DOI:
10.1039/D2MD00154C
(Research Article)
RSC Med. Chem., 2022,
13, 1052-1057
Fragment screening at AstraZeneca: developing the next generation biophysics fragment set†
Received
24th May 2022
, Accepted 29th June 2022
First published on 4th July 2022
Abstract
Fragment based drug discovery is a critical part of the lead generation toolbox and relies heavily on a readily available, high quality fragment library. Over years of use, the AstraZeneca fragment set had become partially depleted and instances of compound deterioration had been found. It was recognised that a redevelopment was required. This provided an opportunity to evolve our screening sets strategy, whilst ensuring that the quality of the fragment set met the robust requirements of fragment screening campaigns. In this communication we share the strategy employed, in particular highlighting two aspects of our approach that we believe others in the community would benefit from, namely that; (i) fragments were selected with input from Medicinal Chemists at an early stage, and (ii) the library was arranged in a layered format to ensure maximum flexibility on a per target basis.
Introduction
Fragment screening at AstraZeneca is celebrating its 20th year in 2022. Over the past two decades a number of important lessons have been learnt at AstraZeneca, both organisationally and scientifically, about how to best apply fragment based drug discovery (FBDD) to deliver a lead series. The lessons shared in previous communications continue to be applied where appropriate, but are continuously improved upon to ensure best practice.1,2 Previously, we highlighted three key changes that significantly impacted the delivery of a lead series from fragment screening, namely; a stronger focus on structurally-enabled targets; a dedicated team of medicinal chemists to work up hits; and a technology-based fragment library.
Whilst the former two points are still fundamental tenants of the approach taken at AstraZeneca, use of our fragment collection over time led to a reduction in the overall quality of both the nuclear magnetic resonance (NMR) and surface plasma resonance (SPR) screening sets. The main issues encountered were the depletion of available stocks and the reduced purity of some of the fragments. Recognising that these needed addressing, we decided to re-evaluate our fragment libraries. A lot has been written about the best approaches for library design3–7 but ultimately the true marker of success is delivery of productive lead series for further optimization and it was felt that the technology-centric strategy employed at AstraZeneca had an excellent track record in that regard. In particular, tailoring the screening sets for a particular project was an approach that still added value. However, with continuing improvements in SPR throughput and sensitivity,8 it was no longer a requirement for these sets to populate different parts of chemical space and to adopt a different number of compounds. It was felt that a layered approach, whereby a single set could be screened sequentially or as a whole would provide the most suitable option. Consequently we opted to evolve our fragment library to a single biophysics fragment set comprised of two layers with layer 1 representing a diverse selection that could be used in both SPR and NMR screens (Fig. 1). This could either be the whole screen or sequentially, before expanding to the wider set. Another consideration was that this could be used as a ‘ligandability’ set.9
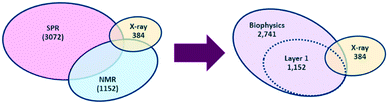 |
| Fig. 1 Transitioning from multiple technology sets to a single layered biophysics fragment set (not to scale). | |
The biophysics fragment set was envisioned to be the workhorse of future fragment screens, that would then be supplemented by a number of target (or technology) specific subsets e.g.19F;10 kinase hinge binder;11 low H-bond donor (HBD);12 epigenetics;13 or metal chelator.14 The X-ray fragment set was not included in this realignment as it was felt that the necessary chemical space for optimal X-ray fragments still required a specific subset (very high solubility, heavy (non-hydrogen) atom count (HAC) <15, molecular weight (MW) <220)15,16 with only partial overlap of our biophysics fragment set. Furthermore, our X-ray collection underwent a refresh in 2019. As a result, the aim of this manuscript is to share with the community the approach taken within AstraZeneca to develop our new biophysics fragment set.
Fragment selection
A central consideration in the approach adopted was ensuring rapid follow up of hits would be possible. Astex and others have reported that synthetic tractability is a key parameter in determining whether fragments can be optimised into lead series.17–20 This is in line with empirical observations at AstraZeneca from previous screening efforts, which had identified validated and well characterised hits, that were then difficult to progress quickly due to a lack of available analogues (near neighbours, NNs) or suitable synthetic methodology. Consequently, we were keen to ensure significant medicinal and synthetic chemistry input was provided to curate and augment the initial diversity based computational selection. This is in contrast to typical approaches that place a higher emphasis on metrics and computational scoring.21,22 Once this initial set of fragments had been identified, they were triaged through a rigorous quality control (QC) process to ensure high fidelity. This was primarily achieved by a liquid chromatography-mass spectrometry (LCMS) purity check, solubility assessment, SPR clean screen, NMR analysis and tris(2-carboxyethyl)phosphine (TCEP) oxidation MS screen (Fig. 2).
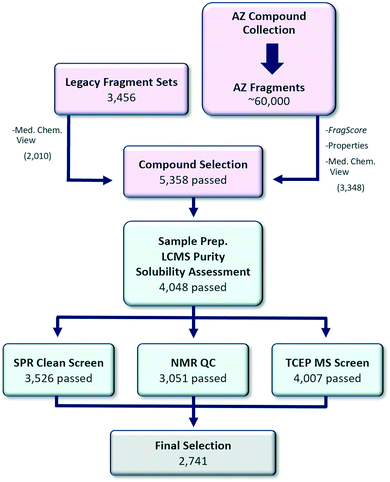 |
| Fig. 2 Overview of the fragment selection process. | |
Computational selection
A set of 5358 candidate fragments progressed to QC came from two sources: (i) the combined AstraZeneca legacy fragment screening sets1 and (ii) the wider AstraZeneca compound collection. First, all available fragments (availability criteria: >30 mg solid or >400 μL 100 mM stock solution) in the legacy screening sets were visually inspected by a team of medicinal chemists to remove compounds that were deemed to either (i) be particularly challenging to follow-up synthetically or (ii) contain undesirable functional groups.23 Of the original 3456 legacy fragments, 2010 remained after the availability and chemistry criteria.
Next, potential replacement fragments in the AstraZeneca compound collection which met the availability requirements were identified. From this selection, approximately 60
000 compounds achieved the following fragment property criteria: (i) HAC: 8–20, (ii) MW: 100–300 Dalton, (iii) clog
P: −2–3, (iv) topological polar surface area (TPSA): <100, (v) number of rotatable bonds (NROT): 0–5, (vi) number of HBD: 0–3, (vii) number of H-bond acceptors (HBA): 0–6, (viii), number of rings (NRings): 0–4, and (ix) passed a set of substructure filters designed to identify unsuitable screening compounds.
Each fragment in the 60
000 set was scored using a multiparameter optimization (MPO) function, FragScore, which was defined as a weighted average of four normalized property scores: LogDScore {1 if AZlog
D7.4 0.5–1.5, 0 if AZlog
D7.4 ≤ 0.5 or AZlog
D7.4 > 3, linear interpolation, weight = 2}, HACScore {1 if HAC 11–14, 0 if HAC < 9 or HAC > 19, linear interpolation, weight = 2}, NRotScore {1 if NROT 0–2, 0 if NROT > 4, linear interpolation, weight = 1}, and HBDScore {1 if HBD 0–2, 0 if HBD > 4, linear interpolation, weight = 1}. The purpose was to be able to rank candidate fragments based on their properties, ranging from ideal (FragScore = 1) to undesirable (FragScore = 0). This MPO aligns with our views on which fragments typically make the most attractive and progressable hits.
Additionally, the respective Bemis–Murcko scaffold (BMS)24 of each of the 60
000 replacement fragments was compared to those of the 2010 legacy fragments, in order to identify fragments with a BMS not represented in the smaller set, and therefore complementary from a structural-diversity viewpoint. In this way, 34
000 fragments were found to contain an unrepresented BMS, covering approximately 16
000 unique BMSs. For each BMS, the fragment with the highest FragScore was identified and visually inspected. Any fragment with an unattractive chemical structure was removed. In this way, 3344 fragment candidates were identified, each with a unique, unrepresented BMS. A further 693 fragments with close to ideal properties (FragScore close to 1) were also identified.
Finally, the 3348 candidate replacement fragments were curated to remove 689 fragments that were deemed to either be very difficult to follow-up synthetically or contained undesirable functional groups. In the end, 5358 fragment candidates were taken forward to QC.
Sample preparation, purity check and solubility assessment
The completed library will be held at two sites (Cambridge, UK and Gothenburg, Sweden) in acoustic tubes holding 200 μL of 100 mM DMSO sample stock. Once prepared, solubility profiling of the final collection was performed by LCMS running each sample in duplicate. Each sample was diluted from its 100 mM starting point down to 1 mM in either water or acetonitrile. The acetonitrile dilution acted as the reference standard for each sample and data from the water dilution was compared for a measurement of the aqueous solubility. The LCMS system has two detectors, ultra-violet (UV) and charged aerosol detection (CAD). Most measurements had both sets of data to give increased confidence in the measurement. This dual detector system also allowed us to prioritize either of the measurements when one detector was saturated or close to its limits of detection. Of the samples tested, 76% (4048) were assessed to have greater than 700 μM solubility with >95% purity by LCMS and these were progressed to the next stages of QC. To expediate the QC process, the next steps occurred in parallel.
SPR clean screen
To evaluate which compounds were suitable for SPR fragment screening, an SPR clean screen was performed. Here, non-specific binding of the fragments to the SPR sensor chip matrix itself is assessed, as this would otherwise lead to interference with the SPR binding signal. The tested surfaces comprise chips modified with 6His–streptavidin, nickel–NTA and a NTA surface on a dextran matrix (NTA series S biosensor chip). The set of 4048 fragments was prepared at a single concentration of 1 mM of which 52 visibly precipitated fragments were removed before the start of the clean screen. Then 470 fragments were identified as outliers using the ‘early stability’ binding signal in at least one of the three surfaces. Overall, during this process 522 fragments (13%) were removed from the library leaving a set of 3526 fragments suitable for SPR fragment screening in terms of the absence of visible precipitation and reference binding.
NMR QC
NMR was used to assess the structure, purity, concentration and propensity for aggregation of the putative members of the fragment collection. Compounds were prepared as 1 mM solutions in a standard screening buffer (TRIS-d11, pH 7.4, see ESI†). The set of 4048 fragments, which passed LCMS purity and solubility criteria, was assessed by a 1D 1H spin echo experiment with excitation sculpting25,26 and a 1D WaterLOGSY experiment.27 Where appropriate, a 1D 19F experiment was also acquired. Data was analysed using ACD Spectrus processor and ACD Spectrus database.28 Compound structure was assessed using the ACD Match factor and subsequent manual assignment and validation, as well as analysis of the 19F data, where appropriate. Purity was assessed by comparing the integral of unassigned peaks to assigned peaks, with a cut-off of >95% purity. The concentration was calculated using a macro within the ACD software where the integral of assigned peaks is compared to the internal reference, DSS (10 μM, 90 μM proton equivalent). WaterLOGSY data was used to exclude compounds showing the presence of soluble aggregates (positive signals compared to negative buffer signals).27,29
After the first round of analysis, 2783 compounds were approved and 650 were rejected (Table 1). The remaining 615, for which the assessor was unable to reach a definitive verdict, were passed to a second assessor for subsequent analysis. At this point the SPR clean screen had read out and 86 compounds were excluded due to having failed this QC (to give 529 for the second-round analysis). From the second assessment, 118 further compounds were approved and 165 rejected. The remaining 246 compounds were typically problematic due to an overlap between buffer signals/residual protons in TRIS-d11, TCEP and the DMSO 13C satellites and aliphatic compound resonances, preventing clear assessment of the structure. Further NMR data was acquired for this subset in deuterated phosphate buffer with compounds dissolved in d6-DMSO. This substantially reduced the signal overlap in the aliphatic region and reduced the suppression of compound signals in the water region, allowing more spectra to be assigned. At this stage, 150 compounds were approved and included in the set.
Table 1 Statistics for the NMR assessment at different stages of QC
|
First-round analysis |
Second-round analysis |
Additional data |
Overall NMR assessment |
First-round QC Query compounds were passed to a second assessor for analysis, excluding 86 which were removed based on SPR results.
Second-round QC Query compounds were selected for more data.
Overall NMR assessment Query compounds represent those excluded due to SPR results where no final NMR conclusion was reached.
|
Not approved |
650 |
165 |
96 |
911 (22.6%) |
Query |
615a |
246b |
0 |
86c (2.1%) |
Approved |
2783 |
118 |
150 |
3051 (75.4%) |
Total |
4048 |
529 |
246 |
4048 |
In the first-round of NMR QC, the greatest source of attrition was due to low purity. Since the compounds had already passed the LCMS purity criterion, we attributed this to; impurities that lacked a chromophore; impurities that co-eluted with the compound; or potential instability in sample buffer. Low concentration provided another source of attrition at this stage with 7% of compounds showing an estimated concentration of <400 μM. Since the samples were prepared in aqueous buffer at a target concentration of 1 mM from 100 mM DMSO stocks, one reason for this could be inorganic impurities. A very small proportion of compounds (<0.5%) showed potential issues with compound aggregation consistent with the stringent selection criteria used in selecting the fragments. Less than 5% of the compounds were rejected due to concerns regarding the structure.
TCEP AMI-MS redox assay
To check that the fragments selected were not redox active, the compounds were tested for redox cycling behaviour using an acoustic-mist ionisation mass spectrometry assay,30 whereby TCEP oxidation is used as a measure of redox cycling.31,32 Compounds were incubated at 10 μM with 0.3 mM TCEP for 24 h prior to data acquisition by AMI-MS. The ion area of m/z peaks representing TCEP and oxidised TCEP (TCEPO), 249 m/z and 265 m/z respectively, were measured. Oxidation of TCEP was calculated using the equation TCEPO / (TCEP + TCEPO). Data was then normalised to positive controls minus neutral controls and presented as % oxidation. Compounds calculated to oxidise over 70% TCEP were flagged as redox cycling compounds and removed from the set. A low hit rate was observed, and 4007 compounds passed this criteria.
Overview of the biophysics fragment set
Since the SPR clean screen, NMR QC and TCEP assay were run in parallel it was then necessary to cross-reference the rejected fragments to collate a final list of compounds. This resulted in a final library size for the biophysics fragment set of 2741 fragments.
As previously discussed, a layered library was desirable to ensure flexibility with the screening approach for different projects. A Pareto optimisation of properties (as represented by FragScore) and the number of unique BMSs was used to select 1152 fragments to utilise as layer 1. It was envisioned this diverse sub-set could be used as the first pass set in any technology or as a ligandability set.9 To add maximum value for NMR screening, the presence of an aromatic CH, to provide a clear reporter signal in NMR assays, was included in the selection criteria.
Fig. 3(A) shows the distribution of the number of fragments per BMS.24 In total, 1798 unique BMSs are represented in the final set of 2741 fragments, indicating a high degree of structural diversity (1.5 fragments/BMS on average). The distribution is uneven, however, with 1568 unique BMSs only represented by a single fragment. The six most represented BMSs are phenyl (98), pyridine (46), pyrimidine (29), benzimidazole (27), indole (25), and quinoline (24). Overall, the set has a good coverage of both MW and clog
P and a suitable distribution of the HAC within the parameters described in FragScore (Fig. 3(B and C)). The trend of the layer 1 compounds to populate the lower MW/HAC space is also reflection of the FragScore MPO function.
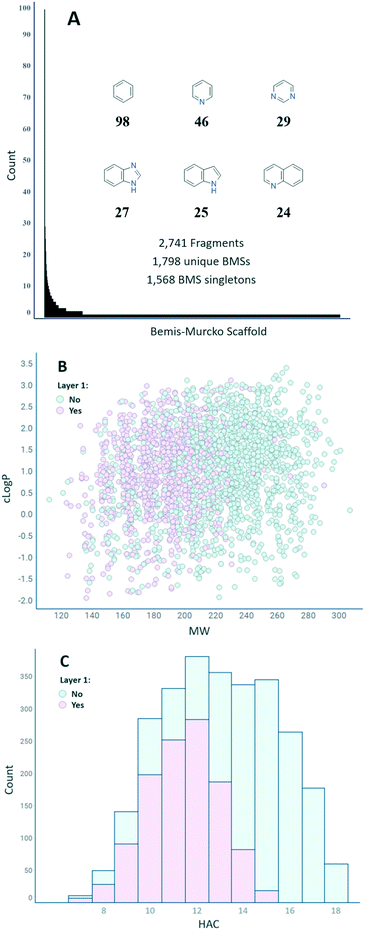 |
| Fig. 3 A: Distribution of unique Bemis–Murcko scaffolds in the biophysics fragment set with the six most well represented scaffold depicted with their count. B: A scatter plot to show distribution of clog P versus MW. C: Distribution of heavy-atom count. Members of layer 1 are indicated by purple symbols. | |
Enabling rapid screening of near neighbours
In the early stages of an FBDD campaign, and indeed any hit finding campaigns, testing close analogues of a hit is a good way to establish early SAR and build confidence in the original hits. To enable rapid follow-up screening around any hits from the biophysics fragment set, the 20 closest NNs for each query compound were identified from the AstraZeneca compound collection using FastROCs.33 A curation of the compounds found, based on FragScore criteria and compound availability, resulted in 16
806 NNs that will also be stored as 100 mM solutions to enable rapid hit expansion.
Case studies
To date, our new generation biophysics fragment set has been screened against four targets with a high degree of success (Table 2). For targets 1–3 the entire library was screened in the first instance, however, in the case of target 4 where protein supply was limited our pre-selected layer 1 made a suitable screening set showing the usefulness of the layered design. The selection of fragments with an aromatic reporter signal was also appropriate for screening by NMR. Hits have been found for all targets with initial hit rates ranging from 2.1–4.2%. Where determined, the potency range of the hits is in the 10 μM–1 mM range expected for fragments. Most importantly, hits have been progressed into medicinal chemistry optimisation from all the screens conducted. This was facilitated by the availability of NNs for the hits which gave us further confidence that the hits were real and early SAR before committing synthetic chemistry resource. We hope to report on the successful conclusion of these campaigns in due course.
Table 2 Screening data from fragment screens against four different targets using the new biophysics fragment set
Target |
Target class |
Screening technique |
Layers used |
Initial hit Ratea (%) |
Affinity range hits (μM) |
Hits progressed |
The initial hit rate is taken from the number of hits progressed for further profiling and different criteria for each target depending on multiple factors. This step involves some subjectivity but indicates that hits were found and importantly progressed.
Affinity values for target 4 hits have not yet been determined.
|
1 |
E3 ligase |
SPR |
All |
2.1 |
250–5000 |
Y |
2 |
Ubiquitin ligase protein |
SPR |
All |
3.7 |
220–980 |
Y |
3 |
Nucleotide exchange factor |
SPR |
All |
3.1 |
49–980 |
Y |
4b |
PPI |
NMR |
Layer 1 |
4.2 |
— |
Y |
Conclusions
Developing a fragment screening library is a fundamental component of enabling FBDD, however, how best to do this remains an open-ended question. We have described the approach taken at AstraZeneca in the hope that it will enrich debate in the literature and provide a signpost for others. Initially a computational selection of diverse fragments (based primarily on BMSs) was manually triaged by the medicinal chemistry team to ensure attractive and synthetically feasible starting point. Once identified, a robust QC process was employed to ensure the integrity of our fragment library. Fragments needed to be pure by both LCMS and NMR (>95%), have high solubility (>700 μM, LCMS) and pass technology (SPR, NMR, TCEP) analyses. In total only 2741 (51%) fragments passed this process and form the basis of our new fragment library – nevertheless, we believe the quality, robustness and synthetic tractability of our new fragment set as well as the selected diversity, is more important than the overall size of the set. Layer 1 was selected using BMS diversity and FragScore to ensure maximum flexibility for screening. Although only time will tell whether the decisions made during this process were judicious, early signs have been encouraging. Four fragment screens have been carried out to date and all of these have resulted in the delivery of hits suitable for further optimisation.
Conflicts of interest
There are no conflicts to declare.
Acknowledgements
The authors would like to thank Santosh Adhikari, J. Henry Blackwell, Claudia De Fusco, Paolo Di Fruscia, Iacovos N. Michaelides, Jen E. Nelson, and Ben Whitehurst for help with the NMR analysis and/or visual inspection of the fragment library.
Notes and references
- S. J. Albert, N. Blomberg, L. A. Breeze, J. H. A. Brown, N. J. Burrows, D. P. Edwards, H. A. R. Folmer, S. Geschwindner, J. E. Griffen, W. P. Kenny, T. Nowak, L.-L. Olsson, H. Sanganee and B. A. Shapiro, Curr. Top. Med. Chem., 2007, 7, 1600–1629 CrossRef PubMed.
- N. Fuller, L. Spadola, S. Cowen, J. Patel, H. Schönherr, Q. Cao, A. McKenzie, F. Edfeldt, A. Rabow and R. Goodnow, Drug Discovery Today, 2016, 21, 1272–1283 CrossRef PubMed.
- Z. Konteatis, Expert Opin. Drug Discovery, 2021, 16, 723–726 CrossRef CAS PubMed.
- G. M. Keserű, D. A. Erlanson, G. G. Ferenczy, M. M. Hann, C. W. Murray and S. D. Pickett, J. Med. Chem., 2016, 59, 8189–8206 CrossRef PubMed.
- Y. Shi and M. von Itzstein, Molecules, 2019, 24, 2838 CrossRef CAS PubMed.
- N. Blomberg, D. A. Cosgrove, P. W. Kenny and K. Kolmodin, J. Comput.-Aided Mol. Des., 2009, 23, 513–525 CrossRef CAS PubMed.
- J. Revillo Imbernon, C. Jacquemard, G. Bret, G. Marcou and E. Kellenberger, RSC Med. Chem., 2022, 13, 300–310 RSC.
- A. Chavanieu and M. Pugnière, Expert Opin. Drug Discovery, 2016, 11, 489–499 CrossRef CAS PubMed.
- F. N. B. Edfeldt, R. H. A. Folmer and A. L. Breeze, Drug Discovery Today, 2011, 16, 284–287 CrossRef CAS PubMed.
- A. Vulpetti and C. Dalvit, Drug Discovery Today, 2012, 17, 890–897 CrossRef CAS PubMed.
- P. Bamborough, M. J. Brown, J. A. Christopher, C.-W. Chung and G. W. Mellor, J. Med. Chem., 2011, 54, 5131–5143 CrossRef CAS PubMed.
- Manuscript in preparation.
- M. M. Vaidergorn, F. da Silva Emery and A. Ganesan, J. Med. Chem., 2021, 64, 13980–14010 CrossRef CAS PubMed.
- A. Agrawal, S. L. Johnson, J. A. Jacobsen, M. T. Miller, L.-H. Chen, M. Pellecchia and S. M. Cohen, ChemMedChem, 2010, 5, 195–199 CrossRef CAS PubMed.
- M. O'Reilly, A. Cleasby, T. G. Davies, R. J. Hall, R. F. Ludlow, C. W. Murray, D. Tisi and H. Jhoti, Drug Discovery Today, 2019, 24, 1081–1086 CrossRef PubMed.
- M. J. Hartshorn, C. W. Murray, A. Cleasby, M. Frederickson, I. J. Tickle and H. Jhoti, J. Med. Chem., 2005, 48, 403–413 CrossRef CAS PubMed.
- C. W. Murray and D. C. Rees, Angew. Chem., Int. Ed., 2016, 55, 488–492 CrossRef CAS PubMed.
- J. D. St. Denis, R. J. Hall, C. W. Murray, T. D. Heightman and D. C. Rees, RSC Med. Chem., 2021, 12, 321–329 RSC.
- P. C. Ray, M. Kiczun, M. Huggett, A. Lim, F. Prati, I. H. Gilbert and P. G. Wyatt, Drug Discovery Today, 2017, 22, 43–56 CrossRef CAS PubMed.
- O. B. Cox, T. Krojer, P. Collins, O. Monteiro, R. Talon, A. Bradley, O. Fedorov, J. Amin, B. D. Marsden, J. Spencer, F. von Delft and P. E. Brennan, Chem. Sci., 2016, 7, 2322–2330 RSC.
- W. F. Lau, J. M. Withka, D. Hepworth, T. V. Magee, Y. J. Du, G. A. Bakken, M. D. Miller, Z. S. Hendsch, V. Thanabal, S. A. Kolodziej, L. Xing, Q. Hu, L. S. Narasimhan, R. Love, M. E. Charlton, S. Hughes, W. P. van Hoorn and J. E. Mills, J. Comput.-Aided Mol. Des., 2011, 25, 621 CrossRef CAS PubMed.
- I. J. Chen and R. E. Hubbard, J. Comput.-Aided Mol. Des., 2009, 23, 603–620 CrossRef CAS PubMed.
- R. F. Bruns and I. A. Watson, J. Med. Chem., 2012, 55, 9763–9772 CrossRef CAS PubMed.
- G. W. Bemis and M. A. Murcko, J. Med. Chem., 1996, 39, 2887–2893 CrossRef CAS PubMed.
- T. L. Hwang and A. J. Shaka, J. Magn. Reson., 1995, 112, 275–279 CrossRef CAS.
- K. Stott, J. Stonehouse, J. Keeler, T.-L. Hwang and A. J. Shaka, J. Am. Chem. Soc., 1995, 117, 4199–4200 CrossRef CAS.
- C. Dalvit, P. Pevarello, M. Tatò, M. Veronesi, A. Vulpetti and M. Sundström, J. Biomol. NMR, 2000, 18, 65–68 CrossRef CAS PubMed.
-
ACD Spectrus, Advanced Chemistry Development, Inc., Toronto, Canada, https://www.acdlabs.com/products/spectrus/ (accessed March 28, 2022) Search PubMed.
- C. Dalvit, G. Fogliatto, A. Stewart, M. Veronesi and B. Stockman, J. Biomol. NMR, 2001, 21, 349–359 CrossRef CAS PubMed.
- I. Sinclair, M. Bachman, D. Addison, M. Rohman, D. C. Murray, G. Davies, E. Mouchet, M. E. Tonge, R. G. Stearns, L. Ghislain, S. S. Datwani, L. Majlof, E. Hall, G. R. Jones, E. Hoyes, J. Olechno, R. N. Ellson, P. E. Barran, S. D. Pringle, M. R. Morris and J. Wingfield, Anal. Chem., 2019, 91, 3790–3794 CrossRef CAS PubMed.
- M. Tarnowski, A. Barozet, C. Johansson, P. O. Eriksson, O. Engkvist, J. Walsh and J. W. M. Nissink, Assay Drug Dev. Technol., 2018, 16, 171–191 CrossRef CAS PubMed.
- C. M. R. Moore, I. Sinclair, G. A. Holdgate and J. Walsh, SLAS Discovery, 2022 DOI:10.1016/j.slasd.2022.06.002.
-
FastROCS Toolkit, OpenEye Scientific Software, Santa Fe, NM, https://www.eyesopen.com (accessed March 31, 2022) Search PubMed.
|
This journal is © The Royal Society of Chemistry 2022 |