DOI:
10.1039/D2LC00276K
(Paper)
Lab Chip, 2022,
22, 2853-2868
Application of a gut–liver-on-a-chip device and mechanistic modelling to the quantitative in vitro pharmacokinetic study of mycophenolate mofetil†
Received
25th March 2022
, Accepted 16th June 2022
First published on 14th July 2022
Abstract
Microphysiological systems (MPS) consisting of multiple linked organ-on-a-chip (OoC) components are highly promising tools with potential to provide more relevant in vitro to in vivo translation of drug disposition, efficacy and toxicity. A gut–liver OoC system was employed with Caco2 cells in co-culture with HT29 cells in the intestinal compartment and single donor primary hepatocytes in the hepatic compartment for the investigation of intestinal permeability, metabolism (intestinal and hepatic) and potential interplay of those processes. The prodrug mycophenolate mofetil was tested for quantitative evaluation of the gut–liver OoC due to the contribution of both gut and liver in its metabolism. Conversion of mycophenolate mofetil to active drug mycophenolic acid and further metabolism to a glucuronide metabolite was assessed over time in the gut apical, gut basolateral and liver compartments. Mechanistic modelling of experimental data was performed to estimate clearance and permeability parameters for the prodrug, active drug and glucuronide metabolite. Integration of gut–liver OoC data with in silico modelling allowed investigation of the complex combination of intestinal and hepatic processes, which is not possible with standard single tissue in vitro systems. A comprehensive evaluation of the mechanistic model, including structural model and parameter identifiability and global sensitivity analysis, enabled a robust experimental design and estimation of in vitro pharmacokinetic parameters. We propose that similar methodologies may be applied to other multi-organ microphysiological systems used for drug metabolism studies or wherever quantitative knowledge of changing drug concentration with time enables better understanding of biological effect.
Introduction
One of the challenges during pre-clinical drug development is the prediction of human pharmacokinetics (PK), which is required for selection of the best drug candidates and the planning of clinical studies. Although animal models are routinely used in drug development, they are often not predictive of human PK.1 Simple in vitro systems such as subcellular fractions (e.g. human liver microsomes) or suspended hepatocytes are valuable for screening large numbers of compounds and selecting the most promising candidates, but they do not always represent the in vivo condition well (e.g. enzymatic expression).2,3 Therefore, there is a great interest in the development of more human-relevant in vitro systems which produce more accurate predictions and may replace or reduce the use of animal models in pre-clinical studies.4 Microphysiological systems (MPSs) include media flow which promotes cell polarization and a more mature cellular phenotype.5 Another important benefit of MPS is the prolonged cell viability which permits extended incubations.6 For example, Rubiano et al. recently reported viability of more than 18 days using a liver-on-a chip device.7 Long term incubations enable measurement of the biotransformation of metabolically stable compounds and metabolite formation. In contrast to traditional in vitro systems, MPS may have multiple cell cultures connected via media flow, enabling assessment of drug metabolism, transport, pharmacology or toxicity which involves more than one tissue. Gut and liver are the organs that play a major role in drug absorption and metabolism following oral administration. The combination of cell cultures representing these tissues in an MPS format is therefore highly relevant. The first application of a gut–liver MPS was reported by Tsamandouras et al. in 2017.8 That study examined the role of the intestinal compartment as a barrier tissue and also the gut and hepatic metabolism of diclofenac and hydrocortisone. A more recent work by Arakawa et al. used a gut–liver MPS for investigation of triazolam PK.9 In both studies, the authors reported modeling of experimental data to estimate PK parameters, but did not use drugs for which intestinal compartment metabolism played an important role.3,4 The work presented in this paper builds upon these achievements, other MPS work involving prodrugs10,11 and also our recently reported liver-on-a-chip MPS validation work,6 with particular emphasis on the mechanistic study of prodrug absorption and activation in the gut, a highly relevant ADME application for gut–liver MPS.
The purpose of this study was to explore and validate the use of a gut–liver MPS for ADME applications, to further develop our MPS modelling approaches and to show whether the in vivo pharmacokinetics of mycophenolate mofetil (MM) could be predicted from a single, two organ, in vitro MPS. This work extends current knowledge and approaches especially in the following areas: 1) the accurate quantification of the intestinal cells and hepatocytes at the end of the experiment in order to scale the PK parameters; 2) the application of detailed modelling to understand and account for the impact of fundamental system parameters (e.g. cell volume, media flow rate) on the PK estimation; 3) the use of in silico modelling to learn from a number of preliminary experiments and to guide experimental design to enable accurate PK parameter estimation. We selected the prodrug mycophenolate mofetil (MM) as a compound that is biotransformed in both gut and liver to form a limited number of well-characterized metabolites of quite different physicochemical properties for which the reference standards are commercially available (Fig. 2).
We tested a prototype ‘Physiomimix’ gut–liver-on-a-chip (gut–liver-OoC) device that is based upon the previously characterized ‘Physiomimix’ liver-on-a-chip device.6 The MPS gut compartment was divided into apical and basolateral sides by a monolayer of cells and the basolateral compartment was linked by an interconnection flow to the liver compartment (Fig. 1). In contrast to standard in vitro systems, the MPS provided media flow to ensure mixing in the gut basolateral compartment without the need for agitation. The gut tissue was represented by a co-culture of colon carcinoma Caco2 and HT29 cells which provided both barrier tissue and metabolic functions. HT29 cells are intestinal derived, mucus-producing cells that provide an additional diffusion barrier. The aim of using this co-culture was to better reproduce the barrier function of the small intestine which contains more than 8 different cells types including absorptive enterocytes and mucus secreting cells.12–16 Therefore, HT29 cells in combination with Caco2 may enable better reproduction of the physiological barrier in the small intestine and thus more relevant intestinal permeability and metabolism.12,17 Following absorption and passage to the basolateral side of the gut, drug was circulated through a system containing cultured primary hepatocytes. In the liver compartment, a flow system distributed drug and media and provided shear stress to the hepatocytes which helped in maintenance of cell viability and phenotype, as reported previously.6,7,18
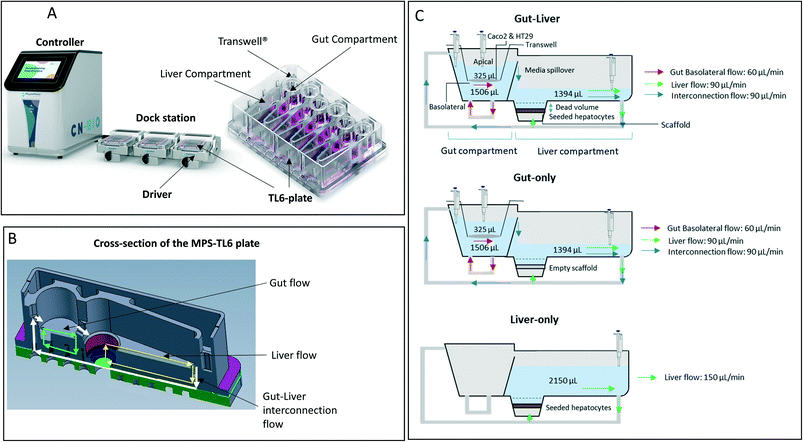 |
| Fig. 1 Representation of the PhysioMimix gut–liver MPS. A: The system is constituted by a controller machine with a system of pumps and a touchscreen to interact with the user. The other components placed into the incubator are the dock station, driver, and TL6 plate. B: Major portions of the system: gut compartment with a transwell which separates the apical and basolateral side of the intestinal system and liver compartment where the hepatocytes are seeded. Three different flow systems can be controlled independently: gut basolateral compartment circulation, liver compartment circulation, and interconnection flow. C: Graphical representation of the well, media flow, and sampling points for all three experimental systems. Initial incubation volumes for each compartment. In the gut–liver and gut-only systems, the well was in the same condition except for the empty scaffold in the gut-only system. In the liver-only system, the gut compartment was not filled with media, there was no basolateral or interconnection media flow and the transwell in the gut compartment was not used. Note; this picture is only a schematic representation of the well in each experimental system, without any intent to report the size on an exact scale. | |
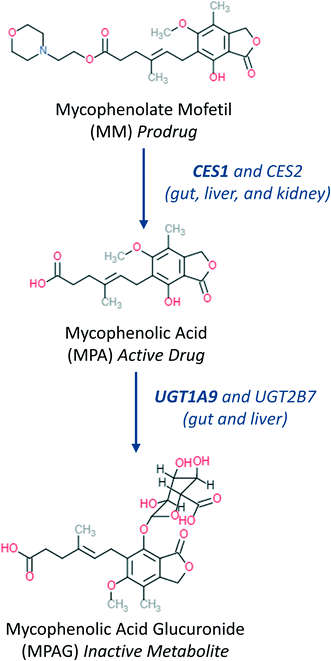 |
| Fig. 2 Main metabolic steps37 of mycophenolate mofetil activation and further metabolism showing main organs and enzymes involved in the metabolic transformation. | |
Materials and methods
Materials
Mycophenolate mofetil, mycophenolic acid and TRITON X 100 were obtained from Sigma-Aldrich (St. Louis, Missouri, US). Mycophenolic acid β-D-glucuronide was obtained from Toronto Research Chemicals (Toronto, Ontario, Canada). PBS, cryopreserved hepatocyte recovery medium, William's E medium, primary hepatocyte plating supplements (solution of FBS, dexamethasone, cocktail-A having penicillin–streptomycin, human recombinant insulin, GlutaMAX™, and HEPES), primary hepatocyte maintenance supplements having dexamethasone and cocktail-B [penicillin–streptomycin, ITS+ (insulin, transferrin, selenium complex), bovine serum albumin (BSA), linoleic acid, GlutaMAX™, and HEPES, penicillin/streptomycin], Dubeccos modified eagle medium (DMEM) high glucose with glutamine, non-essential amino acids, Pierce™ BCA Protein Assay Kit, DAPI (4′,6-diamidino-2-phenylindole) solutions, and phalloidin were purchased from Thermo Fisher Scientific (Waltham, Massachusetts, US). Human hepatocytes (lot: RAS) were purchased from BioIVT (Royston, United Kingdom). Caco2 cells co-cultured with HT29 cells were purchased from ReadyCells (Barcelona, Spain) as pre-seeded in 6.5 mm diameter transwells. All Physiomimix gut–liver on a chip components (controller, docking station and drivers) and consumables (TL-6 plates) were provided by CN Bio Innovations (Cambridge, UK).
Media preparation
The plating medium for the hepatocytes was prepared in William's E medium by adding 5% of fetal bovine serum (FBS), 3.6% of cocktail A, and dexamethasone (final concentration 1 μM). The maintenance medium was prepared in William's E medium by adding 4% of Cocktail B. The Caco2 cell differentiation medium was prepared by dissolving 1% of a solution of penicillin/streptomycin, FBS 10%, and 1% non-essential amino acids in DMEM high glucose with glutamine. The Caco2 cells maintenance medium was prepared dissolving 1% of a solution of penicillin/streptomycin, 1% non-essential amino acids, 1% insulin transferrin solution in DMEM High glucose with glutamine. All fresh media were prepared and micro-filtered before the start of the experiment.
Biological systems in the gut and liver MPS compartments
The intestinal cells used in the experiment were purchased already seeded in a co-culture of Caco2 (immortalized cell line of human colorectal adenocarcinoma cells that expressed CESs19 and UGTs20) and HT29 cells (human colorectal adenocarcinoma cell line with epithelial morphology that also expressed UGTs21) in transwells contained in a 24 well-plate. The cell density ranged between 150
000–180
000 cells/well. Cryopreserved hepatocytes were seeded in the scaffold in the liver compartment as reported previously.6 A detailed description of the system preparation and incubation methods is provided in the ESI.†
Gut–liver-OoC platform description
The multi-organ prototype employed in this work was similar to the CN Bio PhysioMimix system used for liver-on-chip investigations and recently reported by Rubiano et al.7 The system consisted of a controller with an electro-pneumatic system that regulated the media flow in the MPS plates (Fig. 1-A). The experiments were performed with a special plate (TL6) which contained 6 independent incubation chambers. During the experiment, each plate was attached to a dedicated support platform (‘driver’). The driver and the plates were placed inside a 37 °C 5% CO2 atmosphere incubator and attached to the docking station which communicated with the controller by pneumatic and electrical umbilical connector. Each incubation chamber (hereafter referred to as ‘well’ for simplicity) contained a 6.5 mm diameter transwell® with a 0.33 cm2 membrane which was placed in the gut compartment. The transwell created a compartmental separation of the intestinal compartment with independently accessible apical and basolateral sides. In the liver compartment the hepatocytes were seeded on a plastic scaffold having microscopic holes for media flow. The gut basolateral side and the liver chamber had an independent circulating flow system, which provided media flow. In addition to the gut and liver flow systems, an interconnection pump delivered media from the liver chamber into the basolateral compartment (Fig. 1-B). A passive fluidic (overspill) connection flowed from the gut basolateral compartment into the liver compartment (Fig. 1-B). In the gut–liver and gut-only systems the media flowed from the liver to the gut compartment and vice versa: whereas in the liver-only system the interconnection pump was not active since the media was added only in the liver compartment. Exploration of media flow induced shear stress and its effect on hepatocytes was not performed in this work and media flow rates were used as per manufacturers recommendations. The experiment was conducted in three different experimental systems which are described below and schematically reported in Fig. 1-C. The detailed preparation of the plate before the start of the incubation is reported in the ESI.†
Gut–liver experiments.
In this system, both intestinal and hepatic cells were simultaneously included on the chip. The Caco2-cell maintenance media volume at the start of the incubation in the gut apical compartment was 325 μL containing 10 μM mycophenolate mofetil. The total hepatocyte maintenance media volumes in the basolateral side and in the liver compartments were 1506 and 1394 μL, respectively (Fig. 1-C). The volume in the basolateral compartment was essentially constant due to the continuous inflow from the liver compartment and an overspill mechanism for return flow. The interconnection flow was set at 90 μL min−1 which ensured rapid replenishment of the basolateral compartment volume and media circulation within a few seconds following sample collection. The evaporation of the apical media was not measured due to the technical difficulty to recover the residual volume without risking potential damage of the intestinal cells prior to microscopy analysis at the end of the experiment (see ESI†). Although the surface area of the apical compartment is substantially smaller than that of the main liver compartment, it cannot be ruled out that evaporation also occurred here to some extent. The volume in the liver compartment was not constant due to water evaporation from the media and media removal for drug concentration measurements. These changes were taken into account in the data analysis, as reported previously.6 For the entire duration of the incubation the interconnection flow, the basolateral flow and the liver chamber flows were set at 90, 60, and 90 μL min−1, respectively (Fig. 1-C). The media flow rate used in the gut and liver compartment were selected following manufacturers recommendation to guarantee efficient mixing in each compartment. The sampling times in the different compartments are reported in Table 1 and a schematic representation of the plate preparation and incubation is reported in Fig. 3. A detailed description of the incubation protocol is reported in the ESI† in the section S2.
Table 1 Initial drug concentration, compartment volumes, flow rates, and sampling points and their respective compartments for mycophenolate mofetil experiments
|
Gut–liver |
Gut-only |
Liver-only |
Initial concentration (μM) |
Gut apical side |
10 |
10 |
— |
Gut basolateral side |
0 |
0 |
— |
Liver compartment |
0 |
0 |
1 |
Initial volume (μL) |
Gut apical side |
325 |
325 |
— |
Gut basolateral side |
1506 |
1506 |
— |
Liver compartment |
1394 |
1394 |
2150 |
Flow rate (μL min−1) |
Interconnection |
90 |
90 |
— |
Gut basolateral side |
60 |
60 |
— |
Liver chamber |
90 |
90 |
150 |
Sampling times (h) |
Gut apical side |
0, 0.5, 1, 2, 8, 48 |
0, 0.5, 1, 2, 8, 48 |
— |
Gut basolateral side |
0.5, 1, 2, 8, 24, 48 |
0.5, 1, 2, 8, 24, 48 |
— |
Liver compartment |
2, 4, 24, 32, 48 |
2, 4, 24, 32, 48 |
0, 0.5, 1, 2, 8, 24, 32, 48 |
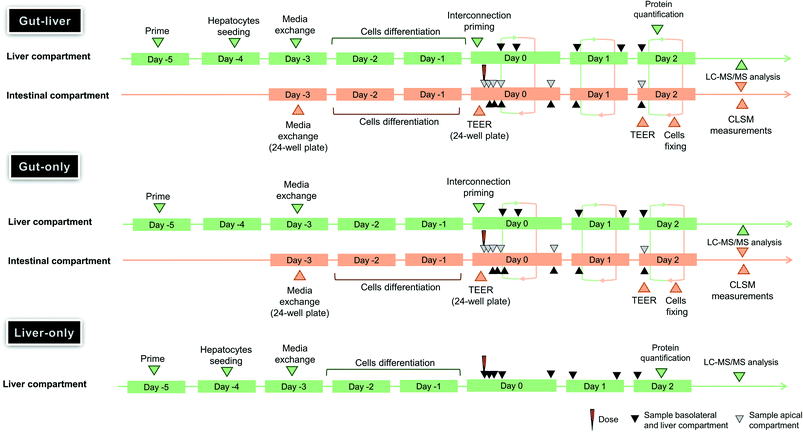 |
| Fig. 3 Timeline and the major events for the assessment of the gut–liver OoC experiment in gut–liver, gut-only, and liver-only systems of mycophenolate mofetil. | |
Gut-only experiment.
In the gut-only experiment (Fig. 3) the same initial 10 μM prodrug concentration, media volumes, flow rates and the sampling times of gut–liver system (Table 1) were applied as in the gut–liver experiments. The only difference was the empty scaffold in the liver compartment which was not seeded with hepatocytes. (Fig. 1-C).
Liver-only experiment.
In the liver-only experiment (Fig. 3) the gut compartment was not used and the entire recirculation flow passed though the hepatocyte culture (Fig. 1-C). The gut compartment remained empty for the entire duration of the experiment and therefore only the recirculating liver compartment pump was used, with a flow rate of 150 μL min−1.
Quantification of drug and metabolite concentrations
Samples of media were removed from each of the compartments of the system at the timepoints specified in Table 1. 25 μL samples were collected and diluted 1
:
5 in a solution of acetonitrile
:
water = 2
:
1. The samples from all compartments (apical, basolateral, and liver chamber) were stored at −20 °C until the end of the experiment when a single sample preparation procedure for the LC-MS/MS analysis was performed. Basolateral and liver samples were centrifuged for 10 minutes at 6300 rpm at 4 °C to remove precipitated BSA and the supernatants collected. The analysis was conducted using liquid chromatography tandem mass spectrometry (LC-MS/MS). The analytical system consisted of a HTS CTC PAL autosampler together with Shimadzu pumps for the HPLC system and a QTRAP 5500 (AB Sciex) equipped with a TurboIonSpray source for the mass spectrometry. The ion acquisition was performed in negative MRM mode for MPA and MPAG and positive MRM mode for MM. Additional details about MRM mode and chromatographic details such as analytical columns, mobile phases, sampling volumes, flow rates, and retention times are reported in the ESI† (Table S3.1).
The quantitative analysis was performed using separate calibration curves for both apical and basolateral media. Dilutions of the samples was performed in order to work in the range of linearity of the calibration curves for all compounds. In addition, an external validation was performed using a mix of all analytes present in the actual samples in their range of linearity. The analytical method was considered robust enough when the 6 control samples of a mixture of analytes at 0.040 μM showed CV% < 5% and accuracy >90%.
Post incubation measurements
Measurement of remaining media volume and calculation of evaporation from the media.
The total residual volume in the plate was measured and the rate of evaporation from the basolateral and liver compartments calculated, assuming a constant rate of evaporation (eqn (1)): | 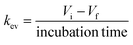 | (1) |
Where kev, Vi and Vf, represented the rate of evaporation, the initial volume, the final volume, respectively.
Measurements of hepatocyte protein amount.
The scaffolds were removed from the plate using the special screwdriver provided by CN Bio and a pair of sharp tweezers. The scaffolds were washed twice in 1000 μL PBS and subsequently placed into 500 μL PBS containing 1% Triton-X. The surface of the scaffold was then thoroughly scratched with a pipette tip to ensure maximal retrieval of cells, and the lysing process was continued for half an hour.6 This process was repeated twice to ensure complete detachment and lysis of the cells. After the scaffold was washed and removed from the cell lysate, total protein concentration was measured with the Pierce™ BCA Protein Assay Kit (Thermo Fisher Scientific), and the incubation cell number calculated using a conversion factor of 1 mg protein per million hepatocytes, as determined previously.6 For the calculations, it was assumed that all attached cells were alive, and that any dead cells were removed from the scaffold during the washing step.
TEER measurement.
Effective barrier function of the intestinal cell monolayer is essential for any gut model system used for drug metabolism applications. Trans-epithelial electrical resistance (TEER) measurements were performed to establish the integrity of the monolayer in the intestinal compartment and enable selection of the best transwells to use in the MPS experiments. Therefore, TEER measurements were performed at the beginning and end of the experiment and the values were compared. The protocol and data from the TEER measurements are described in the ESI† section 4. The TEER values of the selected transwells decreased over the course of the experiment, from ∼300 Ωcm2 initially to ∼180 Ωcm2 at the end of the experiment. Despite this decrease of approximately 40%, TEER was significantly above the manufacturer proposed threshold of 70 Ωcm2 confirming integrity of the monolayer and barrier function of the Caco2 and HT29 co-culture throughout the incubations (Fig. 5-B). As reported in literature, co-culture of Caco2 and HT29 cells reduced the TEER value compared to that from the Caco2 mono-culture.22 Indeed, the use of Caco2 and HT29 cells in co-culture permitted to modify the permeability barrier of the cell monolayers both with respect to paracellular resistance and transport mechanism compared to the singular Caco2 cell monolayer.23 This has been reported to improve the in vivo translatability of the in vitro data and the delivery of a robust experimental methods which are able to provide reproducible results.14,23 In addition to the TEER measurements, imaging of the wells containing the intestinal cells at the end of the experiment was performed using the method reported in the ESI† (section S5 and Fig. S5.1).
Measurement of unbound fraction in the basolateral medium
The fu,inc (fraction unbound in the incubation media) values were determined in the maintenance medium using equilibrium dialysis against 133 mM phosphate buffer at pH 7.4, over 5 hours at 37 °C in a 5% CO2 atmosphere, using a Teflon equilibrium dialysis plate (96-well, 150 μL, half-cell capacity) and cellulose membranes (12–14 kDa molecular weight cut-off) from HT-dialysis (Gales Ferry, Connecticut). The dialysis plate was assembled by placing conditioned membranes between rows of half-wells and tightly clamping the assembled apparatus. Aliquots from the donor and receiver compartments were combined with buffer or blank media to make matrix-matched samples (10% (v/v) media), which were further prepared by the addition of acetonitrile-containing internal standard. Drug concentrations in the samples were determined by LC-MS/MS. Recovery of 80% to 120% and internal standard peak area CV < 20% was required for data acceptance.
Mathematical modelling of in vitro PK data
Development of mechanistic gut–liver OoC model.
Concentration-time profiles for mycophenolate mofetil and its metabolites in all three systems were modelled using a compartmental approach based on ordinary differential equations (ODEs). Four compartments were used for MM, MPA, and MPAG to represent i) apical gut side, ii) intestinal cells, iii) basolateral gut side and iv) liver compartment. Only the concentration in the apical compartment was described in the model for the prodrug since all samples from the other compartments were below the limit of quantification. In the liver-only system, solely the liver compartment was used since the gut compartment was not needed. The models considered the concentrations of all compounds within a compartment to be homogenous (well mixed compartments due to the presence of flow). Based on the data from pilot experiments which showed reproducible intestinal and hepatic cells viability and activity, different models were generated (ESI† in section S6 and Table S6.1) including the most relevant drug metabolism and pharmacokinetic (DMPK) processes for mycophenolate mofetil and its metabolites (data not shown). Identification of the best model was performed by assessment of the goodness of fit based on the Bayesian information criterion (BIC) from several potential candidate models. Model evaluation is reported in section S6 in the ESI,† including the input parameters used in the simulations reported in Table S6.2 and results in Fig. S6.1–6 and Tables S6.1–4.† A lower value of BIC corresponds to a better description of the experimental data. For instance, the model investigated the effect of the processes involved in the drug passage through the intestinal cells which are described by the apparent permeability (Papp) and the efflux ratio (Er). Papp is defined as the permeability of a compound across a cellular membrane normalised by membrane surface area and donor concentration and the Er indicates the presence of active uptake or efflux of the drug, which may have a relevant role in drug absorption and potential for drug–drug interactions.24 The Er having a value > 1 indicates the presence of efflux (i.e. the drug moves from the cell to the lumen) and Er < 1 indicates the active uptake of the drug from the lumen to the cells. For the final models in each of the three experimental systems, the total number of unknown parameters were 7 in the gut–liver system CLapp,gut,u (MM), CLint,gut,u (MPA), CLapp,hep,u (MPA), Papp (MPA), Papp (MPAG), Er (MPA), and Er (MPAG), 6 in the gut-only [as gut–liver but without CLapp,hep,u (MPA)], and 2 for liver only [CLapp,hep,u (MM) and CLapp,hep,u (MPA)].
Intestinal metabolism of MM and hepatic metabolism of MM and MPA were rapid and exceeded permeability of drug into the cells.25 The potential for active efflux (e.g. biliary elimination) of MPAG25 was not explored as the MPS did not have this capability.
System-related features of the mechanistic model.
In addition to the metabolic transformation processes the mechanistic models of the in vitro experiments needed to incorporate MPS specific parameters. These include media and cellular compartment volumes, number of cells and surface area of the transwell. A list of parameters with the respective values is shown in Table 2. Changes in the media volumes in the liver and apical side compartments over time due to media sampling and evaporation were important aspects of the MPS that needed to be captured when modelling the system (Fig. 5-C). The media volume in each compartment throughout the experiment was calculated using eqn (2), in accordance with previous work.6 | Vc(t) = Vi,c − kev·t − nsc(t)·Vs | (2) |
Where Vc(t), Vi,c, nsc, and Vs are the volume at time t, initial volume, the number of samples taken and the volume of sampling in each compartment c, respectively. In addition to the removal of medium (25 μL) removal of drug substance at the time of sampling needed to be considered (Fig. S6.2†). The apical side was considered to be not affected by media evaporation, hence kev was set to 0. Sampling from the basolateral side was compensated for by a rapid replenishment of removed volume from the liver compartment. Although the interconnection flow was interrupted for about 2 min at each sampling time, it was demonstrated by in silico modelling that this did not impact the time vs. concentration profile of the compounds. Details of the model simplifications are reported in the ESI.† The measured input (e.g. number of intestinal and hepatic cells and the constant of evaporation of each well) and system-related input parameters (e.g. Qi and initial compartmental volumes) are reported in Table 2. In order to obtain PK parameters for unbound drug, the ODEs included the experimentally measured unbound fraction (fuinc) of the prodrug and the metabolites in the basolateral media.
Table 2 Input parameters used in the mechanistic model fitting in all three experimental systems. The respective values for the number of hepatocytes and kev for each well were reported and separated by comma for all three experimental systems. In the apical media (free of fetal bovine serum) the fuinc was 1. The fuinc (MM) was used in the liver-only system since the prodrug was directly dosed in the basolateral media
Parameter feature |
Parameter |
Units |
Value |
|
Gut–liver |
Gut-only |
Liver-only |
PK parameter experimentally estimated |
fuinc apical (all compounds) |
|
1 |
— |
fuinc (MM) |
|
— |
0.76 |
fuinc bas (MPA) |
|
0.38 |
— |
fuinc bas (MPAG) |
|
0.70 |
— |
System |
Initial Vapical |
μL |
325 |
— |
Initial Vbasolateral |
μL |
1506 |
— |
Initial Vliver comp. |
μL |
1394 |
2150 |
V intestinal cells |
μL per 106 cells |
2.6 |
|
Surface transwell (SA) |
cm2 |
0.33 |
— |
Interconnection flow (Qi) |
μL min−1 |
90 |
— |
Number intestinal cells (Ne) |
106 cells |
0.45 |
— |
Sample volume (Vs) |
μL |
25 |
Number hepatocytes (Nh) |
106 cells |
0.27, 0.33, 0.30 |
— |
0.38, 0.38, 0.38 |
Media evaporation constant (kev) |
μL min−1 |
0.083, 0.10, 0.096 |
0.11, 0.088, 0.094 |
0.077, 0.095, 0.11 |
Model structure identifiability.
An a priori identifiability indicates whether the model permits an unique determination of the unknown parameters from the given input and measurement data under ideal conditions of noise-free observations and error-free model structure.26 Due to the assumption of ideal conditions, an a priori identifiability is not sufficient to ensure accurate and quantitative parameter determination. However, if the unknown parameters are not uniquely identifiable in the ideal conditions, they will never be identifiable. Therefore, this analysis is a critical quality check to ensure that the model has the capability to estimate the desired parameters from the combination of experimental and system data. The modelling of a complex system such as gut–liver OoC was based on i) input data (drug ‘dose’ and known parameters), ii) observations (samplings), iii) a certain number of ODEs to describe the biological processes and iv) unknown parameters which need to be estimated. The a priori identifiability was performed in DAISY 2.0 (ref. 26 and 27) and the models of gut–liver, gut-only, and liver-only systems were evaluated. All output parameters were found to be globally identifiable.
Fitting and parameter estimation.
The PK estimation was performed in Phoenix 8.2 (Certara, US) using a Naïve Pool approach which excluded the evaluation of inter-well variability and the Sandwich algorithm was applied to calculate the standard deviation of the estimated PK parameters. In order to better explore the parameter uncertainty and their distribution, log likelihood profiling was also performed for all systems investigated (gut-only, liver-only, liver–gut and simultaneous fitting of all available data). Plots of the fitting (exporting the residuals tables from Phoenix) and simulations were generated in R-3.6.1 (ggplot and gridExtra libraries). Graphical representations of concentration-time profiles were generated in GraphPad Prism version 7.04 for Windows (GraphPad Software, La Jolla, CA).
Impact of the gut cell volume and interconnection flow rate on clearance and distribution parameter estimation.
As with the media compartments, the volume of the cellular compartments needs to be considered, especially the gut cellular compartment in the context of MM metabolism. The amount of drug present in a given compartment is equal to the compartment volume multiplied by the compartment drug concentration. The volume of cells often does not impact the Papp estimation since the drug concentration in the cell is equilibrated with the surrounding media and the drug influx due to permeability greatly exceeds drug removal due to metabolic transformation. However, when the metabolite formation rate exceeds the permeability, the drug becomes concentrated in the cells and the cell volume becomes a relevant model input parameter. It was demonstrated that the volume of cells (Ve) impacted the Papp(MPAG) and therefore the application of the best input parameter was included in the fitting model (section S7 in the ESI† and Fig. S7.1 and Table S7.1). Caco2 and HT29 cell volume date were collected from the literature and values of 2.6 and 2.4 μL per 106 cells obtained, respectively.28,29 The volume used in the fitting was 2.6 μL per 106 cells. Additional details about the cellular volumes and their impact on the time-concentration profiles of the prodrug and its metabolites are reported in the ESI.† The impact of the interconnection flow with its associated uncertainty of 20% was investigated by fitting the model with Qi values of 90 μL min−1, 72 μL min−1, and 108 μL min−1. The increase in Qi impacts the estimation of CLapp which asymptotically approaches Qi; results are reported in Table S7.2 in the ESI.†
Global dynamic sensitivity analysis.
Global dynamic sensitivity analysis was performed using data from gut–liver, gut only and liver only experiments in order to assess whether optimal sampling times were chosen for parameter determination in the gut–liver system. It was performed using Morris's screening algorithm which is a variance-based method30 and using the specific library ODEsensitivity in R which allowed the investigation of the signal of each parameter in every compartment at different times during the incubation. The same model applied for the fitting in the gut–liver system having the sampling time and the evaporation of media was used to perform the sensitivity analysis (additional details about the sensitivity analysis outcomes are reported in the section S8 in the ESI†). The sensitivity analysis was performed using a range of values based on the uncertainty (95% confidence interval) derived from the fitting for each parameter in the gut–liver system.
Results and discussion
In vitro pharmacokinetics of mycophenolate mofetil and its metabolites
Gut–liver.
A combination of carboxylesterases (CESs) and uridine 5′-diphospho-glucuronosyltransferases (UGTs)20,31–33 catalyze prodrug hydrolysis to form the active drug mycophenolic acid (MPA) and subsequent conjugation to the inactive metabolite mycophenolic acid glucuronide (MPAG), respectively (Fig. 2). Caco2 and HT29 cells express CES,19,34 as well as UGT1A9 and UGT2B7 (ref. 20 and 21) enzymes, which are responsible for MPA glucuronidation.35 When mycophenolate mofetil (MM) was administered to the gut apical compartment (10 μM) a rapid reduction in prodrug concentration was observed (only 5% of the initial concentration of the prodrug remained in the apical side after 2 h of incubation, Fig. 4). MM did not reach the basolateral side to any measureable extent (all samples contained <2 nM MM), suggesting rapid hydrolysis by carboxylesterases in the gut cells to form the active drug mycophenolic acid (MPA).19,36 To check for any unspecific hydrolysis the chemical stability of the prodrug was also evaluated in the media without cells. This showed a very slow non-enzymatic contribution (∼85% remaining after 5 h) compared with rates catalyzed by the Caco2-HT29 cells. MPA formed within the cells was able to cross both apical and basolateral membranes of the gut cells. MPA entering the basolateral side was diluted into the larger volume of the basolateral/liver compartment (2900 μL) compared with the apical compartment (325 μL) resulting in low basolateral compartment concentrations. In contrast, relatively high MPA concentrations were initially observed in the apical side which peaked shortly after the start of the experiment (∼2.5 μM after 1 h of incubation) then dropped off as the drug diffused back into the gut cells and into the larger basolateral compartment. MPA was metabolized via glucuronidation in both the intestinal and hepatic cells to form the inactive metabolite MPAG. UGT1A9 and to a minor extent UGT2B7 have been identified as the UGT isoforms involved in MPA glucuronidation.35 MPAG accumulated in the gut cells since its formation was faster than its permeation out of the cells, in line with initial decrease in the total drug amount in all media compartments and subsequent increase later in the incubation (Fig. 4). MPAG was also formed in the hepatocytes and by the end of the incubation (48 h) MPA had been completely converted into MPAG. These data confirmed that the Caco2-HT29 cells can qualitatively recapitulate the metabolism of mycophenolate mofetil and MPA in the small intestine.37,38 Only the combined use of gut and liver cells in one device allowed the investigation of the simultaneous contribution of gut and liver to MPA metabolism. This work illustrates the utility of the further gut–liver OoC for practical use in examining combination of gut and liver metabolism as well as clearance and drug–drug interaction studies in future.
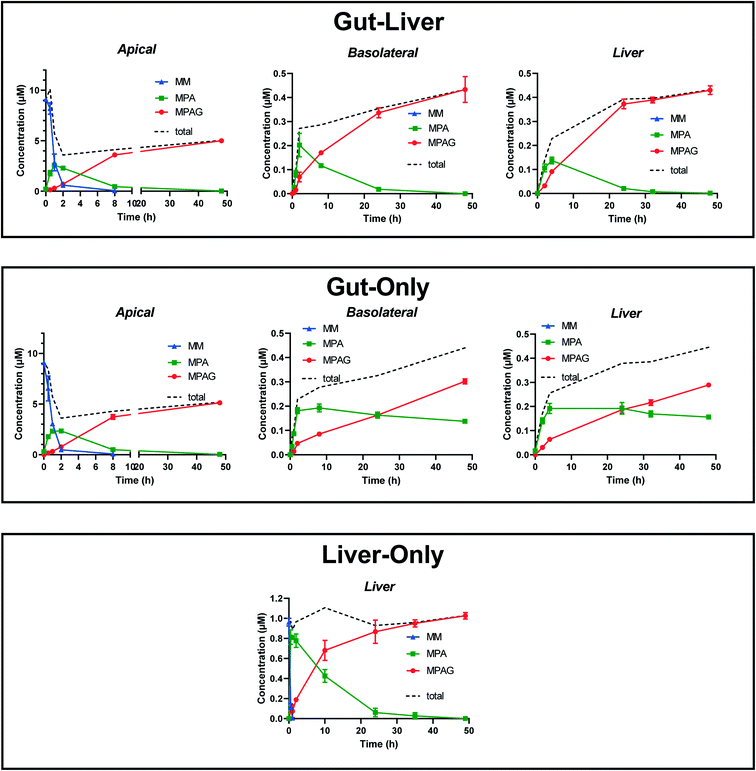 |
| Fig. 4 Time-concentration file of the prodrug mycophenolate mofetil (MM) and its metabolites (MPA and MPAG) in triplicates using three experimental systems: gut–liver, gut-only, and liver-only. The time-concentration profiles are reported in the apical, basolateral and liver compartments. The prodrug was dosed in the apical side medium (10 μM) in order to reproduce an oral administration route in gut–liver and gut-only systems and was added directly in the liver medium in the liver-only system (1 μM). In this system only the liver compartment was used in the experiment. Mycophenolate mofetil was below the limit of quantification in the basolateral and liver compartments in gut–liver and gut-only systems and they were not reported. MM concentration was below the limit of quantification in the liver-only system after 1 h. The dotted line reports the sum of the mean concentrations all compounds across each comportments. | |
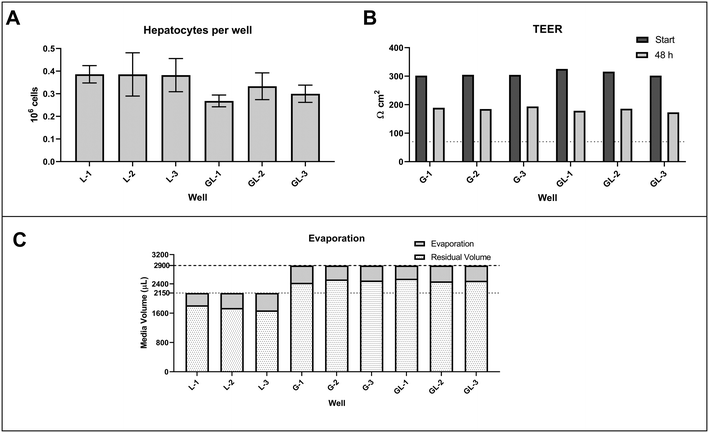 |
| Fig. 5 Histograms reporting the number of hepatocytes (A) and the TEER measurements (B) in the gut compartment at the start and end of the incubation. The abbreviations L, G, and GL corresponded to the experimental systems of liver-only, gut-only, and gut–liver, respectively. The number is associated with the well of the triplicates measurements of each system. The stacked bar chart in panel C shows the media evaporation from the gut basolateral and liver compartment. For a better understanding of the media evaporation, the media removed by sampling was not subtracted from the residual media volume. The dotted and dashed lines represent the total media volume in the liver compartment for the liver-only condition and the total media volume in the liver and basolateral compartment for the gut–liver and gut-only conditions, respectively. | |
Gut-only.
To enhance the detail of model-based understanding of MPS metabolism and disposition processes, additional experiments with individual tissues were performed in parallel. Comparison between gut–liver and gut-only systems highlighted the different contributions of hepatic and intestinal activities, with a significant role of gut metabolism in the glucuronidation of MPA in addition to the hepatic contribution, in accordance with in vivo reports.37 As expected, the apical side showed very similar MM and MPA concentration versus time profiles to those observed in the gut–liver system. However, the incomplete metabolism of MPA to MPAG in the basolateral and liver compartment in the gut-only system clearly showed the contribution of the hepatocytes to the overall metabolism of MPA. Indeed, in contrast to the gut–liver system, unconjugated MPA was still detectable at 48 h at around 0.2 μM, which was around 20% of the amount of MM initially administered in the apical side. The total concentration of compounds in the basolateral side and liver compartment in both systems was very similar (∼0.4 μM) since MPA did not re-diffuse against a concentration gradient into the apical compartment and MPAG permeability is very low (Fig. 4).
Liver-only.
In the liver-only system the prodrug was administrated directly to the liver compartment at a lower concentration (1 μM), but with a similar amount of total drug to reflect the dilution encountered after passing through the intestinal barrier in the gut–liver system (Fig. 4). The prodrug was hydrolyzed very rapidly due to the very high carboxylesterase activity of the hepatocytes. After 1 h of incubation the MM concentration was below the limit of quantification. MPA concentrations peaked at around 0.85 μM after 30 min of incubation and then it was rapidly and completely converted to MPAG by 48 h. The rapid glucuronidation of MPA by the hepatocytes confirmed the role of the liver compartment in the metabolism of MPA in the gut–liver compartment and the relative stability of MPA in the basolateral and liver compartment in gut-only system.
Gut and liver cell number.
Quantification of the number of cells in the intestinal and liver compartments is necessary to express metabolism/transport clearance data in terms of the actual number of cells to enable their quantitative in vitro to in vivo extrapolation (IVIVE) to the whole body situation. In many literature reports using organ on a chip systems, the number of cells was not included in the parameter estimation or was assumed to be the same as the initial seeding number,8,18,39 limiting quantitative extrapolation of in vitro metabolism/transport data generated. Our recent work using a liver-on-a-chip system (analogous to the liver compartment of the gut–liver system employed here) demonstrated that the seeding efficiency was ∼50% of the hepatocytes introduced to the system.6 Similar results were obtained in this study, highlighting the need to measure the actual cell number in each incubation. The number of cells present in the incubation was considered to have been constant throughout the experiment and the number of cells measured at the end of the each experiment (Fig. 4) was used for metabolism rate/clearance calculations. The measured number of hepatocytes was 0.34 million/well, with an inter-well variability of ∼15%. The Caco2-HT29 co-culture seeded on the membrane of the transwell were quantified using CLSM imaging due to the transparency of the membrane (Fig. S5.1†). The cell number in both gut–liver and gut-only systems showed low inter-well variability (∼10%). The number of cells at the end of the incubation was 0.45 ± 0.05 million per well. The experimentally measured values of gut and hepatocyte cell number in each well were used as inputs for the in silico modelling and calculation of intestinal and hepatic metabolic clearances (Fig. 5-A).
Non-specific binding to device surface.
Non-specific binding to the plastic components of the device may reduce the effective concentration of drug and metabolites in the system. The constant total amount of the drug-related material over the incubation time, suggested that the non-specific binding did not impact the PK estimation for MM and metabolites.
Opportunity for media factor cross-talk.
Cross-media exchange and potential impact of gut media factors on hepatocyte activity (and vice versa) in the experiment was not examined in this study. The potential for such exchange to have impacted the results is thought to be low, considering that characterization of cells in gut–liver and individual organ experiments at the end of the experiment (number of hepatocytes, TEER measurements, and the intestinal monolayer imaging) gave the same results and very similar data for MM metabolism in combined and individual organ experiments. Where highly disparate media are employed for longer incubation time periods and in the absence of such controls it may be important to perform media cross-compatibility experiments at the outset as part of the system testing.
Mechanistic PK modelling and quantitative evaluation of the data
Model generation and parameter estimation in gut–liver, gut-only and liver-only experiments.
Application of MPS for drug metabolism and transport studies is dependent upon quantitative outcomes that can be extrapolated to the in vivo situation.4 Due to the combination of incubation system and drug metabolism complexity, mechanistic mathematical PK modelling is needed for extraction of PK parameters from the measured data. It has already been demonstrated that mechanistic mathematical modelling allows simultaneous characterization of various cellular processes relevant for DMPK, such as metabolism and active transport.40 However, a robust in silico procedure to evaluate the gut–liver-OoC system and corresponding PK parameters estimated in this system has not previously been described. Filling this gap enables an important step forward in the development and more effective use of MPS in the DMPK area. The modelling approach illustrated here is not restricted to this experimental system/protocol and can be applied for a large variety of gut–liver OoC or similar systems combining barrier tissues with drug target or metabolism tissues, which might be available in future.
The PK parameters for each experimental system were estimated by fitting the best in silico model which was able to capture all the PK processes observed from the experimental time-concentration profiles (Fig. 6). Modelling of gut-only and liver-only experimental data was used to inform the most complex gut–liver experimental set up and the corresponding model (fitting and graphical representation of the models are reported in Fig. S10.1–2,† whereas data from the gut-only and liver-only experiments are reported in Table 3). Although the gut-only and liver-only experiments were helpful in informing the DMPK processes of the gut–liver model, they were not sufficient to account for the impact of system-related factors on the PK parameter estimates. Some system properties such as the interruption of the basolateral media flow (Qi) during sampling were demonstrated not to impact the model description of MM metabolism and therefore these were not included in the final model. In contrast, other features such as the sampling volume and media evaporation had a significant impact on the parameter estimation and were therefore included in models. Detailed information about the evaluation and the quantitative impact of individual system-related factors on the PK parameter estimation is provided in the ESI.†
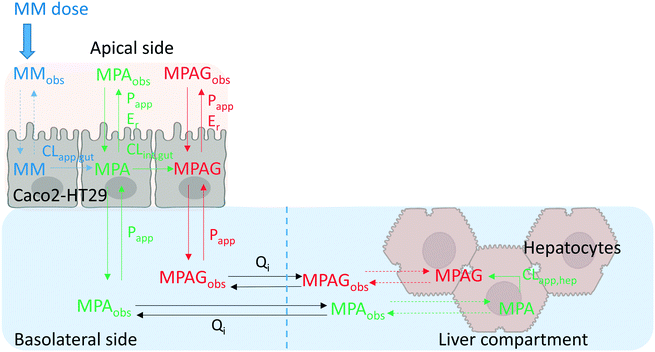 |
| Fig. 6 Schematic representation of the best model for mycophenolate mofetil (MM) which was applied for the gut–liver fitting (differential equations and model in Phoenix were reported in the ESI†). MPA, MPAG, Qi, CL, Papp, and Er referred to mycophenolic acid, mycophenolic acid glucuronide, interconnection flow rate, hepatic or intestinal metabolic clearance, intestinal permeability, and intestinal efflux ratio, respectively. Dashed lines represented processes which were not predictable from the experimental information. The abbreviation “obs” meant that the compound was experimentally detectable and quantified in the respective compartment. Schematic representation of the simultaneous fitting model is provided in Fig. S10.1.† | |
Table 3 Parameter estimates for the mechanism-based pharmacokinetic model of mycophenolate mofetil in the gut–liver OoC system for three experimental systems
Parameter |
Units |
Gut–liver |
Gut–only |
Liver–only |
All |
Estimation |
CVa% |
Estimation |
CV% |
Estimation |
CV% |
Estimation |
CV% |
When a non-normal distribution of parameter values was observed from the log likelihood profiling a range is reported (lowest, highest). The range was determined from the log likelihood profiling with a confidence level (CL) of 95% (ESI†).The related uncertainty of the parameters was reported as ± SD when the evaluation from log likelihood profiling using Wilks's test provided a normal distribution of the parameters (additional material in the ESI† and Fig. S9.1–4). CLint,gut,u CLapp,hep,u, Papp(MPA), and Er of MPA did not show a normal distribution and parametric range uncertainty within 95% of confidential level were reported in Table 1. “ALL” refers to simultaneous fitting of all data generated in the gut-only, liver-only and gut–liver conditions. |
CLint,gut,u(MM) |
μL min−1 per 106 cells |
13 |
11 |
12 |
6.0 |
— |
— |
13 |
6.5 |
CLint,gut,u(MPA) |
μL min−1 per 106 cells |
24 |
(13,48) |
14 |
7.3 |
— |
— |
17 |
10 |
CLapp,hep,u(MPA) |
μL min−1 per 106 cells |
26 |
(18,30) |
— |
— |
24 |
4 |
26 |
3.7 |
CLapp,hep,u(MM) |
μL min−1 per 106 cells |
— |
— |
— |
— |
643 |
(568,1251) |
639 |
(565,1054) |
P
app(MPA) |
nm s−1 |
688 |
(380,1390) |
539 |
18 |
— |
— |
546 |
13 |
P
app(MPAG) |
nm s−1 |
0.37 |
18 |
0.39 |
17 |
— |
— |
0.35 |
15 |
E
r(MPA) |
|
3.7 |
(2.7,5.6) |
2.7 |
18 |
— |
— |
3.0 |
(2.7,3.5) |
E
r(MPAG) |
|
2.9 |
19 |
3.1 |
19 |
— |
— |
3.1 |
15 |
In silico modelling was also applied in order to evaluate the amount of MM and its metabolites in the intestinal cell compartment which was not experimentally accessible. Simulation of the gut–liver system indicated that a significant amount of MPAG (∼30%) was concentrated within the intestinal cells in the interval of time around 2 h. This implied a relatively fast intestinal formation of MPAG from MPA followed by a very slow permeation of MPAG out of the intestinal Caco2-HT29 cells (Fig. S7.1†). The impact of the intestinal cell volume on the MPAG PK was investigated in the gut–liver system using either literature reported values for the Caco2 (2.6 μL per 106 cells)28 and HT29 cells29 (2.4 μL per 106 cells). Relatively small difference between volumes of Caco2 and HT29 cells (8%), resulted in only small differences (<10%) in estimated PK parameters using either of these volumes as input values. Model-based sensitivity analysis was also informative with regards to the impact of the Qi uncertainty (CV = 20%) on the estimation of PK parameters. The Qi was set at 90 μL min−1 in order to provide a low impact on the PK estimation (<10%) as it was demonstrated using Qi ranging from 72 to 108 μL min−1 which represented the expected range from the reported uncertainty. The quantitative Qi and intestinal cell volume effect on the PK parameter estimates are reported in detail in the ESI.†
Gut–liver system modelling.
The fast depletion of MM observed in the time-concentration profile in the gut–liver systems resulted in the estimated intestinal clearance value of 13 μL min−1 per 106 cells (CLint,gut,u MM) (Table 3) (experimental data of gut–liver and fitting of the model are reported in Fig. 7). The CLapp,hep,u value obtained from the liver-only system was 643 μL min−1 per 106 cells, approximately 50-fold higher than that of the intestine. The CLint,gut,u and CLapp,hep,u of MPA were 24 and 26 μL min−1 per 106 cells. The cell membrane Papp of MPA and MPAG were estimated as 688 and 0.39 nm s−1 respectively, indicating a >1000-fold lower permeability of the more polar glucuronide. The mechanistic mathematical modeling enabled an estimate of the intestinal efflux in the experiment to be generated. The intestinal Er values of MPA and MPAG were 3.7 and 2.9, respectively indicating active transport from within the cells to the apical compartment. The intestinal efflux of a drug may result in reduced drug exposure and increased potential for drug–drug interactions.41–43Er values greater than 1 for both MPA and MPAG were in agreement with data reported in vitro and in vivo and suggested that both compounds are substrates of efflux transporters such as P-glycoprotein (P-gp) and Multidrug Resistance-Associated Protein 2 (MRP2).44–47 P-gp and MRP2 are reported to be expressed in the Caco2 and HT29 by the provider.48
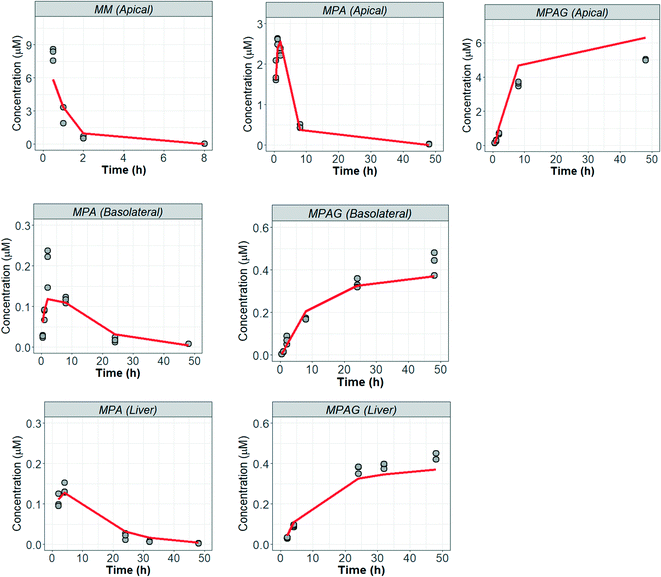 |
| Fig. 7 Mycophenolate mofetil experimental data and model from gut–liver system. Fitting of the gut-only, liver-only and simultaneous fitting of all systems are available in the ESI† (Fig. S10.1–3). MM, MPA, MPAG referred to mycophenolate mofetil, mycophenolic acid, and mycophenolic acid glucuronide, respectively. | |
The steps described above to generate the most appropriate mechanistic model that captures DMPK relevant cellular processes and the system-related parameters were necessary considering the complexity of organ on a chip system. The robustness of parameter estimates was also dependent on the design of the experiment and factors such as inter-well variability, high accuracy and low error in the sampling and analytical method and optimal selection of the sampling times. Choice of sampling times and volumes affect the ability to estimate PK parameters from the measured data. An additional consideration for organ on a chip systems is that the total number of sampling points in each compartment is limited since a minimal working volume of media needs to be maintained throughout the incubation and supplementation with additional media would change drug and metabolite concentrations, perturbing most drug metabolism studies. These technical limitations and a large number of potential combinations of estimated parameters in the gut–liver OoC can be overcome by use of a computational tool and optimization of the sampling times via global dynamic sensitivity analysis using data from a pilot experiment (data not shown). The optimized experimental sampling was confirmed by the fact that majority of parameters obtained for the gut–liver system could be estimated with a relatively low uncertainty considering the complexity of the system (<30%). Exceptions were CLint,gut,u (MPA), Papp(MPA), and Er (MPA), (Table 3) and therefore additional global dynamic sensitivity analysis was performed with the data predicted from the gut–liver system. For each compartment a high
, which assesses the overall influence of the parameter i on the output, showed that the sampling times were well selected considering the limited total sampling volume and the minimum volume of media to perform the analysis (Fig. 8 and S8.1†). Further details on the strategy for the optimization of the sampling points are available in the ESI.†
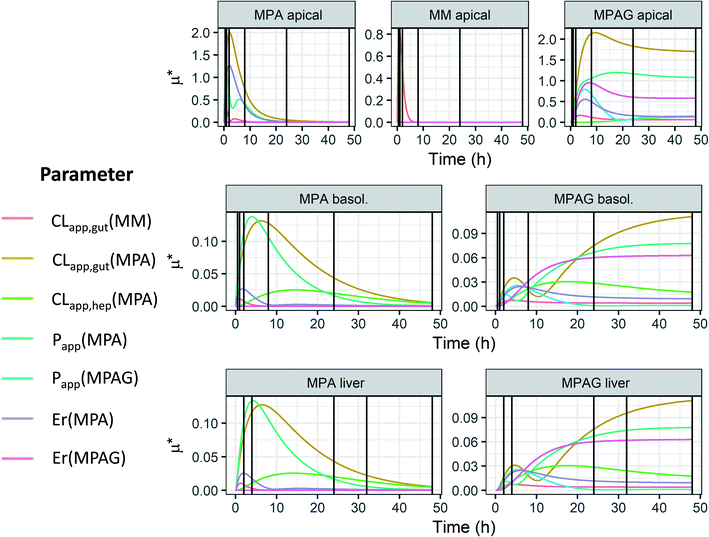 |
| Fig. 8 Global dynamic sensitivity analysis of the seven parameters in the gut–liver system. The sensitivity analysis was applied using Morris's screening for each compartment (row) and compound (column). The vertical lines in each graphs represented the sampling points (note that some of them in the apical and basolateral side are overlapped in the graph) in the respective compartments as reported in Table 1. MM, MPA, MPAG, CL, Papp, and Er referred to mycophenolic acid, mycophenolic acid glucuronide, hepatic or intestinal metabolic clearance, intestinal permeability, and intestinal efflux ratio, respectively. The term “basol.” referred to basolateral side in the gut compartment. | |
Gut–liver, gut-only, and liver-only data comparison.
In the case of mycophenolate mofetil, parameter values and their respective uncertainty showed a non-statistically significant difference, confirming the additive effect of combining the individual systems. In addition to modelling of each individual systems, a simultaneous fitting of the data from all three experimental systems was also performed (model representation and fitting are reported in Fig. S10.1 and S3†). Simultaneous fitting of all three experimental systems resulted in parameter estimates that were in agreement with those estimated from the individual fitting (Table 3), confirming additive trends. However, simultaneous modelling of all available data resulted in less uncertainty in parameter estimates due to the richness of the dataset and increased information available for modelling. For model building and evaluation, the integration of individual system component data, as illustrated here, is highly recommended. In addition, it is important to note that the additive effect observed for mycophenolate mofetil and its metabolites cannot be generalized and must be evaluated on a case-by-case basis, as these will be highly influenced by the experimental protocol, test compound, cells used and the feature of the chip system.
Application to future studies and other OoC systems
The current study demonstrated potential of the gut–liver OoC in combination with in silico modeling as a tool for quantitative estimation of PK parameters of a drug and its metabolites. Building upon promising findings and the encouraging use of in silico modelling, a number of further refinements to the system may be considered to capture better the in vivo physiology, especially in the intestinal component. The co-culture of Caco2 and HT29 cells represents a step forward from previous reports using Caco2 monocultures and it is envisaged that enterocytes/intestinal organoids may be used in future to better reproduce the enzyme and transporter activities of the small intestine. These refinements should increase the translatability of gut–liver OoC data and allow application of the system for the assessment of drug–drug interactions (both for parent drug and metabolites). For example, the current Caco2/HT29 culture did not completely reflect intestinal esterase functionality with higher levels of human carboxylesterase 1 versus carboxylesterase 2 expressed, which may impact the pattern of intestinal versus hepatic hydrolysis for some prodrugs. Developing more quantitative scaling of intestinal metabolic clearance, which needs to take into account the number of enterocytes and regional differences in enzyme expression in the small intestine49,50 will help in future PK predictions. Similarly, the extrapolation of hepatic activities could be made more representative by using a pool of primary hepatocytes donors (≥10 donors), which permits better assessment of the average PK profile in a population. A further improvement would be the investigation of transporter expression and functional activity, including biliary clearance, allowing to broaden the applicability of the system. In addition to the suggested biological refinements, the routine and standardized integration of mathematical modelling for PK parameter extraction and effective MPS experiment planning could be envisaged as a next step from this work. For instance, MPS providers could potentially in future provide such models in the form of system simulation tools to guide researchers towards more optimal experimental designs from the outset. Such tools would also be able to indicate whether the planned measurements enable determination of the desired PK parameters. This is likely to become more important as MPS usage expands and moves on from more basic in vitro pharmacology and toxicology applications (in which constant drug concentrations can often be assumed) to systems in which drug and metabolite concentrations change with time, as they do in vivo.
Conclusion
This work applied a gut–liver OoC device to study the PK of mycophenolate mofetil and its two major metabolites and opens the possibility for future investigations of prodrugs where a combination of intestinal and hepatic processes determine drug and metabolite exposure. In addition, we defined a robust and rigorous in silico modelling strategy which was fundamental for evaluation of the data from the combined gut–liver system. Mechanistic modelling aids study design and is key to optimization of the experimental protocol of such a complex multi-organ system. The current study highlighted the importance of considering drug specific processes such as metabolism and permeability together with system related features such as media flow rate, sampling and evaporation. The demonstrated ability of the gut–liver OoC to capture the intestinal and liver metabolism of mycophenolate mofetil supports its potential for prediction of pharmacokinetics and drug–drug interaction risk of other orally administered drugs with complex in vivo behavior.
Author contributions
Participated in research design: Milani, Parrott, Galetin, Gertz, Fowler. Conducted experiments: Milani. Performed data analysis: Milani, Parrott, Ortiz Franyuti, Gertz, Fowler. Wrote or contributed to the writing of the manuscript: Milani, Parrott, Ortiz Franyuti, Godoy, Galetin, Gertz, Fowler.
Conflicts of interest
NM, NP, PG, DOF, PG, MG and SF are employees of F. Hoffmann-La Roche. AG is an employee of the University of Manchester. The authors do not have any conflicts of interest to declare.
Acknowledgements
Technical support and guidance from NaHong Qiu, Aynur Ekiciler, Vincent Monin, Andreas Götschi, and Charles Alexandre Tournillac is gratefully acknowledged.
References
- M. Cirit and C. L. Stokes, Lab Chip, 2018, 18, 1831–1837 RSC.
- J. P. Wikswo, Exp. Biol. Med., 2014, 239, 1061–1072 CrossRef PubMed.
- J. P. Wikswo, E. L. Curtis, Z. E. Eagleton, B. C. Evans, A. Kole, L. H. Hofmeister and W. J. Matloff, Lab Chip, 2013, 13, 3496–3511 RSC.
- S. Fowler, W. L. K. Chen, D. B. Duignan, A. Gupta, N. Hariparsad, J. R. Kenny, W. G. Lai, J. Liras, J. A. Phillips and J. Gan, Lab Chip, 2020, 20, 446–467 RSC.
- K. Kaarj and J.-Y. Yoon, Micromachines, 2019, 10, 700 CrossRef PubMed.
- L. Docci, N. Milani, T. Ramp, A. A. Romeo, P. Godoy, D. O. Franyuti, S. Krähenbühl, M. Gertz, A. Galetin, N. Parrott and S. Fowler, Lab Chip, 2022, 22, 1187–1205 RSC.
- A. Rubiano, A. Indapurkar, R. Yokosawa, A. Miedzik, B. Rosenzweig, A. Arefin, C. M. Moulin, K. Dame, N. Hartman and D. A. Volpe, Clin. Transl. Sci., 2020, 14, 1049–1061 CrossRef PubMed.
- N. Tsamandouras, W. L. K. Chen, C. D. Edington, C. L. Stokes, L. G. Griffith and M. Cirit, AAPS J., 2017, 19, 1499–1512 CrossRef CAS PubMed.
- H. Arakawa, S. Sugiura, T. Kawanishi, K. Shin, H. Toyoda, T. Satoh, Y. Sakai, T. Kanamori and Y. Kato, Lab Chip, 2020, 20, 537–547 RSC.
- T. Satoh, S. Sugiura, K. Shin, R. Onuki-Nagasaki, S. Ishida, K. Kikuchi, M. Kakiki and T. Kanamori, Lab Chip, 2018, 18, 115–125 RSC.
- K. Shinha, W. Nihei, T. Ono, R. Nakazato and H. Kimura, Biomicrofluidics, 2020, 14, 044108 CrossRef CAS PubMed.
- A. Ferraretto, M. Bottani, P. De Luca, L. Cornaghi, F. Arnaboldi, M. Maggioni, A. Fiorilli and E. Donetti, Biosci. Rep., 2018, 38, 1–16 CrossRef PubMed.
-
J. L. Madara, Physiology of the gastrointestinal tract, 1994, pp. 1577–1612 Search PubMed.
- I. Lozoya-Agullo, F. Araujo, I. Gonzalez-Alvarez, M. Merino-Sanjuan, M. Gonzalez-Alvarez, M. Bermejo and B. Sarmento, Mol. Pharmaceutics, 2017, 14, 1264–1270 CrossRef CAS PubMed.
- Z. M. Al-Majdoub, N. Couto, B. Achour, M. D. Harwood, G. Carlson, G. Warhurst, J. Barber and A. Rostami-Hodjegan, Clin. Pharmacol. Ther., 2021, 109, 1136–1146 CrossRef CAS PubMed.
- M. F. Paine, H. L. Hart, S. S. Ludington, R. L. Haining, A. E. Rettie and D. C. Zeldin, Drug Metab. Dispos., 2006, 34, 880–886 CrossRef CAS PubMed.
- T. J. Franklin, V. N. Jacobs, G. Jones and P. Plé, Drug Metab. Dispos., 1997, 25, 367–370 CAS.
- N. Tsamandouras, T. Kostrzewski, C. L. Stokes, L. G. Griffith, D. J. Hughes and M. Cirit, J. Pharmacol. Exp. Ther., 2017, 360, 95–105 CrossRef CAS PubMed.
- N. Ishiguro, W. Kishimoto, A. Volz, E. Ludwig-Schwellinger, T. Ebner and O. Schaefer, Drug Metab. Dispos., 2014, 42, 250–256 CrossRef CAS PubMed.
- S. Siissalo, H. Zhang, E. Stilgenbauer, A. M. Kaukonen, J. Hirvonen and M. Finel, Drug Metab. Dispos., 2008, 36, 2331–2336 CrossRef CAS PubMed.
- A. Mróz, I. Ryska, H. Sominko, A. Bejrowska and Z. Mazerska, Pharmacol. Rep., 2018, 70, 972–980 CrossRef PubMed.
- A. Wikman-Larhed and P. Artursson, Eur. J. Pharm. Sci., 1995, 3, 171–183 CrossRef CAS.
- C. Hilgendorf, H. Spahn-Langguth, C. G. Regårdh, E. Lipka, G. L. Amidon and P. Langguth, J. Pharm. Sci., 2000, 89, 63–75 CrossRef CAS PubMed.
- A. Ayrton and P. Morgan, Xenobiotica, 2001, 31, 469–497 CrossRef CAS PubMed.
- N. Matsunaga, S. Wada, T. Nakanishi, M. Ikenaga, M. Ogawa and I. Tamai, Mol. Pharmaceutics, 2014, 11, 568–579 CrossRef CAS PubMed.
- G. Bellu, M. P. Saccomani, S. Audoly and L. D'Angiò, Comput. Methods Programs Biomed., 2007, 88, 52–61 CrossRef PubMed.
- M. P. Saccomani and L. D'angiò, IFAC Proceedings Volumes, 2009, 42, 48–53 CrossRef.
- C. W. Tan, B. S. Gardiner, Y. Hirokawa, M. J. Layton, D. W. Smith and A. W. Burgess, PLoS One, 2012, 7, e31882 CrossRef CAS PubMed.
- M. Tahara, T. Inoue, Y. Miyakura, H. Horie, Y. Yasuda, H. Fujii, K. Kotake and K. Sugano, Biochem. Biophys. Res. Commun., 2013, 434, 753–759 CrossRef CAS PubMed.
- M. D. Morris, Technometrics, 1991, 33, 161–174 CrossRef.
- T. Imai, M. Imoto, H. Sakamoto and M. Hashimoto, Drug Metab. Dispos., 2005, 33, 1185–1190 CrossRef CAS PubMed.
- H. Nishimuta, J. B. Houston and A. Galetin, Drug Metab. Dispos., 2014, 42, 1522–1531 CrossRef PubMed.
- K. L. Gill, J. B. Houston and A. Galetin, Drug Metab. Dispos., 2012, 40, 825–835 CrossRef CAS PubMed.
- J. Lamego, P. Ferreira, M. Alves, A. Matias and A. L. Simplício, Mol. Cell. Probes, 2015, 29, 215–222 CrossRef CAS PubMed.
- E. Lévesque, R. Delage, M. O. Benoit-Biancamano, P. Caron, O. Bernard, F. Couture and C. Guillemette, Clin. Pharmacol. Ther., 2007, 81, 392–400 CrossRef PubMed.
- N. Fujiyama, M. Miura, S. Kato, T. Sone, M. Isobe and S. Satoh, Drug Metab. Dispos., 2010, 38, 2210 CrossRef CAS PubMed.
- R. Bullingham, S. Monroe, A. Nicholls and M. Hale, J. Clin. Pharmacol., 1996, 36, 315–324 CrossRef CAS PubMed.
- R. E. Bullingham, A. J. Nicholls and B. R. Kamm, Clin. Pharmacokinet., 1998, 34, 429–455 CrossRef CAS PubMed.
- M. Nakano, N. Kohri, Y. Arakawa and T. Arita, Chem. Pharm. Bull., 1979, 27, 573–577 CrossRef CAS PubMed.
- K. Ménochet, K. E. Kenworthy, J. B. Houston and A. Galetin, J. Pharmacol. Exp. Ther., 2012, 341, 2 CrossRef PubMed.
- C. Zurth, K. Graudenz, K. Denner, T. Korjamo, R. Fricke, G. Wilkinson, F. Seitz and O. Prien, J. Clin. Oncol., 2019, 37, 297 Search PubMed.
- S. Misaka, N. Miyazaki, M. S. Yatabe, T. Ono, Y. Shikama, T. Fukushima and J. Kimura, J. Clin. Pharmacol., 2013, 53, 738–745 CrossRef PubMed.
- X. Chu, A. Galetin, M. J. Zamek-Gliszczynski, L. Zhang and D. J. Tweedie, Clin. Pharmacol. Ther., 2018, 104, 788–792 CrossRef PubMed.
- D. A. Hesselink, R. M. Van Hest, R. A. Mathot, F. Bonthuis, W. Weimar, R. W. De Bruin and T. Van Gelder, Am. J. Transplant., 2005, 5, 987–994 CrossRef CAS PubMed.
- C. G. Patel, K. Ogasawara and F. Akhlaghi, Xenobiotica, 2013, 43, 229–235 CrossRef CAS PubMed.
- J. Wang, M. Figurski, L. M. Shaw and G. J. Burckart, Transplant Immunol., 2008, 19, 192–196 CrossRef CAS PubMed.
- I. S. Westley, L. R. Brogan, R. G. Morris, A. M. Evans and B. C. Sallustio, Drug Metab. Dispos., 2006, 34, 261–266 CrossRef CAS PubMed.
- T. Hirohashi, H. Suzuki, X.-Y. Chu, I. Tamai, A. Tsuji and Y. Sugiyama, J. Pharmacol. Exp. Ther., 2000, 292, 265–270 CAS.
- H. Zhang, C. Wolford, A. Basit, A. P. Li, P. W. Fan, B. P. Murray, R. H. Takahashi, S. C. Khojasteh, B. J. Smith and K. E. Thummel, Drug Metab. Dispos., 2020, 48, 528–536 CrossRef CAS PubMed.
- A. Fritz, D. Busch, J. Lapczuk, M. Ostrowski, M. Drozdzik and S. Oswald, Basic Clin. Pharmacol. Toxicol., 2019, 124, 245–255 CrossRef CAS PubMed.
|
This journal is © The Royal Society of Chemistry 2022 |