DOI:
10.1039/D2RA04402A
(Paper)
RSC Adv., 2022,
12, 29103-29112
Graphene-based strain sensor with sandwich structure and its application in bowel sounds monitoring
Received
16th July 2022
, Accepted 4th October 2022
First published on 12th October 2022
Abstract
Surgery is one of the primary treatment modalities for gastrointestinal tumors but can lead to postoperative ileus (POI), which can aggravate pain and increase costs. The incidence of POI can be effectively reduced by monitoring bowel sounds to assist doctors in deciding the timing of transoral feeding. In this study, we prepared a flexible strain sensor based on a graphene composite material and tested the feasibility of sensor monitoring of bowel sounds using simultaneous stethoscope and sensor monitoring. We found that the time of hearing the bowel sounds (12.0–12.1 s) corresponded to the time of waveform change monitored by the sensor (12.036 s), and the sound tone magnitude corresponded to the waveform amplitude. This proves that the application of sensors to monitor bowel sounds is feasible, which opens up a new field for the application of graphene sensors and provides a new way for clinicians to judge the condition of the intestine.
1. Introduction
Gastrointestinal tumors have become a prevalent type of digestive system disease, and the global cancer statistics in 2018 also show that the incidence and mortality of gastrointestinal cancer are at the forefront.1–3 Surgical resection is currently one of the main treatment methods, but surgery can lead to postoperative ileus (POI) in patients after surgery.4,5 The presence of POI will lead to impaired nutrient absorption and water-electrolyte disturbances, which may further result in serious complications such as incision prolongation, infection, anastomotic fistula, and aspiration pneumonia, which will eventually increase postoperative pain and discomfort, further prolong hospitalization and increase medical costs.6,7 Therefore, reducing the incidence of POI is beneficial to patients and has a catalytic effect on the development of academic and clinical medicine.
The main manifestations of POI are intolerance of oral feeding, inadequate venting, and delayed defecation.8,9 Its occurrence may be related to many factors, such as anesthetic drugs, analgesic drugs, the inflammatory response of the body, and intestinal damage caused by surgery.10 Therefore, most patients require continuous infusion of nutritional fluids to meet their physiological requirements after surgery. Nutritional fluids are not affordable, and prolonged intravenous infusions may cause reactions such as redness and pain in the patient's arm. Therefore, it is essential to shorten the duration of POI, current treatment strategies are limited, and relevant studies have shown that early resumption of oral feeding can reduce the incidence of POI.11,12
However, there is no unified standard to restart feeding, and it is necessary to find an objective indicator to judge the recovery of intestinal motility. Currently, we use the patient's anal discharge as an indicator of the resumption of peristalsis and the time point for the patient to resume oral feeding, but this indicator has a lag and uncertainty. Evaluation can also be performed using gastroscopy, gastrointestinal pressure testing, and other means, but these are complex and invasive. In addition, we can also determine the recovery of the intestinal tract by monitoring bowel sounds, because bowel sounds are produced by the peristaltic movement of the intestinal tract to push the intestinal contents and cause the intestinal contents to hit each other or the intestinal contents to collide with the intestinal wall, which can reflect the intestinal movement.13,14 Currently, clinical auscultation of bowel sounds is mainly performed with a stethoscope, but the judgment of the results depends on the physician's level of experience, and diagnostic errors can occur.15 Moreover, studies have shown that the correct rate of clinician auscultation of bowel sounds is less than 90%.16,17 In addition, Ching et al. applied a 3M™ Littmann® 4100 electronic stethoscope to record and analyze bowel sounds.18 the piezoelectric acoustic sensing device designed by Du et al. can record and classify bowel sounds,19 and Yin et al. designed a wearable bowel sound monitoring system to identify bowel sounds.20 However, the above methods are all monitoring and analysis from the perspective of acoustics, yet the research shows that sound is heard when the intestine can move, but whether it is produced depends on the presence or absence of gas in the intestine, which means that when there is no gas in the intestine, intestinal movement can exist but no bowel sounds are produced.14 Therefore, there is an urgent need to find a non-invasive monitoring method that can be used for real-time and accurate monitoring and is easy to operate.
To achieve this purpose, we attempted to start from the perspective of monitoring the intestinal movement itself and explored the feasibility of using sensors prepared from nanomaterials for monitoring application by utilizing the combination of medicine and materials science. With this research direction, we have noticed that graphene is now a popular player in the field of materials science and condensed matter physics. Graphene is a two-dimensional atomic crystal with high electrical conductivity, excellent mechanical properties, and flexibility as an advanced nanomaterial,21–25 which shows great potential in developing advanced sensors.26–36 Graphene has high sensitivity and is one of the optimal nanomaterials for pressure and strain sensing applications.37,38 The monitoring of signals such as respiration rate,39–41 pulse,42,43 heart rate,44 blood glucose,45 blood pressure,46 body temperature,47,48 and electrocardiogram,49 electroencephalogram,50 electromyography50,51 has been reported based on graphene and its derivatives or graphene composite sensors, which proves that graphene is well suited for sensing applications. The sensor with a sandwich structure designed by Xu et al. can monitor subtle and large body movements such as pulse and knee flexion, demonstrating the potential for versatile applications.52 Polyurethane is a polymer material that is commonly used as a matrix for composite materials and many studies have demonstrated that PU-nanocomposites can improve mechanical properties, electrical conductivity, and sensitivity.53 The strain sensor based on elastomeric/graphene sheet composite film designed by Meng et al. can monitor body movements such as pulse, finger movements, forearm muscle movements, and provides a simple manufacturing method of the composite film sensor.53 The sensor developed by Lv et al. using polyurethane has high sensitivity and large strain range, and the gloves developed based on this can recognize different gestures.54 In addition, the graphene woven fabrics thin-film sensor made by Wang et al. achieved high precision acquisition and recognition of sound signals,55 which in essence is achieved by monitoring the laryngeal muscle movement, and the film cracks internally when stretched thus leading to an increase in resistance by reducing the conductive path. This study also found that the waveform is almost the same when the subject does the same movement with or without sound, which is similar to the theory of our study. Moreover, the suspended SFG designed by Qiao et al. exhibited good acoustic detection skills,56 and the MXene nanoflakes-based artificial eardrum designed by Gou et al. had excellent acoustic sensing ability.57 Therefore we believe that the strain sensor containing graphene nanoplatelets can monitor intestinal movement and make an accurate and objective.
In this study, we fabricated a flexible strain sensor based on PU/GNP composite material and selected the optimal thickness of the composite film through experiments on conductive performance, mechanical properties, and sensitivity. Also, the flexibility, stability and response time of the sensor were examined before performing the monitoring of bowel sounds. The stethoscope was chosen to be performed simultaneously with the sensors, and the feasibility of monitoring was verified, and it was found that different intensities of bowel sounds could be distinguished. This study has opened up new horizons for graphene applications and provided a new method for the evaluation of intestinal motility, and we believe that the sensor has great potential in intestinal monitoring.
2. Experimental section
2.1 Materials
Graphite Intercalation Compounds (GICs) were purchased from Shandong Qingdao Huatai Company. The encapsulation material used in this study is a polyurethane (PU) with a thickness of 0.04 mm, which is commercially available and widely used in the medical and leather industries. The conductive adhesive used in this experiment was ordered from Shenzhen Huijia Tape Products Factory.
2.2 Fabrication of GNP film
Graphene nanoplatelets (GNPs) are prepared using a published thermal expansion-ultrasonic method.58,59 Briefly, the graphite intercalation compounds are thermally expanded in a muffle furnace preheated and warmed to 700 °C for 1 min and cooled in the air to obtain worm-like expanded graphene, then the graphene is prepared after 4 h of sonication and drying. The graphene is added to a glass mold of size 102 × 102 mm and then pressed for the first time with a smooth-surfaced iron block and then pressed for 2.5 min at a pressure of 1.4 MPa using a hydraulic press to form a more compact stack of GNP films, as shown in Fig. 1.
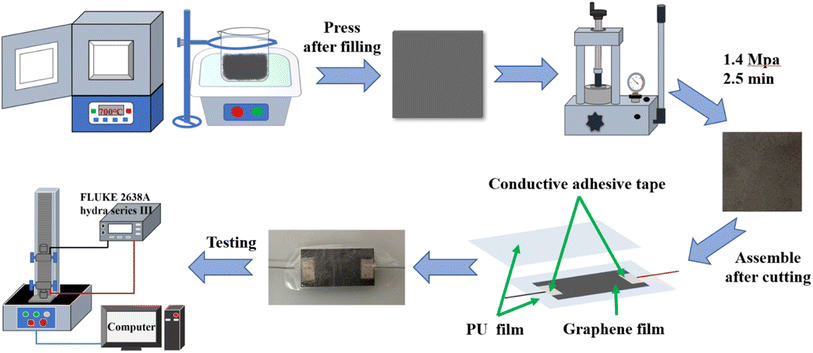 |
| Fig. 1 Schematic diagram of strain sensor preparation and testing. | |
2.3 Fabrication of composite strain sensor
Fig. 1 illustrates the fabrication of a strain sensor based on a PU/GNP composite film. The GNP film and the PU film are first cut to the appropriate size, then the GNP film is placed in the central area of the PU film, the wires are fixed to the two ends of the GNP film with conductive tape, and finally, the other PU film is covered on the other side of the GNP film. The PU–GNP–PU sandwich strain sensor was therefore prepared. The average thickness of the PU film is 0.10 ± 0.002 mm, the thickness of the GNP film is determined by the amount of GNPs added, while the thickness of the composite film is the sum of the two, that is. The thickness of the composite film depends on the weight of the GNPs. In this experiment, five thicknesses of GNP film were prepared 0.12 mm, 0.14 mm, 0.16 mm, 0.18 mm, and 0.20 mm thicknesses were used for the subsequent experiments.
2.4 Characterization
A scanning electron microscope (SEM, JEOL JSM-7800F) at an accelerating voltage of 5 kV was used to present cross-sectional and surface images of the composite films. The thickness of the graphene nanoplatelets was analyzed using an atomic force microscope (AFM, Dimension ICON2-SYS, Brook Corporation, USA) by selecting two positions on each graphene nanoplatelets, measuring at least five sheets, and then determining the average value to obtain the thickness of graphene nanoplatelets.
The mechanical properties were measured using an Instron tensile testing machine of a composite film of size 40 mm × 20 mm at a strain rate of 20 mm min−1 and a scale distance of 30 mm at room temperature, while the FLUKE data acquisition unit was applied jointly and then the stress–strain graph and the resistance change–strain graph were obtained.
The sensitivity of the strain sensor is expressed in terms of the rate of change of resistance versus tensile strain, and the strain acquisition of the composite film with the size of 40 mm × 20 mm was carried out with an Instron stretching machine at a speed of 20 mm min−1 and a gauge length of 30 mm.
The fluke data acquisition unit was used to measure the resistance of the strain sensor simultaneously during the tensile test, and then the data was processed to obtain a graph of the sensitivity performance of this sensor. The cyclic stretching test was performed on the flexible strain sensor under 143% strain for 1000 cycles at a frequency of 0.23 Hz, and the reproducibility of the stability of the sensor was checked by comparing the change in the difference between the waveform shape and the rate of change of resistance before and after 100 cycles.
3. Results and discussion
3.1 Morphological analysis
3.1.1 Morphological of graphene nanoplatelets. The results of AFM is shown in Fig. 2a and we can see the average thickness of the graphene nanoplatelets prepared in this experiment is measured as 3.816 ± 0.059 nm (Fig. 2b). This study shows that each graphene nanoplatelets is composed of 3–5 graphene layers, and the graphene thickness is about ∼1 nm,60 which is closer to the theoretical thickness indicating that our prepared graphene nanoplatelets is of good quality and has a thin and uniform thickness.
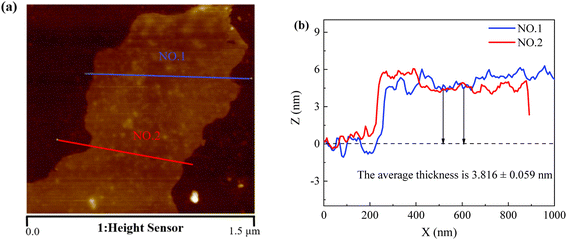 |
| Fig. 2 Morphology of graphene nanoplatelets: (a) high-resolution AFM image and (b) the thickness of GNP sheet. | |
3.1.2 Morphology of PU/GNP composite film. The thickness of the graphene films we prepared was 0.12 mm, 0.14 mm, 0.16 mm, 0.18 mm, and 0.20 mm, accordingly we selected the graphene film with intermediate thicknesses to observe the morphology in this section. We determined that the thickness of the PU film was fixed at 0.1 mm, so the scanning electron microscopy (SEM) imaging of the cross-section was performed for the PU/GNP composite film with a thickness of 0.26 mm, as shown in Fig. 3. Fig. 3a shows the cross-sectional morphology of the composite film, showing the laminar structure consisting of GNP film and PU film. Fig. 3b and c show that GNP was compacted and stacked tightly to form a conductive network. The surface morphology of the GNP film was imaged by SEM using an unencapsulated graphene film with a thickness of 0.16 mm. Under high magnification, images Fig. 3d–f show the surface morphology of the GNP film; Fig. 3d shows the good surface integrity of the GNP film and the tiny cracks and pores formed by the GNPs stacked on top of each other. These micro-cracks and pores will extend when the graphene film is subjected to stresses and other effects, which leads to a change in the conduction path and thus a change in resistance. Fig. 3e shows that the graphene film is made up of many flaky graphene nanoplatelets overlapping each other, thus forming a large number of conductive paths; Fig. 3f enlarges the tight stacking and overlapping between the graphene nanoplatelets, which makes the graphene film have good electrical conductivity.
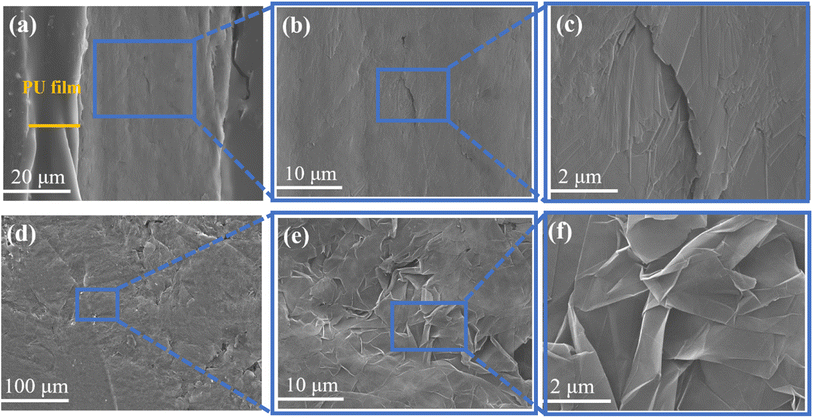 |
| Fig. 3 (a–c) Cross-sectional morphology of the composite film; (d–f) surface morphology of the GNP film. | |
3.2 Electrical conductivity
The conductivity of graphene film is affected by the size of their cross-sectional area, and the size of the mold to which the conductivity measurement instrument adheres to the sample is fixed, so we measured the conductivity of the prepared graphene film of all thicknesses. Fig. 4 shows that the conductivity becomes smaller as the thickness of the graphene film increases, implying that the thinner the graphene film is, the more stable its internal structure is and the better its conductivity. This may be because the increasing thickness of graphene films causes the internal structure to become inhomogeneous, which affects the electrical conductivity.
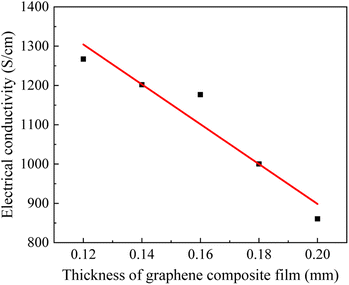 |
| Fig. 4 Electrical conductivity for different thicknesses of GNP films. | |
3.3 Mechanical performance
Fig. 5a shows the change in resistance of the sensor. The sensor is stretched to deform the composite film, which leads to a change in the connection state between GNPs causing microcracks and disconnection between GNPs. At this time, the overlap area between GNPs in the PU will be reduced, and the connection between them can also remain unchanged.
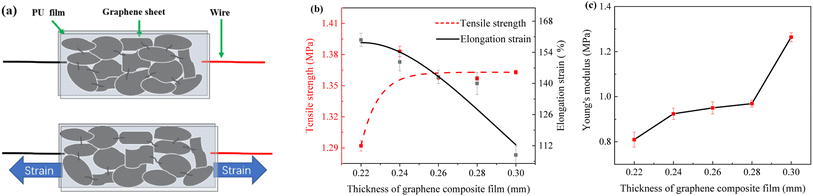 |
| Fig. 5 (a) Schematic of sensor mechanism; (b) tensile strength and elongation strain of GNP composite films with different thicknesses; (c) Young's moduli of GNP composite films with different thicknesses. | |
Fig. 5b displays the curves of tensile strength and elongation strain versus PU/GNP composite film thickness. Fig. 5c shows the curves of Young's moduli of GNP composite films with different thicknesses. As the thickness of the composite film increases, the elongation strain decreases but the tensile strength and Young's moduli increase. 0.22 mm composite film has the highest elongation strain, and 0.30 mm has the lowest elongation strain with a decrease of about 48%. This result may be because the higher graphene content increases the stiffness, resulting in a decrease in strain with increasing thickness. 0.30 mm composite film has the maximum tensile strength with an increase of about 5.4%, probably due to the increase in thickness that enhances the strength of the composite film. Fig. 5b shows that the elevation of tensile strength and the decrease of strain for the graphene composite film with a thickness of 0.26 mm is within the acceptable range.
3.4 Sensing properties of strain sensor
Sensitivity is the most critical performance of strain sensors, and here we use the relationship between resistance change rate and tensile strain to study the sensitivity of strain sensors. Fig. 6a shows the change in resistance versus tensile strain for composite films of different thicknesses. The results show that the resistance change rate increases with increasing strain. When the strain is the same, the rate of change of resistance increases with increasing thickness of the composite film, which means that 0.30 mm composite film has the highest rate of change of resistance and 0.22 mm has the most minor change in resistance. In addition, the measurement range of the composite film with a thickness of 0.30 mm is the smallest, and the measurement range of the rest of the films does not differ significantly. Fig. 6b is the GF calculated by the result in Fig. 6a. In summary, the composite film with 0.26 mm thickness has both a large measurement range and the highest resistance variation.
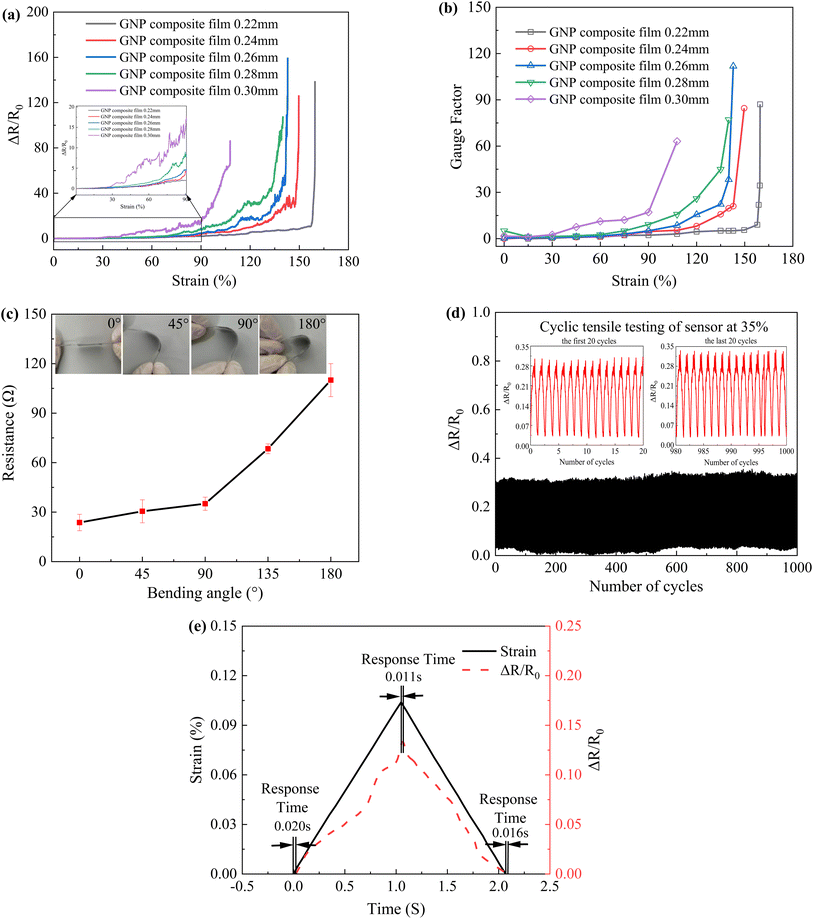 |
| Fig. 6 (a) The curve of resistance changes and strain; (b) the curves of gauge factor and strain; (c) the flexibility of the composite film; (d) cyclic stretching result; (e) response time test under tension and release. | |
Based on the above studies, the 0.26 mm thick composite film not only has excellent electrical conductivity, but also has relatively ideal mechanical properties and high sensitivity. Therefore, we only selected the 0.26 mm thick composite film as a representative for bending test, cyclic tensile test, response time test and bowel sound monitoring.
Fig. 6c shows the change in resistance of the 0.26 mm thickness composite film at different bending angles. It can be seen that the resistance increases with increasing angle, indicating that the composite film has good flexibility and can withstand large strains without loss of performance.
As shown in Fig. 6d, we found that the waveform of the resistance change did not fluctuate much during 1000 cycles, while the waveform shape of the 20 cycles before and after was roughly the same. We randomly selected the three maximum values of the resistance change in the 20 cycles before and after, and obtained that the difference between the maximum values of the resistance change before and after the 20 cycles was 0.02, and the difference change rate was 7%, indicating that the sensor has good stability and reproducibility. The flexibility is mainly due to the relatively good elasticity of the PU film.
Fig. 6e shows the test results of the response time of the strain sensor. By comparing the two load–unload curves of resistance change rate and strain, it can be seen that there are different levels of delays in the sensor's stress application and release phases. The response times at the initial strain, highest strain point, and no strain were 0.020 s, 0.011 s and 0.016 s, respectively, indicating the fast response capability of the sensor.
3.5 Application to bowel sound monitoring
In this experiment, a composite film with optimal mechanical properties and high sensitivity was selected as a strain transducer for monitoring bowel sounds.
Under normal circumstances, the bowel sounds in healthy adults are about 4–5 times per minute and are typically characterized by a considerable variation in frequency sound and tone.19 Fig. 7a is a schematic diagram of bowel sound monitoring using sensors and stethoscopes. Regarding the choice of the monitoring site, Craine et al. suggested that the bowel sounds should be auscultated in the ileocecal and subxiphoid regions.61 However, to avoid interference from heart sounds and abdominal aortic pulsations, we chose to monitor in the ileocecal region. Both conventional and commercial electronic stethoscopes were applied for simultaneous monitoring with the sensor to test the feasibility of sensor monitoring of bowel sounds.
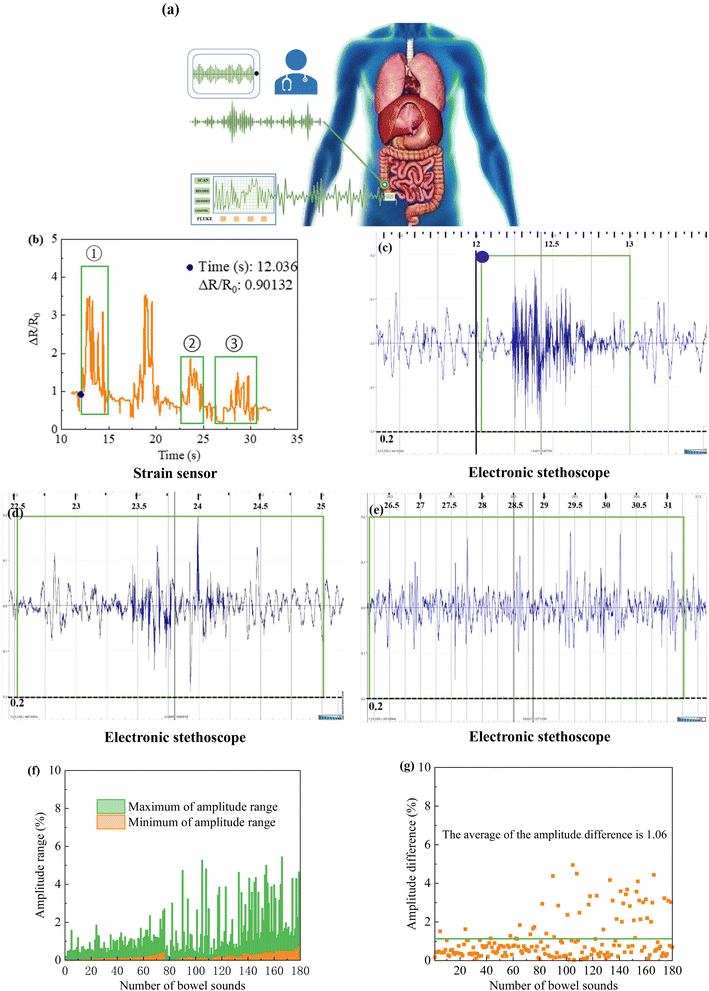 |
| Fig. 7 (a) Schematic diagram of the sensors application and stethoscopes to monitor bowel sounds; (b) waveform diagram of the bowel sounds monitored by the sensor; (c–e) audio graphs of bowel sounds collected by electronic stethoscope; (f) data chart of maximum and minimum amplitude of bowel sounds; (g) difference data chart of bowel sounds amplitude. | |
Fig. 7b shows the waveforms of the sensor-monitored bowel sounds, which were created by finding the waveforms corresponding to the change in sensor resistance based on the time when electronic stethoscope heard the bowel sounds. Fig. 7b is the waveform graph produced by intercepting in chronological order for comparison with Fig. 7c–e. Fig. 7c is a graph of the audio collected the audio data shows that the onset time of hearing bowel sounds is 12.0–12.1 s, while the onset time of waveform change in the first green box in Fig. 7b (marked by the blue dot) is 12.036 s, which is strong evidence to confirm that the flexible strain sensor can monitor bowel sounds. The audio data shows an end time of about 13 s, while the waveform ends at about 15 s, which corresponds to bowel movements when bowel sounds are not present, as mentioned in the previous section. Fig. 7d is an audio map collected by the electronic stethoscope, which corresponds to the second green box in Fig. 7b. Fig. 7e is an audio map collected by the electronic stethoscope, which corresponds to the third green box in Fig. 7b. Comparing the audio intensities in Fig. 7c–e combined with Fig. 7b, it can be found that the amplitude of the bowel sounds corresponds to the pitch of the bowel sounds heard, which shows that the flexible strain sensor can also distinguish bowel sounds of different intensities. For the waveform in Fig. 7b with obvious resistance change but not circled, it means that the bowel sounds is not heard by auscultation at the corresponding time. However, this does not conclude that these waveforms are not bowel sounds because there are limitations in stethoscope auscultation, and combined with the characteristics of the sensor we guess that the sensor is more sensitive than the stethoscope in monitoring bowel sounds.
Fig. 7f–g is a graph of the waveform amplitude data of bowel sounds monitored by a sensor. Fig. 7f shows the maximum and minimum bowel sounds waveform amplitude values. The minimum values range from 0.02 to 0.81, with 98.33% of the minimum values falling within the range of 0.02–0.60. In addition, it can be observed that the maximum value of the bowel sound amplitude varies greatly, Since the bowel sound itself is highly variable, the analysis of the available data suggests that more experiments are needed further to refine the reference range of the maximum value. Fig. 7g is the amplitude difference data plot, ranging from 0.03 to 4.95. The average amplitude differences is 1.06, and most of the difference data can be included when the difference range is set to 0.03–1.06. According to the available data, we preliminarily defined the reference ranges of minimum and difference values of bowel sound amplitude as 0.02–0.60 and 0.03–1.06, respectively.
Thus, flexible strain sensors based on graphene structures can monitor bowel sounds and differentiate between different intensities of bowel sounds, which provides a new way to determine the functional condition of the intestine, but more data need to be collected to continue to revise the reference ranges of the minimum and difference values of bowel sounds amplitude. In addition, we suggest that the clinical application of graphene sensors should be combined with the patient's own perception to make a comprehensive evaluation of intestinal recovery, so that the time point of transoral feeding can be selected more accurately, thus effectively shortening the time of POI and giving full play to the auxiliary role of the sensors.
4. Conclusion
In brief, we have prepared flexible strain sensors based on polyurethane–graphene structures using a simple method that is easy to operate and low cost. Conductivity, mechanical properties, and sensitivity tests were performed after preparing composite films of different thicknesses, and then the best contestant was selected to proceed to the next step. Bending tests, cyclic tensile tests, and response time tests were also performed, which demonstrated fast response capability and the excellent flexibility and stability of the sensor. In the application stage, we used the sensor and stethoscope for simultaneous monitoring, confirming the feasibility of the sensor to monitor bowel sounds and distinguishing bowel sounds of different intensities. In addition, we also preliminarily defined the reference range of the minimum and difference values of the bowel sound amplitude. In conclusion, strain sensors based on graphene composite structure have a broad prospect in clinical intestinal function monitoring and expand new realms for the application of graphene.
Ethical statement
The human experiment was carried out on the author himself, and informed consent has been obtained, which does not involve ethics.
Conflicts of interest
There are no conflicts to declare.
Acknowledgements
This research did not receive any specific grant from funding agencies in the public, commercial, or not-for-profit sectors.
References
- E. C. Smyth, M. Nilsson, H. I. Grabsch, N. C. T. van Grieken and F. Lordick, Lancet, 2020, 396, 635–648 CrossRef CAS.
- E. Dekker, P. J. Tanis, J. L. A. Vleugels, P. M. Kasi and M. B. Wallace, Lancet, 2019, 394, 1467–1480 CrossRef.
- A. P. Thrift and H. B. El-Serag, Clin. Gastroenterol. Hepatol., 2020, 18, 534–542 CrossRef PubMed.
- A. Huge, M. E. Kreis, T. T. Zittel, H. D. Becker, M. J. Starlinger and E. C. Jehle, Dis. Colon Rectum, 2000, 43, 932–939 CrossRef CAS PubMed.
- Y. Jin, R. Geng, Y. Liu, L. Liu, X. Jin, F. Zhao, J. Feng and Y. Wei, Front. Oncol., 2020, 10, 526009 CrossRef PubMed.
- H. Thomas, Nat. Rev. Gastroenterol. Hepatol., 2019, 16, 76 CrossRef PubMed.
- D. Wattchow, P. Heitmann, D. Smolilo, N. J. Spencer, D. Parker, T. Hibberd, S. S. J. Brookes, P. G. Dinning and M. Costa, Neurogastroenterol. Motil., 2021, 33, e14046 CrossRef PubMed.
- N. Stakenborg, E. Labeeuw, P. J. Gomez-Pinilla, S. De Schepper, R. Aerts, G. Goverse, G. Farro, I. Appeltans, E. Meroni, M. Stakenborg, M. F. Viola, E. Gonzalez-Dominguez, G. Bosmans, Y. A. Alpizar, A. Wolthuis, A. D'Hoore, K. Van Beek, S. Verheijden, M. Verhaegen, R. Derua, E. Waelkens, M. Moretti, C. Gotti, P. Augustijns, K. Talavera, P. Vanden Berghe, G. Matteoli and G. E. Boeckxstaens, Gut, 2019, 68, 1406–1416 CrossRef CAS PubMed.
- R. Vather, S. Trivedi and I. Bissett, J. Gastrointest. Surg., 2013, 17, 962–972 CrossRef PubMed.
- E. Mazzotta, E. C. Villalobos-Hernandez, J. Fiorda-Diaz, A. Harzman and F. L. Christofi, Front. Pharmacol., 2020, 11, 583422 CrossRef CAS PubMed.
- T. L. Hedrick, M. D. McEvoy, M. M. G. Mythen, R. Bergamaschi, R. Gupta, S. D. Holubar, A. J. Senagore, T. J. Gan, A. D. Shaw, J. K. M. Thacker, T. E. Miller, P. E. Wischmeyer, F. Carli, D. C. Evans, S. Guilbert, R. Kozar, A. Pryor, R. H. Thiele, S. Everett, M. Grocott, R. E. Abola, E. Bennett-Guerrero, M. L. Kent, L. S. Feldman, J. F. Fiore, Jr. and W. Perioperative Quality Initiative, Anesth. Analg., 2018, 126, 1896–1907 CrossRef PubMed.
- H. Kehlet, Anaesthesia, 2020, 75(Suppl 1), e54–e61 Search PubMed.
- J. K. Nowak, R. Nowak, K. Radzikowski, I. Grulkowski and J. Walkowiak, Sensors, 2021, 21, 5294 CrossRef PubMed.
- C. Wells, L. Tinckler, K. Rawlinson, H. Jones and J. Saunders, Lancet, 1964, 1, 4–10 CrossRef CAS.
- S. Felder, D. Margel, Z. Murrell and P. Fleshner, J. Surg. Educ., 2014, 71, 768–773 CrossRef PubMed.
- Y. Gu, H. J. Lim and M. A. Moser, Dig. Surg., 2010, 27, 422–426 CrossRef PubMed.
- J. Gade, P. Kruse, O. T. Andersen, S. B. Pedersen and S. Boesby, Scand. J. Gastroenterol., 1998, 33, 773–777 CrossRef CAS PubMed.
- S. S. Ching and Y. K. Tan, World J. Gastroenterol., 2012, 18, 4585–4592 CrossRef PubMed.
- X. Du, G. Allwood, K. M. Webberley, A. Osseiran and B. J. Marshall, Sensors, 2018, 18(12), 4240 CrossRef PubMed.
- Y. Yin, H. Jiang, S. Feng, J. Liu, P. Chen, B. Zhu and Z. Wang, Sci. China Inf. Sci., 2018, 61(8), 084301 CrossRef.
- K. S. Novoselov, V. I. Fal'ko, L. Colombo, P. R. Gellert, M. G. Schwab and K. Kim, Nature, 2012, 490, 192–200 CrossRef CAS PubMed.
- Z. Luo, X. Hu, X. Tian, C. Luo, H. Xu, Q. Li, Q. Li, J. Zhang, F. Qiao, X. Wu, V. E. Borisenko and J. Chu, Sensors, 2019, 19(5), 1250 CrossRef CAS PubMed.
- A. K. Geim, Science, 2009, 324, 1530–1534 CrossRef CAS PubMed.
- Q. Meng, J. Jin, J. Ma, R. Wang, H. C. Kuan, N. Kawashima, A. Michelmore, S. Zhu and C. H. Wang, Nanotechnology, 2014, 25, 125707 CrossRef PubMed.
- Q. Meng, H. Wu, Z. Zhao, S. Araby, S. Lu and J. Ma, Composites, Part A, 2017, 92, 42–50 CrossRef CAS.
- Z. Yang, D. Y. Wang, Y. Pang, Y. X. Li, Q. Wang, T. Y. Zhang, J. B. Wang, X. Liu, Y. Y. Yang, J. M. Jian, M. Q. Jian, Y. Y. Zhang, Y. Yang and T. L. Ren, ACS Appl. Mater. Interfaces, 2018, 10, 3948–3954 CrossRef CAS PubMed.
- Z. Yang, Y. Pang, X. L. Han, Y. Yang, J. Ling, M. Jian, Y. Zhang, Y. Yang and T. L. Ren, ACS Nano, 2018, 12, 9134–9141 CrossRef CAS PubMed.
- V. Shirhatti, V. Kedambaimoole, S. Nuthalapati, N. Neella, M. M. Nayak and K. Rajanna, Nanotechnology, 2019, 30, 475502 CrossRef CAS PubMed.
- Y. Li, T. He, L. Shi, R. Wang and J. Sun, ACS Appl. Mater. Interfaces, 2020, 12, 17691–17698 CrossRef CAS PubMed.
- L. Zhang, H. Li, X. Lai, T. Gao and X. Zeng, ACS Appl. Mater. Interfaces, 2020, 12, 44360–44370 CrossRef PubMed.
- Z. Liu, G. Wang, W. Pei, C. Wei, X. Wu, Z. Dou, Y. Li, Y. Wang and H. Chen, J. Mater. Chem. B, 2020, 8, 8794–8802 RSC.
- E. Vermisoglou, D. Panacek, K. Jayaramulu, M. Pykal, I. Frebort, M. Kolar, M. Hajduch, R. Zboril and M. Otyepka, Biosens. Bioelectron., 2020, 166, 112436 CrossRef CAS PubMed.
- I. Jurewicz, A. A. K. King, R. Shanker, M. J. Large, R.
J. Smith, R. Maspero, S. P. Ogilvie, J. Scheerder, J. Han, C. Backes, J. M. Razal, M. Florescu, J. L. Keddie, J. N. Coleman and A. B. Dalton, Adv. Funct. Mater., 2020, 30, 2002473 CrossRef CAS PubMed.
- Q. Meng, Z. Liu, R. Cai, S. Han, S. Lu and T. Liu, Polym. Adv. Technol., 2020, 31, 214–225 CrossRef CAS.
- Q. Meng, Y. Zhao, Z. Liu, S. Han, S. Lu and T. Liu, J. Appl. Polym. Sci., 2019, 136, 47906 CrossRef.
- S. Han, Q. Meng, K. Xing, S. Araby, Y. Yu, A. Mouritz and J. Ma, Compos. Sci. Technol., 2020, 198, 108312 CrossRef CAS.
- H. Huang, S. Su, N. Wu, H. Wan, S. Wan, H. Bi and L. Sun, Front. Chem., 2019, 7, 399 CrossRef CAS PubMed.
- S. Han, Q. Meng, Z. Qiu, A. Osman, R. Cai, Y. Yu, T. Liu and S. Araby, Polymer, 2019, 184, 121884 CrossRef CAS.
- C. S. Boland, U. Khan, C. Backes, A. O'Neill, J. McCauley, S. Duane, R. Shanker, Y. Liu, I. Jurewicz, A. B. Dalton and J. N. Coleman, ACS Nano, 2014, 8, 8819–8830 CrossRef CAS PubMed.
- M. Xu, J. Qi, F. Li and Y. Zhang, Nanoscale, 2018, 10, 5264–5271 RSC.
- Y. Su, C. Li, M. Li, H. Li, S. Xu, L. Qian and B. Yang, Sens. Actuators, B, 2020, 308, 127693 CrossRef CAS.
- T. Yang, X. Jiang, Y. Zhong, X. Zhao, S. Lin, J. Li, X. Li, J. Xu, Z. Li and H. Zhu, ACS Sensors, 2017, 2, 967–974 CrossRef CAS PubMed.
- Y. Pang, K. Zhang, Z. Yang, S. Jiang, Z. Ju, Y. Li, X. Wang, D. Wang, M. Jian, Y. Zhang, R. Liang, H. Tian, Y. Yang and T. L. Ren, ACS Nano, 2018, 12, 2346–2354 CrossRef CAS PubMed.
- N. Karim, S. Afroj, A. Malandraki, S. Butterworth, C. Beach, M. Rigout, K. S. Novoselov, A. J. Casson and S. G. Yeates, J. Mater. Chem. C, 2017, 5, 11640–11648 RSC.
- Z. Pu, J. Tu, R. Han, X. Zhang, J. Wu, C. Fang, H. Wu, X. Zhang, H. Yu and D. Li, Lab Chip, 2018, 18, 3570–3577 RSC.
- Y. Pang, H. Tian, L. Tao, Y. Li, X. Wang, N. Deng, Y. Yang and T. L. Ren, ACS Appl. Mater. Interfaces, 2016, 8, 26458–26462 CrossRef CAS PubMed.
- T. Q. Trung and N. E. Lee, Adv. Mater., 2016, 28, 4338–4372 CrossRef CAS PubMed.
- Z. Wang, W. Gao, Q. Zhang, K. Zheng, J. Xu, W. Xu, E. Shang, J. Jiang, J. Zhang and Y. Liu, ACS Appl. Mater. Interfaces, 2019, 11, 1344–1352 CrossRef CAS PubMed.
- A. Aslan, A. M. Allahverdiyev, M. Bagirova and E. S. Abamor, Curr. Stem Cell Res. Ther., 2018, 13, 447–457 CrossRef CAS PubMed.
- Y. J. Yun, J. Ju, J. H. Lee, S.-H. Moon, S.-J. Park, Y. H. Kim, W. G. Hong, D. H. Ha, H. Jang, G. H. Lee, H.-M. Chung, J. Choi, S. W. Nam, S.-H. Lee and Y. Jun, Adv. Funct. Mater., 2017, 27(33), 1701513 CrossRef.
- B. Sun, R. N. McCay, S. Goswami, Y. Xu, C. Zhang, Y. Ling, J. Lin and Z. Yan, Adv. Mater., 2018, 30, e1804327 CrossRef PubMed.
- J. Xu, H. Chang, B. Zhao, R. Li, T. Cui, J. Jian, Y. Yang, H. Tian, S. Zhang and T.-L. Ren, Chem. Eng. J., 2022, 431(1), 133908 CrossRef CAS.
- Q. Meng, Z. Liu, S. Han, L. Xu, S. Araby, R. Cai, Y. Zhao, S. Lu and T. Liu, J. Mater. Sci., 2019, 54, 10856–10870 CrossRef CAS.
- P. Lv, X. Li, Z. Zhang, B. Nie, Y.-L. Wu, H. Tian, T.-L. Ren and G. Z. Wang, Microelectron. Eng., 2022, 259, 111779 CrossRef CAS.
- Y. Wang, T. Yang, J. Lao, R. Zhang, Y. Zhang, M. Zhu, X. Li, X. Zang, K. Wang, W. Yu, H. Jin, L. Wang and H. Zhu, Nano Res., 2015, 8, 1627–1636 CrossRef CAS.
- Y. Qiao, X. Li, J. Jian, Q. Wu, Y. Wei, H. Shuai, T. Hirtz, Y. Zhi, G. Deng, Y. Wang, G. Gou, J. Xu, T. Cui, H. Tian, Y. Yang and T. L. Ren, ACS Appl. Mater. Interfaces, 2020, 12, 49945–49956 CrossRef CAS PubMed.
- G.-Y. Gou, X.-S. Li, J.-M. Jian, H. Tian, F. Wu, J. Ren, X.-S. Geng, J.-D. Xu, Y.-C. Qiao, Z.-Y. Yan, G. Dun, C. W. Ahn, Y. Yang and T.-L. Ren, Sci. Adv., 2022, 8, eabn2156 CrossRef CAS PubMed.
- Q. Meng, Y. Yu, J. Tian, Z. Yang, S. Guo, R. Cai, S. Han, T. Liu and J. Ma, Nanotechnology, 2020, 31, 465502 CrossRef CAS PubMed.
- G. Shi, Z. Zhao, J. H. Pai, I. Lee, L. Zhang, C. Stevenson, K. Ishara, R. Zhang, H. Zhu and J. Ma, Adv. Funct. Mater., 2016, 26, 7614–7625 CrossRef CAS.
- S. Araby, X. Su, Q. Meng, H. C. Kuan, C. H. Wang, A. Mouritz, A. Maged and J. Ma, Nanotechnology, 2019, 30, 385703 CrossRef CAS PubMed.
- B. L. Craine, M. L. Silpa and C. J. O'Toole, Dig. Dis. Sci., 2002, 47, 1290–1296 CrossRef PubMed.
|
This journal is © The Royal Society of Chemistry 2022 |
Click here to see how this site uses Cookies. View our privacy policy here.