DOI:
10.1039/D2NR00882C
(Paper)
Nanoscale, 2022,
14, 6990-7002
Effect of encapsulated protein on the dynamics of lipid sponge phase: a neutron spin echo and molecular dynamics simulation study†
Received
14th February 2022
, Accepted 19th April 2022
First published on 20th April 2022
Abstract
Lipid membranes are highly mobile systems with hierarchical, time and length scale dependent, collective motions including thickness fluctuations, undulations, and topological membrane changes, which play an important role in membrane interactions. In this work we have characterised the effect of encapsulating two industrially important enzymes, β-galactosidase and aspartic protease, in lipid sponge phase nanoparticles on the dynamics of the lipid membrane using neutron spin echo (NSE) spectroscopy and molecular dynamics (MD) simulations. From NSE, reduced membrane dynamics were observed upon enzyme encapsulation, which were dependent on the enzyme concentration and type. By fitting the intermediate scattering functions (ISFs) with a modified Zilman and Granek model including nanoparticle diffusion, an increase in membrane bending rigidity was observed, with a larger effect for β-galactosidase than aspartic protease at the same concentration. MD simulations for the system with and without aspartic protease showed that the lipids relax more slowly in the system with protein due to the replacement of the lipid carbonyl–water hydrogen bonds with lipid–protein hydrogen bonds. This indicates that the most likely cause of the increase in membrane rigidity observed in the NSE measurements was dehydration of the lipid head groups. The dynamics of the protein itself were also studied, which showed a stable secondary structure of protein over the simulation, indicating no unfolding events occurred.
Polar lipids are key components of cell membranes, which spontaneously self-assemble in an aqueous environment into a wide variety of lipid liquid crystalline (LLC) structures, resulting in a rich phase behaviour even for very simple systems.1 Of particular interest are so-called inverse LLC phases, where the lipid–aqueous interface is curved towards the aqueous phase, thereby forming aqueous cavities. Many of these possible phases are based on a bilayer, the basic structural unit of biological membranes, including the lamellar, bicontinuous cubic and sponge (L3) phases.1–4 The L3 phase, an intermediate mesophase between lamellar and cubic, can be considered a sort of ‘melted’ cubic phase, in that it has a similar short range structure of interconnected water channels, but with no long range order2,5 (Fig. S1†).
The average static structures of lipid bilayer based phases are generally well-established, but, at physiological and near physiological temperatures, the lipid bilayers undergo significant fluctuations in shape and microscopic structure, which are not fully understood in terms how they affect and are affected by e.g. protein interactions.6 At mesoscopic length scales, these fluctuations are caused by the collective motion of the membrane lipids, therefore undulation (bending) and peristaltic (thickness) modes dominate. However, at molecular length scales, the dynamics reflect the motions of individual lipids and therefore protrusion modes become important.6–8 Bilayer fluctuations play an important role in lipid–protein and interbilayer interactions, including in the function of membrane proteins and in interactions with peripheral proteins and other biomolecules, and at longer range, as demonstrated for membranes in a multi-lamellar stack.9–12
The structure formed by, and therefore the dynamics of, the lipid system strongly depends on both the composition of the system and its environment, including temperature, pressure, pH, water content, salt concentration and valency.1–4 The stability of this structure depends on a delicate balance of transverse interactions between neighbouring lipid layers, such as van der Waals forces, hydration, and steric repulsion, and lateral interactions within the bilayers, such as hydrogen bonding.13–16 The water content of the system can modulate its structure via lipid hydration, as hydrogen bonding networks in the hydration layer at the surface of the bilayer help to mediate lipid–lipid associations.17,18 Inclusion of solutes or additives in the aqueous phase can change or disrupt this lipid hydration, through the solute competing with the lipid for water interactions or the solute binding to the lipid head groups, displacing the bound water molecules, resulting in structural changes or even phase transitions.19
The mechanism and such factors that drive lamellar to non-lamellar transitions in these simpler systems can be used to understand similar features in biologically relevant systems, as, although cell membranes are commonly depicted as flat or curved single bilayers, non-lamellar structures have repeatedly been observed in biological systems (ref. 1 and 20 and refs within). Membranous organelles in eukaryotic cells can adapt their morphology in response to changes in their environment, such as in the endoplasmic reticulum, where there is a high rate of reversible lamellar to cubic phase transitions, and the inner membrane of mitochondria, which can reorganise to cubic-like morphologies.20,21 Numerous studies have addressed the flexibility and curvature of mitochondria, both experimentally and through simulations, as mitochondria are considered to be dynamic organelles subject to fusion and fission and these dynamics are believed to be important for cell quality control.22–26
Understanding the dynamics of the system can provide information on the mechanical and elastic properties of the membrane, and consequently interactions between the membrane and additives.8 The undulation modes, for example, can be described by the membrane bending rigidity, which is low for a lipid bilayer, due to the fluid-like nature of the system. It typically ranges from several to tens of kbT, where kb is the Boltzmann constant and T is the absolute temperature.10,27–31 Multiple techniques have been established to measure bending rigidity, including advanced microscopy methods such as flicker spectroscopy, mechanical methods, such as micropipette aspiration, scattering methods including X-ray and neutron scattering techniques, and, more recently atomistic and coarse grained simulations (ref. 8, 32 and 33 and references within).
Neutron spin echo (NSE) spectroscopy is a non-invasive scattering method, which is well-suited for the measurement of thermal fluctuations of lipid membranes, from which the membrane bending rigidity can be extracted. The typical correlation times (0.1 ns to 100 ns) and length scales (10 Å to 500 Å) accessible with this method overlap well with the time and length scales of interest in membrane dynamics.34–37
NSE has been used to investigate lipid membrane dynamics using vesicles and lamellar stacks, which allowed calculations of bending rigidity of lipid bilayers. These studies have demonstrated the capability of the technique to reveal the effect of the system composition, addition of charged components and different solutes (ref. 31 and references within11,29,35,38–48). The majority of these studies have focused on model biomembranes, using biologically relevant lipids and investigating biologically relevant phenomena, although there is also a relatively large body of work regarding lamellar and bicontinuous microemulsions.8,33
As NSE measurements capture the average of a system's dynamics, simulations are useful to isolate the contributions of specific motions to this average. In earlier computational works, it was possible to calculate undulations of lipid bilayers which were used as model systems for cell membranes, outer shells of liposomes and pharmaceutically relevant lipid nano-particles.49 Although computational power limits the size and complexity of the simulated system, with modern hardware and software, it is now possible to simulate larger objects, such as inner parts of lipid nanoparticles with their cargo.50,51
We have previously reported on a food and pharmaceutical grade lipid system, composed of diglycerol monooleate (DGMO), a mixture of mono-, di- and tri-glycerides with the main component glycerol monooleate (GMO-50), and polysorbate 80 (P80), which can form a highly swollen lipid L3 phase.52 It was possible to use this to encapsulate two enzymes of different sizes β-galactosidase from Kluyveromyces lactis (PDB: 3OBA) and aspartic protease from Cryphonectria parasitica (PDB: 1OEW), while retaining enzymatic activity in both systems.53–56 β-Galactosidase, a tetramer of 476 kDa, and aspartic protease, 34.6 kDa, are important industrial enzymes used in various processes in the food industry.57 The L3 phase can also be dispersed into well-defined sponge-like nanoparticles without the use of harsh methods, like sonication, allowing preservation of the activity of the encapsulated enzymes and ensuring a high encapsulation efficiency55,56 (shown in Fig. S2†).
In this paper, we have, for the first time to our knowledge, studied the dynamics of these highly swollen lipid sponge phase nanoparticles (L3NPs) and how they are affected by the encapsulation of two enzymes of different size using NSE spectroscopy. Complementary atomistic molecular dynamics (MD) simulations of the inner parts of the L3NPs were performed for the empty L3NPs and L3NPs containing aspartic protease. The aim was to reveal the effect of the encapsulated proteins on the nature of the dynamic properties of the lipid membranes in water, focussing on membrane bending rigidity.
Materials and methods
Materials
The lipid sponge phase was composed of Capmul GMO-50, DGMO and P80. Capmul GMO-50 (Lot no. 100616-8, Abitec, USA) was made up of 54.7% monoglycerides (mainly glycerol monooleate, GMO), 15% to 35% diglycerides, and 2% to 10% triglycerides with the following fatty acid composition: 84.6% oleic (C18:1), 6.8% linoleic, 0.8% linolenic, and 6.2% saturated acids. In the diglycerol monooleate (DGMO) (Danisco A/S, Brabrand, Denmark), there was 88% diglycerol monoester and 4.9% free glycerol and polyglycerols. The main fatty acid component was oleic acid constituting 90.7%, followed by linoleic (4.2%), saturated (2.9%), eicosenoic (1.2%), and linolenic (0.8%). Polyoxyethylene (20) sorbitan monooleate (P80) was purchased from Sigma Aldrich. D2O was purchased from Cambridge Isotope Laboratories Inc.
Ha-Lactase 5200, generously provided by Chr. Hansen (Horsholm, Denmark), contained 50% glycerol (E422), water and neutral β-galactosidase (from Kluyveromyces lactis). Thermolase, also generously provided by Chr. Hansen (Horsholm, Denmark), contained 50% glycerol (E422), water and aspartic protease (from Cryphonectria parasitica).
All other reagents were of analytical grade and purchased from Sigma Aldrich.
Sample preparation
The preparation of L3NPs containing β-galactosidase and aspartic protease has been described in detail previously,55,56 but has been summarised here for convenience.
Dialysis of thermolase and Ha-lactase 5200.
In order to remove the glycerol from the mixture, Ha-lactase 5200 was dialysed against 50 mM sodium phosphate buffer with 1 mM MgCl2·6H2O (aq) and 0.5 mM NaN3 (aq) at pH 7.3 ± 0.5 for 24 h at 4 °C. Here M indicates molarity and 1 M = 1 mol L−1. After dialysis, the enzyme was mostly present in its dimeric form.55 The resulting dialysed β-galactosidase was then concentrated by centrifugation and diluted to the required concentrations using 50 mM sodium phosphate buffer with 1 mM MgCl2·6H2O (aq). In order to remove glycerol from Thermolase, it was dialysed against 10 mM phosphate buffer at pH 7 over 4 days at 4 °C, with external buffer changes every 8 h to 12 h. The resulting dialysed aspartic protease was freeze dried to obtain a powder, which was then used to prepare the required solution concentrations using Milli Q water. Both of the enzymes retained their enzymatic activity after purification as discussed in previous studies.55,56
Preparation of bulk phases.
A lipid mixture of mass ratio 28/42/30 DGMO/GMO-50/P80 was prepared in a glass vial, which was sealed and left to mix on a roller table for 24 h at 25 °C. The sponge phase was prepared by mixing lipid–polymer mixture/aqueous solution in a mass ratio of 40/60. The lipid-enzyme bulk phases were prepared with two concentrations of enzyme solution: 15 mg ml−1 and 44 mg ml−1. The vials were then sealed, centrifuged up and down, and left to equilibrate for at least 7 days at 25 °C. This method gives bulk phases with final enzyme concentrations of 9 mg ml−1 and 26.4 mg ml−1.
Preparation of nanoparticles.
L3NPs without enzyme were prepared by mixing a mass fraction of 4% (4 wt%) lipid–polymer mixture and 96 wt% D2O. L3NPs with enzyme were prepared by mixing by mass 10 wt% L3 bulk phase with enzyme and 90 wt% D2O, which results in the same lipid–polymer concentration by weight as the empty L3NPs. The sample vials were sealed, vigorously hand shaken and mixed on an orbital shaker for 24 h at 270 rpm and ≈25 °C.
Neutron spin echo (NSE) spectroscopy
Neutron spin echo measurements were performed using the NGA-NSE spectrometer at the National Institute of Standards and Technology (NIST) over the wavevector transfer, q, range 0.04 Å−1 to 0.14 Å−1 and Fourier time range 0.65 ns to 100 ns, resulting in a measurement time of 12 h to 24 h per sample.58 The spin echo data was reduced using DAVE to obtain the normalised intermediate scattering functions (ISFs) I(q,t)/I(q,0).59 The experimental data was fitted in Igor Pro (WaveMetrics Inc., USA), as described in detail below.
Data fitting.
The ISFs were fit according to a modified Zilman and Granek model to determine the bending rigidity of the bilayer, which has since been further developed.60,61 The original model proposed by Zilman and Granek considers the membrane as an ensemble of single non-interacting membrane plaquettes of size L × L, which are randomly oriented and described by the Helfrich bending free energy.30,62 As the size of the L3 nanoparticles was estimated to be approximately 160 nm from previous studies, overall diffusion of the nanoparticles was also considered in the analysis.55,56 The relaxation function due to diffusion and thermal undulations was shown to be: |  | (1) |
where D is diffusion constant (as determined by dynamic light scattering55,56), q is scattering vector, t is Fourier time (ns) and Γ is relaxation rate (ns−1). This expression for S(q,t) was used to fit the ISFs, therefore determine Γ. In the valid q range, the relaxation rate Γ is equal to the relaxation rate for bending fluctuations Γb, given by the expression: | 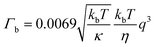 | (2) |
where κ is bilayer bending modulus and η is solvent viscosity. The above expression for Γb was used to calculate bending rigidity. The full derivation of the above expressions and fitted ISFs (Fig. S3†) are included in the ESI,† as well as a comparison to fitting results when excluding the contribution of diffusion (Fig. S6†). It is noted here, however, that the absolute values of the bending modulus calculated using NSE is an ongoing discussion,61 while there is in general consensus that relative trends estimated through an NSE experiment are correct. The relative values were, therefore, calculated and discussed here as follows: |  | (3) |
where κrel is the bending modulus normalised to κempty, the bending modulus for the empty L3NPs.
Simulation
Force field parameterisation.
In order to perform atomistic simulations new numerical models for lipids were derived and added to the existing SLipids force field (FF), using the same philosophy of calculation as in earlier versions of SLipids FF.63 Several model molecules were selected in order to accurately derive partial atomic charges P80, DGMO, GMO, triglycerides, and diglycerides. For simplicity, it was assumed that all lipid tails were mono-unsaturated, as they were the dominant type of hydrocarbon chain in all compounds. The detailed FF can be observed in the zip-archive of the ESI.† All other parameters for bonded interactions and Lennard-Jones potential were taken from the existing SLipids FF. The structures of lipids and other molecules utilized for the partial charge derivation are shown in Fig. S7 in ESI.†
Molecular dynamics simulations.
After derivation of the FF parameters, molecular dynamics (MD) simulations for two systems paralleling two of the experimental samples were set up: one corresponding to the empty L3NPs and one corresponding to the L3NPs with aspartic protease. Both systems contained the same number of lipids: 248 P80 molecules, 705 DGMO molecules, 9815 water molecules and, for the Capmul GMO-50 mixture, 702 molecules of GMO, 257 molecules of a diglyceride and 51 molecules of a triglyceride (see Fig. S7 in ESI† for the lipid structures). Due to high computational costs, a system containing only 1 molecule of the smaller of the two proteins, aspartic protease, was simulated. In this simulation with 1 molecule of aspartic protease, 13 sodium counter ions were added in order to neutralise the protein charge. All molecules were randomly placed in the simulation boxes with a distance of 5 Å around each molecule in order to avoid overlaps and clustering. SLipids FF63 was used to describe the lipids and the CHARMM36 FF64 was used to describe the aspartic protease, due to its compatibility with SLipids FF. TIP3p was selected as the water model.65
Every simulation was carried out for 600 ns, with the last 100 ns considered for analysis. The temperature was 298 K for every system and was controlled by a velocity rescale coupling scheme66 with the time constant set to 0.5 ps. The pressure was 1.013 atm for each simulation. It was regulated by the Berendsen pressure coupling scheme67 with the time constant set to 10 ps. The isotropic pressure coupling type68 was selected for all simulations, since the systems were expected to be disordered. The LINCS algorithm was selected for optimisation of bond lengths with 12 iterations.69 The integrator for Newtonian equations of motion was leap-frog with the time step of 2 fs.70 The software used for simulations was GROMACS-2019.71
Self-intermediate scattering functions and correlation functions.
In order to investigate dynamics in the simulated systems, self-intermediate scattering functions were calculated. In the MD simulations, these functions were computed simultaneously with van Hove's time-dependent correlation functions as per the following equation:72 | Fs(q,t) = N−1〈δρ(q,t)δρ(q,0)〉 | (4) |
where δρ(q,t) = exp(iq·rk(t)), N is the number of particles, q is the wave vector, t is time and rk is the position of particle k.
When calculating the characteristics of motion of the components, groups of interest were selected (instead of whole molecules) in order to avoid high memory costs. Water was selected as a whole group in both simulated systems. For the lipids, both the CH3 group at the end of the tail and the CH2 group next to the carbonyl of the head group were selected, as these groups were common for all lipids.
The rotational motions of the lipid tail were characterised using the rotational time correlation functions (RTCF),73 which can be expressed in its general form using the nth order Legendre polynomial by the following equation:
|  | (5) |
where
Pn is the
nth order Legendre polynomial,
ξ is the time origins,
t is the time-point,
p is the chosen vector. Here the 2
nd order Legendre polynomial was presented.
Error bars for the NSE data represent ±standard deviation and for the simulation data represent ±0.01 standard deviation.
Results and discussion
Dynamic regimes in the sponge phase
Lipid nanoparticles are complex structures where the dynamic footprint reflects the sum of many different types of motions and the dominant bilayer motion depends on the length and time scale studied.6 The Zilman and Granek model describes the regime in which thermal undulations dominate, however, when moving away from this regime, other motions must also be considered. Fig. 1 and the analysis of the data within it are divided and discussed according to these boundary conditions. Previous SANS data reported by Valldeperas et al.,74 for sponge phase nanoparticles with the same lipid composition as in the present study, but with deuterated P80, featured two diffuse peaks at ≈0.05 Å−1 and ≈0.11 Å−1. These peaks were assigned to the L3 cell–cell correlation length and the bilayer thickness, respectively. These q values agree well with previously recorded SAXS data for the bulk sponge phases containing both enzymes measured here, therefore these q values were used to inform the present analysis.55,56
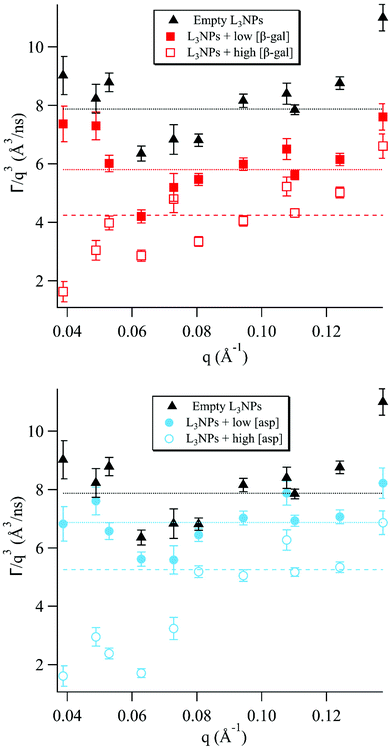 |
| Fig. 1 The normalised relaxation rate Γ/q3vs. q was plotted for sponge phase nanoparticles with different concentrations of encapsulated enzymes. The horizontal lines show the fit of the Zilman and Granek model for the relaxation rate Γ/q3 for bending fluctuations (eqn (2)) to the experimental data in the intermediate q range where the model is valid. | |
It should be noted that, although eqn (1) describes most of the ISFs well, there are also decays for which this is not a good fit. This can be seen, for example, for q = 0.0805 Å−1 in Fig. S3e† showing the ISF for empty L3NPs. It can be assumed that there are additional motions in the sponge phase at this length and time scale, which are not described well by this model. Additionally, this model does not take into account the proteins included in 4 of the 5 samples investigated, which are also likely to contribute directly to the decay curves as well as by affecting the membrane motion.
Low q (q < 0.07 Å−1).
From Fig. 1, it is clear that, for all samples, below 0.07 Å−1, the relaxation rate Γ is not proportional to q3, indicating that this length scale is outside of the range in which the Zilman and Granek model is applicable. When defining their model, Zilman and Granek averaged over an ensemble of single, approximately flat membrane plaquettes of linear size L. The lower q limit for the model, as applied to the sponge phase, naturally corresponds to the unit cell size, as it is assumed that the probe wavelengths are smaller than the model patch size L.62 This q range interrogates a length approaching the sponge phase cell–cell correlation peak in the average static structure, ≈0.05 Å−1 from previous SANS and SAXS measurements. Dynamics in this range are therefore more likely to be dominated by topological membrane changes.36
The sponge phase has a highly dynamic mesoscopic structure, as the bulk phase has a relatively low viscosity and fluid acyl chains, allowing easy making and breaking of water channels.75 It is most likely this motion that results in the high degree of dynamics observed for the samples with the low enzyme concentration and without enzyme in this q range.
The inclusion of both enzymes causes a reduction of the dynamics, due to stiffening of the membrane discussed in a later section, which increases with enzyme concentration. The rate of breaking and reforming the water channels will then naturally be slower compared to the empty sponge phase, as observed for the lower concentrations of both enzymes. For the higher concentrations, however, a more dramatic decrease was observed. This can be discussed in terms of the effect of the enzymes on the lipid phase structure, as it has previously been observed that increasing the concentration of encapsulated enzyme, both in the case of aspartic protease and β-galactosidase, decreases the sponge phase unit cell dimension and for aspartic protease eventually causes a transition to the bicontinuous cubic phase.55,56
The cubic phase structure is more highly ordered and rigid, with slightly less fluidity in the acyl chains and bulk viscoelastic properties similar to a stiff gel, compared to the more fluid sponge phase.75,76 This results in well-defined Bragg peaks in SANS and SAXS diffractograms of cubic phases. Often in scattering studies of dynamics in complex systems, a minimum in dynamics has been observed in proximity to a static structure peak, a phenomenon known as de Gennes narrowing.77 For the higher aspartic protease concentration, which is close to the cubic phase transition boundary, there is a minimum in the Γ/q3versus q plot at q ≈ 0.06 Å−1. This is likely to be a consequence of de Gennes narrowing, which would be much more pronounced for this concentration due to the proximity to the cubic phase transition.
For the higher β-galactosidase concentration, this minimum is likely a result of the large increase in stiffness of the membrane. This can be attributed to partial disruption of the bilayer structure by the enzyme, as discussed in more detail in a later section.
Intermediate q (0.07 Å−1 ≤ q ≤ 0.13 Å−1).
In this range, the bending relaxation rate is proportional to q3, therefore the data agrees well with the Zilman and Granek model (Fig. S4†). It can be observed that increasing the concentration of the enzyme in both cases results in reduced dynamics, more so for β-galactosidase than aspartic protease, indicating a decrease in membrane undulations and stiffening of the membrane. In this region, eqn (2) could be fitted to the data, in order to calculate the bending modulus κ for each sample, as discussed further below.
High q (q > 0.13 Å−1).
In their model, Zilman and Granek describe the system using the Helfrich bending free energy, which uses a continuum model to describe the bilayer. This breaks down in the upper q limit, as the length scale approaches the bilayer thickness, previously determined for this system to be 46 Å, and the finite thickness of the bilayer and thickness fluctuations become important.30,51,52 In multiple NSE studies of lipid membranes, a peak has been observed near the length scale of the membrane thickness, with the excess dynamics assigned to thickness fluctuations.39,60,78,79 Although a similar analysis here is not possible due to the limited q range studied and lack of neutron scattering contrast, a difference can be observed between samples with and without enzyme; the addition of the enzymes clearly results in reduced dynamics. It has previously been observed that the enzymes penetrate into the bilayer, thereby disrupting the acyl chain movement.56,80 It is possible that these interactions between the lipid and the protein, attractive or not, dampen the thickness fluctuations in the bilayer.
Membrane bending moduli
The membrane bending moduli κ for the L3NPs were calculated from fits of eqn (2) to the normalised relaxation rate Γ/q3 in the q range where the Zilman and Granek model is valid (the non-normalised values are plotted in Fig. S5†). The bending rigidities were normalised to the value for the empty sample and are presented plotted against final enzyme concentration in the sponge phase (Fig. 2). As shown in Fig. 2, the increase in the bending modulus κrel with increasing concentration of enzyme is larger when encapsulating β-galactosidase than aspartic protease. The need to consider the effect of specific lipid–protein interactions on membrane rigidity has previously been demonstrated by Agrawal et al.81 They calculated an identical increase in bending modulus of a membrane upon inclusion of a protein modelled as a rigid inclusion, dependant only on protein concentration. The importance of protein specificity is supported by experimental observations in the literature, in which the inclusion of proteins and/or peptides in various lipid membranes has been observed to harden, soften and have no effect on membrane rigidity (ref. 33 and 81 and refs. within).
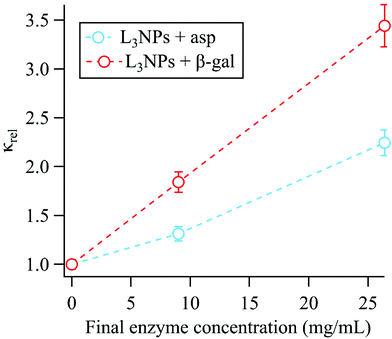 |
| Fig. 2 The membrane bending moduli κrel, extracted from the linear fits of eqn (2) shown in Fig. 1, were normalised to the bending modulus for the empty sponge phase (κempty = 10.8 ± 0.3kbT) and are shown plotted against the final enzyme concentration in the sponge phase nanoparticles. Dotted lines included only to indicate trend. | |
The effect of both aspartic protease and β-galactosidase on the lipid mesostructure has been investigated and the consequent effect on the membrane rigidity can be considered in terms of these interactions.
Aspartic protease (34.6 kDa) has a hydrodynamic diameter in water of 60 Å, compared to the bilayer thickness (46 Å) and the average empty bulk L3 pore size (87 ± 1 Å), therefore could possibly reside completely in the water channels or partially embedded in the bilayer.52,54,56 Previous work by Valldeperas et al.56 showed that when the aspartic protease is encapsulated in the bulk cubic phase, the lipid acyl chain organisation is more disordered and the lipid head group region becomes less hydrated, indicating that the aspartic protease penetrates into the hydrophobic region of the bilayer. This agrees well with complementary neutron reflectometry data for supported lipid bilayers formed from L3NP components, where the aspartic protease was found to gradually penetrate into the lipid bilayer. A perturbation of the acyl chain region and the outer head group layer was observed, leading to an overall thickening of the bilayer like structure.80 Additionally, this penetration results in bilayer asymmetry, which is likely the reason for increasing the bilayer curvature towards the aqueous phase and triggering the transition to cubic phase. As previously mentioned, the cubic phase has a more ordered structure with less fluid acyl chains, therefore a stiffer bilayer.56 It is likely a combination of these effects that causes an increase in bending rigidity upon addition of aspartic protease to the sponge phase.
β-Galactosidase is significantly larger than aspartic protease with the native tetrameric form having a molecular weight of 476 kDa. Under the conditions used here, the enzyme is present as a dimer (238 kDa) with a hydrodynamic diameter of 118 Å. In this case, the enzyme is larger than both the bilayer thickness and the average empty bulk L3 pore size (87 ± 1 Å), therefore, based on size alone, it is most likely that the enzyme resides partially in the water channel and partially in the bilayer.52,55 As the enzyme retains its high activity when encapsulated in the sponge phase with a water soluble substrate, it follows that the active site is exposed to the water channel.55,56 SAXS data for the bulk phase show that addition of β-galactosidase does not cause a phase transition from the sponge phase but does result in a decrease of unit cell size, which is most likely due to dehydration of the lipid head groups.55 Neutron reflectometry data also shows that adding β-galactosidase to a supported bilayer formed from L3NP components causes a thickening of the lipid layer. In addition, the enzyme interaction eventually leads to structural change in the original layer such that a multilayer is formed with a repeat distance of 95 Å.80 It is likely that this thickening contributes to the increase in bending rigidity, however the perturbation of the original bilayer structure is also likely to have an effect.
In addition, the part of the enzyme in the water channel could act similarly to a peripheral protein, which is a protein that is associated with a membrane but is not an integral part of its function.82 Here the relatively high flexibility of the sponge phase is likely to be important. It has been observed that adsorbed or peripheral proteins can rigidify the membrane.33 This was demonstrated in work by Ratanabanangkoon et al.,83,84 where the binding of non-crystalline avidin to biotinylated bilayers and the formation of streptavidin crystals on giant vesicles was investigated. For both systems this resulted in increased membrane rigidity. We also note that for such a large enzyme as β-galactosidase, the free volume available in the water channel for the membrane bending motion will be reduced, which should reduce the motion. Hence, both the parts of the β-galactosidase residing in the water channel and in the bilayer could contribute to the increase in membrane bending rigidity. It is therefore logical that the effect of β-galactosidase on increasing the bending rigidity is larger than for aspartic protease.
Self-intermediate scattering functions and correlation functions
In order to further understand the nature of the interactions between the system components, molecular dynamics (MD) simulations were performed. Neutron scattering techniques provide average values for a system (although different parts can be differently weighted by isotope substitutions), whereas, in MD simulations, functions for various parts of a system can easily be obtained. In simulations, the position of each particle is calculated directly, therefore it is possible to examine the contributions from specific (parts of) compounds of interest and what affects their relaxation rates. Here we focus on the effect of the addition of a molecule of aspartic protease on the relaxation of water and two groups in the lipid tail, as well as the relaxation of the protein itself.
Fig. 3a shows the q-dependent dynamics of water with and without the protein. It can be seen that the inverse relaxation time exhibits a nearly perfect q2 dependence in both systems, indicating that the water dynamics are mainly characterised by translational diffusion. Furthermore, it is evident that the introduction of the protein slows down the water dynamics. This slowing down is caused by the fact that the protein becomes hydrated by water, which, in turn, dehydrates the lipids, as demonstrated in Table S1,† which shows the hydrogen bonds in the simulated systems with and without protein. Thus, the amount of fast “bulk-like” water decreases and the amount of slower “quasi-bound” hydration water increases, which increases the average relaxation time of the water in the protein containing system.
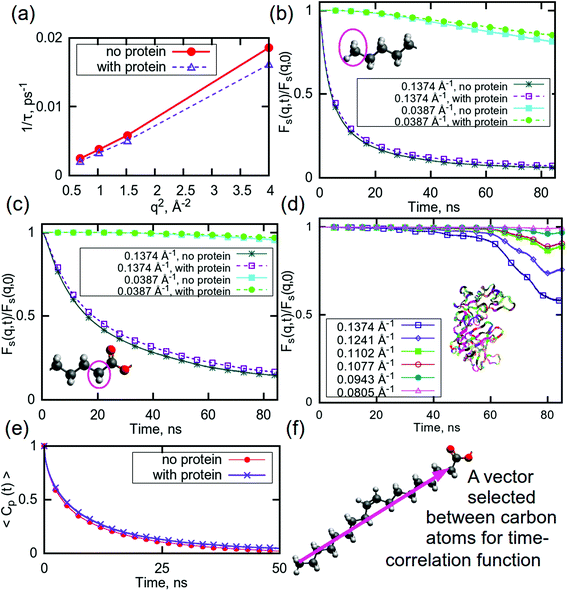 |
| Fig. 3 (a) Relaxation time for water plotted against q for high q-values. Self intermediate scattering (SIS) functions for (b) CH3 groups in lipid tails, (c) CH2 groups in lipid tails, and (d) aspartic protease. (e) Rotational time correlation functions (RTCF) computed using the second order Legendre polynomial for a chosen vector in DGMO molecule, as shown in (f). Colours on molecules indicate the following atoms: red – oxygen, grey– hydrogen, dark grey – carbon. The ribbons on (d) show the protein. For SIS functions for the full q range, see ESI (Fig. S9†). Error bars are within the thickness of the plotted lines. | |
This is also the case for selected parts of the lipid tails: the presence of aspartic protease slows down the relaxation for both selected CH3- and CH2-groups (Fig. 3b and c). The CH3-group exhibits a faster relaxation than the CH2-group for the same q-values, which can likely be explained by the possibility of hydrogen bonding between carbonyl oxygens and water, which are located close to selected CH2-groups, thereby restricting their motion. As previously mentioned, it is probable that these carbonyl groups can bind to the protein, which could be a reason behind the slower relaxation in the system with aspartic protease. These findings are also supported by a Raman spectroscopy study of the same system, which indicates reduced water binding in the carbonyl region as well as direct binding of the aspartic protease.56
In this simulation, the relaxation of the protein itself has also been considered. Fig. 3d shows the relaxation curves for the protein at selected q-values. This process is slow enough that it was not possible to observe the full relaxation of the aspartic protease within the time frame investigated here, especially at the lowest q values. Although the decay is initially negligible for all q values, after 40 ns, there was observable relaxation at the higher q values. The shape of the decay curves suggests a complex, multi-step relaxation process with many contributing motions. This is not surprising as it is well-known that proteins exhibit a wide variety of motions ranging from bond vibrations through to side chain rotation and large conformational changes, such that protein relaxation takes place over a wide range of length and time scales.85,86
Slow dynamics can be observed in the secondary structure of the protein also, as shown in Fig. S8 (ESI†). There are very minor changes occurring in structures of some residues, but the largest part of aspartic protease remains in the same conformations during the last 100 ns of the simulation time, indicating that, although the protein is moving, the secondary structure is stable during this simulation time and no unfolding event occurs. This is consistent with our previous Raman spectroscopy study that shows minute effects on the aspartic protease signature peaks in the spectra, indicating no significant structural changes.56
The rotational motion of the lipid tails was additionally characterised using rotational time correlation functions (RTCFs). Fig. 3e shows example RTCFs for the selected vector between carbons in the lipid tails (Fig. 3f) for DGMO lipids, as the RTCFs had the same shape for P80 and the GMO-50 lipid tails. Considering that the lipid tail is not rigid, it can be concluded that the presence of aspartic protease slows down the rotational motion of lipid tails. This behaviour confirms the observation of decreased flexibility of the acyl chains due to the addition of the protein and suggests the possibility of direct interaction between the lipid tails and the protein.
Pair correlation functions
Pair correlation functions (PCFs) show the probability of finding a particle at a certain distance from another particle. Here, a particle can be defined as an atom, a molecule, or a group of atoms in a molecule. In the case that the particle is made up of multiple atoms, the distance is between the centres of mass of the particles. In order to understand which parts of the protein can bind to the lipid tails, PCFs were calculated for the centres of mass of all of the amino acids in aspartic protease and carbonyl groups from all of the lipid tails. The carbonyl groups were selected due to their ability to be involved in hydrogen bonding, possibly with various parts of the amino acids, and as peaks corresponding to these groups showed the largest change upon addition of aspartic protease in a Raman study of the same system.56 Significant peaks (i.e. with g(r) > 1) were observed for 14 amino acids at a distance of less than 8 Å (Fig. 4a and b), indicating that these residues may undergo hydrogen bonding with lipid carbonyl groups, which is consistent with the results from the aforementioned Raman study of the same system.56 This was investigated further by calculating hydrogen bonds, using a threshold of 3 Å, between the following groups of atoms: lipid head groups, lipid head groups and protein, lipid head groups and water, and protein and water (see Table S1 in ESI†). The number of hydrogen bonds between lipids and water was significantly lower (almost 100 hydrogen bonds less, with the total number of lipids equal to 248) for P80 in the system containing the protein, while for all other lipids the difference in the number of hydrogen bonds was rather insignificant for the two simulated mixtures. Considering the lipid–protein interactions from Table S1,† it can be observed that the head groups of P80, DGMO and GMO-50 bind preferentially to the protein, while di- and triglycerides do not show any binding. This could help explain how the protein affects the motion of lipids which resulted in a slower relaxation, as discussed earlier.
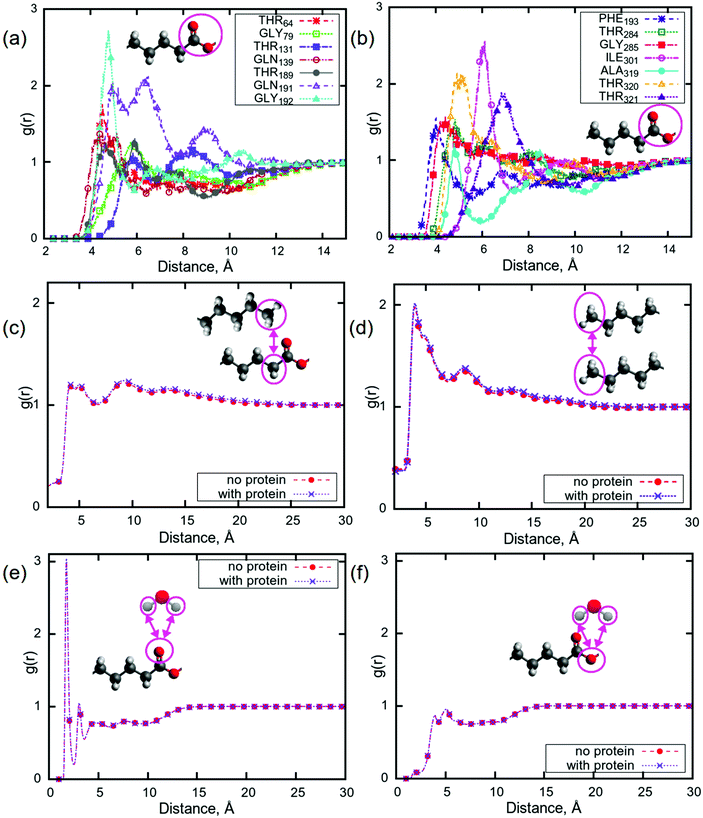 |
| Fig. 4 Pair correlation functions (a) and (b) between centre of mass of amino acids in protein and the carbonyl groups in lipids; (c) between centre of mass of CH3- and CH2-groups in lipid tails; (d) between CH3-groups in lipid tails; (e) and (f) are PCFs between selected oxygens in carbonyl groups and hydrogens in water. Colours on molecules were the following: red – oxygen, grey – hydrogen, dark grey – carbons. Amino acids are labelled with their number in the sequence from the structure from the PDB. | |
Such a binding of the carbonyl groups to the amino acids raises the question of the potential effect of the presence of the aspartic protease on the structure of the lipid membrane. In Fig. 4c, PCFs between the centres of mass of CH2- and CH3-groups at the beginning and the end of the tail, respectively, are presented. There are two well-distinguished significant peaks with the values of g(r) more than 1 at distances of 5 Å and 8 Å, and a third, less pronounced local maximum at approximately 13 Å. The appearance of the peaks at these distances indicate that this lipid membrane may have a different structure than that of phospholipid bilayers,87,88i.e. the leaflets in sponge phase lipid membranes are not well separated and the membrane is quite disordered. This is demonstrated by a comparison between Fig. 4(c) and (d), which show the PCFs between CH3- and CH2-groups and between CH3-groups, respectively. Fig. 4(d) shows that the CH3-groups can be located at the distance of approximately 5 Å apart with the highest probability and with lower probabilities at the distances of 8 Å and 13 Å. Comparing to Fig. 4(c), the positions of the peaks for CH3-groups (distances) are the same as in the case of PCFs between CH2- and CH3-groups. Moreover, the presence of the protein does not seem to affect the structure of the sponge membrane.
The behaviour of water molecules at the lipid–water interface is also of interest, as it was observed that the relaxation for water is slower in the presence of aspartic protease than in its absence. Fig. 4(e and f) shows PCFs between hydrogens in water molecules and the selected carbonyl oxygens in lipid tails, which were the same in all 5 lipids. There are no differences between computed g(r) for the systems with and without protein. As in the case of phospholipid bilayers there may be hydrogen bonds between carbonyl oxygens and water molecules, which could imply that the sponge membrane ‘holds’ due to the existence of water bridges.89,90 As the PCFs are the same for the systems with the protein as without, this indicates that, although the presence of the protein can disrupt these hydrogen bonds, they constitute only a small proportion of the water bridges, and it is the hydrophobic interactions between the acyl-chains that maintains the integrity of the sponge phase. The sponge phase structure is therefore retained, even with protein.
Proposed membrane structure
Although sponge phase lipid nanoparticles are disordered systems, it is still possible to understand their overall structure. Particularly from MD simulations, we can attempt to build a hypothesis about the lipid membrane in the inner part of such a nanoparticle. Fig. 5(a) shows small angle X-ray scattering profiles (SAXS) for both simulated systems (with and without the protein). Calculated profiles are very similar and have a correlation peak at q ≈ 0.14 Å−1. Recalling the PCFs between CH2- and CH3-groups and between CH3-groups, the peak at the distance of 13 Å cannot be related to this peak in the SAXS data. Considering the snapshot in Fig. 5(b) and the disordered structure of the membrane, it can be concluded that correlation peaks from PCFs at distances of about 13 Å may be related to the thickness of a hydrophobic part of the membrane (see Fig. S11†), which is not situated in membrane ‘stacking’ or monolayer thickness, while the correlation peak at a q-value of 0.14 Å−1 is most likely reflecting the bilayer thickness. When additionally considering the PCFs between the water molecules and carbonyl oxygens, which demonstrated a strong potential for hydrogen bonding to the water hydrogens, a schematic structure can be drawn for those thinner membranes, which is shown in Fig. 5(c). We call it a schematic structure, because unsaturated lipid tails do not exist in the bilayer in such a stretched form: they are instead very disordered.91,92
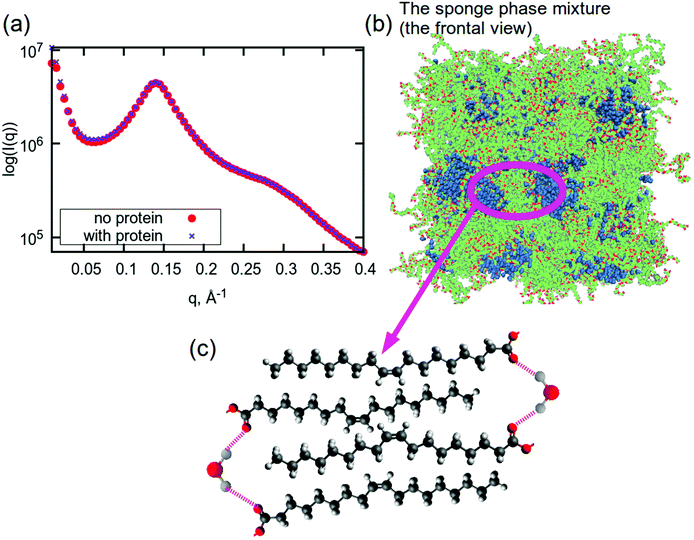 |
| Fig. 5 (a) Small angle X-ray scattering profiles for sponge phase system with and without aspartic protease from MD simulations. (b) A snapshot of a frontal view of the simulated system. A snapshot of the simulated system with protein is shown in Fig. S10.† (c) Proposed schematic structure of lipid tails and water in the sponge membrane. Colours in (b): hydrocarbon lipid tails are green, red atoms are oxygen atoms in lipids, blue molecules are water. Colours in (c): red – oxygen, grey – hydrogen, dark grey – carbon. | |
Nevertheless, some limitations can be named here comparing to the experimental results obtained by Valldeperas et al.,74 which have seen correlation peaks at the q value of about 0.11 Å−1 and related them to bilayer thickness. The sponge phase is a highly disordered phase, as demonstrated by the characteristic broad peaks in its SAXS pattern, therefore there can be significant variation between local regions. Consequently, it is important to consider a sufficiently large sample in order to determine a representative average over the whole system, something that is challenging to achieve in MD simulations due to high computational costs. Additionally, the experimental results are based on the total average values for all L3NPs in the sample volume, whereas the simulations focussed only on the inner structure of a nanoparticle. As a result, it is possible that the amount of water in the MD simulations was different from experiment, which could have an effect on the resulting bilayer thickness. Another dissimilarity could be the amount of the protein: in the simulation, there was only one protein molecule included in the system, due to high computational costs. In the experimental sample, however, it is unlikely that the protein molecules are completely homogeneously distributed throughout the L3NPs, such that there could be L3NPs loaded with several protein molecules and some that contain no protein at all.
Conclusions
In this work, we have for the first time revealed the dynamics of lipid sponge phase nanoparticles with and without the inclusion of the industrially relevant enzymes aspartic protease and β-galactosidase using a combination of experimental and simulation tools. The characterisation of the dynamics of the L3NPs using neutron spin echo spectroscopy demonstrated that the inclusion of both enzymes resulted in reduced dynamics at all length scales measured and that the magnitude of this reduction was dependent on the specific enzyme and its concentration. Using molecular dynamics simulations, it was shown that these reduced dynamics for the system containing aspartic protease were most likely a result of hydrogen bonding between amino acids in the protein and carbonyl groups in the lipid head groups replacing water–carbonyl hydrogen bonds. Additionally, we were able to propose a general schematic structure for the bilayer of the sponge phase from these simulated results. This work has allowed further insight into the relationship between structure and dynamics in a complex lipid system, which is vital to the understanding of the biological function and interactions of LNPs, as well as contributing lipid force fields for the simulation of such systems in the future. For future work, more extensive studies of the system with varying electrolyte concentration and pH will allow us to investigate their effect on the protein–lipid interactions observed here.
Disclaimer
The identification of any commercial product or trade name does not imply endorsement or recommendation by the National Institute of Standards and Technology.
Author contributions
T. N. and M. N. conceptualised the research and planned the NSE experiments and I. E. and J. S. planned the simulations. I. E. designed and performed the simulations and analysed the simulation data. All authors were involved in NSE measurements. J. G. analysed and interpreted NSE data with support from M. N. J. G. and I. E. wrote the manuscript, with critical feedback provided by all the authors.
Conflicts of interest
There are no conflicts to declare.
Acknowledgements
Access to the NGA-NSE instrument was provided by the Center for High Resolution Neutron Scattering, a partnership between the NIST and the National Science Foundation under agreement no. DMR-2010792. The authors thank M. Valldeperas Badell for her valuable input and fruitful discussions regarding experimental design and A. Ridolfi for the use of his render file and indispensable help with plotting the unit cell structures. The project is financed by the Swedish-German Röntgen-Ångström Cluster MEDISOFT. We thank Swedish National Infrastructure for Computing (SNIC) for the following computational and storage resources SNIC2020/5-58, SNIC2020/13-38, SNIC2020/10-22, SNIC2021/5-85, SNIC2020/6-53, SNIC2021/5-80, SNIC2020/5-514. We also thank Å. Sandgren from HPC2N at for the application support.
Notes and references
-
J. M. Seddon and R. H. Templer, Handbook of Biological Physics, Elsevier SPC, 1995, vol. 1, pp. 97–160 Search PubMed.
- S. T. Hyde and G. E. Schröder, Curr. Opin. Colloid Interface Sci., 2003, 8, 5–14 CrossRef CAS.
- X. Mulet, B. J. Boyd and C. J. Drummond, J. Colloid Interface Sci., 2013, 393, 1–20 CrossRef CAS PubMed.
- B. Tenchov and R. Koynova, Eur. Biophys. J., 2012, 41, 841–850 CrossRef CAS PubMed.
- C. V. Kulkarni, W. Wachter, G. Iglesias-Salto, S. Engelskirchen and S. Ahualli, Phys. Chem. Chem. Phys., 2011, 13, 3004–3021 RSC.
- G. Brannigan and F. L. H. Brown, Biophys. J., 2006, 90, 1501–1520 CrossRef CAS PubMed.
- J. N. Israelachvili and H. Wennerstroem, J. Phys. Chem., 1992, 96, 520–531 CrossRef CAS.
- H. Bouvrais, Advances in Planar Lipid Bilayers and Liposomes, 2012, 15, 1–75 CAS.
-
R. B. Gennis, Biomembranes: Molecular Structure and Function, Springer-Verlag New York, 1st edn, 1989, ch. 5, pp. 166–198 Search PubMed.
- H. P. Duwe and E. Sackmann, Physica A, 1990, 163, 410–428 CrossRef CAS.
- H. Seto, N. L. Yamada, M. Nagao, M. Hishida and T. Takeda, Eur. Phys. J. E, 2008, 26, 217–223 CrossRef CAS PubMed.
- W. Helfrich, Z. Naturforsch., A: Phys., Phys. Chem., Kosmophys., 1978, 33, 305–315 Search PubMed.
-
G. Cevc and D. Marsh, Phospholipid Bilayers: Physical Principles and Models, Wiley, 1987 Search PubMed.
- R. P. Rand and V. A. Parsegian, Biochim. Biophys. Acta, Rev. Biomembr., 1989, 988, 351–376 CrossRef CAS.
-
J. N. Israelachvili, Intermolecular and Surface Forces, Academic Press London, 2nd edn, 1991 Search PubMed.
- J. N. Israelachvili, S. Marčelja and R. G. Horn, Q. Rev. Biophys., 1980, 13, 121–200 CrossRef CAS PubMed.
- J. M. Seddon, Biochim. Biophys. Acta, Rev. Biomembr., 1990, 1031, 1–69 CrossRef CAS.
- A. Srivastava and A. Debnath, J. Chem. Phys., 2018, 148, 94901 CrossRef.
- G. Cevc, Chem. Phys. Lipids, 1991, 57, 293–307 CrossRef CAS PubMed.
-
Z. A. Almsherqi, T. Landh, S. D. Kohlwein and Y. B. T. Deng, International Review of Cell and Molecular Biology, Academic Press, 2009, vol. 274, ch. 6, pp. 275–342 Search PubMed.
- E. L. Snapp, R. S. Hegde, M. Francolini, F. Lombardo, S. Colombo, E. Pedrazzini, N. Borgese and J. Lippincott-Schwartz, J. Cell Biol., 2003, 163, 257–269 CrossRef CAS PubMed.
- W. Basu Ball, J. K. Neff and V. M. Gohil, FEBS Lett., 2018, 592, 1273–1290 CrossRef CAS PubMed.
- E. Beltrán-Heredia, F.-C. Tsai, S. Salinas-Almaguer, F. J. Cao, P. Bassereau and F. Monroy, Commun. Biol., 2019, 2, 225 CrossRef PubMed.
- F. Elías-Wolff, M. Lindén, A. P. Lyubartsev and E. G. Brandt, Soft Matter, 2019, 15, 792–802 RSC.
- H. T. McMahon and E. Boucrot, J. Cell Sci., 2015, 128, 1065–1070 CrossRef CAS PubMed.
- B. Westermann, Nat. Rev. Mol. Cell Biol., 2010, 11, 872–884 CrossRef CAS PubMed.
- D. Marsh, Chem. Phys. Lipids, 2006, 144, 146–159 CrossRef CAS PubMed.
- E. Lindahl and O. Edholm, Biophys. J., 2000, 79, 426–433 CrossRef CAS PubMed.
- M. Nagao, Phys. Rev. E: Stat., Nonlinear, Soft Matter Phys., 2009, 80, 31606 CrossRef PubMed.
- W. Helfrich, Z. Naturforsch., C: Biochem., Biophys., Biol., Virol., 1973, 28, 693–703 CrossRef CAS PubMed.
- I. Hoffmann, Colloid Polym. Sci., 2014, 292, 2053–2069 CrossRef CAS.
- M. Doktorova, D. Harries and G. Khelashvili, Phys. Chem. Chem. Phys., 2017, 19, 16806–16818 RSC.
- R. Dimova, Adv. Colloid Interface Sci., 2014, 208, 225–234 CrossRef CAS PubMed.
- M. Mihailescu, M. Monkenbusch, H. Endo, J. Allgaier, G. Gompper, J. Stellbrink, D. Richter, B. Jakobs, T. Sottmann and B. Farago, J. Chem. Phys., 2001, 115, 9563–9577 CrossRef CAS.
- B. Farago, M. Monkenbusch, K. D. Goecking, D. Richter and J. S. Huang, Phys. B, 1995, 213–214, 712–717 CrossRef.
- M. Monkenbusch, O. Holderer, H. Frielinghaus, D. Byelov, J. Allgaier and D. Richter, J. Phys.: Condens. Matter, 2005, 17, S2903–S2909 CrossRef CAS.
-
M. Telling, Dynamics of Biological Macromolecules by Neutron Scattering, Bentham Science Publishers Ltd., 2011, pp. 4–21 Search PubMed.
- Z. Yi, M. Nagao and D. P. Bossev, J. Phys.: Condens. Matter, 2009, 21, 155104 CrossRef PubMed.
- R. Ashkar, M. Nagao, P. D. Butler, A. C. Woodka, M. K. Sen and T. Koga, Biophys. J., 2015, 109, 106–112 CrossRef CAS PubMed.
- M. C. Watson, Y. Peng, Y. Zheng and F. L. H. Brown, J. Chem. Phys., 2011, 135, 194701 CrossRef PubMed.
- B. Brüning, R. Stehle, P. Falus and B. Farago, Eur. Phys. J. E: Soft Matter Biol. Phys., 2013, 36, 77 CrossRef PubMed.
- T. Takeda, Y. Kawabata, H. Seto, S. Komura, S. K. Ghosh, M. Nagao and D. Okuhara, J. Phys. Chem. Solids, 1999, 60, 1375–1377 CrossRef CAS.
- M. C. Watson and F. L. H. Brown, Biophys. J., 2010, 98, L9–L11 CrossRef CAS PubMed.
- R. Bradbury and M. Nagao, Soft Matter, 2016, 12, 9383–9390 RSC.
- M. Nagao, S. Chawang and T. Hawa, Soft Matter, 2011, 7, 6598–6605 RSC.
- L. R. Arriaga, I. López-Montero, G. Orts-Gil, B. Farago, T. Hellweg and F. Monroy, Phys. Rev. E: Stat., Nonlinear, Soft Matter Phys., 2009, 80, 31908 CrossRef PubMed.
- E. G. Kelley, P. D. Butler, R. Ashkar, R. Bradbury and M. Nagao, Proc. Natl. Acad. Sci. U. S. A., 2020, 117, 23365–23373 CrossRef CAS PubMed.
- E. G. Kelley, P. D. Butler and M. Nagao, Soft Matter, 2021, 17, 5671–5681 RSC.
- M. Stepniewski, M. Pasenkiewicz-Gierula, T. Róg, R. Danne, A. Orlowski, M. Karttunen, A. Urtti, M. Yliperttula, E. Vuorimaa and A. Bunker, Langmuir, 2011, 27, 7788–7798 CrossRef CAS PubMed.
- A. K. K. Leung, I. M. Hafez, S. Baoukina, N. M. Belliveau, I. V. Zhigaltsev, E. Afshinmanesh, D. P. Tieleman, C. L. Hansen, M. J. Hope and P. R. Cullis, J. Phys. Chem. C, 2012, 116, 18440–18450 CrossRef CAS PubMed.
- D. Argudo, N. P. Bethel, F. V. Marcoline and M. Grabe, Biochim. Biophys. Acta, Biomembr., 2016, 1858, 1619–1634 CrossRef CAS PubMed.
- M. Valldeperas, M. Wiśniewska, M. Ram-On, E. Kesselman, D. Danino, T. Nylander and J. Barauskas, Langmuir, 2016, 32, 8650–8659 CrossRef CAS PubMed.
- Á. Pereira-Rodríguez, R. Fernández-Leiro, M. I. González-Siso, M. E. Cerdán, M. Becerra and J. Sanz-Aparicio, J. Struct. Biol., 2012, 177, 392–401 CrossRef PubMed.
- P. T. Erskine, L. Coates, S. Mall, R. S. Gill, S. P. Wood, D. A. A. Myles and J. B. Cooper, Protein Sci., 2003, 12, 1741–1749 CrossRef CAS PubMed.
- J. Gilbert, M. Valldeperas, S. K. Dhayal, J. Barauskas, C. Dicko and T. Nylander, Nanoscale, 2019, 11, 21291–21301 RSC.
- M. Valldeperas, M. Talaikis, S. K. Dhayal, M. Velička, J. Barauskas, G. Niaura and T. Nylander, Biophys. J., 2019, 117, 829–843 CrossRef CAS PubMed.
-
B. Law and P. Goodenough, Enzymes in Food Processing, Springer, Boston, MA, 1995, pp. 114–143 Search PubMed.
-
N. Rosov, S. Rathgeber and M. Monkenbusch, Neutron Spin Echo Spectroscopy at the NIST Center for Neutron Research, 1999 Search PubMed.
- R. T. Azuah, L. R. Kneller, Y. Qiu, P. L. W. Tregenna-Piggott, C. M. Brown, J. R. D. Copley and R. M. Dimeo, J. Res. NIST, 2009, 114, 341–358 CrossRef CAS PubMed.
- M. Nagao, E. G. Kelley, R. Ashkar, R. Bradbury and P. D. Butler, J. Phys. Chem. Lett., 2017, 8, 4679–4684 CrossRef CAS PubMed.
- I. Hoffmann, Front. Phys., 2021, 8, 602 Search PubMed.
- A. G. Zilman and R. Granek, Phys. Rev. Lett., 1996, 77, 4788–4791 CrossRef CAS PubMed.
- I. Ermilova and A. P. Lyubartsev, J. Phys. Chem. B, 2016, 120, 12826–12842 CrossRef CAS PubMed.
- J. B. Klauda, R. M. Venable, J. A. Freites, J. W. O'Connor, D. J. Tobias, C. Mondragon-Ramirez, I. Vorobyov, A. D. MacKerell Jr. and R. W. Pastor, J. Phys. Chem. B, 2010, 114, 7830–7843 CrossRef CAS PubMed.
- W. L. Jorgensen, J. Chandrasekhar, J. D. Madura, R. W. Impey and M. L. Klein, J. Chem. Phys., 1983, 79, 926–935 CrossRef CAS.
- G. Bussi, D. Donadio and M. Parrinello, J. Chem. Phys., 2007, 126, 14101 CrossRef PubMed.
-
H. Berendsen, Computer Simulation in Materials Science, Springer, Dordrecht, 1991, pp. 139–155 Search PubMed.
- G. J. Martyna, D. J. Tobias and M. L. Klein, J. Chem. Phys., 1994, 101, 4177–4189 CrossRef CAS.
- B. Hess, H. Bekker, H. J. C. Berendsen and J. G. E. M. Fraaije, J. Comput. Chem., 1997, 18, 1463–1472 CrossRef CAS.
- W. F. Van Gunsteren and H. J. C. Berendsen, Mol. Simul., 1988, 1, 173–185 CrossRef.
- C. Kutzner, S. Páll, M. Fechner, A. Esztermann, B. L. de Groot and H. Grubmüller, J. Comput. Chem., 2019, 40, 2418–2431 CrossRef CAS PubMed.
- G. D. Harp and B. J. Berne, Phys. Rev. A, 1970, 2, 975–996 CrossRef.
- G. Hinze, G. Diezemann and T. Basché, Phys. Rev. Lett., 2004, 93, 203001 CrossRef CAS PubMed.
- M. Valldeperas, A. P. Dabkowska, G. K. Pálsson, S. Rogers, N. Mahmoudi, A. Carnerup, J. Barauskas and T. Nylander, Soft Matter, 2019, 15, 2178–2189 RSC.
- M. Talaikis, M. Valldeperas, I. Matulaitienė, J. L. Borzova, J. Barauskas, G. Niaura and T. Nylander, J. Phys. Chem. B, 2019, 123, 2662–2672 CrossRef CAS PubMed.
- P. Pitzalis, M. Monduzzi, N. Krog, H. Larsson, H. Ljusberg-Wahren and T. Nylander, Langmuir, 2000, 16, 6358–6365 CrossRef CAS.
- P. G. De Gennes, Physica, 1959, 25, 825–839 CrossRef CAS.
- A. C. Woodka, P. D. Butler, L. Porcar, B. Farago and M. Nagao, Phys. Rev. Lett., 2012, 109, 58102 CrossRef PubMed.
- M. Nagao, J. Chem. Phys., 2011, 135, 74704 CrossRef PubMed.
-
M. Valldeperas Badell, Ph.D. thesis, Lund University, 2019.
- H. Agrawal, M. Zelisko, L. Liu and P. Sharma, Sci. Rep., 2016, 6, 25412 CrossRef CAS PubMed.
-
B. A. Seaton and M. F. Roberts, Biological Membranes: A Molecular Perspective from Computation and Experiment, Birkhäuser Boston, Boston, MA, 1996, pp. 355–403 Search PubMed.
- P. Ratanabanangkoon, M. Gropper, R. Merkel, E. Sackmann and A. P. Gast, Langmuir, 2002, 18, 4270–4276 CrossRef CAS.
- P. Ratanabanangkoon, M. Gropper, R. Merkel, E. Sackmann and A. P. Gast, Langmuir, 2003, 19, 1054–1062 CrossRef CAS.
- J. A. McCammon, Rep. Prog. Phys., 1984, 47, 1–46 CrossRef.
- H. Jansson and J. Swenson, Biochim. Biophys. Acta, Proteins Proteomics, 2010, 1804, 20–26 CrossRef CAS PubMed.
- T. Róg and M. Pasenkiewicz-Gierula, FEBS Lett., 2001, 502, 68–71 CrossRef.
- T. Róg, K. Murzyn, R. Gurbiel, Y. Takaoka, A. Kusumi and M. Pasenkiewicz-Gierula, J. Lipid Res., 2004, 45, 326–336 CrossRef PubMed.
- M. Pasenkiewicz-Gierula, Y. Takaoka, H. Miyagawa, K. Kitamura and A. Kusumi, J. Phys. Chem. A, 1997, 101, 3677–3691 CrossRef CAS.
- M. Pasenkiewicz-Gierula, T. Róg, K. Kitamura and A. Kusumi, Biophys. J., 2000, 78, 1376–1389 CrossRef CAS PubMed.
- N. Kučerka, S. Tristram-Nagle and J. F. Nagle, J. Membr. Biol., 2006, 208, 193–202 CrossRef PubMed.
- J. Seelig and N. Waespe-Sarcevic, Biochemistry, 1978, 17, 3310–3315 CrossRef CAS PubMed.
Footnote |
† Electronic supplementary information (ESI) available: Additional experimental details; full derivation of modified Zilman and Granek model; intermediate scattering functions for all samples, including a comparison of fits of modified Zilman and Granek model including and excluding diffusion; parameterisation of model lipids; secondary structure evolution of encapsulated aspartic protease; self-intermediate scattering functions for water and selected parts of lipids at full range of q values; fluctutation of lipid tail length throughout simulation time; comparison of hydrogen bonds in simulated system with and without protein. See DOI: https://doi.org/10.1039/d2nr00882c |
|
This journal is © The Royal Society of Chemistry 2022 |