DOI:
10.1039/D2RA03423A
(Paper)
RSC Adv., 2022,
12, 26016-26022
Determination of 12 anti-obesity drugs in human plasma by a 96-well protein precipitation plate using HPLC-MS†
Received
2nd June 2022
, Accepted 6th September 2022
First published on 13th September 2022
Abstract
An analytical method was developed and validated for the simultaneous determination of 12 anti-obesity drugs (methylephedrine (MER), amphetamine (AMP), fenfluramine (FEN), bupropion (BUP), fluoxetine (FLU), sibutramine (SIBU), bisacodyl (BISA), bumetanide (BUM), lovastatin (LOVA), simvastatin (SIM), rimonabant (RIMO), and fenofibrate (FENO)) in human plasma by a 96-well protein precipitation plate combined with high-performance liquid chromatography-mass spectrometry (HPLC-MS/MS). The 96-well protein precipitation plate was chosen for simultaneous pretreatment of large sample volumes, making the whole process more efficient and faster. Drugs were separated on an Agilent Poroshell 120 EC-C18 column, and detected by MS/MS under multiple reaction monitoring (MRM) mode. The developed method was validated in terms of linearity, matrix effect, accuracy and precision. A good linearity was obtained in the range of 0.1–20.0 ng mL−1 for fenfluramine, bupropion, fluoxetine, sibutramine, bisacodyl, and rimonabant; and 0.5–20.0 ng mL−1 for methylephedrine, amphetamine, bumetanide, lovastatin, simvastatin, and fenofibrate with a correlation coefficient above 0.995. The method was fully validated with an acceptable accuracy of 75.63–108.21%, matrix effect of 80.41–117.71% except for fenofibrate (76.07% at low concentration levels), and precision of 0.32–13.12%. Owing to the advantages of simple operation, high accuracy and sensitivity, this method is suitable for the rapid and simultaneous detection of 12 anti-obesity drugs in human plasma, providing support for clinically monitoring the development of adverse reactions and guiding the rational and appropriate use of weight-loss drugs for obese people.
1. Introduction
Obesity is a chronic metabolic disease, which involves the accumulation of abnormal or excessive fat that can impair health. The number of obese people between the ages of 5 and 19 years increased more than tenfold from 11 million to 124 million worldwide from 1975 to 2016.1 Once considered a problem in high-income countries, obesity is now rising in low- and middle-income countries, especially in urban settings.2 This makes obesity a worldwide health concern.3,4 Obese people have a significantly higher risk of developing non-communicable diseases, such as cardiovascular disease5–7 (mainly heart disease and stroke) and certain cancers7 (endometrial, breast, ovarian, prostate, liver, gallbladder, kidney and colon). Moreover, obesity also has many adverse effects on human fertility.8,9 Further, the psychological effects of obesity are even greater in children and adolescents, who are more likely to suffer from depression.10
Regarding the treatment, diet and exercise are the most commonly used strategies, but they are also difficult to persist, making the use of weight-loss drugs more promising. Long-term, effective and safe anti-obesity medications are more successful when used in combination with behavioural approaches.11 A variety of drugs are available for long-term use, which can be classified based on the mechanism of action, as appetite suppressants such as fenfluramine, sibutramine, rimonabant, and so forth; central excitatory drugs such as methylephedrine, amphetamine, bupropion and fluoxetine, which increase energy expenditure; inhibitors of intestinal digestion and absorption, for example, bisacodyl; diuretics, for example, bumetanide; lipid-lowering medicines, such as lovastatin, simvastatin, and fenofibrate. To make the results more representative and comprehensive, these drugs listed above were selected as analytes for testing.
However, many weight-loss medications can be very harmful. Recently, damage to various organs, such as cardiovascular system,12–14 digestive system,15–17 liver,18 and nervous system,19–21 malnutrition, and reproductive system22 caused by the long-term use of weight-loss pills has been reported. Also, many drugs are dose dependent and addictive with serious side effects, when used in excess. Hence, testing the blood levels of clinically approved and banned weight-loss drugs to monitor the development of adverse reactions is essential.
Various methods are used for determining anti-obesity drugs, including spectroscopic methods, such as near infrared spectroscopy (NIRS) and Raman spectroscopy (RS); chromatographic methods, such as thin-layer chromatography (TLC), liquid chromatography (LC), gas chromatography-tandem mass spectrometry (GC-MS/MS), and liquid chromatography-tandem mass spectrometry (LC-MS/MS);23–26 ion mobility spectrometry (IMS), and so forth. The main target is, however, the illegal addition of slimming drugs in food or drugs. The sample measured in this experiment is blood with a complex matrix. LC-MS/MS has good separation efficacy and high specificity and sensitivity, making it suitable for the accurate quantification of target analytes in blood.
The protein precipitation uses organic solvents miscible with water, such as methanol, acetonitrile, and so forth, to change the hydrogen bonds among protein molecules and thus denature and agglutinate them for the removal of interference from large molecules such as proteins. Based on this theory, the 96-well protein precipitation plate in the experiment can effectively block common protein precipitating agents such as acetonitrile, thus avoiding penetration. If the number of samples is large, the 96-well structure of the plate can effectively save time compared with the traditional protein precipitation method, which makes the whole pre-treatment process more efficient and automated.27,28
By optimizing the chromatographic separation and mass spectrometric detection conditions, an analytical method was developed for the simultaneous determination of 12 diet pills [methylephedrine (MER), amphetamine (AMP), fenfluramine (FEN), bupropion (BUP), fluoxetine (FLU), sibutramine (SIBU), bisacodyl (BISA), bumetanide (BUM), lovastatin (LOVA), simvastatin (SIM), rimonabant (RIMO), and fenofibrate (FENO)] in human plasma. The detection of the 12 anti-obesity drugs could be completed within 20 min. The 96-well protein precipitation was suitable for simultaneous pre-treatment of a large number of complex samples and could effectively extract targeted drugs. This method was sensitive and specific, with a low detection limit and high accuracy, and could determine the target analytes in blood samples.
2. Experimental
2.1. Reagents and instruments
Standards: methylephedrine hydrochloride, bupropion hydrochloride, amphetamine sulfate, fenfluramine, fluoxetine hydrochloride, sibutramine hydrochloride, bixarcomidine, bumetanide, lovastatin, simvastatin and fenofibrate were purchased from the National Institutes for Food and Drug Control (Beijing, China), and rimonabant was purchased from QCC (USA), and the purity was 100%. Chromatographically pure acetonitrile, methanol, formic acid, and acetic acid were purchased from Dima Technology (Beijing, China). Instruments: Agilent 1260 series liquid chromatograph (Agilent), Agilent 6460 triple quadrupole mass spectrometer (Agilent), Synergy UV water purifier (Merck, Germany), TM-1F vortex instrument (Wiggens, Germany), high-speed centrifuge (Hunan Xiang Yi Laboratory Instrument Development Co., Ltd.), SPE-M 96 solid phase extractor device (Agela Technologies), NV-G-S nitrogen blowing device (Agela Technologies), 96-well protein precipitation plate (Nunc, Thermofisher, Pittsburgh, PA).
2.2. HPLC-MS/MS conditions
A HPLC-MS/MS instrument was used to analyse the 12 target compounds. The chromatographic column was an Agilent Poroshell 120 EC-C18 column (3.0 mm × 50 mm, 2.7 μm); the mobile phase A was 0.1% formic acid water (v/v), the mobile phase B was acetonitrile with 0.1% formic acid. The gradient elution procedure was as follows: 0 to 2.0 min, 15% B; 2.0 to 4.0 min, 15% to 90% B; 4.0 to 13.0 min, 90% B; 13.0 to 14.0 min, 90% to 15% B; 14.0 to 20.0 min, 15% B; flow rate was 0.3 mL min−1, the column temperature was 30 °C, and the injection volume was 2 μL.
Electrospray ionization source (ESI); positive ion mode (+); multiple reaction monitoring (MRM); drying gas flow rate: 11 L min−1; drying gas temperature: 300 °C; capillary voltage: 4000 V. The monitored ions, the retention time, collision energy, and fragmentor voltage of analytes are shown in Table 1.
Table 1 The MS/MS monitored ions, fragmentor voltage, collision energy and retention time of the 12 anti-obesity medicines
Compounds |
Precursor ion (m/z) |
Product ion (m/z) |
Fragmentor voltage (V) |
Collision energy (eV) |
Retention time (min) |
Quantitative ion. |
MER |
180.1 |
162.1a |
50 |
13 |
1.571 |
147.0 |
50 |
25 |
AMP |
136.1 |
91.0a |
14 |
21 |
1.766 |
119.0 |
14 |
5 |
FEN |
232.1 |
158.9a |
80 |
33 |
6.624 |
187.0 |
80 |
13 |
BUP |
240.1 |
184.0a |
80 |
9 |
6.473 |
131.0 |
80 |
33 |
FLU |
310.1 |
44.2a |
80 |
13 |
7.120 |
148.0 |
80 |
5 |
SIBU |
280.2 |
124.9a |
80 |
33 |
7.248 |
138.9 |
80 |
13 |
BISA |
362.1 |
183.9a |
80 |
37 |
7.427 |
226.0 |
80 |
17 |
BUM |
365.1 |
240.0a |
80 |
21 |
7.743 |
184.1 |
80 |
25 |
LOVA |
405.3 |
285.1a |
75 |
9 |
9.736 |
199.1 |
75 |
9 |
SIM |
419.3 |
285.0a |
75 |
9 |
10.318 |
199.1 |
75 |
21 |
RIMO |
463.1 |
362.8a |
80 |
37 |
10.433 |
84.1 |
80 |
33 |
FENO |
361.1 |
233.0a |
80 |
17 |
10.886 |
138.9 |
80 |
33 |
2.3. Reference stock solution and quality control sample preparation
The refined weighing MER, AMP, FEN, BUP, FLU, SIBU, BISA, BUM, LOVA, SIM, RIMO and FENO were dissolved in methanol, respectively. Then, a stock solution with a mass concentration of 500 μg mL−1 for each drug was prepared and stored in a −20 °C refrigerator. When in use, the standard curve working solutions of 12 diet drugs were diluted proportionally with methanol. The concentrations of standard curve solutions of BUP, FEN, SIBU, RIMO, FLU, and BISA ranged from 0.1 ng mL−1 to 20.0 ng mL−1 (0.1, 0.5, 1.0, 5.0, 10.0, and 20.0 ng mL−1); MER, AMP, BUM, LOVA, SIM, and FENO ranged from 0.5 ng mL−1 to 20.0 ng mL−1 (0.5, 1.0, 5.0, 10.0, and 20.0 ng mL−1).
Take 100 μL blank plasma, add a certain concentration (the volume was 10 μL) of mixed standard solution to prepare low-, medium- and high-concentration levels of quality control (QC) samples. The low-, medium- and high-concentration levels of MER, AMP, FEN, BUP, FLU, SIBU, BISA, BUM, LOVA, SIM, RIMO and FENO are 0.8,10.0, and 16.0 ng mL−1, respectively.
2.4. Samples
Blood samples were collected from the Physical Examination Centre of the 980th Hospital of the Chinese People's Liberation Army Joint Logistic Support Force (Hebei) in this study. The involving human participants were approved by the Ethics Committee of Hebei Medical University (Approval Number 2020067).
2.5. Sample pre-treatment
The peripheral venous blood collected from volunteers was centrifuged to collect the plasma, which was stored at −20 °C. The plasma samples were naturally thawed, shaken separately to homogenize before use, and processed through a 96-well protein precipitation plate for testing. Then, 300 μL of acetonitrile was added to the 96-well precipitation plate, followed by 100 μL of plasma. The samples were shaken, mixed, and rested for 5 min. The precipitation plate with the collection plate attached was transferred to a positive-pressure device. The solution was collected using positive pressure, blown to dryness at 45 °C under nitrogen, and reconstructed with 200 μL of methanol solution for analysis. If reconstitution volume is any smaller, the target substance will not be completely dissolved.
3. Results and discussion
3.1. Optimization of chromatographic conditions
The 12 anti-obesity drugs have different chemical properties and differ significantly in polarity, which make it more difficult to achieve good separation. After a variety of acetonitrile, 0.1% formic acid water−acetonitrile, 0.1% acetic acid water−acetonitrile, 1.0% formic acid water−acetonitrile, and 0.1% formic acid water-0.1% formic acid acetonitrile, it was found that the peak shape was better when the mobile phase was acetonitrile-water (each containing 0.1% formic acid). After repeated trials, the gradient elution procedure for the experiment was established. The result indicated that the separation of each analyte was good and the baseline was smooth.
3.2. Selection of mass spectrometry conditions
A hydrogen ion could be easily added to all 12 analytes to form a positively charged [M + H]+ ion peak. Therefore, the positive-ion mode was used for detection. The parameters such as declustering potential and collision energy for each analyte were optimized to achieve higher sensitivity, and the fragment ions with the highest response values were selected for quantification.
3.3. Optimization of pre-treatment condition
3.3.1 Selection of sample pre-treatment methods. The present method was compared with the conventional protein precipitation. 100 μL of 10.0 ng mL−1 plasma samples were added into 300 μL of acetonitrile for protein precipitation. The present method was done according to Section 2.5. The traditional protein precipitation involves mixing the precipitant with the sample, centrifuging the mixture, and feeding it into the instrument for testing.29 Then the solution was blown to dryness at 45 °C under nitrogen, and reconstructed with 200 μL of methanol solution for analysis. The efficiency of the pre-treatment was evaluated by comparing the recovery of the target analytes at the same concentration, 10.0 ng mL−1. The results are shown in Fig. 1. The recoveries of the present method were significantly better than those of the conventional protein precipitation method. Almost all target recoveries were increased by 50% and reached 80%. In particular, those of FEN, SIBU, BISA, increased from 20%, 30%, and 20% to 103%, 89%, and 85%, respectively, after using this method. This method, in addition, eliminates the need for centrifugation and filtrate transfer. The filtrate can be collected by applying positive pressure after mixing the precipitant with the sample. The sedimentation plate is a 96-well structure, which can handle 96 samples at the same time. It also shortens the time and improves the laboratory processing sample throughput, effectively speeding up the work process.
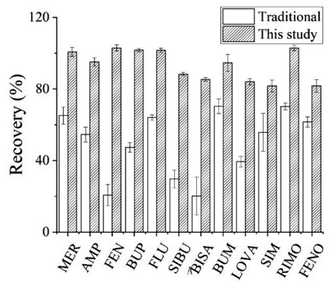 |
| Fig. 1 Effect of two pre-treatment methods on recovery of analytes. | |
3.3.2 Selection of precipitation reagents. 100 μL of 10.0 ng mL−1 plasma samples were added into 300 μL of different precipitation reagents, methanol and acetonitrile for protein precipitation. Then the experiment was done as shown in Section 2.5. 3 replicates were done for every test. The precipitation performance was assessed by comparing the recovery. The result suggested that acetonitrile was used for protein precipitation with better results and higher recovery, as shown in Fig. 2. Therefore, acetonitrile was chosen as the protein precipitant. Solvent volumes for precipitated proteins were in the range of 200–400 μL and a volume ratio of protein precipitant to blood sample was between 2
:
1 and 4.3
:
1.28,30,31 Furthermore, the solvent volumes in this range had little effect on recovery. Hence 300 μL acetonitrile was chosen as the precipitation solvent.
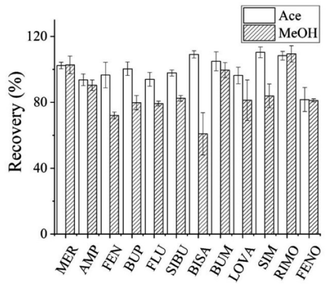 |
| Fig. 2 Effect of different precipitants on the recovery of analytes. | |
3.4. Methodological validation and evaluation
3.4.1 Precision and accuracy. Blank plasma samples were taken to prepare QC samples of low, medium, and high concentrations and processed according to the developed sample pre-treatment method. Three parallel samples were set up for each quality concentration, and each sample was measured three times in parallel, that is to say, each quality concentration was measured nine times; 3 days were measured continuously. The precision of the developed method was evaluated in terms of intra- and inter-day repeatability (n = 3) and is represented as percent relative standard deviation (%RSD). The %RSD values were found to be ≤13.04% for intra-day and ≤13.12% for inter-day. The accuracy of the developed method was evaluated by means of recovery assays on spiked blanks. It was obtained by comparing the added mass concentration with the measured mass concentration of the sample calculated with the corresponding standard curve. The results are shown in Table 2. The accuracy ranged from 75.63% to 108.21%. The results indicated that the method was accurate, reliable, and suitable for the monitoring of 12 diet drugs in plasma.
Table 2 Intra-day and inter-day precision and accuracy of analytes (n = 3)
Compounds |
Spiked (ng mL−1) |
ME (%) |
Precision (RSD%) |
Accuracy (%) |
Inter-day |
Intra-day |
MER |
0.8 |
117.71 |
4.07 |
3.99 |
88.17 |
10.0 |
95.09 |
2.52 |
3.56 |
108.21 |
16.0 |
108.41 |
1.93 |
0.32 |
100.71 |
AMP |
0.8 |
98.19 |
13.12 |
13.04 |
96.23 |
10.0 |
94.37 |
2.37 |
2.98 |
107.41 |
16.0 |
103.00 |
3.61 |
0.59 |
95.11 |
FEN |
0.8 |
108.07 |
2.22 |
2.89 |
102.06 |
10.0 |
99.16 |
1.64 |
0.58 |
102.20 |
16.0 |
111.60 |
1.86 |
2.32 |
102.91 |
BUP |
0.8 |
114.11 |
1.87 |
3.32 |
99.48 |
10.0 |
96.91 |
0.92 |
2.75 |
103.65 |
16.0 |
111.72 |
1.97 |
2.13 |
101.71 |
FLU |
0.8 |
107.50 |
1.53 |
2.64 |
94.00 |
10.0 |
95.76 |
1.15 |
2.64 |
105.49 |
16.0 |
110.99 |
0.97 |
0.17 |
101.65 |
SIBU |
0.8 |
108.78 |
5.38 |
7.22 |
87.29 |
10.0 |
95.43 |
0.84 |
4.50 |
91.37 |
16.0 |
110.12 |
1.85 |
2.86 |
88.32 |
BISA |
0.8 |
98.09 |
2.97 |
1.87 |
86.77 |
10.0 |
96.65 |
1.08 |
1.43 |
85.39 |
16.0 |
110.14 |
1.60 |
0.62 |
102.82 |
BUM |
0.8 |
80.62 |
5.34 |
7.35 |
86.17 |
10.0 |
93.84 |
4.72 |
1.29 |
99.02 |
16.0 |
107.51 |
4.86 |
2.15 |
94.54 |
LOVA |
0.8 |
84.95 |
5.77 |
6.01 |
95.16 |
10.0 |
88.62 |
1.67 |
4.47 |
84.39 |
16.0 |
84.08 |
4.61 |
4.12 |
84.03 |
SIM |
0.8 |
91.76 |
11.92 |
8.33 |
75.63 |
10.0 |
81.79 |
3.36 |
10.15 |
85.72 |
16.0 |
93.07 |
4.42 |
3.63 |
81.65 |
RIMO |
0.8 |
105.96 |
1.42 |
7.83 |
99.92 |
10.0 |
98.58 |
1.45 |
2.14 |
101.99 |
16.0 |
112.72 |
2.01 |
2.51 |
102.87 |
FENO |
0.8 |
76.07 |
5.25 |
6.94 |
84.76 |
10.0 |
80.41 |
3.50 |
7.83 |
83.21 |
16.0 |
84.37 |
8.59 |
4.33 |
76.98 |
3.4.2 Selectivity. Two blank plasma samples were taken, one of which was spiked with the standard working solution mixture (the concentrations of the 12 anti-obesity drugs were all 10 ng mL−1). The two samples were processed according to the sample pretreatment and then analyzed using HPLC-MS/MS. The chromatogram of the spiked sample is shown in Fig. 3.
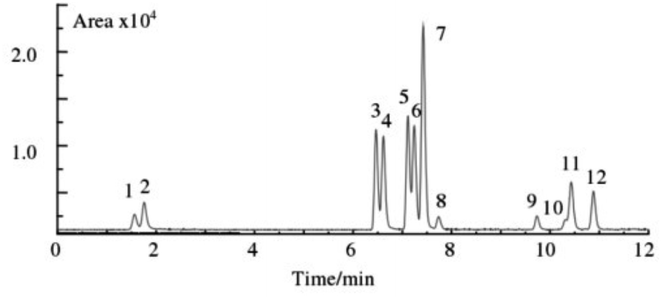 |
| Fig. 3 Typical chromatograms of blank plasma spiked reference compounds (1. MER, 2. AMP, 3. FEN, 4. BUP, 5. FLU, 6. SIBU, 7. BISA, 8. BUM, 9. LOVA, 10. SIM, 11. RIMO, 12. FENO). | |
A substance can be identified as a target substance only if the precursor ion, product ion, and retention time all agree with the target substance. Based on this fact, although the peaks of some target substances were not completely separated, it did not affect the characterization of the interesting substances. For example, for target substances 10 and 11, although the chromatograms were not completely separated, their precursor ions and daughter ions were so different that they were able to be correctly characterized and quantified. In order to cover as many commercially available anti-obesity drugs as possible and to make the study more comprehensive, all 12 weight loss drugs were analysed.
3.4.3 Calibration curve and linearity. Six blank plasma samples were added to the standard working solution mixture and processed according to the method of sample pre-treatment to obtain the standard plasma mixture at the following final concentrations: BUP, FEN, SIBU, RIMO, FLU, and BISA at 0.1, 0.5, 1.0, 5.0, 10.0, and 20.0 ng mL−1, respectively; and MER, AMP, BUM, LOVA, SIM, and FENO at 0.5, 1.0, 2.0, 5.0, 10.0, and 20.0 ng mL−1, respectively. Linear regression analysis was performed with the horizontal coordinate represented by the mass concentration of each analyte in plasma (X) and the vertical coordinate represented by the peak area of the substance (Y). Then, a standard curve was made, and the slope and the correlation coefficient were obtained. The method detection limits (MDL) were calculated at three times the signal-to-noise ratio (S/N), and the method quantification limits (MQL) were calculated at ten times S/N. The results are shown in Table 3. The standard working solution with minimum linear concentrations of each drug was analysed once directly by HPLC/MS. Check the signal-to-noise ratio of the instrument at this concentration. Then calculate the instrumental detection limits (IDL) and instrumental quantification limits (IQL) based on the proportional relationship between the solution concentration and the signal-to-noise ratio. Similarly, IDL were at three times S/N, and IQL were at ten times S/N, as is shown in Table S1.† The targets showed a good linear correlation in a certain concentration range with the correlation coefficients greater than 0.99. The MDL ranged from 0.01 to 0.24 ng mL−1, and the MQL ranged from 0.10 to 0.50 ng mL−1. By comparison with the published literature, such as Júlia Martinelli Magalhães Kahl et al.32 quantified amphetamine in oral liquids using LC/MS in 2021, and LOQ was 20.0 ng mL−1. Martin Kertys et al.33 determined fluoxetine in human plasma by LC-MS/MS, and LOQ was 1.0 ng mL−1. Yijun Li et al.34 detected bumetanide in serum and brain tissue using LC/MS, and LOQ was 1.0 ng mL−1. Hence the LOQ obtained in this experiment are better than other methods and good enough to accomplish the requirements for the analysis of those metabolite in plasma.
Table 3 Regression equations and limit of quantification of analytes
Compounds |
Linear equation |
Linear range (ng mL−1) |
R2 |
LOD (ng mL−1) |
LOQ (ng mL−1) |
MER |
Y= (570 ± 20) x+(400 ± 200) |
0.50–20.0 |
0.9954 |
0.16 |
0.50 |
AMP |
Y= (690 ± 20) x+(300 ± 200) |
0.50–20.0 |
0.9976 |
0.13 |
0.50 |
FEN |
Y= (2870 ± 80) x+(1200 ± 800) |
0.10–20.0 |
0.9966 |
0.03 |
0.10 |
BUP |
Y= (2610 ± 80) x+(600 ± 700) |
0.10–20.0 |
0.9963 |
0.03 |
0.10 |
FLU |
Y= (3840 ± 120) x+(1100 ± 1100) |
0.10–20.0 |
0.9964 |
0.02 |
0.10 |
SIBU |
Y= (2230 ± 60) x+(500 ± 600) |
0.10–20.0 |
0.9971 |
0.04 |
0.10 |
BISA |
Y= (6330 ± 200) x+(2000 ± 2000) |
0.10–20.0 |
0.9959 |
0.01 |
0.10 |
BUM |
Y= (220 ± 10) x+(100 ± 100) |
0.50–20.0 |
0.9984 |
0.22 |
0.50 |
LOVA |
Y= (250 ± 10) x+(100 ± 100) |
0.50–20.0 |
0.9975 |
0.20 |
0.50 |
SIM |
Y = 200x+100 |
0.50–20.0 |
0.9994 |
0.24 |
0.50 |
RIMO |
Y= (1690 ± 60) x+(500 ± 600) |
0.10–20.0 |
0.9952 |
0.05 |
0.10 |
FENO |
Y= (570 ± 20) x+(200 ± 200) |
0.50–20.0 |
0.9969 |
0.16 |
0.50 |
3.4.4 Matrix effect. An appropriate amount of blank human plasma was processed according to sample pre-treatment to obtain a blank plasma matrix solution. Then, the mixed reference working solution of the target analytes was added to it to prepare samples at three mass concentration levels: low, medium, and high for detection. The measured peak area (A) of the tested substance was compared with that (B) obtained by directly injecting the standard solution of the corresponding mass concentration into the LC system, and the matrix effect (ME) (A/B × 100%) of this method was calculated. ME greater than 120% are considered enhanced and less than 80% are inhibited. The finding that ME is from 80 to 120% indicates that the matrix enhancement/inhibitory effect is acceptable. The MEs of each analyte in this experiment at low, medium, and high concentrations were negligible except from FENO, which had a slight matrix inhibition effect (76.07%) at low concentration levels. The remaining 11 analytes were in the range of 80–120%, which was 80.41–117.71%. The results are shown in Table 2.
3.4.5 Method comparison. Analytical performances of the developed LC-MS/MS method for the determining weight loss drugs were mainly compared with the reported similar methods. As shown in Table 4, LLE were the most used pre-treatment methods.35–37 However, compared with LLE, 96-well protein precipitation plate was more convenient, fast and high throughput. More importantly, only the new method provided a comprehensive and detailed quantification of weight loss drugs commercially available.33,35–38 In comparison, the modified method was developed for the determination of 12 anti-obesity drugs in plasma with suitable recoveries and the low LOQs, which showed high throughout and high sensitivity.
Table 4 Comparison of the developed method to the other approaches used in the extraction of anti-obesity drugs in plasma
Instrument |
Pre-processing method |
The number of analytes |
LOQ (ng mL−1) |
Recovery (%) |
Ref. |
LC-MS/MS |
96-Well protein precipitation plate |
12 |
0.1–0.5 |
75.63–108.21 |
This work |
GC- MS |
SPME |
3 |
5.0 |
85.58–108.33 |
38 |
LC–ESI-MS/MS |
LLE |
3 |
0.05 |
88.0–113.1 |
35 |
LC-MS/MS |
LLE |
3 |
0.5–2 |
89–114 |
36 |
LC-HRMS/MS |
LLE |
7 |
1 |
80–120 |
37 |
LC-MS/MS |
Ostro 96-well plate |
5 |
0.2–1.0 |
80.2–91.1 |
33 |
Shortly, the method was simple to operate with low reagent consumption, short analysis time, and simultaneous pre-treatment for large batch samples, greatly saving labour consumption and speeding up the experimental process.
4. Conclusions
A specific and sensitive method was successfully developed for the simultaneous determination of 12 weight-loss drugs in plasma with negligible matrix interference. The samples were processed through a 96-well protein precipitation plate, separated on a Agilent Poroshell 120 EC-C18 column, and detected by HPLC-MS/MS with ESI in positive ion mode under MRM mode. This method was validated regarding its analytical range, precision, recovery, selectivity and ME. Based on the experimental results, the method is simple, rapid, sensitive and could process nearly 100 samples simultaneously, which can be applied in accurate and high throughout detection of anti-obesity drugs, providing support for clinically monitoring the development of adverse reactions and guiding the rational and appropriate use of weight-loss drugs for obese people.
Author contributions
Tengteng Ping: methodology; investigation; validation; data curation; formal analysis; visualization; writing – original draft; Min Zheng: formal analysis, methodology, writing – original draft. Pingping Zhang: methodology, validation, writing – original draft. Tianhao Yan: supervision, validation. Xufeng Miao: methodology, visualization. Ke Wang: data curation, formal analysis, writing – original draft, funding acquisition. Kaoqi Lian: conceptualization, project administration, resources, writing – review & editing. All authors have read and agreed to the published version of the manuscript.
Conflicts of interest
The authors declare no conflict of interest.
Acknowledgements
This work was supported by the Central Government guides the development of local science and technology project of Hebei Province (226Z7708G), and the key research and development program of Hebei Province, China (223777116D).
References
- L. Abarca-Gómez, Z. A. Abdeen, Z. A. Hamid, N. M. Abu-Rmeileh, B. Acosta-Cazares, C. Acuin, R. J. Adams, W. Aekplakorn, K. Afsana, C. A. Aguilar-Salinas, C. Agyemang, A. Ahmadvand, W. Ahrens, K. Ajlouni, N. Akhtaeva and H. M. Al-Hazzaa, Lancet, 2017, 390, 2627–2642 CrossRef.
- L. Li, N. Sun, L. Zhang, G. Xu, J. Liu, J. Hu, Z. Zhang, J. Lou, H. Deng, Z. Shen and L. Han, Global Health Action, 2020, 13, 1795438 CrossRef.
- A. M. Prentice, Int. J. Epidemiol., 2006, 35, 93–99 CrossRef PubMed.
- J. Shen, A. Goyal and L. Sperling, Cardiol. Res. Pract., 2012, 178675 Search PubMed.
- D. Garcia-Labbe, E. Ruka, O. F. Bertrand, P. Voisine, O. Costerousse and P. Poirier, Can. J. Cardiol., 2015, 31, 184–194 CrossRef PubMed.
- M. D. Jensen, D. H. Ryan, C. M. Apovian, J. D. Ard, A. G. Comuzzie, K. A. Donato, F. B. Hu, V. S. Hubbard, J. M. Jakicic, R. F. Kushner, C. M. Loria, B. E. Millen, C. A. Nonas, F. X. Pi-Sunyer, J. Stevens, V. J. Stevens, T. A. Wadden, B. M. Wolfe and S. Z. Yanovski, Circulation, 2014, 129 Search PubMed.
- E. A. Silveira, N. Kliemann, M. Noll, N. Sarrafzadegan and C. de Oliveira, Obes. Rev., 2021, 22, e13088 Search PubMed.
- A. Salas-Huetos, L. Maghsoumi-Norouzabad, E. R. James, D. T. Carrell, K. I. Aston, T. G. Jenkins, N. Becerra-Tomas, A. Z. Javid, R. Abed, P. J. Torres, E. M. Luque, N. D. Ramirez, A. C. Martini and J. Salas-Salvado, Obes. Rev., 2021, 22, e13082 CAS.
- J. Simoes-Pereira, J. Nunes, A. Aguiar, S. Sousa, C. Rodrigues, J. Sampaio Matias and C. Calhaz-Jorge, Endocrine, 2018, 61, 144–148 CrossRef CAS PubMed.
- Y. H. Quek, W. W. S. Tam, M. W. B. Zhang and R. C. M. Ho, Obes. Rev., 2017, 18, 742–754 CrossRef.
- Y. J. Tak and S. Y. Lee, Curr. Obes. Rep., 2021, 10, 14–30 CrossRef PubMed.
- A. M. Chao, T. A. Wadden, R. I. Berkowitz, K. Quigley and F. Silvestry, Expert Opin. Drug Saf., 2020, 19, 1095–1104 CrossRef CAS PubMed.
- R. Khera, A. Pandey, A. K. Chandar, M. H. Murad, L. J. Prokop, I. J. Neeland, J. D. Berry, M. Camilleri and S. Singh, Gastroenterology, 2018, 154, 1309–1319 CrossRef PubMed.
- M. Rodriguez-Guerra, M. Yadav, M. Bhandari, A. Sinha, J. N. Bella and E. Sklyar, Case Rep. Cardiol., 2021, 2021, 8896932 Search PubMed.
- K. A. Packard, R. L. Wurdeman and P. R. Antonio, Ann. Pharmacother., 2002, 36, 1168–1170 CrossRef.
- M. H Amy, A. C Karim, R. M Jennifer, E. C Suzanne and A. Y. Jack, Ann. Pharmacother., 2002, 36, 1003–1005 CrossRef PubMed.
- A. M. Chao, T. A. Wadden and R. I. Berkowitz, Expert Opin. Drug Saf., 2018, 17, 379–385 CrossRef CAS PubMed.
- A. J. Krentz, K. Fujioka and M. Hompesch, Diabetes, Obes. Metab., 2016, 18, 558–570 CrossRef CAS PubMed.
- P. Lauren and A. S. Mike, Neurology, 2013, 80, 773–774 CrossRef PubMed.
- R. A. João and M. Fátima, Curr. Neuropharmacol., 2012, 10, 49–52 CrossRef PubMed.
- H. H. Hansen, J. Perens, U. Roostalu, J. L. Skytte, C. G. Salinas, P. Barkholt, D. D. Thorbek, K. T. G. Rigbolt, N. Vrang, J. Jelsing and J. Hecksher-Sorensen, Mol. Metab., 2021, 47, 101171 CrossRef CAS.
- C. Q. Moreira, M. J. S. S. Faria, J. E. Baroneza, R. J. Oliveira and E. G. Moreira, Hum. Exp. Toxicol., 2005, 24, 397–402 CrossRef CAS PubMed.
- R. L. Cunha, W. A. Lopes and P. A. P. Pereira, Microchem. J., 2016, 125, 230–235 CrossRef CAS.
- M. Sarikaya, H. I. Ulusoy, U. Morgul, S. Ulusoy, A. Tartaglia, E. Yilmaz, M. Soylak, M. Locatelli and A. Kabir, J. Chromatogr. A, 2021, 1648, 462215 CrossRef CAS PubMed.
- C. Viana, G. M. Zemolin, L. S. Muller, T. R. Dal Molin, H. Seiffert and L. M. de Carvalho, Food Addit. Contam., Part A: Chem., Anal., Control, Exposure Risk Assess., 2016, 33, 1–9 CAS.
- J. Yun, K. J. Shin, J. Choi and C. H. Jo, Forensic Sci. Int., 2018, 286, 199–207 CrossRef CAS PubMed.
- T. L. Pedersen, I. J. Gray and J. W. Newman, Anal. Chim. Acta, 2021, 1143, 189–200 CrossRef CAS PubMed.
- Z. Qian, J. Le, X. Chen, S. Li, H. Song and Z. Hong, J. Sep. Sci., 2018, 41, 618–629 CrossRef CAS PubMed.
- L. M. Tazi and S. Jayawickreme, J. Chromatogr. B: Anal. Technol. Biomed. Life Sci., 2016, 1011, 89–93 CrossRef CAS PubMed.
- B. F. Da Silva, A. Ahmadireskety, J. J. Aristizabal-Henao and J. A. Bowden, MethodsX, 2020, 7, 101111 CrossRef PubMed.
- T. Margaryan, M. Sargsyan, A. Gevorgyan, H. Zakaryan, A. Aleksanyan, A. Harutyunyan, Y. Armoudjian and A. Mikayelyan, Biomed. Chromatogr., 2020, 34, e4844 CrossRef CAS PubMed.
- J. M. M. Kahl, K. F. da Cunha, L. C. Rodrigues, K. O. Chinaglia, K. D. Oliveira and J. L. Costa, J. Pharm. Biomed. Anal., 2021, 196, 113928 CrossRef CAS PubMed.
- M. Kertys, M. Krivosova, I. Ondrejka, I. Hrtanek, I. Tonhajzerova and J. Mokry, J. Pharm. Biomed. Anal., 2020, 181, 113098 CrossRef CAS PubMed.
- Y. Li, R. Cleary, M. Kellogg, J. S. Soul, G. T. Berry and F. E. Jensen, J. Chromatogr. B: Anal. Technol. Biomed. Life Sci., 2011, 879, 998–1002 CrossRef CAS.
- J. W. Bae, C. I. Choi, C. G. Jang and S. Y. Lee, Biomed. Chromatogr., 2011, 25, 1181–1188 CrossRef CAS PubMed.
- A. M. Teitelbaum, A. M. Flaker and E. D. Kharasch, J. Chromatogr. B: Anal. Technol. Biomed. Life Sci., 2016, 1017–1018, 101–113 CrossRef CAS.
- L. Wagmann, S. Hemmer, A. T. Caspar and M. R. Meyer, Clin. Chem. Lab. Med., 2020, 58, 664–672 CrossRef CAS PubMed.
- C. Mariotti Kde, R. S. Schuh, P. Ferranti, R. S. Ortiz, D. Z. Souza, F. Pechansky, P. E. Froehlich and R. P. Limberger, J. Anal. Toxicol., 2014, 38, 432–437 CrossRef PubMed.
|
This journal is © The Royal Society of Chemistry 2022 |