DOI:
10.1039/D2RA00160H
(Paper)
RSC Adv., 2022,
12, 6803-6810
Freshness monitoring of raw fish by detecting biogenic amines using a gold nanoparticle-based colorimetric sensor array†
Received
10th January 2022
, Accepted 11th February 2022
First published on 1st March 2022
Abstract
We herein report the quantitative detection of biogenic amines using a gold nanoparticle-based colorimetric chemosensor array for food analysis. The gold nanoparticles are functionalized with carboxylate derivatives, which capture target amines through hydrogen bonds and electrostatic interactions. The simultaneous discrimination of 10 amine derivatives was achieved by a linear discriminant analysis with a 100% correct classification based on the multi-colorimetric response pattern of structural differences. Furthermore, a real sample analysis for raw fish (i.e., tuna) demonstrated highly accurate determination of histamine concentrations by a support vector machine, the result of which was matched with high-performance liquid chromatography. Most importantly, the chemosensor array succeeded in detecting the time-dependent concentration change of histamine in the raw fish, meaning that the decomposition of the fish could be monitored by the colorimetric changes. Hence, the proposed chemosensor array combined with pattern recognition techniques can be a user-friendly analytical method for food freshness monitoring.
1. Introduction
Biogenic amines are representative analytical targets due to not only their crucial roles in our metabolism but also roles of markers in food analysis.1,2 The instrumental techniques such as high-performance liquid chromatography (HPLC),3–5 gas chromatography-mass spectrometry (GC-MS),6 chemiluminescence,7–9 capillary electrophoresis,10 and electrochemical methods,11–13 can be employed to analyze amines with high accuracy, whereas the requirements of complex procedures and technical personnel limit their easy-to-handle applications.14 In this regard, chemosensors can show specific optical responses to the detection of various analytes.15–18 To date, several types of optical chemosensors have been reported for biogenic amines,20,22–24 including aggregation-induced emission (AIE).19,21 However, these chemosensors were designed for highly selective amine detection, which limited the simultaneous recognition for multi-amines. Thus, chemosensor arrays based on a cross-reactivity combined with pattern recognition techniques can overcome the limitations of the chemosensors.25–29 For example, cucurbit[n]uril-based fluorescence probes exhibited the cross-reactive responses derived from the difference in the molecular rigidity of probes, which successfully recognized various amines based on the cavity size-dependency.30–33 However, the emission wavelength originating from the chemosensor is partially out of the visible range, which means that the amine detection by the naked eye could not be easily achieved.32 In this regard, polythiophene-based chemosensors could change visible colors due to the polymer aggregation by adding the analytes,34–36 which simplified the discrimination process of various amines.37–39 Although several polythiophene-based chemosensors have been reported for histamine detection in food samples, the quantitative analysis of amines is still rare.39 Moreover, the polymer-based colorimetric sensors could not cause drastic spectral changes by amine recognition, which limited the application of on-site detection. Thus, it is desirable to establish an easy-to-detect polymer-based sensor for amines with drastic responses.
Gold nanoparticles (AuNPs) have been vigorously employed to prepare naked eye recognizable chemosensors.15,17,40–42 The spectral shift of AuNPs is induced by a change in localized surface plasmon resonance (LSPR) derived from aggregation and dispersion of particles43–45 by chemical stimuli.46 The AuNPs can be easily functionalized with molecular recognition moieties, allowing optical sensing of biogenic amines,47–50 airborne pollutants,51 microorganisms,52 organophosphate pesticides,53 and cells.54 The colorimetric chemosensor arrays based on metal nanoparticles for food analysis applied to the qualitative detection and spike tests of various biogenic amines,55,56 while the freshness monitoring of amines in food samples is still challenging. Herein, we report an AuNP-based colorimetric chemosensor array for the spoilage process monitoring of a raw fish (tuna) by the quantitative detection of the biogenic amine. The chemosensors were prepared by AuNP functionalized with carboxylate derivatives (i.e., 4-mercaptobenzoic acid (S1), 6-mercaptohexanoic acid (S2), and 11-mercaptoundecanoic acid (S3)) for the naked-eye detection of 10 amine derivatives containing diamines, aromatic amines, and polyamines (A1–A10). The different types of functional groups were modified not only to detect target amines at a wide range of concentrations but also to obtain a colorimetric fingerprint-like response pattern. For example, the AuNPs functionalized with 4-mercaptobenzoic acid (S1) and 6-mercaptohexanoic acid (S2) are capable of detecting analytes at low concentrations because the short molecular lengths of those functional groups could facilitate the aggregation manner by adding analytes. In addition, the different rigidity of the functional groups between S1 and S2 could offer the variation of response profiles. In contrast, the AuNP functionalized with a longer alkyl chain such as 11-mercaptoundecanoic acid (S3) enables the detection of analytes at high concentrations due to the difficulty of the sensitive aggregation in the presence of analytes, unlike S1 and S2.57 The AuNPs were aggregated by the addition of the target amines, resulting in drastic color changes (Fig. 1). The qualitative and quantitative detection was processed by the chemometric methods, including a linear discriminant analysis (LDA)25,27 and a supporting vector machine (SVM).31,33 It is worth mentioning that our chemosensor array demonstrated highly accurate determination of histamine concentrations in the raw fish, the result of which was matched with HPLC. Moreover, the chemosensor array successfully monitored time-dependent concentration changes of extracted histamine from the fish stored for 24 h, 48 h, and 72 h at 25 °C, indicating its applicability for a freshness sensor. Thus, the proposed chemosensor array would contribute a one-step-forward for the amine analyses based on nanoparticle technology.
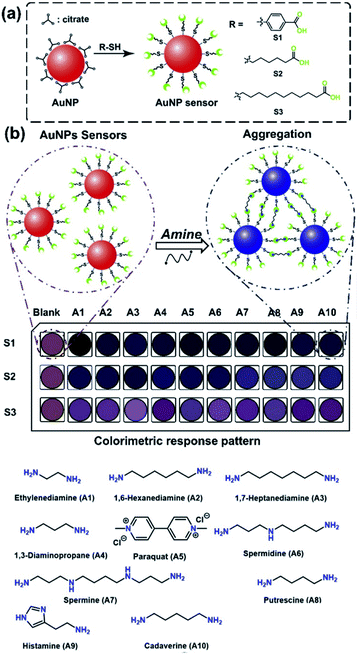 |
| Fig. 1 (a) Schematic structure of the nanoparticle sensors (S1–S3). (b) The feasible colorimetric detection mechanism for the target 10 amine derivatives (A1–A10) utilizing AuNPs functionalized with carboxylate derivatives. | |
2. Experimental
2.1. Materials
Chloroauric acid (HAuCl4, 40–44%) was purchased from Alfa Reagent, Ltd. Histamine (A9, 97%), 4-mercaptobenzoic acid (95%), 6-mercaptohexanoic acid (90%), 11-mercaptoundecanoic acid (98%), and 1,7-heptanediamine (A3, 98%) were purchased from Sigma-Aldrich, Ltd. Trisodium citrate dehydrate (Na3Ct, 98%), methylamine hydrochloride (A11, 99%), dimethylamine hydrochloride (A12, 99%), trimethylamine hydrochloride (A13, 98%), ethylamine hydrochloride (A14, 98%), isopropylamine (A15, 99%), isobutylamine (A16, 98%), hexylamine (A17, 99%), 2-(2-aminoethoxy)ethanol (A18, 98%), trans-4-(aminomethyl)cyclohexanecarboxylic acid (A19, 98%), 2,2-iminodiethanol (A20, 99%), 1-adamantylamine (A21, 98%), 2-(4-hydroxyphenyl)ethylamine (A22, 98%), 1,4-toluidine (A23, 99%), 4-aminophenol (A24, 98%), 4-(aminomethyl)benzoic acid (A25, 98%), 1,4-anisidine (A26, 99%), 1,6-hexanediamine (A2, 99%), trans-1,4-diaminocyclohexane (A27, 98%), 1,3-diaminopropane (A4, 99%), 1,2-phenylenediamine (A28, 99%), N,N,N,N′,N′-pentamethyldiethylenetriamine (A29, 98%), 4-aminoantipyrine (A30, 99%),ornithine monohydrochloride (A31, 98%), methionine (A32, 99%), lysine (A33, 98%), cystine (A34, 99%), alanine (A35, 99%), glycine (A36, 99%), phenylalanine (A37, 99%), histidine (A38, 98%), putrescine (A8, 99%), cadaverine (A10, 98%), trichloroacetic acid (99%), dansyl chloride (98%), proline (99%), hydrochloric acid (37%), sodium hydroxide (96%), n-hexane (99%), methanol (99.9%), acetonitrile (99.9%), ethanol (99.7%), acetone (99.5%), tetrahydrofuran (THF, 99.5%), sodium bicarbonate (99.5%), 2-morpholinoethanesulfonic acid (MES, 99%), sodium carbonate (99.5%), and chloroform (98%) were purchased from Adamas Reagent, Ltd. Ethylenediamine dihydrochloride (A1, 98%), paraquat (A5, 98%), spermidine (A6, 99%) and spermine (A7, 97%) were purchased from Acros Reagent, Ltd. A Milli-Q water system (18.2 MΩ cm) was utilized for preparation of aqueous solutions.
2.2. Measurement
Fourier transform infrared (FT-IR) spectra were obtained using a Thermo Nexus 470 FT-IR instrument by grinding and tableting the sample with KBr. Thermogravimetric analysis was performed using a thermogravimetric analyzer (TGA, TA Q500, TA Instruments) under N2 with a temperature ramp rate of 10 °C min−1 from 30 °C to 800 °C. The microphotographs of the materials were observed through field emission scanning electron microscopy (FE-SEM, S-4800, HITACHI), high angle annular dark-field transmission electron microscopy (HAADF-STEM JEM-2100F, JEOL) with energy dispersive X-ray analysis (EDX). The zeta potential and particle size of the samples were measured by a Zeta-sizer instrument (3000HS, Malvern Ltd., UK). The elemental analyses were carried out by X-ray photoelectron spectroscopy (XPS, ESCALAB 250Xi). Extinction spectra were obtained using a Shimadzu UV 3600 spectrophotometer. The concentration of biogenic amines extracted from a raw fish (i.e., tuna) was determined by HPLC (Agilent 1260 series, Agilent Ltd.). The pH values of the solutions were measured by a pH meter (model: PHS-3E, Inesa scientific instrument Co., Ltd).
2.3. Preparation of gold nanoparticles
An aqueous solution containing Na3Ct (0.65 mmol, 2 mL) was gently added into an aqueous solution of HAuCl4 (0.25 mmol, 98 mL) with stirring. The mixture was further vigorously stirred at 100 °C for 15 min to obtain the directly synthesized gold nanoparticles (DS-AuNPs) with deep red wine color. On the other hand, the inversely synthesized gold nanoparticles (IS-AuNPs) were obtained by the following procedure. The aqueous solution of Na3Ct (0.65 mmol, 99 mL) was kept at 100 °C for 15 min. Then, the aqueous solution of HAuCl4 (0.25 mmol, 1 mL) was added to the above solution with vigorous stirring. After the above reactions, both solutions of DS-AuNPs and IS-AuNPs were cooled down to room temperature. The solutions were filtrated (a membrane filter: Spritzen-Syringe Filter, membrane: PES, pore size: 0.22 μm), and stored at 4 °C until the next step synthesis.
2.4. Synthesis of AuNP-based chemosensors (S1–S3)
The preparation conditions of the AuNPs were selected according to a previous report.58 The obtained IS-AuNPs solution (40 mL) and a phosphate buffer solution (0.01 M, pH 7.0, 40 mL) were mixed and gently stirred under an inert atmosphere. To obtain the functionalized AuNPs (S1, S2, and S3), each ethanol solution (1.0 mL) containing the functional group (4-mercaptobenzoic acid, 6-mercaptohexanoic acid, or 11-mercaptoundecanoic acid) (0.1 mmol) were added to the above mixtures and stirred for 4 h. After this period, the reaction solutions were filtrated using the membrane filter. The products were precipitated by adding acetone and THF, followed by centrifugation at 11
000 rpm. The precipitates were re-dispersed in a MES buffer solution (0.1 M at pH 7.0) and stored at 4 °C.
2.5. HPLC analysis
The target amine in tuna stored for 24 h, 48 h, and 72 h at 25 °C was extracted according to reported procedures.59,60 The fish meat (10 g) was added into trichloroacetic acid (6% (w/v), 25 mL) and stirred for 60 min. Next, the mixture was centrifuged to remove the insoluble matter. The mixture was centrifuged at 6500 rpm for 5 min. The biogenic amine in the obtained supernatant was extracted by n-hexane (10 mL) and vortexed for 5 min. Finally, the organic layer was collected and filtrated using the membrane filter. The extracted amine in the solution was evaluated by HPLC and the chemosensor array. The pH values of the standard amine solutions (1.0 mL) were adjusted to pH 11 by hydrochloric acid (0.1 M) and diluted with a carbonate buffer solution (0.5 M, 1.5 mL) at pH 11 at 25 °C. The standard amine solutions and the extracted amine solutions were preprocessed as follows: (1) a chloroform solution of dansyl chloride (37 mM, 1.0 mL) was added to the above solutions and heated at 45 °C for 1 h under dark conditions. (2) An aqueous solution of proline (0.43 M, 0.4 mL) was added to the mixtures and shaken for 1 h. (3) the mixtures were further extracted by n-hexane (2.0 mL) with shaking for 5 min. The above-mentioned preprocessing was applied to each sample of standard solutions and the extracted solutions, respectively. The final extracted n-hexane solutions (1.0 mL) were dried at 40 °C using a nitrogen blowing concentrator. The residue was dissolved in methanol (1.0 mL), followed by filtration using the membrane filter. The HPLC analysis was performed for the methanol solutions (20 μL) by an Agilent 1260 with a reverse-phase silica gel column (Agilent 959961-902 Zorbax Eclipse Plus C18, 100 × 4.6 mm ID, participle size: 3.5 μm) at 35 °C. The elution was carried out at a flow rate of 0.8 mL min−1 with a gradient mobile phase consisting of acetonitrile and water (Table S4†). The eluted and labelled amines were monitored by a UV detector (λ = 254 nm).
2.6. Data analysis
The inset data for pattern recognition were constructed by using extinction spectra of S1–S3 recorded from 400 nm to 800 nm. The acquired dataset was pre-processed by the Student's t-test, and 4 outlier data points were thus excluded from data points of 24 repetitions. The estimated coefficient of variation among 20 repetitions was lower than 7%. The pre-treated data was applied to the qualitative and semi-quantitative analyses by the linear discriminant analysis (LDA) with a leave-one-out cross-validation protocol (i.e., jackknife method) using SYSTAT13 (Systat Software Inc.). The support vector machine (SVM) with a principal component analysis and an auto-scale pre-processing was carried out using Solo (Eigenvector) for the quantitative assay and the freshness monitoring of the raw fish.
3. Results and discussion
We prepared the AuNPs by direct/inverse synthetic methods based on the reduction of HAuCl4 with citrate (i.e., the different adding order of citrate and HAuCl4).61 In contrast to the DS-AuNPs, the IS-AuNPs resulted in less time-dependent changes of the extinction spectra, which indicated the successful synthesis of the stable IS-AuNPs. In addition, the slight spectral shifts of IS-AuNPs in extinction spectra were observed by the surface functionalization with carboxylate derivatives (S1–S3) (λmax = 525 nm for S1, 524 nm for S2, and 532 nm for S3, respectively) (Fig. S3, ESI†), the deep reddish color of which could be used for colorimetric sensors. Moreover, absorption peaks at 2558 cm−1 and 2670 cm−1 originating from the S–H stretching vibration band in FT-IR spectra disappeared because of the functionalization of the gold surface (Fig. S4, ESI†). Moreover, changes of the weight loss rate (5–10 wt%) in the TGA results of elemental analyses (i.e., XPS) and HAADF-STEM with EDX suggested the successful attachment of the carboxylate derivatives onto the surface of IS-AuNPs (Fig. S5–S7, ESI†). Furthermore, the diameters of nanoparticles were determined by dynamic light scattering (DLS) (Fig. S8, ESI†) and TEM (9.6 ± 1.1 nm, 9.9 ± 1.0 nm, and 9.6 ± 1.0 nm for S1, S2, and S3 (Fig. S9, ESI†)), respectively. The negative ζ-potentials of S1, S2, and S3 also suggested that the carboxylate derivatives were successfully attached to the gold surface (Fig. S10, ESI†).
Next, we evaluated the optical changes of the AuNP-based chemosensors by chemical stimuli (i.e., pH change) in an aqueous solution (Fig. S11 and S12, ESI†). For example, Fig. S11† shows a drastic color change of S2 at the pH range between 4 and 5, which matched with the pKa value of the carboxy group (pKa = 4.8).62–64 Indeed, the ζ-potential of S2 exhibited a gradual decrease with the increase of the pH value due to deprotonation of the carboxy group, which indicated that the nanoparticle with the negative ζ-potential (ca. −30 mV) could be highly dispersed over pH 5. Because the amine protonation occurs under weak-acidic conditions (e.g., histamine: pKa1 = 9.8, pKa2 = 6.0),65 we selected pH 5.5 for sensing applications.
The colorimetric amine detection relied on the aggregation of S1–S3 with amines, thus the aggregation manners of the AuNPs were investigate. For example, the DLS result indicated that the aggregate diameter of S2 with histamine had ca. 43 times enlargement (Fig. 2(a)), which was also supported by TEM (Fig. 2(b)) and field emission scanning electron microscopy (FE-SEM) (Fig. 2(c)). Besides, the ζ-potential of S2 in the presence of amines at pH 5.5 showed nearly neutral or positive (Fig. S41, ESI†).66 Moreover, we observed an elemental peak of nitrogen in the EDX profile of the aggregates with histamine (Fig. S42, ESI†). Notably, S1–S3 displayed distinct color patterns by changing various amine concentrations (Fig. 2(d)). As expected, S1 and S2 showed more sensitive colorimetric responses to target amines rather than S3, which indicated that the AuNP with the short functional group was more suitable for designing highly sensitive sensors.
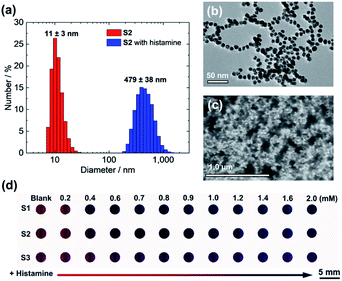 |
| Fig. 2 (a) The histograms of the nanoparticle diameter measured from DLS for S2 (red) and with histamine (blue). [Histamine] = 3.0 mM. (b) TEM image of S2. (c) FE-SEM image of S2 in the presence of histamine. [Histamine] = 3.0 mM. (d) The colorimetric response pattern of the AuNPs with the increase of histamine concentrations. | |
The selectivity of S1–S3 to amines was evaluated by 38 amine and amino acid derivatives (A1–A38). The target amines were selected from the standpoint of the freshness monitoring of fish samples. A1–A4 are interferents to evaluate the freshness of fishes,67 and A6–A10 can be detected in fish products and/or spoiled fish samples. Other amines such as A11–A14 and A16 are also contained in food. In addition, the accumulated A5 in fish samples could cause a potential risk to human body by the ingestion of the contaminated fish samples.68 Thus, A5 was employed to evaluate the diverse detectability of the chemosensors. In this regard, the investigation of the discriminability of S1–S3 based on the difference in the analyte structures (i.e., mono-, di-, and triamines, sterically bulky structures, etc.) was important from the viewpoint of molecular recognition chemists. Therefore, A15 and A17–A30 were selected owing to their unique chemical structures, although these species were not directly related to food analysis. Furthermore, several ubiquitous amino acids (A31–A38) were also used for the test. As a result, A1–A10 caused significant extinction changes, while most of the amine derivatives (A11–A38) showed negligible or slight responses to S1–S3. These weak responses are probably because of the following reasons: (1) monoamines (A11–A26) can only have a single binding site which is hard to induce the aggregation; (2) di- or tri-amines (A27–A30) possessing bulky functional groups are sterically hard to make molecular interactions; (3) the carboxy group in amino acids (A31–A38) induce the electrostatic repulsion between the target and the chemosensors. Given the fact that the low binding affinities to A11–A38 and very low concentrations of A11–A14 and A16 in food (e.g., [A13] < 0.1 mg kg−1),69 we selected A1–A10 as the main target for the qualitative and quantitative assays toward the freshness monitoring of fish samples. Extinction titrations of the amines (A1–A10) were performed in a MES buffer solution (10 mm) at pH 5.5. The AuNPs S1–S3 exhibited significant spectral shifts by the addition of 10 amines (Fig. 3(a) and S14–S33†). Remarkably, the proposed chemosensors exhibited strong responses to polyamines such as spermidine (A6) (Fig. S19, ESI†) and spermine (A7) (Fig. 3a) at micromolar levels. These responses were probably due to the high number of recognition sites, resulting in high sensitivity compared to conventional colorimetric chemosensors.56 As shown in Fig. 3b and S38,† the cross-reactivity of S1–S3 for A1–A10 is suitable to fabricate the chemosensor array for simultaneous recognition of multi-amines.
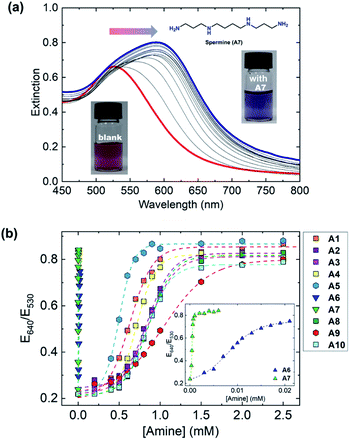 |
| Fig. 3 (a) The changes of extinction spectra of S2 (1.2 × 10−4 g mL−1) upon the addition of spermine (0–10 μM) in a MES buffer solution (10 mM) at pH 5.5 at 25 °C. The extinction spectra of S2 with spermine were recorded after the incubation for 2 min. (b) Cross-reactive response in the extinction ratio (E640 nm/E530 nm) of S2 by the addition of an incremental concentration of amines in a MES buffer solution (10 mM) at pH 5.5 at 25 °C. | |
To highlight the potential of the chemosensors, we decided to fabricate an AuNP-based chemosensor array for simultaneous discrimination of biogenic amines. The chemosensor array was fabricated using a microfluidic robotic dispenser, and the extinction spectra were rapidly recorded using a microplate reader. For pattern recognition, the LDA25,26 was employed to classify multi-amines. The inset data contained the recorded extinction spectra of S1, S2, and S3 in the presence or absence of 10 amines, and then 24 repetitions (including 4 outlier data points) were carried out for each analyte to evaluate the classification accuracy. The LDA result in Fig. 4 revealed that the AuNP-based chemosensor array successfully classified 11 clusters containing 10 amines and a control with the 100% correct classification. Remarkably, the position of each cluster indicates a similarity of amine structures. For example, the polyamine clusters were plotted relatively far from the control, reflecting the sensitive detectability of the chemosensors for polyamines (Fig. 3(b)).
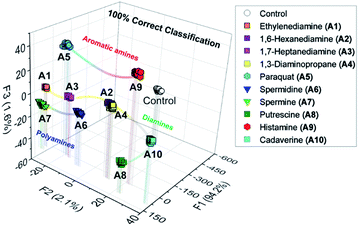 |
| Fig. 4 LDA canonical score plots for the qualitative analysis of 10 amines in a MES buffer solution (10 mM) at pH 5.5 at 25 °C. [S1–S3] = 1.2 × 10−4 g mL−1, [analyte] = 3.0 mM. The measurements of each analyte were replicated 20 times, and the cross-validation routine shows 100% successful classification. | |
Among the biogenic amines, polyamines are found in food, while spermidine and spermine involve in the possibility for carcinogenesis, tumor invasion, and metastasis.70 In addition, histamine is a representative marker for food poisoning and contained in fishes.71–73 Thus, the quantitative and simultaneous analysis for spermine, spermidine, and histamine is important from the standpoint of food analysis and medical fields. Thus, we demonstrated a semi-quantitative assay using the AuNP-based chemosensor array. Fig. 5 displayed a clustering result by the LDA, and indicated the achievement of the accurate concentration-dependent classification. The limit of detection against histamine was estimated to be 7.2 ppm based on the 3σ method,74 which was lower than the defined histamine level by Food and Drug Administration (FDA).75 Thus, the demonstration revealed the potential of the chemosensor array for food analysis.
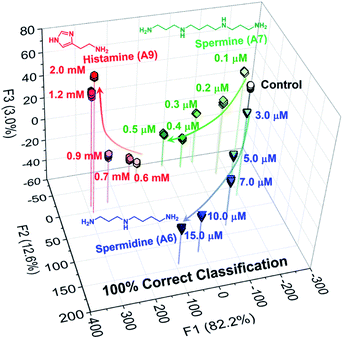 |
| Fig. 5 LDA result of the semi-quantitative assay toward 3 types of biogenic amines (spermidine, spermine, and histamine) in a MES buffer solution (10 mM) at pH 5.5 at 25 °C. In this assay, 20 repetitions were measured for each concentration [S1–S3] = 1.2 × 10−4 g mL−1. | |
To evaluate the quantitative detectability of the chemosensors for 3 amines in the mixtures, we performed the regression analysis using SVM.31,33 The SVM is one of the powerful machine learning algorithms, and the calibration line of which can be built even in mixtures. As shown in Fig. S44,† the chemosensor array with the SVM successfully predicted unknown concentrations of histamine, spermine, and spermidine in the mixtures. Furthermore, a real sample analysis using the raw fish was carried out toward freshness monitoring, the result of which was compared with that of HPLC. In this assay, we attempted to determine time-dependent concentration changes of histamine for freshness monitoring using the chemosensor array. In the spoilage process of a fish sample, the concentration of histamine is time-dependently increased, while the concentrations of spermine and spermidine are not significantly changed.76 Thus, the quantification of histamine in the real fish sample was performed. The target amine was extracted from the raw fish according to reported procedures,59,60 and was analyzed utilizing HPLC (Fig. S46, ESI†) and the chemosensor array. The SVM result indicated the successful determination of the time-dependent concentrations of the extracted amine from each fish stored for 24 h, 48 h, and 72 h by the AuNP-based colorimetric sensing system (Fig. 6). Notably, the accuracy of the AuNP-based chemosensor array was comparable to HPLC, achieving freshness monitoring (Table S5†).
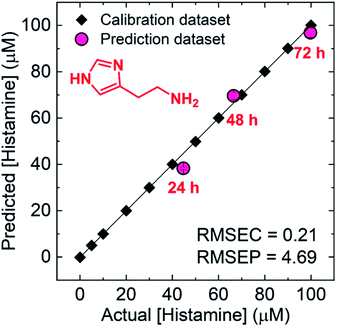 |
| Fig. 6 Real sample analysis using SVM for freshness monitoring of the raw fish [S1–S3] = 1.2 × 10−4 g mL−1. The target histamine was extracted from the raw fish (tuna) stored for 24 h, 48 h, and 72 h at 25 °C. The values of the root-mean-square of calibration (RMSEC) and prediction (RMSEP) prove the high accuracy of the model and its predictive capacity. The actual concentrations of histamine in the fish sample were determined by HPLC. | |
4. Conclusions
In this study, we designed 3 types of AuNP-based chemosensors functionalized with carboxylate derivatives for the qualitative and quantitative analysis of amines. The different lengths of functional groups on the AuNPs contributed to provide a cross-reactive response pattern derived from the various aggregation manners by adding amines. In addition, the visible multi-responses of the chemosensors reflected a difference in both amine structures and their concentrations. The AuNP-based chemosensor array combined with pattern recognition techniques achieved accurate discrimination of 10 amines and regression analysis of biogenic amines in the mixtures. Most importantly, the chemosensor array successfully determined the concentration of extracted histamine from the raw fish stored for 24 h, 48 h, and 72 h at 25 °C, revealing the potential of the chemosensor array for monitoring of food freshness. The proposed simple analytical method by the naked-eye detection would be a novel analytical tool for the food industry.
Author contributions
LD, Yijia Lao, and PG synthesized the AuNP-based chemosensors and performed characterization, qualitative assay, and real sample analysis. This manuscript was written by YS, XL, and SW. TM and Yuanli Liu conceived the entire project. LD and Yijia Lao contributed equally to this work.
Conflicts of interest
There are no conflicts to declare.
Acknowledgements
TM thanks the Japan Society for the Promotion of Science (JSPS KAKENHI Grant No. JP20K21204 and JP21H01780) and JST CREST (Grant No. JPMJCR2011). This work was also financially supported by the National Natural Science Foundation of China (51603050 and 51863006), the Natural Science Foundation of Guangxi (2016GXNSFBA380196 and 2016GXNSFBA380064), and the Foundation of Guilin University of Technology (Grant No. GUTQDJJ2017108).
References
- M. Á. Medina, J. L. Urdiales, C. Rodríguez-Caso, F. J. Ramírez and F. Sánchez-Jiménez, Crit. Rev. Biochem. Mol. Biol., 2003, 38, 23–59 CrossRef CAS PubMed.
- A. R. Shalaby, Int. Food Res. J., 1996, 29, 675–690 CrossRef CAS.
- G.-C. Yen and C.-L. Hsieh, J. Food Sci., 1991, 56, 158–160 CrossRef CAS.
- F. Özogul, K. D. A. Taylor, P. Quantick and Y. Özogul, Int. Food Res. J., 2002, 37, 515–522 Search PubMed.
- N. M. Tamim, L. W. Bennett, T. A. Shellem and J. A. Doerr, J. Agric. Food Chem., 2002, 50, 5012–5015 CrossRef CAS PubMed.
- B. Wzorek, P. Mochalski, I. Sliwka and A. Amann, J. Breath Res., 2010, 4, 026002 CrossRef PubMed.
- Z. Wang, F. Liu and C. Lu, Biosens. Bioelectron., 2014, 60, 237–243 CrossRef CAS PubMed.
- E. Omanovic-Miklicanin and S. Valzacchi, Food Chem., 2017, 235, 98–103 CrossRef CAS PubMed.
- Z. Wu, E. Xu, A. Jiao, Z. Jin and J. Irudayaraj, RSC Adv., 2017, 7, 44933–44944 RSC.
- S. Zhao, Y. Huang, M. Shi and Y.-M. Liu, J. Chromatogr. A, 2009, 1216, 5155–5159 CrossRef CAS PubMed.
- R. Draisci, G. Volpe, L. Lucentini, A. Cecilia, R. Federico and G. Palleschi, Food Chem., 1998, 62, 225–232 CrossRef CAS.
- Y. Li, C.-H. Hsieh, C.-W. Lai, Y.-F. Chang, H.-Y. Chan, C.-F. Tsai, J.-a. A. Ho and L.-c. Wu, Biosens. Bioelectron., 2017, 87, 142–149 CrossRef CAS PubMed.
- J. Dai, Y. Zhang, M. Pan, L. Kong and S. Wang, J. Agric. Food Chem., 2014, 62, 5269–5274 CrossRef CAS PubMed.
- A. Önal, Food Chem., 2007, 103, 1475–1486 CrossRef.
- U. H. F. Bunz and V. M. Rotello, Angew. Chem., Int. Ed., 2010, 49, 3268–3279 CrossRef CAS PubMed.
- H. N. Kim, Z. Guo, W. Zhu, J. Yoon and H. Tian, Chem. Soc. Rev., 2011, 40, 79–93 RSC.
- K. Saha, S. S. Agasti, C. Kim, X. Li and V. M. Rotello, Chem. Rev., 2012, 112, 2739–2779 CrossRef CAS PubMed.
- D. Wu, A. C. Sedgwick, T. Gunnlaugsson, E. U. Akkaya, J. Yoon and T. D. James, Chem. Soc. Rev., 2017, 46, 7105–7123 RSC.
- M. Nakamura, T. Sanji and M. Tanaka, Chem.–Eur. J., 2011, 17, 5344–5349 CrossRef CAS PubMed.
- J. L. Pablos, S. Vallejos, A. Muñoz, M. J. Rojo, F. Serna, F. C. García and J. M. García, Chem.–Eur. J., 2015, 21, 8733–8736 CrossRef CAS PubMed.
- J. Han, Y. Li, J. Yuan, Z. Li, R. Zhao, T. Han and T. Han, Sens. Actuators, B, 2018, 258, 373–380 CrossRef CAS.
- S. Körsten and G. J. Mohr, Chem.–Eur. J., 2011, 17, 969–975 CrossRef PubMed.
- B. Lee, R. Scopelliti and K. Severin, Chem. Commun., 2011, 47, 9639–9641 RSC.
- N. Kaur, S. Chopra, G. Singh, P. Raj, A. Bhasin, S. K. Sahoo, A. Kuwar and N. Singh, J. Mater. Chem. B, 2018, 6, 4872–4902 RSC.
- P. Anzenbacher Jr, P. Lubal, P. Buček, M. A. Palacios and M. E. Kozelkova, Chem. Soc. Rev., 2010, 39, 3954–3979 RSC.
- M. Kitamura, S. H. Shabbir and E. V. Anslyn, J. Org. Chem., 2009, 74, 4479–4489 CrossRef CAS PubMed.
- Z. Li, J. R. Askim and K. S. Suslick, Chem. Rev., 2019, 119, 231–292 CrossRef CAS PubMed.
- H. Singh, G. Singh, N. Kaur and N. Singh, Biosens. Bioelectron., 2022, 196, 113687 CrossRef CAS PubMed.
- K. L. Diehl and E. V. Anslyn, Chem. Soc. Rev., 2013, 42, 8596–8611 RSC.
- D. Lucas, T. Minami, G. Iannuzzi, L. Cao, J. B. Wittenberg, P. Anzenbacher Jr and L. Isaacs, J. Am. Chem. Soc., 2011, 133, 17966–17976 CrossRef CAS PubMed.
- T. Minami, N. A. Esipenko, B. Zhang, M. E. Kozelkova, L. Isaacs, R. Nishiyabu, Y. Kubo and P. Anzenbacher Jr, J. Am. Chem. Soc., 2012, 134, 20021–20024 CrossRef CAS PubMed.
- T. Minami, N. A. Esipenko, B. Zhang, L. Isaacs and P. Anzenbacher Jr, Chem. Commun., 2014, 50, 61–63 RSC.
- T. Minami, N. A. Esipenko, A. Akdeniz, B. Zhang, L. Isaacs and P. Anzenbacher Jr, J. Am. Chem. Soc., 2013, 135, 15238–15243 CrossRef CAS PubMed.
- H. A. Ho and M. Leclerc, J. Am. Chem. Soc., 2003, 125, 4412–4413 CrossRef CAS PubMed.
- H. Jiang, P. Taranekar, J. R. Reynolds and K. S. Schanze, Angew. Chem., Int. Ed., 2009, 48, 4300–4316 CrossRef CAS PubMed.
- C. Li, M. Numata, M. Takeuchi and S. Shinkai, Angew. Chem., Int. Ed., 2005, 44, 6371–6374 CrossRef CAS PubMed.
- M. S. Maynor, T. L. Nelson, C. O'Sulliva and J. J. Lavigne, Org. Lett., 2007, 9, 3217–3220 CrossRef CAS PubMed.
- T. L. Nelson, C. O'Sullivan, N. T. Greene, M. S. Maynor and J. J. Lavigne, J. Am. Chem. Soc., 2006, 128, 5640–5641 CrossRef CAS PubMed.
- T. L. Nelson, I. Tran, T. G. Ingallinera, M. S. Maynor and J. J. Lavigne, Analyst, 2007, 132, 1024–1030 RSC.
- L. K. Bogart, G. Pourroy, C. J. Murphy, V. Puntes, T. Pellegrino, D. Rosenblum, D. Peer and R. Lévy, ACS Nano, 2014, 8, 3107–3122 CrossRef CAS PubMed.
- X. Wei, Y. Wang, Y. Zhao and Z. Chen, Biosens. Bioelectron., 2017, 97, 332–337 CrossRef CAS PubMed.
- P. C. Huang, N. Gao, J. F. Li and F. Y. Wu, Sens. Actuators, B, 2018, 255, 2779–2784 CrossRef CAS.
- N. R. Jana, L. Gearheart and C. J. Murphy, Langmuir, 2001, 17, 6782–6786 CrossRef CAS.
- T. Kim, C.-H. Lee, S.-W. Joo and K. Lee, J. Colloid Interface Sci., 2008, 318, 238–243 CrossRef CAS PubMed.
- Z. Wang and L. Ma, Coord. Chem. Rev., 2009, 253, 1607–1618 CrossRef CAS.
- T. Minami, K. Kaneko, T. Nagasaki and Y. Kubo, Tetrahedron Lett., 2008, 49, 432–436 CrossRef CAS.
- K. M. A. El-Nour, E. T. A. Salam, H. M. Soliman and A. S. Orabi, Nanoscale Res. Lett., 2017, 12, 231 CrossRef CAS PubMed.
- C.-F. Chow, Food Chem., 2020, 311, 125908 CrossRef CAS PubMed.
- H. Kim, B. T. Trinh, K. H. Kim, J. Moon, H. Kang, K. Jo, R. Akter, J. Jeong, E.-K. Lim, J. Jung, H.-S. Choi, H. G. Park, O. S. Kwon, I. Yoon and T. Kang, Biosens. Bioelectron., 2021, 179, 113063 CrossRef CAS PubMed.
- S. Chopra, J. Singh, H. Kaur, N. Singh and N. Kaur, Sens. Actuators, B, 2015, 220, 295–301 CrossRef CAS.
- Z. Li, Z. Wang, J. Khan, M. K. LaGasse and K. S. Suslick, ACS Sens., 2020, 5, 2783–2791 CrossRef CAS PubMed.
- B. Li, X. Li, Y. Dong, B. Wang, D. Li, Y. Shi and Y. Wu, Anal. Chem., 2017, 89, 10639–10643 CrossRef CAS PubMed.
- N. Fahimi-Kashani and M. R. Hormozi-Nezhad, Anal. Chem., 2016, 88, 8099–8106 CrossRef CAS PubMed.
- S. Rana, A. K. Singla, A. Bajaj, S. G. Elci, O. R. Miranda, R. Mout, B. Yan, F. R. Jirik and V. M. Rotello, ACS Nano, 2012, 6, 8233–8240 CrossRef CAS PubMed.
- X. Zhong, D. Huo, H. Fa, X. Luo, Y. Wang, Y. Zhao and C. Hou, Sens. Actuators, B, 2018, 274, 464–471 CrossRef CAS.
- A. Orouji, F. Ghasemi, A. Bigdeli and M. R. Hormozi-Nezhad, ACS Appl. Mater. Interfaces, 2021, 13, 20865–20874 CrossRef CAS PubMed.
- B. L. V. Prasad, S. I. Stoeva, C. M. Sorensen and K. J. Klabunde, Langmuir, 2002, 18, 7515–7520 CrossRef CAS.
- N. R. Nirala, P. S. Saxena and A. Srivastava, Spectrochim. Acta, Part A, 2018, 190, 506–512 CrossRef CAS PubMed.
- H.-F. Kung, L.-T. Chien, H.-J. Liao, C.-S. Lin, E.-T. Liaw, W.-C. Chen and Y.-H. Tsai, Food Chem., 2008, 110, 480–485 CrossRef CAS PubMed.
- A. Naila, S. Flint, G. C. Fletcher, P. J. Bremer and G. Meerdink, Food Chem., 2011, 128, 479–484 CrossRef CAS PubMed.
- I. Ojea-Jiménez, N. G. Bastús and V. Puntes, J. Phys. Chem. C, 2011, 115, 15752–15757 CrossRef.
- H. T. Phan and A. J. Haes, J. Phys. Chem. C, 2018, 122, 14846–14856 CrossRef CAS.
- T. C. Preston, M. Nuruzzaman, N. D. Jones and S. Mittler, J. Phys. Chem. C, 2009, 113, 14236–14244 CrossRef CAS.
- S. M. Ansar, S. Chakraborty and C. L. Kitchens, Nanomaterials, 2018, 8, 339 CrossRef PubMed.
- H. A. De Abreu, W. B. De Almeida and H. A. Duarte, Chem. Phys. Lett., 2004, 383, 47–52 CrossRef CAS.
- X. Zhang, X. Fan, Y. Wang, F. Lei, L. Li, J. Liu and P. Wu, Anal. Chem., 2020, 92, 1455–1462 CrossRef CAS PubMed.
- X. Qi, W.-F. Wang, J. Wang, J.-L. Yang and Y.-P. Shi, Food Chem., 2018, 259, 245–250 CrossRef CAS PubMed.
- T. O. Ikpesu, Environ. Sci. Pollut. Res., 2015, 22, 8517–8525 CrossRef CAS PubMed.
- Z. Yi and J. Xie, Foods, 2021, 10, 2132 CrossRef CAS PubMed.
- B. del Rio, B. Redruello, D. M. Linares, V. Ladero, P. Ruas-Madiedo, M. Fernandez, M. C. Martin and M. A. Alvarez, Food Chem., 2018, 269, 321–326 CrossRef CAS PubMed.
- J. Rosier and C. Van Peteghem, Z. Lebensm.-Unters. Forsch., 1988, 186, 25–28 CrossRef CAS PubMed.
- N. G. Sanceda, E. Suzuki, M. Ohashi and T. Kurata, J. Agric. Food Chem., 1999, 47, 3596–3600 CrossRef CAS PubMed.
- L. Luo, J.-Y. Yang, Z.-L. Xiao, D.-P. Zeng, Y.-J. Li, Y.-D. Shen, Y.-M. Sun, H.-T. Lei, H. Wang and Z.-L. Xu, RSC Adv., 2015, 5, 78833–78840 RSC.
- J. N. Miller and J. C. Miller, Statistics and Chemometrics for Analytical Chemistry, Pearson, Prentice Hall, 2005 Search PubMed.
- I. A. Bulushi, S. Poole, H. C. Deeth and G. A. Dykes, Crit. Rev. Food Sci. Nutr., 2009, 49, 369–377 CrossRef PubMed.
- M. Křížek, F. Vácha, L. Vorlová, J. Lukášová and Š. Cupáková, Food Chem., 2004, 88, 185–191 CrossRef.
Footnotes |
† Electronic supplementary information (ESI) available: Characterization of AuNPs and AuNP-based chemosensors, results of extinction titrations, array experiments and raw fish analysis. See DOI: 10.1039/d2ra00160h |
‡ L. Du and Y. Lao contributed equally to this article. |
|
This journal is © The Royal Society of Chemistry 2022 |