DOI:
10.1039/D2RA04505B
(Paper)
RSC Adv., 2022,
12, 25617-25622
A rapid and sensitive UPLC-MS/MS method for simultaneous determination of four potential mutagenic impurities at trace levels in ripretinib drug substance
Received
21st July 2022
, Accepted 2nd September 2022
First published on 8th September 2022
Abstract
In the synthesis of ripretinib, a new oral tyrosine kinase inhibitor, impurities could arise directly from starting materials, reagents and intermediates. Among these process impurities, four specific intermediate impurities were found to contain the structural alerts of primary aromatic amine and aldehyde groups, triggering the concern of potential mutagenic impurities (PMIs). Two complementary (quantitative) structure–activity relationship [(Q)SAR] evaluation systems (expert rule-based and statistics-based) were subsequently employed to assess and classify the mutagenic risk of the four known impurities. The Sarah prediction results of these four impurities were all positive and they were categorized as class 3, where the threshold of toxicological concern (TTC) of 1.5 μg d−1 would apply. Hereby, a rapid and sensitive UPLC-MS/MS method was developed for the simultaneous and trace level quantification of the four PMIs in ripretinib drug substance. The separation was achieved on a C18 column under the optimized gradient elution program consuming only nine minutes and the four PMIs were all well separated from ripretinib so that they could be easily diverted to waste via a switch valve. The time-segmented multiple reaction monitoring (MRM) mode further improved the sensitivity and allowed for the quantification of the four PMIs as low as 10% of the acceptable limit. The method was fully validated, and proved sufficient in terms of selectivity, sensitivity, linearity, precision and accuracy. The factors involved in the method development and pathways for fragment ions of the four PMIs were also discussed and the study will contribute to risk management of PMIs present in ripretinib.
Introduction
Ripretinib (DCC-2618), a new switch control tyrosine kinase inhibitor for the treatment of gastrointestinal stromal tumors (GIST), is chemically known as 1-(4-bromo-5-[1-ethyl-7-(methylamino)-2-oxo-1,2-dihydro-1,6-naphthyridin-3-yl]-2-fluorophenyl)-3-phenylurea (Fig. 1).1,2 It was discovered and commercialized by Deciphera Pharmaceuticals and approved by the FDA in May 2020 with the brand name of QINLOCK®. The synthesis of ripretinib was reported by Flynn et al. in 2012 over eight steps from the starting material 3-oxo-pentanedioic acid diethyl ester.3,4
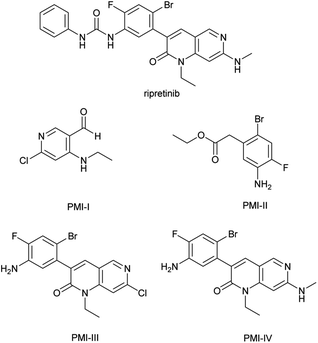 |
| Fig. 1 Structures of ripretinib and the four potential mutagenic impurities (PMIs). | |
Impurities that induce gene mutations and chromosomal aberration are considered as genotoxic impurities (GTIs).5–7 Mutagenic impurities (MIs) refer to the ones that have been demonstrated to be mutagenic in an appropriate mutagenicity test, e.g., bacterial reverse mutation (Ames) assay, which directly cause DNA damage at low levels and therefore, potentially cause cancer.6,7 Other types of GTIs that are non-mutagenic typically have threshold mechanisms and usually do not pose a carcinogenic risk in humans at ordinary levels, as explained in ICH M7(R1) and its question and answer supplement.6,7 Potential mutagenic impurities (PMIs) contain structural alerts but have unknown mutagenic and carcinogenic data.6–8
PMIs in drug substance come from multiple sources like starting materials, intermediates, reagents and byproducts. In particular, the residual of starting materials and intermediates was the direct source.9–11 Among the starting materials and intermediates employed in the industrial production of ripretinib, four impurities (Fig. 1) were found to contain the structural alerts of the primary aromatic amine and aldehyde groups by visual evaluation, triggering the concern of PMIs.12–15 These impurities could remain in the final drug substance as the residual intermediates.
Ripretinib has been approved in several countries and relevant information regarding its toxicity is publicly available. Ripretinib itself has been tested and found to be not mutagenic in an Ames assay or clastogenic in other in vivo and in vitro assay.16 However, toxicity data on pharmaceutical impurities are generally kept proprietary and public information is practically non-existent. Additionally, impurities should not be assessed based solely on a visual structural alert.7,17
(Quantitative) structure–activity relationships [(Q)SAR] technique is a highly recommended method to predict the Ames assay results according to the structural alerts, sequentially estimating and classifying the mutagenic risk of these compounds,9,10,17–20 since it is impractical to perform the toxicity experiment on each impurity. There is an expectation that structural alert assessment should be conducted using two complementary (Q)SAR systems, one expert rule-based and the other statistics-based, as proposed by ICH M7(R1).6,7 Certain commercial (Q)SAR systems, including Derek and Sarah,21 have been validated by the general validation principles set forth by OECD.22
The threshold of toxicological concern (TTC) of 1.5 μg d−1 is proposed to define an acceptable daily intake and applied to control the impurities categorized as class 2 and class 3 by (Q)SAR.6 Since the maximum daily dose (MDD) of ripretinib is 150 mg d−1, the acceptable limit for any PMIs in ripretinib was calculated to be 10 ppm, making it more challenging to quantify the PMIs at trace levels.23–26
To the best of our knowledge, no publication worked on the mutagenicity evaluation, as well as the separation and determination of impurities in ripretinib. In our work, we report the evaluation and classification of these four specific impurities by two (Q)SAR systems, and then present the development of a sensitive and rapid UPLC-MS/MS method for the simultaneous and trace level quantification of the four impurities in ripretinib drug substance.
Experimental
(Q)SAR tools
We followed the ICH M7(R1) guidelines using two in silico methods that complement each other, one expert rule-based and the other statistics-based.
Derek system was employed as the expert rule-based method. Derek version: Derek Nexus 6.1.1, knowledge: Derek KB 2020 1.0, knowledge version: 1.0, knowledge date: 26 March 2020, certified: yes.
Sarah system was employed as the statistics-based method. Sarah version: Sarah Nexus 3.1.1, model: Sarah Model 2020.1, model version: 1.8, certified: yes.
Nexus system, the integration platform of Derek and Sarah, can evaluate and automatically classify the compound mutagenicity as per ICH M7(R1) guidelines. Nexus version: Nexus 2.4.0, species: bacterium, endpoint: mutagenicity in vitro. These commercial software was developed by Lhasa, UK (https://www.lhasalimited.org/).
Reagents and chemicals
Acetonitrile and formic acid were of LC-MS grade and purchased from Thermo Scientific (USA). Water was purified by the Millipore Milli-Q purification system (Merck, France). Reference standards for PMI-I (purity 98.2%), PMI-II (purity 99.3%), PMI-III (purity 98.5%) and PMI-IV (purity 99.1%), as well as the active pharmaceutical ingredient (API) of ripretinib from three batches, were all provided from Yantai Institute of Materia Medica (Yantai, China).
UPLC-MS/MS conditions
Instruments. An UPLC-MS/MS instrument consisting of Waters Acquity I-Class UPLC system with a photo-diode array (PDA) detector and Xevo TQ-S MS system with an ESI ion source was employed.
Separation conditions. The separation was achieved on a Waters Acquity BEH C18 column (100 mm × 2.1 mm, 1.7 μm), using a gradient elution with the run time of 9 min. Mobile phase A was 0.1% formic acid in water (v/v) and mobile phase B was acetonitrile with the following gradient program [time (min)/solvent B (%)]: 0/25, 1/25, 4/40, 8/40, 8.1/25 and 9/25, at 0.3 mL min−1 flow rate. The column temperature was maintained at 30 °C while the sampler temperature was set at 20 °C, with the injection volume of 1 μL.
Mass conditions. ESI ion source parameters were set as follows: capillary voltage: 3 kV for positive mode; desolvation temperature: 500 °C; desolvation gas flow: 1000 L h−1; cone gas flow: 150 L h−1; nebuliser pressure: 7 bar; collision gas flow: 0.15 mL min−1. The time-segmented multiple reaction monitoring (MRM) mode was applied for the quantification of the four PMIs. The corresponding precursor ions, fragment ions, cone voltage, collision energy, segmented monitoring time and other MS parameters for the four PMIs were listed in Table 1. In particular, the flow in 4.5–5.5 min from LC was diverted into waste via a switch valve to protect the MS instrument from the separated ripretinib.
Table 1 Mass spectrometry parameters for the four PMIs
Compound |
Segmented monitoring time (min) |
Precursor ions (m/z) |
Fragment ions (m/z) |
Cone voltage (V) |
Collision energy (eV) |
Dwell time (s) |
Transition for quantification. |
PMI-I |
3.2–4.5 |
185.0 |
121.0a |
56 |
22 |
0.372 |
156.9 |
56 |
18 |
PMI-II |
4.5–7.3 |
276.0 |
201.9a |
18 |
14 |
0.372 |
123.9 |
18 |
32 |
PMI-III |
7.3–10.0 |
396.0 |
288.2a |
50 |
40 |
0.372 |
316.9 |
50 |
22 |
PMI-IV |
0–3.2 |
391.1 |
363.0a |
20 |
28 |
0.372 |
268.9 |
20 |
42 |
Preparation of sample and standard solutions
Diluents. Mobile phase A-mobile phase B (30
:
70, v/v) was used as the diluent.
Sample solutions. A Sample solution of 0.3 mg mL−1 was prepared as follows: about 15 mg of ripretinib API was accurately weighed into a 50 mL volumetric flask and then dissolved in 40 mL diluent by sonication at 30 °C for 30 min. The flask was cooled down to room temperature and filled to the mark with diluent. The resultant solution was mixed and filtered through a 0.22 μm PTFE syringe filter.
Standard solutions. A stock mixture of the four PMIs (75 ng mL−1) was prepared by dissolving appropriate amounts of each impurity in diluent. Lower concentrations were prepared by appropriate dilution of the stock solution with diluent. Concentration of the standard solution was 3 ng mL−1 (10 ppm with respect to 0.3 mg mL−1 of ripretinib).
Results and discussion
(Q)SAR evaluation data
The chemical structures of ripretinib and impurities were drawn by Chemdraw 20.0 and then input into the Nexus 2.4.0 system. The prediction results were shown in Table 2. Derek results were inactive while Sarah results were all positive.
Table 2 (Q)SAR prediction results of the four impurities
Compound |
Derek prediction |
Sarah prediction |
Experimental data |
Similarity to API |
Overall in silico |
ICH M7 class |
PMI-I |
Inactive |
Positive |
Carc/Ames: unspecified |
No Derek alerts found |
Positive |
Class 3 |
PMI-II |
Inactive |
Positive |
Carc/Ames: unspecified |
No Derek alerts found |
Positive |
Class 3 |
PMI-III |
Inactive |
Positive |
Carc/Ames: unspecified |
No Derek alerts found |
Positive |
Class 3 |
PMI-IV |
Inactive |
Positive |
Carc/Ames: unspecified |
No Derek alerts found |
Positive |
Class 3 |
Derek is the expert knowledge-based software that contains data from published sources and confidential and non-confidential data donated by the member organizations. The methodology uses a human-written rules based on empirical observations that are supported by an understanding of the toxicity mechanism or by the rigorous, internal vetting process. The recognition of the structural alerts is based on the expert-defined Markush structure.21 The inactive results mean that the query structures do not match any structural alerts or examples in Derek which show activity in Ames test.
On the other hand, Sarah software includes public data and donated non-confidential data. It uses a fully automated, hierarchical, machine-learning methodology to build a model for Ames test. The Sarah training set contains more than ten thousand individual structures which have been standardized and fragmented to build the Sarah model. Query structures imported into Sarah are standardized and then fragmented. The fragments are refined and ranked depending on the similarity of the query to the training set of compounds. The recognition of the structural alerts is based on machine learnt fragments.21 The positive results of the four impurities mean the query structure is predicted to be positive in a Ames test.
Nexus system, the integration platform of Derek and Sarah, recognized as positive and assigned the four specific impurities as class 3, indicating the potential mutagenicity. A TTC value of 1.5 μg d−1 (10 ppm with respect to MDD of ripretinib) could be applied as the acceptable limit without further testing as per ICH M7(R1) guideline.
Method development and optimization
Optimization of sample preparation. Ripretinib is a lipophilic, weak base compound, practically insoluble in aqueous solutions, exhibiting solubility of only 1.6 μg mL−1 in buffer (pH 2), and lower solubility in neutral or basic media, e.g., solubility of less than 1 μg mL−1 in buffer (pH 6.5). Ripretinib is sparingly soluble in polar aprotic organic solvents, including dimethylformamide, acetonitrile.The higher concentration of ripretinib is crucial to the detection sensitivity. Acidic aqueous solutions and the higher proportion of polar aprotic organic solvents would improve solubility of ripretinib. However, the higher proportion of organic phase could lead to solvent effect in RP chromatography. Various proportions of 0.1% formic acid aqueous solution and acetonitrile were screened and 0.1% formic acid–acetonitrile (30
:
70, v/v) was finally chosen as the diluent to obtain a API concentration of 0.3 mg mL−1 in consideration of the balance of solubility and solvent effect. The PMIs were also soluble in the selected diluents.
Development of chromatographic separation. Ripretinib and relevant four impurities are all weak base compounds with amino groups, thus acid mobile phases contribute to the subsequent ionization and avoid tailing. 0.1% Formic acid aqueous solution, widely used in LC-MS method, as well as acetonitrile was selected as the mobile phase. For the rapid analysis and better separation, various gradient elution programs were attempted in a small particle C18 column (100 mm × 2.1 mm, 1.7 μm). The four PMIs were all well separated from each other and ripretinib under current chromatographic conditions so that the API could be easily diverted to waste and the run time was only 9 min. The different brands and types of C18 columns with the same size specification have litter impact on the separation. In addition, the injection volume was set as 1 μL since the bigger injection volume even only 2 μL would lead to the solvent effect.
Development of MS parameters for quantitation. ESI ion source parameters were set according to the generally recommended values. The precursor ions, fragment ions, and corresponding cone voltage, collision energy for the four PMIs were screened and optimized for the best detection sensitivity. The pathways for fragment ions of the PMIs were demonstrated in Fig. 2.
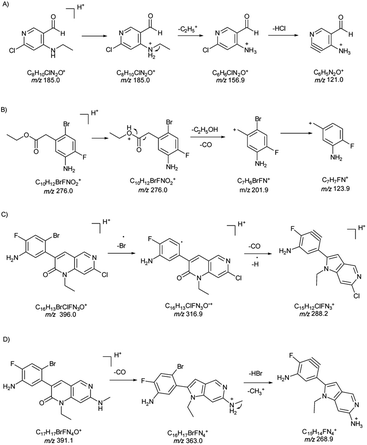 |
| Fig. 2 Pathways for fragment ions of (A) PMI-I, (B) PMI-II, (C) PMI-III and (D) PMI-IV. | |
In particular, the time-segmented MRM mode was applied in MS quantitation. The time-segmented mode assigns longer dwell time to each MRM transition under the condition of a fixed total scan time, thus increasing the signal response and sensitivity. To avoid any pollution from high API concentrations over the MS instrument, the flow in 4.5–5.5 min was diverted to waste via the switch event set in the MS method.
The established method was fully validated as per ICH Q2(R1) guideline in terms of selectivity, sensitivity, linearity, precision and accuracy.
Method validation
Specificity. The specificity of the method was checked by analyzing the blank solution and sample solution spiked with the four PMIs at the specification level (corresponding to 10 ppm), so as to demonstrate the ability of separation from API and other potential interfering impurities in the sample matrix. The monitoring of API could be achieved by the PDA detector at the absorption maximum of 252 nm since it was diverted to waste in the MS method. As a result, no extra peaks were found in the retention time of the PMIs in the blank solution. The four PMIs were eluted as the single peak and well resolved from each other and API. Typical chromatograms of the blank solution and sample solution spiked with the four PMIs were presented in Fig. 3.
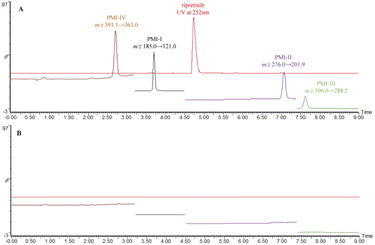 |
| Fig. 3 Typical chromatograms of (A) spiked sample solution and (B) blank solution. | |
LOD, LOQ and linearity. The LOD and LOQ values of the four PMIs were determined based on S/N of 3
:
1 and 10
:
1, by injecting a series of standard solutions diluted to known concentrations. As a result, the LOQ of the four PMIs was as low as 10% of the specification limit. Precision at LOQ level was also carried out by injecting six replicate and calculating % RSD (n = 6) for the areas as listed in Table 3.
Table 3 Validation results of LOD, LOQ, linear regression analysis, recovery and precision data
Parameters |
PMI-I |
PMI-II |
PMI-III |
PMI-IV |
Mean recovery for three determinations. |
LOD (ng mL−1) |
0.030 |
0.089 |
0.091 |
0.091 |
LOQ (ng mL−1) |
0.091 |
0.298 |
0.302 |
0.303 |
Linear range (ng mL−1) |
0.302–6.050 |
0.298–5.956 |
0.302–6.041 |
0.303–6.063 |
Slope |
13 681 |
17 133 |
7816.4 |
26 372 |
Intercept |
+221.76 |
+103.26 |
+279.55 |
−1618.7 |
Correlation coefficient |
1.0000 |
0.9999 |
0.9999 |
0.9992 |
% Y-intercept |
0.5 |
0.2 |
1.2 |
2.2 |
Precision of system (% RSD) |
1.0 |
0.8 |
1.1 |
1.2 |
Repeatability at 50% level (% RSD) |
1.6 |
2.4 |
1.5 |
3.0 |
Repeatability at 100% level (% RSD) |
1.1 |
1.3 |
1.5 |
2.1 |
Repeatability at 150% level (% RSD) |
0.9 |
1.1 |
1.8 |
1.7 |
Intermediate Precision (% RSD) |
2.3 |
1.1 |
1.2 |
1.8 |
Precision at LOQ (% RSD) |
0.9 |
1.7 |
2.2 |
2.9 |
% Recoverya at 50% level & % RSD |
100.1 & 1.6 |
102.6 & 2.5 |
103.2 & 1.5 |
103.5 & 3.0 |
% Recoverya at 100% level & % RSD |
100.1 & 1.1 |
101.5 & 1.3 |
100.9 & 1.5 |
106.5 & 2.0 |
% Recoverya at 150% level & % RSD |
99.2 & 1.0 |
101.8 & 1.0 |
101.0 & 1.8 |
104.8 & 1.7 |
The linear calibration curve was obtained by preparing standard solutions at six levels from 10% to 200% of the concentration limit, i.e. 0.3, 0.6, 1.5, 3.0, 4.5, 6.0 ng mL−1 for all PMIs (corresponding to 1, 2, 5, 10, 15, 20 ppm, respectively). The values of correlation coefficient, slope, intercept and % Y-intercept at concentration limit were calculated by the least-squares linear regression equation as shown in Table 3, which indicated significance linear correlation between the peak areas and concentrations for all four PMIs (P < 0.001).
Precision. The precision of system was evaluated by six-time consecutive injections of the standard solution. The RSD% (n = 6) for peak areas of the four PMIs was below 2.0.The repeatability was conducted by preparing the sample solutions spiked with PMIs at three levels of 50%, 100% and 150% of the concentration limit, i.e. 1.5, 3.0, 4.5 ng mL−1. Each concentration level was prepared in triplicate. The RSD% (n = 3) for peak areas of the four PMIs was calculated in Table 3.
Intermediate precision was examined by analysis of the spiked sample solutions in two different days. Each day six replicates of sample solutions spiked with 10 ppm of four PMIs were prepared by two analysts. The RSD% (n = 12) was shown in Table 3.
Accuracy. The accuracy of the method was checked by analyzing the sample solutions spiked with known amount of PMIs at three levels of 50%, 100% and 150% of the concentration limit, i.e. 1.5, 3.0, 4.5 ng mL−1 (5, 10, 15 ppm, respectively). Each concentration level was prepared in triplicate. Recoveries between the determined content and the added amount and the RSD% (n = 3) of recoveries were presented in Table 3.
Solution stability. Stability of the standard and spiked sample solution was examined by injecting the solution freshly prepared and that after the storage for 3 days in refrigerator (4–8 °C) and at room temperature (20–25 °C). The bias of the concentration of the four PMIs after the storage was less than 10.0%, demonstrating the standard and sample solution were stable for 3 days at least.
Analysis of samples. The developed and validated UPLC-MS/MS method was applied to the determination of the four PMIs in ripretinib API from three batches. In all batches, PMI-III was not detected. PMI-I was detected but in all cases below the LOQ. The amounts of PMI-IV was in the range of 0–4.8 ppm while PMI-II varied from 1.4 to 8.1 ppm, which indicated the four PMIs in all batches met the regulatory limit.
Conclusions
Four specific impurities in the synthesis of ripretinib were evaluated and classified regarding the mutagenicity by the two complementary (Q)SAR systems. The Sarah prediction results of the four impurities were all positive and they were categorized as class 3, indicating the potential mutagenicity. A rapid and sensitive UPLC-MS/MS method was subsequently developed for the simultaneous and trace level quantification of the four PMIs in ripretinib API. The run time was only nine minutes and the four PMIs were all well separated from ripretinib so that the API could be easily diverted to waste via a switch valve. The time-segmented MRM mode further improved the sensitivity and allowed for the quantification of the four PMIs as low as 10% of specification limit. The study will help to risk management of PMIs present in ripretinib.
Author contributions
Yiwen Huang: conceptualization, methodology, validation, formal analysis, investigation, writing – original draft. Qi Xu: software, validation, formal analysis. Zhong Li: resources, data curation. Hui Lu: validation, visualization. Yang Wu: resources, writing – review & editing, supervision, project administration.
Conflicts of interest
There are no conflicts to declare.
Acknowledgements
The authors are thankful to the management of Suzhou Institute for Drug Control for providing necessary facilities.
Notes and references
- B. D. Smith, M. D. Kaufman, W. P. Lu, A. Gupta, C. B. Leary, S. C. Wise, T. J. Rutkoski, Y. M. Ahn, G. Al-Ani, S. L. Bulfer, T. M. Caldwell, L. Chun, C. L. Ensinger, M. M. Hood, A. McKinley, W. C. Patt, R. Ruiz-Soto, Y. Su, H. Telikepalli, A. Town, B. A. Turner, L. Vogeti, S. Vogeti, K. Yates, F. Janku, A. R. Abdul Razak, O. Rosen, M. C. Heinrich and D. L. Flynn, Cancer Cell, 2019, 35, 738 CrossRef CAS PubMed.
- C. C. Ayala-Aguilera, T. Valero, A. Lorente-Macias, D. J. Baillache, S. Croke and A. Unciti-Broceta, J. Med. Chem., 2021, 65, 1047 CrossRef PubMed.
- X. X. Liang, Q. Yang, P. Wu, C. L. He, L. Z. Yin, F. N. Xu, Z. Q. Yin, G. Z. Yue, Y. F. Zou, L. X. Li, X. Song, C. Lv, W. Zhang and B. Jing, Bioorg. Chem., 2021, 113, 105011 CrossRef CAS PubMed.
- D. L. Flynn, M. D. Kaufman and P. A. Petillo, US Pat., 8461179B1, 2013.
- ICH Guideline S2(R1), Guidance on genotoxicity testing and data interpretation for pharmaceuticals intended for human use, 2011. https://database.ich.org/sites/default/files/S2%28R1%29%20Guideline.pdf Search PubMed.
- ICH Guideline M7(R1), Assessment and control of DNA reactive (mutagenic) impurities in pharmaceuticals to limit potential carcinogenic risk, 2017. https://database.ich.org/sites/default/files/M7_R1_Guideline.pdf Search PubMed.
- ICH M7 Q&As, Assessment and control of DNA reactive (mutagenic) impurities in pharmaceuticals to limit potential carcinogenic risk questions & answers, 2020. https://www.ema.europa.eu/en/documents/scientific-guideline/questions-answers-ich-guideline-m7-assessment-control-dna-reactive-mutagenic-impurities_en.pdf Search PubMed.
- C. J. Borths, M. D. Argentine, J. Donaubauer, E. L. Elliott, J. Evans, T. T. Kramer, H. Lee, R. Parsons, J. C. Roberts, G. W. Sluggett, A. Teasdale, M. Urquhart, K. Wang and P. Zhuang, Org. Process Res. Dev., 2021, 25, 831 CrossRef CAS.
- N. K. Lapanja, B. Zupancič, R. T. Časar, S. Jurca and B. Doljak, Org. Process Res. Dev., 2018, 22, 125 CrossRef.
- S. Patil, K. Patel, R. Chadar, S. Ramar, A. Prasad, P. Koppula and S. Koppula, Org. Process Res. Dev., 2021, 25, 1391 CrossRef CAS.
- I. Patel, C. J. Venkatramani, A. Stumpf, L. Wigman and P. Yehl, Org. Process Res. Dev., 2017, 21, 182 CrossRef CAS.
- R. Benigni and C. Bossa, Mutat. Res., 2008, 659, 248 CAS.
- D. J. Snodin, Org. Process Res. Dev., 2010, 14, 960 CrossRef CAS.
- M. Patel, M. Kranz, J. Munoz-Muriedas, J. S. Harvey, A. Giddings, S. Swallow, M. Fellows, R. Naven, A.-L. Werner, D. J. Yeo, F. Bringezu, J. Wichard, A. Sutter, S. Glowienke, L. Whitehead, M. Selby, J. Reuberson, F. Atienzar, H. Gerets, M. O. Kenyon, K. L. Dobo, M. W. Walter, R. A. Jolly, A. Amberg, H.-P. Spirkl, W. Muster and J. Van Gompel, Comput. Toxicol., 2018, 7, 27 CrossRef.
- A. Kalauz and I. Kapui, J. Pharm. Biomed. Anal., 2022, 210, 114544 CrossRef CAS.
- FDA, Drugs@FDA: FDA-approved drugs, 2020. https://www.accessdata.fda.gov/drugsatfda_docs/label/2020/213973s000lbl.pdf Search PubMed.
- A. Myden, S. J. Guesne, A. Cayley and R. V. Williams, Regul. Toxicol. Pharmacol., 2017, 88, 77 CrossRef PubMed.
- N. Greene, K. L. Dobo, M. O. Kenyon, J. Cheung, J. Munzner, Z. Sobol, G. Sluggett, T. Zelesky, A. Sutter and J. Wichard, Regul. Toxicol. Pharmacol., 2015, 72, 335 CrossRef CAS.
- M. Antolčić, M. Runje and N. Galić, Anal. Methods, 2020, 12, 3290 RSC.
- D. Jenkins, C. L. Harmon, X. Jia, A. Kesselring, D. Hatcher, K. Grayson and J. Ayres, J. Pharm. Biomed. Anal., 2020, 187, 113352 CrossRef CAS PubMed.
- Lhasa Limited UK, A comparison of Derek and Sarah Nexus, 2017. https://www.lhasalimited.org/Public/Library/2017/A%20comparison%<?pdb_no 20of?>20of<?pdb END?>%20Derek%20and%20Sarah%20Nexus.pdf Search PubMed.
- OECD, Guidance document on the validation of (quantitative) structure–activity relationships [(Q)SAR] models, 2007. https://www.oecd.org/officialdocuments/publicdisplaydocumentpdf/?doclanguage=en%26cote=env/jm/mono(2007)2 Search PubMed.
- A. Teasdale and D. P. Elder, TrAC, Trends Anal. Chem., 2018, 101, 66 CrossRef CAS.
- V. D'Atri, S. Fekete, A. Clarke, J.-L. Veuthey and D. Guillarme, Anal. Chem., 2019, 91, 210 CrossRef.
- A. V. B. Reddy, J. Jaafar, K. Umar, Z. A. Majid, A. B. Aris, J. Talib and G. Madhavi, J. Sep. Sci., 2015, 38, 764 CrossRef CAS PubMed.
- Y. W. Huang, H. Lu, F. L. Zhang and C. Y. Min, J. Sep. Sci., 2018, 41, 3985 CrossRef CAS PubMed.
|
This journal is © The Royal Society of Chemistry 2022 |