DOI:
10.1039/D2MD00027J
(Review Article)
RSC Med. Chem., 2022,
13, 676-687
A story of peptides, lipophilicity and chromatography – back and forth in time
Received
26th January 2022
, Accepted 18th March 2022
First published on 22nd March 2022
Abstract
Peptides, as part of the beyond the rule of 5 (bRo5) chemical space, represent a unique class of pharmaceutical compounds. Because of their exceptional position in the chemical space between traditional small molecules (molecular weight (MW) < 500 Da) and large therapeutic proteins (MW > 5000 Da), peptides became promising candidates for targeting challenging binding sites, including even targets traditionally considered as undruggable – e.g. intracellular protein–protein interactions. However, basic knowledge about physicochemical properties that are important for a drug to be membrane permeable is missing but would enhance the drug discovery process of bRo5 molecules. Consequently, there is a demand for quick and simple lipophilicity determination methods for peptides. In comparison to the traditional lipophilicity determination methods via shake flask and in silico prediction, chromatography-based methods could have multiple benefits such as the requirement of low analyte amount, insensitivity to impurities and high throughput. Herein we elucidate the role of peptide lipophilicity and different lipophilicity values. Further, we summarize peptide analysis via common chromatographic techniques, in specific reversed phase liquid chromatography, hydrophilic interaction liquid chromatography and supercritical fluid chromatography and their role in drug discovery and development process.
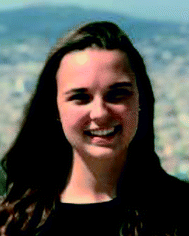 Vanessa Erckes | Vanessa Erckes studied pharmacy and obtained her MSc. at the Swiss Federal Institute of Technology in Zurich (ETHZ), Switzerland. In 2021, she started her doctoral thesis in the pharmaceutical analytics group at ETHZ. Her primary research interests are in peptide analytics and peptide chemistry. |
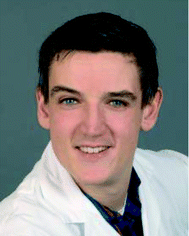 Christian Steuer | Dr. Christian Steuer finished his doctoral thesis at the University of Heidelberg Germany in 2011 and performed postdoctoral studies at the University of Zurich, Switzerland. He joined the institute of pharmaceutical Sciences at ETHZ and has been independent group leader of the pharmaceutical analytics group since 2016. His research interests include analytical method development, quality control of natural products and peptide chemistry. He has co-authored around 40 publications in international peer-reviewed journals. |
1. Introduction
1.1 Peptides in current drug development programs
Peptides are defined as polypeptides with 2–50 amino acids and have usually a molecular weight (MW) between traditional small molecules (MW < 500 Da) and large therapeutic proteins (MW > 5000 Da) such as antibodies.1,2 In general, the early drug discovery process of peptides was usually limited to the understanding of the physiological role of a peptide hormone with subsequent synthesis and therapeutic application. In 1923, the start was made with insulin as the first approved peptide administered by daily injection.1 Since then many more peptides followed up to 106 approved by the end of 2020.3 Although the drug discovery and development process has changed tremendously, there is still only a limited number of peptides orally bioavailable.3 Peptides are handled as promising candidates for targeting challenging binding sites, considered as undruggable, such as intracellular protein–protein interactions (PPI).4–7 PPI are known to play a key role in many pathological conditions. But, both large therapeutic proteins and small molecules have difficulties to target them. In general, large therapeutics are not able to cross membranes and small molecular drugs are often not big enough to interfere sufficiently with the large interaction sites of PPIs.4 As of June 2021, there are 58 peptides (whereof 7 are orally bioavailable) in clinical trials targeting PPI involved in various diseases as cancer, diabetes and bacterial/viral infections.7 Unfortunately, peptides often show inherent weaknesses, especially in their pharmacokinetics (PK) such as low membrane permeability or biological instability due to proteolytic degradation. This means they are usually poorly orally bioavailable and have a short plasma half-life resulting in the necessity of frequent parenteral application as seen for insulin treatment.1,6 Nevertheless, also small molecules and therapeutic proteins have properties, complicating the drug development process. An overview of the individual advantages and difficulties usually faced during the drug development in each drug class is presented in Table 1.
Table 1 Advantages and difficulties of peptides, small molecules and therapeutic proteins in drug development1–6
|
Small molecules |
Peptides |
Therapeutic proteins |
MW < 500 Da |
MW ∼ 500–5000 Da |
MW > 5000 Da |
Chemically unmodified peptides.
|
Advantages |
• Low cost in production and price on market |
• Automated synthesis |
• Very high target affinity, potency, specificity and selectivity due to large interaction site |
• Ease of synthesis |
• High target affinity, potency, specificity and selectivity |
• Long half life |
• Membrane permeability achievable, oral bioavailability and intracellular targets accessible |
• No toxic metabolites |
• Drug safety, no toxic metabolites |
• Known guidelines for drug design, Lipinski rule of 5 |
• Chemical synthesis enables easy structure modifications |
Difficulties |
• Often hepatic metabolism via CYP enzymes: prone to drug–drug interactions |
• Proteolytic instabilitya |
• Expensive in production and on market |
• Drug safety issues due to non-mechanistic-based toxicology (low affinity or selectivity) |
• Rapid clearance, short half-lifea |
• Complex (often recombinant) production, no easy chemical modification |
• Targets with need for large interaction sites precluded |
• Low membrane permeabilitya due to high polarity, no intracellular targets, no CNS targets, no oral absorption |
• Immunogenicity |
• No guidelines for rational drug design |
• No/low membrane permeability due to size, parenteral administration (s.c./i.v.), only extracellular or surface-exposed targets |
• Chemically and physically unstable, elaborated storage (sensitive to heat, pH, oxidation) |
A well-known guideline for oral bioavailability – and consequently membrane permeability – of small molecular drugs is the ‘rule of 5’ (Ro5), also known as ‘Lipinski rules’.8 The authors propose upper limits for log
P (≤5), molecular weight (≤500 Da), hydrogen bond donors (HBD, ≤5) and hydrogen bond acceptors (HBA, ≤10). Other established values for comparison and the determination of drug-likeliness are the topological polar surface area (TPSA, ≤140 Å) and the number of rotatable bonds (RotB, ≤10) as proposed by Veber et al. (2002).9–11 An example for an orally administered peptide small enough to follow the Ro5 is the antihypertensive enalapril which consists of two modified amino acids. Nevertheless, the low oral bioavailability and membrane permeability of the majority of peptides is not very surprising as they are usually very polar and exceed the threshold value for MW of 500 Da.3 Continuous improvements in automated peptide synthesis, ligation strategies and purification render molecular weights beyond 500 Da easily accessible.1,7,10,12–14
However, there are a few exceptions and particularly cyclic peptides are able to bypass the limitations indicated by the Ro5 – especially the upper MW limit of 500 Da,10,15 leading to the idea of a chemical space ‘beyond the rule of 5’ (bRo5), wherein oral bioavailability and membrane permeability is still feasable.12,16–18 A well-known example out of the bRo5 space is the naturally derived immunosuppressant cyclosporine A with an oral bioavailability of up to 80% despite a MW of 1203 Da.15,19 In recent years, medicinal chemists have made significant progress to overcome PK limitations of peptidic drugs by developing novel approaches to protect peptides from rapid degradation by peptidases and to enable membrane permeability. Optimization steps include the insertion of unnatural amino acids, side-chain or backbone modifications, terminus protection via amidation or acetylation and cyclization reactions including novel cyclization techniques such as peptide stapling and head to tail closing.6,20–28 The advantages of these optimized peptidomimetics include higher stability towards proteolysis, higher conformational stability, better transport properties across membranes and a higher target affinity depending on the chosen modification.15,26,27,29,30 This was observed in the optimization process of dengue and West Nile virus protease inhibitor of 763 Da with membrane permeability and metabolic stability containing 4-benzyloxyphenylglycine derivative and a bithiophene N-cap.31 Bird et al. clearly demonstrated that a doubly stapled 37 amino acid alpha-helical peptide with a MW of 4589, was not only resistant to degradation by pepsin and chymotrypsin but was also detected in plasma after oral administration. The linear analog – without modification or stapling – did not show any oral absorption.32 Further methods beyond the scope of medicinal chemistry that can improve the drug-likeness of a peptide are the development of peptide conjugates or the adaptation of their formulation and delivery. Regarding this, nasal and pulmonary delivery for peptide drugs is occasionally considered as an alternative to avoid parenteral application via injection.33–35 Although, successful drug administration and absorption is reported via these alternate routes, applicability as a pill still remains the desired goal due to favourable patient compliance. In 2019, the approval of the GLP-1 receptor agonist Rybelsus (semaglutide, MW 4114 Da) by the Food and Drug Administration led to a breakthrough in the treatment of type 2 diabetes since co-formulation with an absorption enhancer resulted in increased oral bioaivailability.20
Aforementioned examples impressively indicate that there are numerous bRo5 molecules and strategies to allow membrane crossing. Along these lines, peptides offer the possibility to combine the positive properties of the two big drug classes. In detail, peptides could unite oral dosing, ease of synthesis and addressing a broad range of biological targets as well as high target specificity, affinity, drug safety and interaction with large protein surfaces. Unfortunately, guidelines or rules that would enable easy rationale design of new orally available or at least membrane permeable compounds within the bRo5 space are non-existent and basic knowledge about key properties influencing the passive diffusion over a membrane is still missing.16 Although advances are made and support by computational models is given, especially computer assisted strategies reach their boundaries in prediction of key properties as solubility or permeability due to the complexity of the large and often flexible molecules.36–38 Therefore, the characterization of fundamental physicochemical parameters and investigation of underlying mechanisms of bRo5 molecules, especially peptides, to achieve membrane permeability are of great interest for the scientific community.11,20
1.2 Lipophilicity: definition and determination
Lipophilicity is the tendency of a compound to distribute into a nonpolar and lipid environment rather than to an aqueous matrix. It is often used as a measure for a compounds' possibility to cross cell membranes, which is a desired feature for peptides, especially for those targeting the bRo5 space.39 For the passive diffusion across a phospholipid-bilayer the compound needs to be lipophilic enough to interact with the nonpolar lipid chains but should not be too lipophilic, to be trapped in the membrane or to be insoluble in water.40 Lipophilicity is therefore an important property influencing the pharmacokinetic characteristics of a drug by determining all the underlying ADMET processes (absorption, distribution, metabolism, excretion, toxicity).39 A well-known value for lipophilicity is the partition coefficient log
P. It is determined from the partition of a compound between two immiscible phases, a polar aqueous phase and a nonpolar organic phase. It is common to add subscripts to log
P to specify the solvents used for its determination e.g. log
Pow, log
Poct and log
Poct/w indicate the use of octanol as organic solvent, log
Pcyc the use of cyclohexane.40–43 The log
P is defined as the logarithm of the ratio of concentrations of the uncharged molecule in the two phases at equilibrium:40,44 | 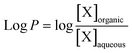 | (1) |
For the distribution between the two phases of an ionizable compound the distribution coefficient log
D is used instead. Peptides often show permanent charges over wide pH ranges due to free C- and N-terminus or acidic and basic side chains. The ionization state of the analyte strongly depends on the pH of the water phase, so it is important to use buffers as aqueous layer to maintain a defined pH. This is in contrast to the log
P value which is only defined for neutral compounds. The log
D is calculated similar to the log
P, but with the ratio of concentrations of all charged species present in the mixture at a certain pH value and not only the neutral compound. X represents the deprotonated and XH the protonated form:40,44 |  | (2) |
Measurements at pH 7.4 are commonly performed, since the behaviour of peptides under physiological conditions are of upmost interest. The pH at which the distribution coefficient was determined is specified by adding subscripts e.g. log
D7.4 means log
D determination at pH 7.4. The organic solvent used for log
P/D determinations is mainly octanol because similar to membrane lipids it contains a nonpolar hydrocarbon chain and an alcohol substructure as HBA and HBD.44 The inclusion of the octanol–water partition coefficient in the Ro5 contributed further to the wide use as a gold-standard. However, the relation between membrane permeability and the log
P/D determined with octanol is not always straightforward. For example, no obvious correlation was found with brain permeation for H2 receptor histamine antagonists or with membrane permeability in general for a series of neutral peptides and a group of cyclic hexapeptides, respectively.42,45,46 However, in all three cases the change to a protic organic solvent without any HBD groups for partition coefficient determination revealed an improved correlation. Solvents investigated included cyclohexane, toluene or 1,9-decadiene.42,45,46
It is also possible to express lipophilicity by the linear solvation energy relationship (LSER):41,47–50 the summands on the right side of eqn (3) account for specific molecular interactions between the solute and a two-phased solvent system resulting in the solute partitioning SP, which can be any measure related to solute partitioning, such as the log
P.
| SP = c + eE + sS + aA + bB + vV + j+J+ + j−J− | (3) |
The capital letters
E,
S,
A,
B and
V are related to the solute's properties while the small letters are the according solvent parameters, except for
c which is a system constant. The
eE term models the polarizability,
sS the dipole interactions,
aA the hydrogen bond accepting ability of the solvent,
bB the hydrogen bond donating ability of the solvent and
vV accounts for molecular size or the cavity formation.
47J+ accounts for electrostatic interactions induced by cations while
J− does the same for anions. For neutral compounds,
J+ and
J− are zero.
48–50 Solvent parameters are specific for a given solvent pair and express to what extent the interaction contributes to the overall solute partition. They reflect the differences between the two solvents whereas a large coefficient represents a large difference to particular interactions. The signs of the coefficients indicate which of the two phases interacts more strongly
via each of the specific interaction.
47 As demonstrated by the LSER, a partition coefficient is the result of many intermolecular forces occurring between the analyte and the two liquid phases and partition coefficients obtained from different solvent systems do not contain the identical structural information. Therefore, the combination of different partition coefficients can also be of value. An example is the Δlog
Poct−cyc that is the difference between log
Poct and log
Pcyc. It was shown that Δlog
P values can be even more accurate in predicting membrane permeability than simple log
P values. A more precise prediction was observed for the H2 receptor histamine antagonists and the series of aforementioned neutral peptides.
45,46 The Δlog
P parameters determined between log
Poct and the log
Pcyc or log
Pisooctane, respectively, had a better correlation with membrane permeation. Another, recently introduced Δlog
P parameter is Δlog
Poct−tol, which is the difference between the log
Poct and the log
Ptoluene. This parameter was shown to describe the propensity of a compound to form intramolecular hydrogen bonds (IMHB),
51 that attracts interest for drug discovery in the bRo5 space and hence for the development of peptides. IMHB can provide environment-dependent ‘chameleonic’ properties – as seen in cyclosporine A – by shielding polar groups when crossing the lipophilic membrane but displaying the hydrogen bond functionalities in aqueous environment.
19,52 A feature, which was also identified in conformationally flexible linear and cyclic peptide model systems to enhance membrane permeability.
11,17,19 The measurement of partition/distribution coefficients is traditionally conducted with the shake-flask method and follows a simple principle, where the analyte is added to a biphasic liquid–liquid system and allowed to partition between the layers until the equilibrium is reached. According to Organization for Economic Cooperation and Development (OECD) guideline for testing chemicals, log
Poct between −2 and 4 can be experimentally determined
via shake flask method without the need for a reference substance.
43 With this method, partition/distribution coefficients in various organic solvents can be easily detected. However, the shake flask method is labour intensive, time-consuming and requires a high amount of pure substance, making the application for peptides expensive. Moreover, only one compound per setup can be analyzed and a further analytical method is needed to determine the concentration of the test substance in both liquid phases.
43,53 To reduce cost and time effort in drug discovery programs, the use of
in silico prediction tools for log
P/
D of peptides would be a desirable add on technology. It is a fast way to determine the lipophilicity for huge compound libraries and could facilitate structure activity relationships. There are various academic and commercial software tools based on different calculation methods and training sets but the training sets are mostly based on small organic molecules.
17,53 There are only few studies using peptides in the training sets for log
P or
D prediction, but mostly short peptides with less than ten amino acids are used.
54 However, three-dimensional structure of the compound is often not taken into account, resulting in inaccurate prediction, especially for longer peptides exposing secondary structure elements. It was shown that peptide conformation can change with changing pH. These observations were made for various peptides with varying length and different secondary structures, including α-helices, β-strands and polyproline-II conformers.
55–57 Additionally, the log
P/
D prediction algorithms are usually not trained to calculate distribution coefficients in other organic phases than octanol as organic solvent.
54,58 This ignores the potential impact of the conformational state on solubility and cell permeability and also the flexibility and structure of the bRo5 molecules depending on their environment. The drawbacks of the shake flask method and
in silico prediction highlight the need for alternative, contemporary methods of lipophilicity determination of peptides. Ideally, this method should require minimal amounts of analyte while being insensitive to impurities, be flexible in the solvents used, include the three-dimensional structure of the compound and allow the automated high throughput of samples containing several analytes. A technique that could meet all these needs is chromatography with its basic principle of the continuous partitioning of solutes between two phases.
59 The fact that chromatographic systems can be characterized by LSER equations and their retention factor can be expressed similar to the log
P shows the connection between these two techniques.
47,59,60 The resulting retention time of an analyte is the sum of numerous interactions with the stationary and mobile phase and therefore includes valuable information about the properties of the analyte such as charge, polarity or hydrophobicity. The value of high performance liquid chromatography for log
Poct determination has already been acknowledged by the inclusion of a method for small molecules in the OECD guidelines.
61 According to these guidelines, log
Poct can be obtained from the linear
eqn (4):
| 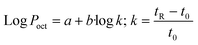 | (4) |
where
a and
b are linear regression coefficients and
k is the retention factor, that can be calculated from the retention time
tR and the holdup time
t0. Because various chromatographic techniques are commonly used in the drug development process of peptides, it would be very convenient to use them for the determination of partition/distribution coefficients as well. Chromatographic systems are very versatile and even though it can be seen as a weakness in the first place because method development can be challenging, it offers the possibility to find solutions precisely suited to a specific research question. The strengths and weaknesses of the methods described above for partition/distribution coefficient determination are summarized in
Table 2. Since chromatography-based methods for the lipophilicity determination of peptides would have great benefits, common chromatographic separation methods for peptides will be described in the following sections. Reports that relate retention behaviour of peptides to their hydrophobicity, membrane permeability or other lipophilicity related properties interesting for peptide characterization are presented accordingly.
Table 2 Strengths and weaknesses of different methods for the determination of partition/distribution coefficients for peptides
|
Shake flask |
In silico prediction |
Chromatography |
Depending on the chromatographic environment.
|
Strengths |
- No reference compounds needed |
- Fast |
- Low amount of analyte needed |
- Includes 3D structure of analyte |
- Cheap |
- Various solvents usable |
- Various solvents usable (for determination of various distribution coefficients) |
- No compound synthesis needed |
- Includes 3D structure of analytea |
- Automated and high throughput |
- Multiple analytes per run |
- Insensitive to impurities |
- Many different techniques and methods |
Weaknesses |
- Labor intensive |
- Often only for octanol as organic solvent |
- Not established or commonly used for analysis of peptide distribution coefficients yet |
- Low throughput |
- 3D structure of analyte not included |
- Requires high amount of pure substance |
- Training sets based on small molecules or peptides with low numbers of amino acids |
- Only one analyte per setup |
- Need of additional analysis method for concentration determination in both phases |
2. Peptide analysis and lipophilicity determination with chromatography
Chromatography in combination with various detection systems is an important tool in small molecule and peptide drug development. It is used for purification, identification and impurity profiling. In the following, we will focus on the three main chromatographic techniques: reversed phase liquid chromatography (RPLC), hydrophilic interaction liquid chromatography (HILIC) and supercritical fluid chromatography (SFC).
2.1 Reversed phase liquid chromatography (RPLC)
RPLC is certainly the most important chromatographic technique for peptide separation due to the excellent resolving power, instrumental convenience, analytical stability and versatility.60,62 Analytes are separated in order of increasing molecular hydrophobicity. Retention is initially generated by hydrophobic interaction of the hydrophobic analyte with the mobile phase and subsequently by dispersive interactions with the nonpolar stationary phase.60 Separation conditions include stationary phases based on silica, mostly bonded with C4-, C8- and C18-alkyl chains and a mobile phase consisting of water, a water-miscible organic solvent such as acetonitrile (ACN) or methanol (MeOH) and an ion pairing reagent.60,62–65 As ion pairing reagent most commonly trifluoroacetic acid (TFA) 0.05–0.1% (v/v) is added to the mobile phase, but also formic acid (FA) or phosphoric acid (H3PO4) are possible.66 TFA decreases peptide hydrophilicity and increases the affinity of the peptide for the hydrophobic stationary phase and improves separation.66 Moreover, TFA neutralizes positively charged residues of peptides and maintains a low pH of 2, thereby preventing undesirable ionic interactions between protonated side chains and free silanol groups of the stationary phase.62,64 Sufficient separation of peptides under isocratic elution is rarely achieved and the retention is very sensitive to small changes in solvent strength. It is therefore common to apply linear gradients of increasing organic solvent concentration from 0–100% in 30 to 120 min.62,63 However, it should be noted that an organic solvent as well as the nonpolar stationary environment of an RPLC can influence conformation.63,64 Depending on the peptide sequence, ACN, C18 alkyl chains and ion paring reagents are able to stabilize secondary structures in peptides or induce an alpha helical and beta-hairpin conformation, respectively.67–71
The use of RPLC for log
P determination of small molecules is described in scientific literature and also promoted by international organizations.61,72–74 For accurate log
Poct determination, the international guidelines recommend the use of packed C8 or C18 reversed phase columns as stationary phase. Elution should be performed under isocratic conditions using MeOH or ACN with a minimum concentration of 25% water. The use of further additives in the mobile phase should be omitted. If the use is unavoidable, the effect of the additive on the interaction between stationary phase and analyte and the resulting influence on the retention time should be evaluated carefully. RPLC determination is suitable for log
Poct values between 0 and 6 but should not be used for strong acids, bases or surface-active reagents. Correlation of retention times and log
P values is done by linear regression (eqn (4)). Training sets should be based on references which are structurally related to the test substances. A list of recommended reference substances is provided by the OECD guideline.61 However, chromatographic conditions for peptide analysis contradict the recommendations mentioned before. Usually, peptides are eluted from RP-columns in gradient mode and with the use of mobile phase additives like TFA. Additionally, peptides often show acidic or alkaline properties. The absence of peptides in the recommended training sets further complicates straight-forward log
P determination by linear regression. RPLC has been used multiple times to investigate intrinsic amino acid side-chains hydrophilicity/hydrophobicity in peptides. The accurate determination of these values could provide fundamental understanding in peptide–protein or protein–protein interactions. Different hydrophilicity/hydrophobicity scales obtained with RPLC were compared in a review by Mant et al. (2009).75 On the basis of their comparisons they were able to identify aspects which are important regarding RPLC separation conditions to obtain reliable hydrophilicity/hydrophobicity scales correlated to retention time. Those aspects could be helpful for eventual partition/distribution coefficient determination of peptides with RPLC as well. In general, poor correlations were obtained when the nature of peptide mixture was random in terms of varying peptide size and types of structure, especially for small sample pools (<100 peptides). In randomly selected peptides it was additionally shown, that the nature of the stationary phase (e.g. C8 vs. C18) can lead to wide variations in the coefficients, probably due to differential induction of secondary structure between different columns. Furthermore, higher content of organic modifier but also elevated temperature can lead to conformational changes.76,77 This effect can be evaluated by the use of reference peptides adopting the same conformation under selected RP environment.78 But even in set of well-defined model peptides, the secondary structure should be monitored carefully. The replacement of a single amino acid in the sequence can alter the whole conformation. It was demonstrated that the introduction of a D-amino acid instead of an L-amino acid simultaneously leads to a disruption of the alpha-helical structure and a shift towards lower retention times, respectively.79 Another factor that should be considered is the presence of ionizable side chains in the amino acid sequence in connection with pH of the mobile phase and ion pairing reagents. A low pH of 2 assures the protonation of the acidic side chains aspartate and glutamate (pKa ∼ 4) as well as the protonation of histidine (pKa ∼ 6), whereas analysis at physiological pH 7 leads to their deprotonation and a change in hydrophobicity. Peptides containing the basic residues lysine and arginine are positively charged at pH 2 and pH 7 and therefore they are sensitive for the addition of ion pairing reagents. Despite the helpful properties of the ion pairing reagent mentioned above, the interaction with positive charged amino acid residues increases the retention time and can change the relative elution order of peptides. The strength of this effect depends on ion pairing reagent concentration and hydrophobicity.66 TFA was shown to mask the polarity of peptides in such a way that the retention time of a +5 charged peptide could exceeded the ones of lower charged peptides (+1, +3). Nevertheless, the effect of the ion pairing reagent was also shown to be identical per net positive charge, meaning it is constant within a group of equally charged sequences.66 Depending on the nature of ion pairing reagents (H3PO4 instead of TFA at pH 2 or NaCl instead of NaClO4 at pH 7), net effect on analyte's hydrophobicity is less prominent. The elution order of uncharged random coil peptides on the other hand was independent of mobile phase pH and ion pairing reagent.75 Nevertheless, it should be mentioned that the presence of different types of counterions such as chloride, FA or TFA can have an impact on secondary structure elements.71,80 Finally, in the case of alpha helical amphipathic peptides it was shown that their preferred binding domain to the stationary phase is their nonpolar site. RPLC is consequently more sensitive to hydrophobicity changes on the nonpolar site of peptides compared to changes on the polar site.79 An approach to use RPLC as a rapid method to characterize macrocyclic peptides and facilitate their drug discovery process was proposed by Wang et al. (2015).81 62 neutral cyclic hexapeptides were analyzed on a C18 solid phase with an ACN/water mixture containing 0.05% TFA. A positive but weak correlation between the retention factor log
k of and cell permeability was obtained, indicating that log
k or retention time could be used as a first filter to guide the design of membrane-permeable peptides. Similar results were obtained by an analysis of six hydrocarbon stapled peptides and derivatives with a C4 column and a linear gradient from 5 to 95% ACN in water, both containing 0.1% TFA. It was shown that a higher retention time correlated with higher cellular uptake. Interestingly the results also indicated that hydrophobicity had a higher impact on membrane permeability compared to a constrained structure. The peptides, modified with R/S-pentenylalanine but not cyclized via ring closing metathesis, showed a higher retention time in RPLC and also a higher permeability as the fully stapled peptide.82 Recently the influence of C- and N-methylation in cyclic peptide isomers with identical Ro5 parameters (MW, calculated log
P, HBA, HBD and also RotB and TPSA) was investigated.83 It was possible to show that peptides, differing only in the location of one methyl group had different membrane permeability in correlation to the retention time in RPLC analysis on a C18 column. A larger coherent hydrophobic area was discussed as a possible cause for beneficial membrane permeability. It would also explain higher retention times on a reversed phase stationary phase due to the increased hydrophobic interactions.83 This highlights again the importance of three-dimensional structure of target analytes.38,83
2.2 Hydrophilic interaction liquid chromatography (HILIC)
HILIC is a type of aqueous normal phase liquid chromatography (NPLC) and has in contrast to RPLC a polar stationary phase. Hence it is a valuable technique for the separation of polar and ionized compounds such as amino acids and peptides,84 which are difficult to separate in RPLC.85 In HILIC nonpolar compounds elute first and polar analytes are retained longer on the column. Hence, in general analytes with negative log
P/D values are preferably analyzed in this chromatographic setting. Characteristic for HILIC is the solvent composition of the mobile phase. Although the types of organic solvents are the same as in RPLC, the HILIC mobile phase consists of high levels of organic solvents (typically ACN > 70% v/v) in combination with water or a buffer (at least 2.5% v/v) to maintain the mobile phase pH.60,63 Water has the highest elution force in HILIC. As polar phases, many different chemistries are available including bare silica or silica modified with amino, amide, cyano, diol, ion exchange or zwitterionic functional groups.85,86 The specific mobile phase conditions are required in order to preserve the distinctive retention mechanism of HILIC, involving the formation of a water rich layer at the solid phase surface.60,86 Thus retention of a solute is the result of its adsorption to the stationary phase via hydrogen bonding, dipole/dipole interactions or electrostatic forces combined with the partitioning between the organic layer and the water enriched layer immobilized on the surface of the polar stationary support.60 Depending on the type of surface modifications, the contribution of intermolecular forces leading to retention vary strongly as can be seen in the retention models based on LSER, published by Schuster and Lindner (2013).87,88 The type of attracted charge, either positive or negative, changes when using different column chemistries. It should be noted that the ionization state of the stationary phase itself can change upon varying mobile phase pH, leading to an altered retention. Peptide separation with HILIC is usually initiated with an organic solvent content of 80–95%.89 Subsequent elution of the peptides is accomplished by an increase of the mobile phase polarity, either by increasing the amount of water or by running an increasing salt gradient.63 When comparing the separation of five peptides, only slightly differing in their hydrophobicity, on a C18 column (RPLC, gradient elution with increasing ACN content, 0.05% TFA) with their separation on a polyhydroxyethylaspartamide column (HILIC, gradient elution with decreasing ACN content, 0.2% aq. phosphoric acid) a reverse of elution order can be observed. In RPLC the peptides were eluted with increasing hydrophobicity, while in HILIC the hydrophobicity was, as expected, decreasing.90 Similar to RPLC separation, the pH of the mobile phase, as well as the organic solvent content, can influence peptide charge and structure and therefore change the retention behavior. The analysis of five peptides, differing in their charge and amino acid composition, on a ZIC-HILIC column (zwitterionic sulfoalkybetaine) at different pH values (pH 3, 4.5, 6.8, 8), revealed a change in relative elution order. Consequently, a constant pH during peptide separation should be maintained, which is achieved by the use of buffers e.g. with phosphoric acid, ammonium formate or ammonium acetate.89 It is well known that type and concentration of salt can alter peptide structure.80 NaClO4 can be used for peptide elution purposes91 and simultaneously stabilizing an alpha helical conformation.92 The time required for peptide separation with HILIC is comparable to RPLC and suitable for high throughput analysis.63 Depending on the scope of the experiments, shorter or longer chromatographic run times can be applied.
A few studies investigating the relation between HILIC retention time and the partition/distribution coefficient for small molecules have been published. Kadar et al. (2008) evaluated the relation between HILIC retention and log
D3.0 utilizing ACD Labs log
D suite prediction.93 The retention factors of 30 pharmaceutically relevant compounds were measured on an Atlantis HILIC column (bare silica) under three different isocratic ACN conditions (85%, 90%, 95% v/v) with 10 mM ammonium formate aqueous buffer. The highest correlation factor between log
k and log
D3.0 obtained was 0.751 with 85% ACN in the mobile phase. Especially for positively charged basic compounds, the correlation was weak. Experiments to determine the log
Poct of basic compounds with HILIC were conducted by Bard et al. (2009).94 They examined log
k values of 39 basic drugs (log
P range from −1.31 to 4.62) and compared it to log
Poct values from literature. Retention coefficients were measured on a ZIC-pHILIC column with 95% (log
k95) and with 0% (log
k0) ACN and a 100 mM TFA/ammonia buffer at pH 2. The resulting correlation between the partition coefficient of charged as well as neutral molecules and log
k (log
k95 as well as log
k0) was low. The best correlation was obtained for the calculated Δlog
k0–95 and the log
Poct with a correlation coefficient of 0.93. Finally, Bard et al. (2012) showed that the correlation between the alkane/water partition coefficient and HILIC retention of 44 polar neutral compounds is better compared to the correlation with log
Poct.95 A ZIC-pHILIC as stationary phase and 90% ACN with 20 mM TFA/sodium hydroxide (pH 2) or ammonium acetate/formic acid (pH 7) were chosen as experimental conditions. The measured correlation coefficient for the relation between log
k and log
Palk was 0.87 (compared to 0.55 for the relation with log
Poct). In these experiments, it was shown that because of the general polar character of HILIC stationary phases it is possible to determine log
D values since charge is taken into account during separation. In 1991 the laboratory of Mant and Hodges introduced the novel peptide separation method mixed-mode hydrophilic interaction/cation exchange chromatography (HILIC/CEX).90 A summary of their experiments with HILIC/CEX including detailed discussion of the results can be found in a review from the same authors in 2008.96 HILIC/CEX is basically cation exchange chromatography (CEX) in the presence of high ACN concentrations. A possible column is the strong CEX stationary phase Poly-Sulfoethyl A, where peptides are eluted with increasing salt concentrations e.g. with NaClO4. In general, HILIC/CEX separates peptides in groups of increasing charge and within these groups in order of increasing hydrophilicity. For peptides with the same positive charge and only small deviations in hydrophilicity/hydrophobicity it was shown that applying high concentrations of ACN on a CEX column (resulting in HILIC/CEX) can improve the separation because hydrophilic interactions are promoted. With low ACN concentration peptides are simply separated in CEX-mode by charge, leading to a low resolution for equally charged peptides.97 HILIC/CEX analysis of amphipathic alpha helical peptides on a Poly-Sulfoethyl A column revealed that HILIC/CEX is more sensitive to resolve peptide elution, where single amino acid substitutions are made on the polar face of the molecule.79 On the other hand, RPLC was better suited for the separation for peptides with substitution on the non-polar phase. This indicates that amphipathic molecules preferably interact with the face complementary to the stationary phase and the retention time represents the change in hydrophilicity at the interaction site instead of representing the overall hydrophilicity/hydrophobicity of the molecule. Similar results were obtained for cyclic peptides, where different peptides with modifications on the polar face co-eluted by RPLC but were well separated by HILIC/CEX.98 This indicates that the combination of RPLC and HILIC analysis can provide especially for amphipathic molecules valuable information. The fact that HILIC and RPLC separations provide complementary information and are not just inverted techniques is also shown by the application of the combination of HILIC and RPLC in coupled column systems (2D-HPLC) in proteomics. HILIC × RPLC shows a high degree of orthogonality, thereby reducing the complexity of proteomics samples prior to mass spectrometry analysis.99
2.3 Supercritical fluid chromatography (SFC)
The characteristic feature of SFC is the use of a mobile phase in supercritical fluid state. In most cases, CO2 is used at a critical pressure of 7.3 MPa and a critical temperature of 31 °C, respectively.100 Fluids in supercritical state have on one hand the characteristics of a gaseous phase resulting in a low pressure in the system and allowing high flow rates up to 5 mL min−1 but on the other hand show a density and solvating power comparable to a liquid mobile phase.101 The use of CO2 as mobile phase is favourable because it is further considered as a green solvent and keeps the consumption of organic solvent even at high flow rates very low100,101 The feasibility of SFC for analysis of peptides with up to 40 amino acids with acidic and basic side chains was shown by Zheng et al. (2006).102 An important advantage of SFC for peptide separation compared to RPLC and HILIC is the very short analysis time. This was shown by a direct comparison of Tognarelli et al. (2010) where the separation of an assorted 5 peptide mixture was achieved in less than 12 minutes, while the separation using RPLC took 50 minutes.103 However, elution order was changed in SFC, probably due to the use of a different stationary phase. The main limitation for the analysis of compounds is their solubility in the CO2 mobile phase. CO2 has a very low polarity, comparable to alkanes.100,104,105 Hence the analysis of peptides, which are relatively polar, requires the addition of polar organic solvent modifiers in the mobile phase, such as MeOH, ethanol or ACN. The modifier improves polar compound solubility, enhances solvent strength and avoids sample precipitation. Gradient elution with increasing modifier proportion from 5–50% to accelerate elution is also common.100,105 Moreover, acid, base or salt additives, typically 0.05–0.1%, can be added to change the pH of the mobile phase, change the ionization state of compounds and stationary phase or form ion pairs to improve the solubility of the compound further.100 Possible additives are TFA, methanesulfonic acid, ammonia acetate, ammonium hydroxide or iso-propylamine.106,107 Due to its limited miscibility with CO2, water is not preferred as a modifier but was shown to improve the separation of uncapped charged 12mer peptides nevertheless.108 TFA or the combination of TFA and ammonia as additives, each 0.1%, were shown to improve peak shapes of small macrocyclic peptides as well as of basic and acidic peptides up to 40 amino acids on various columns.109 But, as already mentioned for RPLC, the effect of additives on retention is strongly dependent on the functional groups of the analytes.110 Additionally, it has to be kept in mind that the conformation of the analyzed peptides probably changes upon alteration of its ionization state or solvent pH, what influences retention behavior.109 Even though multiple parameters can be modified in the mobile phase Patel et al. (2011) concluded, after analysis of two isomeric peptide pairs with SFC, that choosing the right type of stationary phase is more critical.111 Stationary phases utilized in SFC include a wide range of different types. There are SFC specific columns e.g. based on 2-ethylpyridine (2-EP) or naphthyl bonded silica, which can be summarized as ‘aromatic’ columns. But also NPLC columns e.g. bare silica as well as HILIC columns e.g. diol and nonpolar C18 columns usually seen in RPLC separations can be used.105 The attempt to separate the peptide pairs with a 4-ethylpyridine column was not successful, while separation with a HA-pyridine column showed good results independent of the used additive.100,105 West and Lesellier presented the LSER for nonpolar,112 polar113,114 and aromatic column types.115 A comparison of the LSER of a C18 and a bare silica column in SFC mode with RP and NP revealed that similar retention patterns were achieved.100 The aromatic columns, including the 2-EP bounded silica column, which is commonly used for peptide separation,103,109 showed a retention pattern that is neither RP nor NP type but unique to SFC. Applications that would use SFC for log
P/D determination were not found in literature. However, Goetz et al. (2014)116 developed an SFC method for the high throughput identification of IMHB in pharmaceutically relevant small molecules and introduced the EPSA value. Historically, Fourier transform infrared spectroscopy (FTIR) and nuclear magnetic resonance (NMR) were used for detection of IMHB, but they do not enable measurement in a physiologically relevant environment. Hence the new method, introduced by Shalaeva et al. (2013)51 based on measuring Δlog
Poct−tol is an improvement, but the weaknesses of the shake flask method were already elucidated above. The development of an SFC method allowed the analysis of IMHBs in 12 minutes at 40 °C and a flow rate of 5 mL min−1. A Pirkle Chirex 3014 column (silica bonded (S)-valine and (R)-1-(α-naphthyl)ethylamine with urea linkage) was used as stationary phase and the mobile phase consisted of 5–50% MeOH gradient in CO2. Due to the water-free environment IMHB within the analyte are not disrupted and molecules offering a hidden polarity by means of forming IMHB elute earlier from the column. The lower retention time then corresponds to a lower EPSA value. This method was successfully applied to cyclic peptides where in general an EPSA value below 80 was a good indicator for membrane permeability.117 This indicates that SFC is already a valuable tool in the drug discovery process of membrane permeable bRo5 molecules including peptides.
Conclusions
Peptide drug discovery has gained a lot of attention in recent years since peptides provide unique properties in the bRo5 space. New fields of drug targets, such as intracellular PPI, are explored and there is a growing need for easy methods to characterize basic chemical properties such as lipophilicity as a metric for membrane permeability. Even though research in the bRo5 space seems to slowly drift away from traditional log
Poct determination, the characterization by distribution coefficients or combinations of them is nevertheless an important and easy tool for the prediction of complex interactions involved in basic pharmacokinetic processes. It is especially valuable for sophisticated molecules such as peptides, where flexibility and 3D structure are crucial and the accuracy of computational methods is still limited. Particularly chromatography-based techniques could play a key role in upcoming years for lipophilicity assessment by fast and easy compound screening in the peptide drug development process. Due to the countless opportunities chromatographic techniques provide, many different aspects of lipophilicity can be characterized. RPLC with its nonpolar stationary phases can be used for log
P determination from 0 to 6 and was shown to display especially hydrophobic peptide regions carefully. HILIC on the other hand is used for the analysis of polar and charged compounds contrary to RPLC, but does still provide a certain orthogonality. With SFC there is the unique possibility to create a water free environment for analysis, which was shown to encourage IMHB and display chameleonic properties. The latter in particular was only recently described as a possible important property for peptide membrane permeability in the bRo5 chemical space. Throughout all chromatographic techniques and analyses it became clear that peptide properties are easily influenced by its surrounding. However, chromatography-based techniques were able to prove that they are sensitive enough to monitor even minor changes in peptide sequence, charge and structure while mimicking many different environments. Therefore, chromatographic techniques will certainly support the much-demanded easy characterization of physicochemical properties in rational peptide drug design in the future.
Author contributions
VE and CS contributed equally to the manuscript. Both authors reviewed the final version of the manuscript.
Conflicts of interest
There are no conflicts to declare.
Acknowledgements
We are grateful to Justine Räber for fruitful discussions and for reading our manuscript.
References
- A. Henninot, J. C. Collins and J. M. Nuss, J. Med. Chem., 2018, 61, 1382–1414 CrossRef CAS PubMed.
- J. L. Lau and M. K. Dunn, Bioorg. Med. Chem., 2018, 26, 2700–2707 CrossRef CAS PubMed.
- V. D'Aloisio, P. Dognini, G. A. Hutcheon and C. R. Coxon, Drug Discovery Today, 2021, 26, 1409–1419 CrossRef PubMed.
- D. E. Scott, A. R. Bayly, C. Abell and J. Skidmore, Nat. Rev. Drug Discovery, 2016, 15, 533–550 CrossRef CAS PubMed.
- G. J. B. Philippe, D. J. Craik and S. T. Henriques, Drug Discovery Today, 2021, 26, 1521–1531 CrossRef CAS PubMed.
- A. C. Lee, J. L. Harris, K. K. Khanna and J. H. Hong, Int. J. Mol. Sci., 2019, 20, 2383 CrossRef PubMed.
- W. Cabri, P. Cantelmi, D. Corbisiero, T. Fantoni, L. Ferrazzano, G. Martelli, A. Mattellone and A. Tolomelli, Front. Mol. Biosci., 2021, 8, 697586 CrossRef CAS PubMed.
- C. A. Lipinski, F. Lombardo, B. W. Dominy and P. J. Feeney, Adv. Drug Delivery Rev., 2001, 46, 3–26 CrossRef CAS PubMed.
- D. F. Veber, S. R. Johnson, H. Y. Cheng, B. R. Smith, K. W. Ward and K. D. Kopple, J. Med. Chem., 2002, 45, 2615–2623 CrossRef CAS PubMed.
- M. D. Shultz, J. Med. Chem., 2019, 62, 1701–1714 CrossRef CAS PubMed.
- G. Caron, J. Kihlberg, G. Goetz, E. Ratkova, V. Poongavanam and G. Ermondi, ACS Med. Chem. Lett., 2021, 12, 13–23 CrossRef CAS PubMed.
- M. Tyagi, F. Begnini, V. Poongavanam, B. C. Doak and J. Kihlberg, Chemistry, 2020, 26, 49–88 CrossRef CAS PubMed.
- R. Behrendt, P. White and J. Offer, J. Pept. Sci., 2016, 22, 4–27 CrossRef CAS PubMed.
- S. S. Kulkarni, J. Sayers, B. Premdjee and R. J. Payne, Nat. Rev. Chem., 2018, 2, 0122 CrossRef CAS.
- D. S. Nielsen, N. E. Shepherd, W. Xu, A. J. Lucke, M. J. Stoermer and D. P. Fairlie, Chem. Rev., 2017, 117, 8094–8128 CrossRef CAS PubMed.
- P. Matsson and J. Kihlberg, J. Med. Chem., 2017, 60, 1662–1664 CrossRef CAS PubMed.
- P. Matsson, B. C. Doak, B. Over and J. Kihlberg, Adv. Drug Delivery Rev., 2016, 101, 42–61 CrossRef CAS PubMed.
- S. D. Kramer, H. E. Aschmann, M. Hatibovic, K. F. Hermann, C. S. Neuhaus, C. Brunner and S. Belli, Adv. Drug Delivery Rev., 2016, 101, 62–74 CrossRef CAS PubMed.
- D. Lee, S. Lee, J. Choi, Y. K. Song, M. J. Kim, D. S. Shin, M. A. Bae, Y. C. Kim, C. J. Park, K. R. Lee, J. H. Choi and J. Seo, J. Med. Chem., 2021, 64, 8272–8286 CrossRef CAS PubMed.
- M. J. Blanco and K. M. Gardinier, ACS Med. Chem. Lett., 2020, 11, 228–231 CrossRef CAS PubMed.
- C. R. Coxon and W. D. G. Brittain, Chem. – Eur. J., 2022, 28, e202103305 Search PubMed.
- F. Yang, F. Yin and Z. Li, Methods Mol. Biol., 2022, 2371, 391–409 CrossRef PubMed.
- K. Bozovicar and T. Bratkovic, Int. J. Mol. Sci., 2021, 22, 1611 CrossRef CAS PubMed.
- M. G. Ricardo, A. M. Ali, J. Plewka, E. Surmiak, B. Labuzek, C. G. Neochoritis, J. Atmaj, L. Skalniak, R. Zhang, T. A. Holak, M. Groves, D. G. Rivera and A. Domling, Angew. Chem., Int. Ed., 2020, 59, 5235–5241 CrossRef CAS PubMed.
- J. Chatterjee, C. Gilon, A. Hoffman and H. Kessler, Acc. Chem. Res., 2008, 41, 1331–1342 CrossRef CAS PubMed.
- E. Lenci and A. Trabocchi, Chem. Soc. Rev., 2020, 49, 3262–3277 RSC.
- C. Morrison, Nat. Rev. Drug Discovery, 2018, 17, 531–533 CrossRef CAS PubMed.
- S. U. Vetterli, K. Moehle and J. A. Robinson, Bioorg. Med. Chem., 2016, 24, 6332–6339 CrossRef CAS PubMed.
- M. Moiola, M. G. Memeo and P. Quadrelli, Molecules, 2019, 24, 3654 CrossRef CAS PubMed.
- Y. Li, Y. Zhang, M. Wu, Q. Chang, H. Hu and X. Zhao, ACS Chem. Biol., 2019, 14, 516–525 CrossRef CAS PubMed.
- M. A. Behnam, D. Graf, R. Bartenschlager, D. P. Zlotos and C. D. Klein, J. Med. Chem., 2015, 58, 9354–9370 CrossRef CAS PubMed.
- G. H. Bird, N. Madani, A. F. Perry, A. M. Princiotto, J. G. Supko, X. He, E. Gavathiotis, J. G. Sodroski and L. D. Walensky, Proc. Natl. Acad. Sci. U. S. A., 2010, 107, 14093–14098 CrossRef CAS PubMed.
- B. von Mentzer, A. F. Russo, Z. Zhang, A. Kuburas, P. M. Killoran, V. D’Aloisio, L. Nizic, V. Capel, D. A. Kendall, C. R. Coxon and G. A. Hutcheon, J. Pharm. Pharmacol., 2020, 72, 1352–1360 CrossRef CAS PubMed.
- J. Liu, T. Gong, H. Fu, C. Wang, X. Wang, Q. Chen, Q. Zhang, Q. He and Z. Zhang, Int. J. Pharm., 2008, 356, 333–344 CrossRef CAS PubMed.
- G. Scheuch, M. J. Kohlhaeufl, P. Brand and R. Siekmeier, Adv. Drug Delivery Rev., 2006, 58, 996–1008 CrossRef CAS PubMed.
- V. Poongavanam, Y. Atilaw, S. Ye, L. H. E. Wieske, M. Erdelyi, G. Ermondi, G. Caron and J. Kihlberg, J. Pharm. Sci., 2021, 110, 301–313 CrossRef CAS PubMed.
- F. Begnini, V. Poongavanam, Y. Atilaw, M. Erdelyi, S. Schiesser and J. Kihlberg, ACS Med. Chem. Lett., 2021, 12, 983–990 CrossRef CAS PubMed.
- B. Over, P. Matsson, C. Tyrchan, P. Artursson, B. C. Doak, M. A. Foley, C. Hilgendorf, S. E. Johnston, M. D. T. Lee, R. J. Lewis, P. McCarren, G. Muncipinto, U. Norinder, M. W. Perry, J. R. Duvall and J. Kihlberg, Nat. Chem. Biol., 2016, 12, 1065–1074 CrossRef CAS PubMed.
- S. D. Kramer, D. Lombardi, A. Primorac, A. V. Thomae and H. Wunderli-Allenspach, Chem. Biodiversity, 2009, 6, 1900–1916 CrossRef PubMed.
- X. Liu, B. Testa and A. Fahr, Pharm. Res., 2011, 28, 962–977 CrossRef CAS PubMed.
- M. H. Abraham, H. S. Chadha, G. S. Whiting and R. C. Mitchell, J. Pharm. Sci., 1994, 83, 1085–1100 CrossRef CAS PubMed.
- M. R. Naylor, A. M. Ly, M. J. Handford, D. P. Ramos, C. R. Pye, A. Furukawa, V. G. Klein, R. P. Noland, Q. Edmondson, A. C. Turmon, W. M. Hewitt, J. Schwochert, C. E. Townsend, C. N. Kelly, M. J. Blanco and R. S. Lokey, J. Med. Chem., 2018, 61, 11169–11182 CrossRef CAS PubMed.
-
OECD, Test No. 107: Partition Coefficient (n-octanol/water): Shake Flask Method, 1995.
- A. Oliva, C. Monzon, A. Santovena, J. B. Farina and M. Llabres, J. Sep. Sci., 2016, 39, 2689–2701 CrossRef CAS PubMed.
- P. S. Burton, R. A. Conradi, N. F. Ho, A. R. Hilgers and R. T. Borchardt, J. Pharm. Sci., 1996, 85, 1336–1340 CrossRef CAS PubMed.
- R. C. Young, R. C. Mitchell, T. H. Brown, C. R. Ganellin, R. Griffiths, M. Jones, K. K. Rana, D. Saunders, I. R. Smith, N. E. Sore and
et al.
, J. Med. Chem., 1988, 31, 656–671 CrossRef CAS PubMed.
- M. Vitha and P. W. Carr, J. Chromatogr. A, 2006, 1126, 143–194 CrossRef CAS PubMed.
- M. H. Abraham and Y. H. Zhao, J. Org. Chem., 2004, 69, 4677–4685 CrossRef CAS PubMed.
- M. H. Abraham and W. E. Acree, Jr., J. Chromatogr. A, 2016, 1430, 2–14 CrossRef CAS PubMed.
- M. H. Abraham and W. E. Acree, Jr., ACS Omega, 2019, 4, 2883–2892 CrossRef CAS PubMed.
- M. Shalaeva, G. Caron, Y. A. Abramov, T. N. O'Connell, M. S. Plummer, G. Yalamanchi, K. A. Farley, G. H. Goetz, L. Philippe and M. J. Shapiro, J. Med. Chem., 2013, 56, 4870–4879 CrossRef CAS PubMed.
- M. Rossi Sebastiano, B. C. Doak, M. Backlund, V. Poongavanam, B. Over, G. Ermondi, G. Caron, P. Matsson and J. Kihlberg, J. Med. Chem., 2018, 61, 4189–4202 CrossRef CAS PubMed.
-
L. Di and E. H. Kerns, in Drug-Like Properties. Concepts, Structure Design and Methods from ADME to Toxicity Optimization, Elsevier, London, 2nd edn, 2016, ch. 23, pp. 299–306 Search PubMed.
- M. D. Allenspach, J. A. Fuchs, N. Doriot, J. A. Hiss, G. Schneider and C. Steuer, J. Pept. Sci., 2018, 24, e3113 CrossRef PubMed.
- E. Schievano, T. Calisti, I. Menegazzo, R. Battistutta, E. Peggion, S. Mammi, G. Palu and A. Loregian, Biochemistry, 2004, 43, 9343–9351 CrossRef CAS PubMed.
- F. Fan and K. H. Mayo, J. Biol. Chem., 1995, 270, 24693–24701 CrossRef CAS PubMed.
- K. I. Oh, K. K. Lee, E. K. Park, D. G. Yoo, G. S. Hwang and M. Cho, Chirality, 2010, 22(Suppl 1), E186–E201 CrossRef CAS PubMed.
- A. Visconti, G. Ermondi, G. Caron and R. Esposito, J. Comput.-Aided Mol. Des., 2015, 29, 361–370 CrossRef CAS PubMed.
- A. Berthod and S. Carda-Broch, J. Chromatogr. A, 2004, 1037, 3–14 CrossRef CAS PubMed.
-
S. Fanali, in Liquid chromatography. Applications, Elsevier, Amsterdam, 2nd edn, 2017, ch. 5, pp. 107–157 Search PubMed.
-
OECD, Test No. 117: Partition Coefficient (n-octanol/water), HPLC Method, 2004.
- S. Fekete, J. L. Veuthey and D. Guillarme, J. Pharm. Biomed. Anal., 2012, 69, 9–27 CrossRef CAS PubMed.
-
M. I. Aguilar, HPLC of peptides and proteins: basic theory and methodology, 2004 Search PubMed.
-
C. T. Mant, Y. Chen, Z. Yan, T. V. Popa, J. M. Kovacs, J. B. Mills, B. P. Tripet and R. S. Hodges, in Peptide characterization and application protocols, ed. G. B. Fields, Humana Press, Totowa, N.J., 2007, pp. 3–55 Search PubMed.
- C. S. Neuhaus, G. Gabernet, C. Steuer, K. Root, J. A. Hiss, R. Zenobi and G. Schneider, Angew. Chem., Int. Ed., 2019, 58, 1674–1678 CrossRef CAS PubMed.
- M. Shibue, C. T. Mant and R. S. Hodges, J. Chromatogr. A, 2005, 1080, 68–75 CrossRef CAS PubMed.
- E. Lazoura, I. Maidonis, E. Bayer, M. T. Hearn and M. I. Aguilar, Biophys. J., 1997, 72, 238–246 CrossRef CAS PubMed.
- S. E. Blondelle, J. M. Ostresh, R. A. Houghten and E. Perez-Paya, Biophys. J., 1995, 68, 351–359 CrossRef CAS PubMed.
- V. Antonucci, M. B. Hicks, Z. Lin and R. A. Reamer, J. Chromatogr. A, 2003, 983, 73–82 CrossRef CAS PubMed.
- C. J. Smith, A. F. Drake, B. A. Banfield, G. B. Bloomberg, M. S. Palmer, A. R. Clarke and J. Collinge, FEBS Lett., 1997, 405, 378–384 CrossRef CAS PubMed.
- S. Roux, E. Zekri, B. Rousseau, M. Paternostre, J. C. Cintrat and N. Fay, J. Pept. Sci., 2008, 14, 354–359 CrossRef CAS PubMed.
- C. Liang, J. Q. Qiao and H. Z. Lian, J. Chromatogr. A, 2017, 1528, 25–34 CrossRef CAS PubMed.
- R. Pascale, M. A. Acquavia, T. R. I. Cataldi, A. Onzo, D. Coviello, S. A. Bufo, L. Scrano, R. Ciriello, A. Guerrieri and G. Bianco, Anal. Bioanal. Chem., 2020, 412, 3005–3015 CrossRef CAS PubMed.
- A. Kaune, M. Knorrenschild and A. Kettrup, Fresenius' J. Anal. Chem., 1995, 352, 303–312 CrossRef CAS.
- C. T. Mant, J. M. Kovacs, H. M. Kim, D. D. Pollock and R. S. Hodges, Biopolymers, 2009, 92, 573–595 CrossRef CAS PubMed.
- C. T. Mant, Y. Chen and R. S. Hodges, J. Chromatogr. A, 2003, 1009, 29–43 CrossRef CAS PubMed.
- Y. Chen, C. T. Mant and R. S. Hodges, J. Chromatogr. A, 2003, 1010, 45–61 CrossRef CAS PubMed.
- J. M. Kovacs, C. T. Mant and R. S. Hodges, Biopolymers, 2006, 84, 283–297 CrossRef CAS PubMed.
- R. S. Hodges, Y. Chen, E. Kopecky and C. T. Mant, J. Chromatogr. A, 2004, 1053, 161–172 CrossRef CAS PubMed.
- K. Sikora, M. Jaskiewicz, D. Neubauer, D. Migon and W. Kamysz, Pharmaceuticals, 2020, 13, 442 CrossRef CAS PubMed.
- C. K. Wang, S. E. Northfield, J. E. Swedberg, B. Colless, S. Chaousis, D. A. Price, S. Liras and D. J. Craik, Eur. J. Med. Chem., 2015, 97, 202–213 CrossRef CAS PubMed.
- K. Sakagami, T. Masuda, K. Kawano and S. Futaki, Mol. Pharmaceutics, 2018, 15, 1332–1340 CrossRef CAS PubMed.
- H. N. Hoang, T. A. Hill and D. P. Fairlie, Angew. Chem., Int. Ed., 2021, 60, 8385–8390 CrossRef CAS PubMed.
- O. D. Monera, T. J. Sereda, N. E. Zhou, C. M. Kay and R. S. Hodges, J. Pept. Sci., 1995, 1, 319–329 CrossRef CAS PubMed.
- P. Hemstrom and K. Irgum, J. Sep. Sci., 2006, 29, 1784–1821 CrossRef PubMed.
- P. Jandera, Anal. Chim. Acta, 2011, 692, 1–25 CrossRef CAS PubMed.
- G. Schuster and W. Lindner, J. Chromatogr. A, 2013, 1301, 98–110 CrossRef CAS PubMed.
- G. Schuster and W. Lindner, J. Chromatogr. A, 2013, 1273, 73–94 CrossRef CAS PubMed.
- A. Periat, I. S. Krull and D. Guillarme, J. Sep. Sci., 2015, 38, 357–367 CrossRef CAS PubMed.
- B.-Y. Zhu, C. T. Mant and R. S. Hodges, J. Chromatogr., 1991, 548, 13–24 CrossRef CAS PubMed.
- C. T. Mant, Z. Jiang, B. E. Boyes and R. S. Hodges, J. Chromatogr. A, 2013, 1277, 15–25 CrossRef CAS PubMed.
- M. Hoshino, N. Yumoto, S. Yoshikawa and Y. Goto, Protein Sci., 1997, 6, 1396–1404 CrossRef CAS PubMed.
- E. P. Kadar, C. E. Wujcik, D. P. Wolford and O. Kavetskaia, J. Chromatogr., B, 2008, 863, 1–8 CrossRef CAS PubMed.
- B. Bard, P. A. Carrupt and S. Martel, J. Med. Chem., 2009, 52, 3416–3419 CrossRef CAS PubMed.
- B. Bard, P. A. Carrupt and S. Martel, J. Chromatogr. A, 2012, 1260, 164–168 CrossRef CAS PubMed.
- C. T. Mant and R. S. Hodges, J. Sep. Sci., 2008, 31, 2754–2773 CrossRef CAS PubMed.
- C. T. Mant, J. R. Litowski and R. S. Hodges, J. Chromatogr. A, 1998, 816, 65–78 CrossRef CAS PubMed.
- C. T. Mant, L. H. Kondejewski and R. S. Hodges, J. Chromatogr. A, 1998, 816, 79–88 CrossRef.
- M. Gilar, P. Olivova, A. E. Daly and J. C. Gebler, Anal. Chem., 2005, 77, 6426–6434 CrossRef CAS PubMed.
- E. Lesellier and C. West, J. Chromatogr. A, 2015, 1382, 2–46 CrossRef CAS PubMed.
- A. Van Eeckhaut and D. Mangelings, J. Pharm. Biomed. Anal., 2015, 113, 181–188 CrossRef CAS PubMed.
- J. Zheng, J. D. Pinkston, P. H. Zoutendam and L. T. Taylor, Anal. Chem., 2006, 78, 1535–1545 CrossRef CAS PubMed.
- D. Tognarelli, A. Tsukamoto, J. Caldwell and W. Caldwell, Bioanalysis, 2010, 2, 5–7 CrossRef CAS PubMed.
- E. Lesellier, Bioanalysis, 2011, 3, 125–131 CrossRef CAS PubMed.
- V. Desfontaine, D. Guillarme, E. Francotte and L. Novakova, J. Pharm. Biomed. Anal., 2015, 113, 56–71 CrossRef CAS PubMed.
- G. L. Losacco, J. O. DaSilva, J. Liu, E. L. Regalado, J. L. Veuthey and D. Guillarme, J. Chromatogr. A, 2021, 1642, 462048 CrossRef CAS PubMed.
- C. West, J. Melin, H. Ansouri and M. Mengue Metogo, J. Chromatogr. A, 2017, 1492, 136–143 CrossRef CAS PubMed.
- M. A. Patel, F. Riley, M. Ashraf-Khorassani and L. T. Taylor, J. Chromatogr. A, 2012, 1233, 85–90 CrossRef CAS PubMed.
- M. Ventura, J. Pharm. Biomed. Anal., 2020, 185, 113227 CrossRef CAS PubMed.
- J. A. Blackwell, R. W. Stringham and J. D. Weckwerth, Anal. Chem., 1997, 69, 409–415 CrossRef CAS PubMed.
- M. A. Patel, F. Riley, J. Wang, M. Lovdahl and L. T. Taylor, J. Chromatogr. A, 2011, 1218, 2593–2597 CrossRef CAS PubMed.
- C. West and E. Lesellier, J. Chromatogr. A, 2006, 1110, 181–190 CrossRef CAS PubMed.
- C. West, S. Khater and E. Lesellier, J. Chromatogr. A, 2012, 1250, 182–195 CrossRef CAS PubMed.
- C. West and E. Lesellier, J. Chromatogr. A, 2006, 1110, 200–213 CrossRef CAS PubMed.
- C. West and E. Lesellier, J. Chromatogr. A, 2006, 1115, 233–245 CrossRef CAS PubMed.
- G. H. Goetz, W. Farrell, M. Shalaeva, S. Sciabola, D. Anderson, J. Yan, L. Philippe and M. J. Shapiro, J. Med. Chem., 2014, 57, 2920–2929 CrossRef CAS PubMed.
- G. H. Goetz, L. Philippe and M. J. Shapiro, ACS Med. Chem. Lett., 2014, 5, 1167–1172 CrossRef CAS PubMed.
|
This journal is © The Royal Society of Chemistry 2022 |
Click here to see how this site uses Cookies. View our privacy policy here.