DOI:
10.1039/D2LC00640E
(Paper)
Lab Chip, 2022,
22, 4950-4961
A 3D-printed microfluidic gradient generator with integrated photonic silicon sensors for rapid antimicrobial susceptibility testing†
Received
12th July 2022
, Accepted 27th October 2022
First published on 22nd November 2022
Abstract
With antimicrobial resistance becoming a major threat to healthcare settings around the world, there is a paramount need for rapid point-of-care antimicrobial susceptibility testing (AST) diagnostics. Unfortunately, most currently available clinical AST tools are lengthy, laborious, or are simply inappropriate for point-of-care testing. Herein, we design a 3D-printed microfluidic gradient generator that automatically produces two-fold dilution series of clinically relevant antimicrobials. We first establish the compatibility of these generators for classical AST (i.e., broth microdilution) and then extend their application to include a complete on-chip label-free and phenotypic AST. This is accomplished by the integration of photonic silicon chips, which provide a preferential surface for microbial colonization and allow optical tracking of bacterial behavior and growth at a solid–liquid interface in real-time by phase shift reflectometric interference spectroscopic measurements (PRISM). Using Escherichia coli and ciprofloxacin as a model pathogen-drug combination, we successfully determine the minimum inhibitory concentration within less than 90 minutes. This gradient generator-based PRISM assay provides an integrated AST device that is viable for convenient point-of-care testing and offers a promising and most importantly, rapid alternative to current clinical practices, which extend to 8–24 h.
Introduction
The emergence of antimicrobial resistance in bacterial and fungal pathogens caused by the extensive use and misuse of antimicrobials has led to the emergence of ‘superbugs’ and a global health crisis.1,2 According to a recent study by the antimicrobial resistance collaborators, ∼1.3 million deaths were directly attributed to antimicrobial resistance in 20193 and by 2050, antimicrobial-resistant infections could even come to surpass cancer as the leading cause of mortality.4 This concerning situation is only further exacerbated by the lack of a robust pipeline for developing new antimicrobials – which underscores the pressing need for proper antimicrobial stewardship (especially efforts to curb the excessive use of antimicrobials and the spread of drug resistance by encouraging physicians to prescribe suitable antimicrobials only when needed).5,6 An essential part of effective antimicrobial stewardship is clinical antimicrobial susceptibility testing (AST). In these assays, pathogens are exposed to a panel of antimicrobials at varying concentrations so that the minimum inhibitory concentration (MIC) can be determined. This value is typically defined as the lowest drug concentration that inhibits the pathogens' growth and helps physicians to differentiate between resistant and susceptible isolates and choose the adequate antimicrobial for treatment.13–15 Yet gold-standard reference AST techniques, such as broth microdilution (BMD) and agar-based methods in which growth can be determined by absorbance measurements16 or visually,17,18 are labor-intensive procedures with long (≥16 h) wait times and are not well-suited for point-of-care (PoC) purposes.16–19
To overcome these limitations, extensive research is directed towards the development of microfluidic devices for miniaturized and rapid AST assays20,21 using various sensing approaches (e.g., chromogenic agar,22 oxygen consumption,23,24 membrane integrity,25 single-cell imaging,26 or metabolic activity/metabolite analysis27–29). However, in most studies, the total assay time (including sample preparation and time readout) is still in the range of several hours, and sample preparation should be further simplified.20 To answer these challenges and make AST procedures more appropriate for deployment at PoC, the integration of microfluidic gradient generators (GG) in AST systems is suggested.7–12,30–32 These GG devices are designed to establish a desired antimicrobial dilution series – combining advantages of automation, and minimized sample and reagents consumption. The automatically generated concentrations are then collected and used for BMD testing, or the AST procedure is performed within the microfluidic system. Yet, existing microfluidic GG AST systems still suffer from different shortcomings; for example, in diffusion-based assays, the diffusion behavior of each antimicrobial agent must be well known to accurately predict the specific concentration in the area of interest.30,31 By contrast, convective gradient generators allow a high control of the respective concentration without a precise determination of the antimicrobials' diffusive behavior. Yet, tree-like gradient generators (based on a cascade of divisions and reunions with an additional branch at every level) that represent the standard system of such convective gradient generators are often constrained by a maximal flow rate (as thorough mixing is required between each reunion and division).33 Moreover, reported convective GG AST systems only apply non-clinically relevant linear concentration gradients,7,8,10 require inconvenient off-chip testing after antimicrobial solution collection,8,11 need to be coupled with sophisticated microscopy analysis,7,10 or provide AST results within ≥8 h8,9 which in a clinical setting often corresponds to next-day results only.13 See Table 1 for a summary of previously reported relevant convective GG devices for AST.
Table 1 Relevant convective microfluidic GG systems for AST assays previously reported in the literature. The gradient generator devices are ordered according to their reported assay time, from the lengthiest to the most expeditious
Principle |
Gradient |
Flow rate (μL min−1) |
AST method |
Label |
Assay time (h) |
Ref. |
Tree-like |
Linear |
0.167 |
Fluorescence imaging of stained biofilm |
Yes |
>24 |
7
|
One-step inlet position |
Linear |
2280 |
Off-chip turbidity |
No |
Overnight |
8
|
Vacuum driven |
Exponential (two-fold dilution) |
No data |
Fluorescence detection (resazurin-based redox indicator) |
Yes |
8 |
9
|
Acoustic waves |
Linear |
3.3 |
Time-lapse microscopy (covered area) |
No |
5.5 |
10
|
3D tree-like |
Symmetric gradient of 3 fluids |
1000 |
Off-chip fluorescence detection (resazurin-based redox indicator) |
Yes |
5 |
11
|
Tree-like |
Exponential (two-fold dilution) |
1.5 |
Microscopy (cell counting) |
No |
3 |
12
|
One-step parallel channels |
Exponential (two-fold dilution) |
1000 |
PRISM |
No |
≤1.5 |
This study |
In this work, we build a 3D-printed GG system for creating clinically relevant two-fold dilution series of antimicrobials and integrate it with an on-chip phenotypic AST, allowing for an automatic rapid susceptibility determination (within <90 min). The microfluidic GG principle relies on adjusting the channel length ratios of parallel channels33 and flow rate ratios to generate defined concentrations in one step. The device is fabricated from a biocompatible and heat-resistant34 polyacrylate material by high-resolution 3D printing – a technique that enables the manufacturing of microfluidic devices and has accordingly assumed increasing importance in many biotechnology-related fields, ranging from diagnostics to cell culture.35–39 Compared to standard fabrication processes such as polydimethylsiloxane-based soft-lithography,10,12,40 3D printing offers rapid prototyping of complex structures in a single step,38,41 the use of various and versatile materials,34,40,42 and does not require sophisticated microfabrication facilities.12,38 The functionality of the GG is first established off-chip, see Fig. 1A, for various clinically relevant microorganisms (bacterial and fungal) using the gold standard BMD assay. In these tests, two-fold serial dilutions of antimicrobials are prepared in a liquid growth medium and inoculated with a predefined and standardized cell number. Next, we interface the gradient generator with an array of photonic silicon chips (Fig. 1B-i), consisting of microwell diffraction gratings, which provide a preferential surface for microbial colonization and act as an optical transducer element and previously enabled real-time and rapid AST of Escherichia coli (E. coli) in 2–3 h and Aspergillus niger (A. niger) in 10–12 h.43,44 In this label-free PRISM (phase shift reflectometric interference spectroscopic measurement) method, continuous reflectivity measurements (Fig. 1B-ii) track bacterial growth in the presence of different antibiotic concentrations within the microwell structure of photonic silicon chips. After applying frequency analysis, single peaks are obtained (Fig. 1B-v and -vi) where the peak position corresponds to 2nL (n is the refractive index of the medium within the grating and L represents the height of the microstructures). At antibiotic concentrations ≥ MIC (Fig. 1B-iii), bacterial growth is inhibited and the 2nL value remains largely unchanged (Fig. 1B-v), whereas uninhibited bacterial growth (Fig. 1B-iv) results in an increase of the 2nL due to refractive index changes (Fig. 1B-vi). Susceptibility and corresponding MIC values are determined by the extent of antimicrobial-hindered bacterial growth within the grating by monitoring the 2nL value over time (Fig. 1B-vii). The resulting integrated platform, combining the microfluidic GG device and PRISM sensors, allows for rapid (90 min) phenotypic AST in a label-free format, which is highly applicable in PoC settings.
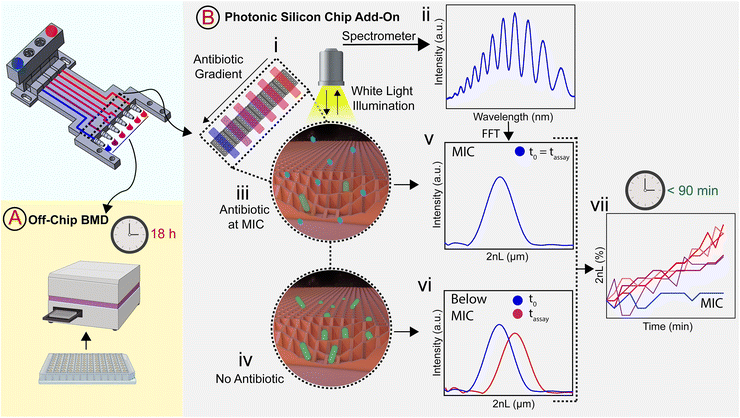 |
| Fig. 1 (A) First, the functionality of the GG device is established by the gold-standard BMD method. The GG is used to generate two-fold dilutions of antimicrobials that are tested in a 96 well plate against three different microorganisms (E. coli, S. marcescens, and C. auris). The MIC is determined after incubation by absorbance measurements or visually. (B-i) Second, the integration of photonic silicon chips consisting of microwell diffraction gratings for rapid optical AST (PRISM) is demonstrated. (B-ii) Illumination of the photonic silicon chips by a white light source results in characteristic reflectivity spectra exhibiting interference fringes as light is partially reflected from the top and bottom surfaces of the silicon microwell diffraction grating. These reflectivity spectra are recorded and analysed in real time, enabling label-free monitoring of bacterial growth in the presence of varying antibiotic concentrations generated by the GG device. (B-iii) Bacterial growth is inhibited at antibiotic concentrations ≥ MIC, while at (B-iv) subinhibitory concentrations, the bacteria grow and proliferate within the microwells. To monitor this bacterial behaviour, the reflectivity spectrum (at each time point) is analysed by frequency analysis (FFT), yielding a single peak where the peak position corresponds to the 2nL value (n = refractive index within the microwells; L = height of the microstructure). When bacterial growth is inhibited, (B-v) this 2nL value remains unchanged, while (B-vi) it increases when bacteria grow inside the microwells and induce changes in the average refractive index values. (B-vii) The 2nL is monitored in real-time and used to track bacterial growth at varying antimicrobial concentrations to determine the MIC value within less than 90 min. | |
Experimental
Materials, media, and microbial strains
VisiJet® M2S-HT90 3D printing material and wax support material VisiJet® M2 SUP were purchased from 3D Systems Inc. (SC, USA). Cation-adjusted Muller Hinton broth (CAMHB), Roswell Park Memorial Institute medium (RPMI 1640), 3-(N-morpholino)propanesulfonic acid (MOPS), glutaraldehyde, D-glucose, voriconazole, and ciprofloxacin were supplied by Sigma-Aldrich, Israel. All buffer salts were obtained from Merck, Germany. Potato dextrose agar (PDA), bacto agar, and Luria-Bertani (LB) broth were supplied by Becton Dickinson (Difco, USA). Absolute ethanol was purchased from Gadot, Israel. Detergent fairy ultra plus was purchased from Procter and Gamble (Cincinnati, USA), gentamicin sulfate from AppliChem GmbH (Darmstadt, Germany), Pelikan ink 156372 (designated blue dye) with an estimated diffusion coefficient45 of ∼10−10 m2 s−1 from Pelikan Group GmbH (Falkensee, Germany), and paraffin oil of low viscosity from Carl Roth GmbH + Co. K.G. (Karlsruhe, Germany). All aqueous solutions and media were prepared in deionized water (18.2 MΩ cm). Phosphate buffered saline (PBS) was constituted of 137 mM NaCl, 2.7 mM KCl, 1.8 mM KH2PO4, and 10 mM Na2HPO4. RPMI 1640 2% G medium (RPMI 1640 medium supplemented with 2% D-glucose) was composed of 10.4 g L−1 RPMI 1640, 34.5 g L−1 MOPS, and 18 g L−1D-glucose. LB agar was made from 25 g L−1 LB broth and 15 g L−1 bacto agar. PDA plates and CAMHB medium were prepared according to the manufacturer's instructions. All media and buffer were heat-steam sterilized or sterile-filtered before use. E. coli K12 and Serratia marcescens (S. marcescens) were generously provided by Prof. Sima Yaron (Department of Biotechnology and Food Engineering, Technion – Israel Institute of Technology). Candida auris (C. auris) DSM 21092 was obtained from the German Collection of Microorganisms and Cell Cultures.
Preparation of microbial cultures
Microbial cultures were stored as cryo cultures at −70 °C. Prior to AST experiments, the cells were sub-cultured onto LB agar (bacteria) or PDA (yeast) and incubated at 37 °C for 16–24 h. Colonies from these agar plates were directly used to obtain the cell suspensions used in AST experiments.
3D printing and post-processing
The computer-aided design (CAD) model was created using SolidWorks 2020 (Dassault Systèmes SolidWorks Corp, Waltham, MA, USA). The model was saved as an .STL file for printing purposes, and as an .SAT file for simulations. All files are provided in the ESI.† Models were printed using a high-resolution 3D printer (ProJet® MJP 2500 Plus, 3D Systems, SC, USA) with a xyz resolution of 32, 28 and 32 μm, respectively. The GG was printed with its inlets facing up, as shown in Fig. S1.† Further information on the accuracy of the printing process is given in Fig. S2 and Table S1.† The printed device was removed from the printing platform after incubation at −18 °C for 10 min. Afterward, all pieces were placed in EasyClean units from 3D systems (water vapor bath and hot paraffin oil bath at 65 °C) to remove the wax support material. The interior structures were flushed at least three times with hot paraffin oil using a syringe. To remove oil residues, the parts were then submerged in an ultrasonic bath (Elma Elmasonic S30, Elma, Schmidbauer GmbH, Singen, Germany) with water and detergent at 50 °C for at least three times. Subsequently, the device was cleaned with deionized water and dried at 70 °C for 1 hour.
Computational fluid dynamics (CFD) simulations
The functionality of the design was tested via running a computational fluid dynamics (CFD) simulation using COMSOL® Multiphysics 5.5 (COMSOL Inc., Stockholm, Sweden). General simulation settings were chosen according to Enders et al.41 As physical properties, a density of 1 g cm−3 and a dynamic viscosity of 10−3 kg m−1 s−1 were chosen for water. For inlet A, the concentration of a fictive substance with a diffusion constant of 10−9 m2 s−1 was set to 1 mol m−3, while the concentration of inlet B was defined as 0 mol m−3. To simulate the performance of the designed GG, the inflows at inlet A and inlet B were defined as 323 μL min−1 and 677.3 μL min−1, respectively.
Experimental gradient generating accuracy studies
The source fluids – blue dye (ink; 1
:
30 diluted), glucose (6 g L−1), and ciprofloxacin (1 mg L−1) – were introduced into the device by a syringe pump into inlet A at a flow rate of 323 μL min−1, while the sink fluid water was introduced by a second syringe pump into inlet B at a flow rate of 677.3 μL min−1. After 5 min, the first fractions were collected from the six outlets, the pumps were stopped, and the solutions were transferred into a 96 well plate or reaction tubes. Next, the pumps were started again, and after 30 s a second batch was collected. This procedure was repeated again, for the collection of a third batch from every outlet. The dye was quantified at 600 nm (NanoDrop™ 2000 spectrophotometer, Thermo Fisher Scientific GmbH, Dreieich, Germany), the glucose concentration was measured using a Cedex BioAnalyzer (Roche Diagnostics Deutschland GmbH, Mannheim, Germany), and ciprofloxacin was quantified via a fluorescence assay (λex 272 nm, λem 421 nm; Varioskan Flash, Thermo Scientific, USA). For every source fluid, a different GG device was used. The photograph of the dye-water gradient was obtained using a VHX-6000 digital microscope (Keyence Deutschland GmbH, Neu-Isenburg, Germany).
Broth microdilution
Gold standard BMD was performed using the protocols that are recommended by EUCAST, namely ISO 20776-1:2020 standard for bacteria18 and the EUCAST AFST protocol for yeast.16 Two-fold dilutions of the antimicrobials in growth medium (CAMHB for bacteria and RPMI 2% G medium for yeast) were prepared in 96 well plates and tested against a standardized cell density of 5 × 105 cells mL−1 (bacteria) or 105 cells mL−1 (yeast) unless otherwise stated. For bacterial species, the MIC was determined visually after 18 h incubation at 37 °C supported by absorbance measurements at 600 nm (Varioskan Flash, Thermo Scientific, USA). The MIC of C. auris (yeast) was determined by absorbance measurements at 530 nm after incubation for 24 h at 37 °C. Every gold standard BMD test was repeated at least three times with n ≥ 3 for every concentration tested. Before obtaining the antimicrobial dilutions for the GG-based BMD, the GG device was heat-steam sterilized and employed as described in the previous section. Accordingly, the source fluids ciprofloxacin (1 mg L−1), gentamicin (1 mg L−1), and voriconazole (0.25 mg L−1) in growth medium were diluted by the sink fluid (CAMHB or RPMI 2% G medium) to obtain the desired two-fold dilutions.
Photonic silicon Chip integration
Silicon chips with microwells (width of approx. 4 μm and depth of approx. 4 μm) were fabricated by laser writing and reactive ion etching technologies at the micro- and nano-fabrication and printing unit (Technion – Israel Institute of Technology.44 A stepwise protocol for the fabrication procedure of the chips is given in the ESI† (section S2). The chips (diced into 5 × 5 mm) were individually placed into the square-shaped cavities (5.1 × 5.1 mm) of the GG. UV-curable glue (Norland Optical Adhesive 72, Norland Products Inc., NJ, USA) was carefully applied to the chip backside of the photonic silicon sensor and allowed to cure for 30 min under UV-light at 365 nm with 1.5 mW cm−2 (VL-6.LC UV lamp 365/254 nm 6 W, Vilber Lourmat, Collégien, France) to seal the system leakage-free. To improve the optical properties, UV-curable lacquer (luxaprint® shellac, DETAX GmbH, Ettlingen, Germany) was applied into rectangular depressions on top of the 3D-printed surfaces above the integrated photonic silicon chips and cured for 5 min under UV-light using the same conditions as for the Norland Optical Adhesive.
Gradient generator-based PRISM assay
The GG device with integrated photonic silicon chips was placed onto a heat-controlled aluminium sub-structure (37 °C) and connected via a 3D-printed adapter to a motorized linear stage (Thorlabs, Inc., USA) for movement control. 70% ethanol was used to disinfect the system before growth medium (CAMHB) was introduced – allowing devices, temperature, and medium to equilibrate. Subsequently, suspensions of E. coli (McFarland 0.5) and 1 mg L−1 ciprofloxacin (source fluid) and E. coli (McFarland 0.5) without antibiotic (sink fluid) were introduced for 5 min. The outlets were then closed (using silicone tubing and Luer adapters), and the bacteria were given 10 min to settle within the microstructure before the optical assay (PRISM) was initiated. Data acquisition and analysis were performed according to our previous work:43,44 a bifurcated fibre optic (Ocean Optics, USA) equipped with a collimating lens was arranged normal to the photonic silicon chips. Through the bifurcated fibre, the chips were illuminated via a HL-2000 white light source (Ocean Optics, USA), and the reflected light was transmitted to a USB4000 CCD spectrometer (Ocean Optics, USA). Fast Fourier transform (FFT) frequency analysis was performed on the acquired spectra in the range of 450–900 nm. In the resulting peak, the peak position corresponds to the 2nL, where n represents the refractive index of the medium filling the microstructure and L the height of the microwells. The 2nL values were plotted versus time to monitor bacterial growth. The percent changes of 2nL (Δ2nL) were calculated as follows:
where 2nL is the 2nL value at a given time, and 2nL0 corresponds to the 2nL when the PRISM assay was started.
Photonic silicon Chip characterization
The photonic silicon microstructure was studied by high-resolution scanning electron microscopy (HR-SEM) using a Zeiss Ultra Plus high-resolution scanning microscope (Carl Zeiss, Germany).
Results and discussion
Gradient generator principle and design
The design of the 3D-printed GG consists of different functional units (modular design), as illustrated in Fig. 2A. Both source (e.g., antimicrobial solution) and sink (e.g., buffer or medium) fluids are introduced from the outer inlets into the device. The inner inlets serve as a port through which ethanol can be introduced to remove air bubbles and disinfect the device. The gradient generating unit is the core module of the device – this is where the dosing of the required concentrations (i.e., two-fold dilutions) is achieved. In Fig. 2B, the gradient generating principle is schematically illustrated: the source fluid is introduced via a syringe pump (at a constant flow rate) into the reservoir A/inlet A, while a second syringe pump likewise introduces the sink fluid into the reservoir B/inlet B. Both these reservoirs are connected by a series of parallel microfluidic channels where the source and sink fluid combine and mix with each other. The concentration dosing is achieved by adjusting the length ratios of these connected channels, based on the simple principle of fluid mechanics, which holds that the longer the channel, the higher the hydrodynamic resistance – resulting in reduced flow rates in comparison to shorter channels.33,46 For example, when the length of both channels is equivalent (see the first horizontal channel in the center), the sink and source fluids merge at the same flow rate, and dosing of 50% (relative concentration of the source fluid) is achieved. However, when the channel from the sink fluid reservoir is shorter than the channel that transfers the source fluid (see the fourth horizontal channel in the center), the latter is diluted to much lower concentrations (here 6.25%) since it perfuses at a much lower flow rate. Please note that a higher volume of the sink fluid, compared to the source fluid, is required to achieve a two-fold dilution series, and, accordingly, the sink fluid is introduced at a higher flow rate (677.3 μL min−1) than the source fluid (323 μL min−1). For a detailed step-by-step explanation of the gradient generating principle and how the parallel channel length rations are adjusted, the reader is referred to the ESI† (see section S3 for equations and description). Also, this section (Fig. S3†) provides additional detailed technical drawings of the GG unit and the micromixers.
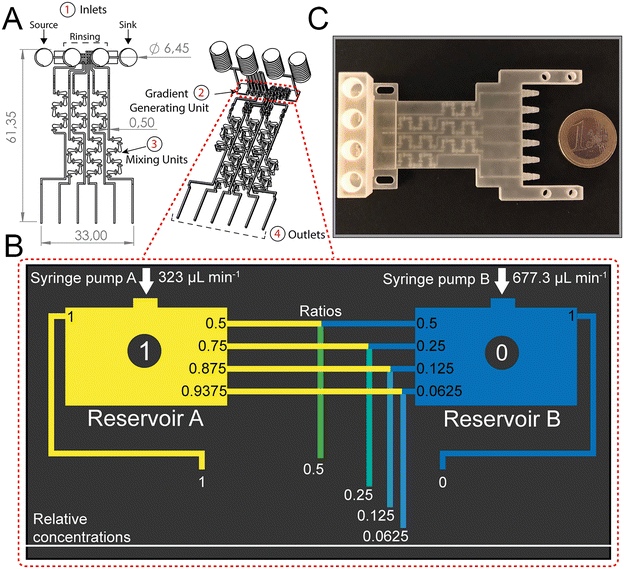 |
| Fig. 2 Design and principle of the 3D-printed GG for two-fold dilutions dosing. (A) The technical drawing depicts the different modules of the device: (1) inlets to introduce source and sink fluids and rinsing with ethanol, (2) gradient generating unit where the dosing of the required concentrations is achieved, (3) mixing unit with integrated micromixers facilitates homogenous mixing and (4) outlets from where the solutions are collected. Dimensions are shown in millimeters (mm). If not further specified, the microfluidic channels were printed with dimensions of 0.5 mm × 0.5 mm (width × height). The internal volume of the whole device is 455 μL. (B) Schematic presenting the principles of the gradient generating unit. The source fluid is introduced into reservoir A, whereas the sink fluid is pumped into reservoir B. White numbers within the black circle represent the relative concentrations of the source fluid in reservoir A and reservoir B. By adjusting the flow rate ratio according to the channel length ratios (black annotations) of the conjoining horizontal channels, a two-fold dilution series is obtained (white lettering). (C) Photograph of the final 3D-printed GG device after post-processing procedure. | |
From the GG unit, the merged fluids are then transferred to the mixing unit, where the integration of passive HC-micromixers41 enables homogenous mixing of these solutions emphasized by computational fluid dynamic (CFD) simulations as depicted in Fig. S4.† Homogenous mixing is critical when surface-based sensing elements such as the PRISM silicon chips are integrated into the device for bioanalytical analysis. Our group47 and others48,49 have demonstrated that providing uniform analyte concentration to the silicon sensor surface is crucial in minimizing the analyte depletion and hindered mass transfer. After leaving the mixing unit, the fluids flow towards the outlets, where they are collected for quantification or subsequent AST. According to the GG design, the calculated flow rate at every outlet is ∼166.7 μL min−1, and indeed Fig. S5† shows equivalent flow rates at the various outlets with an overall outlet flow rate (940 μL min−1) that is consistent with the total inlet flow rate (∼1000 μL min−1).
Gradient generator characterization
The dosing accuracy of the GG was studied by conducting CFD simulations and experimental analyses of various model solutions (i.e., dye, glucose, and ciprofloxacin) with distinct properties. For example, dyes are often used to investigate the (mixing) performance in microfluidic systems;11,31,41 in this work, the dye (blue ink) concentration was quantified by absorbance measurements at 600 nm. Glucose served as a hydrophilic model compound to reflect the behavior of aminoglycoside antibiotics, such as gentamicin, and its concentration was determined using a photometric enzymatic (hexokinase) assay.50 As a relevant model antibiotic, which is also used in the subsequent AST assays, ciprofloxacin is employed, harnessing its intrinsic fluorescence.51Fig. 3A presents a CFD simulation of the device at the designated flow rates and the corresponding photograph of the generated gradient using an aqueous dye solution. Fig. 3B compares the simulated concentration values to those collected experimentally for all three model solutions.
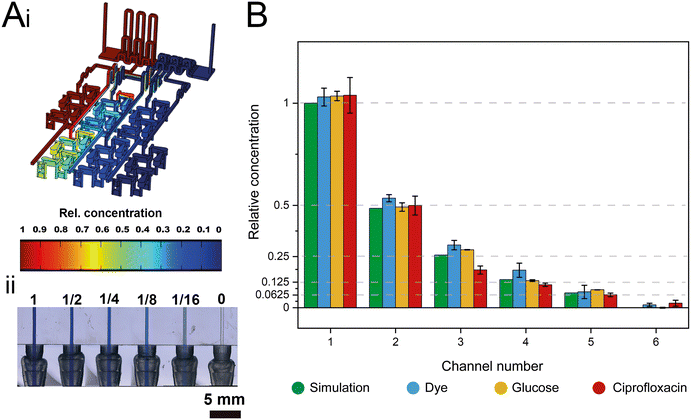 |
| Fig. 3 Characterization of the gradient generator using simulation and experimental gradient generating accuracy studies. (A-i) CFD simulation using COMSOL Multiphysics® at a total flow rate of 1000 μL min−1. (A-ii) Photograph at the outlets of the 3D-printed gradient generator depicting a two-fold dilution gradient using dye (source) and water (sink). (B) Relative concentrations for the six channels determined by CFD simulation and experimental studies using a dye (blue ink), glucose, and ciprofloxacin as source solutions. Error bars indicate the standard deviation of three consecutive experiments. Dashed lines indicate desired concentrations for each outlet. | |
The measured concentrations of the generated solutions depict an accurate two-fold dilution series with high values of the coefficient of determination (R2 simulation = 0.9995; R2 dye = 0.9974, R2 glucose = 0.998, and R2 ciprofloxacin = 0.9929) which are comparable to accuracies presented in previously published GG systems.8,12 The results also suggest that possible adsorption effects of the solutions to the 3D-printing material are negligible. The small differences in the determined concentrations for the different source fluids are ascribed to slight deviations in the channel dimensions that may influence the network of finely balanced channel lengths (ratios) and effectively also the concentrations at the outlets. Please refer to Table S1† for further information on the printing accuracy.
GG-enabled AST
The successful miniaturization, integration, and automation of the various steps required in AST methods (e.g., generation of two-fold antimicrobial dilution series, cell inoculum, sensing, and MIC determination) are considered essential prerequisites for providing rapid and easy-to-use AST assays for point-of-care applications.14,52 We thus envisioned a microfluidic system that automatically generates the desired antimicrobial concentrations and also integrates the sensing elements for convenient and time-saving real-time optical AST directly within the microfluidic channels of the GG-system. Before integrating photonic silicon chips as sensing elements for on-chip AST into our device, we first test the compatibility of the 3D-printed GG with the gold-standard BMD assay (schematically illustrated in Fig. 1A). We used three clinically-relevant model pathogen-drug combinations – namely, S. marcescens vs. ciprofloxacin, E. coli vs. gentamicin, and C. auris vs. voriconazole. S. marcescens and E. coli are both Gram-negative bacteria that can cause, among other serious ailments, urinary tract infections (UTIs), diarrheal diseases, pneumonia, and sepsis;53,54 their susceptibility was tested against antibiotics with different modes of action. While ciprofloxacin (fluoroquinolone) inhibits the gyrase enzyme, which plays an essential role in DNA replication,55 gentamicin (aminoglycoside) binds to the 30S subunit of the ribosomes and thus inhibits the protein biosynthesis.56 As a fungal pathogen, C. auris (an opportunistic yeast) causes severe invasive infections and shows alarming rates of acquired antifungal resistance;57,58 it was studied against the antifungal drug voriconazole, which inhibits the ergosterol synthesis (crucial cell wall component) and is applied for invasive candidiasis and aspergillosis treatment.59 From the gradient generator, five consecutively obtained fractions (F1–F5) were collected for every tested concentration (GG-enabled BMD), and the attained relative growth values for all pathogen-drug combinations are presented in Fig. 4 and compared to reference BMD results (see grey panels). Although at least at subinhibitory antimicrobial concentrations, the growth behaviour can deviate between fractions, the MIC values that were calculated for the GG-enabled BMD – defined as the lowest drug concentration which completely inhibits the growth (bacteria) or induces 50% growth inhibition (yeast) – were accurately determined. Moreover, they agree with the reference BMD and MIC values published by the European Commission for Antimicrobial Susceptibility Testing (EUCAST); see Table S3† for a detailed summary and comparison. Accordingly, we determined that this GG allows for MIC determination of antimicrobials in a concentration range of 0.016 mg L−1 to 0.125 mg L−1, which is suitable for the vast majority of drug-pathogen combinations according to EUCAST.60 To allow for a complete on-chip AST, we modified the 3D-printed GG to include “growth chambers”, which house the PRISM photonic silicon chips, as shown in Fig. 5A-i and S6A.† These PRISM chips are diffraction gratings consisting of periodic arrays of square-shaped microwells, with dimensions of ∼4 × 4 μm, as depicted by the scanning electron micrograph in Fig. 5A-ii.
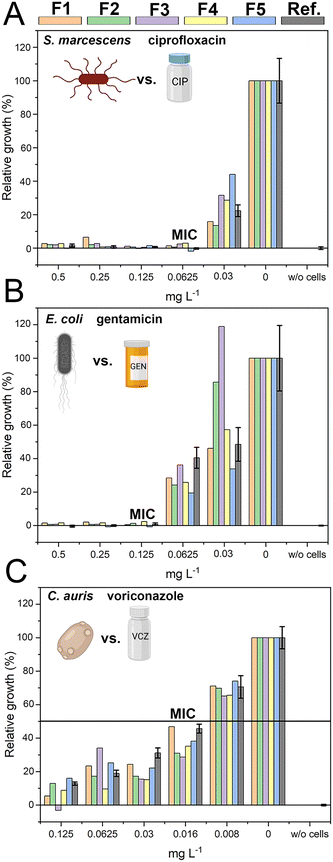 |
| Fig. 4 Comparison of GG-enabled BMD and manual reference BMD for AST of (A) S. marcescens and ciprofloxacin, (B) E. coli K12 and gentamicin and (C) C. auris and voriconazole. For bacterial species, the MIC is defined as the lowest drug concentration that inhibits bacterial growth determined visually and supported by absorbance measurements at 600 nm. For C. auris, the MIC is defined as the lowest drug concentration that induces a 50% growth inhibition obtained from absorbance measurements at 530 nm, as emphasized by the threshold line. For the GG-enabled BMD, five separately and consecutively collected fractions (F1–F5) were tested against the respective pathogens. For all reference BMD tests (ref.), every concentration was tested with n ≥ 3; error bars depict the standard deviation of these measurements. Growth in the absence of drug was defined as 100% growth. | |
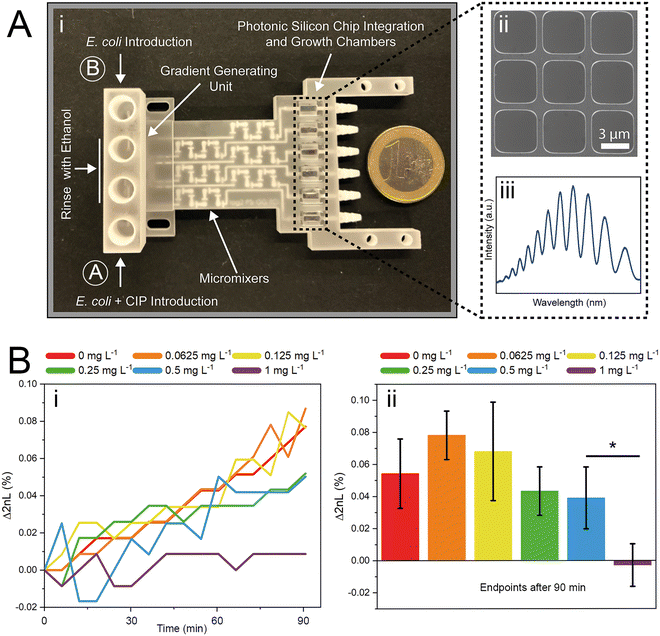 |
| Fig. 5 3D-printed gradient generator for PRISM experiments. (A-i) A photograph of the 3D-printed gradient generator device depicts the different modules of the device, including a square-shaped (5.01 × 5.01 mm) unit for photonic silicon chip integration. The black dashed rectangle emphasizes the integrated photonic silicon chips that (A-ii) feature a silicon diffraction grating of microwells with a width of ∼4 μm and a depth of ∼4 μm and (A-iii) allows obtaining reflectivity spectra with characteristic interference fringes. (B-i) Characteristic PRISM growth curves (Δ2nL over time) from the six growth chambers of the gradient generator for a two-fold dilution series (0–1 mg L−1) of ciprofloxacin (CIP) against E. coli. (B-ii) Δ2nL (%) endpoints from three experiments (error bars = standard deviation) demonstrate that the MIC value can be determined within 90 min. | |
We demonstrate that the integration of these optical sensing elements does not impair the dosing performance of the 3D-printed GG (see Fig. S6B†), where the obtained coefficients of determination (R2) are >0.994, similar to the results obtained for the GG itself (Fig. 3B). Next, we conducted a proof-of-concept on-chip AST study, where we chose E. coli as our model pathogen, in light of the major role it plays in causing UTIs (85–95% of reported cases) and severe sepsis (17% of reported cases)61 and the emergence of drug-resistant E. coli variants.62,63 The principle of the on-chip PRISM assay was as follows: In the GG-based PRISM AST, E. coli and the highest tested antibiotic concentration (here ciprofloxacin at 1 mg L−1) were both introduced into inlet A (Fig. 5A-i), while only E. coli was introduced into inlet B. Both suspensions were introduced into the heat-controlled device at the designated flow rates for 5 min; the dosing was achieved in the GG unit, and in the mixing unit, the suspensions were homogeneously mixed before being transferred onto the “growth chambers” that house the photonic silicon chips. Please refer to the ESI† (Fig. S7) demonstrating that such an experimental procedure in which E. coli is exposed to the highest tested ciprofloxacin concentration for 5 min before being diluted to the designated antibiotic concentrations does not result in flawed MIC values. The assay was operated at a cell density value of McFarland 0.5 (a turbidity standard corresponding to ∼108 cells mL−1), which is of high clinical relevance and used to standardize the cell density in most clinical AST assays18,64,65 and is either directly used for inoculation (agar-based E-test)65 or further diluted (BMD and state-of-the-art automated Vitek 2),18,66 as detailed in the ESI,† Table S4. By avoiding the additional dilution step and directly using a cell suspension at McFarland 0.5, the assay is simplified and tailored for current clinical procedures and involves minimal handling, which improves reproducibility and allows for high throughput. Also, higher cell densities potentially allow a faster time to growth detection and may better reflect clinically relevant scenarios as microbial infections in the human body are often caused by bacterial biofilms (large number of interacting cells).67
The PRISM assay enables real-time monitoring of bacterial growth by analyzing changes in the reflectance spectra of the photonic chip over time.43,68Fig. 5A-iii shows a characteristic reflectance spectrum at a single time point, which is analyzed to track bacterial growth by monitoring the 2nL value (where n is the refractive index of the medium within the periodic microwells, and L represents the depth of the wells) as detailed in Fig. 1B. The resulting PRISM curves, depicting a continuous change in 2nL vs. time, allow sensitive and quantitative detection of changes in bacterial growth upon exposure to antibiotics (see Fig. 5B-i). The MIC value is defined as the lowest drug concentration at which no increase in the 2nL occurs. Fig. 5B-i displays characteristic PRISM curves of E. coli upon exposure to varying ciprofloxacin concentrations generated on-chip (at a range of 0–1 mg L−1), where the percent changes in 2nL (Δ2nL) are plotted over time. For both no and low antibiotic concentrations (0–0.125 mg L−1), a continuous increase in Δ2nL is observed (slope: ∼0.05 Δ2nL h−1), while at elevated drug concentrations (0.25–0.5 mg L−1), the slope values decrease (∼0.03 Δ2nL h−1), indicative of hindered bacteria growth. At a concentration of 1 mg L−1 ciprofloxacin, the slope remains largely unchanged, and as such, the PRISM MIC value is determined to be 1 mg L−1. Fig. 5B-ii summarizes the attained Δ2nL values after 90 min from three independent PRISM experiments, and statistical analysis (t-test) reveals significant differences between the MIC and subinhibitory concentrations within 90 min.
Note also that the MIC determined by this on-chip PRISM assay is higher compared to reference methods (see Table 2), including both BMD and the automated Vitek 2 test. This deviation is mainly attributed to differences in the inoculum size, where larger initial inoculum is shown to result in higher MIC values for many antibiotics,69 including ciprofloxacin.70–72 For example, Davey and Barza demonstrated that increasing the inoculum from 105 to 107 CFU mL−1 results in a 4 to 32-fold higher MIC value for E. coli and ciprofloxacin depending on the tested strain.71 We demonstrate that this effect is also apparent for the BMD (Table 2); when changing the cell density from 5 × 105 cells mL−1 to ∼108 (McFarland 0.5), the MIC value increases from 0.016 to 0.25–0.5 mg L−1.
Table 2 MIC value for E. coli and ciprofloxacin obtained from the GG-based PRISM assay compared to other (reference) AST methods
Method |
Inoculum |
MIC |
Time |
The reduced assay time of 90 min compared to 2–3 h is ascribed to the higher cell concentration (shift from 107 cells mL−1 to ∼108 cells mL−1), which facilitates a more rapid signal detection.
Value retrieved from our previous work.43
|
PRISM (gradient generator) |
McF. 0.5 (∼108 cells mL−1) |
1 mg L−1 |
90 mina |
Non-gradient PRISM (aluminum flow cell) |
107 cells mL−1 |
0.05 mg L−1b |
2–3 ha,b |
Vitek 2 |
∼107 cells mL−1 |
≤0.25 mg L−1b |
8 hb |
BMD |
5 × 105 cells mL−1 (used in reference BMD) |
0.016 mg L−1 |
18 h |
BMD |
McF. 0.5 (∼108 cells mL−1) |
0.25–0.5 mg L−1 |
18 h |
Yet, the obtained MIC for the GG-enabled PRISM assay is ∼two-fold higher (1 mg L−1) in comparison to the latter values; this is ascribed to the obvious differences in the growth environment. While the BMD analyzes bacterial growth in a liquid medium, the PRISM assay monitors bacteria behavior on and within the silicon microtopologies – which, in fact, may reflect more clinically-relevant scenarios since an estimated ∼80% of chronic and recurrent microbial infections in the human body are due to bacterial biofilms.67 Thus, the higher MIC values did not impair the functionality of the assay, and we suggest calibrating our system for important pathogen-drug combinations with strains that are known to be susceptible or resistant. Isolates with unknown behavior could be classified in accordance with their MIC into “resistant” and “susceptible” categories while still benefitting from the profoundly accelerated (≤90 min) MIC determination at higher cell densities. It should be noted that such calibration is required for all AST methods, including the gold-standard BMD,73 as well as the automated systems, such as the Vitek 2.74
In comparison to other recently developed microfluidic AST systems, which often still require several hours for completion,9,10,22,24,28 our GG-based PRISM assay enables rapid analysis within 90 min with minimal sample handling. It is equivalent or inferior in terms of assay time to some microfluidic AST methods relying on sophisticated single-cell analyses.13,75,76 For example, single-cell imaging of bacteria entrapped in microfluidic channels allowed to differentiate between resistant and susceptible isolates by monitoring their growth at breakpoint antibiotic concentrations within only 30 min.26 Indeed, working at the single cell level allows to detect changes at high resolution (e.g., in the cell morphology) in short timescales, in contrast to phenotypic tests based on detecting bulk bacterial growth. Yet, it is it controversial whether the behavior of single immobilized or confined (in a microchannel) cells is representative of a bacterial population,76 and as such how many cells should be analyzed.75 In contrast to the latter methods, the PRISM signal represents the averaged behavior of a large population of cells, colonizing on the chip that are free to move, interact and form a community. Moreover, the method is label-free and does not require additional reagents as many microfluidic-assisted phenotypic AST techniques e.g., resazurin for metabolic analysis,28,75,76 and it employs a simple and portable optical setup, which can be further miniaturized.
Thus, while assay time is a critical parameter for AST, there are many considerations that should be carefully weighed when designing a clinically applicable AST technique for point-of-care settings.
Conclusions
In this paper, we demonstrate a 3D-printed microfluidic gradient generator that is suitable for rapid and label-free phenotypic AST using integrated photonic silicon chips. Both the performance and accuracy of this GG device were comprehensively assessed and confirmed using several different model fluids, and GG-enabled BMD testing was conducted using three different pathogen-drug combinations.
For rapid optical AST, the bacteria were colonized on microstructured photonic silicon chips – which, when integrated into the GG-system, also provided the optical transducer elements. Bacterial growth at defined antibiotic concentrations was monitored in real-time by observing changes in the reflectivity spectra collected from the photonic silicon chips. Using the GG-based PRISM assay and E. coli and ciprofloxacin as a model pathogen-drug combination, we demonstrated that MIC value determination is feasible within 90 min. Therefore, this assay is significantly faster than the current gold standard BMD and classical agar-based methods (≥16 h), and we believe that it paves a clear path toward more convenient and expeditious point-of-care AST procedures.
To the best of our knowledge, this is the first demonstration of a microfluidic GG-device that automatically creates desired and clinically relevant two-fold antimicrobial dilutions integrated with an optical sensing element for label-free phenotypic monitoring of bacterial behavior. While this assay was demonstrated for E. coli and ciprofloxacin, we envision that it could potentially be extended to a number of other species and antimicrobials as well – since we have shown that AST of different pathogen-drug combinations on these photonic silicon chips is feasible.43,44
We acknowledge that the MIC value obtained from the GG-based PRISM assay is higher than that obtained using reference methods, and also that calibration of our system will be required to guide clinical treatment decisions. Accordingly, future research on calibrating our platform with pathogens of known resistant or susceptible behavior will be required. In the future, however, we envision that GG design will be flexibly adjusted (i.e., via rapid prototyping achieved through 3D printing) for other gradients (e.g., linear) as well as through the integration of different sensing elements. This would facilitate rapid characterization of various (bio)sensors – in terms of both binding affinities and performance – under varying conditions, including different buffer compositions and target concentrations.
Author contributions
C. H. and J.-A. P. contributed equally. Conceptualization: C. H. and J.-A. P.; methodology: C. H., J.-A. P., and M. B.; investigation: C. H., J.-A. P., and M. B.; data curation: C. H.; writing-original draft preparation: J.-A. P. and C. H.; writing-review and editing: C. H., J.-A. P., T. S., E. S., and J. B.; visualization: C. H. and J.-A. P.; supervision: E. S., T. S., and J. B.; project administration: E. S. and J. B.; funding acquisition: J. B., T. S. and E. S.
Conflicts of interest
There are no conflicts to declare.
Acknowledgements
This research was funded by the German Research Foundation (DFG) via the Emmy Noether program (project ID 346772917), by the VolkswagenStiftung via the program “Niedersächsisches Vorab: Research Cooperation Lower Saxony—Israel”, and by the DFG via the grant SCHE 279/32-2. We also wish to thank Dima Peselev and Orna Ternyak of the Technion's at the micro- and nano-fabrication and printing unit for the microfabrication of the photonic silicon chips. Part of the cartoons in Fig. 1 and 4 were obtained from https://BioRender.com under a publishing license. The open access publication of this article was supported by the DFG sponsored Open Access Fund of the University of Augsburg.
References
- T. K. Burki, Lancet Respir. Med., 2018, 6, 668 CrossRef.
- D. A. Gray and M. Wenzel, ACS Infect. Dis., 2020, 6, 1346–1385 CrossRef CAS.
- Antimicrobial Resistance Collaborators, Lancet, 2022, 399, 629–655 CrossRef.
- Interagency Coordination Group on Antimicrobial Resistance, No time to wait: Securing the future from drug-resistant infections. Report to the secretary-general of the United Nations, https://www.who.int/docs/default-source/documents/no-time-to-wait-securing-the-future-from-drug-resistant-infections-en.pdfsfvrsn=5b424d7_6, (accessed 17 June 2022).
- S. Doron and L. E. Davidson, Mayo Clin. Proc., 2011, 86, 1113–1123 CrossRef.
- J. Carlet, V. Jarlier, S. Harbarth, A. Voss, H. Goossens and D. Pittet, Antimicrob. Resist. Infect. Control, 2012, 1, 1–13 CrossRef.
- M. DiCicco and S. Neethirajan, BioChip J., 2014, 8, 282–288 CrossRef CAS.
- H. Shi, Z. Hou, Y. Zhao, K. Nie, B. Dong, L. Chao, S. Shang, M. Long and Z. Liu, Chem. Eng. J., 2019, 359, 1327–1338 CrossRef CAS.
- W. Zeng, P. Chen, S. Li, Q. Sha, P. Li, X. Zeng, X. Feng, W. Du and B.-F. Liu, Biosens. Bioelectron., 2022, 205, 114100 CrossRef CAS PubMed.
- Y. Im, S. Kim, J. Park, H. J. Sung and J. S. Jeon, Lab Chip, 2021, 21, 3449–3457 RSC.
- E. Sweet, B. Yang, J. Chen, R. Vickerman, Y. Lin, A. Long, E. Jacobs, T. Wu, C. Mercier, R. Jew, Y. Attal, S. Liu, A. Chang and L. Lin, Microsyst. Nanoeng., 2020, 6, 92 CrossRef PubMed.
- S. C. Kim, S. Cestellos-Blanco, K. Inoue and R. N. Zare, Antibiotics, 2015, 4, 455–466 CrossRef PubMed.
- H. Leonard, R. Colodner, S. Halachmi and E. Segal, ACS Sens., 2018, 3, 2202–2217 CrossRef CAS PubMed.
- C. Heuer, J. Bahnemann, T. Scheper and E. Segal, Small Methods, 2021, 5, 2100713 CrossRef CAS.
- A. van Belkum, C. A. D. Burnham, J. W. A. Rossen, F. Mallard, O. Rochas and W. M. Dunne, Nat. Rev. Microbiol., 2020, 18, 299–311 CrossRef CAS PubMed.
- EUCAST, EUCAST definitive document E.DEF 7.3.2 Method for the determination of broth dilution minimum inhibitory concentrations of antifungal agents for yeasts, https://www.eucast.org/fileadmin/src/media/PDFs/EUCAST_files/AFST/Files/EUCAST_E_Def_7.3.2_Yeast_testing_definitive_revised_2020.pdf, (accessed 17 June 2022).
- EUCAST, EUCAST disk diffusion method for antimicrobial susceptibility testing, https://www.eucast.org/fileadmin/src/media/PDFs/EUCAST_files/Disk_test_documents/2022_manuals/Manual_v_10.0_EUCAST_Disk_Test_2022.pdf, (accessed 17 June 2022).
- International Organization for Standardization, ISO 20776-1:2020.
- CLSI, M100Ed32 | Performance Standards for Antimicrobial Susceptibility Testing, 32nd Edition, https://clsi.org/standards/products/microbiology/documents/m100/, (accessed 17 June 2022).
- N. Qin, P. Zhao, E. A. Ho, G. M. Xin and C. L. Ren, ACS Sens., 2021, 6, 3–21 CrossRef CAS.
- Z. A. Khan, M. F. Siddiqui and S. Park, Biotechnol. Lett., 2019, 41, 221–230 CrossRef CAS PubMed.
- L. Y. Ma, M. Petersen and X. N. Lu, Appl. Environ. Microbiol., 2020, 86, e00096-20 CrossRef.
- Y. Liu, T. Lehnert, T. Mayr and M. A. M. Gijs, Lab Chip, 2021, 21, 3520–3531 RSC.
- W. T. Qiu and S. Nagl, ACS Sens., 2021, 6, 1147–1156 CrossRef CAS.
- D. C. Spencer, T. F. Paton, K. T. Mulroney, T. J. J. Inglis, J. M. Sutton and H. Morgan, Nat. Commun., 2020, 11, 5328 CrossRef CAS PubMed.
- Ö. Baltekin, A. Boucharin, E. Tano, D. I. Andersson and J. Elf, Proc. Natl. Acad. Sci. U. S. A., 2017, 114, 9170–9175 CrossRef.
- K. W. Chang, H. W. Cheng, J. Shiue, J. K. Wang, Y. L. Wang and N. T. Huang, Anal. Chem., 2019, 91, 10988–10995 CrossRef CAS.
- J. Avesar, D. Rosenfeld, M. Truman-Rosentsvit, T. Ben-Arye, Y. Geffen, M. Bercovici and S. Levenberg, Proc. Natl. Acad. Sci. U. S. A., 2017, 114, E5787–E5795 CrossRef CAS PubMed.
- K. Churski, T. S. Kaminski, S. Jakiela, W. Kamysz, W. Baranska-Rybak, D. B. Weibel and P. Garstecki, Lab Chip, 2012, 12, 1629–1637 RSC.
- M. Azizi, A. V. Nguyen, B. Dogan, S. Zhang, K. W. Simpson and A. Abbaspourrad, ACS Appl. Mater. Interfaces, 2021, 13, 19581–19592 CrossRef CAS PubMed.
- M. Azizi, B. Davaji, A. V. Nguyen, S. Zhang, B. Dogan, K. W. Simpson and A. Abbaspourrad, ACS Sens., 2021, 6, 1560–1571 CrossRef CAS.
- M. H. Tang, X. Y. Huang, Q. Chu, X. H. Ning, Y. Y. Wang, S. K. Kong, X. P. Zhang, G. H. Wang and H. P. Ho, Lab Chip, 2018, 18, 1452–1460 RSC.
- J. M. Cabaleiro, Chem. Eng. J., 2020, 382, 122742 CrossRef CAS.
- 3D systems, VisiJet M2S-HT90 (MJP), https://www.3dsystems.com/materials/visijet-m2s-ht90-mjp, (accessed 17 June 2022).
- T. Habib, C. Brämer, C. Heuer, J. Ebbecke, S. Beutel and J. Bahnemann, Lab Chip, 2022, 22, 986–993 RSC.
- S. Arshavsky-Graham, A. Enders, S. Ackerman, J. Bahnemann and E. Segal, Microchim. Acta, 2021, 188, 1–12 CrossRef.
- A. Enders, J. A. Preuss and J. Bahnemann, Micromachines, 2021, 12, 1060 CrossRef PubMed.
- S. R. Bazaz, O. Rouhi, M. A. Raoufi, F. Ejeian, M. Asadnia, D. Y. Jin and M. E. Warkiani, Sci. Rep., 2020, 10, 5929 CrossRef.
- P. Panjan, V. Virtanen and A. M. Sesay, Analyst, 2018, 143, 3926–3933 RSC.
- N. Bhattacharjee, A. Urrios, S. Kanga and A. Folch, Lab Chip, 2016, 16, 1720–1742 RSC.
- A. Enders, I. G. Siller, K. Urmann, M. R. Hoffmann and J. Bahnemann, Small, 2019, 15, 1804326 Search PubMed.
- N. P. Macdonald, J. M. Cabot, P. Smejkal, R. M. Guijt, B. Paull and M. C. Breadmore, Anal. Chem., 2017, 89, 3858–3866 CrossRef CAS.
- H. Leonard, S. Halachmi, N. Ben-Dov, O. Nativ and E. Segal, ACS
Nano, 2017, 11, 6167–6177 CrossRef CAS PubMed.
- C. Heuer, H. Leonard, N. Nitzan, A. Lavy-Alperovitch, N. Massad-Ivanir, T. Scheper and E. Segal, ACS Infect. Dis., 2020, 6, 2560–2566 CrossRef CAS PubMed.
- M. H. V. Werts, V. Raimbault, R. Texier-Picard, R. Poizat, O. Français, L. Griscom and J. R. G. Navarro, Lab Chip, 2012, 12, 808–820 RSC.
- K. W. Oh, K. Lee, B. Ahn and E. P. Furlani, Lab Chip, 2012, 12, 515–545 RSC.
- S. Arshavsky Graham, E. Boyko, R. Salama and E. Segal, ACS Sens., 2020, 5, 3058–3069 CrossRef CAS PubMed.
- Y. Zhao, G. Gaur, S. T. Retterer, P. E. Laibinis and S. M. Weiss, Anal. Chem., 2016, 88, 10940–10948 CrossRef CAS PubMed.
- T. M. Squires, R. J. Messinger and S. R. Manalis, Nat. Biotechnol., 2008, 26, 417–426 CrossRef CAS PubMed.
- Roche Custom Biotech, Glucose Bio Test kit for the Cedex Bio Analyzer, https://custombiotech.roche.com/global/en/products/cb/glucose-bio-3810241.html, (accessed 17 June 2022).
- S. N. Muchohi, N. Thuo, J. Karisa, A. Muturi, G. O. Kokwaro and K. Maitland, J. Chromatogr., B, 2011, 879, 146–152 CrossRef CAS.
- A. Vasala, V. P. Hytonen and O. H. Laitinen, Front. Cell. Infect. Microbiol., 2020, 10, 308 CrossRef CAS PubMed.
- S. D. Mahlen, Clin. Microbiol. Rev., 2011, 24, 755–791 CrossRef.
- J. B. Kaper, J. P. Nataro and H. L. T. Mobley, Nat. Rev. Microbiol., 2004, 2, 123–140 CrossRef CAS PubMed.
- L. M. Fisher, J. M. Lawrence, I. C. Josty, R. Hopewell, E. E. C. Margerrison and M. E. Cullen, Am. J. Med., 1989, 87, S2–S8 CrossRef.
- K. M. Krause, A. W. Serio, T. R. Kane and L. E. Connolly, Cold Spring Harbor Perspect. Med., 2016, 6, a027029 CrossRef PubMed.
- A. S. Sultan, T. Villa, D. Montelongo-Jauregui and M. A. Jabra-Rizk, Clin. Infect. Dis., 2021, 72, 178–179 Search PubMed.
- B. L. Wickes, J. Clin. Microbiol., 2020, 58, e02083-19 CrossRef PubMed.
- L. B. Johnson and C. A. Kauffman, Clin. Infect. Dis., 2003, 36, 630–637 CrossRef CAS PubMed.
- EUCAST, EUCAST wild-type distribution, https://mic.eucast.org/search/?search%5Bmethod%5D=mic&search%5Bantibiotic%5D=-1&search%5Bspecies%5D=261&search%5Bdisk_content%5D=-1&search%5Blimit%5D=50, (accessed 17 June 2022).
- T. A. Russo and J. R. Johnson, Microbes Infect., 2003, 5, 449–456 CrossRef.
- Z. Peng, Z. Hu, Z. Li, X. Zhang, C. Jia, T. Li, M. Dai, C. Tan, Z. Xu, B. Wu, H. Chen and X. Wang, Nat. Commun., 2022, 13, 1116 CrossRef CAS PubMed.
- A. McNally, T. Kallonen, C. Connor, K. Abudahab, D. M. Aanensen, C. Horner, S. J. Peacock, J. Parkhill, N. J. Croucher and J. Corander, MBio, 2019, 10, e00644-19 CrossRef PubMed.
- E. A. Idelevich, I. Schüle, B. Grünastel, J. Wüllenweber, G. Peters and K. Becker, J. Clin. Microbiol., 2014, 52, 4058–4062 CrossRef.
- bioMérieux, Etest Application Guide, https://www.biomerieux-usa.com/sites/subsidiary_us/files/supplementary_inserts_-_16273_-_b_-_en_-_eag_-_etest_application_guide-3.pdf, (accessed 17 June 2022).
- M. Ligozzi, C. Bernini, M. G. Bonora, M. de Fatima, J. Zuliani and R. Fontana, J. Clin. Microbiol., 2002, 40, 1681–1686 CrossRef CAS PubMed.
- D. Sharma, L. Misba and A. U. Khan, Antimicrob. Resist. Infect. Control, 2019, 8, 1–10 CrossRef.
- H. Leonard, X. Jiang, S. Arshavsky-Graham, L. Holtzman, Y. Haimov, D. Weizman, S. Halachmi and E. Segal, Nanoscale Horiz., 2022, 7, 729–742 RSC.
- I. Brook, Rev. Infect. Dis., 1989, 11, 361–368 CrossRef CAS PubMed.
- I. Morrissey and J. T. George, J. Antimicrob. Chemother., 1999, 43, 423–425 CrossRef CAS.
- P. G. Davey and M. Barza, J. Antimicrob. Chemother., 1987, 20, 639–644 CrossRef CAS PubMed.
- R. J. Fass, Antimicrob. Agents Chemother., 1983, 24, 568–574 CrossRef CAS PubMed.
- EUCAST, Clinical breakpoints - breakpoints and guidance, https://www.eucast.org/clinical_breakpoints/, (accessed 17 June 2022).
- bioMérieux, How does VITEK® 2 Generate MIC Values? - BIOMÉRIEUX iNEWS, https://www.biomerieux-microbio.com/how-does-vitek-2-generate-mic-values/, (accessed 17 June 2022).
- W. Postek, N. Pacocha and P. Garstecki, Lab Chip, 2022, 22, 3637–3662 RSC.
- K. Syal, M. N. Mo, H. Yu, R. Iriya, W. W. Jing, G. Sui, S. P. Wang, T. E. Grys, S. E. Haydel and N. J. Tao, Theranostics, 2017, 7, 1795–1805 CrossRef CAS.
Footnotes |
† Electronic supplementary information (ESI) available. See DOI: https://doi.org/10.1039/d2lc00640e |
‡ Christopher Heuer and John-Alexander Preuss contributed equally to this work. |
|
This journal is © The Royal Society of Chemistry 2022 |