DOI:
10.1039/D2RA02425J
(Paper)
RSC Adv., 2022,
12, 15133-15144
2-Methoxy-1,4-naphthoquinone regulated molecular alternation of Fusarium proliferatum revealed by high-dimensional biological data†
Received
15th April 2022
, Accepted 2nd May 2022
First published on 17th May 2022
Abstract
Fungi Fusarium proliferatum and the toxins it produces are hazardous to agricultural plants, animals, and human health. However, there is a lack of more effective and environment-friendly natural anti-F. proliferatum agents. In the search for natural anti-fungal agents, we found that naturally originated 2-methoxy-1,4-naphthoquinone (MNQ) with a minimal inhibitory dose of 8.0 mg L−1 possessed a potential inhibitory effect on F. proliferatum. The results of transcriptomic, proteomic, and metabolomic reveal a total of 1314 differential expression genes (DEGs, 873 up-regulated and 441 down-regulated), 259 differential expression proteins (DEPs, 104 up-regulated and 155 down-regulated), and 86 differential accumulation metabolites (DAMs, 49 up-regulated and 37 down-regulated) in MNQ-induced F. proliferatum. Further, the correlation analysis of transcriptomic, proteomic, and metabolomic indicated that these DEGs, DEPs, and DAMs were co-mapped in the pathways of glyoxylate and dicarboxylate metabolism, glycine, serine, and threonine metabolism, and pyruvate metabolism that linked to the TCA cycle. Furthermore, the key DEGs of the significantly co-mapped pathways were verified with qPCR analysis, which was related to the permeability of the cell membrane of F. proliferatum. Thus, these findings will provide fundamental scientific data on the molecular shifts of MNQ-induced F. proliferatum.
1 Introduction
Fungi exist extensively in nature; some of these can seriously harm agricultural plants and the toxins they produce are harmful to animal and human health.1 Fusarium proliferatum, belonging to the Fusarium genus, is a worldwide fungal pathogen that can parasitize a variety of other plants, such as maize, onion, sorghum, pearl millet, and date palm,2–5 and the fumonisins it produces also pose a potential threat to agricultural product safety.6 At present, industrial fungicides are used to manage Fusarium as agricultural techniques and biological methods only give little alleviation from the disease.7 Fusarium is only controlled with a few industrial fungicides. However, these widely used chemical fungicides are limited by several serious problems,8 such as serious residual toxicity, the development of drug resistance, environmental pollution, and health hazards. Natural products as prospective safe fungicides have lately gotten a lot of interest because of their low toxicity or non-toxicity when compared to synthetic commercial fungicides.9,10 Therefore, it is urgent to find anti-fungal drugs with more effective and less poisonous to defend against F. proliferatum.
2-Methoxy-1,4-naphthoquinone (MNQ) (Fig. 1) is a phytochemical found in the Impatiens balsamina Linn,11 as well as a marine microbe Nocardiopsis alba.12,13 MNQ has shown anti-Helicobacter pylori capabilities comparable to that of amoxicillin,14 and have shown anti-pruritic,15 anti-inflammatory16 and anti-fungal17 qualities in previous research. Although some research on the antibacterial mechanisms of MNQ such as anti-Penicillium italicum and anti-P. digitatum has been reported,13,18 the anti-F. proliferatum action and molecular mechanisms of MNQ have yet to be investigated. Therefore, the specific molecular details behind the alterations in the genes, proteins, and metabolites in F. proliferatum treated with MNQ should be studied.
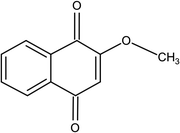 |
| Fig. 1 Chemical structure of 2-methoxy-1,4-naphthoquinone (MNQ). | |
In this research, the inhibitory impact and molecular details of MNQ on F. proliferatum were elucidated via high-dimensional biodata including transcriptomics, proteomics, and untargeted metabolomics, which can give details of differently enriched genes, differently enriched proteins, and differently enriched metabolites of F. proliferatum treated with MNQ. Furthermore, the key differently enriched genes related to the pathways of glyoxylate and dicarboxylate metabolism, glycine, serine, and threonine metabolism, and pyruvate metabolism were verified using qPCR. These analyzed data will aid in hypothesizing the anti-F. proliferatum activity of MNQ, which is beneficial for developing MNQ as a natural precursor of an environment-friendly anti-fungal agent.
2 Materials and methods
2.1 Microorganism and minimum inhibitory concentration (MIC) tests
F. proliferatum applied in this research was provided by the South China Botanical Garden, Chinese Academy of Sciences (Guangzhou, China). F. proliferatum was maintained in PDA (HuanKai Microbial, China) at 28 °C. Effects of MNQ (≥98%, Sigma, USA) on the mycelial growth of F. proliferatum were determined in vitro by the MIC test.19,20 PDA (10 mL) was poured into a sterile Petri dish with a diameter of 60 mm and measured amounts of MNQ (dissolved in DMSO) were added to PDA media to give desired concentrations of 0.0, 0.5, 1.0, 2.0, 4.0, 8.0, 16.0, and 32.0 mg L−1. The spores of F. proliferatum were washed down with sterile water from the PDA plate and diluted to a concentration of 1 × 107 conidia per mL. Then, the above 10 μL of conidial suspensions were evenly smeared onto the surface of each plate. Finally, all the culture plates were treated for 48 hours at 28 °C. Three biological replicates were conducted.
2.2 MNQ treatment
2 mL of the diluted spore suspension (1 × 107 conidia per mL) was inoculated in the 200 mL Czapek's broth medium (CB, 30 g L−1 sucrose, 3 g L−1 NaNO3, 1 g L−1 K2HPO4, 0.5 g L−1 MgSO4·7H2O, 0.5 g L−1 KCl, 0.01 g L−1 FeSO4, pH 7.2). The conical flasks with spores were incubated at 28 °C with 140 rpm, which were shaken for sporulation in the dark. After 48 h of cultivation, the suspensions were then treated by MNQ at two concentrations (0 and 5 mg L−1) and incubated at 28 °C with 140 rpm for another 48 h for molecular analyses. Ultimately, the mycelium was washed by PBS for omics analysis.
2.3 Transcriptome profiling
The Trizol reagent (Invitrogen, CA, USA) was used to extract total RNA from frozen mycelium. The stability and concentration of RNA were determined using the NanoPhotometer® spectrophotometer (Implen, CA, USA), Qubit® 2.0 fluorometer (Life Technologies, CA, USA), and Agilent Bioanalyzer 2100 system. RNA Library Prep Kit (Illumina, CA, USA) was used to create cDNA libraries for sequencing from 3 μg of total RNA. The library fragments were purified using the AMPure XP system to identify cDNA fragments with a length preference of 250–300 bp. The cDNA libraries were then enriched using PCR amplification, and the quality of the cDNA libraries was determined using the Agilent Bioanalyzer 2100 system. Finally, the Illumina platform was used to sequence the cDNA libraries, providing paired-end reads of 125 bp/150 bp.
All downstream analyses were based on clean readings, which were trimmed from the raw data. The read counts mapped to each gene were counted using FeatureCounts v1.5.0-p3. Then, depending on the length of the gene and the number of reads mapped to it, the fragments per kilobase per million (FPKM) of each gene was determined.21 The Benjamini and Hochberg strategy for controlling the false discovery rate was used to alter the p-value for the comparison of the reads per kilobase per million (RPKM) between the treatment and the control groups. Differentially expressed genes were classified as those with an adjusted p-value < 0.05 and |log
2 fold change| > 1.
2.4 Proteomics experiments
As previously indicated, the mycelium was treated for complete protein extraction.22 In a nutshell, 200 μL of pre-chilled lysis solution (4% SDS, 100 mM DTT, 150 mM Tris–HCl, pH 8.0) was added to the mycelium. A homogenizer was used to smash the mycelium, which was then placed in boiling water for 5 min. The ultrasonically broken dissolved products were then returned to boiling water for another 5 min. The supernatant was then collected and evaluated using the BCA Protein Assay Kit (Bio-Rad, CA, USA) following centrifugation at 14
000 rpm for 15 min. The proteins were then digested with trypsin (Promega, WI, USA), and the peptide concentration was determined using a 280 nm UV light spectral density. Each sample's peptide was desalted using C18 Cartridges (Sigma, MO, USA), then vacuum centrifuged and reconstituted in 0.1% (v/v) trifluoroacetic acid. A Q Exactive mass spectrometer coupled to an Easy nLC (Thermo Fisher Scientific, MA, USA) was used to examine the peptides. The mass spectrometry data were compared to the NCBI F. proliferatum ET1 database and processed and quantified using a MaxQuant 1.3.0.5.23 Protein abundance was estimated using the normalized spectral protein intensity (LFQ intensity), and the statistical significance of the data was determined using Student's t-test. Proteins with a p-value < 0.05 and fold change > 2.0 (up-regulated) or fold change < 0.5 (down-regulated) were defined as differentially expressed.
2.5 Metabolomics analysis
The samples were homogenized in water before being combined in a 1
:
1 mixture of methanol and acetonitrile. The materials were then homogenized twice more using ultrasonication at 4 °C for 30 min each time. The mixture was centrifuged for 20 min (14
000 rcf, 4 °C) after being stored at −20 °C for 1 hour to remove the protein. The supernatant was collected and then dried in a vacuum centrifuge. The samples were re-dissolved in 100 μL acetonitrile/water (1
:
1, v/v) solvent for LC-MS analysis. Quality control (QC) samples were created by pooling 10 μL of each sample and analyzing them together with the other samples to monitor the instrument's stability and repeatability. The QC samples were introduced regularly. In Shanghai Applied Protein Technology Co., Ltd., analyses were carried out utilizing a UHPLC (1290 Infinity LC, Agilent Technologies) linked to a quadrupole time-of-flight (AB Sciex TripleTOF 6600). A 2.1 mm × 100 mm ACQUIY UPLC BEH 1.7 μm column (Waters, Ireland) was used to examine samples for HILIC separation. The mobile phase in both the positive and negative ESI modes contained A = 25 mM ammonium acetate and 25 mM ammonium hydroxide in water, as well as B = acetonitrile. For 1 min, the gradient was 85% B, linearly lowered to 65% in 11 min, reduced to 40% in 0.1 min and held for 4 min, and then raised to 85% in 0.1 min with a 5 minute re-equilibration interval. The following were the electrospray ionization (ESI) source conditions: Ion Source Gas1 (Gas1) was set to 60, Ion Source Gas2 (Gas2) was set to 60, curtain gas (CUR) was set to 30, source temperature was set to 600 °C, and IonSpray Voltage Floating (ISVF) was set to ±5500 V. The instrument was configured to acquire across the m/z range 60–1000 Da in MS alone acquisition, and the accumulation time for Time-off-Flight Mass Spectrometer (TOF MS) scan was set at 0.20 s per spectra. The instrument was configured to acquire across the m/z range 25–1000 Da in auto MS/MS acquisition, and the accumulation time for product ion scan was set at 0.05 s per spectra. Information dependent acquisition (IDA) with high sensitivity mode was used to obtain the product ion scan. The following settings were used: collision energy (CE) was set to 35 V with 15 eV; declustering potential (DP) was set to 60 V (+) and −60 V (−); isotopes inside 4 Da were excluded; candidate ions to monitor each cycle: 10.
2.6 RT-qPCR analysis
Total RNA Kit (Gbcbio, China) was used to extract total RNA from each sample, and the RNA concentration was determined using a Nanodrop 2000C spectrophotometer (Thermo Scientific, USA). Hiscript II 1st Strand cDNA Synthesis Kit (Vazyme, China) was used to make the first-strand cDNA template. The genes' relative expression levels were chosen at random. The reaction system was SYBRgreen real-time PCR super Mix (Mei5 Biotechnology, China) and PCR conditions were 95 °C for 15 s, 55 °C for 15 s, 72 °C for 42 s, 40 cycles. NCBI was used to design the primers (Table S2†). The expression levels of the target genes were determined using the 2−ΔΔCt technique, with histone H3 served as an internal reference gene.
2.7 Malondialdehyde (MDA) and H2O2 content analysis
The Malondialdehyde Assay Kit (Solarbio, China) was used to determine MDA concentration in 0.1 g powders from F. proliferatum using the thiobarbituric acid (TBA) technique. SpectraMax i3x determination of absorbance and calculation of MDA content according to the instructions were carried out. On a fresh weight basis, the MDA content was given as μmol g−1. Hydrogen Peroxide Assay Kit (Solarbio, China) was used to evaluate the H2O2 concentration in 0.1 g powders from F. proliferatum. Finally, the H2O2 content was determined using SpectraMax i3x according to the instructions. On a fresh weight basis, the H2O2 content was represented as μmol g−1.
2.8 Relative electrical conductivity analysis
F. proliferatum was inoculated in PDA dishes and cultured for 48 h. After that, F. proliferatum mycelium blocks were obtained by punching holes in the well-growing colonies with a 5 mm perforator. Mycelium blocks were put into a beaker containing 20 mL 8 mg L−1 MNQ solution and 20 mL deionized water, respectively. MNQ solution was added as the treatment group and deionized water as the control group. The electrical conductivity of F. proliferatum was measured with a conductivity meter after 0 h and 2 h treatment. The beaker should be fully shaken before measurement. After 10 h treatment, the beaker was heated in boiling water for 5 min and the electrical conductivity was measured again. The calculation formula of relative conductivity is as follows: C (relative conductivity) = (C − C0)/(D − C0) × 100%, where C0 represents the conductivity of 0 h treatment, C represents the conductivity of 2 h treatment, and D represents the conductivity of 10 h treatment and heating.
3 Results
3.1 Effects of MNQ on F. proliferatum
As depicted in Fig. 2, the minimum inhibitory concentration (MIC) of MNQ was found to be 8.0 mg L−1 because F. proliferatum grew at 4.0 mg L−1 and did not grow at 8.0 mg L−1. To establish the optimal timing for mycelium control and extraction, a concentration of MNQ of 8.0 mg L−1 was used to treat F. proliferatum within the MIC range. According to MIC, F. proliferatum was treated with the control group without MNQ and the treatment group with 8.0 mg L−1 MNQ. The findings indicated that the growth of F. proliferatum in the treatment group was slow and the color of the culture medium was yellow, while the growth of F. proliferatum in the control group was faster and the color of the culture medium was green. Subsequently, the anti-microbial mechanism of MNQ was studied according to the MIC of MNQ against F. proliferatum.
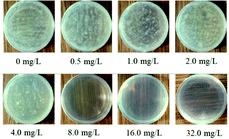 |
| Fig. 2 MIC of MNQ against F. proliferatum (48 h). | |
3.2 Transcriptomic analysis
A total of 15
319 genes were detected in the control and treatment groups. Based on the padj < 0.05 and |log
2 fold change| > 1 for the significance of different standards, screening different genes between the treatment group (T1, T2, and T3) and the control group (C1, C2, and C3) was done. As shown in Fig. 3A, a total of 1314 DEGs were screened, with 873 being up-regulated and 441 being down-regulated. The cluster analysis of different genes in the treatment group and the control group can more intuitively describe the situation of different genes in F. proliferatum. The DEseq normalized value of the differentially expressed genes was taken as the expression level, and the expression level was normalized. Clustering results were obtained by hierarchical clustering analysis; different clustering information is presented in different colors, with the red portion representing the up-regulated gene and another part representing the down-regulated gene (Fig. 3B). Different genes in the same group may have the same function or the same biological characteristics. The similarity of DEGs within the groups was higher, and the trend was opposite between the groups.
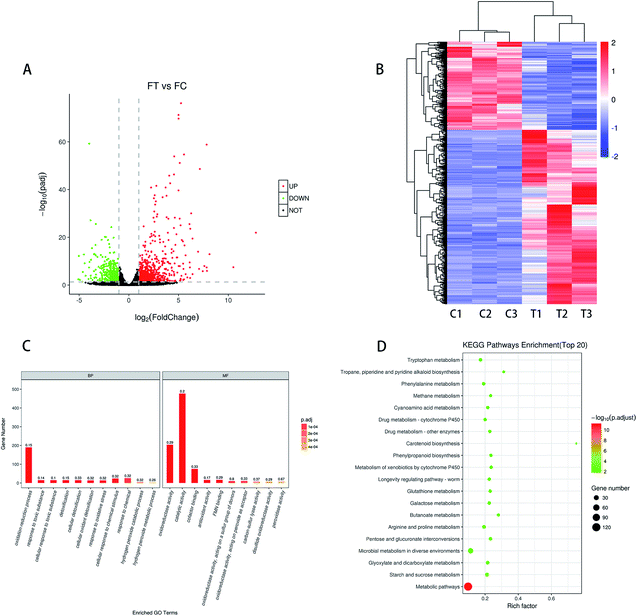 |
| Fig. 3 (A) Volcano maps of differentially expressed genes; (B) hierarchical cluster analysis was conducted for the DEGs, C and T represented the control group and the treatment group; (C) enriched GO terms of total DEGs; (D) enriched KEGG pathways of total differential genes (top 20). | |
3.2.1 Gene ontology (GO) annotation. As the GO enrichment data illustrates in Fig. 3C, based on significant enrichment analysis (p < 0.05) of the total DEGs and the up-regulated DEGs in the MNQ treated F. proliferatum, the genes primarily changed the catalytic activity of the enzymes and oxidoreduction reactions. Simultaneously, the oxidation–reduction process, cofactor binding, FMN binding, as well as cellular detoxification may all have a role in inhibiting F. proliferatum proliferation.
3.2.2 Kyoto encyclopedia of genes and genomes (KEGG) annotation. The KEGG pathway research was applied to the different genes of the treatment group and the control group, and 20 pathways with the highest significant enrichment were selected to create a scatter KEGG pathway plot (Fig. 3D). A total of 1314 DEGs were enriched into 37 signaling pathways (p < 0.05), including 11 pathways of energy and carbon metabolism, 10 pathways of amino acid metabolism, and 6 pathways of substance degradation and secondary metabolite synthesis, indicating that MNQ has a stronger impact on the energy and carbon metabolism genes, as well as amino acid metabolism genes. As shown in the scatter diagram of the total differentially expressed genes KEGG pathway (Fig. 3D), MNQ treatment showed the most significant enrichment effect on the metabolic pathways with 121 differentially expressed genes involved in this pathway. As shown in the scatter diagram of the up-regulated genes KEGG pathway (Fig. S1a†), the up-regulated genes were involved in 22 signaling pathways after MNQ treatment (p < 0.05), including microbial metabolism in diverse environments, starch and sucrose metabolism, galactose metabolism, as well as glyoxylate and dicarboxylate metabolism. There were 20, 13, 9, and 6 differential genes involved in these pathways, respectively.As shown in the scatter diagram of the down-regulated genes KEGG pathway (Fig. S1b†), the down-regulated genes were involved in 21 signaling pathways after MNQ treatment (p < 0.05), which were contained in the biosynthesis of secondary metabolites, carbon metabolism, alanine, aspartate and glutamate metabolism, tyrosine metabolism, valine, leucine and isoleucine degradation, and pyruvate metabolism. There were 16, 9, 8, 6, 5, and 5 differential genes involved in these pathways, respectively. Most of the pathways related to up-regulation genes belong to carbohydrate metabolism, while the majority of pathways related to down-regulation genes belong to amino acid metabolism.
3.3 Proteomics analysis
In the control and treatment groups, a total of 2627 proteins were found. Based on the fold change > 2 or fold change < 0.5, and p < 0.05 for the significance of the different standards, screening the control group (C) and the treatment group (T) gave the difference between the proteins. As shown in Fig. 4A, a total of 259 DEPs were screened, among which 104 were up-regulated and 155 were down-regulated. In general, after MNQ treatment, the number of down-regulated proteins in F. proliferatum was more than the up-regulated proteins. Clustering results were obtained by hierarchical clustering analysis; different clustering information is presented in different colors, where the red part and the blue part represent the up and down-regulated proteins, respectively (Fig. 4B). Different proteins in the same group may have the same function or the same biological characteristics.
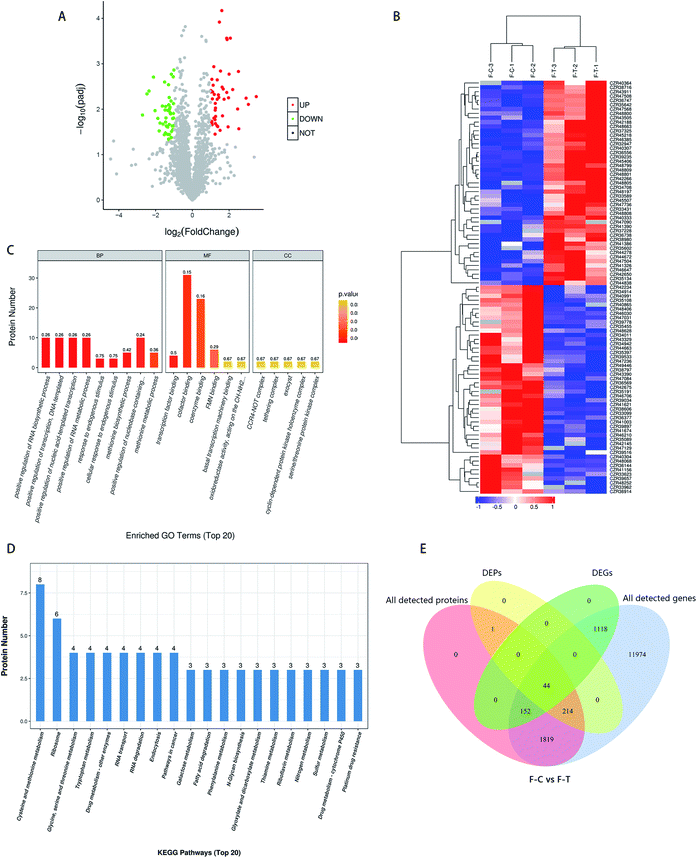 |
| Fig. 4 (A) Volcano maps of differentially expressed proteins; (B) the DEPs were subjected to hierarchical cluster analysis, C and T represented the control group and the treatment group, respectively; (C) enriched GO terms (the rich factor was represented by the number above the bar, and the color of the bar reflects the value of p); (D) enriched KEGG pathways of total differential proteins; (E) Venn diagram of all the detected genes, DEGs, all the detected proteins, and DEPs. (F-C) Control group, (F-T) treatment group. | |
3.3.1 GO annotation. All the different proteins between the treatment group and the control group were mapped to the GO database, and the number of proteins in each term was counted to find the significantly changed terms (FDR < 0.05). As shown in Fig. 4C, after MNQ treatment, the functions of DEPs enriched in BP were mainly related to nucleic acid transcription and amino acid metabolism. For example, the positive regulation of nucleic acid-templated transcription involved 10 different proteins, and the aspartate family amino acid metabolic process involved 6 and the sulfur amino acid metabolic process involved 5. The functions of differential proteins enriched in MF are mainly related to the terms of cofactor binding, transition metal ion binding, FMN binding, and transcription factor binding, which involve 31, 17, 6, and 4 different proteins, respectively. However, the functions of DEPs enriched in cellular component (CC) are mainly related to the terms of cyclin-dependent protein kinase holoenzyme complex and serine/threonine protein kinase complex, which just involve 2 and 2 different proteins, respectively. The biological functions of differential genes' enrichment were mainly the catalytic activity and oxidoreduction activity, while the biological functions of DEPs enrichment were mainly transcriptional regulation and substance binding, indicating that MNQ treatment may cause different changes in genes and proteins.
3.3.2 KEGG annotation. In the DEPs of the treatment and the control groups, a total of 130 pathways were activated. However, only two pathways, namely, cysteine and methionine metabolism, and riboflavin metabolism, were significantly enriched, which involved 8 and 3 differential proteins, respectively (p < 0.05). In addition, the pathways such as ribosome, glycine, serine, and threonine metabolism, tryptophan metabolism, drug metabolism-other enzymes, RNA transport, galactose metabolism, glyoxylate and dicarboxylate metabolism, and drug metabolism-cytochrome P450 were also affected by MNQ (Fig. 4D). DEGs were enriched in amino acid metabolism and carbohydrate metabolism pathways, while DEPs were enriched in amino acid metabolism and nucleic acid metabolism pathways. These findings showed that MNQ may inhibit the growth of F. proliferatum by altering the amino acids, nucleic acids, and carbohydrate metabolism.
3.4 Combined analysis of transcriptomics and proteomics
To compensate for data difficulties caused by a lack of data, noise, or other variables in a single piece of data, multiple sets of data must be integrated and analyzed. At the same time, the reciprocal verification of various sets of data resources can decrease the false positives created by a single set of analyses. First, we conducted a transcriptome and proteome correlation study. We discovered 2230 genes linked to the protein; however, only 44 of them were DEGs. We discovered that 1819 proteins remained unchanged, and their transcription levels remained consistent. Among the 1314 DEGs, there were 1118 DEGs with no protein matches in the proteome data and 152 DEGs with no alterations in their protein products. Only 44 of the 259 DEPs had substantial transcriptional alterations, whereas 214 had no variations in their transcription levels (Fig. 4E). The changes in these 259 DEP levels might be influenced by post-transcriptional mechanisms. Although proteins changed less in the proteomic (259) than the transcriptomic (1314) region, the fraction of DEPs (259/2230) was greater in the transcriptome (1314/15
321). Our proteome exhibits limited association with the transcriptome, which is consistent with various prior research on the mismatch between transcriptomes and proteomes. The biological functions of differential gene enrichment were mainly in the catalytic activity and oxidoreduction activity, while the biological functions of differential protein enrichment were mainly in transcriptional regulation and substance binding, indicating that MNQ treatment may cause different changes in genes and proteins.
3.5 Metabolomics analysis
3.5.1 Multi-dimensional statistical analysis. PCA and OPLS-DA models were used for multi-dimensional statistical analysis to evaluate the test reliability after the original data were preprocessed. In the PCA score graph, t[1] represented the first principal component in the abaxial coordinate, and t[2] represented the second principal component in the vertical coordinate. Meanwhile, the model is more stable and reliable when the R2X value is more close to 1 (Fig. 5A and B). In the OPLS-DA score graph, t[1] represented the first principal component in both the abaxial and vertical coordinates; meanwhile, the model was more stable and accurate when the R2Y and Q2 values were closer to 1 (Fig. 5C and D). In the PCA model, the R2X value in the positive and negative ion modes was 0.508 and 0.621, respectively (Table 1). In the OPLS-DA model, the R2Y value in the positive and negative ion modes was 0.995 and 0.995; meanwhile, the Q2 value in the positive and negative ion modes was 0.773 and 0.760, respectively (Table 1). These data meant that both the models were stable and reliable, which could fully explain the difference between the treatment and the control groups.
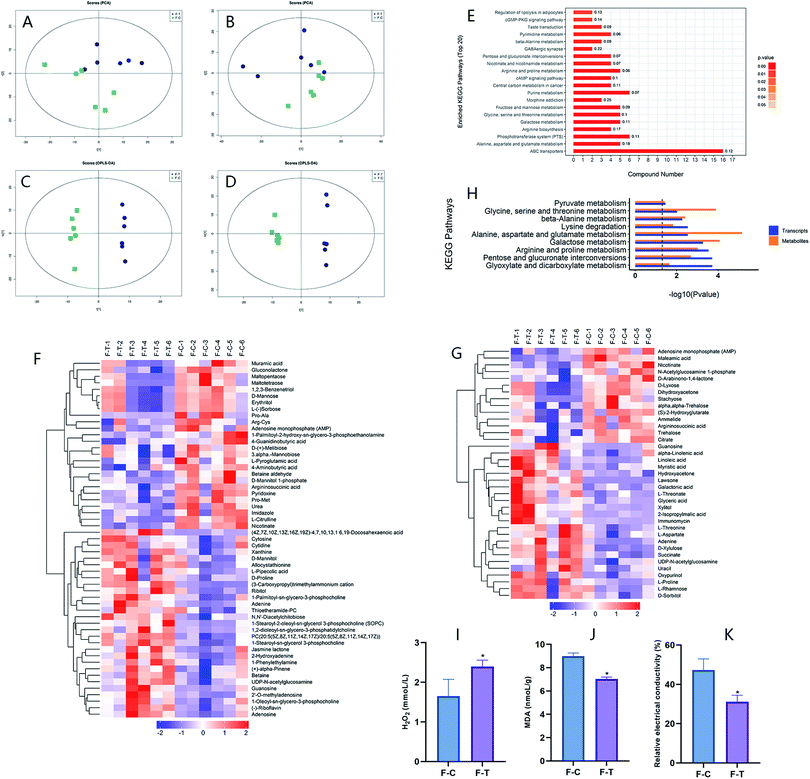 |
| Fig. 5 (A and B) PCA score graph: positive modes (A) and negative modes (B); (C and D) OPLS-DA score graph: positive modes (C) and negative modes (D); (E) enriched KEGG pathways of differential metabolites; (F and G) hierarchical cluster heat map of differential metabolites in the positive mode (F) and the negative mode (G); (H) enriched KEGG pathways of both transcriptome and metabolomics; (I–K) H2O2 content (I), malondialdehyde (MDA) content (J) and relative electrical conductivity (K). (F-C) represented the control group, and (F-T) represented the treatment group. * meant p < 0.05. | |
Table 1 PCA and OPLS-DA model parameters
Model |
PCA |
OPLS-DA |
R2X |
R2Y |
Q2 |
Positive mode |
0.508 |
0.995 |
0.773 |
Negative mode |
0.621 |
0.995 |
0.760 |
Differential metabolites were screened according to the Variable Importance for the Projection (VIP) in the OPLS-DA model: VIP > 1 and p < 0.1. On account of the significantly different metabolites of the samples between the two groups, the rationality of the identified metabolites was assessed. The connection between F. proliferatum and the metabolites in F. proliferatum was clarified by hierarchical cluster analysis. This approach was conducive to researching the changes in the candidate metabolic processes and the accurate selection of marker metabolites (Fig. 5F and G). As shown in Table S1,† a total of 86 differential accumulation metabolites (DAMs, 49 up-regulated and 37 down-regulated) were screened, which can be divided into 8 categories, which are carbohydrates, lipids, amino acids, nucleic acids, peptides, cofactors/vitamins, energy, and others. Many differential metabolites belong to the carbohydrates and lipids categories.
3.5.2 KEGG annotation. On the different metabolites in the treatment and control groups, a KEGG pathway research was applied, and a total of 95 pathways were involved in the differential metabolites, and 41 pathways were significantly enriched (p < 0.05), including 9 material transport and signal transduction pathways, 7 amino acid metabolism pathways, 7 carbohydrate metabolism pathways, 3 lipid metabolism pathways, 3 nucleic acid metabolism pathways, 2 cofactors and vitamin metabolism pathways. Among them, the cAMP signal pathway, cGMP-PKG signal pathway, as well as the phosphotransferase system, were involved in material transport and signal transduction pathways. Alanine, aspartate, and glutamate metabolism pathway, arginine biosynthesis pathway, glycine, serine, and threonine metabolism pathway, as well as arginine and proline metabolism pathway, were involved in amino acid metabolism pathways. Galactose metabolism pathway, fructose and mannose metabolism pathway, as well as pentose and glucuronate interconversions pathway were involved in carbohydrate metabolism pathways, which also included citrate cycle pathway, glyoxylate and dicarboxylate metabolism pathway, and pyruvate metabolism pathway. The biosynthesis of unsaturated fatty acids pathway, linoleic acid metabolism pathway, and glycerolipid metabolism pathway was involved in the lipid metabolism pathways. The top 20 pathways with the most significant enrichment are shown in Fig. 5E.
3.6 Combined analysis and validation of omics
3.6.1 RT-qPCR verification of DEGs in metabolism pathways. Differential genes, proteins, and metabolites were respectively enriched into 37, 2, and 41 metabolic pathways (p < 0.05). Glyoxylate and dicarboxylate metabolism pathway, glycine, serine and threonine metabolism pathway, and pyruvate metabolism pathway were enriched in both transcriptome and metabolomics (Fig. 5H) and presented in proteomics (Fig. 4D).To verify whether MNQ treatment can promote these metabolism pathways of F. proliferatum, we selected a total of 10 differential genes as the key genes from the transcriptome result for RT-qPCR verification, which were involved in the pathways above. As shown in Table 2, among them, glutathione S-transferase (GST), formate dehydrogenase (FDH), catalase (CAT), 5-oxoprolinase, glutathione reductase (GR), and formamidase were up-regulated at the mRNA level, which is consistent with the transcriptome results. Peroxidase, phosphoenolpyruvate carboxykinase (PEPCK), glycine hydroxymethyltransferase (glyA), and acetyl-CoA synthetase (ACS) were down-regulated at the mRNA level, which is consistent with the transcriptome results. It suggests that the effects of MNQ on F. proliferatum were related to the pathways of glyoxylate and dicarboxylate metabolism, glycine, serine and threonine metabolism, and pyruvate metabolism.
Table 2 RT-qPCR verification
Gene name |
RT-qPCR |
Transcriptome |
Glutathione S-transferase (GST) |
33.65 |
2.52 |
Formate dehydrogenase (FDH) |
28.15 |
3.83 |
Catalase (CAT) |
8.34 |
3.98 |
5-Oxoprolinase |
5.65 |
3.17 |
Glutathione reductase (GR) |
4.07 |
2.00 |
Formamidase |
3.33 |
1.47 |
Peroxidase |
0.59 |
−2.41 |
Phosphoenolpyruvate carboxykinase (PEPCK) |
0.38 |
−1.62 |
Glycine hydroxymethyltransferase (glyA) |
0.30 |
−1.88 |
Acetyl-CoA synthetase (ACS) |
0.24 |
−1.64 |
3.6.2 Permeability verification of cell membrane. According to the integrated analysis of multiple omics results, the catalase (CAT) activity may be caused by excessive H2O2.24 As a major enzyme for the elimination of H2O2, CAT was involved in several biological pathways including glyoxylate and dicarboxylate metabolism.25 H2O2 can cause membrane lipid oxidation, while malondialdehyde (MDA) is the product of membrane lipid peroxidation, which is an important indicator for the identification of membrane peroxidation.26 The permeability of the cell membrane will be changed and the permeability of cell contents will increase when the membrane structure was destroyed, which will lead to an increase in the relative electrical conductivity.27 Therefore, it is necessary to further determine the H2O2 content, the MDA content, and relative conductivity of F. proliferatum to clarify the inhibition mechanism of MNQ on F. proliferatum. As shown in Fig. 5I–K, the H2O2 content was considerably greater in the treatment group than in the control group but the MDA content and relative electrical conductivity were decreased in the treatment group.
4 Discussion
MNQ could be considered as a natural anti-microbial agent from its ability to inhibit F. proliferatum. In this study, MIC was used as an indicator to identify the activity against F. proliferatum. Previous studies have found that tebuconazole is one of the most widely used anti-fungal compounds in agriculture in many European nations to control fungal diseases in cereals and other crops, and it is particularly efficient against Fusarium species.28,29 The MIC value of tebuconazole fungicide on F. proliferatum was greater than 10 mg mL−1.7,30 Compared with tebuconazole, we found that the inhibition effect depends on the concentration of MNQ. F. proliferatum was completely killed by MNQ at the concentration of 8 mg L−1, which may be more effective and safer. Through the multi-omics analysis of GO and KEGG enrichment, we found that it had a high proportion of correlation with glyoxylate and dicarboxylate metabolism pathway, glycine, serine and threonine metabolism pathway, and pyruvate metabolism pathway.
4.1 TCA cycle of F. proliferatum affected by MNQ
From the RT-qPCR data of DEGs, we proved that the pathway of MNQ on F. proliferatum was related to the pathways of glyoxylate and dicarboxylate metabolism, glycine, serine, and threonine metabolism, and pyruvate metabolism. Based on the existing studies, these pathways were all found to be related to energy metabolism and the TCA cycle, which were required to decrease acetyl-CoA and provide the reducing equivalents involved in creating ATP.18,31–34
In the glyoxylate and dicarboxylate metabolism pathway, the genes of catalase (CAT), formate dehydrogenase (FDH), and formamidase were up-regulated, while glycine hydroxymethyltransferase (glyA) and acetyl-CoA synthetase (ACS) were down-regulated. Also, the differential metabolites included succinate, citrate, and glyceric acid. Succinate and citrate are important intermediates in both the glyoxylate cycle and TCA cycle (Fig. 6), which is the most efficient way to obtain energy in vivo, and the ultimate metabolic pathway for carbohydrates, lipids, and amino acids.35,36 By the down-regulated differential metabolite citrate, both the glyoxylate cycle and TCA cycle may be restrained. The glyoxylate cycle, such as the TCA cycle, starts with the conversion of oxaloacetate and acetyl-CoA to isocitrate.37,38 Some microorganisms can use dicarboxylate as a material to synthesize dicarboxylate and tricarboxylic acid through the glyoxylate and dicarboxylate metabolism pathway, and can also make acetate into acetyl-CoA catalyzed by ACS to supplement compounds in the TCA cycle. At the same time, in microorganisms, the exchange of lipids and sugars can be carried out through the glyoxylate and dicarboxylate metabolism pathway. Lipid metabolites will be converted to acetyl-CoA via β-oxidation, and then passed through the glyoxylate and dicarboxylate metabolism pathway, as well as the TCA cycle, to generate energy.39 From the results of this study, the TCA cycle can be restrained by the down-regulated of acetyl-CoA synthetase, which may play role in the glyoxylate and dicarboxylate metabolism pathway and the TCA cycle.
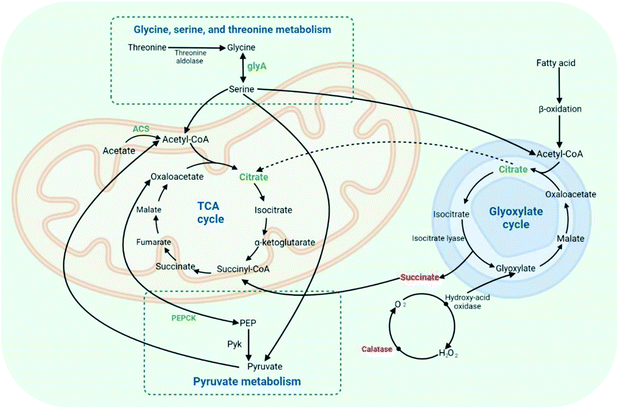 |
| Fig. 6 Mechanism diagram of the TCA cycle, glyoxylate cycle, pyruvate metabolism and glycine, serine, and threonine metabolism. Down-regulated genes are displayed as green, and up-regulated genes are displayed as red. ACS, acetyl-CoA synthetase; glyA, glycine hydroxymethyltransferase; PEPCK, phosphoenolpyruvate carboxykinase; Pyk, pyruvate kinase. | |
The production and catabolism of the proteinogenic amino acids required for protein synthesis were involved in glycine, serine, and threonine metabolism. There were several routes for these amino acids, starting with threonine, which can be broken into glycine and acetaldehyde by threonine aldolase.40,41 Glycine hydroxymethyltransferase (glyA) may convert glycine to serine, a reversible and interchangeable process. Then, serine may turn into the TCA cycle by combining with oxaloacetate.42,43 From the results of RT-qPCR, glycine was inhibited to turn into serine with the down-regulated glyA so that the TCA cycle may not be activated by the glycine, serine, and threonine metabolism pathways. Oxaloacetate is likely to be converted by phosphoenolpyruvate carboxykinase (PEPCK) to phosphoenolpyruvate (PEP) in the pyruvate metabolism pathway.44 Pyruvate kinase (Pyk) is an important enzyme for converting PEP to pyruvate and ATP. The decrease in PEPCK causes the inhibition of pyruvate and ATP production. Pyruvate is the starting substrate for the TCA cycle and plays a central role in the intermediary metabolism.45,46 From the results of RT-qPCR, the TCA cycle and pyruvate metabolism may be inhibited by the down-regulated PEPCK. As shown in Fig. 6, the effect of MNQ on F. proliferatum was achieved mainly by the disruption of the TCA cycle with the effect of glyoxylate and dicarboxylate metabolism, glycine, serine, and threonine metabolism, and pyruvate metabolism.
4.2 Permeability of cell membrane of F. proliferatum affected by MNQ
The transcriptome results showed that the genes of catalase (CAT), glutathione reductase (GR), and glutathione S-transferase (GST) in F. proliferatum after MNQ treatment were up-regulated. The proteomics results showed that catalase (CAT) was increased. The metabolomics results showed that 13 lipids including α-linolenic acid and linoleic acid were increased, and 10 nucleic acids including adenine, uracil, cytosine, guanosine, and cytidine were increased.
According to the findings, we revealed that MNQ treatment caused F. proliferatum to produce a large number of unsaturated fatty acids, which resulted in the formation of a large amount of H2O2 and led to the breakdown of genetic materials; furthermore, it caused the nucleic acids to increase. Due to excessive H2O2, the catalase activity was accelerated, which influenced the glyoxylate and dicarboxylate metabolism pathway. H2O2 is a relatively stable intracellular reactive oxygen species (ROS) that can be converted into hydroxyl radicals catalyzed by enzymes. Hydroxyl radicals have high oxidizing power that promotes cell depletion.47 The increase in the intracellular ROS can cause oxidative damage, which changes the biological structure and function of macromolecular substances such as DNA, lipids, and proteins.48 The intracellular ROS can be eliminated by metabolites such as glutathione, ascorbic acid, and carotenoids.49,50 5-Oxoproline is an amino acid that is an intermediary in the production and catabolism of glutathione. As a result, glutathione can be catabolized by the up-regulated of 5-oxoprolinase,51 which means that the down-regulation of glutathione reduces ROS inhibition.
As the results showed, to eliminate H2O2 in the cells, catalase (CAT), glutathione reductase (GR), and other enzymes in F. proliferatum were up-regulated. Fatty acids are one of the essential components of the cell membrane. Unsaturated fatty acids such as linolenic acid and linoleic acid will produce H2O2 and free radicals, damaging the structure of the cell membrane.52 The permeability of the cell membrane will be changed and the permeability of the cell contents will increase when the membrane structure was destroyed, which will lead to an increase in the relative electrical conductivity.27 However, metabolites such as betaine, L-proline, succinate, and 2-isopropylmalic acid increased, altering the membrane lipid peroxidation and osmotic pressure, resulting in reduced MDA concentration and relative electrical conductivity in the treatment group compared to the control group. Betaine has a similar chemical structure to amino acids and acts as a buffer against osmotic stress. When the osmotic pressure changes, cells can produce or absorb betaine to maintain normal osmotic pressure to prevent water shunting out of cells and salt invasion. At the same time, betaine can improve the potassium and sodium pump function of the cell membrane.53 Interestingly, proline is an osmotic protectant that can regulate the osmotic potential, remove ROS, stable proteins, and subcellular structure.54,55 The increase in the proline is also the reason for the lower MDA content and relative electrical conductivity in the treatment group compared with the control group.
5 Conclusion
Overall, our present investigation revealed that MNQ had a significant anti-F. proliferatum activity with an MIC value of 8.0 mg L−1. Combining the analysis by transcriptome-proteomic-metabolomic, glyoxylate, and dicarboxylate metabolism pathway, glycine, serine, and threonine metabolism pathway, and pyruvate metabolism pathway, which were enriched in three omics, were all correlated with the TCA cycle pathway. Thus, we confirmed that the mRNA of the TCA cycle and the gene of transcriptome were on the same trend by RT-qPCR. Moreover, by verifying the content of H2O2, MDA, and relative electrical conductivity; it was proved that MNQ treatment influenced glyoxylate and dicarboxylate metabolism pathway, which also affected the structure and permeability of the cell membrane. According to the above results, we speculated that the inhibition mechanism of MNQ on F. proliferatum is due to excessive unsaturated fatty acids promote the production of H2O2 by the cells, which affects the permeability of the cell membrane and leads to the degradation of genetic material, thus inhibiting the growth and reproduction of F. proliferatum. Thus, these findings will contribute to a further understanding of the molecular details of MNQ against F. proliferatum, which are beneficial for improving MNQ as a precursor of an environment-friendly anti-fungal agent.
Author contributions
R. M. Huang conceived and designed the study; J. J. Yang, X. W. Xia, M. X. Guo, and L. Zhong contributed to the acquisition, analysis, and interpretation of data. J. J. Yang and M. X. Guo wrote the manuscript; X. Y. Zhang, X. W. Duan, J. Liu and R. M. Huang reviewed the paper and provided comments, and all of the authors reviewed the manuscript.
Conflicts of interest
The authors declare that they have no competing interests.
Acknowledgements
This work was financially supported by the Natural Science Foundation of Guangxi Province, grant number 2021GXNSFDA075010; the Program of Department of Natural Resources of Guangdong Province, grant numbers GDNRC(2021)53; the Guangdong Provincial Special Fund for Modern Agriculture Industry Technology Innovation Teams, grant numbers 2021KJ122; the Key-Area Research and Development Program of Guangdong Province, grant numbers 2020B1111030004; the general project of Guangdong Medical Science and Technology Research Foundation, grant number A2021207; the Characteristic Innovation Project of Colleges and Universities in Guangdong Province, grant number 2020KTSCX041; the Finance Special Project of Zhanjiang City, grant number 2021A05095; the Discipline construction project of Guangdong Medical University, grant number 4SG21279P.
Notes and references
- M. Jurado, P. Marin, C. Callejas, A. Moretti, C. Vazquez and M. T. Gonzalez-Jaen, Food Microbiol., 2010, 27, 50–57 CrossRef PubMed.
- E. Oldenburg, F. Hoppner, F. Ellner and J. Weinert, Mycotoxin Res., 2017, 33, 167–182 CrossRef CAS PubMed.
- K. D. Beck, C. Reyes-Corral, M. Rodriguez-Rodriguez, C. May, R. Barnett, M. K. Thornton, A. A. Bates, J. W. Woodhall and B. K. Schroeder, Plant Dis., 2021, 105, 494 CrossRef PubMed.
- H. F. Vismer, G. S. Shephard, L. van der Westhuizen, P. Mngqawa, V. Bushula-Njah and J. F. Leslie, Int. J. Food Microbiol., 2019, 296, 31–36 CrossRef CAS PubMed.
- M. Y. Abdalla, A. Al-Rokibah, A. Moretti and G. Mule, Plant Dis., 2000, 84, 321–324 CrossRef CAS PubMed.
- E. Wang, W. P. Norred, C. W. Bacon, R. T. Riley and A. H. Merrill, J. Biol. Chem., 1991, 266, 14486–14490 CrossRef CAS PubMed.
- E. Cendoya, M. J. Nichea, M. D. P. Monge, V. G. L. Zachetti, S. M. Chiacchiera and M. L. Ramirez, Rev. Argent. Microbiol., 2021, 53, 64–74 Search PubMed.
- F. Tini, G. Beccari, A. Onofri, E. Ciavatta, D. M. Gardiner and L. Covarelli, Pest Manag. Sci., 2020, 76, 3738–3748 CrossRef CAS PubMed.
- W. K. Mousa, A. Schwan, J. Davidson, P. Strange, H. Liu, T. Zhou, F. I. Auzanneau and M. N. Raizada, Front. Microbiol., 2015, 6, 1157 Search PubMed.
- N. Witaszak, J. Lalak-Kanczugowska, A. Waskiewicz and L. Stepien, Toxins, 2020, 12, 95 CrossRef CAS PubMed.
- H. F. Jiang, Z. H. Zhuang, B. W. Hou, B. J. Shi, C. J. Shu, L. Chen, G. X. Shi and W. M. Zhang, Evid. Based Complement. Alternat. Med., 2017, 2017, 4245830 Search PubMed.
- Q. Zhang, S. Li, Y. Chen, X. Tian, H. Zhang, G. Zhang, Y. Zhu, S. Zhang, W. Zhang and C. Zhang, J. Antibiot., 2013, 66, 31–36 CrossRef CAS PubMed.
- M. Guo, X. Zhang, M. Li, T. Li, X. Duan, D. Zhang, L. Hu and R. Huang, Int. J. Mol. Sci., 2019, 20, 3459 CrossRef CAS PubMed.
- Y. C. Wang, W. Y. Li, D. C. Wu, J. J. Wang, C. H. Wu, J. J. Liao and C. K. Lin, Evid. Based Complement. Alternat. Med., 2011, 2011, 704721 Search PubMed.
- H. Oku and K. Ishiguro, Phytother. Res., 2001, 15, 506–510 CrossRef CAS PubMed.
- W. Nittayananta, S. Limsuwan, T. Srichana, C. Sae-Wong and T. Amnuaikit, Arch. Oral Biol., 2018, 90, 80–85 CrossRef CAS PubMed.
- Y. C. Wang and Y. H. Lin, Fitoterapia, 2012, 83, 1336–1344 CrossRef CAS PubMed.
- M. Guo, J. Liu, Z. Xu, J. Wang, T. Li, H. Lei, X. Duan, Y. Sun, X. Zhang and R. Huang, J. Agric. Food Chem., 2020, 68, 9697–9706 CrossRef CAS PubMed.
- L. Miao, J. Xu, Z. W. Yao, Y. Jiang, H. R. Zhou, W. Jiang and K. M. Dong, Biotechnol. Biotechnol. Equip., 2017, 31, 1007–1015 CrossRef CAS.
- N. G. Tao, Q. L. OuYang and L. Jia, Food Control, 2014, 41, 116–121 CrossRef CAS.
- B. ter Horst, B. L. Feringa and A. J. Minnaard, Chem. Commun., 2010, 46, 2535–2547 RSC.
- P. Solar, V. Sackova, G. Hrckova, V. Demeckova, M. Kassayova, B. Bojkova, D. Mudronova, S. Gancarcikova, R. Jendzelovsky and P. Fedorocko, Oncol. Rep., 2017, 37, 368–378 CrossRef PubMed.
- T. Gall, G. Lehoczki, G. Gyemant, T. Emri, Z. M. Szigeti, G. Balla, J. Balla and I. Pocsi, Acta Microbiol. Immunol. Hung., 2016, 63, 475–489 CrossRef CAS PubMed.
- A. Bignucolo, V. P. Appanna, S. C. Thomas, C. Auger, S. Han, A. Omri and V. D. Appanna, J. Biotechnol., 2013, 167, 309–315 CrossRef CAS PubMed.
- T. Yanik and R. P. Donaldson, Arch. Biochem. Biophys., 2005, 435, 243–252 CrossRef CAS PubMed.
- M. W. Davey, E. Stals, B. Panis, J. Keulemans and R. L. Swennen, Anal. Biochem., 2005, 347, 201–207 CrossRef CAS PubMed.
- J. Kang, W. Jin, J. Wang, Y. Sun, X. Wu and L. Liu, LWT--Food Sci. Technol., 2019, 101, 639–645 CrossRef CAS.
- S. G. Edwards and N. P. Godley, Food Addit. Contam., Part A: Chem., Anal., Control, Exposure Risk Assess., 2010, 27, 629–635 CrossRef CAS PubMed.
- C. Müllenborn, U. Steiner, M. Ludwig and E.-C. Oerke, Eur. J. Plant Pathol., 2008, 120, 157–166 CrossRef.
- P. Marín, A. de Ory, A. Cruz, N. Magan and M. T. González-Jaén, Int. J. Food Microbiol., 2013, 165, 251–258 CrossRef PubMed.
- Z. Lin, W. Ye, X. Zu, H. Xie, H. Li, Y. Li and W. Zhang, Sci. Rep., 2018, 8, 6619 CrossRef PubMed.
- V. Singh, S. V. Satheesh, M. L. Raghavendra and P. P. Sadhale, Fungal Genet. Biol., 2007, 44, 563–574 CrossRef CAS PubMed.
- S. Mok, B. H. Stokes, N. F. Gnadig, L. S. Ross, T. Yeo, C. Amaratunga, E. Allman, L. Solyakov, A. R. Bottrill, J. Tripathi, R. M. Fairhurst, M. Llinas, Z. Bozdech, A. B. Tobin and D. A. Fidock, Nat. Commun., 2021, 12, 530 CrossRef CAS PubMed.
- A. Crousilles, S. K. Dolan, P. Brear, D. Y. Chirgadze and M. Welch, J. Biol. Chem., 2018, 293, 14260–14269 CrossRef CAS PubMed.
- E. Mills and L. A. O'Neill, Trends Cell Biol., 2014, 24, 313–320 CrossRef CAS PubMed.
- M. Akram, Cell Biochem. Biophys., 2014, 68, 475–478 CrossRef CAS PubMed.
- A. Hartig, M. M. Simon, T. Schuster, J. R. Daugherty, H. S. Yoo and T. G. Cooper, Nucleic Acids Res., 1992, 20, 5677–5686 CrossRef CAS PubMed.
- M. Kunze and A. Hartig, Front. Physiol., 2013, 4, 204 Search PubMed.
- S. O. Curreem, J. L. Teng, H. Tse, K.-Y. Yuen, S. K. Lau and P. C. Woo, Cell Biosci., 2011, 1, 16 CrossRef CAS PubMed.
- J. G. Petersen, M. C. Kielland-Brandt, T. Nilsson-Tillgren, C. Bornaes and S. Holmberg, Genetics, 1988, 119, 527–534 CrossRef CAS PubMed.
- C. Bornaes, J. G. Petersen and S. Holmberg, Genetics, 1992, 131, 531–539 CrossRef CAS PubMed.
- M. Ogawa, J. Moreno-Garcia, L. C. M. Joseph, J. C. Mauricio, J. Moreno and T. Garcia-Martinez, Metabolites, 2021, 11, 150 CrossRef CAS PubMed.
- E. K. Kastanos, Y. Y. Woldman and D. R. Appling, Biochemistry, 1997, 36, 14956–14964 CrossRef CAS PubMed.
- S. Malone, Z. H. Chen, A. R. Bahrami, R. P. Walker, J. E. Gray and R. C. Leegood, Plant Cell Physiol., 2007, 48, 441–450 CrossRef CAS PubMed.
- L. de Bari, D. Valenti, R. Pizzuto, A. Atlante and S. Passarella, Biochim. Biophys. Acta, 2007, 1767, 281–294 CrossRef CAS PubMed.
- S. Y. Kim, J. S. Choi, C. Park and J. W. Jeong, Cancer Lett., 2010, 295, 236–241 CrossRef CAS PubMed.
- M. Schimmel and G. Bauer, Oncogene, 2002, 21, 5886–5896 CrossRef CAS PubMed.
- L. A. Del Rio and E. Lopez-Huertas, Plant Cell Physiol., 2016, 57, 1364–1376 CAS.
- A. J. Meyer, J. Plant Physiol., 2008, 165, 1390–1403 CrossRef CAS PubMed.
- M. A. Gammone, G. Riccioni and N. D'Orazio, Mar. Drugs, 2015, 13, 6226–6246 CrossRef CAS PubMed.
- A. Kumar and A. K. Bachhawat, FEMS Yeast Res., 2010, 10, 394–401 CrossRef CAS PubMed.
- Y. J. Ma, X. P. Li, Y. Wang and J. W. Wang, Microb. Cell Fact., 2021, 20, 92 CrossRef CAS PubMed.
- N. A. Banu, A. Hoque, M. Watanabe-Sugimoto, K. Matsuoka, Y. Nakamura, Y. Shimoishi and Y. Murata, J. Plant Physiol., 2009, 166, 146–156 CrossRef PubMed.
- L. Szabados and A. Savoure, Trends Plant Sci., 2010, 15, 89–97 CrossRef CAS PubMed.
- J. Yang, F. Wang, Y. Wen, S. Gao, C. Lu, Y. Liu and H. Liu, Plant Dis., 2020, 105, 1210 Search PubMed.
|
This journal is © The Royal Society of Chemistry 2022 |