49.6% in municipal funding schools, while 17.4% were enrolled in private funding schools, and less than 1% of the total enrolments were in federal funding schools (Brasil, 2021). The Brazilian educational system is fundamentally divided into primary (nine years) and secondary education levels. The secondary education level covers the three-year high school period, which can be complemented by vocational and technical studies offered in federal institutes funded by the federal government. Although some basic chemistry concepts are presented to the pupils in their last year of studies in primary schools, chemistry is only systematically taught at the secondary school level. Unlike the curriculum structure of other countries such as the US, the high-school curriculum in Brazil is largely discipline-focused and chemistry is a mandatory subject for all students during the three years of high school, regardless of the type of institution (private, state or federal institutions). In this sense, when investigating high-school students’ motivation to learn chemistry in Brazil, the reference of analysis is specifically centered around the chemistry classes taken by students in high school and it is not confined to the chemistry contents in science classes or in a general field per se in which chemistry is included.
Questionnaire translation
First, the authors of the SMQ II were contacted to check whether there was a Brazilian version of the instrument and to seek their permission to translate the instrument culturally to suit the Brazilian context. As pointed out above, the SMQ II can be adapted to specific disciplines by replacing the word “science” in each item with the name of the discipline of interest. The authors of the Brazilian version of the instrument replaced “science” with “chemistry” throughout the questionnaire. The questionnaire items were initially translated independently into Brazilian Portuguese by three bilingual translators in order to create the Brazilian version of the Chemistry Motivation Questionnaire II (CMQ-II), which consisted of a Likert-type scale ranging from 1 (never) to 5 (always).
After that, two doctoral students, both of whom were familiar with education, motivation theories and literature on questionnaire development, back-translated the questionnaire into English. The doctoral students compared the back-translated content with the translated version and the original items. Subsequently, a specialist (with a PhD degree) in chemistry education who is a Full Professor of Chemistry at the University of Sao Paulo (for over nine years) compared the translated version of the instrument with the original version. A consensus was then reached on the final form of the translated scale, and the meaning equivalence of the words in the original and the translated versions of the CMQ-II was thoroughly confirmed. Attempts were made in order to ensure a balance between a literal and a culturally specific translation of the words in the instrument so that the translation of items were duly appropriate for Brazilian students within the Brazilian context.
Procedures
Due to the COVID-19 pandemic, data collection was carried out between August 2020 and November 2021 – during the quarantine period in Brazil, with the aid of Google Forms. High school teachers across the country were contacted during this period. The teachers were thoroughly informed about the study objectives and the methodology adopted for carrying out the study. The high school students from the participating institutions were also informed about the study and were invited to complete an on-line survey through a link that was sent to them by the teachers. The first part of the survey was related to the aim of the research and the Terms of Free and Informed Consent (TFIC). The students were only allowed to respond to the survey once they have consented to participate. The students were informed that none of the results of the investigation would influence their school grades and were thanked for their collaboration.
The study sample consisted of 514 responses obtained from Brazilian high-school students. Out of the total number of students who took part in the survey, 38.1% were boys (n = 196), 60.5% were girls (n = 311), and 1.4% were classified as others (n = 7). The sample race/ethnicity was distributed between 54.9% White (Branco) (n = 282), 29.6% Brown (Pardo) (n = 152), 10.1% Black (Preto) (n = 52), 1.2% Indigenous (Indígena) (n = 6), 1.6% Yellow (Asians) (n = 8), and 2.7% (n = 14) were not racially/ethnically identified. The participating students came from forty-two schools distributed between three main types of institutions: 37.9% from federal-funded schools (n = 195), 37% from private-funded schools (n = 193), and 24.3% from state-funded schools (n = 125).
Data analysis
Data analyses were performed in three stages. Initially, prior to performing the main statistical analyses, all the data collected was screened for normality and possible outliers. Univariate normality was assessed by examining the indicators of skewness and kurtosis for each motivational construct. We considered that values lower than a magnitude of 2 implied that the data did not significantly depart from a normal distribution (Pituch and Stevens, 2015). The data was then examined for univariate outliers using z-scores. Z-values above or below ±3.29 were considered univariate outliers (Tabachnick and Fidell, 2001). Multivariate outliers were examined by calculating Mahalanobis distances (p < 0.001).
After initial screening of the data, the statistical analyses of the data were performed using Exploratory Factor Analysis (EFA), Confirmatory Factor Analysis (CFA) and Model Invariance Analysis in order to confirm whether the variables remained intact after the translation of the instrument (Hair et al., 2009). The EFA was conducted using principal components analysis (PCA) and the factors were extracted using the Kaiser–Guttman rule, selecting only the factors with eigenvalues greater than 1 and resorting to a scree-plot to check the solution. Following the guidelines of several authors (Brown, 2006; Hair et al., 2009; Blunch, 2008), the criteria used to determine the factors used were: criteria of Kaiser–Meyer–Olkin test (KMO) with values greater than 0.60 and Bartlett's sphericity test with p < 0.00. Factorial loading of items ≥0.40 was established. Inexistence of items with factor weights with some relevance (>0.40) in more than one factor. If this happens and if the difference between them is significant (cross-loadings ≤0.10), it is suggested that the item be eliminated. The following conditions were employed for the analysis: criteria of Kaiser–Meyer–Olkin test (KMO) with values greater than 0.60 and Bartlett's sphericity test with p < 0.00. Factorial loading of items ≥0.35 was established. The variance explained by the factors retained was at least 40%. The total internal consistency and internal consistencies of the subscales were also calculated (Cronbach's α) using the criteria of values ≥0.70. The alpha coefficient was calculated based on raw scores (Cronbach, 1951).
The CFA was carried out according to the guidance and recommendations of a wide range of authors (e.g., Hooper et al., 2008; Hair et al., 2009; Byrne, 2010; Kline, 2011). The analysis was carried out based on the maximum likelihood (ML) method, using the chi-squared (χ2) testing of the respective degrees of freedom (df) and the level of significance (p). The following adjustment quality indices were also used: Standardized Root Mean Square Residual (SRMR), Comparative Fit Index (CFI), Tucker–Lewis Index (TLI), Root Mean Square Error of Approximation (RMSEA), and the respective Confidence Interval (90% CI). The most known cutoff values are from Hu and Bentler (1999). They conducted simulation studies and recommend some cutoff values considered as a good fit for a model. These values are: SRMR close to 0.08 or below; RMSEA close to 0.06 or below; and CFI and TLI close to 0.95 or more. There are others recommendations, such as ranges of fit index values. MacCallum et al. (1996) suggest RMSEAs in the range of 0.08–0.10 would be a “mediocre” fit and RMSEA ≤ 0.08 as a good fit. Steiger (2007) recommended a value of RMSEA ≤ 0.07. Browne and Cudeck (1993) recommended RMSEA between 0.05 to 0.08, with a 90% confidence interval of the RMSEA upper limit below 0.1. CFI and TLI values in the range of or equal to 0.90 and 0.95 can be interpreted as an acceptable model fit (Bentler, 1990). As cited by Brown (2015), there is little consensus on recommended cutoff for a good fit index. So, in this paper a range of adjustment acceptable values is used.
To carry out the model invariance analysis, two groups were formed (Boys vs. Girls) and the following criteria for the model invariance (Putnick and Bornstein, 2016) was adopted: (1) individual CFA groups (where the model is expected to have a good fit in each group); (2) multigroup analysis by restricting the model parameters: free parameters model (i.e., configural invariance), fixed factor loadings (i.e., metric invariance), fixed intercepts and factor loadings (i.e., scalar invariances), fixed factorial, intercepts and error measurement model (i.e., residual invariance). As for the CFA, we chose not-so-conservative cutoff values for model invariance: the difference in values between the configural model (free parameters model) and the restricted model (fixed parameters) should be ΔCFI ≤ 0.01 (Cheung and Rensvold, 2002). ΔRMSEA ≤ 0.015 at all levels of invariance tests (Chen, 2007).
After the instrument structure was established, in the final stage Multivariate analyses of variance (MANOVA) were then conducted in order to examine the gender effect on students’ motivation to learn chemistry. Follow-up analyses of variance (ANOVA) were also performed. In these analyses, differences were considered statistically significant when p < 0.05. Effect sizes were also reported using partial η2 considering 0.01 as a small effect, 0.06 as a moderate effect, and 0.14 as a large effect (Cohen, 1988). Finally, the data was analysed based on descriptive statistics with the calculation of mean, standard deviation and correlations.
Results
Preliminary results
All the variables were found to exhibit values of skewness (from −0.72 to −0.12) and kurtosis (from −1.06 to −0.08) within acceptable ranges; this shows that the variable scores were nearly univariate normally distributed. Univariate outliers were identified in 4 cases in “Grade Motivation”. Mahalanobis distances indicated there were no significant multivariate outliers in this data set (p < 0.001). As these 4 cases indicated some form of systematic answer (e.g. the same response for all items), we decided to remove them from subsequent analyses. This resulted in a sample of 510 students that was used for the conduct of further descriptive and multivariate analyses. For factorial analyses, the total sample was randomly split between two samples for CFA (n = 256) and EFA (n = 254). As it supported the same 5-factor solution for both halves of the data and there were no modifications in terms of removing items, another CFA was done. We used the full data (n = 505), without respondents’ gender classified as “others” (n = 5), for further model invariance analysis.
Main analysis
Principal component analysis.
Using PCA with an orthogonal rotation (varimax), a five-factor structure was extracted from the 25 items which constituted the Brazilian version of the CMQ-II (Table 1 and Fig. 1). Eigenvalues >1.0 (Kaiser, 1960) explained 69.6% of the total variance. The Kaiser–Meyer–Olkin test measured the sampling adequacy of the analysis (KMO = 0.924). Based on the Barlett's test of sphericity, χ2(300) = 4232.373, and p < 0.001. Factor loadings for each item measure the percentage of variance accounted for by the factors. In this study, all items have acceptable factor loadings in their respective factors, all higher than 0.52. As for item Q19, its elimination could be considered, given that this item loads in the SE and IM factors, but the difference between them is not significant (>0.10). The five factors extracted from the data through the PCA and their corresponding explained variance and eigenvalues were as follows: career motivation (42.4%; 10.6), self-efficacy (9.3%; 2.3), self-determination (7.8%; 1.9), intrinsic motivation (5.7%; 1.4) and grade motivation (4.6%; 1.1).
Table 1 Items and factor loadings (n = 254)
Item |
|
CM |
SE |
SD |
IM |
GM |
Q10 |
Knowing chemistry will give me a career advantage |
0.86
|
|
|
|
|
Q13 |
Understanding chemistry will benefit me in my career |
0.83
|
|
|
|
|
Q25 |
I will use chemistry problem-solving skills in my career |
0.82
|
|
|
|
|
Q23 |
My career will involve chemistry |
0.82
|
|
|
|
|
Q7 |
Learning chemistry will help me get a good job |
0.71
|
|
|
|
|
Q9 |
I am confident I will do well in chemistry tests |
|
0.81
|
|
|
|
Q18 |
I believe I can score an ‘‘A’’ in chemistry |
|
0.76
|
|
|
|
Q14 |
I am confident I will do well in chemistry labs and projects |
|
0.73
|
|
|
|
Q15 |
I believe I can master chemistry knowledge and skills |
|
0.72
|
|
|
|
Q21 |
I am sure I can understand chemistry |
|
0.72
|
|
|
|
Q22 |
I study hard to enable me to understand chemistry |
|
|
0.85
|
|
|
Q11 |
I spend a lot of time learning chemistry |
|
|
0.75
|
|
|
Q16 |
I prepare well before going in for chemistry tests |
|
|
0.75
|
|
|
Q5 |
I put enough effort into learning chemistry |
|
|
0.66
|
|
|
Q6 |
I use strategies to learn chemistry well |
|
|
0.52
|
|
|
Q17 |
I am curious about discoveries in chemistry |
|
|
|
0.70
|
|
Q1 |
The chemistry I learn at school is relevant to my life |
|
|
|
0.69
|
|
Q3 |
Learning chemistry is interesting |
|
|
|
0.68
|
|
Q12 |
Learning chemistry makes my life more meaningful |
|
|
|
0.66
|
|
Q19 |
I enjoy learning chemistry |
|
0.44 |
|
0.60
|
|
Q8 |
It is important that I get an ‘‘A’’ in chemistry |
|
|
|
|
0.76
|
Q24 |
Scoring good grades in chemistry tests and labs matters to me |
|
|
|
|
0.72
|
Q2 |
I like to do better than other students in chemistry tests |
|
|
|
|
0.69
|
Q4 |
Getting a good grade in chemistry is important to me |
|
|
|
|
0.68
|
Q20 |
I think about the grade I will get in chemistry |
|
|
|
|
0.55
|
α
|
Total: 0.94 |
0.92 |
0.89 |
0.85 |
0.89 |
0.81 |
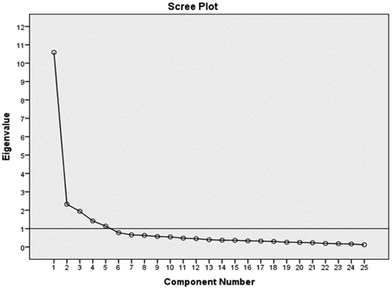 |
| Fig. 1 Scree plot showing five-factor solution as a good fit. | |
Confirmatory factorial analysis
Based on the results obtained in the PCA, we were able to perform Confirmatory Factor Analysis (CFA), with the aid of the SPSS Amos software (version 23), and consistent results were obtained. In order to obtain the best results and explanations for the model, the correlated five-factor model was subjected to four tests: Standardized Root Mean Square Residual (SRMR), Tucker–Lewis Index (TLI), Comparative Fit Index (CFI) and Root Mean Square Error Approximation (RMSEA), which together made it possible to measure the goodness of fit. For this analysis, the following cutoff values were employed: SRMR ≤ 0.08 proposed by Hu and Bentler (1999); RMSEA between 0.05 to 0.08, with a 90% confidence interval of the RMSEA upper limit below 0.1 (Browne and Cudeck, 1993); values of TLI and CFI between or equal to 0.90 and 0.95 (Bentler, 1990). Table 2 and Fig. 2 shows the results obtained which are in line with the reports in the literature. The results for both models represent an acceptable fit.
Table 2 Confirmatory factor analysis results
Model |
χ
2
|
df |
SRMR |
TLI |
CFI |
RMSEA |
RMSEA 90% CI |
χ
2 = chi-squared; SRMR = standardised root mean square residual; TLI = Tucker–Lewis index; CFI = comparative fit index; RMSEA = root mean squared error of approximation; CI = confidence interval. |
Model 1 (n = 256 with no modifications) |
605.577 |
265 |
0.05 |
0.90 |
0.91 |
0.07 |
0.064–0.078 |
Model 1 (n = 505 with no modifications) |
894.140 |
265 |
0.05 |
0.91 |
0.092 |
0.07 |
0.064–0.074 |
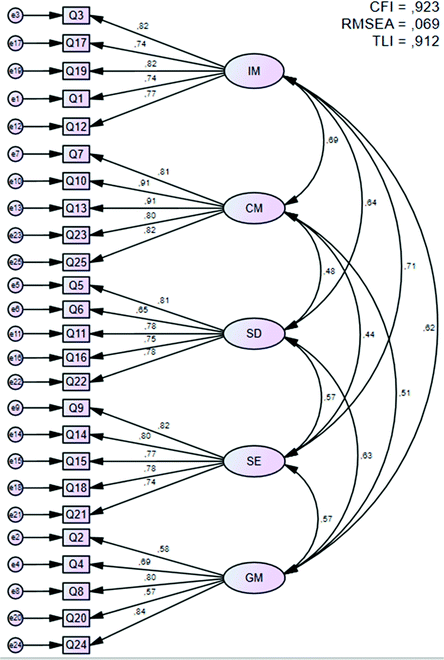 |
| Fig. 2 CFA diagram with model loadings (n = 505). | |
Measurement of model invariance
The objective of the analysis of model invariance is to evaluate whether the structure of the measurement model is equivalent (invariant) in different groups for the same population with different characteristics – in this case, boys vs. girls. To perform the analysis of model invariance, the sample used to carry out the confirmatory factor analysis (n = 505) was divided by gender (boys and girls). There were 194 male respondents and 311 female respondents. The values related to χ2, SRMR, TLI, CFI and RMSEA were calculated for both genders (Table 3). The same cutoff values for CFA were considered and the models indicated a slightly worst fit for both genders in comparison with the initial model with n = 505. However, the values were still found to be within the range that is considered a reasonable fit. TLI value for boys’ model presented a value a little below the acceptable and recommended. The others fit index values were acceptable. For this reason, we decided not to reject the model and proceed with the invariance analysis.
Table 3 Confirmatory factor analysis results obtained for boys and male
Model |
χ
2
|
df |
SRMR |
TLI |
CFI |
RMSEA |
RMSEA 90% CI |
Caption. χ2 = chi-squared; SRMR = standardized root mean square residual; TLI = Tucker–Lewis index; CFI = comparative fit index; RMSEA = root mean squared error of approximation; CI = confidence interval. |
Boys |
560.607 |
265 |
0.06 |
0.89 |
0.90 |
0.08 |
0.067–0.085 |
Girls |
733.771 |
265 |
0.05 |
0.90 |
0.91 |
0.08 |
0.069–0.082 |
Individually, the CFA groups (Boys vs. Girls) exhibited a model with reasonable fit; as a result, we went ahead with our analyses and tested the criteria for the model invariance. Table 4 shows the results obtained for the model invariance analysis.
Table 4 Model invariance analysis
B vs. G |
M C |
χ
2
|
df |
RMSEA |
ΔRMSEA |
CFI |
ΔCFI |
χ
2 = chi-squared; CFI = comparative fit index; RMSEA = root mean squared error of approximation. |
1. Configural invariance |
|
1294.378 |
530 |
0.054 |
|
0.907 |
|
2. Metric invariance |
2 vs. 1 |
1307.618 |
550 |
0.052 |
0.002 |
0.907 |
0.000 |
3. Scalar Invariance |
3 vs. 2 |
1415.944 |
575 |
0.054 |
0.002 |
0.897 |
0.010 |
4. Residual Invariance |
4 vs. 3 |
1519.893 |
615 |
0.054 |
0.000 |
0.889 |
0.008 |
Gender differences and descriptive statistics
For the sample related to gender differences, the correlations (Table 5) obtained for all five factors ranged between 0.40 for Self-Efficacy and Career Motivation and 0.64 for Self-Efficacy and Intrinsic Motivation (p < 0.01). The total mean values obtained ranged from 3.89 (Grade Motivation) to 3.19 (Career Motivation). MANOVA results (n = 505) indicated an overall statistically significant effect of gender on students’ motivation to learn chemistry – F(5, 499) = 16.43, p < 0.001, Wilks's Λ = 0.86, partial η2 = 0.14. Follow-up univariate analyses showed that girls scored higher in Career Motivation (F(1, 503) = 5.35, p = 0.02, η2 = 0.01), Self-Determination (F(1, 503) = 21.53, p < 0.01, partial η2 = 0.04), and Grade Motivation (F(1, 503) = 20.65, p < 0.01, partial η2 = 0.04), while boys scored higher in Self-Efficacy (F(1, 503) = 6.49, p = 0.01, partial η2 = 0.01). However, there was no significant gender effect on Intrinsic Motivation (F(1, 503) = 1.01, p = 0.28, partial η2 = 0.002).
Table 5 Mean, standard deviation and correlation values obtained for the CMQ-II subscales
Subscales |
r
|
|
Boys (n = 194) |
Girls (n = 311) |
Total (n = 505)a |
1 |
2 |
3 |
4 |
5 |
We excluded from these analyses responses in which the respondents’ gender was classified as “others” (n = 5), though we considered this category when calculating for total mean scores. **p < 0.01.
|
|
1. Career motivation |
|
0.40** |
0.44** |
0.63** |
0.44** |
M |
3.06 |
3.29 |
3.19 |
|
SD |
1.08 |
1.16 |
1.13 |
|
|
2. Self-efficacy |
|
|
|
|
|
0.50** |
0.64** |
0.48** |
M |
3.63 |
3.43 |
3.50 |
|
|
SD |
0.84 |
0.89 |
0.88 |
|
|
|
3. Self-determination |
|
|
|
|
|
|
0.57** |
0.53** |
M |
3.15 |
3.49 |
3.35 |
|
SD |
0.83 |
0.77 |
0.81 |
|
|
4. Intrinsic motivation |
|
|
|
|
|
|
|
0.52** |
M |
3.47 |
3.56 |
3.52 |
|
SD |
0.88 |
0.93 |
0.91 |
|
|
5. Grade motivation |
|
|
|
|
|
|
|
|
M |
3.68 |
4.01 |
3.89 |
|
SD |
0.86 |
0.77 |
0.83 |
|
Discussions
Factor analysis
The results obtained after carrying out psychometric analyses (including EFA, assessment of reliability, and internal consistency of the variables and factors) of the data were considered satisfactory. The CMQ-II designed for the Brazilian context and used for assessing the motivation to learn chemistry among Brazilian high school students exhibited a five-factor structure (self-determination, self-efficacy, intrinsic motivation, grade motivation and career motivation), with factor loadings ranging from 0.52 to 0.86. The results obtained in this study were found to be perfectly in line with a greater portion of the criteria proposed in another study of translation of the CMQ II with Spanish high school students (Ardura and Pérez-Bitrián, 2018). Among the results obtained include the following: (i) eigenvalues > than 1; (ii) variation explained by the five retained factors = 69.6% (>40%) – this is slightly lower compared to the value reported in the CMQ II Spanish study (69.7%) (Ardura and Pérez-Bitrián, 2018). The internal consistency indices (Cronbach α) found for each motivational factor also point to the suitability of the instrument. The general average of the Cronbach α obtained (0.94) was found to be within the acceptable range and was slightly higher than the value reported in other studies: 0.92 (Glynn et al., 2011), 0.91 (Salta and Koulougliotis, 2015), 0.87 and 0.93 (Dong et al., 2020). The “grade motivation” and “career motivation” in the present study recorded the lowest (α = 0.81) and the highest (a = 0.92) internal consistency index, respectively. Ardura and Pérez-Bitrián (2018) obtained similar results during their statistical analysis of CQM II in Spanish.
The confirmatory factor analysis presented in this study considered the separation of the five factors (components) generated by the scale with the five domains and their respective items, according to the initial instrument. As in the sample of n = 256, the sample with full data presented good model fit values. The factor loadings were found to be adequate and were maintained as in the original scale on both analysis. As highlighted by Komperda et al. (2020), most SMQ II or CMQ II studies do not meet the Hu and Bentler cutoff criteria. It was also the case of this study, however, we obtained good values from the proposed model, which is the same as the original study.
The high value of χ2 recorded in the study is thus not regarded as a problem in this context. According to Jöreskog and Sörbom (1989), χ2 has serious limitations when dealing with variables such as human values. Several studies published in the literature have also reported similar observations in their statistical analysis of the transcultural adaptation of SQM II or CMQ II (Glynn et al., 2011; Salta and Koulougliotis, 2015; Ardura and Pérez-Bitrián, 2018; Dong et al., 2020).
With regard to model invariance analysis, Cheung and Rensvold (2002) demonstrated that it is reasonable to consider this type of analysis when it comes to differences in CFI (ΔCFI). According to Cheung and Rensvold (2002), the difference in values between the configural model (free parameters model) and the restricted model (fixed parameters) should be ΔCFI ≤ 0.01. Also, Chen (2007) recommends ΔRMSEA ≤ 0.015. Based on the recommendations of Cheung and Rensvold (2002) and Chen (2007), as can be observed, the results obtained in the present study shows that there were no gender differences – between boys and girls’ respondents. This clearly helps to argue for the reliability of the data collected by the questionnaire for this research.
Descriptive statistics and correlations
The results obtained in this study show that high school students' motivation to learn chemistry is more oriented toward obtaining grades; this is in line with the findings reported by Schumm and Bogner (2016), Salta and Koulougliotis (2015), Ardura and Pérez-Bitrián (2018), and Komperda et al. (2020). The correlation analysis conducted showed that while autonomous forms of motivation, such as self-determination, self-efficacy, and intrinsic motivation, are positively related, they are also positively related to less autonomous forms of motivation, such as career and grade-related motivations. For instance, high correlation values were obtained between intrinsic motivation and career motivation and between intrinsic motivation and self-efficacy. Thus, these subscales may not be mutually exclusive and high school students can simultaneously exhibit autonomous and controlled motivations for learning Chemistry.
Gender differences
Overall, the results obtained from the MANOVA showed that there were significant differences between Brazilian high-school male and female students in terms of the motivation to learn chemistry. Intrinsic motivation was the only factor in which we did not find any significant differences between the genders. Overall, boys scored higher for “Self-Efficacy” while girls scored higher in both controlled forms of regulation (Grade Motivation) and autonomous regulation (Self-Determination and Career Motivation). These results show that Brazilian high-school boys are slightly more inclined to believe in their abilities to succeed in chemistry classes, yet they seem less inclined to study because of their imagined future career or to obtain a good grade. It is clearly plausible to infer that by believing more in their abilities to do well in chemistry, boys tend to be less worried about putting in much more efforts in their studies to obtain a good grade as opposed to girls, and this may explain their having a lower score in Grade Motivation. In contrast, because of their lower self-efficacy compared to boys, girls seem to be more worried about grades, and this boosts their motivation to study chemistry – Grade motivation. Still, unlike boys, girls also tend to adopt more autonomous forms of regulation to study by focusing on the importance of chemistry for their imagined future career and using self-regulated strategies to learn chemistry. While some studies in the literature show boys’ greater inclination to pursue a science-related career (DeWitt and Archer, 2015), our results indicate that this might no be necessarily the case for chemistry in Brazil; thus, in essence, the girls’ main motives of studying chemistry are related to their perception of the importance of chemistry for a professional occupation in a science/chemistry-related field. Also, a higher career motivation for studying chemistry can be directly related to the need for obtaining good grades as an important element for the students to be considered good learners (of chemistry). This is in stark contrast to boys despite the fact that they exhibit higher confidence in chemistry classes. This finding points to the existence of some potential inequalities in chemistry education in the sense that although girls are generally motivated to study chemistry for both controlled and autonomous reasons, they still believe less in their abilities to succeed in this discipline.
The gender differences observed in the present study were found to be in line with some of the findings reported by studies conducted using the CMQ-II based on the USA (Glynn et al., 2011), Spanish (Ardura and Pérez-Bitrián, 2018), and German (Schumm and Bogner, 2016) contexts, although it is in stark contrast with some of the results obtained by Salta and Koulougliotis (2015) under the Greek context who reported to have found no significant differences between genders in terms of self-efficacy and grade motivation. However, our findings still agrees with Salta and Koulougliotis (2015) analysis which showed that girls scored higher in self-determination. Similarly, some of our results were in line with some of the findings of Ardura and Pérez-Bitrián (2018) and Schumm and Bogner (2016). For example, both studies pointed to the existence of significant gender differences when it comes to self-efficacy (higher for boys) and self-determination to study chemistry (higher for girls).
These differences in the results obtained from the application of the CMQ II in different countries somewhat point to the importance of conducting cross-cultural studies targeted at investigating motivational variations between countries. Remarkably, the similarities of the results found in terms of gender differences point to the robustness of the CMQ II when applied toward investigating how boys and girls relate to chemistry in Brazil; it is clear that these outcomes can be attributed to the existing contextual similarities in chemistry classes between the countries investigated. However, following Cohen's (1988) interpretation, one should be cautioned when analysing these results as the strength of the differences was generally small (partial η2 = 0.01) for Career Motivation and Self-Efficacy, while for Self-Determination and Grade Motivation the strength of the differences were between small and moderate (partial η2 = 0.04). With that in mind, we can argue that, at least for this sample of Brazilian high-school students, there is not a considerable gender gap in the motivation to learn chemistry. This finding agrees with Salta and Koulougliotis (2020) study that showed a small gender effect on the overall motivation to learn chemistry between undergraduate students.
Limitations
Brazil is a country of more than 200 million inhabitants. By virtue of that, it would be empirically interesting to assess students’ motivation to learn chemistry based on a larger and more heterogeneous sample. Clearly, this would allow one to conduct a much deeper investigation regarding the suitability of the instrument for measuring students’ motivation to learn chemistry in the Brazilian context. Due to the COVID-19 pandemic, during the course of this research, the majority of public and private secondary schools suspended their classes; this certainly undermined the smooth collection of data. Also, the collection period was too long (15 months). Student motivation is a construct that does not stay constant over time; therefore, the period of data collection was also considered a limitation. Regarding the translation and back-translation of the instrument to Portuguese, future studies should also include a response process interview with students to strengthen their results (AERA et al., 2014; Deng et al., 2021).
Conclusions
The results obtained from the exploratory factor analysis, confirmatory factor analysis and the analysis of model invariance between genders conducted in this study give support to the applicability of the Brazilian version of the CMQ-II for measuring the motivation to learn chemistry among high school students in Brazil. The sensitivity of the instrument was found to be clearly evident when it was applied for the analysis of the differences between boys and girls in terms of motivation to study chemistry. The findings of the study also point to significant gender differences when it comes to students’ motivation to study chemistry; although Brazilian boys students believe more in their ability to learn chemistry, the girls students were found to exhibit much stronger motivation to study chemistry for an imagined future career. Finally, we certainly believe the instrument can be easily translated to the Portuguese language spoken in Portugal, and the conduct of a cross-cultural analysis between these countries can further contribute toward making the instrument more robust.
Conflicts of interest
There are no conflicts to declare.
Acknowledgements
The authors are grateful to the students that took part in the study. This work was financially supported by the São Paulo Research Foundation (FAPESP) [grant numbers 2017/10118-0, 2014/02522-7, 2018/20145-7, 2019/22340-4], the Coordenação de Aperfeiçoamento de Pessoal de Nível Superior – Brasil (CAPES) – Finance Code 001 and the Learning in the Community Program of the Dean of Undergraduate Studies of the São Paulo University (USP).
Notes and references
- AERA, APA and NCME, (2014), Standards for educational and psychological testing, Washington, DC: American Educational Research Association.
- Ardura D. and Pérez-Bitrián A., (2018), The effect of motivation on the choice of chemistry in secondary schools: adaptation and validation of the Science Motivation Questionnaire II to Spanish students, Chem. Educ. Res. Pract., 19(3), 905–918.
- Artelt C., (2005), Cross-cultural approaches to measuring motivation. Educ. Assess., 10(3), 231–255.
- Bandura A., (1986), Social foundations of thought and action: A social cognitive theory, Englewood Cliffs, N.J.: Prentice-Hall.
- Bandura A., (1989), Human agency in social cognitive theory, Am. Psychol., 44(9), 1175–1184. Available at: http://www.stiftelsenhvasser.no/documents/Bandura_Human_Agency_in_social_Cognitiv_theory.pdf.
- Bandura A., (2002), Social Cognitive Theory of Mass Communication, In: J. Bryant and M. B. Oliver (ed.), Media Effects: Advances in Theory and Research, New York: Routledge, pp. 94–124.
- Bandura A., (2005), The evolution of social cognitive theory, Great minds in management: the process of theory development. Oxford: Oxford University Press.
- Bentler P. M., (1990), Comparative Fit Indexes in Structural Models, Psychological Bulletin, 107(2), 238–246.
- Black A. E. and Deci E. L., (2000), The effects of instructors’ autonomy support and students’ autonomous motivation on learning organic chemistry: A self-determination theory perspective, Sci. Educ., 84(6), 740–756.
- Broussard S. C. and Garrison M. E., (2004), The Relationship Between Classroom Motivation and Academic Achievement in Elementary-School-Aged Children, Family Consumer Sci. Res. J., 33(2), 106–120.
- Blunch N., (2008), Introduction to structural equation modelling using SPSS and AMOS, London: Sage.
- Brasil, (2021), Instituto Nacional de Estudos e Pesquisas Educacionais Anísio Teixeira (Inep), Resumo Técnico: Censo Escolar da Educação Básica 2021 [National Institute of Educational Studies and Research Anísio Teixeira (Inep). Technical brief: schooling census for basic education 2021], Brasília, DF: Inep.
- Brown T., (2006), Confirmatory factor analysis for applied research, New York: The Guilford Press.
- Brown T., (2015), Confirmatory factor analysis for applied research, 2nd edn, New York: The Guilford Press.
- Browne M. W. and Cudeck R., (1993), Alternative Ways of Assessing Model Fit, In: K. Bollen and J. Long (ed.), Testing Structural Equation Models, Newbury Park, CA: Sage, pp. 136–162.
- Byrne B. M., (2010), Structural equation modeling with AMOS: Basic concepts, applications, and programming, 2nd edn, Routledge: Taylor & Francis.
- Chen F. F., (2007), Sensitivity of goodness of fit indexes to lack of measurement invariance. Struct. Eq. Model.: Multidisciplinary J., 14(3), 464–504.
- Cheung G. W. and Rensvold R. B., (2002), Evaluating Goodness-of-Fit Indexes for Testing Measurement Invariance, Structural Equation Modeling: A Multidisciplinary Journal, 9(2), 223–255.
- Covert H., Ilunga Tshiswaka D., Ramkissoon I., Sisskin E., Lichtveld M. and Wickliffe J., (2019), Assessing science motivation among high school students participating in a supplemental science programme: the Emerging Scholars Environmental Health Sciences Academy. Int. J. Sci. Educ., 41(17), 2508–2523.
- Cohen J., (1988), Statistical power analysis for the behavioural sciences, 2nd edn, Hillsdale, NJ: Lawrence Erlbaum Associates.
- Cronbach L. J., (1951), Coefficient alpha and the internal structure of tests, Psychometrika, 16(3), 297–334.
- Deci E. L. and Ryan R. M., (1985), Intrinsic motivation and self-determination in human behavior, New York: Springer Science and Business Media.
- Deng J. M., Streja N. and Flynn A. B., (2021), Response process validity evidence in chemistry education research, J. Chem. Educ., 98(12), 3656–3666.
- DeWitt J. and Archer L., (2015), Who aspires to a science career? A comparison of survey responses from primary and secondary school students, Int. J. Sci. Educ., 37(13), 2170–2192.
- Dong Z., Li M., Minstrell J. and Cui Y., (2020), Psychometric properties of Science Motivation Questionnaire II-Chinese version in two waves of longitudinal data, Psychol. Sch., 57(8), 1240–1256.
- Ferrell B., Phillips M. M. and Barbera J., (2016), Connecting achievement motivation to performance in general chemistry. Chemistry Education Research and Practice, 17(4), 1054–1066.
- Glynn S. M. and Koballa T. J., (2006), Motivation to Learn College Science. In: J. J. Mintzes and W. H. Leonard (ed.), Handbook of college science teaching, Arlington: National Science Teachers Association Press, pp. 25–32.
- Glynn S. M., Taasoobshirazi G. and Brickman P., (2009), Science Motivation Questionnaire: Construct validation with nonscience majors, J. Res. Sci. Teach., 46(2), 127–146. https://onlinelibrary.wiley.com/doi/abs/10.1002/tea.20267.
- Glynn S. M., Brickman P., Armstrong N. and Taasoobshirazi G., (2011), Science motivation questionnaire II: Validation with science majors and nonscience majors, J. Res. Sci. Teach., 48(10), 1159–1176.
- Hair Jr. J. F., Black W. C., Babin B. J. and Anderson R. E., (2009), Multivariate Data Analysis, 7th edn, Upper Saddle River: Prentice Hall.
- Hooper D., Coughlan J. and Mullen M. R., (2008), Structural Equation Modelling: Guidelines for Determining Model Fit, Electron. J. Bus. Res. Methods, 6, 53–60.
- Hu L. T. and Bentler P. M., (1999), Cutoff criteria for fit indexes in covariance structure analysis: Conventional criteria versus new alternatives, Structural Equation Modeling, 6(1), 1–55.
- Jöreskog K. G. and Sörbom D., (1989), LISREL 7: A Guide to the Program and Applications, Chicago, IL: SPSS.
- Kaiser H. F., (1960), The application of electronic computers to factor analysis, Educ. Psychol. Meas., 20, 141–151.
- Kline R. B., (2011), Principles and Practice of Structural Equation Modeling, 3rd edn, New York: Guilford Press.
- Komperda R., Hosbein K. N., Phillips M. M. and Barbera J., (2020), Investigation of evidence for the internal structure of a modified science motivation questionnaire II (mSMQ II): a failed attempt to improve instrument functioning across course, subject, and wording variants, Chem. Educ. Res. Pract., 21(3), 893–907.
- Lavigne G. L., Vallerand R. J. and Miquelon P., (2007), A motivational model of persistence in science education: A self-determination theory approach, Eur. J. Psychol. Educ., 22(3), 351–369.
- Lawson A. E., Banks D. L. and Logvin M., (2007), Self-efficacy, reasoning ability, and achievement in college biology. J. Res. Sci. Teach., 44(5), 706–724.
- MacCallum
R. C., Browne M. W. and Sugawara H. M., (1996), Power Analysis and Determination of Sample Size for Covariance Structure Modeling, Psychological Methods, 1(2), 130–149.
- Mujtaba T., Sheldrake R., Reiss M. J. and Simon S., (2018), Students’ science attitudes, beliefs, and context: associations with science and chemistry aspirations, Int. J. Sci. Educ., 40(6), 644–667.
- OECD, (2016), PISA 2015 results (volume I): excellence and equity in education, PISA: OECD Publishing, Paris.
- Pajares F. and Olaz F., (2008), Teoria Social Cognitiva e autoeficácia: umavisãogeral [Social Cognitive Theory and self-efficacy: an overview], In: A. Bandura, R. G. Azzi, and S. Polydoro (ed.), Teoria Social Cognitiva: conceitosbásicos, [Social Cognitive Theory: basic concepts], São Paulo: Artmed, pp. 97–114.
- Patall E. A., Steingut R. R., Vasquez A. C., Trimble S. S., Pituch K. A. and Freeman J. L., (2018), Daily autonomy supporting or thwarting and students’ motivation and engagement in the high school science classroom, J. Educ. Psychol., 110(2), 269.
- Pituch K. A. and Stevens J. P., (2015), Applied multivariate statistics for the social sciences: Analyses with SAS and IBM's SPSS, Routledge.
- Putnick D. L. and Bornstein M. H., (2016), Measurement invariance conventions and reporting: The state of the art and future directions for psychological research. Dev. Rev., 41, 71–90.
- Reeve J., (2009), Understanding Motivation and Emotion, 5th edn, Danvers: John Wiley & Sons.
- Ryan R. M. and Deci E. L., (2000) Intrinsic and extrinsic motivations: Classic definitions and new directions, Contemporary Educ. Psychol., 25(1), 54–67.
- Salta K. and Koulougliotis D., (2015), Assessing motivation to learn chemistry: adaptation and validation of Science Motivation Questionnaire II with Greek secondary school students, Chem. Educ. Res. Pract., 16(2), 237–250.
- Salta K. and Koulougliotis D., (2020), Domain specificity of motivation: chemistry and physics learning among undergraduate students of three academic majors, Int. J. Sci. Educ., 42(2), 253–270.
- Schumm M. F. and Bogner F. X., (2016), Measuring adolescent science motivation, Int. J. Sci. Educ., 38(3), 434–449.
- Simpkins S. D., Price C. D. and Garcia K., (2015), Parental support and high school students' motivation in biology, chemistry, and physics: Understanding differences among Latino and Caucasian boys and girls, J. Res. Sci. Teach., 52(10), 1386–1407.
- Steiger J. H., (2007), Understanding the limitations of global fit assessment in structural equation modelling, Pers. Individ. Differ., 42(5), 893–898.
- Tabachnick B. G. and Fidell L. S., (2001), Using multivariate statistics, 4th edn, Boston: Allyn and Bacon.
- Tai R. H., Qi Liu C., Maltese A. V. and Fan X., (2006), Planning early for careers in science, Science, 312(5777), 1143–1144.
- Vedder-Weiss D. and Fortus D., (2011), Adolescents' declining motivation to learn science: Inevitable or not?, J. Res. Sci. Teach., 48(2), 199–216.
- Vedder-Weiss D. and Fortus D., (2013), School, teacher, peers, and parents' goals emphases and adolescents' motivation to learn science in and out of school, J. Res. Sci. Teach., 50(8), 952–988.
- Wardhany I. I., Subita G. P. and Maharani D. A. (2018) Cross-Cultural Adaptation and Psychometric Properties of the Science Motivation Questionnaire-II: Indonesian Version, Pesquisa Brasileira em Odontopediatria e Clinica Integrada, 18(1). 4294.
- Wang M. T., Guo J. and Degol J. L., (2020), The role of sociocultural factors in student achievement motivation: A cross-cultural review, Adolesc. Res. Rev., 5(4), 435–450.
|
This journal is © The Royal Society of Chemistry 2022 |