Considering alternative reaction mechanisms: students’ use of multiple representations to reason about mechanisms for a writing-to-learn assignment
Received
8th November 2021
, Accepted 14th February 2022
First published on 23rd February 2022
Abstract
Organic reaction mechanisms are often represented by the electron-pushing formalism and reaction coordinate diagrams. These representations pose a challenge to students because valuable information is encoded within each representation, and students must know how to reason about mechanisms using both. Hence, it is important to understand whether and how students consider these two representations when reasoning about reaction mechanisms. We have collected responses to a writing-to-learn assignment administered in a second-semester organic chemistry laboratory course to investigate students’ reasoning. The assignment was designed to elicit students’ reasoning about the most likely of two mechanisms for a catalyzed intramolecular aldol reaction when given the electron-pushing scheme and reaction coordinate diagram for both mechanisms. As part of the assignment, students submitted initial drafts, participated in content-focused peer review, and submitted revised drafts. We analyzed each component using a mixed methods approach to identify students’ reasoning about the most likely reaction pathway and how their reasoning changed after peer review and revision. In this article, we present a quantitative overview of changes students made about their decisions for the most likely reaction pathway and how these changes are related to providing and receiving feedback. Additionally, we present our analysis of the features of representations students used to reason about the likelihood of alternative reaction mechanisms. This study demonstrates how existing research about students’ reasoning with representations was operationalized for classroom practice using writing-to-learn. Furthermore, the analysis illustrates how writing-to-learn to can be used to develop students’ reasoning and offers implications for teaching students to reason about reaction mechanisms using multiple representations.
Introduction
Students typically encounter two representations of organic reaction mechanisms in introductory organic chemistry courses: the electron-pushing formalism (EPF) and reaction coordinate diagrams (RCDs). A growing body of research examines how students reason about organic reaction mechanisms with the EPF (Graulich, 2015). More recently, attention has focused on how students reason with RCDs (Lamichhane et al., 2018; Popova and Bretz, 2018a, 2018c), with one study examining how students match reactions to RCDs (Popova and Bretz, 2018b). However, few existing studies explore how introductory organic chemistry students reason with both of these representations together. As such, the goal of this study is to explore students’ reasoning in writing when considering both representations of reaction mechanisms. We achieve this through a writing-to-learn (WTL) assignment that asked students to reason about the most likely of two mechanisms for a single transformation, given the EPF schemes and RCDs for both mechanisms. The WTL assignment was implemented with peer review and revision, which allowed us to further investigate (1) how students’ reasoning with these representations changed during the weeks spanning the assignment and (2) how changes in students’ reasoning from their initial to final drafts might be influenced by the peer review process. In this work, we present our analysis of students’ reasoning as presented across their initial and final responses to the WTL assignment. We additionally present our analysis of the influence of the peer review process. This research demonstrates the operationalization of chemistry education research findings to inform the design of WTL and to further investigate students’ reasoning with mechanistic representations when they write about organic reactions.
Reasoning with mechanistic representations in organic chemistry
The Next Generation Science Standards identifies multiple scientific practices that science educators should focus on for improving STEM education. One of these scientific practices is “developing and using models” (NGSS Lead States, 2013). In this context, a “model” is not only the representation that depicts a phenomenon but also the cognitive processes involved in developing and using said representation. In other words, models include the graphs, figures, and/or structures that scientists use to depict a phenomenon along with the epistemic practices for developing models and using them to explain or predict phenomena (Passmore et al., 2014, 2016). As such, researchers emphasize that instruction in alignment with this scientific practice should not only encompass what a model is of, but also what it is for (Gouvea and Passmore, 2017).
Models and representations are central to reasoning in organic chemistry, as evident by the nature of chemical knowledge spanning the submicroscopic, macroscopic, and symbolic domains described by Johnstone's triangle (Johnstone, 1991; Taber, 2013). Representations lie within the symbolic domain and are used to represent and bridge between submicroscopic and macroscopic concepts (Johnstone, 1991). There are multiple representations within the symbolic domain in the context of organic reaction mechanisms, including energy graphs and molecular structures (Taber, 2013). The two symbolic representations of organic reaction mechanisms often taught in introductory organic chemistry courses are the EPF and RCDs. Both representations provide different information about reactions that reflect organic chemists’ conceptions of chemical transformations (Goodwin, 2012). As such, the learning goals for teaching these representations are for students to understand (1) how the representations align with chemical ideas or concepts and (2) how the representations can be used to construct claims, predictions, or explanations (Kozma et al., 2000; Kozma and Russell, 2005; Popova and Jones, 2021).
Students’ reasoning with the EPF has received significant attention in the literature. Many studies examine how students make connections between the EPF representation and the underlying chemical properties that guide the proper use of the EPF. These studies indicate that students often focus on surface features, such as charges, when reasoning through reaction mechanisms (Anzovino and Bretz, 2015; Galloway et al., 2017; Caspari et al., 2018; Watts et al., 2020). Students also have challenges making connections between structure and function when reasoning about reaction mechanisms using key concepts including resonance (Ferguson and Bodner, 2008; Finkenstaedt-Quinn et al., 2020a; Petterson et al., 2020), nucleophilicity (Strickland et al., 2010; Cruz-Ramírez De Arellano and Towns, 2014; Anzovino and Bretz, 2015, 2016; Dood et al., 2020; Watts et al., 2020), and sterics (Bodé et al., 2019). Other studies similarly suggest that students focus more on the surface features and structures of the representation rather than the implicit chemical properties and functions (Strickland et al., 2010; Graulich and Bhattacharyya, 2017; Dood et al., 2020). This understanding of how students conceptualize the EPF provides a valuable basis for exploring how students use the EPF when reasoning, making claims, or constructing explanations.
Studies in the literature also examine students’ understandings of RCDs with investigations into how students interpret the meaning of RCD surface features (Lamichhane et al., 2018; Popova and Bretz, 2018a, 2018b, 2018c; Atkinson et al., 2020, 2021; Atkinson and Bretz, 2021). The studies by Popova and Bretz (2018a, 2018b, 2018c) specifically report several findings about how organic chemistry students understand RCDs. For example, Popova and Bretz (2018a) identified that students in their study often viewed RCDs as encoding information that reflects only the major reacting species rather than all components of a reaction, often not considering the submicroscopic level. In another article, Popova and Bretz (2018b) identified that students demonstrated challenges when translating between mechanisms and RCDs for substitution and elimination reactions due to incomplete understandings of the information communicated by RCD surface features. They also found students have difficulty with this task because their reasoning with the mechanisms was often product-oriented and focused on the surface features of reactants (Popova and Bretz, 2018b). The Popova and Bretz articles (2018a, 2018b, 2018c) were all in the context of a course that primarily taught RCDs alongside reactions in first-semester organic chemistry (e.g., substitution, elimination, and addition reactions) but not in the second semester of instruction. Hence, their findings suggest the need to provide students with further opportunities to develop their reasoning with RCDs. Furthermore, across the articles describing students’ interpretations of RCD surface features, researchers identified that students often conflate transition states with intermediates, do not note energy changes encoded on the y-axis, and view the x-axis as corresponding to time (Lamichhane et al., 2018; Popova and Bretz, 2018c; Atkinson et al., 2021; Parobek et al., 2021). The findings regarding students’ understandings of RCD surface features and how they connect to mechanisms suggest a need to further support students’ use and understanding of RCDs within the organic chemistry curriculum. Furthermore, the findings provide a baseline for understanding how students might use these representations in their reasoning.
As the existing literature indicates, researchers are focused on how students connect the surface features of both representations to chemical ideas, properties, and concepts. However, further research is necessary to understand how students use these representations in their reasoning. Some existing studies provide insight into how students reason in chemistry (Kraft et al., 2010; Christian and Talanquer, 2012; Sevian and Talanquer, 2014; Weinrich and Talanquer, 2016; Lieber and Graulich, 2022). Specifically, studies demonstrate that few students reason based on mental models that relate structure to reactivity, while many students rely on memorized rules or cases (Kraft et al., 2010; Christian and Talanquer, 2012). Similarly, students often face challenges with integrating multiple variables into their reasoning (Sevian and Talanquer, 2014; Weinrich and Talanquer, 2016) or using reasoning to connect evidence to claims (Lieber and Graulich, 2022). The challenges students have with reasoning in organic chemistry specifically may be related to a tendency for rote memorization rather than meaningful learning (Grove and Bretz, 2012). More recent studies examined students’ engagement with contrasting cases and how these types of problems can encourage students to consider multiple conceptual factors when producing an explanation (Caspari et al., 2018; Bodé et al., 2019; Caspari and Graulich, 2019; Watts et al., 2021). Nevertheless, students in these studies still often exhibited limited complexity in their reasoning or explanations. Altogether, these studies provide evidence of students’ abilities for both reasoning and providing explanations in organic chemistry across different problem types. These studies point to the need for further research into understanding how students reason and develop explanations when using two common mechanistic representations in organic chemistry. The goal of this study is to address this need through implementing a WTL assignment in the classroom that targets this aspect of students’ reasoning.
Using writing-to-learn, peer review, and revision to access students’ reasoning
Prior research demonstrates the analysis of students’ writing to access their reasoning about STEM content in organic chemistry (Watts et al., 2020; Brandfonbrener et al., 2021) and other content areas (Grimberg and Hand, 2009; Moon et al., 2019; Moreira et al., 2019). Some of these studies specifically elicited students’ reasoning through WTL (Moon et al., 2019; Watts et al., 2020; Brandfonbrener et al., 2021), an instructional practice that emphasizes the role of writing assignments in supporting students’ conceptual understanding (Anderson et al., 2015; Gere et al., 2019). Studies demonstrate that WTL is effective for supporting understanding in a variety of STEM courses, including chemistry, biology, materials science, and statistics (Finkenstaedt-Quinn et al., 2017; Halim et al., 2018; Moon et al., 2018; Schmidt-McCormack et al., 2019; Finkenstaedt-Quinn et al., 2020b; Finkenstaedt-Quinn et al., 2021a; Marks et al., 2022). In addition to the writing assignments supporting students’ conceptual understanding, WTL also incorporates peer review and revision that provide further learning opportunities. This aspect of WTL pedagogy can also explain how students’ reasoning might change for specific content due to these structures (Finkenstaedt-Quinn et al., 2021b). As these prior studies suggest, students’ responses to the WTL process are a valuable source of data for accessing students’ reasoning.
Existing studies of WTL in STEM courses examine the role of peer review in supporting students’ conceptual understanding and the revisions students make (Halim et al., 2018; Finkenstaedt-Quinn et al., 2019; Finkenstaedt-Quinn et al., 2020b; Finkenstaedt-Quinn et al., 2021a). Specifically, studies demonstrate that students can use the peer review process to provide content-focused, constructive feedback (Finkenstaedt-Quinn et al., 2019; Finkenstaedt-Quinn et al., 2020b). However, students do not always indicate incorrect content when commenting on other students’ drafts (Finkenstaedt-Quinn et al., 2020b). In addition, while students tend to make revisions in general, their revisions do not always necessarily align with the peer review comments they received (Finkenstaedt-Quinn et al., 2019; Schmidt-McCormack et al., 2019). Furthermore, peer review and revision can serve to both remediate some misunderstandings while also surfacing additional misunderstandings that were not present in students’ initial responses (Halim et al., 2018). Altogether, these studies suggest that receiving peer review comments is valuable for encouraging revision but not necessarily for remediating students’ incorrect understanding. However, studies do suggest that reading other students’ work and providing feedback may have more influence on students’ revisions compared to receiving feedback, in STEM courses (Finkenstaedt-Quinn et al., 2021a), writing courses (Lundstrom and Baker, 2009; Cho and MacArthur, 2010; Cho and Cho, 2011), and during hypothetical peer review (Berg and Moon, 2022). In addition to supporting students’ conceptual learning, there is also evidence that peer review can support students’ positive affective experiences with WTL assignments in a way that supports meaningful learning (Gupte et al., 2021; Petterson et al., 2022). With the existing evidence for the role of WTL with peer review and revision supporting students’ learning, it is necessary to further explore WTL assignments and the peer review process in the context of students’ reasoning with representations in organic chemistry, which is the goal of this study. Furthermore, using WTL assignments in this way demonstrates how the existing research findings pertaining to students’ reasoning with representations and case comparisons can be operationalized and implemented within the classroom to further investigate students’ reasoning.
Theoretical perspectives
This study is informed by the representational competence framework and the cognitive process theory of writing. Representational competence provides explanatory power for investigating students’ reasoning with multiple representations, which we captured in their writing. The cognitive process theory of writing is a complementary perspective that we used to uncover students writing processes as they engaged with this WTL assignment.
Representational competence
As described by Kozma and Russell (2005), representational competence is the ability to use representations to describe and explain chemical phenomena; this aligns the idea that the scientific practice of “developing and using models” includes both the representation of a phenomenon and how the representation is used in practice (Passmore et al., 2014, 2016; Gouvea and Passmore, 2017). Kozma and Russel's framework recognizes that much of chemistry instruction surrounds the various ways that chemists represent sub-microscopic phenomena. All representations in the context of chemistry require an understanding of the related chemical concepts, including the two representations central to this study, the EPF and RCDs. As described in the literature review, students’ use of these representations in organic chemistry tends to emphasize surface features rather than underlying chemical concepts, which is a reflection of novice representational use (Kozma and Russell, 1997, 2005; Kozma et al., 2000). However, another feature of representations that merits further study is that they are inherently required for communication, supporting claims, or making predictions (Kozma et al., 2000; Kozma and Russell, 2005). For instance, the EPF is useful for explaining or predicting the chemical structure of reaction products; similarly, RCDs are useful for explaining the thermodynamic and kinetic parameters that control the products of a reaction (Raker et al., 2013). Representational competence is also necessary for communicating concepts, ideas, or claims surrounding chemical phenomena (Kozma et al., 2000; Kozma and Russell, 2005). Beyond being able to use representations to support claims or make predictions, representational competence also encompasses selecting the appropriate representation for making particular claims or predictions (Kozma and Russell, 2005). This latter ability reflects the idea that, in many cases, more than one representation can be used to describe the same chemical phenomena—and that two representations of the same phenomenon can provide both similar and unique information (Kozma and Russell, 2005). Because of this, it is important to investigate how students use multiple representations when supporting claims or making predictions in organic chemistry. Furthermore, it is necessary to extend the existing research literature investigating students’ representational competence so instructors can better inform their practice of teaching, eliciting, and assessing representational competence in the classroom (Popova and Jones, 2021).
Cognitive process theory of writing
The other theoretical framework guiding this study supports the utilization of WTL both within the classroom and for investigating students’ reasoning. As described in previous studies using data from WTL assignments (Watts et al., 2020; Brandfonbrener et al., 2021), the cognitive process theory of writing underpins the analysis of written responses for accessing students’ understanding (Flower and Hayes, 1981, 1984; Hayes, 1996). The cognitive process theory suggests that students’ written responses reflect the concepts and knowledge they used throughout the process of writing and revising in response to an assignment. Furthermore, the theory emphasizes writing as a recursive process in which planning, drafting, and revising occur throughout all stages of the writing process. As cognitive writing processes require producing internal representations of knowledge that engage both long- and short-term memory, the texts that students produce make visible the concepts used to respond to a writing task. The cognitive process theory also suggests the value of implementing writing assignments with peer review and revision to further support students’ learning, as these structures provide further opportunity to engage with the cognitive writing processes. With the focus on recursive processes and revision, the cognitive process theory aligns with the model of cognition which suggests that the concepts and ideas used to respond to a task are activated within a specific context and can change across time (Hammer and Elby, 2003; Hammer et al., 2004). Hence, cognitive process theory provides a lens through which to understand how writing about representations engages students in the aforementioned aspects of representational competence across the initial draft and revision components of WTL assignments.
Research questions
This study examines introductory organic chemistry students’ reasoning when considering multiple representations of organic reaction mechanisms. Through our analysis of students’ reasoning as presented in their responses to a WTL assignment, we seek to address the following research questions:
(1) What features of multiple mechanistic representations do students use in their writing when reasoning about organic reaction mechanisms?
(2) What changes do students make in the features present in their writing after peer review and revision?
(3) How are students’ revisions linked to the components of the peer review process?
Methods
Instructional setting
This research took place within a second-semester organic chemistry laboratory course at a large, Midwestern research university. The laboratory course included a weekly, one-hour lecture component that covered content and procedures relevant for the weekly, four-hour laboratory component. The lecture component was taught across three sections by faculty and postdoctoral instructors, while the laboratory component was taught across multiple smaller sections by graduate student instructors. The coursework included a laboratory notebook, quizzes, and three writing assignments. The writing assignments accounted for thirty percent of students’ final grade for the course. Explicit instruction on using the EPF and interpreting RCDs took place near the beginning of the prerequisite first-semester organic chemistry lecture course (typically introduced during the second and fifth weeks of the course, respectively). RCDs are typically covered alongside substitution, elimination, and addition reactions. Instruction across the lecture and laboratory sequence incorporated using the EPF and RCDs to explain relevant phenomena; as such, students were expected to have enough familiarity with these representations to complete the WTL assignment described below. The course was affected by the onset of the COVID-19 pandemic, and the final weeks of the course were completed remotely. Therefore, the described WTL assignment and associated data collection took place entirely during remote instruction. All WTL assignments in the course were already administered asynchronously online through the learning management system, so no change was needed to the WTL implementation when changing to remote instruction.
Writing-to-learn assignment and implementation
The WTL assignment for this study was the final of three. The writing task was designed to afford students the opportunity to practice using the EPF and RCD representations to explain relevant phenomena. The assignment had the specific goal to support students in developing representational competence, particularly the abilities to (1) use representations to support their reasoning and (2) to select the appropriate representation for a task (Kozma and Russell, 2005). The assignment introduced students to a triazabicyclodecene (TBD) catalysed intramolecular aldol reaction and two of its possible mechanistic pathways as identified by Hammar et al. (2010). The EPF schemes and RCDs were provided for both mechanisms, as presented in Fig. 1. The focus of analysis for this study was the portion of the assignment that required students to identify which of the two mechanistic pathways they thought to be the most likely and to explain their choice. As described in the conclusion to the article by Hammar et al., the most likely pathway is Mechanism A, based on the density functional theory (DFT) calculations used to determine the energy values represented in the RCDs (Hammar et al., 2010). Note that students were not expected to write about DFT in their responses but that students were expected to be able to provide a response based on the information given in the assignment. The assignment was designed to incorporate features demonstrated to support students’ learning, including the opportunity to apply content knowledge to a meaning-making writing task and structures for peer interaction and revision (Anderson et al., 2015; Gere et al., 2019; Finkenstaedt-Quinn et al., 2021b). The full text of the assignment is available in Appendix 1.
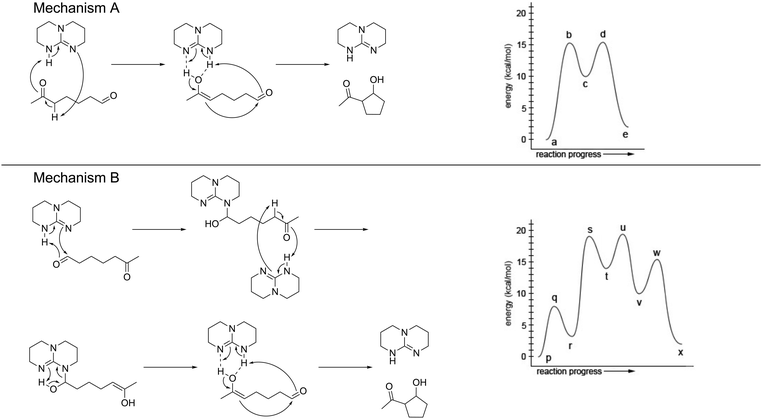 |
| Fig. 1 The EPF schemes and RCDs provided for both mechanisms within the WTL assignment, as shown to students. | |
The assignment was available to students in the online learning management system, and students had one week to submit an initial draft. Students then underwent an automated, double-blind peer review process in which each student provided and received feedback from typically three peers. Peer review was content-focused in that students provided feedback in alignment with the concepts targeted by the assignment. Students provided peer review comments by responding to the questions in the peer review guidelines (available in Appendix 1). Following the peer review process, students had three days to revise their response and submit final drafts. Throughout the weeks the assignment was open, students had access to support from undergraduates who were previously successful in the course and trained as writing fellows to support students with the WTL assignments. The peer review process and availability of writing fellows were intended to provide structure for interactive writing processes and to encourage metacognition and reflection (Anderson et al., 2015; Gere et al., 2019). The assessment process for the assignment was independent of the presented analysis, though students were assessed based on whether they incorporated reasoning rather than the correctness of their response.
Participants and data collection
The participants for this study included 456 students out of the 771 students who received a final grade for the course. The 456 students were those who consented to participate in the study, submitted a first and revised draft, and both provided and received peer review feedback to/from other consenting students. The data collected for this study included the first drafts, peer review comments, and final drafts for the WTL assignment. Data were collected following Institutional Review Board approval for human subjects research.
Data analysis
Data was analysed using a mixed methods approach, through which qualitative analysis of students' work was followed by quantitative transformation for further statistical analysis (Miles et al., 2014). The mixed methods approach was chosen to enable us to view the data through multiple approaches and perspectives, allowing for better understanding of the complexities present in students’ writing and their peer review interactions (Greene, 2008). The qualitative and quantitative analysis stages are described below.
Qualitative analysis.
The qualitative analysis took part in three stages: (1) identifying the mechanism students chose as the most likely in their initial and revised drafts, (2) qualitatively coding the initial and revised drafts for features of the mechanistic representations students wrote about to guide their choice, and (3) qualitatively coding peer review comments for whether students indicated agreement or disagreement with the mechanism chosen as the most likely.
The three coding schemes for the qualitative analysis are presented in Appendix 2. All coding schemes were developed by two members of the research team (FW and GP). The first coding scheme was used to identify students’ initial and revised responses for whether the student selected Mechanism A, Mechanism B, both, or neither as the most likely mechanism (Appendix 2, Table 4). At this stage of the analysis, responses from 456 students (both initial and final drafts for a total of 912 drafts) were analysed. The second coding scheme was developed through inductively coding students’ initial and revised drafts (Miles et al., 2014). The inductive coding scheme was developed to identify how students were using features of the mechanistic representations (e.g., peaks on the RCDs or functional groups in the EPF schemes) within their justifications for the reaction pathway they indicated as the most likely to occur. Through multiple rounds of open coding and discussions with the research team, the coding scheme was revised and modified until saturation was reached and all codes were clearly defined (Appendix 2, Table 5). The coding scheme sought to identify the different aspects of students’ writing that related to the features of mechanistic representations and/or their reasoning for their selection of the most likely pathway. For example, some codes captured students’ use of specific words or phrases within their justification (e.g., the “thermodynamics” and “kinetics” codes), while others captured students’ more specific reasoning for their selection of the most likely mechanistic pathway (e.g., the “general energy” and “counting” codes). Responses from 164 students (both initial and final drafts, for a total of 328 drafts and 1594 sentences) were analysed with the finalized coding scheme. This coding was done on a sentence level using NVivo 12 (QSR International Pty Ltd, 2018), with the allowance that each sentence could be coded with all applicable codes. Two examples of a coded response (initial and revised drafts) are provided in Appendix 3, Fig. 9. The third coding scheme was developed to analyse the peer review comments students wrote in response to the fourth peer review criterion, which asked students to comment on whether the author selected the appropriate choice for the most likely mechanism. The coding scheme was used to categorize the comments as providing agreement, disagreement, or a neutral response (Appendix 2, Table 6). The peer review comments received by all 456 students were analysed (a total of 1361 comments).
Reliability.
Efforts were taken to establish reliability throughout the qualitative analysis process (Watts and Finkenstaedt-Quinn, 2021). For each stage, a subset of at least 20% of the total analysed data was independently analysed by two authors (FW and GP for stages 1 and 3; FW and MP for stage 2). The percent agreement and an appropriate IRR measure was calculated for each stage (Table 1). The calculated agreement measure for each stage of analysis indicates strong agreement (Watts and Finkenstaedt-Quinn, 2021).
Table 1 Details for the efforts to establish reliability for each stage of the analysis
Data analysis stage |
N (students) |
Data analysed |
N (analysis units) |
Reliability subset |
Percent agreement (%) |
IRR measure |
Cohen's kappa, for when only one code is applied to each unit of analysis (Cohen, 1960).
Fuzzy kappa, for when more than one code can be applied to a single unit of analysis (Kirilenko and Stepchenkova, 2016).
|
(1) Mechanism choice |
456 students |
Initial and revised drafts, at the document level |
912 drafts |
280 drafts (31%) |
99 |
0.94a |
(2) Features of mechanistic representations |
164 students |
Initial and revised drafts, at the sentence level |
1594 sentences |
334 sentences (21%) |
82 |
0.80b |
(3) Peer review comments |
456 students |
Peer review comments received |
1361 comments |
277 comments (20%) |
90 |
0.80a |
Quantitative analysis.
The results of the qualitative coding were transformed into quantitative data for further analysis (Miles et al., 2014). The sentence-level coding from the second coding scheme of the qualitative analysis was used to determine frequencies with which each code appeared in each student’s first and second drafts (since each code could be applied to multiple sentences and each sentence could have multiple codes). Students’ sentence-level revisions were represented by subtracting the frequency with which each code appeared in their first drafts from the frequencies in their revised drafts. These data were used to perform the quantitative analyses focused on the features students included in their initial drafts and revisions, completed in R using RStudio (RStudio Team, 2018). Statistical significance for all analyses was set at α = 0.05.
The statistical analyses for initial drafts and revisions were performed to identify sentence-level differences between groups of students based on the mechanism they selected as the most likely. To analyse initial drafts, we grouped students by whether they selected Mechanism A or Mechanism B. This allowed for identifying the connections students made between the features of representations (identified with the coding scheme in Table 5) and the mechanistic pathway they identified as most likely (identified with the coding scheme in Table 4). To analyse students’ revisions, students were grouped by degree of global revisions, which are revision activities beyond the sentence level (Hayes, 1996). For the context of this study, global revisions were characterized by whether students revised to select a different mechanism as most likely (Table 2).
Table 2 Groupings of students by global revisions
Global revision group |
Mechanism selected, initial draft |
Mechanism selected, revised draft |
1 |
A |
A |
2 |
A |
B |
3 |
B |
A |
4 |
B |
B |
The statistical analyses were selected and conducted as described by Sheskin (2011). Statistics first involved Shapiro–Wilk tests for normality, which indicated the distributions for the total number of codes in students’ initial drafts were non- normally distributed (W = 0.93, p < 0.001). Hence, non-parametric tests were used for each analysis. Next, Mann–Whitney U tests were used to identify differences between the two groups of students on their initial drafts. Finally, Kruskal–Wallis one-way analysis of variance by ranks tests were used to identify differences in sentence-level revisions between the four groups of students based on their global revisions. For statistically significant results on the Kruskal–Wallis tests, post hoc pairwise Mann–Whitney U tests were performed to identify the specific revision groups between which the differences were significant. For all tests in which multiple hypothesis tests were conducted simultaneously, p-values were corrected using Bonferroni's procedure to adjust for the family-wise Type I error rate (Sheskin, 2011).
Lastly, we used logistic regression analysis to identify relationships between the global revisions and the two components of the peer review process (receiving feedback and reading other students’ drafts). The logistic regression models took the general form of
Revisions = Initial Draft Mech. + Comments Received + Drafts Reviewed |
where
Revisions is the outcome variable capturing whether students made global revisions (
i.e., whether students chose a different mechanism as most likely in their revised draft). This variable was coded as
Revisions = 1 if students made global revisions (
i.e., for groups 2 and 3 in
Table 2) and as
Revisions = 0 if students did not make global revisions (
i.e., for groups 1 and 4 in
Table 2). The
Initial Draft Mech. predictor variable served as a binary indicator of the mechanism students selected as most likely in their initial draft. Note that students who selected both or neither mechanism in either draft were excluded from all regression models. The
Comments Received predictor variable indicates the number of peer review comments students received that included a disagreement with the mechanism selected as most likely. Similarly, the
Drafts Reviewed predictor variable indicates the number of drafts students reviewed that selected a different mechanism as most likely. Three logistic regression models were calculated and are described in detail in the results. Odds ratios were used to interpret the logistic regression models, which were calculated by exponentiating the coefficients of non-interaction terms. Odds ratios are interpreted as the factor by which each predictor variable influenced the odds of students making global revisions (
Jaccard, 2011).
Results
The central goal of this research is to identify how students use the two common representations of organic reaction mechanisms (RCDs and EPFs) to reason in writing about the likelihood of alternative reaction mechanisms. This analysis is set within students’ responses to a WTL assignment in which they had the opportunity to revise their responses after undergoing a peer review and revision process. As such, this study also aims to identify how students’ reasoning changes following peer review and revision. We first present the results of the initial stage of our analysis, in which we identified the mechanistic pathway students selected as most likely in their initial and final drafts. Following this, we address our three research questions to (1) describe the features of RCDs and EPFs students used to justify their decisions in their initial drafts, (2) describe the changes students made in their explanations after peer review and revision, and (3) identify the degree to which components of the peer review process—both receiving and providing feedback—influenced students’ revisions. The results are interpreted and situated within the literature and theoretical frameworks in the discussion section.
The mechanistic pathway students selected as most likely in their initial and final drafts
As presented in Fig. 2, most students selected Mechanism A as most likely in their first draft (n = 336), while most remaining students chose Mechanism B (n = 114). Few students did not clearly indicate a choice (n = 6). After the peer review process, few students who initially chose Mechanism A revised to choose Mechanism B in their revised draft (n = 5). In contrast, slightly less than half of the students who initially chose Mechanism B revised to choose Mechanism A in their revised draft (n = 46).
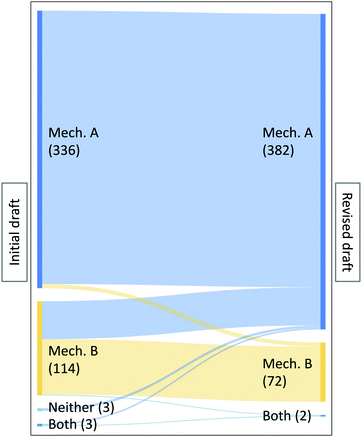 |
| Fig. 2 Sankey diagram representing the pathway students selected as most likely in their initial and revised drafts, illustrating the proportion of students making different types of global revisions. The total sample size reflected in the diagram is N = 456. | |
Research question one: what features of the RCDs and EPFs do students use in their writing when reasoning about organic reaction mechanisms?
We present our analysis of the features present in students’ initial drafts as captured by the qualitative coding process to address this research question. Each code corresponds to one or both of the mechanistic representations students used to support their identification of the most likely mechanistic pathway. We sought to identify differences between students who identified different mechanisms as most likely. First, there is no significant difference between the total number of codes between students selecting the different mechanisms (Mann–Whitney U test, W = 2331, p = 0.25). This finding indicates that students selecting one mechanism did not tend to incorporate more features in their writing, as captured by the coding scheme, compared to students selecting the other mechanism. Next, we sought to identify if there were differences in the specific features students incorporated based on the chosen mechanism. The average frequency of each code appearing across students’ initial drafts is presented in Fig. 3. The significance levels are shown from the outcome of the Mann–Whitney U tests for differences in students’ first drafts depending on whether they selected Mechanism A or Mechanism B as the likely mechanistic pathway. The relevant data for this research question, including mean values, standard deviations, and Bonferroni corrected p-values, is also presented in Appendix 4, Table 7. These results indicate the specific features of students’ writing they incorporated to support their choice of the likely mechanistic pathway and which features were significantly different among students selecting the different mechanisms as most likely (with the significant codes being TBD adding and functional group).
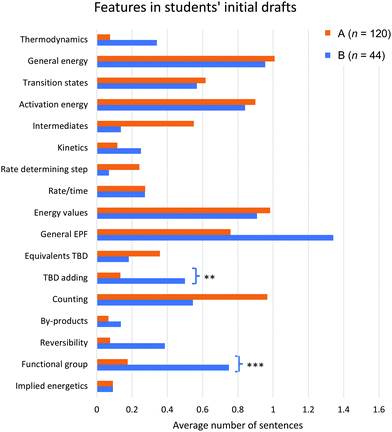 |
| Fig. 3 The average frequency of sentences for each code appearing in students’ initial drafts, separated by whether students indicted Mechanism A or Mechanism B as most likely. Definitions for each code can be found in Appendix 2, Table 5. Significant differences between groups are indicated with *p < 0.05, **p < 0.01, ***p < 0.001. | |
Research question two: what changes do students make in the features present in their writing after peer review and revision?
We performed a similar analysis to investigate the features students used to guide their choice of the most likely mechanism in their revised drafts. For each student, the frequency of each code applied to their initial draft was subtracted from the frequency of each code applied to their revised draft, resulting in a value that indicates the frequency with which each code was added (or removed) upon revision. The average change in the frequency of each code appearing in students’ revisions, across the four revision groups in Table 2, are presented in Fig. 4. The significance levels are shown from the outcome of the Kruskal–Wallis tests for differences in students’ revisions across the four groups. The relevant data for this research question, including mean values, standard deviations, and Bonferroni corrected p-values, is also presented in Appendix 4, Table 8. For the three codes with significant differences across revision groups, the results of the post hoc pairwise Mann–Whitney U tests are presented in Appendix 4, Table 9. These provide further specification about the groups between which the differences in revisions were significant. Altogether, the results for this research question indicate the specific codes with significant differences in the frequencies of revisions across the four revision groups (with the significant codes being TBD adding, counting, and functional group).
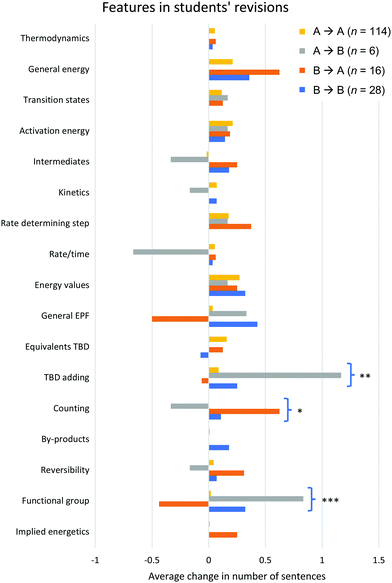 |
| Fig. 4 The average change in frequency of sentences for each code appearing in students’ revisions, separated by the nature of students’ global revisions. Definitions for each code can be found in Appendix 2, Table 5. Significant differences between groups are indicated with *p < 0.05, **p < 0.01, ***p < 0.001. | |
Research question three: How are students’ revisions linked to the components of the peer review process (both receiving and providing feedback)?
The final stage of the analysis sought to identify if and how the global revisions students made were connected to the two components of the peer review process: receiving feedback from peers and reviewing peers’ drafts. To do this, we performed three logistic regression analyses. The outcome variable for all regressions was whether students revised to select another mechanism. The predictor variables for the regressions were (1) the mechanism students indicated as most likely in their initial drafts, (2) the instances of disagreements encountered in peer review comments received, and (3) the instances of reviewing drafts that selected the opposite mechanism as most likely. The results of the three logistic regressions are presented in Table 3. The descriptive statistics for the variables included in the regression analysis are in Appendix 4, Table 10.
Table 3 Summary of the logistic regression models
Predictor |
Coeff. (st. err.) |
Exponent of coeff. |
p-Value |
N = 449. *p < 0.05, **p < 0.01, ***p < 0.001. |
Model 1. Logistic regression analysis without interaction terms
|
Initial draft mech. (A = 0, B = 1) |
1.44 (0.58) |
4.24 |
0.013* |
Comments received (freq. of disagreement) |
0.88 (0.24) |
2.41 |
<0.001*** |
Drafts reviewed (freq. of disagreement) |
1.45 (0.30) |
4.26 |
<0.001*** |
Intercept |
−6.58 (0.81) |
0.00 |
<0.001*** |
|
Model 2. Interaction analysis with students who initially selected Mechanism A as most likely as the reference group
|
Initial draft mech. (A = 0, B = 1) |
2.14 (1.71) |
8.51 |
0.210 |
Comments received (freq. of disagreement) |
1.16 (0.72) |
3.19 |
0.108 |
Drafts reviewed (freq. of disagreement) |
1.55 (0.50) |
4.72 |
0.002** |
Initial draft mech. × Comments received |
−0.33 (0.76) |
0.72 |
0.665 |
Initial draft mech. × Drafts reviewed |
−0.19 (0.63) |
0.83 |
0.766 |
Intercept |
−6.99 (1.29) |
0.00 |
<0.001*** |
|
Model 3. Interaction analysis with students who initially selected Mechanism B as most likely as the reference group
|
Initial draft mech. (A = 1, B = 0) |
−2.14 (1.71) |
0.12 |
0.210 |
Comments received (freq. of disagreement) |
0.82 (0.25) |
2.29 |
<0.001*** |
Drafts reviewed (freq. of disagreement) |
1.37 (0.38) |
3.92 |
<0.001*** |
Initial draft mech. × Comments received |
0.33 (0.76) |
1.39 |
0.665 |
Initial draft mech. × Drafts reviewed |
0.19 (0.63) |
1.21 |
0.766 |
Intercept |
−4.85 (1.13) |
0.01 |
<0.001*** |
Model 1 included only the three predictor variables, with Initial Draft Mech. = 0 for students who indicated Mechanism A and Initial Draft Mech. = 1 for students who indicated Mechanism B as most likely. Model 1 indicates that all three predictor variables significantly predict whether students will make global revisions to select the opposite mechanism in their final draft. The exponent of the coefficients (the odds ratios) of the predictor variables for Model 1 indicate the size of the influence. For example, the odds ratio of 4.24 for initial draft mechanism choice in Model 1 indicates that a student who initially selected Mechanism B as most likely has a 4.24 odds of making global revisions over a student who initially selected Mechanism A. Similarly, the odds ratio of 2.41 for the Comments Received variable in Model 1 indicates that a one unit increase in the number of peer review comments that state a disagreement increases the odds that the student will make global revisions by a factor of 2.41.
Model 2 and Model 3 included interaction terms to investigate the relationship between Initial Draft Mech. with the Comments Received and Drafts Reviewed variables. Model 2 used students who initially selected Mechanism A as the reference group (i.e., Initial Draft Mech. = 0 for students who initially indicated Mechanism A as most likely). Because of this, the exponent of the coefficients for the non-interaction terms (the odds ratios) are interpreted conditionally for students who initially selected Mechanism A (Jaccard, 2011). For example, the odds ratio of 4.72 for the Drafts reviewed variable in Model 2 indicates that, for students who initially selected Mechanism A as most likely, each one unit increase in the drafts Reviewed that selected Mechanism B increases the odds of global revisions by a factor of 4.72.
For Model 3, the odds ratios are interpreted conditionally for students who initially selected Mechanism B, as the model was calculated with students who initially selected Mechanism B as the reference group (i.e., Initial Draft Mech. = 0 for students who initially indicated Mechanism B as most likely). For example, the odds ratio of 3.92 for the Drafts Reviewed variable in Model 3 indicates that, for students who initially selected Mechanism B as most likely, each one unit increase in the drafts reviewed that selected Mechanism A corresponds to an increase in the odds of global revisions by a factor of 3.92. Note that the exponents of the interaction terms for Models 2 and 3 are not odds ratios and are therefore not to be interpreted in the same manner (Jaccard, 2011).
Model 2 results indicate that the only significant predictor variable for students who initially selected Mechanism A is Drafts Reviewed. However, the results for Model 3 indicate that both the Comments Received and Drafts Reviewed variables are significant predictors of global revisions for students who initially selected Mechanism B. Together, the results of all three models indicate a positive direction of influence for both components of the peer review process on the outcome of revising to select a different mechanism as most likely. That is, encountering a disagreement in both comments received or drafts reviewed influenced students’ revisions to select the mechanism that matched their peers. Thus, the results of Model 2 and Model 3 specifically provide insight into the nature of the interaction between the mechanism students initially selected as most likely and the influence of both components of the peer review process.
Discussion
The presented results indicate the general trends across students’ initial responses, revisions, and interactions in the peer review process. These trends point to the key findings and claims we can make from our analysis, considering the existing literature and theoretical frameworks guiding this study. The following discussion is organised by the key findings and claims.
Students largely selected the favoured mechanistic pathway as the most likely mechanism in both their initial and revised responses
This finding is promising as, at the end of the WTL process, most students successfully selected the most likely mechanistic pathway (n = 382, Fig. 2). Furthermore, most students who revised their choice of the most likely mechanistic pathway transitioned to select the appropriate pathway (n = 51 of 57 students, Fig. 2). That students made global revisions provides evidence that the WTL process encouraged reflection and revision for these students, an intended goal of WTL assignments (Hayes, 1996; Anderson et al., 2015; Gere et al., 2019). This result provides further evidence for the value of WTL in organic chemistry (Schmidt-McCormack et al., 2019; Watts et al., 2020; Gupte et al., 2021; Petterson et al., 2022), while extending the findings of prior studies by demonstrating students’ engagement with WTL on a task that required consideration of two reaction mechanisms, represented by both the EPF and RCDs. Additionally, this finding provides evidence for using the WTL process with peer review and revision to support students’ conceptual engagement within the organic chemistry course context.
Students across the dataset incorporated features from both representations in their responses
The closer analysis of students’ initial and revised drafts further supports the claim that the WTL assignment supported students’ conceptual engagement. The results for research questions two and three indicate that students across the dataset incorporated evidence from both the RCDs and EPF schemes to support their claim of the most likely mechanistic pathway. This indicates that the WTL assignment supported students’ use of representations in their reasoning, moving beyond engaging students with representations as simply being of a phenomenon (Kozma and Russell, 2005; Gouvea and Passmore, 2017). However, the findings indicate differences among students both within and between the groups who selected different mechanisms as most likely, suggesting nuance in students’ developing representational competence. Notably, different students who selected the same mechanism (e.g., different students who selected Mechanism A at some stage of the WTL process) occasionally exhibited different reasoning. This finding is described in detail in the following paragraphs and provides support for the calls in the literature to emphasize and evaluate the process of students’ reasoning rather than the final outcome or product of their reasoning (Anderson and Bodner, 2008; Grove and Bretz, 2012; Watts et al., 2020, 2021).
Students who selected Mechanism A as most likely reasoned by appealing to both chemically accurate and chemically inaccurate reasoning
In general, students who selected Mechanism A as most likely included two specific reasons: that Mechanism A had lower activation energy and that Mechanism A had fewer steps. For instance, one student reasoning with activation energy wrote:
“Mechanism A is more likely to occur because it has a lower activation energy and proceeds through lower energy intermediates and transition states than Mechanism B.”
Students who used this reasoning demonstrated an appropriate interpretation of the RCD representation. However, this finding is complicated because students often discussed energy in broader terms (as captured by the general energy code). Students’ explanations that referred to energy both in general terms and specifically with the phrase “activation energy” align with the research from both Lamichhane et al. (2018) and Popova and Bretz (2018c) by suggesting that some students may not have an understanding of activation energy in alignment with chemists’ interpretations. The result of the present study extends these findings by illustrating that some students could identify the appropriate concept and representation for completing the writing task (i.e., energetics derived from the RCDs) but may not have been able to connect the concept to the specific, appropriate feature of the RCD representation (i.e., peaks representing the activation energy). Students’ continued discussion of energy in general terms, rather than specific terms, after the cognitive processes of writing and revising indicates an area where instruction can be improved to support students’ representational competence (Flower and Hayes, 1981, 1984; Hayes, 1996; Kozma and Russell, 2005).
The other common reasoning among students who selected Mechanism A was based on the number of steps in the reaction. For example, one student wrote:
“This mechanism also has fewer steps which makes it more favorable when synthesizing for real application.”
This finding suggests a different approach to the task in comparison to the reasoning based on energetic considerations. Specifically, students who reasoned based on the number of steps demonstrated focus on a representational surface feature that could be drawn from either the RCD or EPF. Students’ writing that appealed to the surface features of the representations aligns with prior studies indicating students’ reliance on surface features for both RCDs (Lamichhane et al., 2018; Popova and Bretz, 2018a, 2018b, 2018c; Atkinson et al., 2021; Parobek et al., 2021) and the EPF (Strickland et al., 2010; Anzovino and Bretz, 2015; Galloway et al., 2017; Graulich and Bhattacharyya, 2017; Caspari et al., 2018; Dood et al., 2020; Watts et al., 2020). Students’ reliance on representational surface features is valuable to identify, as a key component of developing representational competence is being able to interpret the chemical meaning of representations when supporting their reasoning (Kozma and Russell, 2005). Specifically, the reasoning that the likelihood of alternative reaction mechanisms is based on the number of mechanistic steps is notable, as it represents a naïve view of mechanism that does not incorporate chemical reasoning. This approach to reasoning is in alignment with the type of rule-based reasoning that neglects chemical understanding, which has been reported in the literature (Kraft et al., 2010; Christian and Talanquer, 2012; Grove and Bretz, 2012; Cruz-Ramírez De Arellano and Towns, 2014; Watts et al., 2021). Furthermore, that the WTL assignment elicited this type rule-based of reasoning suggests the value of WTL for eliciting students’ understanding that may be difficult to elicit through other assessment approaches, which has been suggested in a prior study of WTL (Halim et al., 2018).
Students who selected Mechanism B as most likely reasoned by appealing more to the EPF than students who selected Mechanism A as most likely
Students who reasoned that Mechanism B was most likely incorporated reasoning more focused on the EPF, particularly with respect to the first step of Mechanism B (in which the TBD catalyst acts as a nucleophile and adds to the aldehyde). The only two significantly different codes identified in research question one related to this reasoning. This result suggests that students who selected Mechanism B were also writing about the features that guided other students’ choice of Mechanism A as most likely (e.g., features as captured by the codes general energy, activation energy, or counting). However, the students who selected Mechanism B also incorporated significantly more reasoning captured by the codes TBD adding and Functional group. The TBD adding code is exemplified by one student's response that.
“Mechanism B is the most likely pathway because unlike Mechanism A, TBD reacts with the aldehyde side of the ketoaldehyde first which prevents the possibility of the aldehyde reacting with TBD and forming an enol.”
Similarly, another student wrote:
“In Mechanism B, however, the first step eliminates this type of reactivity at the aldehyde since of the binding TBD which makes it an alcohol. This is much less likely to occur at the ketone due to steric hindrance, therefore making Mechanism B more selective than Mechanism A.”
This reasoning is drawn from the EPF and reflects the idea that TBD must first react with the aldehyde to act as a protecting group before the reaction can occur at the more sterically hindered ketone. Such reasoning focused on sterics has been demonstrated by students in a prior study (Bodé et al., 2019). While this reasoning about the EPF is reasonable—and can be supported by comparing the transition state energies for the initial steps for the two reaction mechanisms—it is notable that students based their selection of the most likely pathway on this feature of the EPF rather than the more appropriate features of the RCDs. This reflects students’ appropriate interpretation of the EPF representation, but their inappropriate selection of the representation most suited for the task of selecting the most likely reaction pathway. Hence, these students exhibited some aspects of representational competence, but not the ability to select the appropriate representation of a phenomenon for a specific task (Kozma and Russell, 2005).
The other code significantly more common among students who selected Mechanism B as most likely was the Functional group code. This is exemplified by a student who wrote:
“I believe that Mechanism B is more likely to occur because aldehydes are usually more reactive than ketones.”
This reasoning, similar to the TBD adding code, is focused on the idea that aldehydes are more reactive than ketones—but without the explicit reference to the steric hinderance argument. These students suggested that because aldehydes are more reactive, the reaction that starts with the aldehyde is more likely. This reasoning aligns with prior studies of students’ reasoning with functional group reactivity trends rather than the actual functions of said functional groups (Strickland et al., 2010; Watts et al., 2021). Furthermore, this reasoning focused primarily on reactivity trends is aligned with the rule-based reasoning strategy demonstrated by students in prior studies of their reasoning in organic chemistry (Kraft et al., 2010; Christian and Talanquer, 2012; Grove and Bretz, 2012; Cruz-Ramírez De Arellano and Towns, 2014; Watts et al., 2021). In addition, the focus on functional group is explicitly tied to features of the EPF (i.e., identifying functional groups) and plausibly tied to students’ connection-making between the EPF and RCDs (i.e., examining the reacting functional group on the EPF and the corresponding transition state energy on the RCD). Nevertheless, students exhibiting this reasoning included additional focus on the EPF, the representation less suited for the task of selecting the more likely reaction pathway—suggesting that these students are still developing their ability to select the most appropriate representation for a given task (Kozma and Russell, 2005).
Students’ revisions revealed similar trends in reasoning for selecting both Mechanism A and Mechanism B, while both reducing and eliciting students’ inaccurate reasoning
Similar trends as those identified from the sentence-level analysis of students’ first drafts were identified in students’ revisions. Students across the dataset generally revised to incorporate more features in their responses, with some exceptions (as seen in Fig. 4). However, the differences between students adding or removing features were not significant for most features identified in students’ writing. Three features, however, were significantly different across the different groups of students based on their global revisions: the features captured by the TBD adding, Functional group, and Counting codes. These features mirror the trends identified in students’ initial drafts. Students who initially selected Mechanism B and revised to select Mechanism A tended to revise their writing to remove the features corresponding to the relative reactivities of aldehydes and ketones while adding the reasoning based on counting the number of steps. It is notable that, through the cognitive processes of writing (Flower and Hayes, 1981, 1984; Hayes, 1996), many students reduced the prevalence of their reasoning about the EPF schemes that was less appropriate for the writing task when revising to select Mechanism A. However, the reverse was true for students who initially selected Mechanism A and revised to select Mechanism B. This trend follows from the results of analysing students’ initial drafts by suggesting that these features guided students’ decisions to identify Mechanism B as more likely. Altogether, the nature of students’ revisions suggests how the peer review and revision process might serve to elicit some students’ inappropriate reasoning that was not elicited in their initial drafts. This finding extends the WTL literature to support the notion that peer review and revision are useful for identifying reasoning that might be challenging to elicit through other means of assessment (Halim et al., 2018). Furthermore, this finding also indicates that while students may select the more likely mechanistic pathway, they do not always exhibit accurate chemical reasoning even after the complete WTL process. This supports the emphasis present in the literature to focus on eliciting, emphasizing, and evaluating students’ reasoning itself rather than the products or outcomes of their reasoning (Anderson and Bodner, 2008; Grove and Bretz, 2012; Watts et al., 2020, 2021).
Students’ global revisions are influenced by both reviewing their peers’ work and by receiving peer review comments
The final set of findings relates to students’ interactions within the peer review process and how it influenced students’ decisions to make global revisions. The logistic regression models indicate similar trends and key differences based on the mechanistic pathway students indicated as most likely in their initial drafts. For both groups of students, disagreements in the drafts reviewed significantly predicted students’ decisions to switch which mechanism they selected as most likely within their revisions. Furthermore, the odds ratios were higher for the disagreements in drafts reviewed compared to disagreements in comments received. Together, each of these findings indicate that students are influenced to a higher degree by the drafts they review than by the comments they received. The finding that student are influenced more by the drafts they review was suggested in prior studies of peer review, both in STEM WTL contexts (Finkenstaedt-Quinn et al., 2021a) and traditional writing courses (Lundstrom and Baker, 2009; Cho and MacArthur, 2010; Cho and Cho, 2011). However, for students who incorrectly selected Mechanism B as the more likely mechanism in their initial drafts, disagreements in the peer reviewer comments received were also statistically significant predictors of students’ global revisions. The same was not true for students who initially selected Mechanism A. This result suggests two claims: (1) that peer review comments can influence students’ decisions to revise (though a smaller effect than the drafts students review) and (2) that disagreements in peer review comments have more influence for students who initially display inaccurate reasoning. Altogether, the peer review analysis results indicate that students are influenced to make global revisions from both receiving comments and reviewing others’ drafts, and that the influence differs based on which mechanism they selected as most likely in their initial response. This aligns with previous studies of writing assignments which emphasize how the social components of writing can influence students’ engagement with all aspects of the WTL process, creating space to encourage reflection and metacognition (Anderson et al., 2015; Gere et al., 2019). Furthermore, this finding extends the literature by identifying a possible interaction between peer feedback received and the accuracy of students’ reasoning in their initial drafts.
Limitations
There are limitations associated with this study. First, the study took place within one laboratory course at a single institution, and thus findings may not be generalizable to all students or instructional settings. Students’ responses may have been influenced by the writing assignment taking place within a laboratory course; for example, writing assignments (e.g., laboratory reports) are more typical in laboratory courses compared to lecture courses at the study institution, so students may have been more receptive to completing the writing assignment in the laboratory course setting. Furthermore, data collection took place during remote learning due to the COVID-19 pandemic, which may have influenced the results. For instance, students may have engaged in fewer informal discussions surrounding the WTL assignment (e.g., while waiting for class to start) than might have been expected with in-person learning. This may have influenced students’ responses and/or the degree to which students engaged in the peer review process. There are additional limitations imposed by the approach to data collection and analysis. First, only students’ responses to the WTL process were collected. These responses serve as evidence of students’ reasoning, but no other data such as interviews were collected to triangulate the findings. Nevertheless, students’ abilities to use representational features to make claims suggest evidence of students’ reasoning through the cognitive processes of writing. Additionally, due to qualitatively coding on the sentence level where multiple codes could be applied to each sentence, the analysis is not suited for making claims about the different ways students reasoned within groups. For example, the analysis does not allow for strict categorization of students between the different reasonings for selecting Mechanism A (i.e., based on activation energy vs. based on counting the number of steps). However, this method of analysis was employed to account for all features students incorporated in their writing and to gain detailed perspective on the nature of students’ revisions. Furthermore, there are limitations associated with quantifying and performing statistical tests on qualitative data. Specifically, any biases that may have affected the qualitative data analysis would be carried through into the quantified data and ensuing statistical analyses, which may have influenced the nature of the results. A final limitation of the analysis is that the goal was to generalize students’ responses across the hundreds of participants in the study. Because of this, any individual student's response might represent reasoning that differs from the general trends identified in the analysis.
Conclusions and implications
This study describes our analysis of all components of a WTL assignment (students’ initial drafts, peer review, and revised drafts) in which students were given a writing task to consider two common mechanistic representations in organic chemistry (the EPF and RCDs) in their reasoning about the likelihood of two alternative mechanisms for a single transformation. The analysis indicated that many students correctly selected the mechanistic pathway accepted as most likely in both their initial and revised responses, with slightly less than half of the students who selected the less likely pathway revising to select the more appropriate choice. Students across the dataset drew on features of both the RCD and EFP representations in their responses. Students who selected the more appropriate pathway (Mechanism A) reasoned appropriately by appealing to the RCDs and comparisons of activation energy; students in this group also reasoned less appropriately about the number of steps in the mechanism. Students who selected the less likely pathway (Mechanism B) reasoned by appealing to the EPF, discussing both steric considerations and reflecting knowledge about general reactivity trends for aldehydes and ketones. These findings suggest students who incorporated more reasoning with the EPF tended to select the less likely pathway, indicating the need to develop their representational competence skill of selecting the most appropriate representation for the task of identifying the more likely reaction mechanism. Students’ revisions revealed similar trends in reasoning, with some students incorporating revisions that reflected inappropriate reasoning that was not revealed in their initial drafts. Finally, the peer review analysis indicates the potential influences of peer review on students’ revisions, providing evidence that reading drafts with different perspectives has a higher odds of influencing students’ global revisions compared to receiving feedback with disagreements. Altogether, these findings extend the literature by providing insight into organic chemistry students’ representational competence as presented in their responses to a WTL assignment. Further, the findings provide key implications for research and practice both for WTL interventions and for teaching organic chemistry.
Implications for research
The results of this study further the understanding of how students engage with representations in organic chemistry to support their reasoning about reaction mechanisms. The study expands the growing literature that utilizes writing analysis to access students’ engagement with disciplinary skills (Moon et al., 2019; Moreira et al., 2019; Watts et al., 2020). The present study specifically used writing analysis to provide insight into students’ representational competence through examining all components of a WTL assignment, a methodology that can similarly be used for future studies. Writing analysis of this type enables researchers to analyse the reasoning of large numbers of students participating in a course and is a strategy that can overcome some of the limitations of interview analyses which often include a smaller set of self-selected participants. Future research is merited to further investigate organic chemistry students’ representational competence. While many existing studies provide insight into how students interpret the features of organic chemistry representations, the present study indicates how students use representations for a specific task. Future research is necessary to explore how students use representations for other tasks similar to the work of practicing chemists. Additionally, further research is necessary for investigating other aspects of students’ developing representational competence, as outlined by Kozma and Russell (2005), such as how students make connections between representations in their reasoning. There is also a need for further research into the components of the WTL process, including studies that investigate more specifically what influences students to make both global and sentence-level revisions across the peer review process.
Implications for practice
There are a variety of implications for teaching associated with this study. First, the study provides details on students’ representational competence with the primary representations for organic reaction mechanisms. Understanding how students think about these representations at the introductory level is important for knowing how students might think about and approach different problems when learning organic chemistry. For example, knowing that some students may think that reaction mechanisms with fewer steps are more likely is valuable for teaching other reaction mechanisms in organic chemistry where alternative mechanistic pathways have different numbers of steps, such as substitution and elimination reactions. This study also provides a WTL assignment that engaged students with developing their representational competence, specifically with the ability to select the appropriate representation when completing a specific task. Activities which engage students in this type of task are especially important, as this is a component of representational competence that may not often be emphasized in introductory organic chemistry (Popova and Jones, 2021). The results of the analysis of students’ responses to this assignment suggest that teaching should specifically target the different uses for different representations, in alignment with the representational competence framework and calls to teach the epistemic practices surrounding developing and using models (Kozma and Russell, 2005; NGSS Lead States, 2013; Passmore et al., 2014, 2016; Gouvea and Passmore, 2017). Specifically, instruction could be improved by exposing students to both common representations of organic reaction mechanisms throughout the introductory organic chemistry curriculum with emphasis on what information each representation provides and how each representation can be used for different problems in organic chemistry. This study also provides insight into the value of using WTL assignments within the organic chemistry classroom, particularly in that writing assignments can elicit students’ inappropriate or non-chemical reasoning that might be difficult to elicit through other assignments or assessments. This study specifically suggests the value of implementing peer review and revision with WTL assignments for identifying how students reason with multiple representations. Lastly, this study provides support for the key implication of evaluating students’ reasoning itself rather than the product of students’ reasoning, as the findings indicate that students can use inaccurate reasoning to arrive at correct answers.
Conflicts of interest
There are no conflicts to declare.
Appendix 1. WTL assignment and peer review criteria
Exploring possible reaction pathways for a catalyzed intramolecular aldol reaction
Ivermectin is a drug used to treat onchocerciasis, a parasitic disease commonly known as river blindness. While the disease is rare in the United States, it is especially prevalent in Ghana, where more than 15% of the population is affected. As a lab technician for Médecins Sans Frontieres (Doctors Without Borders), you have traveled to Ghana to collaborate on a study initiated by biochemists at the University of Ghana who are working to develop a more efficient synthesis of ivermectin. The biochemists you are working with have identified a new strategy to perform intramolecular aldol reactions that uses the catalyst triazabicyclodecene (TBD). The TBD-catalyzed aldol reaction could be used in the place of the traditional aldol reaction for an early synthetic step in the synthesis of ivermectin. Using TBD will replace the need of strong acids and bases in this synthetic step, which will limit undesired side reactions. An example of a TBD-catalyzed aldol reaction with a simplified starting material is shown in Fig. 5.
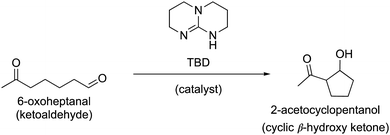 |
| Fig. 5 The intramolecular, TBD-catalyzed aldol reaction of 6-oxoheptanal produces 2-acetocyclopentanol. | |
The biochemists you are working for have asked you to research the mechanisms for the reaction. This will help them determine the feasibility of applying it to the synthesis of ivermectin. You have identified two potential mechanistic pathways, shown below in Fig. 6 and 7.
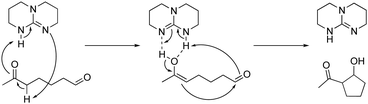 |
| Fig. 6 Proposed Mechanism A. | |
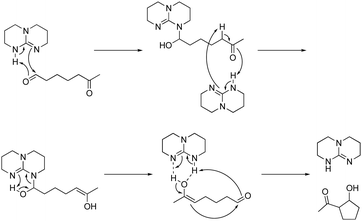 |
| Fig. 7 Proposed Mechanism B. | |
For each proposed pathway, you have performed computer simulations to determine their energy profiles. The results of your calculations are shown in Fig. 8, where each reaction coordinate diagram is presented side-by-side.
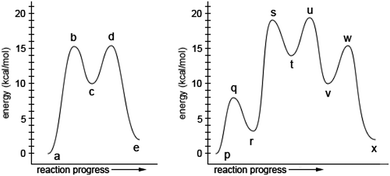 |
| Fig. 8 Reaction coordinate diagrams for Mechanism A (left) and Mechanism B (right). Note that claims about reaction times between Mechanism A and B can’t be made since the units on the horizontal axes aren’t specified. | |
At the end of the summer, you will write a brief report to summarize your findings, suggest the most likely pathway, and share your part of the project with the rest of the team. You should provide a detailed explanation of the mechanisms for both reaction pathways. Also, your argument for the most likely pathway should be supported by the mechanisms and the reaction coordinate diagrams. The report is directed toward the biochemists and other concerned parties who will use your recommendations to decide the feasibility of applying this reaction to the more complicated synthesis of ivermectin. Therefore, they may not be experts when it concerns mechanisms or organic-specific terms. Use clear and concise language, striking a balance between organic jargon and oversimplified explanations.
Your report should be approximately between 500–700 words (1–2 pages) in length. It should address the following points:
1. Discuss how each mechanism correlates with the corresponding energy diagram.
a. Summarize the findings.
b. Specifically, explain how the transition states and intermediates of the mechanisms correspond to features on the diagrams.
c. Take care to translate which specific step in the mechanism corresponds to which specific feature of the associated reaction coordinate diagram.
2. Identify which reaction pathway you think is most likely to occur. You will be evaluated on the explanation of your choice, not the choice itself.
3. When discussing mechanisms, be sure to write about the structural features and electronics of the molecules involved. Include descriptions of how the molecules interact in the mechanism and how they change in structure as a result of their interactions.
You can and should include figures of schemes, structures, mechanisms, or reaction coordinate diagrams, if that supports your response. We suggest that you have the figure(s) in front of you—ready to color-code or mark-up in various ways—and that you use your visible thinking to guide your audience through your explanation. Any images that you include in your response, including the figures in this prompt or those that you draw in ChemDraw or on paper, must have the original source cited using either ACS or APA format. Given your audience, your written response should suffice so that the explanations can be understood without the figures. You will be graded only on your written response.
Peer review guidelines
• Print and read over your peer's essay to quickly get an overview of the piece.
• Read the essay more slowly keeping the rubric in mind.
• Highlight the pieces of texts that let you directly address the rubric prompts in your online responses.
• In your online responses, focus on larger issues (higher order concerns) of content and argument rather than lower order concerns like grammar and spelling.
• Be very specific in your responses, referring to your peer's actual language, mentioning terms and concepts that are either present or missing, and following the directions in the rubric.
• Use respectful language whether you are suggesting improvements to or praising your peer.
1. In what ways does the author discuss the structural features of the molecules and the changes that result from the interactions in Mechanism A? Suggest ways the author could improve their mechanistic description.
2. In what ways does the author discuss the structural features of the molecules and the changes that result from the interactions in Mechanism B? Suggest ways the author could improve their mechanistic description.
3. How does the author relate the mechanistic details to the corresponding energy diagram for each mechanism? Suggest specific ways the author could relate each mechanism to features of its energy diagram.
4. Which mechanistic pathway did the author choose as the most likely? State what choice you think the author made and whether or not you think the author made the correct choice. Provide an explanation for why you think this way.
5. How did the author justify their choice of the most likely mechanistic pathway? Suggest ways the author could use details from their mechanism and energy diagram descriptions to better explain their choice.
Appendix 2. Coding schemes
Table 4 Coding scheme for the first analysis stage, in which initial and revised drafts were coded for the mechanism students indicated as most likely
Code |
Definition |
Exemplar |
Mechanism A |
The student indicated Mechanism A as the most likely mechanism for the reaction |
“My belief is that the reaction undergoes Mechanism A because it requires much less energy than Mechanism B and does not form as many stabilized intermediates as Mechanism B.” |
|
Mechanism B |
The student indicated Mechanism B as the most likely mechanism for the reaction. |
“Although it involves more steps, I believe that Proposed Mechanism B is more likely to occur.” |
|
Both mechanisms |
The student indicated, in different parts of their response, both Mechanism A and Mechanism B as the most likely mechanism for the reaction |
“Based on the energy diagrams, it seems most likely that Mechanism B occurs over Mechanism A… As a result, Mechanism A requires marginally less energy to progress past the rate-determining step and is more likely to occur than Mechanism B.” |
|
Neither mechanism |
The student did not clearly indicate either mechanism as the most likely mechanism for the reaction in any part of their response |
N/A |
Table 5 Coding scheme for the second analysis stage, in which initial and revised drafts were coded at the sentence level for features guiding students’ responses
Code |
Definition |
Exemplar |
Thermodynamics |
The student uses the word “thermodynamics” to describe the thermodynamics of the reactions |
“Thermodynamically speaking, both reactions are equally favorable since both have a ΔG° of 2 kcal mol−1.” |
|
General energy |
The student uses the word “energy” OR the student gives a generic description of the energy required for the reaction. Can include comparing “energy” between different points on the RCD |
“Mechanism A will be favored because it is much more energetically favored than Mechanism B.” |
|
Transition states |
The student considers the highest energy or the transition state peaks on the RCDs, including phrases like “first peak.” |
“In determining the success of either mechanism in producing the desired product, one must consider the energy levels of transition states in either reaction and their levels of reversibility.” |
|
Activation energy |
The student refers to the activation energy of the reaction. This can include mentions of the “first activation energy” or “sum of activation energies.” |
“This indicates that this pathway is more likely to progress because the overall sum of activation energies is lower than for B.” |
|
Intermediates |
The student describes the energy level for the intermediates of the mechanism |
“Also, in Mechanism B, intermediate R is nearly as stable as the desired product and it would take 16 kcal mol−1 to continue the reaction forward–this means that intermediate R would probably form in high amounts, and it would probably reverse back to the starting material as well.” |
|
Kinetics |
The student uses the word “kinetics” to describe the kinetics of the reactions |
“Thus, Mechanism B is more kinetically favored and more likely to occur.” |
|
Rate determining step |
The student uses the phrase “rate determining step” to specifically describe a mechanistic step |
“The rate determining step(s) for Mechanism A is 15 kcal mol−1, compared to 19 kcal mol−1 in Mechanism B.” |
|
Rate/time |
The student refers to the rate, time, or speed of the reactions. (As the literature indicates students’ challenges with interpreting the x-axis on RCDs, these features were captured by a single code so as not to interpret unintended meaning in responses referring to the rate, time, or speed of reactions.) |
“Mechanism A is preferred because it is selective for the desired keto–aldol product, has lower energy transition states and has a faster overall reaction time.” |
|
Energy values |
The student uses specific energy values or labels from the reaction coordinate diagram |
“Mechanism A's rate-determining step requires 15 kcal mol−1 of energy and occurs between the reactant a and intermediate c, while Mechanism B's rate-determining step uses 17 kcal mol−1 of energy and occurs between intermediate r and intermediate t.” |
|
General EPF |
The student uses general features of the EPF schemes, including descriptions of electron-pushing or changes in bonding, to justify their choice of the most likely mechanism |
“Mechanism A occurs without forming and breaking a bond between the catalyst and reagent and only uses one equivalent of TBD, making this mechanism much more favorable and cost-effective for your company.” |
|
Equivalents TBD |
The student refers to the equivalents of TBD catalyst added to the reaction |
“Additionally, Pathway A only requires one equivalent of TBD where Pathway B requires two.” |
|
TBD adding |
The student describes the addition of TBD to the aldehyde in Mechanism B and/or specifically uses the words or phrases “attaching,” “acting as a nucleophile,” “protecting group,” or “complexation” to describe the addition of TBD to the aldehyde |
“All the steps seen in Mechanism A occur in Mechanism B, however, Mechanism B involves additional steps that involve the addition and removal of TBD for the protecting and deprotecting of the aldehyde.” |
|
Counting |
The student counts transition states, intermediates, or reaction steps OR uses words, such as “fewer,” “more,” “additional,” or “only,” referring to the number of steps in each reaction |
“This mechanism has less steps than Mechanism A, and therefore the reaction would take less energy to create the product.” |
|
By-products |
The student refers to the formation of unwanted by-products, usually when students make an argument that more steps lead to more unwanted by-products |
“Mechanism A involves only one intermediate, but with a higher activation energy, while Mechanism B offers a lower activation energy for its first step, but with several more intermediate structures/byproducts.” |
|
Reversibility |
The student refers to the reversibility or irreversibility of the reaction, describing whether products are likely (or unlikely) to revert back to reactants OR uses words “reversible” or “irreversible.” |
“In determining the success of either mechanism in producing the desired product, one must consider the energy levels of transition states in either reaction and their levels of reversibility.” |
|
Functional group |
The student refers to a functional group (commonly ketone, aldehyde, or enol) to make an argument about the reactivity, stability, favourability, or likelihood of one reaction pathway compared to the other |
“The aldehyde is much more reactive than the ketone and therefore would use less energy.” |
|
Implied energetics |
The student uses the words “stable,” “unstable,” “favorable,” “unfavorable,” “more likely,” or “less likely” to make an argument about the likelihood of the chosen reaction pathway, without referring specifically to energy levels, features on the energy diagram, or to specific functional groups |
“This intermediate in Mechanism A is more stable than those in B's due to hydrogen bonding and is therefore more favorable.” |
Table 6 Coding scheme for the third analysis stage, in which peer review comments were coded for instances of clear agreement or clear disagreement
Code |
Definition |
Exemplar |
Agree |
Within the provided peer review comment, the student indicated clear agreement with the mechanism the author of the reviewed draft indicated as most likely |
“They chose Mechanism A as the pathway that the molecules would most likely go through… I think the author made the correct choice, but there isn't much evidence to back up their claim…” |
|
Disagree |
Within the provided peer review comment, the student indicated clear disagreement with the mechanism the author of the reviewed draft indicated as most likely |
“The author chose Mechanism B as the preferred method… I personally disagree since more equivalents of TBD were required…” |
|
Neutral |
Within the provided peer review comment, the student indicated neither clear agreement nor clear disagreement with the mechanism the author of the reviewed draft indicated as most likely |
“The author chose Mechanism B and it seems reasonable how product formation has become selective with the creation of an alcohol. My explanation was for Mechanism A and how a lower transition state energy would be more easier to overcome and thus at a higher probability rate.” |
Appendix 3. Examples of coded responses
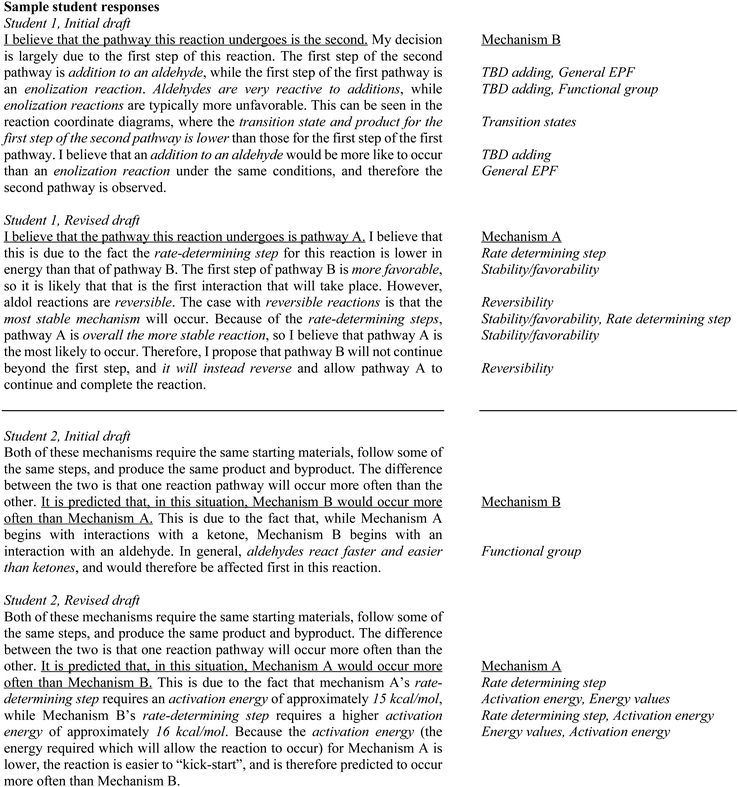 |
| Fig. 9 Two examples of students' initial and revised drafts with the codes applied from the first and second analysis stages. | |
Appendix 4. Tabular results and statistics
Table 7 Mean and standard deviation for each coded feature of students’ responses among students selecting either Mechanism A or Mechanism B as most likely. Reported p-values are from the Mann–Whitney U tests with the Bonferroni correction for multiple hypothesis tests
Code |
Selected Mechanism A (n = 120), Mean (St. dev.) |
Selected Mechanism B (n = 44), mean (St. dev.) |
p-Values |
*p < 0.05, **p < 0.01, ***p < 0.001. |
Thermodynamics |
0.08 (0.29) |
0.34 (0.86) |
0.062 |
General energy |
1.01 (0.89) |
0.95 (1.12) |
1.000 |
Transition states |
0.62 (0.79) |
0.57 (0.97) |
1.000 |
Activation energy |
0.90 (1.23) |
0.84 (1.27) |
1.000 |
Intermediates |
0.55 (0.99) |
0.14 (0.51) |
0.074 |
Kinetics |
0.12 (0.41) |
0.25 (0.72) |
1.000 |
Rate determining step |
0.24 (0.74) |
0.07 (0.25) |
1.000 |
Rate/time |
0.28 (0.62) |
0.27 (0.66) |
1.000 |
Energy values |
0.98 (1.17) |
0.91 (1.20) |
1.000 |
General EPF |
0.76 (1.17) |
1.34 (1.63) |
0.878 |
Equivalents TBD |
0.36 (0.58) |
0.18 (0.58) |
0.310 |
TBD adding |
0.13 (0.47) |
0.50 (0.88) |
0.006** |
Counting |
0.97 (0.89) |
0.55 (0.66) |
0.107 |
By-products |
0.07 (0.28) |
0.14 (0.51) |
1.000 |
Reversibility |
0.08 (0.32) |
0.39 (0.89) |
0.077 |
Functional group |
0.18 (0.67) |
0.75 (0.99) |
<0.001*** |
Implied energetics |
0.09 (0.32) |
0.09 (0.29) |
1.000 |
Total number of codes |
7.39 (3.67) |
8.27 (4.41) |
1.000 |
Table 8 Mean and standard deviation for the changes in each coded feature of students’ revisions, among the groups of students by the nature of their global revisions. Reported p-values are from the Kruskal–Wallis one-way analysis of variance by ranks tests adjusted with the Bonferroni correction for multiple hypothesis tests
Code |
Change in number of codes for A → A (n = 114), Mean (St. dev.) |
Change in number of codes for A → B (n = 6), Mean (St. dev.) |
Change in number of codes for B → A (n = 16), Mean (St. dev.) |
Change in number of codes for B → B (n = 28), Mean (St. dev.) |
p-Value |
*p < 0.05, **p < 0.01, ***p < 0.001. |
Thermodynamics |
0.05 (0.22) |
0.00 (0.00) |
0.06 (0.25) |
0.04 (0.43) |
1.000 |
General energy |
0.21 (0.84) |
0.00 (0.63) |
0.63 (1.15) |
0.36 (0.95) |
1.000 |
Transition states |
0.11 (0.56) |
0.17 (0.98) |
0.13 (0.89) |
0.00 (0.47) |
1.000 |
Activation energy |
0.21 (0.95) |
0.17 (0.41) |
0.19 (1.17) |
0.14 (0.65) |
1.000 |
Intermediates |
−0.02 (0.44) |
−0.33 (1.03) |
0.25 (0.68) |
0.18 (0.55) |
1.000 |
Kinetics |
0.07 (0.34) |
−0.17 (0.41) |
0.00 (0.00) |
0.07 (0.38) |
1.000 |
Rate determining step |
0.18 (0.57) |
0.17 (0.41) |
0.38 (0.62) |
0.00 (0.00) |
0.697 |
Rate/time |
0.05 (0.35) |
−0.67 (1.21) |
0.06 (0.68) |
0.04 (0.19) |
1.000 |
Energy values |
0.27 (0.67) |
0.17 (0.41) |
0.25 (1.13) |
0.32 (0.94) |
1.000 |
General EPF |
0.04 (0.40) |
0.33 (0.52) |
−0.50 (1.26) |
0.43 (1.26) |
1.000 |
Equivalents TBD |
0.16 (0.43) |
0.00 (0.63) |
0.13 (0.81) |
−0.07 (0.26) |
1.000 |
TBD adding |
0.09 (0.37) |
1.17 (1.17) |
−0.06 (1.00) |
0.25 (0.75) |
0.003** |
Counting |
0.04 (0.73) |
−0.33 (0.52) |
0.63 (1.02) |
0.11 (0.42) |
0.028* |
By-products |
0.01 (0.16) |
0.00 (0.00) |
0.00 (0.00) |
0.18 (0.94) |
1.000 |
Reversibility |
0.04 (0.24) |
−0.17 (0.41) |
0.31 (0.87) |
0.07 (0.72) |
1.000 |
Functional group |
0.02 (0.13) |
0.83 (1.33) |
−0.44 (0.81) |
0.32 (0.82) |
<0.001*** |
Implied energetics |
0.01 (0.31) |
0.00 (0.00) |
0.25 (0.77) |
0.00 (0.27) |
1.000 |
Total number of codes |
1.54 (2.50) |
1.33 (3.14) |
2.25 (3.42) |
2.43 (3.17) |
1.000 |
Table 9 The p-values for the post hoc pairwise Mann–Whitney U tests for statistically significant codes in the Kruskal–Wallis tests. The p-values are adjusted using the Bonferroni correction for multiple hypothesis tests
Code |
|
A → A |
A → B |
B → A |
*p < 0.05, **p < 0.01, ***p < 0.001. |
TBD adding |
A → B |
<0.001*** |
— |
— |
B → A |
1.000 |
0.099 |
— |
B → B |
1.000 |
0.101 |
1.000 |
|
Counting |
A → B |
0.520 |
— |
— |
B → A |
0.008** |
0.124 |
— |
B → B |
1.000 |
0.066 |
0.018* |
|
Functional group |
A → B |
<0001*** |
— |
— |
B → A |
<0.001*** |
0.205 |
— |
B → B |
0.009** |
1.000 |
0.031* |
Table 10 Descriptive statistics for variables included in the logistic regression analysis
Variable |
Mean |
St. dev. |
Min |
Max |
Initial draft mech. (A = 0, B = 1) |
0.25 |
0.43 |
0 |
1 |
Comments received (freq. of disagreement) |
0.57 |
0.79 |
0 |
4 |
Drafts reviewed (freq. of disagreement) |
1.15 |
1.04 |
0 |
3 |
Acknowledgements
The authors would like to thank the Keck Foundation and the University of Michigan Third Century Initiative for funding. This material is based upon work supported by the National Science Foundation Graduate Research Fellowship Program under Grant No. DGE 1256260. The authors would additionally like to thank Solaire A. Finkenstaedt-Quinn, Jennifer A. Schmidt-McCormack, Ina Zaimi, Ashley Karlin, Atia Sattar, Barry C. Thompson, and Anne Ruggles Gere for their input on the WTL assignment central to this investigation. Furthermore, we would like to thank Amber Dood, Eleni Zotos, and members of the Shultz grouup for discussions related to the preparation of this manuscript.
References
- Anderson T. L. and Bodner G. M., (2008), What can we do about “Parker”? A case study of a good student who didn’t “get” organic chemistry, Chem. Educ. Res. Pract., 9(2), 93–101.
- Anderson P., Anson C. M., Gonyea R. M. and Paine C., (2015), The contributions of writing to learning and development: Results from a large-scale multi-institutional study, Res. Teach. Engl., 50(2), 199–235.
- Anzovino M. E. and Bretz S. L., (2015), Organic chemistry students’ ideas about nucleophiles and electrophiles: The role of charges and mechanisms, Chem. Educ. Res. Pract., 16(4), 797–810.
- Anzovino M. E. and Bretz S. L., (2016), Organic chemistry students’ fragmented ideas about the structure and function of nucleophiles and electrophiles: A concept map analysis, Chem. Educ. Res. Pract., 17(4), 1019–1029.
- Atkinson M. B., Popova M., Croisant M., Reed D. J. and Bretz S. L., (2020), Development of the reaction coordinate diagram inventory: Measuring student thinking and confidence, J. Chem. Educ., 97(7), 1841–1851.
- Atkinson M. B. and Bretz S. L., (2021), Measuring changes in undergraduate chemistry students’ reasoning with reaction coordinate diagrams: A longitudinal, multi-institution study, J. Chem. Educ., 98(4), 1064–1076.
- Atkinson M. B., Croisant M. and Bretz S. L., (2021), Investigating first-year undergraduate chemistry students’ reasoning with reaction coordinate diagrams when choosing among particulate-level reaction mechanisms, Chem. Educ. Res. Pract., 22(1), 199–213.
- Berg S. A. and Moon A., (2022), Prompting hypothetical social comparisons to support chemistry students’ data analysis and interpretations, Chem. Educ. Res. Pract, 23(1), 124–136.
- Bodé N. E., Deng J. M. and Flynn A. B., (2019), Getting past the rules and to the WHY: Causal mechanistic arguments when judging the plausibility of organic reaction mechanisms, J. Chem. Educ., 96(6), 1068–1082.
- Brandfonbrener P. B., Watts F. M. and Shultz G. V., (2021), Organic chemistry students’ written descriptions and explanations of resonance and its influence on reactivity, J. Chem. Educ., 98(11), 3431–3441.
- Caspari I., Kranz D. and Graulich N., (2018), Resolving the complexity of organic chemistry students’ reasoning through the lens of a mechanistic framework, Chem. Educ. Res. Pract., 19(4), 1117–1141.
- Caspari I. and Graulich N., (2019), Scaffolding the structure of organic chemistry students’ multivariate comparative mechanistic reasoning, Int. J. Phys. Chem. Educ., 11(2), 31–43.
- Cho K. and MacArthur C., (2010), Student revision with peer and expert reviewing, Learn. Instr., 20(4), 328–338.
- Cho Y. H. and Cho K., (2011), Peer reviewers learn from giving comments, Instr. Sci., 39(5), 629–643.
- Christian K. and Talanquer V., (2012), Modes of reasoning in self-initiated study groups in chemistry, Chem. Educ. Res. Pract., 13(3), 286–295.
- Cohen J., (1960), A coefficient of agreement for nominal scales, Educ. Psychol. Meas., 20(1), 37–46.
- Cruz-Ramírez De Arellano D. and Towns M. H., (2014), Students’ understanding of alkyl halide reactions in undergraduate organic chemistry, Chem. Educ. Res. Pract., 15(4), 501–515.
- Dood A. J., Dood J. C., Cruz-Ramírez De Arellano D., Fields K. B. and Raker J. R., (2020), Analyzing explanations of substitution reactions using lexical analysis and logistic regression techniques, Chem. Educ. Res. Pract., 21(1), 267–286.
- Ferguson R. and Bodner G. M., (2008), Making sense of the arrow-pushing formalism among chemistry majors enrolled in organic chemistry, Chem. Educ. Res. Pract., 9(2), 102–113.
- Finkenstaedt-Quinn S. A., Halim A. S., Chambers T. G., Moon A., Goldman R. S., Gere A. R. and Shultz G. V., (2017), Investigation of the influence of a writing-to-learn assignment on student understanding of polymer properties, J. Chem. Educ., 94(11), 1610–1617.
- Finkenstaedt-Quinn S. A., Snyder-White E. P., Connor M. C., Gere A. R. and Shultz G. V., (2019), Characterizing peer review comments and revision from a writing-to-learn assignment focused on Lewis structures, J. Chem. Educ., 96(2), 227–237.
- Finkenstaedt-Quinn S. A., Halim A. S., Kasner G., Wilhelm C. A., Moon A., Gere A. R. and Shultz G. V., (2020a), Capturing student conceptions of thermodynamics and kinetics using writing. Chem. Educ. Res. Pract., 21, 922–939.
- Finkenstaedt-Quinn S. A., Watts F. M., Petterson M. N., Archer S. R., Snyder-White E. P. and Shultz G. V., (2020b), Exploring student thinking about addition reactions, J. Chem. Educ., 97(7), 1852–1862.
- Finkenstaedt-Quinn S. A., Petterson M., Gere A. and Shultz G., (2021a), Praxis of writing-to-learn: A model for the design and propagation of writing-to-learn in STEM, J. Chem. Educ., 98(5), 1548–1555.
- Finkenstaedt-Quinn S. A., Polakowski N., Gunderson B., Shultz G. V. and Gere A. R., (2021b), Utilizing peer review and revision in STEM to support the development of conceptual knowledge through writing, Writ. Commun, 38(3), 351–379.
- Flower L. and Hayes J. R., (1981), A cognitive process theory of writing, Coll. Compos. Commun., 32(4), 365–387.
- Flower L. and Hayes J. R., (1984), Images, plans, and prose: The representation of meaning in writing, Writ. Commun., 1(1), 120–160.
- Galloway K. R., Stoyanovich C. and Flynn A. B., (2017), Students’ interpretations of mechanistic language in organic chemistry before learning reactions, Chem. Educ. Res. Pract., 18(2), 353–374.
- Gere A. R., Limlamai N., Wilson E., MacDougall Saylor K. and Pugh R., (2019), Writing and conceptual learning in science: An analysis of assignments, Writ. Commun., 36(1), 99–135.
- Goodwin W., (2012), Mechanisms and chemical reaction, in Hendry R. F., Needham P. and Woody A. I. (ed.), Philosophy of Chemistry, Elsevier BV, pp. 309–327.
- Gouvea J. and Passmore C., (2017), ‘Models of’ versus ‘models for’: Toward an agent-based conception of modeling in the science classroom, Sci. Educ., 26(1–2), 49–63.
- Graulich N., (2015), The tip of the iceberg in organic chemistry classes: How do students deal with the invisible? Chem. Educ. Res. Pract., 16(1), 9–21.
- Graulich N. and Bhattacharyya G., (2017), Investigating students’ similarity judgments in organic chemistry, Chem. Educ. Res. Pract., 18(4), 774–784.
- Greene J. C., (2008), Is mixed methods social inquiry a distinctive methodology? J. Mix. Methods Res., 2(1), 7–22.
- Grimberg B. I. and Hand B., (2009), Cognitive pathways: Analysis of students’ written texts for science understanding, Int. J. Sci. Educ., 31(4), 503–521.
- Grove N. P. and Bretz S. L., (2012), A continuum of learning: From rote memorization to meaningful learning in organic chemistry, Chem. Educ. Res. Pract., 13(3), 201–208.
- Gupte
T., Watts F. M., Schmidt-McCormack J. A., Zaimi I., Gere A. R. and Shultz G. V., (2021), Students’ meaningful learning experiences from participating in organic chemistry writing-to-learn activities, Chem. Educ. Res. Pract., 22, 396–414.
- Halim A. S., Finkenstaedt-Quinn S. A., Olsen L. J., Gere A. R. and Shultz G. V., (2018), Identifying and remediating student misconceptions in introductory biology via writing-to-learn assignments and peer review, CBE Life Sci. Educ., 17(2), 1–12.
- Hammer D. and Elby A., (2003), Tapping epistemological resources for learning physics, J. Learn. Sci., 12(1), 53–90.
- Hammer D., Elby A., Scherr R. E. and Redish E. F., (2004), Resources, framing, and transfer, in Mestre J. (ed.), Transfer of Learning: Research and Perspectives, Greenwich, CT: Information Age Publishing.
- Hammar P., Ghobril C., Antheaume C., Wagner A., Baati R. and Himo F., (2010), Theoretical mechanistic study of the TBD-catalyzed intramolecular aldol reaction of ketoaldehydes, J. Org. Chem., 75(14), 4728–4736.
- Hayes J. R., (1996), A new framework for understanding cognition and affect in writing, in Levy C. M. and Ransdell S. (eds.), The Science of Writing: Theories, Methods, Individual Differences, and Applications, Mahwah, New Jersey: Lawrence Erbaum Associates, pp. 1–27.
- Jaccard J., (2011), Interactions between qualitative and quantitative/continuous predictors, in Interaction Effects in Logistic Regression, Thousand Oaks: SAGE Publications, Inc., pp. 31–41.
- Johnstone A. H., (1991), Why is science difficult to learn? Things are seldom what they seem, J. Comput. Assist. Learn., 7, 75–83.
- Kirilenko A. P. and Stepchenkova S., (2016), Inter-coder agreement in one-to-many classification: Fuzzy kappa, PLoS One, 11(3), 1–14.
- Kozma R. B. and Russell J., (1997), Multimedia and understanding: Expert and novice responses to different representations of chemical phenomena, J. Res. Sci. Teach., 34(9), 949–968.
- Kozma R., Chin E., Russell J. and Marx N., (2000), The roles of representations and tools in the chemistry laboratory and their implications for chemistry learning, J. Learn. Sci., 9(2), 105–143.
- Kozma R. and Russell J., (2005), Students Becoming Chemists: Developing Representational Competence, in Gilbert J. K. (ed.), Visualization in Science Education. Models and Modeling in Science Education, Dordrecht: Springer, vol. 1.
- Kraft A., Strickland A. M. and Bhattacharyya G., (2010), Reasonable reasoning: Multi-variate problem-solving in organic chemistry, Chem. Educ. Res. Pract., 11(4), 281–292.
- Lamichhane R., Reck C. and Maltese A. V., (2018), Undergraduate chemistry students’ misconceptions about reaction coordinate diagrams, Chem. Educ. Res. Pract., 19(3), 834–845.
- Lieber L. and Graulich N., (2022), Investigating students’ argumentation when judging the plausibility of alternative reaction pathways in organic chemistry, Chem. Educ. Res. Pract, 23(1), 38–54.
- Lundstrom K. and Baker W., (2009), To give is better than to receive: The benefits of peer review to the reviewer's own writing, J. Second Lang. Writ., 18(1), 30–43.
- Marks L., Lu H., Chambers T., Finkenstaedt-Quinn S. and Goldman R. S., (2022), Writing-to-learn in introductory materials science and engineering, MRS Commun., DOI: 10.1557/s43579-021-00114-z.
- Miles M. B., Huberman A. M. and Saldana J., (2014), Qualitative data analysis: A methods sourcebook, 3rd edn, Los Angeles, CA: Sage.
- Moon A., Zotos E., Finkenstaedt-Quinn S., Gere A. R. and Shultz G., (2018), Investigation of the role of writing-to-learn in promoting student understanding of light–matter interactions, Chem. Educ. Res. Pract., 19(3), 807–818.
- Moon A., Moeller R., Gere A. R. and Shultz G. V., (2019), Application and testing of a framework for characterizing the quality of scientific reasoning in chemistry students’ writing on ocean acidification, Chem. Educ. Res. Pract., 20(3), 484–494.
- Moreira P., Marzabal A. and Talanquer V., (2019), Using a mechanistic framework to characterise chemistry students’ reasoning in written explanations, Chem. Educ. Res. Pract., 20(1), 120–131.
- NGSS Lead States, (2013), Next Generation Science Standards: For States, By States, Washington: The National Academies Press.
- Parobek A. P., Chaffin P. M. and Towns M. H., (2021), Location-thinking, value-thinking, and graphical forms: combining analytical frameworks to analyze inferences made by students when interpreting the points and trends on a reaction coordinate diagram, Chem. Educ. Res. Pract, 22(3), 697–714.
- Passmore C., Gouvea J. S. and Giere R., (2014), Models in science and in learning science: Focusing scientifi c practice on sense-making, in Matthews M. R. (ed.), International Handbook of Research in History, Philosophy and Science Teaching, Dordrecht: Springer Scinece + Business Media, pp. 1171–1202.
- Passmore C., Schwarz C. V and Mankowski J., (2016), Developing and using models, in Schwarz C., Passmore C. and Reiser B. J. (ed.), Helping Students Make Sense of the World Using Next Generation Science and Engineering Practices, NSTA Press, pp. 109–134.
- Petterson M. N., Watts F. M., Snyder-White E. P., Archer S. R., Shultz G. V. and Finkenstaedt-Quinn S. A., (2020), Eliciting student thinking about acid–base reactions via app and paper–pencil based problem solving, Chem. Educ. Res. Pract., 21(1), 878–892.
- Petterson M. N., Finkenstaedt-Quinn S. A., Gere A. R. and Shultz G. V., (2022), The role of authentic contexts and social elements in supporting organic chemistry students’ interactions with writing-to-learn assignments, Chem. Educ. Res. Pract, 23(1), 189–295.
- Popova M. and Bretz S. L., (2018a), “It's only the major product that we care about in organic chemistry”: An analysis of students’ annotations of reaction coordinate diagrams, J. Chem. Educ., 95(7), 1086–1093.
- Popova M. and Bretz S. L., (2018b), Organic chemistry students’ challenges with coherence formation between reactions and reaction coordinate diagrams, Chem. Educ. Res. Pract., 19(3), 732–745.
- Popova M. and Bretz S. L., (2018c), Organic chemistry students’ interpretations of the surface features of reaction coordinate diagrams, Chem. Educ. Res. Pract., 19(3), 919–931.
- Popova M. and Jones T., (2021), Chemistry instructors’ intentions toward developing, teaching, and assessing student representational competence skills, Chem. Educ. Res. Pract, 22(3), 733–748.
- QSR International Pty Ltd, (2018), NVivo qualitative data analysis software (Version 12).
- Raker J. R., Holme T. A. and Murphy K. L., (2013), The ACS exams institute undergraduate chemistry anchoring concepts content map IV: Physical chemistry, J. Chem. Educ., 95(2), 238–241.
- RStudio Team, (2018), RStudio: Integrated Development for R.
- Schmidt-McCormack J. A., Judge J. A., Spahr K., Yang E., Pugh R., Karlin A., et al., (2019), Analysis of the role of a writing-To-learn assignment in student understanding of organic acid–base concepts, Chem. Educ. Res. Pract., 20(2), 383–398.
- Sevian H. and Talanquer V., (2014), Rethinking chemistry: A learning progression on chemical thinking, Chem. Educ. Res. Pract., 15(1), 10–23.
- Sheskin D. J., (2011), Handbook of Parametric and Nonparametric Statistical Procedures, 5th edn, Boca Raton: CRC Press.
- Strickland A. M., Kraft A. and Bhattacharyya G., (2010), What happens when representations fail to represent? Graduate students’ mental models of organic chemistry diagrams, Chem. Educ. Res. Pract., 11(4), 293–301.
- Taber K. S., (2013), Revisiting the chemistry triplet: Drawing upon the nature of chemical knowledge and the psychology of learning to inform chemistry education, Chem. Educ. Res. Pract., 14(2), 156–168.
- Watts F. M. and Finkenstaedt-Quinn S. A., (2021), The current state of methods for establishing reliability in qualitative chemistry education research articles, Chem. Educ. Res. Pract., 22, 565–578.
- Watts F., Schmidt-McCormack J., Wilhelm C., Karlin A., Sattar A., Thompson B., et al., (2020), What students write about when students write about mechanisms: Analysis of features present in students’ written descriptions of an organic reaction mechanism, Chem. Educ. Res. Pract., 21, 1148–1172.
- Watts F. M., Zaimi I., Kranz D., Graulich N. and Shultz G. V., (2021), Investigating students’ reasoning over time for case comparisons of acyl transfer reaction mechanisms, Chem. Educ. Res. Pract., 22, 364–381.
- Weinrich M. L. and Talanquer V., (2016), Mapping students’ modes of reasoning when thinking about chemical reactions used to make a desired product, Chem. Educ. Res. Pract., 17(2), 394–406.
|
This journal is © The Royal Society of Chemistry 2022 |