DOI:
10.1039/D1MO00106J
(Research Article)
Mol. Omics, 2022,
18, 19-30
Targeting coenzyme Q10 synthesis overcomes bortezomib resistance in multiple myeloma†
Received
31st March 2021
, Accepted 18th August 2021
First published on 26th August 2021
Abstract
During the development of drug resistance, multiple myeloma (MM) cells undergo changes to their metabolism. However, how these metabolic changes can be exploited to improve treatment efficacy is not known. Here we demonstrate that targeting coenzyme Q10 (CoQ) biosynthesis through the mevalonate pathway works in synergy with the proteasome inhibitor bortezomib (BTZ) in MM. We show that gene expression signatures relating to the mitochondrial tricarboxylic acid (TCA) cycle and electron transport chain (ETC) predispose to clinical BTZ resistance and poor prognosis in MM patients. Mechanistically, BTZ-resistant cells show increased activity of glutamine-driven TCA cycle and oxidative phosphorylation, together with an increased vulnerability towards ETC inhibition. Moreover, BTZ resistance is accompanied by high levels of the mitochondrial electron carrier CoQ, while the mevalonate pathway inhibitor simvastatin increases cell death and decreases CoQ levels, specifically in BTZ-resistant cells. Both in vitro and in vivo, simvastatin enhances the effect of bortezomib treatment. Our study links CoQ synthesis to drug resistance in MM and provides a novel avenue for improving BTZ responses through statin-induced inhibition of mitochondrial metabolism.
Introduction
Multiple myeloma (MM), the second most common haematologic malignancy,1 is an ideal model to study drug resistance. It follows a relapsing course, with the majority of patients experiencing multiple relapses from different drugs, and is characterised by the presence of multiple clones with differing degrees of drug sensitivity at the time of diagnosis.1,2
Over the past decade, proteasome inhibitors and immunomodulatory drugs have become the pillars of MM treatment regimes, which have improved treatment outcomes and overall survival. However, despite these recent breakthroughs, MM is still an incurable cancer as most patients develop refractory disease that cannot be cured.
Outcomes for MM patients have improved significantly with the introduction of novel agents, such as the proteasome inhibitor Bortezomib (BTZ). However, not all patients respond to BTZ and many initial responders develop resistance during the course of therapy.3,4 A better understanding of MM development can help find prognostic biomarkers for optimal treatment, or novel therapeutic targets to enhance efficiency of current MM therapy.
Changes in metabolism are increasingly regarded as one of the hallmarks of cancer cells, including MM.5 Malignant cells rewire multiple aspects of their metabolism to fulfil the higher demand for energy, to produce building blocks for proliferation and to maintain their redox balance. Several studies have shown that serum from MM patients have altered levels of amino acids and lipids.6,7 On a cellular level, MM is associated with higher glycolysis, coupled to increased glucose uptake and higher lactate production.8–10 At the same time, MM cells show an addiction for the amino acid glutamine,11 the main carbon source for the tricarboxylic acid (TCA) cycle in many cancer cells, including MM.11–13 However, the TCA cycle can use different carbon sources, including pyruvate derived from glycolysis. Metabolic flux studies using 13C stable isotopes have shown that glutamine is dominant as a source for the TCA cycle in MM as compared to glucose.14
Recent studies show that metabolism is further adjusted when cells become resistant to BTZ treatment.15–18 In a previous study, we have shown that BTZ-resistant cells use glucose to fuel the pentose phosphate pathway (PPP) and serine synthesis pathway (SSP), resulting in enhanced antioxidant capacity.18 Other studies suggest that BTZ-resistant cells have increased TCA cycle and oxidative phosphorylation (OXPHOS), resulting in increased ATP production.16,17,19,20 Proteasome inhibition induces mitochondrial damage, which results in oxidative stress and eventually leads to apoptosis.21 It is therefore likely that BTZ-resistant cells upregulate their mitochondrial energy production and anti-oxidant capacity to counteract these effects. In addition, increased OXPHOS has shown to promote protein folding, mediating BTZ resistance.22 Although these studies point to a clear association between energy metabolism and proteasome-inhibitor response, whether and how energy metabolism can be exploited to improve BTZ treatment efficacy remains unclear.
Here, we characterize mitochondrial energy metabolism in BTZ-resistant cells by using a multi-omics approach. Our data indicate that a metabolic signature of TCA cycle and OXPHOS gene expression predisposes to clinical BTZ resistance and poor prognosis in MM patients. We show that this signature is linked to elevated levels of the mitochondrial electron carrier coenzyme Q10 (CoQ), a downstream product of the mevalonate pathway. We further demonstrate that inhibition of the mevalonate pathway with simvastatin lowers CoQ levels in BTZ-resistant cells, inducing apoptosis. Our results position the combination of BTZ with statin-induced OXPHOS inhibition as a novel strategy to improve MM treatment outcome.
Material and methods
Reagents
Human MM RPMI-8226 WT and BTZ-resistant cells were a kind gift from Gerrit Jansen (VU University Medical Center, Amsterdam, The Netherlands). AMO-1 and ARH-77 WT, BTZ- and carfilzomib-resistant cells were kindly provided by C. Driessen (Kantonsspital St. Gallen, Switzerland). BTZ was purchased from Selleck Chemicals (Houston, TX, USA). All solvents were obtained from Biosolve (Valkenswaard, The Netherlands) and all other chemicals were obtained from Sigma-Aldrich (Zwijndrecht, The Netherlands), unless stated otherwise.
Cell culture
BTZ and carfilzomib-resistant cells were obtained as described previously.23,24 Cells were maintained in suspension culture in RPMI-1640 (Lonza, Basel, Switzerland) medium supplemented with 2 mM L-glutamine (Lonza), 10% fetal bovine serum (FBS) (Gibco) and 100 μg ml−1 penicillin/streptomycin (Lonza) and were kept at 37 °C in humidified 5% CO2 atmosphere. BTZ- and carfilzomib resistant cells were continuously cultured in the presence of BTZ or carfilzomib. Cells were cultured without drugs 3–5 days prior to experiments. Media were supplemented with 10% FBS and 100 μg ml−1 penicillin/streptomycin unless stated otherwise.
LC-MS based metabolomics
LC-MS based metabolomics were performed as described.18,25 For metabolic screens, cells were resuspended in fresh RPMI medium at a density of 1 × 106 cells per ml. After 2 hours, samples were washed with PBS and harvested for LC-MS analysis.
For 13C-tracer tracer studies, cells were resuspended in DMEM containing 2 mM [U-13C]L-glutamine (Cambridge Isotopes, Tewksbury, MA, USA) or with 25 mM [U-13C]D-glucose and 12C-glutamine and harvested after 4 hours. For glutamine starvation experiments, medium consisted of DMEM, supplemented with 25 mM [U-13C]D-glucose and 10% dialyzed FBS in the presence or absence of 2 mM L-glutamine. LC-MS analysis was performed on an Exactive mass spectrometer (Thermo Scientific, Breda, The Netherlands) coupled to a Dionex Ultimate 3000 autosampler and pump (Thermo Scientific). Peak intensities were normalised based on median peak intensity. Isotope distributions were corrected for natural abundance and data are plotted as relative peak area compared to WT cells under control conditions. See ESI,† for more detailed information.
Proteomics with membrane fractionation
RPMI-8226 WT and BTZ-resistant cells were seeded in RPMI medium at a density of 1 × 106 cells per ml and incubated for 2 hours. Per cell line, four biological replicates of 3 × 107 cells were washed with PBS and harvested for fractionation using a plasma membrane isolation kit (Abcam, ab65400). Proteomics data were acquired using an UHPLC 1290 system (Agilent, Santa Clara, CA, USA) coupled to an Orbitrap Q Exactive Plus mass spectrometer (Thermo Scientific). See ESI,† for more detailed information.
Extracellular flux analysis
Extracellular flux analysis was performed using a Seahorse Bioscience XFe24 Analyzer. RPMI-8226 WT and BTZ-resistant cells were resuspended at a density of 1 × 106 cells per ml in Seahorse XF Base medium (Seahorse Bioscience), supplemented with 25 mM glucose and 1 M NaOH, in the presence or absence of 2 mM L-glutamine. 1 × 105 cells (100 μl) were added to 5 wells of XF24 cell culture microplates (Seahorse Bioscience, Agilent). After 30 minutes, microplates were centrifuged at 400 rpm to ensure cells were adhered to the bottom of the plates. 425 μl of corresponding media was added and cells were incubated for 60 min at 37 °C. The oxygen consumption rate (OCR) in pmol O2/min was measured in triplicate at basal conditions, and after the injection of 1 μM oligomycin, 0.1 μM FCCP and 2 μM of Rotenone and Antimycin A after 18, 36 and 54 min, respectively. After injections, measurements of 3 min were performed in triplicate, preceded by 1 min of mixture time and 2 min waiting time. OCR coupled ATP production was calculated as the difference in OCR at basal conditions and after the addition of oligomycin.
Cell viability and cell growth assays
Cells were suspended in triplicate at a density of 2–5 × 105 cells per ml in RPMI-1640 medium in 96-well plates and incubated with drugs at the indicated concentrations for 24–48 hours. Cell growth was monitored continuously with the IncuCyte live-cell imager system (Essen Bioscience, Hertfordshire, UK). Images were acquired every 2 hours for 1–2 days, and analysed using the IncyCyte Zoom software (Essen Bioscience). Cell growth was defined as population doublings per 24–48 hours and calculated based on increased confluence.
Cell death was assessed after 24–48 hours by incubating each well with 30 μM propidium iodide and measuring fluorescence after 15 minutes using the IncuCyte instrument. Cell death was calculated based on the area of the fluorescent signal, normalized to confluency of the wells. For experiments using a combination of simvastatin and BTZ, cells were suspended in triplicate at a density of 1 × 105 cells per ml in RPMI-1640 medium in 96-well plates and pre-incubated with simvastatin with the stated concentrations. After 24 hours, BTZ was added and cell viability was measured after 48 hours by incubation of cells with 50 μM resazurin for 2–4 hours, after which absorption was measured at 570 nm and 600 nm using a microplate reader. Results were calculated by subtraction of background absorbance at 600 nm from absorbance at 570 nm.
Gene expression analysis
Gene expression data from clinical MM patients treated with BTZ or dexamethasone were obtained from a public domain Gene Expression Omnibus dataset (accession number GSE9782).26 In this genomics study with patients from four clinical trials, purified MM samples were collected prior to treatment with BTZ (188 patients) or dexamethasone (DEX) (76 patients). Patients were classified based on their response to therapy: Complete response, partial response, minimal response, no change, or progressive disease. Patients with no change or progressive disease were considered non-responders.26
The population-wide expression of each gene was normalized to mean = 0 and SD = 1 and the mean expression of the OXPHOS and TCA cycle KEGG pathways were calculated for each patient. This patient-mean pathway expression was compared between responders and non-responders using t-tests and boxplots. The odds of non-response based on higher vs. lower than average pathway expression was estimated with a logistic regression model. The overall survival of patients with a higher vs. lower than average pathway expressions was compared using Cox proportional hazard models and survival curves. The proportionality assumption was checked by testing the linearity of the Schoenfeld residuals against log time and was valid for all reported models (P > 0.05).
All gene expression analyses were done in R using the Bioconductor platform27 and the GEOquery28 and GAGE29 packages.
Cholesterol and CoQ analysis by LC-MS
Cells were resuspended in fresh RPMI medium at a density of 1 × 106 cells per ml in the presence of absence of 1 μM simvastatin, atorvastatin or rosuvastatin. After 24 hours, samples were washed with PBS and harvested by centrifugation for 5 min at 1000 g at 4 °C. Cells were resuspended in 800 μL PBS and transferred to round bottom glass tube. Cholesterol and CoQ were extracted according to Bligh and Dyer.30 LC-MS analysis was performed on an Q-Exactive HF mass spectrometer (Thermo Scientific) coupled to a Vanquish autosampler and pump (Thermo Scientific). See ESI,† for more detailed information.
5T33 MM in vivo studies
C57BL/KalwRij mice (Envigo Laboratories, Horst, Holland) were housed and treated according to procedures approved by the Ethical Committee for Animal Experiments of the Vrije Universiteit Brussel (project number: 19-281-5). Female mice were injected intravenously (i.v.) on day 0 with 5 × 105 5T33MM cells and divided into four groups: vehicle, single agent groups: BTZ or simvastatin alone and combinations of simvastatin with BTZ. Starting from day 1, mice were treated either with 40 milligrams per kilograms (mpk) simvastatin 6×/week intraperitoneally (IP) for the designated treatment groups. Starting from day 2, 0.6 mpk bortezomib was given twice per week subcutanously (SC). Injection volumes did not exceed 100 μl. The vehicle for simvastatin is composed of 10% DMSO, 10% Tween 80 and saline. At day 18, spleens were harvested and weighed, the percent plasma cells in the BM was determined on May Grunwald-Giemsa stained BM smears from one femur, and the M spike was measured as described.31
Results
Expression of mitochondrial gene signatures is associated with poor response and outcome to BTZ treatment specifically
Several studies have linked increased activity of metabolic pathways in mitochondria to BTZ resistance in MM.15–17 We first questioned whether such mitochondrial signatures are specifically associated with BTZ response or a general feature of drug-resistant MM. Therefore, we correlated gene-expression signatures of metabolic pathways to treatment outcome and overall survival of treatment-naïve MM patients on BTZ (n = 188) or dexamethasone (n = 76) therapy, using a public set of genome-wide data from four clinical trials.26 The expression of both OXPHOS enzymes (KEGG hsa00190, 132 genes, 110 measured) and TCA cycle enzymes (KEGG hsa00020, 30 genes, 29 measured) were associated with BTZ response and patient survival (Fig. 1). Patients with higher than average OXPHOS expression had 2.2-fold odds of being unresponsive to BTZ (95%CI 1.2–4.1) and a 1.6-fold hazard of death (95%CI 1.1–2.2) compared to those with lower than average expression (Fig. 1a and b). Patients with higher than average TCA cycle expression had 1.9-fold odds of being unresponsive to BTZ (95%CI 1.0–3.5) and a 2.1-fold hazard of death (95%CI 1.4–3.0) compared to those with lower than average expression (Fig. 1c and d). For patients treated with dexamethasone instead of BTZ, no association between OXPHOS or TCA cycle expression and treatment response or overall survival was observed (Fig. S1, ESI†). Thus, these mitochondrial gene-expression signatures are specific to BTZ resistance, rather than a general marker of poor prognosis. Our results indicate that for treatment-naïve patients, a high expression of OXPHOS and TCA cycle-related genes could be used to predict responses to BTZ therapy specifically.
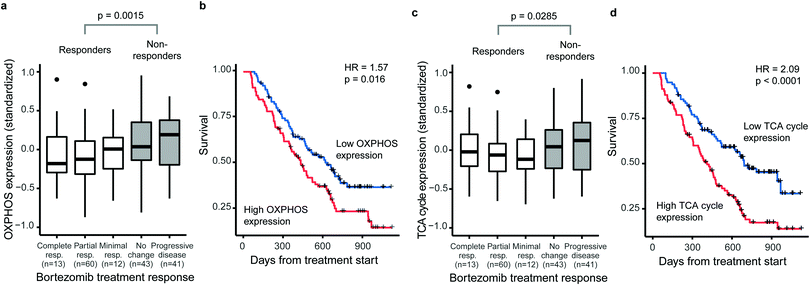 |
| Fig. 1 OXPHOS and TCA cycle overexpression is associated with bortezomib response and survival in multiple myeloma patients. Tumour cell gene expression data was available for 169 multiple myeloma patients before the start of BTZ therapy. After standardising the population-wide expression of each gene to mean = 0, SD = 1, the mean expression of the KEGG oxidative phosphorylation pathway (132 genes, 110 measured) and the KEGG TCA cycle pathway (30 genes, 29 measured) was calculated for each patient. (a) Expression of the OXPHOS pathway before the start of therapy was associated with treatment response to bortezomib. (b) Patients with higher than average OXPHOS pathway expression had worse overall survival under bortezomib therapy. (c) Expression of the TCA cycle pathway before the start of therapy was associated with treatment response to bortezomib. (d) Patients with higher than average TCA cycle pathway expression had worse overall survival under bortezomib therapy. Expression by treatment response was compared using t-tests, survival was compared using a Cox Proportional Hazards model. Crosses indicate censoring of survival information. | |
BTZ-resistant cells upregulate the entire mitochondrial metabolic machinery
To investigate whether specific mitochondrial proteins or complexes were upregulated in BTZ resistance, we performed proteomics and metabolomics analysis on BTZ-sensitive wild-type (WT) and BTZ-resistant (BTZ/100) RPMI-8226 MM cells. BTZ/100 cells grow in the presence of 100 nM BTZ23 and have a 48 hour IC50 value ∼250 nM, against ∼7 nm for WT (Fig. S2a, ESI†).18 To improve proteomics coverage of metabolic enzymes, a fractionation strategy was used, separating cytosolic from membrane fractions before measuring these separately. A total of 7210 proteins were identified, with >5380 proteins quantified in at least 2 out of 4 biological replicates. Amongst these, 1807 proteins were involved in cellular metabolism (based on KEGG pathways; Fig. S2b, ESI†). Gene Ontology (GO) enrichment analysis of differentially expressed metabolic enzymes (≥2-fold, p < 0.05) revealed that different processes related to mitochondrial energy metabolism were amongst the highest enriched GO processes in BTZ-resistant cells (Fig. S2c, ESI†). Almost all proteins (Fig. 2a) and metabolites (Fig. 2b) across the TCA cycle and ETC complexes were significantly upregulated in BTZ-resistant cells compared to WT cells. Functionally, BTZ-resistant cells showed an increased basal oxygen consumption rate (OCR) (Fig. 2c) and increased levels of ATP-coupled respiration (Fig. 2d), in line with previous studies.16,17 Combined, these data show that BTZ-resistant cells upregulate the entire mitochondrial energy metabolism machinery, resulting in a more active TCA cycle coupled to increased mitochondrial ATP production (Fig. 2e).
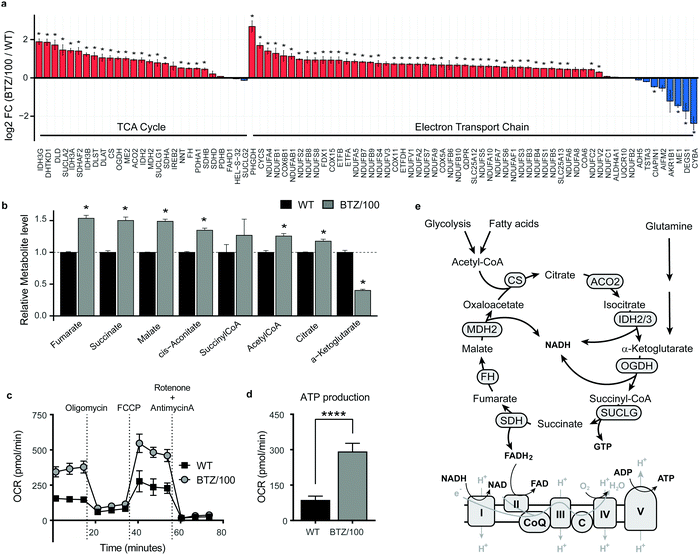 |
| Fig. 2 Bortezomib-resistant cells have higher activity of mitochondrial energy metabolism. (a) Bar graphs display the average log 2 fold change (FC) of metabolic enzymes involved in the TCA cycle (GO:0006099) and electron transport chain (ETC) (GO:0022900). Enzymes that were significantly up- (red) or downregulated (blue) in RPMI-8226 bortezomib-resistant (BTZ/100) cells compared to wild type (WT) are marked with * (b) Bar graphs display the relative metabolite levels of TCA cycle intermediates in RPMI-8226 wild type (WT) cells (black) and bortezomib-resistant (BTZ/100) cells (grey). (c and d) Seahorse XF Cell Mito Stress Test of WT and BTZ/100 cells (c) and ATP production (d). WT and BTZ/100 cells were plated in XF base medium exposed to the Seahorse XF Cell Mito Stress Test, consisting of automated treatment with oligomycin, FCCP, and the combination of antimycin A and rotenone at the indicated times. The oxygen consumption rate (OCR) was measured over time using the XFe24 Analyzer. (e) Schematic overview of the TCA cycle and ETC. Data are mean OCR ± SD (n = 5). One-way ANOVA tests were performed (**** = p < 0.0001, *** = p < 0.001). | |
BTZ-resistant cells use glutamine to sustain a high TCA cycle activity, but are not dependent on glutamine for survival
Successful intervention in mitochondrial energy metabolism requires a detailed understanding of its metabolic wiring to identify potential metabolic dependencies. To investigate which pathways are feeding into mitochondrial metabolism in BTZ-sensitive and -resistant, WT and BTZ/100 cells were grown in the presence of [U-13C]-glucose or [U-13C]-glutamine and the incorporation of 13C–carbon in citrate was followed using mass spectrometry (Fig. 3a). As expected, glutamine was the main carbon source for the TCA cycle in both WT and BTZ/100 cells, as evidenced by the high fraction of labelled citrate from or [U-13C]-glutamine (Fig. 3b). In contrast, BTZ/100 cells showed less incorporation of 13C-glucose as compared to WT cells (Fig. 3c), in line with our previous findings, where we showed that BTZ/100 cells use glucose predominantly to fuel anti-oxidant pathways.18 In addition, BTZ-resistant cells displayed a higher uptake of extracellular glutamine as compared to WT cells (Fig. 3d), together with significantly upregulated levels of GPT2, GOT2 and GLUD1 (≥2-fold, p < 0.05; Fig. S2d, ESI†). Although both WT and BTZ/100 MM cells predominantly use glutamine to fuel their TCA cycle, the higher TCA cycle activity in BTZ/100 cells likely necessitates an increased consumption of glutamine. OCR measurements indeed confirmed that glutamine is a major source for mitochondrial energy production in BTZ/100 cells, as starving the cells from glutamine resulted in a severe drop in ATP production in BTZ/100 cells specifically (Fig. 3e and f).
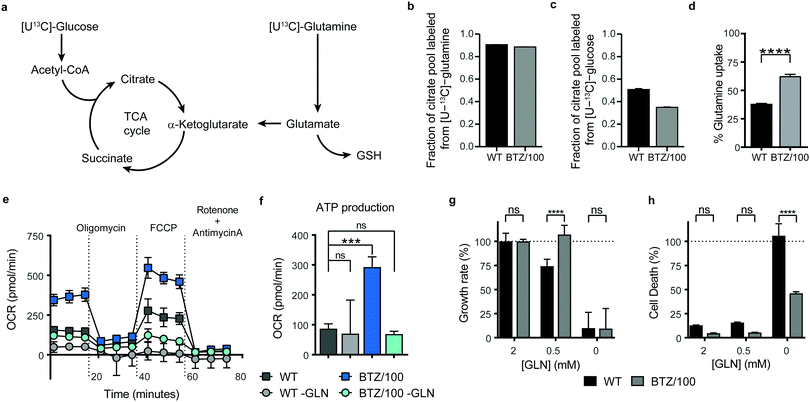 |
| Fig. 3 Bortezomib-resistant cells are less dependent on extracellular glutamine for survival. (a) Schematic overview of the TCA cycle and the two important carbon sources glucose and glutamine. (b and c) LC-MS analysis of intracellular citrate fractions in RPMI-8226 wild type (WT) (black) and bortezomib resistant (BTZ/100) cells (grey). Cells were grown in complete medium containing 25 mM [U-13C]-D-glucose and 2 mM 12C-glutamine (b) or 25 mM 12C-D-glucose and 2 mM [U-13C] glutamine (c). Intracellular metabolites were extracted after 4 hours, followed by LC-MS analysis. Data are means ± SD (n = 3) of 13C-labeled fraction of total citrate pool. (d) LC-MS analysis of extracellular glutamine levels in the culture medium of RPMI-8226 wild type (WT) and bortezomib resistant (BTZ/100) cells. Cells were suspended in RPMI medium containing 1 mM L-glutamine. Media samples were collected after 8 hours. Results represent uptake of glutamine as compared to cell-free media (n = 3). (e and f) Seahorse XF Cell Mito Stress Test of WT and BTZ/100 cells and ATP production in the presence and absence (–GLN) of extracellular glutamine, consisting of automated treatment with oligomycin, FCCP, and the combination of antimycin A and rotenone at the indicated times. Data are mean OCR ± SD (n = 5). (g and h) Growth rate (g) and cell death (h) of WT and BTZ/100 cells after a 24 hour treatment with decreasing concentrations of glutamine (GLN) present in the culture medium. Results represent % growth rate (g) or % cell death (h) ± SD compared to non-treated controls (n = 3). One-way ANOVA tests were performed (**** = p < 0.0001, *** = p < 0.001). | |
We next investigated whether the increased glutamine consumption of BTZ/100 cells also constituted a metabolic vulnerability by culturing cells under glutamine-starved conditions. Surprisingly, lowering the extracellular glutamine concentration to 0.5 mM resulted in a significant growth inhibition in WT cells only (Fig. 3g). Moreover, complete glutamine starvation induced significantly more cell death in WT cells compared to BTZ-resistant cells (Fig. 3h) and re-addition of extracellular glutamine after a 24 h period of glutamine starvation restored cell proliferation in BTZ/100 cell only (Fig. S3a, ESI†). The same phenotype was seen when we treated WT and BTZ/100 cells with the allosteric glutaminase inhibitor BPTES. While BPTES reduced cell viability in both WT and BTZ/100 cells (Fig. S3b, ESI†), no cell death was seen in BTZ/100 cells (Fig. S3c, ESI†). Finally, while WT cells showed no measurable basal respiration in the absence of glutamine, the OCR of BTZ/100 cells without glutamine was comparable to the OCR of WT cells under control conditions (Fig. 3e). To study how BTZ/100 cells maintain mitochondrial activity in the absence of glutamine, we cultured cells for 4 hours under glutamine-starved conditions in the presence of 13C-glucose. In the absence of glutamine, the levels of TCA cycle intermediates dropped in both cell types (Fig. S3d, ESI†), as expected. However, in line with the mitochondrial activity measurements BTZ/100 cells maintained significantly higher levels citrate and succinate than WT cells (Fig. S3d, ESI†). In addition, NADH levels in BTZ/100 cells remained stable, while these significantly decreased in WT cells (Fig. S3d, ESI†). Notably, a large part of citrate and succinate remained unlabeled from 13C-glucose, also in the absence of glutamine (Fig. S3d, ESI†), suggesting that BTZ/100 cells can switch carbon sources other than glucose and glutamine for the production of TCA cycle intermediates. Together, our results demonstrate that under normal conditions, BTZ-resistant cells use more extracellular glutamine for metabolic processes in the mitochondria, but also have a higher metabolic flexibility, allowing these cells to cope with glutamine starvation.
The mevalonate pathway through CoQ production is an important metabolic driver of BTZ resistance
As BTZ-resistant cells have developed a metabolic plasticity that allows them to counteract interventions in TCA-cycle feeder pathways, we next turned our attention to OXPHOS and performed cell growth assays in the presence of ETC complex inhibitors (Fig. 4a). Complex I inhibitors showed 25–50 fold lower IC50 values on cell growth in BTZ/100 cells compared to WT cells (Phenformin: 193 μM vs. 3.86 μM (Fig. 4b); Metformin: 19.4 mM vs. 0.81 mM; Rotenone: 236 nM vs. 5.65 nM (Fig. S4a, ESI†)), whereby complex I inhibition appeared cytostatic rather than cytotoxic (Fig. S4b, ESI†). In contrast, Complex III (Antimycin A) and ATP synthase (Oligomycin) inhibitors showed no differences IC50 values between both cell types (2.28 nM vs. 1.46 nM and 4.78 nM vs. 4.27 nM respectively; Fig. 4b), suggesting that the first steps in the ETC potentially constitute a metabolic vulnerability in BTZ-resistant cells.
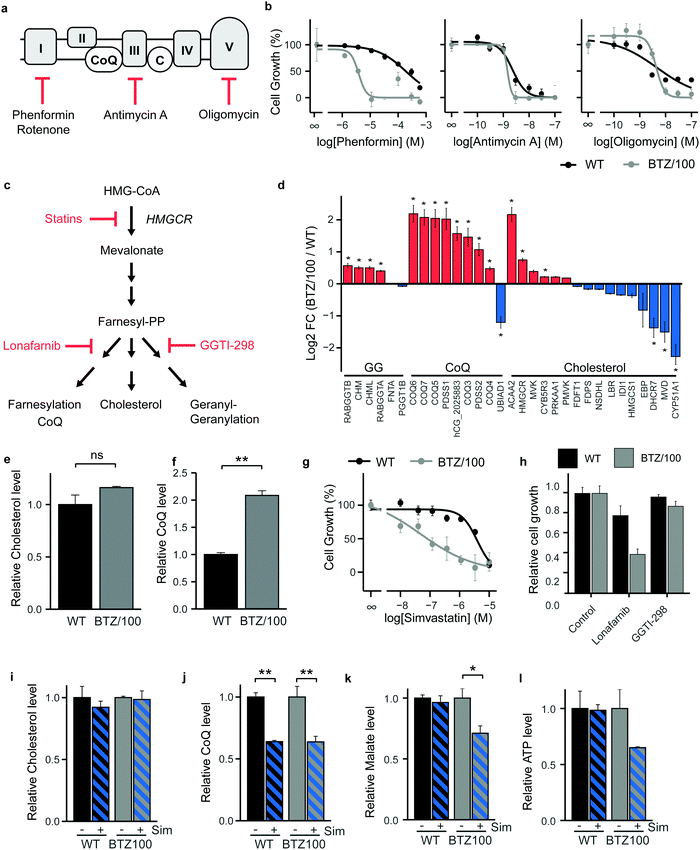 |
| Fig. 4 Bortezomib-resistant cells have elevated Coenzyme Q levels and are more vulnerable to simvastatin-induced OXPHOS inhibition. (a) Schematic representation of the electron transport chain and used inhibitors. (b) Growth rate of RPMI-8226 wild type (WT) (black) and bortezomib-resistant (BTZ/100) (grey) cells after a 48 hour treatment with increasing concentrations of Phenformin, Antimycin A and Oligomycin. Results represent % growth rate ± SD compared to non-treated controls (n = 3). (c) Schematic representation of mevalonate pathway and inhibitors. Statins inhibit HMG-CoA reductase (HMGCR), the rate limiting step of the mevalonate pathway. (d) Bar graphs display the average log 2 FC of metabolic enzymes involved in the Geranylgeranylation (GO:0018344), CoQ biosynthesis (GO:0006744) and cholesterol biosynthesis (GO:0006695). Enzymes that were significantly up- (red) or downregulated (blue) in BTZ/100 cells compared to WT are marked with *. (e and f) LC-MS analysis of intracellular cholesterol (e) and CoQ (f) levels in WT (black) and BTZ/100 cells (grey). (g) Growth rate of WT (black) and bortezomib-resistant (BTZ/100) cells after a 48 hour treatment with increasing concentrations of simvastatin. Results represent % growth rate ± SD compared to non-treated controls (n = 3). (h) Growth rate of WT and bortezomib-resistant (BTZ/100) cells after a 48 hour treatment with 1 μM of Lonafarnib or GGTI-298. Results represent % growth rate ± SD compared to non-treated controls (n = 3). (i and j) LC-MS analysis of intracellular cholesterol (i) and CoQ (j) levels in WT (black) and BTZ/100 cells (grey) after a 24 hour treatment with 1 μM simvastatin (k and l) LC-MS analysis of intracellular Malate (k) and ATP (l) levels in WT (black) and BTZ/100 cells (grey) after 24 hours treatment with 1 μM simvastatin. Data represents relative peak area of metabolite ± SD compared to non-treated controls (n = 3). One-way ANOVA tests were performed (* = p < 0.05). | |
Coenzyme Q10 (CoQ) acts as a mitochondrial electron carrier and is therefore important for ETC complex I and complex II activity (Fig. 4a). CoQ is a downstream product of the mevalonate pathway, which is also involved in synthesis of cholesterol, dolichols and prenyl units (Fig. 4c). Notably, our proteomics data identified enzymes selectively involved in CoQ synthesis to be amongst the highest upregulated proteins in BTZ-resistant cells (Fig. 4d). Proteins that are shared between the cholesterol and CoQ biosynthesis pathways (HMGCR, ACAA2 and MVK) were also upregulated, while downstream enzymes that are specifically involved in cholesterol synthesis were downregulated in BTZ/100 cells. In line with the proteomics data, intracellular levels of CoQ were significantly upregulated in BTZ/100 cells as compared to WT cells (Fig. 4e and f), while no significant difference in cholesterol was seen (Fig. 4f and e). To investigate whether increased CoQ levels are a general feature of BTZ-resistant cells, we also included BTZ-sensitive AMO-1 MM cells and its BTZ- and carfilzomib-resistant counterpart, and WT and BTZ-resistant ARH-77 cells.16,24 Both AMO-1 and ARH-77 BTZ-resistant cells had no increased levels of CoQ (Fig. S4c, ESI†). However, higher levels of enzymes involved in CoQ synthesis were found in AMO-1 BTZ-resistant MM cells in another study, as well as in BTZ-resistant HL-60 cells,16 suggesting that BTZ-resistant cells have higher activity CoQ biosynthesis, to support increased OXPHOS.
While the HMG-CoA reductase (HMGCR) inhibitor simvastatin is widely used as a cholesterol blocker, recent studies show that in cancer, simvastatin predominantly lowers CoQ levels, while less effect is seen on cholesterol levels.32 Simvastatin, via CoQ, therefore provides an alternative route for ETC inhibition in cancer cells.32–35 We next questioned whether the mevalonate pathway and downstream CoQ synthesis can be targeted in BTZ/100 cells to overcome resistance. WT and BTZ/100 cells were first exposed to several statins (HMGCR inhibitors). BTZ/100 cells showed an increased sensitivity for simvastatin (Fig. 4g) and atorvastatin compared to WT cells, while rosuvastatin was not effective in either cell line (Fig. S4d, ESI†). In contrast to the cytostatic complex I inhibitor phenformin, simvastatin showed cytotoxic effects on BTZ/100 but not WT cells (Fig. S4b, ESI†). While AMO-1 and ARH-77 BTZ-resistant cells showed no increased intracellular CoQ levels, simvastatin treatment resulted in a significant decrease in cell viability, as compared to their WT counterpart (Fig. S4e, ESI†), confirming that BTZ-resistant cells are more sensitive to simvastatin.
To demonstrate that the effect of simvastatin is via its action on CoQ synthesis, rather than other downstream pathways of the mevalonate pathway, we tested the effect of Lonafarnib (Farnesyl-transferase inhibitor) and GGTI-298 (Geranylgeranyltransferase inhibitor) (Fig. 4c and h). Indeed, GGTI-298 showed no effect on cell growth in both WT and BTZ/100 cells. Lonafarnib on the other hand induced a similar reduction of cell growth as simvastatin.
To further support the hypothesis that simvastatin acts on BTZ/100 cells via interfering with CoQ synthesis and that this, in turn, lowers mitochondrial metabolism, we performed a metabolomic screen of WT and BTZ-resistant cells in the presence and absence of simvastatin. Simvastatin significantly lowered CoQ, but not cholesterol levels in both WT and BTZ/100 cells (Fig. 4i and j), as well as in the panel of AMO-1 and ARH-77 cells (Fig. S4f, ESI†). The effect of simvastatin on CoQ levels in AMO-1 cells is smaller than in the other cell lines, which corresponds to the lower effect of simvastatin on cell viability in these cells. Atorvastatin and rosuvastatin also lowered CoQ levels in WT and BTZ/100 cells, although the effect of rosuvastatin was less pronounced, in line with the effect on cell growth (Fig. S4g, ESI†). Together, these data indicate that the effect of statins in BTZ-resistant cells is correlated to the decrease in intracellular CoQ levels.
In addition, the TCA cycle intermediate malate decreased upon simvastatin treatment in BTZ/100 but not WT cells, indicative of lower TCA cycle activity (Fig. 4k). The substantially, albeit not significantly, lower levels of ATP in BTZ/100 cells further support the notion that mitochondrial energy metabolism is affected in BTZ/100 but not WT cells (Fig. 4l). BTZ/100 cells also showed a higher secretion of lactate after simvastatin treatment (Fig. S4h, ESI†), indicating that BTZ-resistant cells increase their glycolytic activity in response to simvastatin, likely to compensate for the decrease in mitochondrial energy production.32,35
Simvastatin enhances the effect of Bortezomib in vitro and in vivo
Because high levels of CoQ appear to be important for cells to become resistant to BTZ, we next investigated if lowering CoQ levels with simvastatin would enhance the effect of BTZ. Hence, we pre-incubated WT and BTZ-resistant cells with simvastatin for 24 hours, before exposing cells to BTZ. Indeed, preincubation with simvastatin enhanced the sensitivity towards BTZ of both sensitive and resistant RPMI-8226 cells (Fig. 5a) and in AMO-1 WT, ARH-77 WT and BTZ-resistant cells (Fig. S4i, ESI†). No significant effect was seen in AMO-1 BTZ-resistant cells, but simvastatin also resulted in a minor decrease in CoQ levels in these cells (Fig. S4f, ESI†).
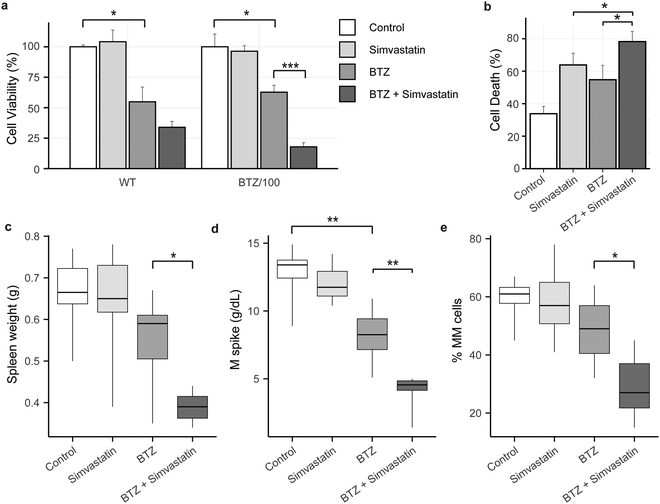 |
| Fig. 5 Simvastatin synergizes with Bortezomib in vitro and in vivo. (a) Cell viability of WT (black) and bortezomib-resistant (BTZ/100) cells after a 48 hour treatment with bortezomib (5 nM and 200 nM respectively) with and without a 24 hour preincubation with 100 nM Simvastatin. Results represent % cell viability ± SD compared to non-treated controls ((n = 3).). (b) Cell death of 5T33MMvv cells after 24 hour treatment with 5 μM simvastatin, 2 nM Bortezomib or the combination. Results represent % cell death ± SD (n = 3). (c–e) The effect of simvastatin with and without bortezomib treatment on different multiple myeloma parameters in mice. Data are means ± SD (n = 9) of each treatment group. (c) Spleen weight (g) as indication of tumour load. (d) M-spike, which was analysed by protein electrophoresis on collected serum (g dl−1). (e) Analysis of plasmacytosis on May-Grunwald-Giemsa stained cytosmears (% MM cells on total number of cells). One-way ANOVA tests were performed (* = p < 0.05, ** = p < 0.01). | |
Finally, we investigated whether simvastatin, in combination with BTZ, could reduce tumour load in vivo. We used the 5T33MM mouse model, which is an immune competent model that faithfully recaptures the human disease, with MM tumours secreting M-proteins, residing in the bone marrow, and migrating to hematopoietic organs including the spleen.31 The combination of BTZ and simvastatin significantly enhanced cell death in 5T33MM cells in vitro (Fig. 5b), confirming that the combination of simvastatin and BTZ works in vitro in this model system. In vivo, BTZ treatment reduced the tumour burden in the bone marrow by 19% (Fig. 5e) and serum M protein levels by 37% (Fig. 5d), while simvastatin alone had no effect on these parameters. The combination of simvastatin and BTZ significantly reduced the serum M protein level, tumour burden and spleen weight as compared to BTZ treatment alone (tumourload: 28.6% MM cells in combo compared to 48.5% in BTZ group; M spike: 4.2 g dl−1vs. 8.1 g dl−1; spleen weight: 0.38 g vs. 0.54 g) (Fig. 5c–e). Together, these results show that simvastatin synergizes with BTZ both in vitro and in vivo and pose mevalonate pathway inhibitors as novel compounds for combination treatment with BTZ or to treat BTZ-resistant patients.
Discussion
Despite the positive developments due to targeted cancer therapies, resistance to therapeutic agents is acknowledged as a major problem. This is especially true for MM, which remains an incurable disease mainly due to the occurrence of drug resistance. Considering that metabolism plays an essential role in resistance against anti-cancer drugs,36 we aimed to identify metabolic pathways involved in BTZ resistance, targeting of which would work synergistically with BTZ treatment to ultimately improve treatment efficacy. Publicly-available gene-expression data from MM patient samples identified a priori upregulated TCA cycle and OXPHOS pathways as predictive of intrinsic clinical BTZ resistance and poor prognosis, as has previously been described for a mitochondrial biogenesis signature.26,37 Importantly, signatures are specific to BTZ resistance, rather than a general marker of poor prognosis. The same pattern is visible also in cell lines that acquired BTZ resistance through exposure to the drug, suggesting that an upregulated TCA cycle and ETC activity is core feature of BTZ-resistant MM.
Mechanistically, we show that BTZ-resistant cells in vitro have higher activity of the TCA cycle and that glutamine is the major source for TCA cycle in both BTZ-sensitive and -resistant cells. Many cancer cells are highly dependent on glutamine for survival and proliferation12,38 and withdrawal of glutamine from the culture media results in growth arrest and cell death of (drug-sensitive) MM cells.11,13 Our results show that upon acquiring BTZ-resistance, MM cells remain dependent on glutamine for proliferation, but no longer for survival. Moreover, our data show that under conditions of glutamine starvation, BTZ-resistant cells are able to retain higher levels of TCA cycle intermediates and mitochondrial oxygen consumption rates. Although the exact pathways that fuel to TCA cycle under these conditions remain to be identified, oxidation of branched chain amino acids or fatty acids and scavenging pathways that use macromolecules to generate metabolites39,40 are all likely candidates. A previous study showed that proteasome inhibitor-resistant AMO-1 cells are more sensitive to glutaminase inhibition compared to wild-type cells,17 especially in combination with carfilzomib. It is not clear why proteasome-inhibitor resistant AMO-1 appear to react differently to inhibition of glutamine metabolism. However, RAS mutations are known to modulate glutamine metabolism and the sensitivity to glutamine metabolism inhibitors.41,42 Although the RAS status in the bortezomib-resistant cell lines is not known, their WT counterparts harbour different KRAS mutations.43 It is therefore conceivable that a different mutational KRAS status contributes to the differences in sensitivity between these two cell lines. The sensitivity to glutamine metabolism inhibition could also vary depending on the proteasome inhibitor used. The overexpression of the drug efflux transporter ABCB1/P-glycoprotein, an ATP-dependent efflux pump, is an important underlying mechanism of carfilzomib resistance.16,44 This dependence on ATP production may make carfilzomib-resistant cells particularly sensitive to the sudden decrease in ATP-production capacity that would result from glutamine starvation or glutaminase inhibition.
Importantly, we are the first to link high CoQ levels to BTZ response and show that inhibition of CoQ synthesis through the HMGCR inhibitor simvastatin or the farnesyl-transferase inhibitor lonafarnib is more effective in BTZ-resistant cells. Simvastatin strongly enhanced the effect of BTZ in both WT and BTZ-resistant cell lines in vitro and in vivo, proposing combination treatments of BTZ and mevalonate pathway inhibition as a novel strategy to treat (intrinsically) BTZ-resistant patients. The identified TCA cycle/OXPHOS signature could be of use to predict BTZ response in patients and stratify patients that would particularly benefit from combined simvastatin/bortezomib treatment.
Simvastatin is an approved drug for the treatment of hypercholesterolaemia and is currently under investigation for cancer therapy.45,46 Besides lowering cholesterol, statins lower the levels of CoQ in serum47,48 and are known to decrease mitochondrial respiration and increase oxidative stress.33,34 Recent studies show that simvastatin has limited effect on cholesterol levels in cancer cells and that the effect in cancer is merely a result of lowering CoQ levels, resulting in impaired OXPHOS.32,35 In line with these findings, our data also point towards changes in CoQ synthesis rather than cholesterol in the mode of action of simvastatin in BTZ-resistant MM.
Statins have been shown to overcome cell adhesion-mediated drug resistance in MM by geranylgeranylation in a co-culture system of MM and bone marrow stromal cells49 and a small pilot study showed that simvastatin overcomes drug resistance in refractory MM.50 However, the RPMI-8226 cells used in our study are not adherent and no stromal cells were present during the experiments. A recent study showed that MM are dependent on the mevalonate pathway, resulting to statin sensitivity, acting via geranylgeranylation.51 Geranylgeranylation inhibition with GGTI-298 had no effect in both WT and BTZ-resistant cells, indicating that geranylgeranylation does not play a role in BTZ-resistant RPMI-8226 cells. Instead, our data support inhibition of CoQ synthesis as a novel mechanism via which statins can overcome drug resistance. Statins are associated with a 24% reduction in the hazard of multiple-myeloma specific mortality in a propensity-matched cohort of 2656 patients,52 supporting preclinical data. Our data provides novel insights in the relation of simvastatin and BTZ response in patients and we are the first to link energy metabolism, CoQ synthesis and statin treatment to BTZ resistance.
Conclusions
In conclusion, our findings demonstrate that an increased TCA cycle activity is associated with higher energy production via the ETC and that this is a core feature of both intrinsic and acquired drug resistance. Importantly, we show that CoQ pathway inhibition with simvastatin enhances the effect of BTZ both in vitro and in vivo. Our data provide a rationale for the combination of simvastatin and BTZ as a novel therapy to target BTZ resistance.
Ethics approval and consent to participate
The genome-wide expression data of MM tumour samples was obtained from the NCBI Gene Expression Omnibus. Ethics approval and informed consent was obtained as described in the original study.26
Data availability
Relevant data and materials within this work are made available in this manuscript and its supplementary information files. The genome-wide expression data of MM tumour samples was obtained from the NCBI Gene Expression Omnibus, accession number GSE9782. The mass spectrometry proteomics data were deposited to the public database ProteomeXchange via the PRIDE partner repository, project PXD20850. Any additional information is available from the corresponding author on reasonable request.
Authors’ contributions
CRB and EAZ developed this study, designed the experiments and wrote the manuscript. EAZ, PL, SL, GJJvS and WW performed the experiments. IO and EM performed in vivo studies. HJdG performed gene expression analysis. JWAJ performed cholesterol and CoQ analysis. EAZ, WW and CRB performed data analysis and interpretation. All authors approved the final manuscript.
Conflicts of interest
All authors declare to have no conflict of interest regarding this manuscript.
Acknowledgements
The authors would like to thank Boudewijn Burgering and Maaike Meerlo for their assistance in extracellular flux analysis, Christoph Driessen for BTZ- and CFZ-resistant cell lines, and Albert Heck for constructive discussions. IO was supported by FWO (Vlaanderen), EM by Kom Op Tegen Kanker (Vlaanderen) and the International Myeloma Foundation.
References
- S. K. Kumar, V. Rajkumar, R. A. Kyle, M. van Duin, P. Sonneveld, M.-V. Mateos, F. Gay and K. C. Anderson, Nat. Rev. Dis. Prim., 2017, 3, 17046 CrossRef PubMed.
- A. Gulla and K. C. Anderson, Haematologica, 2020, 105, 2358–2367 CrossRef CAS PubMed.
- K. C. Anderson, J. Clin. Oncol., 2012, 30, 445–452 CrossRef CAS PubMed.
- R. Z. Orlowski and D. J. Kuhn, Clin. Cancer Res., 2008, 14, 1649–1657 CrossRef CAS PubMed.
- D. Hanahan and R. A. Weinberg, Cell, 2011, 144, 646–674 CrossRef CAS PubMed.
- L. Puchades-Carrasco, R. Lecumberri, J. Martínez-López, J.-J. Lahuerta, M.-V. Mateos, F. Prósper, J. F. San-Miguel and A. Pineda-Lucena, Clin. Cancer Res., 2013, 19, 4770–4779 CrossRef CAS.
- C. Ludwig, D. S. Williams, D. B. Bartlett, S. J. Essex, G. McNee, J. W. Allwood, E. Jewell, A. Barkhuisen, H. Parry, S. Anandram, P. Nicolson, C. Gardener, F. Seymour, S. Basu, W. B. Dunn, P. A. H. Moss, G. Pratt and D. A. Tennant, Blood Cancer J., 2015, 5, e359 CrossRef CAS PubMed.
- S. Fujiwara, Y. Kawano, H. Yuki, Y. Okuno, K. Nosaka, H. Mitsuya and H. Hata, Br. J. Cancer, 2013, 108, 170–178 CrossRef CAS.
- C. El Arfani, K. De Veirman, K. Maes, E. De Bruyne and E. Menu, Int. J. Mol. Sci., 2018, 19(4), 1200 CrossRef PubMed.
- D. Rizzieri, B. Paul and Y. Kang, J. Cancer Metastasis Treat., 2019, 5, 139–148 Search PubMed.
- M. Bolzoni, M. Chiu, F. Accardi, R. Vescovini, I. Airoldi, P. Storti, K. Todoerti, L. Agnelli, G. Missale, R. Andreoli, M. G. Bianchi, M. Allegri, A. Barilli, F. Nicolini, A. Cavalli, F. Costa, V. Marchica, D. Toscani, C. Mancini, E. Martella, V. Dall’Asta, G. Donofrio, F. Aversa, O. Bussolati and N. Giuliani, Blood, 2016, 128, 667–679 CrossRef CAS PubMed.
- R. J. DeBerardinis, A. Mancuso, E. Daikhin, I. Nissim, M. Yudkoff, S. Wehrli and C. B. Thompson, Proc. Natl. Acad. Sci. U. S. A., 2007, 104, 19345–19350 CrossRef CAS PubMed.
- R. Bajpai, S. M. Matulis, C. Wei, A. K. Nooka, H. E. Von Hollen, S. Lonial, L. H. Boise and M. Shanmugam, Oncogene, 2016, 35, 3955–3964 CrossRef CAS.
- W. I. Gonsalves, V. Ramakrishnan, T. Hitosugi, T. Ghosh, D. Jevremovic, T. Dutta, D. Sakrikar, X.-M. Petterson, L. Wellik, S. K. Kumar and K. S. Nair, JCI Insight, 2018, 3, 1–16 CrossRef PubMed.
- P. Maiso, D. Huynh, M. Moschetta, A. Sacco, Y. Aljawai, Y. Mishima, J. M. Asara, A. M. Roccaro, A. C. Kimmelman and I. M. Ghobrial, Cancer Res., 2015, 75, 2071–2082 CrossRef CAS PubMed.
- G. P. Soriano, L. Besse, N. Li, M. Kraus, A. Besse, N. Meeuwenoord, J. Bader, B. Everts, H. den Dulk, H. S. Overkleeft, B. I. Florea and C. Driessen, Leukemia, 2016, 30, 2198–2207 CrossRef CAS PubMed.
- R. M. Thompson, D. Dytfeld, L. Reyes, R. M. Robinson, B. Smith, Y. Manevich, A. Jakubowiak and M. Komarnicki, Oncotarget, 2017, 8, 35863–35876 CrossRef.
- E. A. Zaal, W. Wu, G. Jansen, S. Zweegman, J. Cloos and C. R. Berkers, Cancer Metab, 2017, 5, 7 CrossRef PubMed.
- I. S. Song, H. K. Kim, S. R. Lee, S. H. Jeong, N. Kim, K. S. Ko, B. D. Rhee and J. Han, Int. J. Cancer, 2013, 133, 1357–1367 CrossRef CAS PubMed.
- P. Tsvetkov, A. Detappe, K. Cai, H. R. Keys, Z. Brune, W. Ying, P. Thiru, M. Reidy, G. Kugener, J. Rossen, M. Kocak, N. Kory, A. Tsherniak, S. Santagata, L. Whitesell, I. M. Ghobrial, J. L. Markley, S. Lindquist and T. R. Golub, Nat. Chem. Biol., 2019, 15, 681–689 CrossRef CAS PubMed.
- S. Maharjan, M. Oku, M. Tsuda, J. Hoseki and Y. Sakai, Sci. Rep., 2014, 4, 5896 CrossRef CAS PubMed.
- L. Besse, A. Besse, M. Mendez-Lopez, K. Vasickova, M. Sedlackova, P. Vanhara, M. Kraus, J. Bader, R. B. Ferreira, R. K. Castellano, B. K. Law and C. Driessen, Haematologica, 2019, 104, e415–e419 CrossRef CAS.
- N. E. Franke, D. Niewerth, Y. G. Assaraf, J. van Meerloo, K. Vojtekova, C. H. van Zantwijk, S. Zweegman, E. T. Chan, C. J. Kirk, D. P. Geerke, A. D. Schimmer, G. J. L. Kaspers, G. Jansen and J. Cloos, Leukemia, 2012, 26, 757–768 CrossRef CAS.
- T. Rückrich, M. Kraus, J. Gogel, A. Beck, H. Ovaa, M. Verdoes, H. S. Overkleeft, H. Kalbacher and C. Driessen, Leukemia, 2009, 23, 1098–1105 CrossRef.
- J. M. Buescher, M. R. Antoniewicz, L. G. Boros, S. C. Burgess, H. Brunengraber, C. B. Clish, R. J. DeBerardinis, O. Feron, C. Frezza, B. Ghesquiere, E. Gottlieb, K. Hiller, R. G. Jones, J. J. Kamphorst, R. G. Kibbey, A. C. Kimmelman, J. W. Locasale, S. Y. Lunt, O. D. K. Maddocks, C. Malloy, C. M. Metallo, E. J. Meuillet, J. Munger, K. Nöh, J. D. Rabinowitz, M. Ralser, U. Sauer, G. Stephanopoulos, J. St-Pierre, D. A. Tennant, C. Wittmann, M. G. Vander Heiden, A. Vazquez, K. Vousden, J. D. Young, N. Zamboni and S.-M. Fendt, Curr. Opin. Biotechnol, 2015, 34, 189–201 CrossRef CAS PubMed.
- G. Mulligan, C. Mitsiades, B. Bryant, F. Zhan, W. J. Chng, S. Roels, E. Koenig, A. Fergus, Y. Huang, P. Richardson, W. L. Trepicchio, A. Broyl, P. Sonneveld, J. D. Shaughnessy, P. L. Bergsagel, D. Schenkein, D. Esseltine, A. Boral and K. C. Anderson, Blood, 2007, 109, 3177–3188 CrossRef CAS PubMed.
- W. Huber, V. J. Carey, R. Gentleman, S. Anders, M. Carlson, B. S. Carvalho, H. C. Bravo, S. Davis, L. Gatto, T. Girke, R. Gottardo, F. Hahne, K. D. Hansen, R. A. Irizarry, M. Lawrence, M. I. Love, J. MacDonald, V. Obenchain, A. K. Oleś, H. Pagès, A. Reyes, P. Shannon, G. K. Smyth, D. Tenenbaum, L. Waldron and M. Morgan, Nat. Methods, 2015, 12, 115–121 CrossRef CAS PubMed.
- S. Davis and P. S. Meltzer, Bioinformatics, 2007, 23, 1846–1847 CrossRef PubMed.
- W. Luo, M. S. Friedman, K. Shedden, K. D. Hankenson and P. J. Woolf, BMC Bioinf., 2009, 10, 161 CrossRef PubMed.
- E. G. Bligh and W. J. Dyer, Can. J. Biochem. Physiol., 1959, 37, 911–917 CrossRef CAS.
- K. Vanderkerken, H. De Raeve, E. Goes, S. Van Meirvenne, J. Radl, I. Van Riet, K. Thielemans and B. Van Camp, Br. J. Cancer, 1997, 76, 451–460 CrossRef CAS PubMed.
- G. H. McGregor, A. D. Campbell, S. K. Fey, S. Tumanov, D. Sumpton, G. R. Blanco, G. Mackay, C. Nixon, A. Vazquez, O. J. Sansom and J. J. Kamphorst, Cancer Res., 2020, 80, 175–188 CrossRef CAS PubMed.
- R. A. Vaughan, R. Garcia-Smith, M. Bisoffi, C. A. Conn and K. A. Trujillo, Eur. J. Pharmacol., 2013, 711, 1–9 CrossRef CAS PubMed.
- A. Kettawan, T. Takahashi, R. Kongkachuichai, S. Charoenkiatkul, T. Kishi and T. Okamoto, J. Clin. Biochem. Nutr., 2007, 40, 194–202 CrossRef CAS PubMed.
- C. F. Christie, D. Fang, E. G. Hunt, M. E. Morris, A. Rovini, K. A. Heslop, G. C. Beeson, C. C. Beeson and E. N. Maldonado, FASEB J., 2019, 33, 8186–8201 CrossRef CAS PubMed.
- E. A. Zaal and C. R. Berkers, Front. Oncol., 2018, 8, 1–15 CrossRef PubMed.
- X. Zhan, W. Yu, R. Franqui-machin, M. L. Bates, K. Nadiminti, H. Cao, B. A. Amendt, Y. Jethava, I. Frech, F. Zhan and G. Tricot, Oncotarget, 2017, 8, 111213–111224 CrossRef PubMed.
- D. R. Wise and C. B. Thompson, Trends Biochem. Sci., 2010, 35, 427–433 CrossRef CAS PubMed.
- E. Michalopoulou, V. Bulusu and J. J. Kamphorst, Br. J. Cancer, 2016, 115, 635–640 CrossRef CAS.
- J. R. Mayers, M. E. Torrence, L. V. Danai, T. Papagiannakopoulos, S. M. Davidson, M. R. Bauer, A. N. Lau, B. W. Ji, P.
D. Dixit, A. M. Hosios, A. Muir, C. R. Chin, E. Freinkman, T. Jacks, B. M. Wolpin, D. Vitkup and M. G. Vander Heiden, Science, 2016, 353, 1161–1165 CrossRef CAS PubMed.
- E. White, Genes Dev., 2013, 27, 2065–2071 CrossRef CAS PubMed.
- A. C. Kimmelman and E. White, Cell Metab., 2017, 25, 1037–1043 CrossRef CAS PubMed.
- Y. H. T. Lin, G. P. Way, B. G. Barwick, M. C. Mariano, M. Marcoulis, I. D. Ferguson, C. Driessen, L. H. Boise, C. S. Greene and A. P. Wiita, Blood Adv., 2019, 3, 3214–3227 CrossRef PubMed.
- S. E. Verbrugge, Y. G. Assaraf, B. A. C. Dijkmans, G. L. Scheffer, M. Al, D. den Uyl, R. Oerlemans, E. T. Chan, C. J. Kirk, G. J. Peters, J. W. van der Heijden, T. D. de Gruijl, R. J. Scheper and G. Jansen, J. Pharmacol. Exp. Ther., 2012, 341, 174–182 CrossRef CAS PubMed.
- U. E. Martinez-Outschoorn, M. Peiris-Pagés, R. G. Pestell, F. Sotgia and M. P. Lisanti, Nat. Rev. Clin. Oncol., 2017, 14, 11–31 CrossRef CAS.
- L. Galluzzi, O. Kepp, M. G. Vander Heiden and G. Kroemer, Nat. Rev. Drug Discovery, 2013, 12, 829–846 CrossRef CAS PubMed.
- S. Passi, A. Stancato, E. Aleo, A. Dmitrieva and G. P. Littarru, BioFactors, 2003, 18, 113–124 CrossRef CAS PubMed.
- H. Mabuchi, T. Higashikata, M. Kawashiri, S. Katsuda, M. Mizuno, A. Nohara, A. Inazu, J. Koizumi and J. Kobayashi, J. Atheroscler. Thromb., 2005, 12, 111–119 CrossRef CAS PubMed.
- R. Schmidmaier, P. Baumann, M. Simsek, F. Dayyani, B. Emmerich and G. Meinhardt, Blood, 2004, 104, 1825–1832 CrossRef CAS PubMed.
- R. Schmidmaier, P. Baumann, I. Bumeder, G. Meinhardt, C. Straka and B. Emmerich, Eur. J. Haematol, 2007, 79, 240–243 CrossRef CAS PubMed.
- J. Longo, P. Smirnov, Z. Li, E. Branchard, J. E. van Leeuwen, J. D. Licht, B. Haibe-Kains, D. W. Andrews, J. J. Keats, T. J. Pugh, S. Trudel and L. Z. Penn, Leukemia, 2021, 35(3), 796–808 CrossRef CAS PubMed.
- K. M. Sanfilippo, J. Keller, B. F. Gage, S. Luo, T.-F. Wang, G. Moskowitz, J. Gumbel, B. Blue, K. O’Brian and K. R. Carson, J. Clin. Oncol., 2016, 34, 4008–4014 CrossRef CAS PubMed.
Footnotes |
† Electronic supplementary information (ESI) available. See DOI: 10.1039/d1mo00106j |
‡ These authors contributed equally to this work. |
|
This journal is © The Royal Society of Chemistry 2022 |
Click here to see how this site uses Cookies. View our privacy policy here.