DOI:
10.1039/D1MO00282A
(Research Article)
Mol. Omics, 2022,
18, 57-70
The effect of key DNA methylation in different regions on gene expression in hepatocellular carcinoma†
Received
2nd August 2021
, Accepted 31st October 2021
First published on 16th November 2021
Abstract
Hepatocellular carcinoma (HCC) is a common cancer with high morbidity and mortality. As we all know, the alteration of DNA methylation has a crucial impact on the occurrence of HCC. However, the mechanism of the effect of DNA methylation in different regions on gene expression is still unclear. Here, by computing and analyzing the distribution of differential methylation in 12 different regions in HCC tissues and adjacent normal tissues, not only the hypermethylation of CpG islands and global hypomethylation were found, but also a stable distribution pattern of differential methylation in HCC was found. Then the correlations between DNA methylations in different regions and gene expressions were calculated, and the diversity of correlations in different regions was determined. The key genes of differential methylation and differential expression related to the survival of HCC patients were obtained by using Cox regression analysis, a four-gene prognostic risk scoring model was constructed, and the prognostic performance was well verified. The regions of the differentially methylated CpG sites corresponding to the four key genes were located and their influences on the expression were analyzed. The results indicate that the promoter, first exon, 5′UTR, sixth exon, N_Shore, and S_Shore hypomethylation promotes the expression of key oncogenes, which together lead to the occurrence of HCC. These results might help to study the role of DNA methylation in HCC and provide potential biomarkers for the diagnosis of HCC.
1. Introduction
Hepatocellular carcinoma (HCC) is the sixth most commonly diagnosed and the fourth most common cancer in the world.1 The statistics of HCC patients showed that HCC is the fastest growing cancer among all cancer types, and the 5 year survival rate is the lowest among all diagnosis stages, i.e. only 18%.2 A variety of chronic liver diseases and hepatitis viruses are the inducements, leading to the occurrence and development of HCC. More and more studies have also confirmed that epigenetic modification alterations are a key event in the occurrence and development of HCC. Therefore, studying the epigenetic modification alterations of HCC may provide better diagnostic and treatment tools for HCC.
DNA methylation and histone modification are common epigenetic modifications. The effect of histone modification on gene expression has been analyzed in our previous study,3,4 so here we focus on the analysis of DNA methylation. DNA methylation usually occurs on the 5th carbon atom of cytosine in the CpG sequence to produce 5-methylcytosine. The regions rich in CpG dinucleotides are called CpG islands (CGIs), which are mainly located in the promoter and the first exon (1st-exon) regions, and are the focus of DNA methylation research.5 Recent studies have shown that DNA methylation alterations occur not only in CGIs and promoters, but also in the sequences up to 2 kb from CGIs, which are called CpG island shores.6 Furthermore, the study of DNA methylation has been extended to CpG island shelves, which are at a distance of 2 kb from the upstream and downstream of the CpG island shores.7,8 The DNA methylations in different regions have different effects on gene expressions. In HCC and most cancers, it has been found that specific promoter hypermethylation and global hypomethylation are related to the inactivation of tumor suppressor genes and chromatin instability, respectively.
DNA methylation alterations can lead to HCC. It has been found that certain abnormal DNA methylation can silence the transcription of tumor suppressor genes,9 inhibit the expression of genes related to cell cycle regulation, DNA mismatch repair and apoptosis, and lead to HCC.10 Hypermethylation of the CGI in the promoter region inhibits the expression of RASSF1A, DLC-1, P53, GSTP1, and BCLB, which are related to precancerous lesions, molecular characteristics, and prognosis of HCC.11–15 The hypermethylation inhibits the expression of CCND1, AR, ESR1, and TRIM58, and is related to the occurrence and metastasis of HCC.16–19HOXD10 inhibits HCC through ERK signaling, and its expression is regulated by methylation in the promoter region.20 The promoter methylation levels of IRAK3 and VIM are significantly increased in HCC, which is closely related to tumor stage and size.21,22 These studies indicate that hypermethylation has an inhibitory effect on gene expression. On the other hand, hypomethylation promotes cancer by affecting chromosomal stability. Hypomethylation is usually more obviously related to tumor progression and malignancy.23,24 But, in general, it is thought that DNA methylation has a negative correlation with gene expression. Only a few studies have shown that DNA methylation may have a positive correlation with gene expression. Studies have found that CGI hypermethylation in the gene-body region can predict the increase of gene expression levels in cancer.25 These results indicated that DNA methylation may have multiple effects on gene expression in HCC. However, there are few related studies. In addition, abnormal DNA methylation that results in tumors can also be detected in CpG island shores, CpG island shelves and other regions besides the CGI.8 Therefore, it is of great significance to study DNA methylation alterations in different regions. At present, most studies focus on DNA methylation alterations in the promoter region and CGI; however, studies on methylation in other regions are still lacking.
In this study, in order to understand the effect of DNA methylation in different functional regions on the occurrence of HCC, we analyzed the DNA methylation data of 430 samples in 12 different functional regions. By comparing the differential methylation in different regions, it was found that hypermethylation is significant in the CGI, and abnormal methylation presents a stable distribution state in HCC. Then we obtained the diversity relationship between DNA methylation and gene expression through correlation analysis. Finally, the effect of important genes related to cancer on the survival of HCC patients was analyzed, and the four key genes that are related to survival were obtained. The key regions where the methylation sites of four key genes were located, and the relationships between the DNA methylation of different regions and gene expression were found. Our results reveal methylation biomarkers associated with HCC.
2. Materials and methods
2.1. Data sources
The DNA methylation data (Illumina Infinium Human Methylation 450K), gene expression data, and clinical data of human LIHC (liver hepatocellular carcinoma) tissue samples and adjacent normal tissue samples were downloaded from the TCGA database (https://cancergenome.nih.gov/) (hg38).26 The DNA methylation data include 380 HCC tissue samples and 50 adjacent normal tissue samples. There are 485
577 CpG sites in the DNA methylation data of each sample. After deleting the missing rows of methylation data, the remaining 365
748 CpG sites were used for the subsequent analysis. The count data and normalized FPKM data of gene expression include 374 tumor samples and 50 normal samples, and all expression data samples are included in the DNA methylation data samples. There are 372 clinical data samples corresponding to DNA methylation data and gene expression data, of which 364 samples contain overall survival time and survival status (Table S1, ESI†).
2.2. Division of different regions
The human reference genome annotation file (RefSeq, hg38) was downloaded from the UCSC database (http://genome.ucsc.edu/), and only 19
484 genes at the beginning of NM were reserved for the analysis. Based on the annotation file, the genome was divided into the following different regions. The definition of the promoter is from 1500 bp upstream to 1500 bp downstream of the transcription start site (TSS) (TSS ± 1500 bp). 5′UTR was defined from the TSS to the coding region start site (CDS) (TSS-CDS). 3′UTR was defined from the coding region end site (CDE) to the transcription termination site (TTS) (CDE-TTS). The intergenic region was defined from the TTS of one gene to the TSS of the nearest gene. The enhancer region location file of human was downloaded from the FANTOM5 database (https://fantom.gsc.riken.jp/5/data/).27 The distances from the TSS of each gene to the center of each enhancer region were calculated, and the closest gene to an enhancer was defined as the target gene of that enhancer. The annotation file of the CGI region was downloaded from the UCSC database. Then the 2 kb regions of the CGI boundary were defined as CpG island shores, where the upstream and downstream 2 kb were the north shore (N_Shore) (CGI − 2 kb) and the south shore (S_Shore) (CGI + 2 kb), respectively. The 4 kb regions upstream and downstream of the CpG island were defined as the CpG island shelves, which were the north shelf (N_Shelf) (CGI − 4 kb) and south shelf (S_Shelf) (CGI + 4 kb), respectively.7,8 In short, we divided into a total of 12 regions: promoter, 5′UTR, exon, intron, 3′UTR, intergenic, enhancer, CGI, N_Shore, S_Shore, N_Shelf and S_Shelf regions.
2.3. Analysis of differentially methylated CpG sites (DMCs) and differentially expressed genes (DEGs)
When the absolute methylation difference between the two tissues reaches 0.2 (|Δβ| > 0.2) and adjusted P value <0.01, they were defined as DMCs.28 Through differential methylation analysis (Limma package, R) on the methylation data of 380 tumor samples and 50 normal samples, there are 5334 hyper-methylated CpG sites and 42
060 hypo-methylated CpG sites.29 The DEG analysis (DESeq2, R) was performed on the gene expression count data of tumor tissues and normal tissues, with P value <0.05 and |log2(fold change)| > 1.3 as the threshold value.
2.4. Calculation of the distribution density of DMCs in different regions
The DMCs were dropped into the above 12 regions, and the density of the DMCs in each region was calculated using eqn (1): | 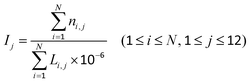 | (1) |
where i denotes the i-th gene (or CGI), j denotes the j-th region, ni,j denotes the number of DMCs of the i-th gene (or CGI) in the j-th region, N denotes the number of all genes (or CGIs), Li,j denotes the length of the i-th gene (or CGI) in the j-th region, and Ij denotes the density of the DMCs in the j-th region. In order to avoid information loss, when a CpG site was mapped to multiple genes, all genes were selected corresponding to the CpG site.
2.5. Calculation of the distribution density for DMCs in site-specific regions
The promoter region was evenly divided into 30 bins, where each bin was 100 bp. The other 11 regions were normalized into 10 bins. The 140 bins were called site-specific regions. The DMCs were dropped into each bin, and the distribution density (Ik) of the DMCs in each bin was calculated using eqn (2): | 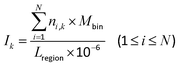 | (2) |
where i and N are the same as in eqn (1), k denotes the k-th bin, Mbin denotes the total number of bins in each region, ni,k denotes the number of DMCs in the k-th bin of the i-th gene (or CGI), and Lregion denotes the total length of each region.
2.6. Calculation of the DNA methylation level for each bin in site-specific regions
In order to calculate the DNA methylation level in each bin, we first calculated the average levels of methylation for all samples. Then the DMCs were matched to each bin of regions. The average levels of methylation
for the k-th bin in different regions were calculated using eqn (3): | 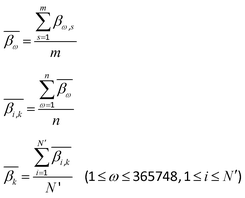 | (3) |
where i and k are the same as in eqn (2), ω denotes the ω-th CpG site, s denotes the s-th sample, m denotes the total number of samples, βω,s represents the methylation level of the ω-th CpG site in the s-th sample,
denotes the average level of methylation for the ω-th CpG site, n denotes the total number of CpG sites that fall into the k-th bin of the i-th gene (or CGI),
denotes the average value of methylation on the k-th bin of the i-th gene (or CGI), and N′ denotes the number of all non-zero effective methylation genes (or CGIs) in the k-th bin.
2.7. The correlation between DNA methylation and gene expression
In order to clarify the relationships between DEGs and DMCs in different regions, the DMCs were matched to the corresponding genes, and the Spearman correlation (rω) between the methylation level of each CpG site and the gene expression level of the corresponding gene for all the same samples was calculated according to eqn (4): | 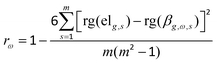 | (4) |
where ω, s, and m are the same as in eqn (3), g denotes the g-th gene, elg,s is the gene expression level of the g-th gene in the s-th sample, βg,ω,s is the methylation level of the ω-th CpG site corresponding to the g-th gene in the s-th sample, and rg(elg,s) and rg(βg,ω,s) are, respectively, the rank numbers of elg,s and βg,ω,s.
2.8. Known genes associated with HCC
There are 13, 629, 623, and 611 genes related to HCC according to the COSMIC (https://cancer.sanger.ac.uk/census), CCGD (http://ccgd-starrlab.oit.umn.edu/download.php), DISEASES (https://diseases.jensenlab.org/Downloads), and OncoDB.HCC (http://oncodb.hcc.ibms.sinica.edu.tw/index.htm) databases, respectively. After synthesizing these databases and deleting the duplicated genes, there are 1761 remaining genes related to HCC.
2.9. Survival analysis
Univariate Cox and multivariate Cox proportional hazard regressions were used to assess the relationship between the expression levels of genes related to abnormal methylation and survival rates, and the hazard ratio (HR) and 95% confidence interval (CI) were calculated. Based on the coefficients of multivariate Cox analysis and the expression levels of genes associated with abnormal methylation, a prognostic risk scoring (RS) model was constructed, and the formula is as follows: | 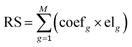 | (5) |
where g denotes the g-th gene, M denotes the total number of related genes, coefg denotes the regression coefficient and represents the contribution of gene g to the prognostic risk score in the multivariate Cox analysis, and elg denotes the expression level of the g-th gene.
Patients were divided into high-risk and low-risk groups using the median RS. Kaplan–Meier was used to calculate the survival rate of the high-risk and low-risk groups. Finally, the performance of the prognostic RS model was evaluated using the time-dependent receiver operating characteristic (ROC) curve.
3. Results
3.1. Distribution density of DMCs in different regions
To compare the distribution density of DMCs in different regions, we matched the DMCs to the enhancer, promoter, 5′UTR, exon, intron, 3′UTR, intergenic, N_Shelf, N_Shore, CGI, S_Shore, and S_Shelf regions, then normalized all regions, and calculated the distribution density of DMCs in each region using eqn (1). The distribution density of DMCs is the highest in the CGI region and the distribution density of hypomethylated CpG sites is higher, indicating that there is a global hypomethylation status in HCC (Fig. 1A). Then we calculated the percentage of distribution density for hypermethylated CpG sites and hypomethylated CpG sites, and found that hypermethylation is significantly enriched in the CGI region (Fig. 1B), which is consistent with the fact that the CGI of cancer is hypermethylated.
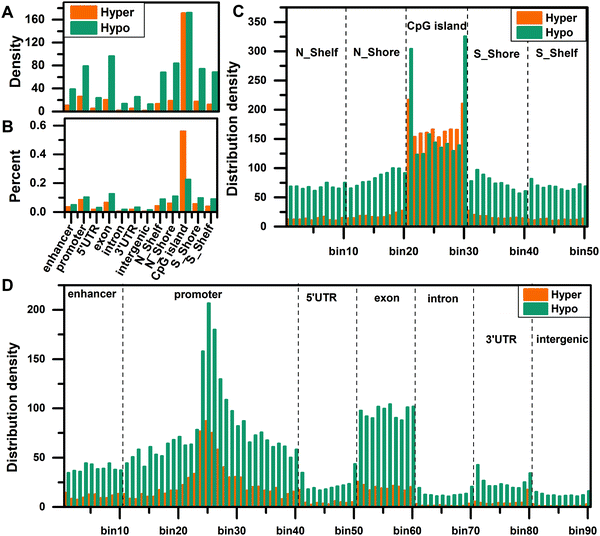 |
| Fig. 1 Distribution density of differentially methylated CpG sites. (A) The density distribution of hypermethylated and hypomethylated CpG sites in 12 different regions. (B) The percentage of distribution density for hypermethylated CpG sites and hypomethylated CpG sites. (C) The density distribution of hypermethylated and hypomethylated CpG sites in the site-specific regions of CpG islands and surrounding regions. (D) The density distribution of hypermethylated and hypomethylated CpG sites in seven site-specific regions of the genome. | |
In order to understand the distribution density of DMCs in site-specific regions, we further divided all regions into smaller bins and calculated the distribution density of DMCs in these site-specific regions according to eqn (2). First, we compared the distribution density of overall DMCs in the site-specific region. For the functional regions around the CGI, the distribution of DMCs in the CGI region is significantly enriched, especially the edge regions on both sides of the CGI (Fig. 1C). In the genome regions (enhancer, promoter, 5′UTR, exon, intron, 3′UTR, and intergenic), the DMCs are more abundant in the enhancer, promoter and exon regions, especially in the vicinity of the TSS (bin25) (Fig. 1D). Second, we compared the distribution density of hypermethylated CpG sites and hypomethylated CpG sites in the site-specific regions. The results show that the distribution density of hypomethylated CpG sites is higher than that of hypermethylated CpG sites in the N_Shelf, N_Shore, S_Shore, and S_Shelf regions. In the edge regions on both sides of the CGI, the hypomethylated CpG sites are more abundant than the hypermethylated CpG sites. On the contrary, the distribution density of hypermethylated CpG sites is more than that of hypomethylated CpG sites in the interior of the CGI (Fig. 1C). The distribution density of hypomethylated CpG sites is greater than that of hypermethylated CpG sites in different regions of the genome (Fig. 1D).
3.2. Distribution of methylation levels for DMCs in different regions
By analyzing the distribution density of DMCs, in order to further understand the degree of difference in methylation levels, we calculated the methylation levels of DMCs in different regions according to eqn (3). The result shows that the distribution patterns of methylation levels for hypermethylated CpG sites or hypomethylated CpG sites in the two tissues are similar (Fig. 2A and B). In the CGI region, the methylation level of the hypermethylated CpG sites in the edge regions on both sides of the CGI is higher than that of the internal region (Fig. 2A), while the methylation level of the hypomethylated CpG sites in the edge regions on both sides of the CGI is lower than that of the internal region (Fig. 2B). On the whole, the methylation levels are diverse in normal tissues, while the methylation levels increased (0.2 vs. 0.5) or decreased (0.8 vs. 0.5) to a stable level (0.5) in tumor tissues (Fig. 2C). That is to say, the original methylation levels in normal tissues are very low (or high) but the methylation levels increase (or decrease) during tumor development, and finally a stable methylation level is reached in the tumor. This stable distribution pattern of methylation levels may be beneficial to the occurrence of HCC.
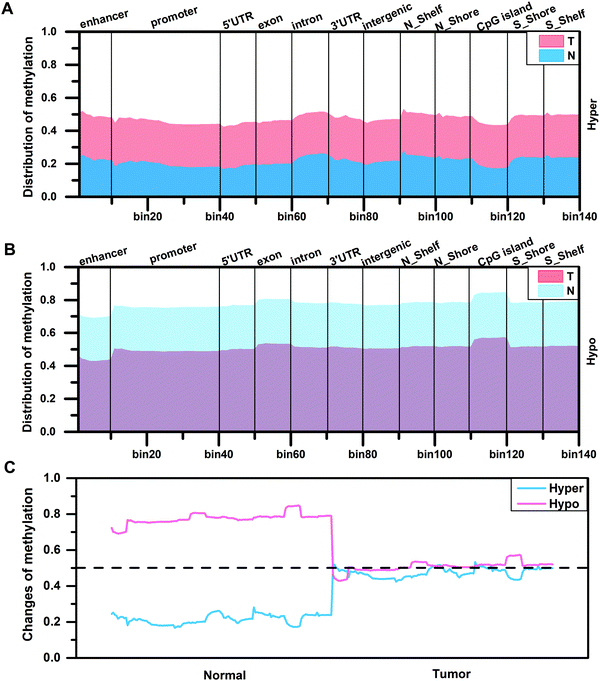 |
| Fig. 2 The distribution of methylation levels for differentially methylated CpG sites in different regions of two tissues. (A and B) Representation of the distribution of methylation levels for hypermethylated CpG sites and hypomethylated CpG sites in different regions of two tissues. (C) The changes of methylation levels for abnormally methylated CpG sites in two tissues. | |
3.3. The correlation between methylation and gene expression for different types of genes
Based on the differential expression analysis of genes for all normal and tumor samples, a total of 2495 up-regulated genes and 777 down-regulated genes were obtained (Fig. 3A). We selected, respectively, the top 50 genes with the most up-regulation and down-regulation, and performed a cluster analysis of the gene expression in the two tissues. It can be seen that there are obvious differences between normal and tumor tissues for gene expression (Fig. 3B and C). Then, the DMCs of the enhancer, promoter, 5′UTR, exon, intron, 3′UTR, N_Shore, N_Shelf, CGI, S_Shore, and S_Shelf regions were matched to the corresponding genes, which were called differentially methylated genes (DMGs). Taking the intersection of DMGs and DEGs in these regions, we obtained four types of differential gene sets: Hyper_up, Hyper_down, Hypo_up, and Hypo_down (Fig. 3D).
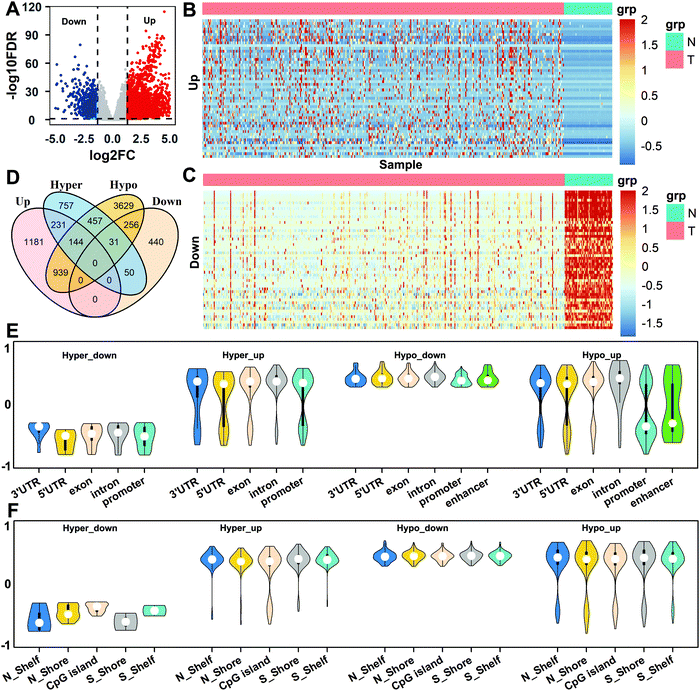 |
| Fig. 3 The analysis of gene expression levels and methylation. (A) The volcano map of differentially expressed genes in HCC and normal tissues. (B and C) Representation of the expression of up-regulated and down-regulated genes in two tissues. (D) Venn diagram of differentially methylated genes and differentially expressed genes. (E) The correlations between gene expression levels and methylation in different regions of the genome. (F) The correlations between gene expression levels and methylation in CpG islands and surrounding regions. | |
In order to understand the correlations between gene expression and methylation for different types of genes, we selected the above four types of genes and calculated the Spearman correlations between the CpG methylation in different regions and the corresponding gene expressions for all the same samples according to eqn (4). The genes with strong correlations (|rω| > 0.3, P value <0.01) were selected as important genes, and a total of 674 genes were obtained. For the different regions of the genome, the results show that the expression levels of Hyper_down genes are negatively correlated with methylation in the 3′UTR, 5′UTR, exon, intron, and promoter regions, and the expression levels of Hyper_up and Hypo_down genes are mainly positively correlated with methylation in all genome regions. But the correlations of methylation with the expression of Hypo_up genes show obvious regional differences, which are mainly positively correlated in the 3′UTR, 5′UTR, exon, and intron regions, while they are negatively correlated in the promoter and enhancer regions (Fig. 3E).
For the CGI and surrounding regions, the correlations between the expression levels of Hyper_down genes and CpG methylation are negative. But the correlations between methylation and the expression levels of Hyper_up, Hypo_down, and Hypo_up genes are mainly positive in all regions (Fig. 3F). It can also be seen that the expression levels of the gene with methylation show diverse relationships in different regions.
3.4. Functional enrichment analysis of important genes
To further understand the biological functions of these important genes that are DEGs related to abnormal methylation, KEGG and GO enrichment analyses were performed. The KEGG pathway shows that these important genes are mainly enriched in the neuroactive ligand–receptor interaction, proteoglycans in cancer, PI3K-Akt signaling pathway, pathways in cancer, cAMP signaling pathway, calcium signaling pathway, other cancer-related pathways, and important pathways related to the basic functions of the liver such as axon guidance, etc. (Fig. 4A). GO biological processes show that these genes are involved in cell morphogenesis involved in differentiation, modulation of chemical synaptic transmission, negative regulation of cell differentiation, response to growth factor, MAPK cascade and other biological processes (Fig. 4B). The cellular components are mainly related to various synapses involved in neuromodulation and the extracellular matrix, and the molecular functional enrichment of these important genes is mainly related to the activity of transportation channels inside and outside the cell (Fig. 4C and D). The results show that these important genes play an important role in the occurrence of cancer.
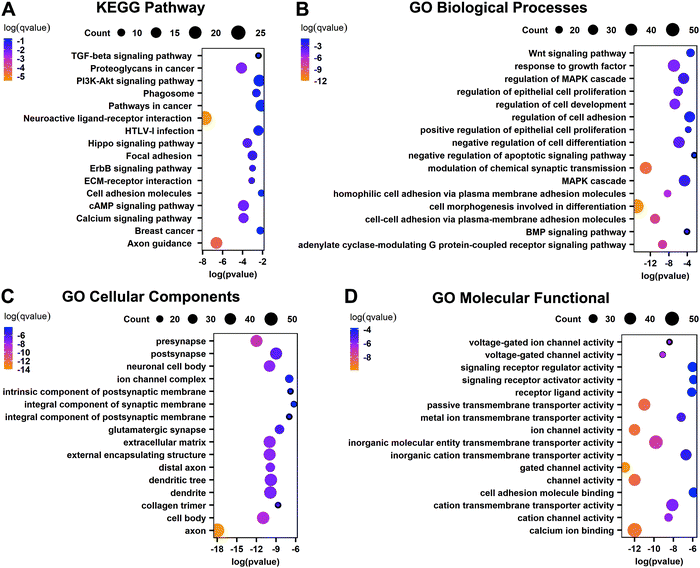 |
| Fig. 4 Functional analysis of differentially expressed genes related to abnormal methylation. (A) KEGG pathway of important genes. (B) GO biological processes of important genes. (C) GO cellular components of important genes. (D) GO molecular functions of important genes. | |
3.5. Survival analysis of important genes related to HCC
By intersecting the 674 important genes with strong correlations and the 1761 known HCC-related genes, we obtained 98 genes as important genes related to HCC for the subsequent analysis. We selected the clinical data of 364 samples corresponding to DNA methylation and gene expression, and performed univariate Cox regression analysis on the expression of these 98 genes in all samples. The Wald test (P < 0.01) was used to select genes related to prognosis. Among the 98 genes, 21 genes were found to be associated with prognosis (Table S2, ESI†). The results of univariate Cox regression were screened using the RSF function,30 and then multivariate Cox regression analysis was performed on these genes. Four key genes that are significantly correlated with prognosis were found (likelihood ratio test, P = 3 × 10−8), which can be used as independent prognostic factors. According to eqn (5), we constructed a RS model by using the coefficients of multivariate Cox analysis and the expression levels of four independent HCC-related genes to assess the prognostic risk of HCC patients as follows:
RS = (0.087 × elSPP1) + (0.246 × elCDC20) + (0.002 × elSFN) + (0.083 × elLAPTM4B) |
In the RS model, the coefficients of SPP1, CDC20, SFN, and LAPTM4B are positive, indicating that they may be risk factors because their high expression is associated with poor prognosis.
By using the prognostic RS model established above to perform RS, the patients were divided into the high-risk group and low-risk group by a median risk score of 1.54621785. The group in which the patient score is equal to or lower than the threshold is the low-risk group, and the other group is the high-risk group. The Kaplan–Meier analysis shows that the survival rate of patients in the low-risk group is significantly higher than that in the high-risk group (P < 0.0001, log-rank test). The 5 year survival rate of the high-risk group is 6.6%, and that of the low-risk group is 15.4% (Fig. 5A). Then we predicted the survival status of the patients based on the risk score, where the standard deviation (SD) of the “dead” samples (SDdead = 0.61) is 1.13 times (SDdead/SDalive = 1.13) that of the “alive” samples (SDalive = 0.54).31 The area under the curve (AUC) from the ROC curve analysis of the risk score over time is 0.76, indicating that the model has high sensitivity and specificity, and the prognostic performance is good (Fig. 5B). We sorted patients according to their risk scores, observed the expression levels of the four key genes, and found that the expression levels of key genes changed with the risk score (Fig. 5C). Then we analyzed the expression levels of the four key genes in high-risk and low-risk groups. The expression levels of SFN, SPP1, CDC20, and LAPTM4B in the high-risk group are significantly higher than those in the low-risk group (Fig. 5D). We also compared the expression levels of the four key genes in tumor and normal tissues, and found that their expression levels in tumor tissues were significantly higher than those in normal tissues (Fig. 5E). In addition, we divided the samples into high-risk and low-risk groups based on the expression levels of the four key genes and conducted the survival analysis. We found that the high expression of these four key genes significantly reduced the survival rate, further confirming that they are risk factors (Fig. S1, ESI†).
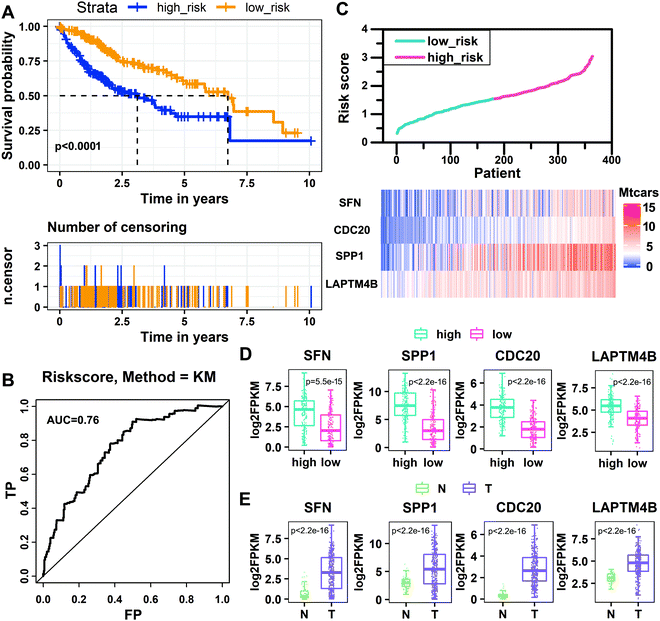 |
| Fig. 5 Performance evaluation of the risk scoring model. (A) Kaplan–Meier estimates of the survival rate of patients in the low-risk and high-risk groups. (B) Time-dependent receiver operating characteristic curve analysis of the risk score. (C) The changes of expression levels for key genes with increasing risk score. (D) The distribution of the expression of key genes in the high-risk and low-risk groups of HCC patients. (E) The distribution of the expression of key genes in tumor and normal tissues of HCC patients. | |
3.6. Validation of the risk scoring model
To further verify the prognostic performance of the RS model, we used the model to assess the risk of patients with clinically relevant characteristics, such as age, gender, pathologic M, N, and T, and stage. The median risk score is still used to divide patients into high-risk and low-risk groups. Kaplan–Meier curve results show that the high-risk group has a shorter median survival time than the low-risk group in the age ≤ 50, age > 50, male, female, pathologic_M0, pathologic_N0, pathologic_stage I/II, pathologic_stage III/IV, pathologic_T1/2, and pathologic_T3/4 groups, and the high-risk group is closely related to poor prognosis (Fig. 6). By comparing the pathologic stage, it was found that the median survival time of patients with stage III/IV is significantly shorter than that of stage I/II. Comparing the pathologic T, it was also found that T3/4 patients have a shorter median survival time than T1/2 patients. These results indicate that the RS model has good prognostic performance.
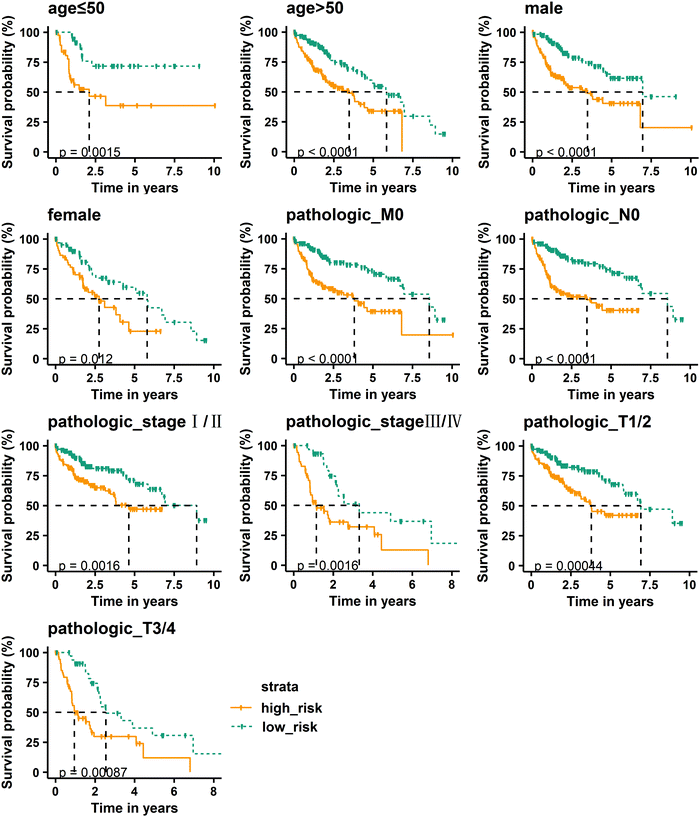 |
| Fig. 6 Kaplan–Meier curve analysis of overall survival in high-risk and low-risk groups for clinically relevant characteristics. | |
3.7. Methylation location analysis of four key genes related to prognosis
In order to find out which regions of the four key genes are affected by methylation, we located the methylated CpG sites of these genes and calculated the Spearman correlations between the gene expression levels and the corresponding CpG methylation levels. It was found that the correlations between the methylation levels of all CpG sites and the expression levels of SFN, SPP1, CDC20, and LAPTM4B were negative (Fig. 7). Further, we located the methylated CpG sites corresponding to these genes and found that the hypomethylation of the region around the CGI in the promoter activates the expression of these oncogenes (SFN, SPP1, and CDC20) (Table 1). In addition, the cg13466284, cg17330303, cg07786675, and cg13374701 of SFN and the cg15460348 and cg20261167 of SPP1 were located in the 1st-exon region, and the cg13466284, cg17330303, cg15460348, and cg20261167 were located in the 5′UTR region. The cg05525368 of CDC20 was located in the sixth exon (6th-exon) region and the cg19726712 of LAPTM4B was located in the S_Shore region. This indicates that the occurrence of HCC may be caused by the high expression of oncogenes due to hypomethylation in different regions of oncogenes.
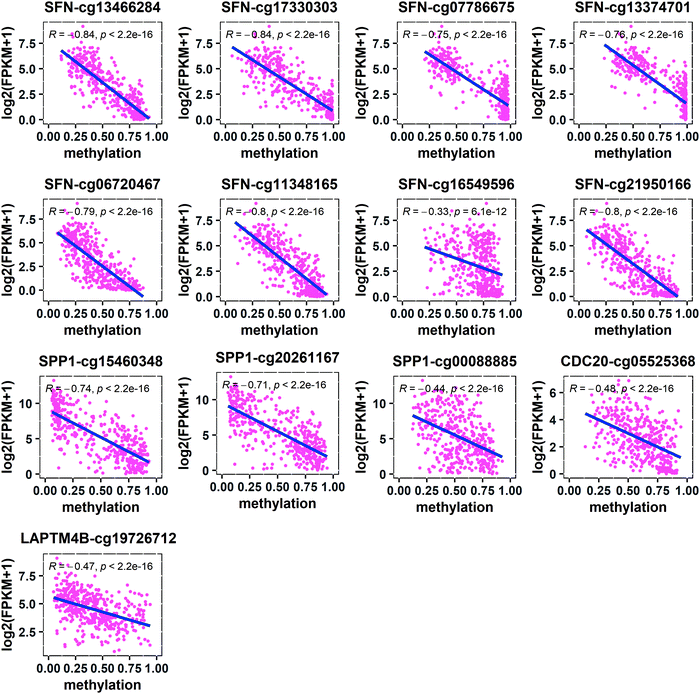 |
| Fig. 7 Spearman correlations between the expression levels of key genes and the corresponding CpG methylation levels. | |
Table 1 Information of four key genes and their corresponding CpG methylation sites
Gene |
CpG site |
Chr |
Position |
Region |
Gene type |
r
|
P value |
Abbreviations: region: the region where the methylated CpG site is located; r is the Spearman correlation coefficient between the gene expression level and the CpG methylation level; 5′UTR: 5′ untranslated region; CGI: CpG island; 1st-exon: the first exon; 6th-exon: the sixth exon region. |
SFN
|
cg13466284 |
Chr1 |
26863188 |
5′UTR, 1st-exon, promoter, N_Shore |
Hypo_up |
−0.84 |
0.0 |
SFN
|
cg17330303 |
Chr1 |
26863157 |
5′UTR, 1st-exon, promoter, N_Shore |
Hypo_up |
−0.84 |
0.0 |
SFN
|
cg07786675 |
Chr1 |
26863494 |
1st-exon, promoter, CGI, N_Shore |
Hypo_up |
−0.75 |
0.0 |
SFN
|
cg13374701 |
Chr1 |
26863597 |
1st-exon, promoter, CGI, N_Shore |
Hypo_up |
−0.76 |
0.0 |
SFN
|
cg06720467 |
Chr1 |
26862949 |
Promoter, N_Shore |
Hypo_up |
−0.79 |
0.0 |
SFN
|
cg11348165 |
Chr1 |
26862779 |
Promoter, N_Shore |
Hypo_up |
−0.80 |
0.0 |
SFN
|
cg16549596 |
Chr1 |
26862096 |
Promoter, N_Shelf, N_Shore |
Hypo_up |
−0.33 |
6.1 × 10−12 |
SFN
|
cg21950166 |
Chr1 |
26862858 |
Promoter, N_Shore |
Hypo_up |
−0.80 |
0.0 |
SPP1
|
cg15460348 |
Chr4 |
87975757 |
5′UTR, 1st-exon, promoter |
Hypo_up |
−0.74 |
0.0 |
SPP1
|
cg20261167 |
Chr4 |
87975790 |
5′UTR, 1st-exon, promoter |
Hypo_up |
−0.71 |
0.0 |
SPP1
|
cg00088885 |
Chr4 |
87975482 |
Promoter |
Hypo_up |
−0.44 |
0.0 |
CDC20
|
cg05525368 |
Chr1 |
43360233 |
6th-exon, promoter, S_Shore |
Hypo_up |
−0.48 |
0.0 |
LAPTM4B
|
cg19726712 |
Chr8 |
97778099 |
S_Shore |
Hypo_up |
−0.47 |
0.0 |
4. Discussion
In this study, we analyzed DNA methylation, gene expression and clinical data of HCC. By analyzing the distribution of abnormal methylation in 12 functional regions, we found the stable distribution pattern of abnormal methylation in HCC. Through the analysis of the correlation between abnormal methylation in different regions and gene expression, we found that DNA methylation has diverse effects on gene expression. Then a four-gene prognostic RS model was constructed by Cox regression analysis on important genes related to HCC, and the prognostic performance was well verified in the clinical characteristics of patients. Finally, the four key genes related to survival were further analyzed and their regions of abnormal methylation were located. These results provide potential biomarkers for further studies of HCC and provide new insights into the role of DNA methylation in gene regulation.
Genome-wide hypomethylation and CGI hypermethylation of the promoter region are common events in cancer32 and hypomethylation is common in gene-body regions for HCC.33,34 In our study, similar results were obtained by analyzing the distribution density of DMCs in 12 regions. In addition, the distribution of the methylation levels for DMCs in different regions was calculated, and it was found that the levels of abnormal methylation have reached a stable distribution pattern in HCC. We suspect that this may be the cause of HCC, but this mechanism is still unclear.
DNA methylation plays a vital role in the occurrence and development of cancer. It has been observed that alterations of DNA methylation are the cause of cancer. DNA methylation is an important regulator for gene expression. It is generally believed that hypermethylation inhibits gene expression, hypomethylation promotes gene expression, and DNA methylation has a negative regulatory effect on gene expression. For example, the CGI hypermethylation in the promoter region leads to the inhibition of transcription.35,36 Previous studies have also found that promoter hypomethylation leads to the increase of HAI1 expression, which is related to the differentiation state of HCC tissue.37 The effect of DNA demethylation of CD147 on the progression of HCC has been reported.38 In addition, some studies have shown that DNA methylation in the 1st-exon is negatively correlated with the gene expression level.39 Similarly, it has been reported that 1st-exon methylation prevents transcript initiation, while the hypomethylation of the 5′UTR and 1st-exon regions leads to up-regulation of gene expression.40,41 Another study has shown that methylation in CGIs, CpG island shores, and the 1st-exon is closely related to gene suppression.42 In our results, it was found that the hypomethylation of the promoter, 1st-exon, 5′UTR, N_Shore, and S_Shore regions of key oncogenes activates gene expression. This result is consistent with the predecessors, indicating that DNA methylation is negatively correlated with gene expression and confirms that methylation inhibits gene expression from the opposite perspective.
This study identified four key genes as potential biomarkers for HCC. Some of these genes have been reported in studies related to HCC. It is well known that SPP1 can induce inflammation and liver fibrosis, and is related to physiological processes such as drug resistance, cell proliferation, invasion, and tumor metastasis.43 A large amount of clinical evidence indicates that SPP1 is a therapeutic target to prevent HCC metastasis and proliferation.44–47 Many studies have found that SPP1 is hypomethylated in HCC, and its expression level is higher than that of non-tumor tissues.43,48,49 In our results, SPP1 is also hypomethylated and highly expressed in HCC. CDC20 is a cell cycle regulator, and abnormal expression of CDC20 has been detected in many cancers.50,51 Studies have shown that silencing the expression of CDC20 can inhibit the proliferation and invasion of HCC cells and prevent cell cycle progression.52,53 The high expression of CDC20 is related to the shortening of the overall survival of HCC patients and is a potential therapeutic target,54,55 which is consistent with our results. SFN is significantly related to the overall survival rate of HCC patients and is a candidate biomarker for HCC.56 We found that hypomethylation promotes the expression of SFN in HCC. Studies have shown that LAPTM4B can promote HCC cell proliferation, migration, and invasion in vitro. LAPTM4B is overexpressed in HCC and is a poor prognostic factor for HCC.57,58 The same results were obtained in our research. It can be seen that these key genes play an important role in the occurrence, proliferation and metastasis of HCC.
Although, in our study, we found prognostic biomarkers related to methylation in HCC and identified the key regions where abnormal methylation regulates gene expression, due to the limitation of conditions, our research is limited to the level of theoretical analysis. Therefore, further experimental studies are needed to verify our results.
5. Conclusions
In this study, we conducted a systematic and comprehensive analysis of DNA methylation, gene expression, and clinical data of HCC. Four key genes were identified in HCC, and the effect of promoter, 1st-exon, 5′UTR, 6th-exon, N_Shore and S_Shore hypomethylation on the expression of key genes was found. These may provide new insights and potential biomarkers for exploring the occurrence of HCC.
Abbreviations
HCC | Hepatocellular carcinoma |
CGIs | CpG islands |
5′UTR | 5′ untranslated regions |
3′UTR | 3′ untranslated regions |
TSS | Transcription start site |
CDS | Coding region start site |
CDE | Coding region end site |
TTS | Transcription termination site |
DMCs | Differentially methylated CpG sites |
DEGs | Differentially expressed genes |
RS | Risk scoring |
ROC | Receiver operating characteristic |
DMGs | Differentially methylated genes |
1st-exon | The first exon |
6th-exon | The sixth exon |
Data availability
We have used the DNA methylation data, gene expression data, and clinical data from the TCGA database (https://cancergenome.nih.gov/), the genomic data from the UCSC database (http://http:/genome.ucsc.edu/), the enhancer region location file from the FANTOM5 database (https://fantom.gsc.riken.jp/5/data/), and known cancer genes from the COSMIC (https://cancer.sanger.ac.uk/census), CCGD (http://ccgd-starrlab.oit.umn.edu/download.php), DISEASES (https://diseases.jensenlab.org/Downloads), and OncoDB.HCC (http://oncodb.hcc.ibms.sinica.edu.tw/index.htm) databases. These data are publicly available.
Author contributions
Yu-Xian Liu: conceptualization, formal analysis, investigation, methodology, software, validation, visualization, methodology, writing – original draft, writing – review and editing. Qian-Zhong Li: conceptualization, funding acquisition, methodology, supervision, writing – review and editing. Yan-Ni Cao: methodology, validation, visualization, writing – review and editing. All authors read and approved the final manuscript.
Conflicts of interest
There are no conflicts to declare.
Acknowledgements
This work was supported by the National Natural Science Foundation of China [grant numbers 32160216, 31870838, and 61861035].
References
- F. Bray, J. Ferlay, I. Soerjomataram, R. L. Siegel, L. A. Torre and A. Jemal, Global cancer statistics 2018: GLOBOCAN estimates of incidence and mortality worldwide for 36 cancers in 185 countries, Ca-Cancer J. Clin., 2018, 68, 394–424 CrossRef PubMed.
- R. L. Siegel, K. D. Miller and A. Jemal, Cancer statistics, 2020, Ca-Cancer J. Clin., 2020, 70, 7–30 CrossRef PubMed.
- Y. X. Liu, Q. Z. Li, Y. N. Cao and L. Q. Zhang, Identification of key genes and important histone modifications in hepatocellular carcinoma, Comput. Struct. Biotechnol. J., 2020, 18, 2657–2669 CrossRef CAS.
- W. Jin, Q. Z. Li, Y. Liu and Y. C. Zuo, Effect of the key histone modifications on the expression of genes related to breast cancer, Genomics, 2020, 112, 853–858 CrossRef CAS PubMed.
- K. Jabbari and G. Bernardi, Cytosine methylation and CpG, TpG (CpA) and TpA frequencies, Gene, 2004, 333, 143–149 CrossRef CAS PubMed.
- R. A. Irizarry, C. Ladd-Acosta, B. Wen, Z. Wu, C. Montano, P. Onyango, H. Cui, K. Gabo, M. Rongione, M. Webster, H. Ji, J. Potash, S. Sabunciyan and A. P. Feinberg, The human colon cancer methylome shows similar hypo- and hypermethylation at conserved tissue-specific CpG island shores, Nat. Genet., 2009, 41, 178–186 CrossRef CAS.
- J. Sandoval, H. Heyn, S. Moran, J. Serra-Musach, M. A. Pujana, M. Bibikova and M. Esteller, Validation of a DNA methylation microarray for 450,000 CpG sites in the human genome, Epigenetics, 2011, 6, 692–702 CrossRef CAS PubMed.
- M. Bibikova, B. Barnes, C. Tsan, V. Ho, B. Klotzle, J. M. Le, D. Delano, L. Zhang, G. P. Schroth, K. L. Gunderson, J. B. Fan and R. Shen, High density DNA methylation array with single CpG site resolution, Genomics, 2011, 98, 288–295 CrossRef CAS PubMed.
- N. Yamada, K. Yasui, O. Dohi, Y. Gen, A. Tomie, T. Kitaichi, N. Iwai, H. Mitsuyoshi, Y. Sumida, M. Moriguchi, K. Yamaguchi, T. Nishikawa, A. Umemura, Y. Naito, S. Tanaka, S. Arii and Y. Itoh, Genome-wide DNA methylation analysis in hepatocellular carcinoma, Oncol. Rep., 2016, 35, 2228–2236 CrossRef CAS PubMed.
- U. Schagdarsurengin, L. Wilkens, D. Steinemann, P. Flemming, H. H. Kreipe, G. P. Pfeifer, B. Schlegelberger and R. Dammann, Frequent epigenetic inactivation of the RASSF1A gene in hepatocellular carcinoma, Oncogene, 2003, 22, 1866–1871 CrossRef CAS PubMed.
- X. Zhang, C. Guo, X. Wu, A. X. Li, L. Liu, W. Tsark, R. Dammann, H. Shen, S. L. Vonderfecht and G. P. Pfeifer, Analysis of Liver Tumor-Prone Mouse Models of the Hippo Kinase Scaffold Proteins RASSF1A and SAV1, Cancer Res., 2016, 76, 2824–2835 CrossRef CAS PubMed.
- C. A. Ishak, A. E. Marshall, D. T. Passos, C. R. White, S. J. Kim, M. J. Cecchini, S. Ferwati, W. A. MacDonald, C. J. Howlett, I. D. Welch, S. M. Rubin, M. R. W. Mann and F. A. Dick, An RB-EZH2 Complex Mediates Silencing of Repetitive DNA Sequences, Mol. Cell, 2016, 64, 1074–1087 CrossRef CAS PubMed.
- C. M. Wong, J. M. Lee, Y. P. Ching, D. Y. Jin and I. O. Ng, Genetic and epigenetic alterations of DLC-1 gene in hepatocellular carcinoma, Cancer Res., 2003, 63, 7646–7651 CAS.
- S. P. Hussain, J. Schwank, F. Staib, X. W. Wang and C. C. Harris, TP53 mutations and hepatocellular carcinoma: insights into the etiology and pathogenesis of liver cancer, Oncogene, 2007, 26, 2166–2176 CrossRef CAS PubMed.
- X. Liu, X. Hu, Y. Kuang, P. Yan, L. Li, C. Li, Q. Tao and X. Cai, BCLB, methylated in hepatocellular carcinoma, is a starvation stress sensor that induces apoptosis and autophagy through the AMPK-mTOR signaling cascade, Cancer Lett., 2017, 395, 63–71 CrossRef CAS.
- F. Karami, S. Mohammadi-Yeganeh, N. Abedi, A. Koochaki, V. Kia and M. Paryan, Bioinformatics Prediction and In Vitro Analysis Revealed That miR-17 Targets Cyclin D1 mRNA in Triple Negative Breast Cancer Cells, Chem. Biol. Drug Des., 2016, 87, 317–320 CrossRef CAS.
- H. Zhang, X. X. Li, Y. Yang, Y. Zhang, H. Y. Wang and X. F. S. Zheng, Significance and mechanism of androgen receptor overexpression and androgen receptor/mechanistic target of rapamycin cross-talk in hepatocellular carcinoma, Hepatology, 2018, 67, 2271–2286 CrossRef CAS PubMed.
- N. Heldring, A. Pike, S. Andersson, J. Matthews, G. Cheng, J. Hartman, M. Tujague, A. Ström, E. Treuter, M. Warner and J. A. Gustafsson, Estrogen receptors: how do they signal and what are their targets, Physiol. Rev., 2007, 87, 905–931 CrossRef CAS PubMed.
- X. Qiu, Y. Huang, Y. Zhou and F. Zheng, Aberrant methylation of TRIM58 in hepatocellular carcinoma and its potential clinical implication, Oncol. Rep., 2016, 36, 811–818 CrossRef CAS.
- Y. Guo, Y. Peng, D. Gao, M. Zhang, W. Yang, E. Linghu, J. G. Herman, F. Fuks, G. Dong and M. Guo, Silencing HOXD10 by promoter region hypermethylation activates ERK signaling in hepatocellular carcinoma, Clin. Epigenet., 2017, 9, 116 CrossRef.
- C. C. Kuo, Y. L. Shih, H. Y. Su, M. D. Yan, C. B. Hsieh, C. Y. Liu, W. T. Huang, M. H. Yu and Y. W. Lin, Methylation of IRAK3 is a novel prognostic marker in hepatocellular carcinoma, World J. Gastroenterol., 2015, 21, 3960–3969 CrossRef CAS.
- S. Zhang, Y. Zhou, Y. Wang, Z. Wang, Q. Xiao, Y. Zhang, Y. Lou, Y. Qiu and F. Zhu, The mechanistic, diagnostic and therapeutic novel nucleic acids for hepatocellular carcinoma emerging in past score years, Brief. Bioinform., 2021, 22, 1860–1883 CrossRef CAS PubMed.
- A. Eden, F. Gaudet, A. Waghmare and R. Jaenisch, Chromosomal instability and tumors promoted by DNA hypomethylation, Science, 2003, 300, 455 CrossRef CAS PubMed.
- M. Ehrlich, DNA hypomethylation in cancer cells, Epigenomics, 2009, 1, 239–259 CrossRef CAS PubMed.
- M. Arechederra, F. Daian, A. Yim, S. K. Bazai, S. Richelme, R. Dono, A. J. Saurin, B. H. Habermann and F. Maina, Hypermethylation of gene body CpG islands predicts high dosage of functional oncogenes in liver cancer, Nat. Commun., 2018, 9, 3164 CrossRef PubMed.
- Cancer Genome Atlas Research Network, Comprehensive and Integrative Genomic Characterization of Hepatocellular Carcinoma, Cell, 2017, 169, 1327–1341 CrossRef .e23.
- R. Andersson, C. Gebhard, I. Miguel-Escalada, I. Hoof, J. Bornholdt, M. Boyd, Y. Chen, X. Zhao, C. Schmidl, T. Suzuki, E. Ntini, E. Arner, E. Valen, K. Li, L. Schwarzfischer, D. Glatz, J. Raithel, B. Lilje, N. Rapin, F. O. Bagger, M. Jørgensen, P. R. Andersen, N. Bertin, O. Rackham, A. M. Burroughs, J. K. Baillie, Y. Ishizu, Y. Shimizu, E. Furuhata, S. Maeda, Y. Negishi, C. J. Mungall, T. F. Meehan, T. Lassmann, M. Itoh, H. Kawaji, N. Kondo, J. Kawai, A. Lennartsson, C. O. Daub, P. Heutink, D. A. Hume, T. H. Jensen, H. Suzuki, Y. Hayashizaki, F. Müller, A. R. R. Forrest, P. Carninci, M. Rehli and A. Sandelin, An atlas of active enhancers across human cell types and tissues, Nature, 2014, 507, 455–461 CrossRef CAS.
- X. Yang, L. Gao and S. Zhang, Comparative pan-cancer DNA methylation analysis reveals cancer common and specific patterns, Brief. Bioinform., 2017, 18, 761–773 CAS.
- M. E. Ritchie, B. Phipson, D. Wu, Y. Hu, C. W. Law, W. Shi and G. K. Smyth, Limma powers differential expression analyses for RNA-sequencing and microarray studies, Nucleic Acids Res., 2015, 43, e47 CrossRef PubMed.
- J. M. Taylor, Random Survival Forests, J. Thorac. Oncol., 2011, 6, 1974–1975 CrossRef PubMed.
- N. J. Wald and J. P. Bestwick, Is the area under an ROC curve a valid measure of the performance of a screening or diagnostic test?, J. Med. Screen., 2014, 21, 51–56 CrossRef CAS PubMed.
- S. Sharma, T. K. Kelly and P. A. Jones, Epigenetics in cancer, Carcinogenesis, 2010, 31, 27–36 CrossRef CAS.
- J. Cheng, D. Wei, Y. Ji, L. Chen, L. Yang, G. Li, L. Wu, T. Hou, L. Xie, G. Ding, H. Li and Y. Li, Integrative analysis of DNA methylation and gene expression reveals hepatocellular carcinoma-specific diagnostic biomarkers, Genome Med., 2018, 10, 42 CrossRef.
- F. Fang, X. Wang and T. Song, Five-CpG-based prognostic signature for predicting survival in hepatocellular carcinoma patients, Cancer Biol. Med., 2018, 15, 425–433 CrossRef CAS PubMed.
- I. Keshet, Y. Schlesinger, S. Farkash, E. Rand, M. Hecht, E. Segal, E. Pikarski, R. A. Young, A. Niveleau, H. Cedar and I. Simon, Evidence for an instructive mechanism of de novo methylation in cancer cells, Nat. Genet., 2006, 38, 149–153 CrossRef CAS PubMed.
- K. P. Nephew and T. H. Huang, Epigenetic gene silencing in cancer initiation and progression, Cancer Lett., 2003, 190, 125–133 CrossRef CAS.
- X. Du, L. Wu and M. S. Ur Rahman, Promoter Hypomethylation Is Responsible for Upregulated Expression of HAI-1 in Hepatocellular Carcinoma, Dis. Markers, 2019, 2019, 9175215 Search PubMed.
- L. M. Kong, C. G. Liao, L. Chen, H. S. Yang, S. H. Zhang, Z. Zhang, H. J. Bian, J. L. Xing and Z. N. Chen, Promoter hypomethylation up-regulates CD147 expression through increasing Sp1 binding and associates with poor prognosis in human hepatocellular carcinoma, J. Cell. Mol. Med., 2011, 15, 1415–1428 CrossRef CAS PubMed.
- S. Li, J. Zhang, S. Huang and X. He, Genome-wide analysis reveals that exon methylation facilitates its selective usage in the human transcriptome, Brief. Bioinform., 2018, 19, 754–764 CrossRef CAS PubMed.
- F. Brenet, M. Moh, P. Funk, E. Feierstein, A. J. Viale, N. D. Socci and J. M. Scandura, DNA methylation of the first exon is tightly linked to transcriptional silencing, PLoS One, 2011, 6, e14524 CrossRef CAS PubMed.
- B. Ozer and U. Sezerman, Analysis of the interplay between methylation and expression reveals its potential role in cancer aetiology, Funct. Integr. Genomics, 2017, 17, 53–68 CrossRef CAS PubMed.
- C. J. Lee, J. Evans, K. Kim, H. Chae and S. Kim, Determining the effect of DNA methylation on gene expression in cancer cells, Methods Mol. Biol., 2014, 1101, 161–178 CrossRef CAS PubMed.
- Y. Komatsu, T. Waku, N. Iwasaki, W. Ono, C. Yamaguchi and J. Yanagisawa, Global analysis of DNA methylation in early-stage liver fibrosis, BMC Med. Genomics, 2012, 5, 5 CrossRef CAS PubMed.
- Y. Zhu, X. M. Gao, J. Yang, D. Xu, Y. Zhang, M. Lu, Z. Zhang, Y. Y. Sheng, J. H. Li, X. X. Yu, Y. Zheng, Q. Z. Dong and L. X. Qin, C-C chemokine receptor type 1 mediates osteopontin-promoted metastasis in hepatocellular carcinoma, Cancer Sci., 2018, 109, 710–723 CrossRef CAS PubMed.
- Q. Z. Dong, X. F. Zhang, Y. Zhao, H. L. Jia, H. J. Zhou, C. Dai, H. J. Sun, Y. Qin, W. D. Zhang, N. Ren, Q. H. Ye and L. X. Qin, Osteopontin promoter polymorphisms at locus -443 significantly affect the metastasis and prognosis of human hepatocellular carcinoma, Hepatology, 2013, 57, 1024–1034 CrossRef CAS PubMed.
- R. Jia, Y. Liang, R. Chen, G. Liu, H. Wang, M. Tang, X. Zhou, H. Wang, Y. Yang, H. Wei, B. Li, Y. Song and J. Zhao, Osteopontin facilitates tumor metastasis by regulating epithelial-mesenchymal plasticity, Cell Death Dis., 2016, 7, e2564 CrossRef CAS PubMed.
- J. Wang, F. Hao, X. Fei and Y. Chen, SPP1 functions as an enhancer of cell growth in hepatocellular carcinoma targeted by miR-181c, Am. J. Transl. Res., 2019, 11, 6924–6937 CAS.
- J. Long, P. Chen, J. Lin, Y. Bai, X. Yang, J. Bian, Y. Lin, D. Wang, X. Yang, Y. Zheng, X. Sang and H. Zhao, DNA methylation-driven genes for constructing diagnostic, prognostic, and recurrence models for hepatocellular carcinoma, Theranostics, 2019, 9, 7251–7267 CrossRef CAS PubMed.
- C. A. Moylan, H. Pang, A. Dellinger, A. Suzuki, M. E. Garrett, C. D. Guy, S. K. Murphy, A. E. Ashley-Koch, S. S. Choi, G. A. Michelotti, D. D. Hampton, Y. Chen, H. L. Tillmann, M. A. Hauser, M. F. Abdelmalek and A. M. Diehl, Hepatic gene expression profiles differentiate presymptomatic patients with mild versus severe nonalcoholic fatty liver disease, Hepatology, 2014, 59, 471–482 CrossRef CAS PubMed.
- M. Kapanidou, N. L. Curtis and V. M. Bolanos-Garcia, Cdc20: At the Crossroads between Chromosome Segregation and Mitotic Exit, Trends Biochem. Sci., 2017, 42, 193–205 CrossRef CAS PubMed.
- Y. Kim, J. W. Choi, J. H. Lee and Y. S. Kim, Spindle assembly checkpoint MAD2 and CDC20 overexpressions and cell-in-cell formation in gastric cancer and its precursor lesions, Hum. Pathol., 2019, 85, 174–183 CrossRef CAS PubMed.
- S. Shan, W. Chen and J. D. Jia, Transcriptome Analysis Revealed a Highly Connected Gene Module Associated With Cirrhosis to Hepatocellular Carcinoma Development, Front. Genet., 2019, 10, 305 CrossRef CAS PubMed.
- J. Li, J. Z. Gao, J. L. Du, Z. X. Huang and L. X. Wei, Increased CDC20 expression is associated with development and progression of hepatocellular carcinoma, Int. J. Oncol., 2014, 45, 1547–1555 CrossRef CAS PubMed.
- G. Fan, Y. Tu, C. Chen, H. Sun, C. Wan and X. Cai, DNA methylation biomarkers for hepatocellular carcinoma, Cancer Cell Int., 2018, 18, 140 CrossRef PubMed.
- W. X. Yang, Y. Y. Pan and C. G. You, CDK1, CCNB1, CDC20, BUB1, MAD2L1, MCM3, BUB1B, MCM2, and RFC4 May Be Potential Therapeutic Targets for Hepatocellular Carcinoma Using Integrated Bioinformatic Analysis, Biomed. Res. Int., 2019, 2019, 1245072 Search PubMed.
- Y. Zheng, Q. Huang, Z. Ding, T. Liu, C. Xue, X. Sang and J. Gu, Genome-wide DNA methylation analysis identifies candidate epigenetic markers and drivers of hepatocellular carcinoma, Brief. Bioinform., 2018, 19, 101–108 CAS.
- H. Su, T. Xu, X. Huang, S. Zang, B. Wang, Y. Huang, J. Liu and A. Huang, Correlation of lysosome-associated protein transmembrane-4β gene overexpression with the malignant phenotypes of hepatocellular carcinoma, Pathol. Res. Pract., 2017, 213, 1536–1541 CrossRef CAS PubMed.
- Y. Meng, L. Wang, D. Chen, Y. Chang, M. Zhang, J. J. Xu, R. Zhou and Q. Y. Zhang, LAPTM4B: an oncogene in various solid tumors and its functions, Oncogene, 2016, 35, 6359–6365 CrossRef CAS PubMed.
Footnote |
† Electronic supplementary information (ESI) available. See DOI: 10.1039/d1mo00282a |
|
This journal is © The Royal Society of Chemistry 2022 |