DOI:
10.1039/D1RA07447D
(Paper)
RSC Adv., 2022,
12, 2741-2750
Molecular dynamics simulations and MM-GBSA reveal novel guanosine derivatives against SARS-CoV-2 RNA dependent RNA polymerase
Received
7th October 2021
, Accepted 6th December 2021
First published on 20th January 2022
Abstract
According to the World Health Organization (WHO), SARS-CoV-2 is responsible for more than 5 M deaths and is reported in 223 countries infecting 250+ M people. Despite the current vaccination momentum, thousands of people die every day by COVID-19. Suggesting possible blockers of the viral RNA-dependent RNA polymerase is highly needed for potential effective therapeutics against SARS-CoV-2. This study utilizes combined molecular dynamics simulation and molecular docking to test novel guanosine derivatives against SARS-CoV-2 RdRp. Results reveal the binding potency of nineteen guanosine derivatives against SARS-CoV-2 solved structures. The bulky moieties (hydroxyl or fluorated phenyl moieties) added to the 2′ position of the ribose ring positively impacted the binding affinity to RdRp. The current in silico study represents a one-step-ahead for suggesting new possible blockers of SARS-CoV-2 RdRp that are yet to be verified in the wet lab. It offers new potential binders or blockers of RdRp that bind to the protein active site tighter than remdesivir. The latter was approved by the food and drug administration (FDA) for emergency use against COVID-19 last year.
Introduction
The viral pandemic Severe Acute Respiratory Syndrome Coronavirus 2 (SARS-CoV-2) has killed over 5 million people in 223 countries since it was first reported in Wuhan, China, in December 2019. According to the WHO website, the infection and death numbers are continuing to grow (https://www.who.int/emergencies/diseases/novel-coronavirus-2019, accessed 12 November 2021).1,2 The SARS-CoV-2 virus is a new strain of betacoronaviruses that causes the deadly COVID-19 disease. Betacoronaviruses are a family of coronaviruses (crown-like virus) that can cause respiratory and gastrointestinal illnesses in humans and animals, unlike gammacoronaviruses and deltacoronaviruses, which infect birds and possibly mammals but have never been linked to human illness.3 SARS-CoV (2003) and the Middle East Respiratory Syndrome coronavirus (MERS-CoV) (2012) are two other betacoronaviruses that were reported to cause human epidemic diseases in the past two decades.1,4–6
The SARS-CoV-2 genome contains two large genes, ORF1a and ORF1b, which code for 16 non-structural proteins (NSP1–NSP16), including NSP12, which codes for RNA-dependent RNA polymerase (RdRp).4 RdRp is a crucial enzyme for RNA viruses, including the human coronaviruses. It promotes viral gene transcription and replication in collaboration with other viral (nsp7 and nsp8) and host-cell factors.7,8 It has no human homolog, so this paves the way for antiviral drug production and lowers the chances of a protein being harmed in human cells.9,10 RdRp was targeted in different RNA viruses such as Hepatitis C Virus (HCV), Ebola Virus (EBOV), Zika Virus (ZIKV), and human coronaviruses (HCoVs).11–17
Many directly acting antiviral drugs are officially approved against different viruses, such as sofosbuvir and ribavirin against RdRp of HCV and remdesivir against EBOV.11,18 These drugs are modified nucleotides that compete with physiological nucleotides for the RdRp active site. Ribavirin is a wide-acting antiviral drug used to combat a wide range of viruses in combination with immunomodulators or other antiviral medications, while remdesivir is a nucleotide analog used against EBOV, Marburg virus, MERS-CoV, and SARS-CoV.19–22 Ribavirin reported a half-maximal Effective Concentration (EC50) against SARS-CoV-2 of 109.5 μM, while remdesivir reported an EC50 of 1.76 μM.23,24 This study focuses on nucleotide inhibitors because of the strong evidence of inhibition against viral RdRps. After Molecular Dynamics Simulation (MDS) for up to 50 ns for each structure, seventy-six guanosine triphosphate derivatives are tested against ten different solved structures of SARS-CoV-2 RdRp. After cluster analysis of the RdRp structures' trajectories, molecular docking is utilized to test the binding affinity and the guanosine derivatives' compulsory mode against SARS-CoV-2 RdRp. Finally, the top hits are subjected to additional 50 ns MDS followed by binding free energy calculations.
Materials and methods
Structural retrieval and preparation
Ten different SARS-CoV-2 RNA-dependent RNA polymerase structures were downloaded from the Protein Data Bank (PDB) database.25 The PDB ID codes for the structures were 6M71, 6XEZ, 6XQB, 6YYT, 7BTF, 7BV1, 7BV2, 7BW4, 7BZF, and 7C2K with resolutions of 2.90, 3.50, 3.40, 2.90, 2.95, 2.80, 2.50, 3.70, 3.26, and 2.93 Å, respectively.26–31 The structures were solved experimentally using the cryo-EM technique, yet they maintained their high resolution. The PyMOL software was utilized to prepare the structures for docking and molecular dynamics simulation studies.32 Water and ligands were removed, while any missing hydrogen atoms were added to the structures. The structures that contain other cofactors were prepared by removing any cofactors or nucleic acids before the simulation studies.
The structures of the ligands used in this study were drawn using SCIGRESS (version FJ 2.9.1) software.33 The ligands were modified guanosine derivatives based on the guanosine triphosphates (GTP) modified at position 2′ while the hydroxyl group was replaced by a bulky group, as shown in Fig. 1. The structures were geometry optimized first using the classical mechanical force field (MM3) then using the semi-empirical parameterization method 6 (PM6) before the docking study.34,35
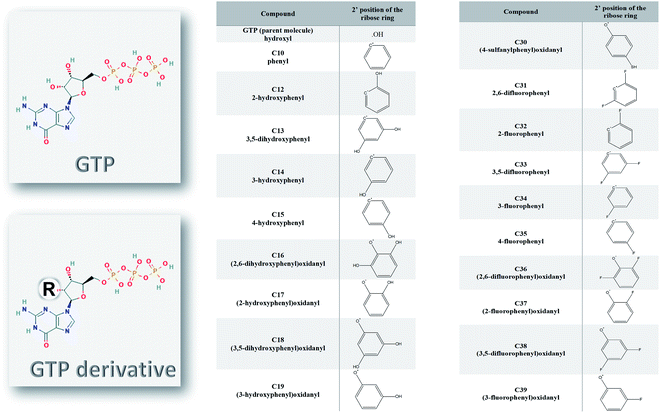 |
| Fig. 1 The guanosine triphosphate (GTP) and its derivative compounds (C10, C12–C19, and C30–C39). The modifications (R) are in the 2′ position of the ribose ring instead of the hydroxyl group in the GTP. | |
Molecular dynamics simulation
The ten SARS-CoV-2 RdRp structures were examined using 50 ns molecular dynamics simulation runs with the help of the Nanoscale Molecular Dynamics (NAMD) 2.14 software (CHARMM 36 force field).36,37 Visualizing Molecular Dynamics (VMD) 1.9.3 software was utilized to build the input files and analyze the trajectories along with some in-house codes.38,39 Before the production run, the structures were solvated using the TIP3P water model, while Na and Cl ions were added to keep the system's charge neutral.40 Additionally, the system was minimized for 10
000 steps using the steepest descent algorithm, followed by gradually increasing the system temperature until the physiological temperature of 310 K at the equilibration step. While the number of atoms, the pressure, and temperature were kept constant (NPT) during the equilibration period (1.1 ns), the volume was adjusted and kept constant (NVT) during the production runs (50 ns).
After MDS, cluster analyses of the trajectories were performed using TTClust software.41 Two clusters were defined for each protein, and a representative conformation was randomly selected from each cluster for the docking study. A total of twenty different conformations of the RdRps were used to test the guanosine derivatives.
Molecular docking
After MDS trajectory clustering, the docking experiment was performed using the twenty structural conformations of SARS-CoV-2 RdRps with the help of AutoDock Vina software.42 Seventy-six guanosine derivatives were tested against the different conformations of the SARS-CoV-2 RdRps. Guanosine triphosphate (GTP), sofosbuvir, ribavirin, and remdesivir were used as positive controls, while cinnamaldehyde and thymoquinone were selected as negative controls.8,15 AutoDock Tools 1.5.6 was utilized to prepare ligands and the different protein conformations (merging Gasteiger charges and generating dockable PDBQT format for molecular docking).43 The receptor grid boxes for the twenty conformations of SARS-CoV-2 RdRp were adjusted to cover the binding site represented by D760 and D761, which were treated as flexible during the docking simulations. The box size was set to 30 × 30 × 30 Å3 centered between the D760 and D761 residues that have different coordinates in each protein conformation. The calculations were done on eight cores, and the default exhaustiveness value of 8 was set in all docking calculations.
After docking, SARS-CoV-2 RdRp structures were mined for the established interactions between ligands and proteins using the Protein–Ligand Interaction Profiler (PLIP) web server (Technical University of Dresden) and then tabulated.44,45
MDS of the best two complexes and free energy calculations
The best two complexes in binding RdRps (C13 and C14) were subjected to 100 ns MDS run following the same protocol using NAMD 2.13 software. This was followed by Molecular Mechanics Generalized Born Surface Area (MM-GBSA) calculation using MMPBSA.py script implemented in amber tools 17.46 All frames (1000) were used in the calculations. The salt concentration was set to 0.154 M, while all other settings were kept as default.
Results and discussion
2′ analog as a potent nucleotide derivative
The nucleotide modified at its 2′ position of the ribose ring was found successful as an inhibitor against different viral polymerases, including Hepatitis C virus (HCV), Zika virus, and human coronaviruses (such as MERS-CoV, SARS-CoV, and SARS-CoV-2).8,12,47,48 Sofosbuvir, for example, was approved by the Food and Drugs Administration (FDA) against HCV NS5B RdRp eight years ago.15,49,50 In addition, it showed excellent in silico binding affinity against other viruses and pathogenic fungi.17,51–53 In Fig. 1, the guanosine derivatives giving good binding energies are listed with the modifications added at the ribose 2′ position instead of the hydroxyl group in the parent compound Guanosine Triphosphate (GTP). The added bulky groups (phenyl or OH, SH, F substituted phenyl moieties) will interfere with RNA recognition after the addition of the guanosine derivative to the primer RNA at the viral RdRp active site.
Molecular dynamics simulation of SARS-CoV-2 structures
The ten RdRp solved structures are equilibrated for 50 ns MDS as shown from the average Root Mean Square Deviation (RMSD) curve of Fig. 2A. The blue curve is the average over the ten RMSD curves for individual protein structure versus time, while the error bars represent the standard deviation values. The average RMSD reflects that the protein systems are equilibrated during the first 30 ns, and the average RMSD curve reaches a saturation value of 2.75 Å. The protein systems are also stable during the dynamics simulation as deduced from the average per-residue Root Mean Square Fluctuations (RMSF) curve in Fig. 2B. Again, the blue curve is the average RMSF, while the error bars are the standard deviation values. The majority of the protein residues are stable during the dynamic's simulation except for the highly fluctuating N and C terminals (about 50 residues at the beginning and 50 at the end of the RMSF curve) which reach up to <5 Å. The active site residues D760 and D761 exhibit minor fluctuations (average RMSF up to 1.13 Å), while the regions F334–V338 (red), V359–S364 (yellow), Q822–D825 (blue), and D846–M855 (magenta) show moderate average RMSF values (<2 Å). These four regions are apart from the active site D760 & D761 (black sticks) and represent movable turns and loops as shown in the structure of SARS-CoV-2 RdRp (PDB ID: 6XEZ) in Fig. 2B.
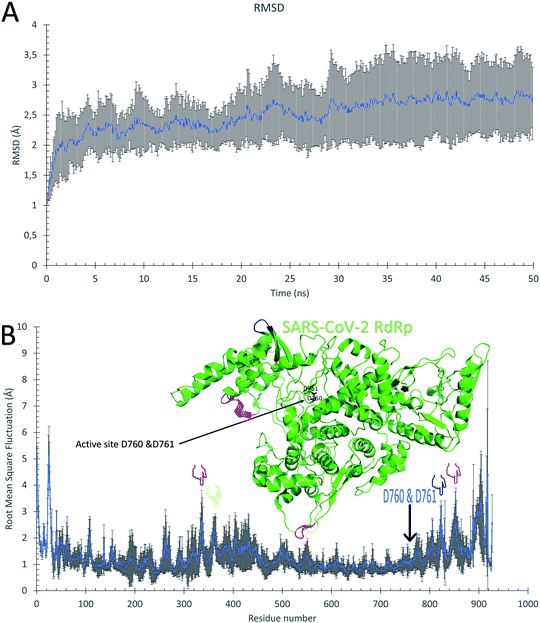 |
| Fig. 2 Molecular dynamics simulation of the SARS-CoV-2 RdRp structures. (A) The average root mean square deviations (RMSD) in Å versus simulation time in ns. (B) The average per-residue root mean square fluctuations (RMSF) in Å. The error bars are the standard deviations. The structure of the RdRp (PDB ID: 6XEZ) is shown in the green cartoon, while the highly fluctuating regions F334–V338, V359–S364, Q822–D825, and D846–M855 are in red, yellow, blue, and magenta cartoons, respectively. The four regions are also shown in the RMSF by colored hands. The active site residues are shown in the structure with black sticks and also by an arrow in the RMSF. | |
Binding affinities of the guanosine derivatives against SARS-CoV-2 RdRps
The 50 ns trajectories of the ten RdRps are subjected to cluster analysis using TTClust, and two clusters are defined for each structure. The binding affinities of the GTP and its 76 derivatives against SARS-CoV-2 RdRps conformations are calculated using the AutoDock Vina software. Fig. 3 shows the average binding affinities for the best 19 derivatives in blue alongside the GTP in green, the positive controls (sofosbuvir, remdesivir, and ribavirin) in yellow, and the negative controls (cinnamaldehyde and thymoquinone) in red. The colored bars represent the average values (in kcal mol−1), while the error bars represent the standard deviations. One can notice that the guanosine derivatives have average binding affinities comparable to that of the approved drug remdesivir (−7.70 ± 0.58 kcal mol−1) and to those of the other positive controls sofosbuvir (−7.55 ± 0.43 kcal mol−1) and ribavirin (−7.35 ± 0.48 kcal mol−1). This indicates that these guanosine derivatives could be successful therapeutic agents against SARS-CoV-2 RdRp as its binding is better than or equal to the approved drug remdesivir. We discussed in a previous study the appropriate use of anti-HCV, anti-HIV, and anti-EBOV drugs against SARS-CoV-2 RdRp, but our study was based on in silico built models of the RdRp.9,10 Fortunately, the current research reproduced almost the same binding affinity for remdesivir and sofosbuvir using ten different solved structures of the RdRp. This indicates the effectiveness of our previous model of RdRp to test anti-COVID-19 therapeutics. Additionally, we compared the remdesivir and sofosbuvir against RdRp of SARS-CoV-2 in an earlier study, and it gave the same binding affinity to the current research.8 Therefore, even the interacting residues are conserved in the case of SARS-CoV-2 RdRp against remdesivir and sofosbuvir.
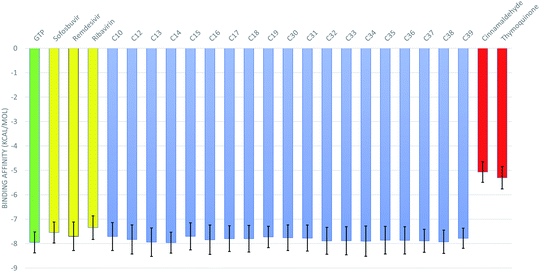 |
| Fig. 3 The binding affinity of the guanosine derivatives (blue), positive control drugs sofosbuvir, remdesivir, ribavirin (yellow), the physiological guanosine triphosphate (green), and the negative control compounds cinnamaldehyde and thymoquinone (red). Error bars represent the standard deviation. | |
As shown in Table 1, the nineteen guanosine derivatives successfully establish strong contacts with the active site of the RdRp of SARS-CoV-2. Hydrogen bonds or salt bridges' formation is the primary type of interaction found between the protein and the guanosine derivatives, with few hydrophobic contacts as a secondary type of interaction. On average, nine H-bonds or salt bridges are formed, and two hydrophobic contacts are established between the guanosine derivatives and the RdRps. The most-reported residues from the RdRps that form H-bonds with the guanosine derivatives ranked based on the sum of the number of formed H-bonds are D761 (23), D760 (20), E811 (15), R555 (10), D623 (10), S682 (8), S814 (8), W800 (7), N691 (5), and D618 (4). Simultaneously, the most reported residue that forms hydrophobic contact with the guanosine derivatives is the active site residue D760 (8 connections). Additionally, other residues from RdRps contribute to developing four (Y619 and D761) or three (D623 and N691) hydrophobic contacts with the guanosine derivatives.
Table 1 The interactions that were established upon docking the guanosine triphosphate and its derivatives into the SARS-CoV-2 RdRp active site. Docking scores are calculated using AutoDock Vina software, while PLIP is used to get the interactions established. The interactions of positive (sofosbuvir, remdesivir, and ribavirin) and negative (cinnamaldehyde and thymoquinone) control compounds are listed as well in the table
Compound |
AutoDock score (kcal mol−1) |
H-Bonding/salt bridges |
Hydrophobic interaction |
No. |
Amino acids involved |
No. |
Amino acids involved |
GTP |
−8 |
16 |
Y619, D623, N691, S759(2), D760(2), D761(2), A762, V763, W800, E811(2), C813, S814 |
2 |
D760, D761 |
Sofosbuvir |
−7.5 |
7 |
R555, D618, C622, D761, E811, C813, S814 |
1 |
K621 |
Remdesivir |
−7.5 |
12 |
T246(3), T248, R249, T462(2), M463, D760, N790, N791(2) |
3 |
T246, M463(2) |
Ribavirin |
−7.3 |
10 |
R555(2), W617, D618, D761(2), A762, W800, C813, S814 |
0 |
— |
10 phenyl |
−7.7 |
11 |
D760, D761(4), W800, E811(2), C813, S814(2) |
0 |
— |
C12 |
−7.8 |
16 |
R555, C622, D623, S682(2), A685, T687, A688, N691, S759, D760, D761(2), E811, C813, S814 |
1 |
A688 |
 |
−7.9 |
12 |
D623, N691, S759, D760(5), D761, W800, H810, E811 |
2 |
L758(2) |
 |
−8 |
6 |
W617, Y619, D623, S682, N691, A762 |
3 |
Y619, D623, N691 |
C15 |
−7.7 |
10 |
D618, K621, D623, R624, R624, Y680(2), D760(2), D761 |
1 |
E811 |
C16 |
−7.9 |
6 |
R555, W617, D760, E811, S814(2) |
2 |
Y619, D760 |
C17 |
−7.8 |
9 |
R555(3), D618, K621(2), R624, T680, D760 |
0 |
— |
C18 |
−8.1 |
7 |
R555, 617W, 621K, 623D(2), D761 |
0 |
— |
C19 |
−7.9 |
12 |
K621, C622, D623(2), D761(2), W800, E811(2), C813, S814(2) |
2 |
Y619, D760 |
C30 |
−7.8 |
8 |
R553, W617(2), Y619, D761(2), A762, W800 |
1 |
D760 |
C31 |
−7.8 |
5 |
D623, D760, E811(2), C813 |
1 |
D761 |
C32 |
−7.9 |
10 |
Y619, K621, R624(2), S682, N691, S759(2), D760, D761 |
1 |
R555 |
C33 |
−7.6 |
8 |
K551, R555, D618, D760, W800, H810(2), E811 |
2 |
D760, D761 |
C34 |
−7.9 |
6 |
D760(2), D761(2), K798, S814 |
4 |
, D761, K798, E811 |
C35 |
−7.8 |
7 |
D761, A762, W800, H810, E811(3) |
2 |
D760, D761 |
C36 |
−7.9 |
11 |
D618, K621, C622, D623, S682(2), N691, S759, D761(3) |
4 |
I589(2), L758(2) |
C37 |
−7.9 |
8 |
R555(2), R624, T680, S681, S682, D760(2) |
2 |
N691, D760 |
C38 |
−7.9 |
11 |
W617, Y619(2), D760, D761(3), A762, W800, E811(2) |
1 |
D760 |
C39 |
−7.7 |
7 |
R555, R624, Y680(2), S682, S759, D760 |
5 |
Y619, D623(2), N691, D760 |
Cinnamaldehyde |
−5 |
3 |
C813, S814(2) |
3 |
D761, V763, F812 |
Thymoquinone |
−5.3 |
2 |
C813, S814 |
2 |
V763, E811 |
The best two guanosine derivatives are the C13 (3,5-dihydroxy phenyl substitution) and C14 (3-hydroxyphenyl substitution) with average binding affinities of −7.94 ± 0.58 and −7.96 ± 0.43 kcal mol−1, respectively. Fig. 4 shows a graphical representation of the interactions between the best two compounds and selected RdRps. The RdRp residues are labeled by their one-letter codes and represented in blue sticks, while the guanosine derivatives are in yellow sticks. H-Bonds and salt bridges are depicted by blue lines and dashed-yellow lines, respectively, while dashed-gray lines represent the hydrophobic contacts. Notably, the added bulky moiety at the 2′ position of the ribose contributes to the binding against the protein's active site pocket residues.
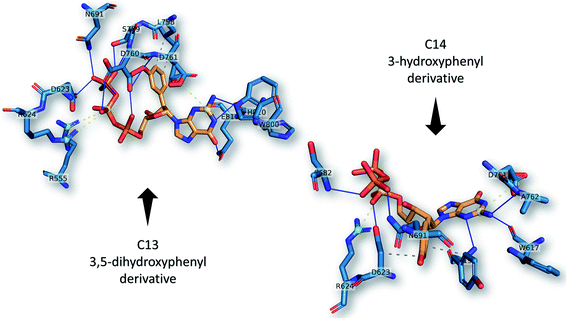 |
| Fig. 4 The interactions that were established after docking the guanosine derivatives C13 and C14 (see Fig. 1) (orange sticks) against SARS-CoV-2 RdRp active site pocket residues (blue sticks). H-Bonds in solid blue lines while hydrophobic interactions are in dashed-gray lines. Salt bridges are in yellow spheres connected by dashed lines. | |
MDS and binding free energy calculation for C13 and C14 RdRp complexes
MDS for the best two complexes (C13 and C14 against RdRp) and the GTP–RdRp complex are performed for 100 ns using NAMD 2.13 software, followed by MM-GBSA calculations for the same complexes using Amber tools. The residual contribution for the binding of RdRp against the GTP–RdRp complex and the best two compounds (C13 and C14) are listed in Table 2 and ranked according to their binding energy values (ascending). The active site aspartates (D760 and D761) are shown in bold and underlined in the table as well. For the RdRp–GTP complex, which represents the positive control compound, F812, C813, S814, R836, and G616 are the most important residues in binding the GTP with binding energies −3.98, −3.17, −2.52, −0.95 and −0.95 kcal mol−1, respectively. On the other hand, in the RdRp–C13 complex, D760, C313, and L758 are the main contributors to binding (−1.58, −1.57, and −0.81 kcal mol−1, respectively). While for RdRp–C14 complex, R555, G683, T556, S682, R624, K621, Y619, T680, and V557 are the main contributors (−10.55, −3.04, −2.54, −2.49, −2.22, −1.67, −1.48, −1.23, and −0.93 kcal mol−1, respectively) to binding. The contribution of the ligands (LIG) is important in the case of GTP and C14 complexes with binding energy contributions of −4.11 and −20.93, respectively. On the other hand, in the C13 complex, the LIG contribution to binding is +2.26. This suggests the superiority of C14 over C13 in binding the RdRp. Additionally, active site aspartate (D760 and D761) contributions in binding are +1.89, −1.58, and +1.55 in GTP, C13 and C14, respectively. Some other residues have a negative impact on the binding (shown in red in Table 2) these residues were D623, H816, K545, E811, and D618 in GTP–RdRp complex, D865, D618, K545, and D623 in RdRp–C13 complex, and D623 and D452 with a total contribution to binding of +3.45, +1.19, and +1.7 kcal mol−1, respectively.
Table 2 The MM-GBSA calculations for the best two complexes (C13 and C14 complexes with RdRp) after 100 ns MDS. Red coloured residues represent the residue have negative contribution on the binding (positive binding energies). The average binding free energies and its individual terms are shown at the bottom for each complex with its standard deviations
Thus, the total binding energy for the RdRp–C14 complex is lower (−46.95 ± 6.3 kcal mol−1) than RdRp–C13 and the RdRp-positive control (GTP) (−5.14 ± 6.6 and −13.82 ± 6.2 kcal mol−1, respectively); hence it is suggested to be the best SARS-CoV-2 RdRp potential inhibitor in the current study. Therefore, we recommend testing this compound experimentally against SARS-CoV-2 RdRp.
Fig. 5 supports the docking data as reflected from the flattened RMSD curves (Fig. 5A) of the GTP and the two complexes (C13 and C14) versus SARS-CoV-2 RdRp. The three complexes were equilibrated during the 100 ns as reflected from the flattened RMSD (A), the equilibrated Radius of Gyration (RoG) (B), and Surface Accessible Surface Area (SASA) (C) curves. The systems RMSD, RoG, and SASA mean values are equilibrated at around 2.5 Å, 29.7 Å, and 40
000 Å3, respectively. The GTP–RdRp complex possesses elevated RoG and SASA values compared to the C13 and C14 complexes. The total number of the formed H-bonds is almost stable around 1300 during the simulation period for C13 and C14 complex but 1500 for the GTP complex, as shown in Fig. 5D. The per-residue RMSF is shown in Fig. 5E for the two complexes, where C13–RdRp is represented by the orange line, while C14–RdRp is represented by the gray one. Apart from the N and C-terminals, the RMSF show high fluctuations (RMSF < 3) in the D469–G480 region in the case of the C13–RdRp complex compared to the C14–RdRp. Additionally, the C14–RdRp complex shows lower RMSF values in 460 and 560 residues and is more stable than the C13–RdRp complex. These regions are apart from the active site aspartates (D760 & D761) that are shown in black sticks in the structure at the top of the RMSF curves. So, C14–RdRp is a more stable complex than C13–RdRp complex, which supports our calculated binding energies.
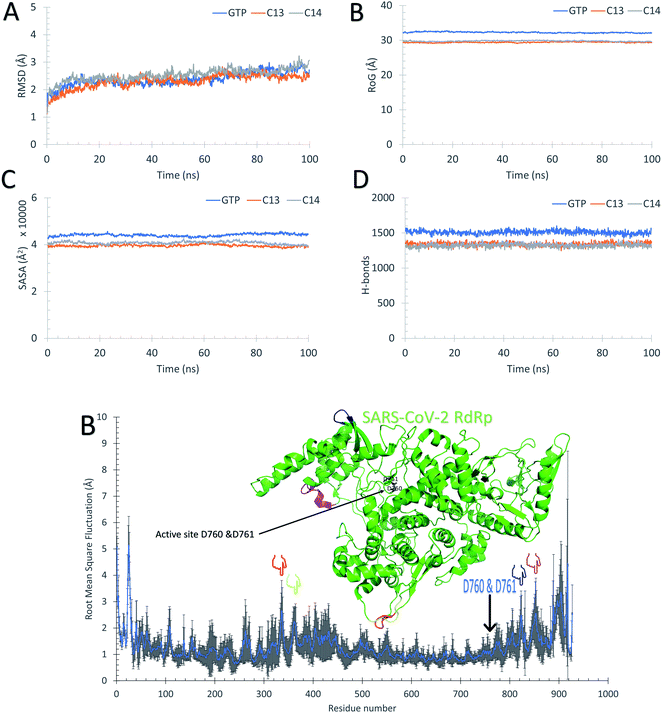 |
| Fig. 5 Molecular dynamics simulation of the C13–RdRp and C14–RdRp complexes. (A) The root mean square deviations (RMSD) in Å, (B) the radius of gyration (RoG) in Å, (C) the surface accessible surface area (SASA) in Å3, and (D) the total number of H-bonds versus simulation time in ns. (E) The per-residue root mean square fluctuations (RMSF) in Å alongside the structure of C14–RdRp complex shown in colored cartoon representation. The active site residues (D760 and D761) are shown in black sticks, while the marked region of the RMSF are depicted in the structure by the blue cartoons. | |
Fig. 5B, C, and D reflect that the GTP complex has higher RoG, SASA, and H-bonds. This, in fact, is due to the protein size used in this complex which is 925 residues (PDB ID: 6XEZ), while for C13–RdRp and C14–RdRp complexes, the protein size is 833-residues (PDB ID: 7BV1). In general, all the nineteen guanosine derivatives presented in Fig. 3 could be successful candidates against SARS-CoV-2 RdRp, while compound C14 is the best in binding SARS-CoV-2 RdRp based on the calculated MM-GBSA and the RMSF curves. Therefore, C14 should be tested experimentally to prove its binding affinity and binding mode against the SARS-CoV-2 RdRp active site.
Conclusion
The pandemic SARS-CoV-2 is still causing everyday life casualties since its emergence in December 2019. The availability of solved structures for SARS-CoV-2 RdRp allows us to test a bunch of new compounds, based on guanosine triphosphate, as possible RdRp blockers. Based on the current computational study, nineteen of the guanosine derivatives show promising binding affinities against SARS-CoV-2 RdRp solved structures (−7.83 ± 0.08 kcal mol−1) comparable to that of remdesivir (−7.70 ± 0.58 kcal mol−1), sofosbuvir (−7.55 ± 0.43 kcal mol−1), and ribavirin (−7.34 ± 0.49). The added bulky groups at the 2′ position of the ribose ring positively impact the binding affinity. C14 (3-hydroxyphenyl substitution at 2′ position) shows the tightest binding affinity to the RdRp using MM-GBSA calculations and hence is suggested as a potent binder to the viral protein. However, this is yet to be confirmed experimentally.
Author contributions
A. E. designed the research study, made the tables & figures and wrote the draft. H. M. & I. I. performed the calculations and retrieve the data. W. E. supervised H. M. and revised the manuscript. M. I. received funding and logistics. All authors have read and approved the final manuscript.
Conflicts of interest
All the authors declare that there is no conflict of interest for this work.
Acknowledgements
Shaheen supercomputer of King Abdullah University of Science and Technology (KAUST) is utilized to perform the after dockings MDS calculations (under Project Number k1482). Additionally, the Bibliotheca Alexandrina HPC is used to perform the core MDS calculations. This work is supported by the Cairo University COVID-19 fund received by AE.
References
- D. S. Hui, W. I. Azhar, T. A. Madani, F. Ntoumi, R. Kock and O. Dar, et al., The continuing 2019-nCoV epidemic threat of novel coronaviruses to global health – The latest 2019 novel coronavirus outbreak in Wuhan, China, Int. J. Infect. Dis., 2020, 91, 264–266 CrossRef CAS PubMed.
- I. I. Bogoch, A. Watts, A. Thomas-Bachli, C. Huber, M. U. G. Kraemer and K. Khan, Pneumonia of unknown aetiology in Wuhan, China: potential for international spread via commercial air travel, J. Travel Med., 2020, 27(2), taaa008 CrossRef PubMed.
- A. Nassar, I. M. Ibrahim, F. G. Amin, M. Magdy, A. M. Elgharib and E. B. Azzam, et al., A Review of Human Coronaviruses' Receptors: The Host-Cell Targets for the Crown Bearing Viruses, Molecules, 2021, 26(21), 6455 CrossRef CAS PubMed.
- A. A. Elfiky, S. M. Mahdy and W. M. Elshemey, Quantitative structure–activity relationship and molecular docking revealed a potency of anti-hepatitis C virus drugs against human corona viruses, J. Med. Virol., 2017, 89(6), 1040–1047 CrossRef CAS PubMed.
- J. F. Chan, S. K. Lau, K. K. To, V. C. Cheng, P. C. Woo and K.-Y. Yuen, Middle East respiratory syndrome coronavirus: another zoonotic betacoronavirus causing SARS-like disease, Clin. Microbiol. Rev., 2015, 28(2), 465–522 CrossRef CAS PubMed.
- WHO, Middle East respiratory syndrome coronavirus (MERS-CoV), WHO, 2016, updated 23 June 2016 Search PubMed.
- Y. Jiang, W. Yin and H. E. Xu, RNA-dependent RNA polymerase: Structure, mechanism, and drug discovery for COVID-19, Biochem. Biophys. Res. Commun., 2021, 538, 47–53 CrossRef CAS PubMed.
- A. A. Elfiky, E. B. Azzam and M. W. Shafaa, The anti-HCV, Sofosbuvir, versus the anti-EBOV Remdesivir against SARS-CoV-2 RNA dependent RNA polymerase in silico, Mol. Diversity, 2021 Search PubMed.
- A. A. Elfiky, Reply to a letter to the editor, Life Sci., 2020, 252, 117715 CrossRef CAS PubMed.
- A. A. Elfiky, Corrigendum to “Ribavirin, Remdesivir, Sofosbuvir, Galidesivir, and Tenofovir against SARSCoV-2 RNA dependent RNA polymerase (RdRp): A molecular docking study” [Life Sci. 253 (2020) 117592], Life Sci., 2020, 258, 118350 CrossRef CAS PubMed.
- A. A. Elfiky and A. Ismail, Molecular dynamics and docking reveal the potency of novel GTP derivatives against RNA dependent RNA polymerase of genotype 4a HCV, Life Sci., 2019, 238, 116958 CrossRef CAS PubMed.
- A. A. Elfiky, Novel Guanosine Derivatives as Anti-HCV NS5b Polymerase: A QSAR and Molecular Docking Study, Med. Chem., 2019, 15(2), 130–137 CrossRef CAS PubMed.
- A. Ganesan and K. Barakat, Applications of Computer-Aided Approaches in the Development of Hepatitis C Antiviral Agents, Expert Opin. Drug Discovery, 2017, 12(4), 407–425 CrossRef CAS PubMed.
- A. A. Elfiky and A. M. Ismail, Molecular Modeling and Docking revealed superiority of IDX-184 as HCV polymerase Inhibitor, Future Virol., 2017, 12(7), 339–347 CrossRef CAS.
- A. A. Elfiky and W. M. Elshemey, IDX-184 is a superior HCV direct-acting antiviral drug: a QSAR study, Med. Chem. Res., 2016, 25(5), 1005–1008 CrossRef CAS PubMed.
- A. A. Elfiky and W. M. Elshemey, Molecular dynamics simulation revealed binding of nucleotide inhibitors to ZIKV polymerase over 444 nanoseconds, J. Med. Virol., 2018, 90(1), 13–18 CrossRef CAS PubMed.
- A. A. Elfiky, Zika virus: novel guanosine derivatives revealed strong binding and possible inhibition of the polymerase, Future Virol., 2017, 12(12), 721–728 CrossRef CAS.
- P. L. Yang, M. Gao, K. Lin, Q. Liu and V. A. Villareal, Anti-HCV drugs in the pipeline, Curr. Opin. Virol., 2011, 1(6), 607–616 CrossRef CAS PubMed.
- P. Glue, The clinical pharmacology of ribavirin, Semin. Liver Dis., 1999, 19(1), 17–24 CAS.
- M. P. Manns, J. G. McHutchison, S. C. Gordon, V. K. Rustgi, M. Shiffman and R. Reindollar, et al., Peginterferon alfa-2b plus ribavirin compared with interferon alfa-2b plus ribavirin for initial treatment of chronic hepatitis C: a randomised trial, Lancet, 2001, 358(9286), 958–965 CrossRef CAS.
- E. J. Gane, C. A. Stedman, R. H. Hyland, X. Ding, E. Svarovskaia and W. T. Symonds, et al., Nucleotide polymerase inhibitor sofosbuvir plus ribavirin for hepatitis C, N. Engl. J. Med., 2013, 368(1), 34–44 CrossRef CAS PubMed.
- T. K. Warren, R. Jordan, M. K. Lo, A. S. Ray, R. L. Mackman and V. Soloveva, et al., Therapeutic efficacy of the small molecule GS-5734 against Ebola virus in rhesus monkeys, Nature, 2016, 531(7594), 381–385 CrossRef CAS PubMed.
- M. Wang, R. Cao, L. Zhang, X. Yang, J. Liu and M. Xu, et al., Remdesivir and chloroquine effectively inhibit the recently emerged novel coronavirus (2019-nCoV) in vitro, Cell Res., 2020, 30(3), 269–271 CrossRef CAS PubMed.
- W. Markland, T. J. McQuaid, J. Jain and A. D. Kwong, Broad-spectrum antiviral activity of the IMP dehydrogenase inhibitor VX-497: a comparison with ribavirin and demonstration of antiviral additivity with alpha interferon, Antimicrob. Agents Chemother., 2000, 44(4), 859–866 CrossRef CAS PubMed.
- H. Berman, K. Henrick and H. Nakamura, Announcing the worldwide Protein Data Bank, Nat. Struct. Biol., 2003, 10(12), 980 CrossRef CAS PubMed.
- Q. Wang, J. Wu, H. Wang, Y. Gao, Q. Liu and A. Mu, et al., Structural Basis for RNA Replication by the SARS-CoV-2 Polymerase, Cell, 2020, 182(2), 417–428 CrossRef CAS PubMed.
- Q. Wang, J. Wu, H. Wang, Y. Gao, Q. Liu and A. Mu, et al., Structural Basis for RNA Replication by the SARS-CoV-2 Polymerase, Cell, 2020, 182(2), 417–428 CrossRef CAS PubMed.
- W. G. Guntheroth, The cost and benefits of segregation (by age), Am. Heart J., 1979, 97(5), 549–550 CrossRef CAS PubMed.
- W. Yin, C. Mao, X. Luan, D. D. Shen, Q. Shen and H. Su, et al., Structural basis for inhibition of the RNA-dependent RNA polymerase from SARS-CoV-2 by remdesivir, Science, 2020, 368(6498), 1499–1504 CrossRef CAS PubMed.
- Y. Gao, L. Yan, Y. Huang, F. Liu, Y. Zhao and L. Cao, et al., Structure of the RNA-dependent RNA polymerase from COVID-19 virus, Science, 2020, 368(6492), 779–782 CrossRef CAS PubMed.
- H. S. Hillen, G. Kokic, L. Farnung, C. Dienemann, D. Tegunov and P. Cramer, Structure of replicating SARS-CoV-2 polymerase, Nature, 2020, 584(7819), 154–156 CrossRef CAS PubMed.
- D. Seeliger and B. L. de Groot, Ligand docking and binding site analysis with PyMOL and Autodock/Vina, J. Comput.-Aided Mol. Des., 2010, 24(5), 417–422 CrossRef CAS PubMed.
- K. L. Summers, A. K. Mahrok, M. D. Dryden and M. J. Stillman, Structural properties of metal-free apometallothioneins, Biochem. Biophys. Res. Commun., 2012, 425(2), 485–492 CrossRef CAS PubMed.
- J. H. Lii and N. L. Allinger, Molecular mechanics. The MM3 force field for hydrocarbons. 3. The van der Waals' potentials and crystal data for aliphatic and aromatic hydrocarbons, J. Am. Chem. Soc., 1989, 111(23), 8576–8582 CrossRef CAS.
- Z. Bikadi and E. Hazai, Application of the PM6 semi-empirical method to modeling proteins enhances docking accuracy of AutoDock, J. Cheminf., 2009, 1(1), 15 Search PubMed.
- J. C. Phillips, R. Braun, W. Wang, J. Gumbart, E. Tajkhorshid and E. Villa, et al., Scalable molecular dynamics with NAMD, J. Comput. Chem., 2005, 26(16), 1781–1802 CrossRef CAS PubMed.
- J. Huang and A. D. MacKerell Jr, CHARMM36 all-atom additive protein force field: validation based on comparison to NMR data, J. Comput. Chem., 2013, 34(25), 2135–2145 CrossRef CAS PubMed.
- W. Humphrey, A. Dalke and K. Schulten, VMD: visual molecular dynamics, J. Mol. Graphics, 1996, 14(1), 33–38 CrossRef CAS PubMed.
- A. A. Elfiky and I. M. Ibrahim, Host-cell recognition through GRP78 is enhanced in the new UK variant of SARS-CoV-2, in silico, J. Infect., 2021, 82(5), 186–230 CrossRef CAS PubMed.
- P. Mark and L. Nilsson, Structure and dynamics of the TIP3P, SPC, and SPC/E water models at 298 K, J. Phys. Chem. A, 2001, 105(43), 9954–9960 CrossRef CAS.
- T. Tubiana, J. C. Carvaillo, Y. Boulard and S. Bressanelli, TTClust: A Versatile Molecular Simulation Trajectory Clustering Program with Graphical Summaries, J. Chem. Inf. Model., 2018, 58(11), 2178–2182 CrossRef CAS PubMed.
- O. Trott and A. J. Olson, AutoDock Vina: improving the speed and accuracy of docking with a new scoring function, efficient optimization, and multithreading, J. Comput. Chem., 2010, 31(2), 455–461 CAS.
- G. M. Morris, R. Huey, W. Lindstrom, M. F. Sanner, R. K. Belew and D. S. Goodsell, et al., AutoDock4 and AutoDockTools4: Automated docking with selective receptor flexibility, J. Comput. Chem., 2009, 30(16), 2785–2791 CrossRef CAS PubMed.
- S. Salentin, S. Schreiber, V. J. Haupt, M. F. Adasme and M. Schroeder, PLIP: fully automated protein–ligand interaction profiler, Nucleic Acids Res., 2015, 43(W1), W443–W447 CrossRef CAS PubMed.
- M. F. Adasme, K. L. Linnemann, S. N. Bolz, F. Kaiser, S. Salentin and V. J. Haupt, et al., PLIP 2021: expanding the scope of the protein–ligand interaction profiler to DNA and RNA, Nucleic Acids Res., 2021, 49(W1), W530–W534 CrossRef CAS PubMed.
- S. Genheden and U. Ryde, The MM/PBSA and MM/GBSA methods to estimate ligand-binding affinities, Expert Opin. Drug Discovery, 2015, 10(5), 449–461 CrossRef CAS PubMed.
- A. S. Mayhoub, Hepatitis C RNA-dependent RNA polymerase inhibitors: a review of structure–activity and resistance relationships; different scaffolds and mutations, Bioorg. Med. Chem., 2012, 20(10), 3150–3161 CrossRef CAS PubMed.
- A. A. Elfiky and A. M. Ismail, Molecular docking revealed the binding of nucleotide/side inhibitors to Zika viral polymerase solved structures, SAR QSAR Environ. Res., 2018, 29(5), 409–418 CrossRef CAS PubMed.
- A. M. Lam, C. Espiritu, S. Bansal, H. M. Micolochick Steuer, C. Niu and V. Zennou, et al., Genotype and subtype profiling of PSI-7977 as a nucleotide inhibitor of hepatitis C virus, Antimicrob. Agents Chemother., 2012, 56(6), 3359–3368 CrossRef CAS PubMed.
- E. Murakami, T. Tolstykh, H. Bao, C. Niu, H. M. Steuer and D. Bao, et al., Mechanism of activation of PSI-7851 and its diastereoisomer PSI-7977, J. Biol. Chem., 2010, 285(45), 34337–34347 CrossRef CAS PubMed.
- C. Q. Sacramento, G. R. de Melo and C. S. de Freitas, The clinically approved antiviral drug sofosbuvir inhibits Zika virus replication, Sci. Rep., 2017, 7, 40920 CrossRef CAS PubMed.
- K. M. Bullard-Feibelman, J. Govero, Z. Zhu, V. Salazar, M. Veselinovic and M. S. Diamond, et al., The FDA-approved drug sofosbuvir inhibits Zika virus infection, Antiviral Res., 2017, 137, 134–140 CrossRef CAS PubMed.
- A. A. Elfiky, The antiviral Sofosbuvir against mucormycosis: an in silico perspective, Future Virol., 2019, 14(11), 739–744 CrossRef CAS.
|
This journal is © The Royal Society of Chemistry 2022 |
Click here to see how this site uses Cookies. View our privacy policy here.