DOI:
10.1039/D1LC00689D
(Paper)
Lab Chip, 2022,
22, 225-239
Microfluidic organ-on-chip system for multi-analyte monitoring of metabolites in 3D cell cultures†
Received
3rd August 2021
, Accepted 3rd November 2021
First published on 1st December 2021
Abstract
Three-dimensional cell cultures using patient-derived stem cells are essential in vitro models for a more efficient and individualized cancer therapy. Currently, culture conditions and metabolite concentrations, especially hypoxia, are often not accessible continuously and in situ within microphysiological systems. However, understanding and standardizing the cellular microenvironment are the key to successful in vitro models. We developed a microfluidic organ-on-chip platform for matrix-based, heterogeneous 3D cultures with fully integrated electrochemical chemo- and biosensor arrays for the energy metabolites oxygen, lactate, and glucose. Advanced microstructures allow straightforward cell matrix integration with standard laboratory equipment, compartmentalization, and microfluidic access. Single, patient-derived, triple-negative breast cancer stem cells develop into tumour organoids in a heterogeneous spheroid culture on-chip. Our system allows unprecedented control of culture conditions, including hypoxia, and simultaneous verification by integrated sensors. Beyond previous works, our results demonstrate precise and reproducible on-chip multi-analyte metabolite monitoring under dynamic conditions from a matrix-based culture over more than one week. Responses to alterations in culture conditions and cancer drug exposure, such as metabolite consumption and production rates, could be accessed quantitatively and in real-time, in contrast to endpoint analyses. Our approach highlights the importance of continuous, in situ metabolite monitoring in 3D cell cultures regarding the standardization and control of culture conditions, and drug screening in cancer research. Overall, the results underline the potential of microsensors in organ-on-chip systems for successful application, e.g. in personalized medicine.
1. Introduction
Three dimensional (3D) cell cultures have become essential in vitro models, e.g. in cancer research and drug development. Microfluidic organ-on-chip platforms allow patterning and compartmentalization of 3D cultures to study responses to stimuli such as drug exposure at minimal volumes, control of culture conditions, and cellular interactions. Integrated microsensors for real-time monitoring of both culture conditions and cell metabolism promise unprecedented insight into these complex in vitro models. In cancer research, the use of patient-derived stem cells that recapitulate the original patient's tumour from a single stem cell level onwards promises truly individualized medicine.1,2 Such models are of particular importance in the development of new and personalized medications, as they offer the possibility for fast, cost-effective and parallelized testing of potential drug candidates in vitro, without negative side effects for the patient and with the perspective to also reduce animal experiments. The triple-negative breast cancer stem cell (BCSC) model used in this study consists of isolated cells from individual patients after undergoing chemotherapy.3 Focus on such stem cells is essential because they drive metastasis and are therapy resistant, meaning traditional treatments may not be effective. In current practice, in order to test e.g. the efficacy of a chemotherapeutic drug, cells are cultured in microtiter plates, then exposed to the chemotherapeutic agent, and then cultured again while cell viability is tracked by e.g. spheroid growth or staining methods. In some cases, cells are transplanted into mice as xenografts in order to recapitulate the original tumour. Such procedures are time and resource consuming, aside from the ethical concerns of animal models. It would therefore be beneficial to perform some of these analyses on-chip. One central aspect is hypoxia as the cells are typically cultured in hypoxia incubators, although it is not often clear which exact oxygen concentration occurs within the 3D matrix. In a stop/flow regimen within an organ-on-chip system, e.g. oxygen consumption as a quantitative measure for cell viability can be determined within minutes from a small cell number in a minimal volume. Besides continuous and online tracking of metabolic parameters, microfluidic systems allow an advanced level of control of culture conditions including in situ verification thereof.
Along with the extensive effort put into improvement of in vitro models in order to make them resemble the in vivo situation as closely as possible, it became evident that traditional 2D cultures consisting of adherent cell monolayers can replicate the complex reality within the human body only to a limited extent. It has been shown that cells of the same type behave fundamentally different depending on how they are cultured.4 Within a 2D culture, cell interactions occur only laterally across the cell membranes and through signalling molecules that spread within the comparably large volume of cell culture medium. Within static 3D cultures, however, cells are embedded within an artificial extracellular matrix (ECM) and develop into spherical structures called spheroids, in which cell–cell interactions and concentration gradients of metabolites and waste products are found as under physiological conditions.5,6 Furthermore, 3D models were equipped with medium supply channels for active7,8 or passive9,10 perfusion in order to mimic the dynamic situation and concentration gradients within living tissue even better. In such microfluidic platforms, the ECM is partitioned from medium channels by means of photolithographic ECM patterning or specific barrier structures, manufactured in a wide range of dimensions and shapes.10–14 Trietsch et al. introduced their concept of stratified cell culture, in which ECM-gels are separated from fluid channels by microstructured phaseguides in a microtiter plate format.10 ECM-free spheroid generation approaches have been elaborated, based on the agglomeration of cells, rather than their formation from single cells, within round-bottom ultralow attachment plates, spinning bioreactors, hanging droplets, microengineered traps, magnetic levitation and fluidically generated droplets.15 Such approaches accelerate spheroid formation, but, self-assembly of cells into patient-specific tumour organoids cannot be studied in more detail under these accelerated conditions.
Characterization of the cell state has traditionally been dominated by optical methods such as staining, combined with microscopy and endpoint analysis. In recent years, it has been shown, that the monitoring of metabolic parameters and culture conditions by means of embedded sensors can provide many benefits in terms of information content and quality of drug screening experiments, as well as fundamental metabolic characteristics of tissues.16 Sensors for many different parameters such as oxygen,17–27 superoxide,28 nitric oxide (NO),29 pH,20,25,27,30–32 transepithelial electrical resistance (TEER),33–36 lactate,19,23,31,37 glucose19,31,37 and other biochemically relevant substances were developed and used for examination of static and dynamic 2D and 3D in vitro models. Optical sensors measure variations in optical properties, e.g. luminescence of sensing layer, caused by analyte concentration changes. Their limitations include their undefined zero-point, integration capability into full-wafer fabrication processes and inherent non-linear transfer function, which restricts them from use at low concentrations. Deposition of microelectrodes by means of thin-film technology established in microfabrication, on the other hand, enables the integration of sensor arrays with a high spatial resolution within a miniaturized environment. Besides rather basic electrical sensors for impedance measurement to assess barrier properties of adherent cells, this approach allows the integration of electrochemical sensors for measurement of a variety of different dissolved species at micromolar concentrations and second-by-second temporal resolution. Their successful integration into both static17,24,25,38 and dynamic31 2D cell culture, as well as hanging droplet systems,37 has already been demonstrated. Bavli et al. showed luminescence-based oxygen sensor beads integrated into multiple spheroids in a bioreactor, together with external downstream electrochemical glucose and lactate biosensors. Misun et al. integrated glucose and lactate biosensors in their hanging droplet system to measure single spheroid metabolism in situ over hours. Both approaches include isolated spheroids in an open or gas-permeable system environment. Microsensor integration into platforms for dynamic, matrix-based cultivation of cells in 3D, on the other hand, is rather challenging. Compartments for reliable cell-laden gel integration have to be formed. Dynamic, reliable and efficient fluidic access has to be provided to supply the cells with medium, and meaningful measurement signals have to be extracted from the heterogeneous culture by integrated sensors or via microfluidics.
In this work, we introduce a transparent, state-of-the-art organ-on-chip platform for matrix-based organoid cultivation from single cells with integrated highly sensitive and long-term stable electrochemical microsensors for real-time measurement of oxygen, lactate and glucose. Cell cultivation is performed within culture compartments enclosing the ECM, separated by structured microchannels to enable medium perfusion, introduction of external stimuli and different microfluidic operation modes. The presented technology links microsensors with compartmentalized cell culture, allowing culture conditions, including hypoxia, to be specifically adjusted and monitored. Besides optical inspection of spheroid development, the transparent platform enables determination of cellular oxygen and glucose consumption as well as lactate production with stable sensors over a relevant period of more than one week. To underline the utility of such a technology for drug-screening experiments during personalized therapy, patient-derived triple-negative breast cancer stem cells (BCSC1) are used as a clinically relevant cell model and exposed to drugs and hypoxia. Besides drug screening and toxicity studies, organ-on-chip systems with integrated electrochemical sensors, as introduced in the following, can contribute to standardization of in vitro models and to more fundamental research on cellular metabolism and interactions between co-cultures.
2. Methods
2.1. Chip fabrication
Fabrication of the organ-on-chip system began on wafer-level with a 500 μm thick, 4-inch Pyrex borosilicate glass wafer (Fig. 1c). First, the wafer was coated with a 500 nm silicon nitride insulating layer using plasma enhanced physical vapour deposition (PECVD). The image reversal resist AZ 5214E (Clariant, Switzerland) was spin-coated to a thickness of 1.4 μm and patterned by UV light with the mask aligner MA6B (Süss MicroTec, Germany) using a chromium mask. Subsequently, 50 nm titanium as adhesion promoter, 100 nm platinum as electrode material and 20 nm titanium as capping layer were evaporated and structured in a lift-off process. In a further PECVD step, the wafer surface was covered with an insulation stack of 800 nm silicon nitride and 200 nm silicon oxide. Reactive ion etching (RIE) through the previously patterned AZ 1518 (Clariant, Switzerland) positive resist mask was used to open the insulation and the titanium layer in regions on the wafer where contact pads and electrodes are located.
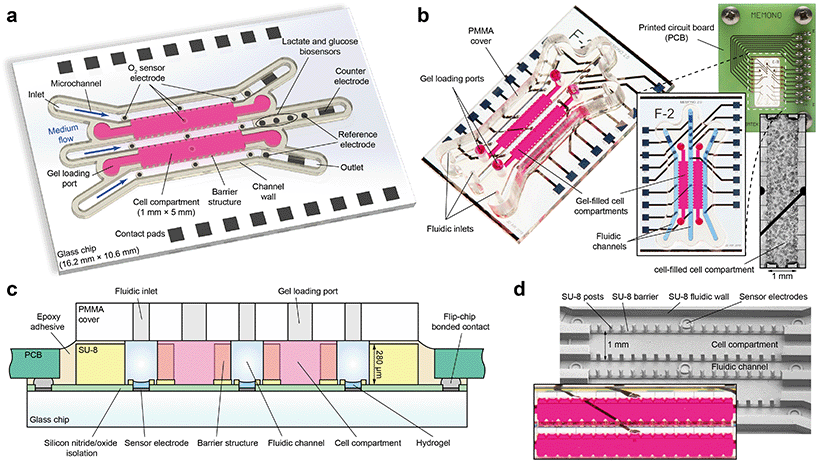 |
| Fig. 1 System design, configuration and microfabrication. (a) Top-view illustration of the sensor glass chip comprising two cell compartments for matrix-based spheroid cultivation with three adjacent microchannels for medium supply and fluidic control of culture conditions. Electrochemical microsensors for oxygen, lactate and glucose are integrated for continuous metabolic monitoring. (b) Photographs of an assembled device filled with coloured hydrogel and with attached PMMA-cover. Electrical sensor read-out is done by means of a custom-made printed circuit board. Phase-contrast micrograph shows breast cancer stem cell (BCSC1) spheroids within a spheroid compartment after five days of culture. (c) Schematic cross-sectional view, including materials and technologies used. (d) SEM-micrograph of patterned SU-8 structures composing the fluidic structures. Barrier structures allow leakage-free filling of extracellular matrix hydrogels. The photograph shows gel-filled cell compartments. | |
The fluidic walls, barrier structures and electrode rims of the platform were formed using the permanent negative epoxy resist SU-8 3000 as structural material (Fig. 1c and d). The complex structures were created by 365 nm UV light exposure of multiple SU-8 layers through two different chrome masks. A first 40 μm thick layer of SU-8 3025 was spin-coated, soft-baked and exposed to 360 mJ cm−2 UV light to generate circular structures around sensor and reference electrodes, as well as the continuous part of the barrier structures. A post exposure bake step was followed by spin-coating of three consecutive layers of SU-8 3050 at 80 μm thickness each. In between each layer deposition, a soft bake was conducted for 5 min at 65 °C and 25 min at 95 °C. The temperature was ramped at 2 °C min−1 in all baking steps in order to avoid thermal stress-induced delamination of the already cross-linked structures of the first SU-8 layer. After coating the wafer with all layers, the SU-8 stack with a total thickness of 280 μm was exposed through a second chrome mask with 990 mJ cm−2 UV light and post exposure baked at 65 °C for 5 h. This step patterns the fluidic walls as well as the triangular shaped barrier structures. Development in 1-methoxy-2-propanol acetate (Sigma-Aldrich, USA) for 75 min on an orbital shaker revealed the cross-linked structures by dissolving unexposed SU-8, which were finally hard baked for 3 h at 150 °C.
2.2. Sensor electrode modification
Silver/silver chloride reference electrodes were fabricated by electroplating of a 10 μm thick silver layer at −16 mA cm−2 for 10 min in Arguna S solution (Umicore Galvanotechnik, Germany). This layer was then partially converted to silver chloride in 0.1 M KCl solution at 1.6 mA cm−2 for 20 min. The last fabrication step on wafer-level was the deposition of a permselective membrane onto the biosensor electrodes. Therefore, m-poly(phenylenediamine) was deposited by electropolymerization during cyclic voltammetry using a 3 mM m-phenylenediamine (Sigma-Aldrich, USA) solution. Wafer dicing separated the 20 chips per wafer. Electrical connection to the system was ensured by flip-chip bonding to a printed circuit board with the electrically conductive adhesive Polytec EC 242 (Polytec PT, Germany) and curing at 100 °C for 20 min. Since all subsequent fabrication steps were carried out at room temperature, electrodes could now be further equipped with hydrogels, which partly contain temperature-sensitive enzymes.
Oxygen sensor electrodes were modified through deposition of a diffusion-limiting membrane by manual dispensing and subsequent UV-curing of poly(2-hydroxyethyl methacrylate) (pHEMA). The pHEMA precursor solution consisted of 28% HEMA, 26% pHEMA, 42% ethylene glycol, 3% triethylene glycol dimethacrylate (TEGDMA) and 1% Irgacure 651 in water. Circular SU-8 rims around the electrodes define the volume and protect the membrane during microfluidic operation. Platinum counter electrodes were left untreated. For biosensor fabrication, pHEMA with entrapped lactate oxidase (Sekisui Chemical, Japan) or glucose oxidase (BBI Solutions, UK) was dispensed onto the previously applied electropolymer membrane. If an increase of the linear range of the sensor was necessary, an additional diffusion-limiting pHEMA membrane without embedded enzymes was applied. Blank electrodes consisted of exactly the same configuration but without lactate oxidase or glucose oxidase in the first pHEMA membrane.
2.3. Device assembly and electrical read-out
The final fabrication step was the permanent attachment of a computer numerical control (CNC) milled poly(methylmethacrylate) (PMMA) cover (2 mm thickness) to the fluidic unit (Fig. 1b and c). The cover contained all fluidic in- and outlet ports and was placed manually on the fluidic walls using a stereo microscope. Both components of the system were attached by underfilling of the biocompatible epoxy-based adhesive Epo-Tek 302-3M (Epoxy Technology, USA) and subsequent curing at room temperature for 24 h. The same approach was used to seal the electrical connections between glass chip and PCB (Fig. 1b).
The multi-channel potentiostat MultiPalmSens4 (Palmsens, The Netherlands) was used in all electrochemical measurements in a 3-electrode setup using on-chip reference and counter electrodes. Oxygen sensor operation was performed at an acquisition rate of 10 Hz using the following three-step chronoamperometric protocol: (1) 0.7 VAg/AgCl for 2 s, (2) −0.4 VAg/AgCl for 2 s, (3) −0.3 VAg/AgCl for 6 s. Each measurement cycle was ended at open circuit conditions, whose duration defined the time between two oxygen concentration readings and lasted 50 s or 110 s, depending on the experiment. Biosensors were operated at a constant potential of 0.5 VAg/AgCl and an acquisition rate of 2 Hz.
2.4. Cell culture
The fluorescent-labelled breast cancer stem cell line 1 (BCSC1 eGFP) was utilized for all cell-related experiments. Passage 30–40 cells were cultured in a 2D environment in mammary stem cell (MSC) medium with 2% Matrigel (Corning, USA) at 37 °C inside a hypoxia box (Billups-Rothenberg, USA) and low oxygen conditions (3% O2, 5% CO2, 92% N2). The cell culture medium consisted of mammary epithelial basal medium (Lonza Group, Switzerland) and was supplemented with 2% B27 (Gibco-BRL, USA), 1% amphotericin B (Sigma Aldrich, USA), 1% penicillin/streptomycin (Sigma-Aldrich, USA), 20 ng ml−1 epidermal growth factor (PeproTech, USA), 4 μg ml−1 heparin (Sigma-Aldrich, USA), 20 ng ml−1 fibroblast growth factor (PeproTech, USA), 35 μg ml−1 gentamicin (Gibco, USA) and 500 nM rho kinase inhibitor H-1152 (Calbiochem, USA). Cells were split as soon as the confluence reached 70%.
In order to isolate single cells from the 2D cell culture, cells were incubated with accutase (Life Technologies, USA) for 20 min at 37 °C. After cell counting, the desired cell number was suspended in 25% MSC medium and 75% Matrigel on ice. A volume of 3.5 μl of the suspension was filled into each cell compartment through the gel loading ports using a 10 μl pipette and pre-cooled tips. The cell-laden chip was incubated at 37 °C for additional 30 min to let the Matrigel solidify. The polyimide tape HB830 (Hi-Bond Tapes, UK) was used in order to seal all ports in a gas-tight manner on the top side of the platform which are not connected during microfluidic operation. Drug exposure experiments were performed using doxorubicin hydrochloride (Actavis, USA) and antimycin A (Sigma Aldrich, USA).
2.5. Microfluidic measurements
Demonstration of fluidic compartment separation was performed with spheroid compartments containing 0.5% agarose (Sigma-Aldrich, USA). Depending on the scenario, either pure phosphate-buffered saline (PBS) or PBS with 100 μM H2O2 (Perhydrol, Merck, Germany) was perfused at 2 μl min−1 through the respective channel by means of two precision syringe pumps (PHD 2000 Infusion/Withdraw, Harvard Apparatus, USA and Cavro XL 3000, Tecan Group, Switzerland).
Throughout all cell-based and sensor-related measurements, the programmable syringe pump Cavro XL 3000 was used. Pump protocols were implemented with the software bioMON 4 (Jobst Technologies, Germany).
Oxygen calibration was performed in PBS at 37 °C and Matrigel-filled spheroid compartments. The electrolyte reservoir was purged with a mixture of air and nitrogen using the gas mixing station GMix41 (Hitec-Zang, Germany). Biosensors were calibrated before and after an experiment using aliquots of MSC medium containing different concentrations of sodium L-lactate (Sigma-Aldrich, USA) and D-(+)-glucose-monohydrate (Honeywell Fluka, USA).
After platform seeding with the desired cell density, the system was placed in an oven at 37 °C and electrically connected to the potentiostat. Unless otherwise stated, the inlet of the middle channel was connected to a reservoir filled with 15 ml MSC medium via microfluidic tubing. An atmosphere consisting of 95% air and 5% CO2 supplied by the gas mixing station was created within the reservoir. A syringe pump was connected to the same channel's outlet in order to aspirate the cell culture medium in stop/flow (2 μl min−1) cycles. The inverted microscope Nikon Eclipse TE2000-U (Nikon, Japan) was used to acquire phase-contrast and fluorescence images.
2.6. Computational modelling of mass transport
We performed computational modelling of the mass transport in the microfluidic organ-on-chip system using finite element analysis with COMSOL Multiphysics 4.2 (COMSOL, USA). The physics interface “Transport of Diluted Species” bearing Fick's law was chosen under the assumption of diffusion limited mass transport within the two-dimensional model. No further interaction of the molecule with the hydrogel was assumed. Concentration changes within the supply channel were implemented with a transition time of 20 s. Hydrogen peroxide and doxorubicin, with diffusion coefficients in water modelled as 2 × 10−9 m2 s−1 (ref. 39, 40) and 0.58 × 10−9 m2 s−1,41 respectively, were used as representative substances for small and larger molecules. Diffusion coefficients for the substances in 75% Matrigel in the cell chambers are not available in the literature. Based on our own measurements, we estimated the diffusion coefficients in 75% Matrigel to be 25% lower than in water, which is in good agreement with the literature42 for 100% Matrigel. Additional analyses for various diffusion coefficients, chip locations and diffusion times can be found in the ESI.†
3. System concept and design
The presented microsystem is designed for three-dimensional, matrix-based cell cultivation and sensor-assisted characterization of the metabolic microenvironment. Preliminary results were introduced earlier.43 Simple, microstructure-guided gel integration allows the embedment of artificial extracellular matrices (ECM) commonly used for in vitro cell cultivation44 into the closed platform, such as Matrigel, but also agarose or polyethylene glycol (PEG). Within these compartments, single cells of mono- or co-cultures develop into organoids with heterogeneous 3D structures during microfluidic operation, while cell culture medium is supplied via adjacent microchannels (Fig. 1a and b). The same channels permit the targeted control of culture conditions, insertion of external stimuli such as drugs or hypoxia, and a fluidic separation of culture compartments for investigation of cell–cell interactions. In contrast to open systems such as hanging droplet platforms or systems made of PDMS, an accurate control of the dissolved gas composition and the ability to measure cellular respiration rates is enabled by the gas-tight sealing of the culturing milieu. Electrochemical microsensors, directly integrated into the culture environment, monitor both culture conditions and cellular metabolism. The metabolic parameters oxygen, lactate and glucose are measured continuously in a stop/flow perfusion concept for renewal of the medium without exposing cells to flow-induced shear stress. Additionally, the design also allows passive pumping schemes, e.g. by gravity.
The fluidic unit of the system is disposed on a 16.2 × 10.6 mm2 Pyrex borosilicate glass chip using microfabrication processes and consists of two 1.4 μl cell compartments surrounded by three 500 μm wide microchannels (Fig. 1a). Within the platform, electrochemical microsensors are integrated into the bottom of the glass chip. Three amperometric oxygen sensor electrodes (Ø 200 μm) are located in each of the three channels in close vicinity of the compartments. In another design variation, oxygen sensors are additionally located in the spheroid compartments' bottom in order to access pericellular oxygen levels. Three enzyme-based biosensor electrodes for glucose, lactate and the blank signal are present downstream the middle channel. Biosensors are located just outside the cell culture area so that the occurring reactions do not influence the cells, but close enough that undiluted low-volume medium can be measured. Additionally, the biosensor technology allows the potential detection of additional molecules such as pyruvate, glutamate45 or superoxide28 by changing the embedded enzyme. Each microchannel has a downstream rectangular-shaped counter (0.35 mm2) and circular silver/silver chloride (Ag/AgCl) reference electrode in order to measure the analytes in a 3-electrode setup. Biosensor and reference electrodes have a diameter of 300 μm.
Based on previous studies of Bohl et al.46 and Rubehn et al.,47 a multi-layer photo-lithography process was elaborated to pattern the structures which form the fluidic unit from permanent epoxy photoresist (SU-8). The interface between compartment and microchannel, which is defined by barrier structures that guide leakage-free filling of the liquid cell/matrix suspension, ensures diffusion of dissolved molecules into and out of the compartments during culture. Barrier structures are composed of a 40 μm high step with superimposed equilateral triangles of 100 μm edge length and 200 μm spacing (Fig. 1d). The height of the triangles extends up to the entire height (280 μm) of the inner volume of the platform, which can be adjusted with a high precision and reproducibility by means of the multi-layer fabrication process. In combination with the flat step, the integrity of the introduced gel is not affected by fluidic operation for more than one week. The top part of the platform is formed by a milled PMMA cover including all fluidic ports and is hermetically bonded to the fluidic unit (Fig. 1c). The design is such that all fluidic structures could be included in the cover alone, e.g. as an injection moulded part, for more efficient fabrication.
Single cells suspended in an ice-cold Matrigel/medium mixture are introduced with a standard laboratory 10 μl pipette via cell-loading inlet ports on the topside of the platform (see Movie S1†). The guidance of the barrier structures provides leakage-free fluid propagation through the spheroid compartments (Movie S2†) with a homogeneous cell distribution. The modular arrangement of compartments and channels permits upscaling if additional cultures are to be studied. An internal volume of below 10 μl allows rapid changes of substance concentrations and reactions to external stimuli, using small amounts of fluids. Culture conditions during drug screening experiments can thus be changed dynamically within short time intervals.
4. Results
4.1. Mass transport and microfluidic compartmentalization
The chip layout allows generation of different microenvironments in both spheroid compartments, as well as control of the communication between the embedded cells by directing fluid flow to specific channels. To illustrate the system's capabilities, Fig. 2 shows two possible operation modes together with electrochemical measurements under microfluidic operation, in which each mode was performed in sequence. Hydrogen peroxide (H2O2) in phosphate buffered saline (PBS), which occurs as a reactive species and behaves similarly to oxygen in terms of diffusion, was used as test substance for these diffusion analyses.
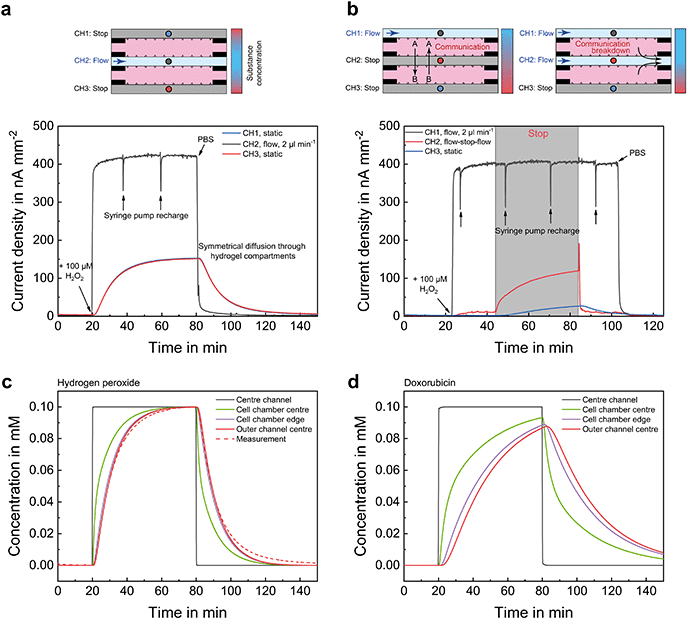 |
| Fig. 2 Mass transport control study. H2O2 in PBS was used as test substance and measured with microsensors located in the centre of each microchannel. (a) Liquid perfusion is exclusively performed within the middle channel leading to identical microenvironments inside both spheroid compartments, reflected by the overlapping sensor signals. (b) Second operation mode in which a constant flow is applied to an outer channel. If perfusion within the middle channel is stopped, concentration gradients form across both compartments permitting communication of different cell types. Application of a flow to the same channel interrupts the communication by fluidic separation. (c) Computational modelling of mass transport for hydrogen peroxide (Dliq = 2.50 × 10−9 m2 s−1, Dgel = 1.875 × 10−9 m2 s−1) diffusion from the centre channel into the cell chamber. The measurement results fit the modelled behaviour very well, confirming that the sensor signals follow the underlying principles, and that mass transport is dominated by diffusion. (d) Computational modelling of the mass transport of a larger molecule, the cancer drug doxorubicin (Dliq = 0.58 × 10−9 m2 s−1, Dgel = 4.35 × 10−10 m2 s−1) into the cell chamber. | |
Experimental study.
Fig. 2a shows the first operation mode in which the two outer channels are kept static, while the middle channel is perfused. This leads to equal substance distribution into both spheroid compartments due to the axial symmetry of the chip. Depending on the diffusivity of the individual solute within the used hydrogel, concentration gradients develop from the centre to the outer walls of the chip. This was demonstrated with an electrochemical measurement, in which all sensors showed a signal close to zero at the beginning, since no oxidizable substance was present in PBS. As soon as H2O2 entered the platform via the middle channel, the sensor located within it showed a rise in signal which was dependent on the analyte concentration and the flow rate. With a lag of around 70 s, the sensors near the outer walls of the chip showed an increase in signal current, as H2O2 molecules diffused through the hydrogel containing compartments leading to a rise in concentration. Thereby, the courses and final values of both sensor signals were similar, indicating a symmetrical substance distribution within the chip and a highly reproducible sensor performance. After pure PBS was perfused again in the middle channel, it took 30 min (t90%–10%) until the hydrogels released all H2O2. Sensor signals increased and decreased according to t(−1/2) matching the one-dimensional analytical solution of Fick's second law.48 Hereby, we show how integrated sensor measurements can enable the assessment of mass transport in organ-on-chip devices.
Fig. 2b shows the case where the supply of nutrients and oxygen is ensured via one outer channel, while the other two channels are kept static without flow. Thus, concentration gradients range over the entire width of the chip. In addition, communication between the compartments can then take place by diffusion of signalling molecules through the static middle channel. Applying a flow to this channel leads to an interruption of the communication and isolates both compartments fluidically. The application of the middle channel for such fluidic separation was validated by a measurement with H2O2, which can be seen as a signalling molecule equivalent with a comparatively high diffusivity due to its smaller size. After adding H2O2 to the outer channel, it diffused through compartment A and reached the middle channel, where liquid flow prevented further diffusion into compartment B. By stopping the flow in the middle channel, the H2O2 entered the static channel through compartment B, as indicated by the increase of the sensor signal within this channel. Re-establishment of the flow resulted in a removal of H2O2 from compartment B and re-isolation of both compartments. Hereby, we show the successful manipulation of mass transport and thus culture conditions by compartmentalization and microfluidics.
Computational study.
Fig. 2c shows the simulated mass transport of a small molecule, e.g. hydrogen peroxide, recapitulating the experiment in Fig. 2a. As expected, there is an almost immediate concentration change in the centre channel and an increasingly slower change, the larger the distance to the centre channel becomes. The dashed line shows the normalized experimental data from Fig. 2a for the hydrogen peroxide measurement in the outer channel. It can be observed that the experimental data follow the simulated curve very closely. The main qualitative difference is observed in the higher signal at the end during the removal of the substance, which can be explained by an ongoing release of substance from the dead ends of the fluid channels that were not modelled. The degree of quantitative match between experiment and simulation lies in the accuracy of the used diffusion coefficients. Data for different combinations of diffusion coefficients and the influence on diffusion times are found in the ESI.† Overall, it can be shown that our sensor data follow the underlying physical principles, which are dominated by diffusion within the cell compartments, and reflect the expected mass-transport situation very well, both qualitatively and quantitatively. Furthermore, it was shown that in situ sensors can provide valuable information by verifying parameters used in computational models, such as the diffusion coefficient, which is extremely challenging to measure but has strong influence on the mass transport.
Fig. 2d shows the same situation as Fig. 2c, just for a much slower diffusing substance, such as the drug doxorubicin. As expected, a much slower concentration change can be observed. Overall, it can be assumed that concentrations of small molecules such as oxygen are fully exchanged in the cell compartments within a typical flow phase of 30 min. For matrix-based 3D cell culture systems, it should be considered that diffusion is comparably slow if there is no forced mass transport, e.g. by flow, and diffusion times even in highly permeable ECM gels are in the range of minutes to hours depending on the molecule size. Further details regarding system geometry, diffusion coefficients and diffusion times are found in the ESI.†
4.2. Oxygen sensor characterization
The applied amperometric oxygen sensor principle relies on the electrochemical reduction of oxygen at the platinum surface. Concentration changes are therefore determined by measuring the mass transport change to the electrode. For this purpose, an optimized chronoamperometric protocol17 was applied (Fig. 3a), which is based on the unique characteristic features of platinum electrochemistry. Initially, platinum oxide is formed at an anodic potential. The formed oxide layer is reduced in the following step by lowering the potential to a cathodic region. As a result, a bare platinum surface is present at the electrode–electrolyte interface, which acts as a catalyst for the oxygen reduction reaction. In a last upward step by 100 mV to achieve faster sensor stabilization, the actual oxygen measurement takes place. A typical current response is shown in Fig. 3a for oxygen concentrations at atmospheric and anoxic conditions. Oxygen-free conditions result in a sensor output close to zero allowing one-point calibration. An approximately 20 μm thick pHEMA-based diffusion limitation membrane is dispensed into the SU-8 polymer rims surrounding the sensor electrodes (Fig. 1c) in order to obtain a defined diffusion profile above the electrode and thereby increase the stability of the sensor response.
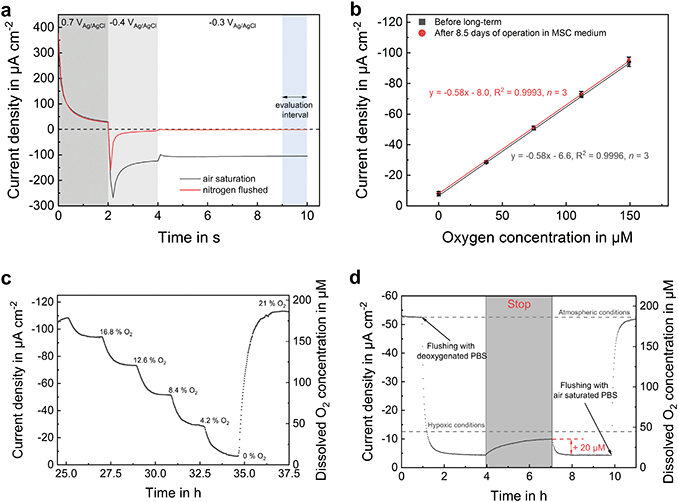 |
| Fig. 3 Oxygen sensor and gas-tightness characterization. Medium flow was applied to the middle channel at 10 μl min−1. Both outer channels were kept static. (a) Sensor response for the chronoamperometric oxygen measurement protocol in air-saturated and nitrogen-flushed PBS. The mean current density of the last second is taken for data evaluation. (b) Oxygen sensor calibration before and after long-term measurement in MSC medium. Error bars are s.d. (c) Transient oxygen calibration. (d) Gas-tightness verification by lowering the oxygen concentration via fluidics and subsequent stopping of the perfusion. Dissolved oxygen concentration remains in hypoxic regime within the spheroid compartment even after 3 h without active supply of oxygen-free electrolyte. | |
By cyclic formation and removal of the oxide layer, the electrode is permanently cleaned during the measurement. This leads to a remarkably high long-term stability of the sensor without measurable drift over one week, even within protein-containing cell culture medium, as demonstrated in Fig. 3b. Sensitivity did not change after more than 8 days of continuous operation. These results underline the stable electrochemical protocol and the protection of the electrodes by the membranes, which successfully prevent electrode fouling and attachment of blocking substances. Additionally, sensitivity can be adjusted by the membrane thickness. In the shown example, the sensitivity was −0.58 μA cm−2 μM−1 with a relative error of 0.8% ± 0.4% before and 1.2% ± 0.4% after long-term measurement (n = 6 × 3, based on 6 different concentration values in 3 independent calibration runs) with respect to the measurement range. All electrodes showed a detection limit below 1 μM (based on 3 σ of the blank). Low standard deviations of the measurement points indicate a highly reproducible measurement of the oxygen concentration within the platform.
4.3. Gas tightness
In many microfluidic systems for culturing and monitoring spheroid cultures that are made of gas-permeable materials (such as PDMS) or open systems (such as hanging droplets), the gas composition in the cell culture medium is regulated by gas exchange with the incubator environment. This is a convenient approach, however unlike the in vivo situation, if a constant and reproducible oxygen concentration is desired for all tissue types in the system, especially if several systems are used in parallel for a high throughput evaluation. We intentionally used gas-impermeable materials to have a targeted control of the gas composition through external gassing of the medium in reservoirs outside the microsystem. In addition, this enables the determination of cellular respiration rates, since the controlled influx of new medium is the only source of oxygen molecules.
The ability of the system to maintain hypoxic conditions within a spheroid compartment was verified with an experiment (Fig. 3d) in which the oxygen concentration was first lowered to below 15 μM by perfusing oxygen-free electrolyte. Upon stopping the flow for 3 h, oxygen has increased to a final concentration of 35 μM, remaining in hypoxic regime. Most likely, this slight increase is caused by oxygen dissolution from microbubbles which remain after the sealing of inlet and outlet ports, rather than by oxygen diffusion through the compartment surrounding materials. However, in practical operation during cell culture, typical stop phases do not last longer than 30 min, which leads to a maximum oxygen concentration increase by 10 μM, if there is no cellular oxygen consumption.
4.4. Formation of breast cancer stem cell spheroids
The cell model used in this work consists of triple-negative breast cancer stem cells, more specifically of the breast cancer stem cell line 1 (BCSC1), one of five extensively characterized BCSC lines isolated from primary invasive tumours of individual patients.3,49,50 BCSCs are of particular interest in the development of personalized chemotherapy approaches for triple-negative breast cancer patients, as they are considered to be responsible for both cancer metastasis and therapy resistance.51
Fig. 4a shows the development of single BCSC1 cells into spheroids with a mean diameter of approximately 50 μm after four days of culture under dynamic fluidic operation in which cell culture medium was perfused through the middle channel of the chip. By variation of different seeding densities, an optimum was found between 0.5 × 106 and 2 × 106 cells per ml, resulting in a total cell number of 3500 to 15
000 cells per platform. In this range, there were enough cells for a sufficiently high measurement signal and at the same time no lack of nutrients occurred, and enough room for growth remained over a time span of at least five days. Due to the axial symmetry along the central channel, the same concentration gradients develop in both cell chambers, as confirmed in Fig. 2a. Thus, spheroids in compartment A and B experience the same culture conditions during growth and do not differ in size or amount (Fig. 4b and c). Furthermore, on-chip grown spheroids have the same morphology and size distribution as within a standard well-plate format (Fig. 4c). Typical for cultures in which spheroids develop from ECM-embedded single cells, the spheroid size distribution varies more widely compared to methods where individual spheroids are formed from aggregation of a defined number of cells using microfluidic structures and techniques.52 In order to study spheroids with a uniform size distribution or larger diameter for higher metabolic activity instead of the development from single cells, spheroids can be grown outside the platform, sorted by a cell strainer with defined mesh size and transferred into the platform.
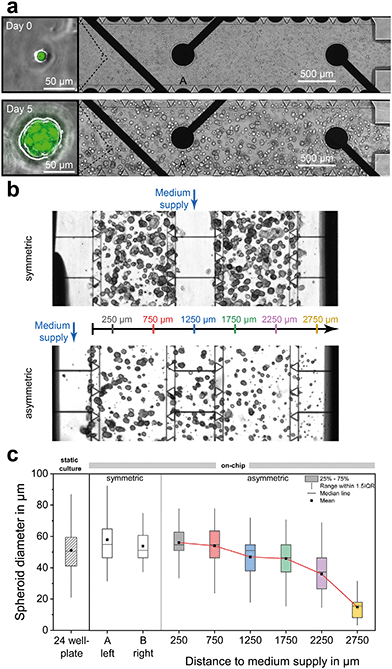 |
| Fig. 4 On-chip tumour spheroid formation. (a) Phase-contrast micrographs of single breast cancer stem cells (BCSC1) embedded in 75% Matrigel right after seeding and gelation at a seeding density of 2 × 106 cells ml−1. During dynamic supply with cell culture medium over days, spheroids develop within compartments. Scale bar, 500 μm. Inserts show micrograph overlays (phase-contrast and green fluorescence) of a single cell and a spheroid. Scale bars, 50 μm. (b) Phase-contrast micrographs showing spheroids on day 5 of culture which have grown under two different medium supply situations. Seeding densities: 1.5 × 106 cells ml−1 (top) 0.5 × 106 cells ml−1 (bottom). (c) Comparison of spheroid diameter distribution for static conditions within a traditional well-plate (seeding density: 1.5 × 106 cells ml−1) and for both dynamic situations on-chip (n ≥ 58). | |
The lower micrograph of Fig. 4b shows an example where the whole inner volume of the platform was filled with cell-laden Matrigel, with the exception of one outer channel which was used for medium perfusion. This asymmetric supply with fresh medium results in substance gradients across the entire width of the platform, as demonstrated in Fig. 2b. Previous studies demonstrated for adherent cell monolayers53 and matrix-embedded cancer cells54 that such gradients lead to nutrient-dependent metabolic profiles and cell viability. Consequently, spheroids developing closer to the supply were exposed to more favourable growth conditions than cells growing more distant, as reflected in their structure. This is also evident from the spheroid diameter (see Fig. 4c), which was significantly smaller when the spheroids were located more than 1.75 mm from the supply channel. Since in all experiments we observed that BCSC1 spheroids grew largely independent of the oxygen concentration, we speculate that other factors such as the accumulation of waste products leads to inhibition of spheroid growth. These findings underline the importance of selecting appropriate scaling of cell culture environments, considering the diffusion properties of nutrients and cellular waste products within the used ECM, and verifying culture conditions in situ with integrated sensors.
4.5. Measurement of cellular oxygen consumption
Fig. 5 shows the signal of an oxygen sensor located in the centre of the perfusion channel, in between of both cell compartments. During growth, the culture medium was aspirated through the platform in stop/flow cycles with each phase lasting for 30 min. In the stop phases, active supply of medium with atmospheric oxygen concentration was stopped. The change in oxygen concentration within the chip was then solely caused by the cells and showed a steady decrease over time (Fig. 5b). Oxygen sensor consumption during a stop phase was only 0.3% with respect to the overall oxygen content within the chip and can therefore be neglected. During flow phases, cells were re-supplied with oxygen-rich medium, which passed over the sensor at 2 μl min−1, bringing its signal back to baseline. Since the signal is superimposed by a flow-dependent offset, the sensor showed a higher oxygen concentration than the expected 188.7 μM at atmospheric conditions (19.9% O2, 5% CO2). The gap in the oxygen measurement after addition of the drug resulted from electrical disconnection of the system from the potentiostat to characterize the state of the cells under the microscope and subsequent recalibration of the biosensors.
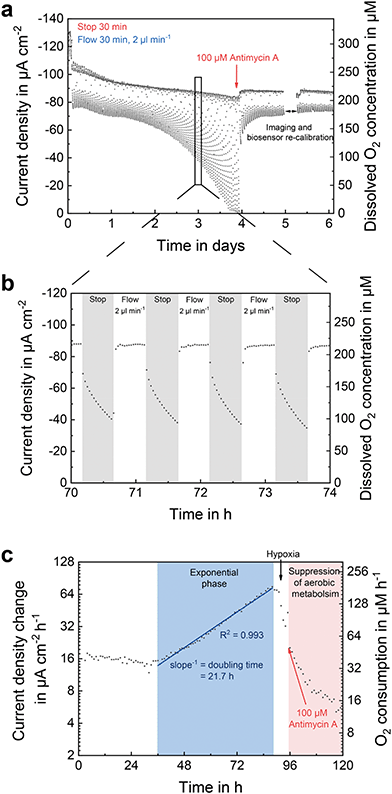 |
| Fig. 5 In-line oxygen monitoring. Oxygen monitoring in the central perfusion channel during the formation of breast cancer stem cell (BCSC1) spheroids at a seeding density of 2 × 106 cells ml−1. MSC cell culture medium was perfused in stop/flow cycles. Oxygen concentration was measured every 2 minutes. (a) Oxygen measurement over 6 days during spheroid formation including exposure to the metabolism altering drug antimycin A. (b) Section over 4 hours on day 3 of the same measurement. Each measurement point represents the oxygen concentration at the given time. (c) Oxygen consumption over time, whereby the slopes of the linear fits of the last 5 concentration values of each stop phase were taken for data evaluation. | |
Over time, the oxygen demand of the cells increased, due to proliferation and exponential growth of the cell number within the forming spheroids. This is also reflected in the decrease of oxygen concentration during the stop phases in the course of culture time (Fig. 5a). After 3.75 days, all available oxygen molecules were metabolized during the stop phases, as indicated by a sensor current of −1.2 μA cm−2, which represents 1.5% of atmospheric conditions matching the sensor output at anoxic conditions during calibration (Fig. 3a). Oxygen consumption increased with a doubling time of 21.7 h (Fig. 5c). After 4 days of culture, 100 μM antimycin A was added to the cell culture medium leading to an abrupt rise in oxygen concentration to atmospheric conditions. As antimycin A inhibits mitochondrial electron transfer,55 the aerobic cell metabolism was suppressed, as shown by the drastic decrease in oxygen consumption. A cellular response to the drug occurred within a 1 hour stop/flow cycle (Fig. 5a). By integration of oxygen sensors into the perfusion channel of such dynamic cell culture systems, the oxygen concentration of the inflowing medium can be monitored. At the same time, oxygen consumption rates can be determined very accurately and used for revealing drug-induced effects on the respiratory metabolism.
4.6. Pericellular monitoring and exposure to doxorubicin
While the previous example covered the oxygen concentration change within the medium supply channel of the system, Fig. 6a shows a long-term oxygen measurement in the centre of a spheroid compartment in close vicinity to the spheroids. By integration into the cell compartment, the sensor's signal is not flow-dependent and indicates the actual oxygen concentration experienced by the cells even during flow phases. During the first three days of culture, flow phases re-established conditions at 19.9% oxygen. Afterwards, this baseline was no longer reached due to the high oxygen uptake by the cells, as shown in Fig. 6b, where stop/flow sections at the end of the respective day are summarized. This observation underlines the importance of positioning sensors for oxygen monitoring within the cell embedding ECM to access the realistic culture microenvironment experienced by the cells even during active medium perfusion.
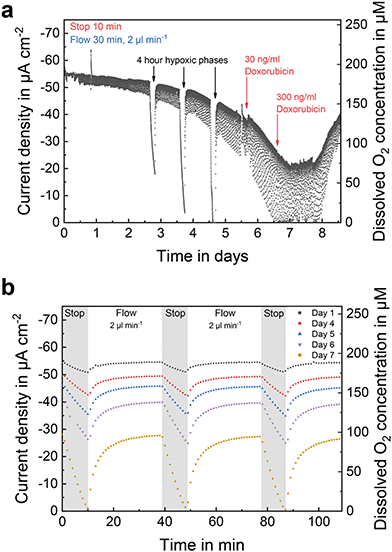 |
| Fig. 6 Long-term oxygen monitoring. (a) Pericellular oxygen monitoring within a spheroid compartment at a seeding density of 1.5 × 106 cells ml−1. Oxygen concentration was measured every minute. During measurement, cells were exposed to three hypoxic phases and two exposures to different concentrations of doxorubicin. (b) Course of the oxygen concentration at the respective day of culture. | |
During spheroid development, three experimental phases were included, in which the supply with fresh medium was interrupted for 4 h (Fig. 6a) in order to mimic acute cyclic hypoxia as seen in real tumours during accelerated growth compared to healthy tissue.56 After each phase, the oxygen concentration returned to values as before stopping the flow, and the cellular oxygen consumption did not change. Since the oxygen consumption was also not affected, it is shown that BCSC1 cells have an adapted metabolism and tolerate hypoxia well, whereas oxygen consumption does not depend on the overall oxygen concentration.
On the sixth day of culture (Fig. 6a), 30 ng ml−1 of the chemotherapeutic drug doxorubicin, frequently used for the treatment of breast cancer and based on the intercalation into the DNA,57 was added to the cell culture medium. At this critical concentration, BCSC1 cells show a significantly inhibited cell proliferation when cultivated in 2D.49 In contrast, the BCSC1 spheroids showed no change in metabolism even more than 24 h post drug exposure. After increasing that concentration by a factor of 10, the oxygen concentration course changed to higher values. As verified by microscopy the cells began to die, whereby the oxygen concentration within the compartment successively began to rise. Comparing the onset of action on oxygen uptake for antimycin A (Fig. 5a) and doxorubicin (Fig. 6a) indicates, that antimycin A induces a drug response much faster reflecting the differences in the mechanisms of action. Such acquisition of additional information on pharmacodynamics highlights the fundamental benefit of sensor-based pericellular metabolic monitoring. In comparison to a traditional experiment where cells are exposed to a drug, re-seeded and proliferation is monitored over days, our method allows real-time, online and quantitative access to cell metabolism.
4.7. Glucose and lactate monitoring
Biosensors are based on the equimolar conversion of glucose or lactate into H2O2 inside the pHEMA-based hydrogel, which is immobilized on the electrodes and entraps the enzymes glucose oxidase or lactate oxidase, respectively. The concentration of the analyte is then determined by the current density for the oxidation of the formed H2O2 at a potential of 0.5 VAg/AgCl. Sensor calibration for glucose and lactate sensors are shown in Fig. 7a and b, respectively. During measurement, a blank-signal was recorded by an electrode without embedded enzyme, in order to subtract unspecific background signals. The developed biosensors showed a highly linear, reversible and selective behaviour within the physiological range. The limit of detection was 7.6 μM (±3.7 μM, n = 3) in case of glucose and 6.1 μM (±4.2 μM, n = 3) for lactate sensors. Sensitivity, limit of detection and linear range can be adjusted for the desired application by varying the height of the diffusion-limiting membrane within the membrane stack. An individual calibration was performed prior and after each experiment. Drift correction was performed under the assumption of linear sensitivity decay for long-term measurement evaluation.
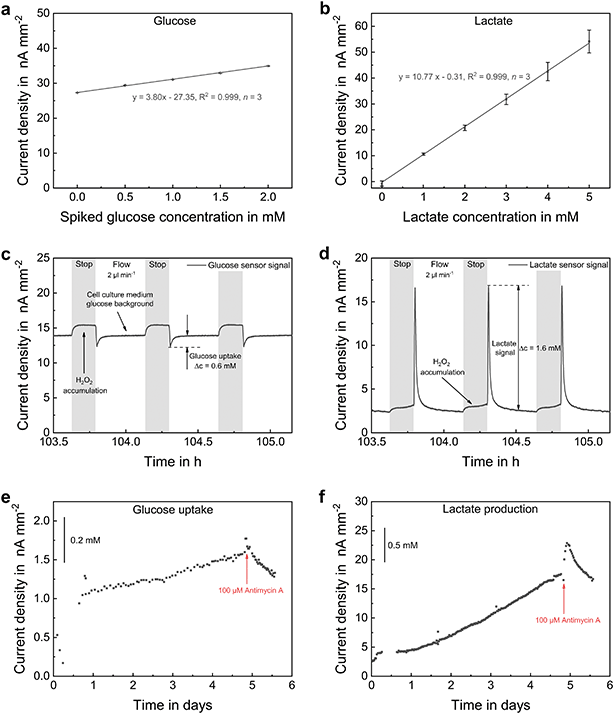 |
| Fig. 7 Biosensor characterization and long-term measurements. (a) Spiked glucose biosensor calibration before and after operation within long-term culture. Error bars are s.d. (b) Lactate biosensor calibration before and after operation within long-term culture. Error bars are s.d. (c) Glucose and (d) lactate signal simultaneously recorded during microfluidic operation (stop 10 min/flow 30 min). (e) Glucose uptake and (f) lactate production during long-term culture and their change upon addition of 100 μM antimycin A. | |
Fig. 7c and d shows typical biosensor signals during spheroid cultivation. Due to the high glucose background concentration in MSC medium and the cellular lactate secretion, both analytes were always present and converted by the enzyme membranes. This resulted in a H2O2 accumulation over the sensor electrodes and a signal increase during stop phases. Biosensors were placed outside the cell compartments downstream the microchannel in order to prevent H2O2 diffusion into the inner volume of the platform. At the beginning of a flow phase, the consumed medium was removed from the culture volume and passed over the sensor. As glucose was metabolized by the cells, a signal drop relative to the background was detected, and its uptake was quantified by subtraction of both signal values. In case of lactate, a distinct signal peak appeared at the initiation of perfusion. Both glucose consumption and lactate secretion increased with rising cell density and decreased again after the addition of a lethal dose of antimycin A. Compared to the example in Fig. 5, the stop phase duration was reduced to 10 minutes, so that oxygen-rich medium was perfused more frequently. Consequently, the oxygen concentration was within the normoxic range throughout the entire culturing duration (Fig. 6a). However, a remarkably high lactate signal was measured indicating that the cells show a substantial nonoxidative metabolism of glucose besides aerobic respiration, as known to be a typical characteristic of cancerous tissue.58 Regarding the ratio of glucose consumption (Fig. 6e) to lactate production (Fig. 6f), we observed a slightly lower glucose consumption than a 1
:
2 ratio. It has to be noted that the absolute quantification of pericellular levels in the matrix-based culture by microfluidic measurement is highly complex. Glucose has a slower diffusion in comparison to lactate because it is the larger molecule, and glucose is supplied with the medium while lactate is only produced by the cells. During the stop phase, exchange of the medium with the gel compartments occurs only near the gel edges. Therefore, our glucose measurements may represent a slight underestimation of the actual cellular consumption. Still, a quantitative comparison of the two metabolite concentrations is possible. Further, we have demonstrated for the first time that a quantitative and online on-chip measurement of these parameters from heterogeneous matrix-based cultures is possible using biosensors that are located outside the cell culture areas.
5. Conclusions
We have developed a microfluidic organ-on-chip platform with integrated electrochemical microsensor arrays for compartmentalized matrix-based organoid cultivation from single stem cells for acquisition of relevant metabolic parameters in real-time. The developed transparent technology is based on a multi-layer microfabrication process for an advanced, modular microfluidic unit on a glass chip. Any matrix-based culture can be integrated by simple, barrier structure-guided filling of the microliter-scale cell compartments with standard laboratory equipment. Introduction of single triple-negative breast cancer stem cells into the low-volume microsystem results in a heterogeneous spheroid culture from a small cell number of less than 15
000 cells during microfluidic operation. These patient-derived stem cells recapitulate the individual patient's tumour in vitro and are therefore an ideal model for testing both drug efficacy and tolerance towards individualized chemotherapy. In many organ-on-chip systems culture conditions and cell metabolism are not accessible on a pericellular level, and can be neither controlled nor verified in situ.
The chip design, comprising two cell compartments with adjacent microchannels, permits the incorporation of co-cultures in combination with individual microfluidic accessibility. Notably, unlike PDMS-based or open systems, our gas-tight system allows the generation of hypoxia on-chip and the control of culture conditions entirely via the medium. Such a design enables replication of a microenvironment as found in tumours. We demonstrated the flow-controlled fluidic separation of the compartments, which can be utilized, e.g., for investigation of the intercellular communication. Computational modelling confirmed that sensor signals precisely reflect the mass transport by diffusion. Hereby, we have shown how integrated sensors can contribute to design considerations of organ-on-chip systems. Hypoxia is essential in cancer, and we have shown how culture conditions can influence spheroid growth on chip. Integrated sensors can deliver important real-time information on culture conditions and cell metabolism, which is currently still underrepresented in the increasingly popular microphysiological and organ-on-chip systems.
We developed precise and long-term stable electrochemical sensors for oxygen, lactate and glucose and successfully applied them over more than one week of culture. While other systems have focused on single or multiple spheroids, we have now demonstrated that it is possible to determine consumption and production rates from heterogeneous, matrix-based 3D culture reversibly and absolute quantitatively, enabling conclusions about cell state in real-time. Unlike classical macroscopic cell culture models, the high ratio of cell number to medium volume resulted in high concentration changes of metabolites within short time intervals. This provides a higher temporal resolution of cellular metabolic changes and, e.g. the exponential growth phase of the spheroids can be determined directly, beyond microscopy and optical inspection. Our results also show the implications for sensor locations in matrix-culture. Sensor integration into perfusion channels can be used for controlling the oxygen concentration of the medium supply. Sensors in the cell chambers measure pericellular values, while sensors in the microfluidics monitor the medium supply but also can be used to extract meaningful metabolic signals, especially biosensors which are preferably located outside the cell compartments.
Besides monitoring of culture conditions, sensor array measurements can reveal cellular effects to drug exposure. Substantial differences in drug resistance of spherically grown patient-derived, triple-negative breast cancer stem cells compared to their two-dimensional equivalents were observed based on cellular metabolic activity measured by embedded sensors. The ability to perform such investigations is essential in the development of personalized treatment options. In contrast to endpoint analyses, cell metabolism can be observed continuously. Since culture conditions significantly affect the properties of cancer and other cells, standardization of their microenvironment is essential and can be greatly enhanced by means of such sensor integration. In summary, by accurate monitoring of metabolic parameters in a clinically relevant tumour organoid model, we have shown how our sensor-integrated organ-on-chip platform extends the state-of-the-art in the context of patient-specific chemotherapy towards personalized medicine.
Author contributions
Conceptualization: JD, JK, JM, AW; investigation: JD, HM, AW; writing – original draft preparation: JD; writing – review & editing: JD, JK, HM, JM, GAU, AW; visualization: JD, AW; supervision: JK, JM, GAU, AW.
Conflicts of interest
The Baden-Württemberg Stiftung gGmbH has filed a patent application related to this work. AW, JD, JK, JM and GAU are co-inventors of this patent application.
Acknowledgements
Financing by Baden-Württemberg Stiftung within the project MIVT-1, MEMONO, is gratefully acknowledged.
References
- A. van den Berg, C. L. Mummery, R. Passier and A. D. van der Meer, Lab Chip, 2019, 19, 198–205 RSC
.
- V. van Duinen, S. J. Trietsch, J. Joore, P. Vulto and T. Hankemeier, Curr. Opin. Biotechnol., 2015, 35, 118–126 CrossRef CAS PubMed
.
- E. Metzger, S. S. Stepputtis, J. Strietz, B. T. Preca, S. Urban, D. Willmann, A. Allen, F. Zenk, N. Iovino, P. Bronsert, A. Proske, M. Follo, M. Boerries, E. Stickeler, J. Xu, M. B. Wallace, J. A. Stafford, T. Kanouni, J. Maurer and R. Schüle, Cancer Res., 2017, 77, 5900–5912 CrossRef CAS PubMed
.
- L. A. Low, C. Mummery, B. R. Berridge, C. P. Austin and D. A. Tagle, Nat. Rev. Drug Discovery, 2021, 20, 345–361 CrossRef CAS PubMed
.
- M. Kapałczyńska, T. Kolenda, W. Przybyła, M. Zajączkowska, A. Teresiak, V. Filas, M. Ibbs, R. Bliźniak, Ł. Łuczewski and K. Lamperska, Arch. Med. Sci., 2018, 14, 910–919 Search PubMed
.
- A. Sontheimer-Phelps, B. A. Hassell and D. E. Ingber, Nat. Rev. Cancer, 2019, 19, 65–81 CrossRef CAS PubMed
.
- C. K. Byun, K. Abi-Samra, Y. K. Cho and S. Takayama, Electrophoresis, 2014, 35, 245–257 CrossRef CAS PubMed
.
- K. Renggli, N. Rousset, C. Lohasz, O. T. P. Nguyen and A. Hierlemann, Adv. Biosyst., 2019, 3, 1900018 CrossRef PubMed
.
- J. H. Sung, C. Kam and M. L. Shuler, Lab Chip, 2010, 10, 446–455 RSC
.
- S. J. Trietsch, G. D. Israëls, J. Joore, T. Hankemeier and P. Vulto, Lab Chip, 2013, 13, 3548–3554 RSC
.
- C. P. Huang, J. Lu, H. Seon, A. P. Lee, L. A. Flanagan, H. Y. Kim, A. J. Putnam and N. L. Jeon, Lab Chip, 2009, 9, 1740–1748 RSC
.
- Y. C. Toh, C. Zhang, J. Zhang, Y. M. Khong, S. Chang, V. D. Samper, D. van Noort, D. W. Hutmacher and H. Yu, Lab Chip, 2007, 7, 302–309 RSC
.
- Y. Wang, L. Wang, Y. Zhu and J. Qin, Lab Chip, 2018, 18, 851–860 RSC
.
- I. K. Zervantonakis, S. K. Hughes-Alford, J. L. Charest, J. S. Condeelis, F. B. Gertler and R. D. Kamm, Proc. Natl. Acad. Sci. U. S. A., 2012, 109, 13515–13520 CrossRef PubMed
.
- D. Liu, S. Chen and M. Win Naing, Biotechnol. Bioeng., 2021, 118, 542–554 CrossRef CAS PubMed
.
- J. Kieninger, A. Weltin, H. Flamm and G. A. Urban, Lab Chip, 2018, 18, 1274–1291 RSC
.
- J. Kieninger, K. Aravindalochanan, J. A. Sandvik, E. O. Pettersen and G. A. Urban, Cell Proliferation, 2014, 47, 180–188 CrossRef CAS PubMed
.
- Y. S. Zhang, J. Aleman, S. R. Shin, T. Kilic, D. Kim, S. A. M. Shaegh, S. Massa, R. Riahi, S. Chae, N. Hu, H. Avci, W. Zhang, A. Silvestri, A. S. Nezhad, A. Manbohi, F. De Ferrari, A. Polini, G. Calzone, N. Shaikh, P. Alerasool, E. Budina, J. Kang, N. Bhise, J. Ribas, A. Pourmand, A. Skardal, T. Shupe, C. E. Bishop, M. R. Dokmeci, A. Atala and A. Khademhosseini, Proc. Natl. Acad. Sci. U. S. A., 2017, 114, E2293–E2302 CrossRef CAS PubMed
.
- D. Bavli, S. Prill, E. Ezra, G. Levy, M. Cohen, M. Vinken, J. Vanfleteren, M. Jaeger and Y. Nahmias, Proc. Natl. Acad. Sci. U. S. A., 2016, 113, E2231–E2240 CrossRef CAS PubMed
.
- C. Wenzel, B. Riefke, S. Gründemann, A. Krebs, S. Christian, F. Prinz, M. Osterland, S. Golfier, S. Räse, N. Ansari, M. Esner, M. Bickle, F. Pampaloni, C. Mattheyer, E. H. Stelzer, K. Parczyk, S. Prechtl and P. Steigemann, Exp. Cell Res., 2014, 323, 131–143 CrossRef CAS PubMed
.
- C. J. Ochs, J. Kasuya, A. Pavesi and R. D. Kamm, Lab Chip, 2014, 14, 459–462 RSC
.
- H. Zirath, M. Rothbauer, S. Spitz, B. Bachmann, C. Jordan, B. Müller, J. Ehgartner, E. Priglinger, S. Mühleder, H. Redl, W. Holnthoner, M. Harasek, T. Mayr and P. Ertl, Front. Physiol., 2018, 9, 815 CrossRef PubMed
.
- A. Weltin, S. Hammer, F. Noor, Y. Kaminski, J. Kieninger and G. A. Urban, Biosens. Bioelectron., 2017, 87, 941–948 CrossRef CAS PubMed
.
- J. Marzioch, J. Kieninger, A. Weltin, H. Flamm, K. Aravindalochanan, J. A. Sandvik, E. O. Pettersen, Q. Peng and G. A. Urban, Lab Chip, 2018, 18, 3353–3360 RSC
.
- P. Mestres and A. Morguet, Expert Opin. Drug Discovery, 2009, 4, 785–797 CrossRef CAS PubMed
.
- C. Gehre, M. Flechner, S. Kammerer, J. H. Küpper, C. D. Coleman, G. P. Püschel, K. Uhlig and C. Duschl, Sci. Rep., 2020, 10, 13700 CrossRef CAS PubMed
.
- E. Tanumihardja, R. H. Slaats, A. D. van der Meer, R. Passier, W. Olthuis and A. van den Berg, ACS Sens., 2021, 6, 267–274 CrossRef CAS PubMed
.
- H. Flamm, J. Kieninger, A. Weltin and G. A. Urban, Biosens. Bioelectron., 2015, 65, 354–359 CrossRef CAS PubMed
.
- E. Tanumihardja, A. P. Rodríguez, J. T. Loessberg-Zahl, B. Mei, W. Olthuis and A. van den Berg, Sens. Actuators, B, 2021, 334, 129631 CrossRef CAS
.
- T. Liang, C. Gu, Y. Gan, Q. Wu, C. He, J. Tu, Y. Pan, Y. Qiu, L. B. Kong, H. Wan and P. Wang, Sens. Actuators, B, 2019, 301, 127004 CrossRef CAS
.
- A. Weltin, K. Slotwinski, J. Kieninger, I. Moser, G. Jobst, M. Wego, R. Ehret and G. A. Urban, Lab Chip, 2014, 14, 138–146 RSC
.
- F. Hafner, Biosens. Bioelectron., 2000, 15, 149–158 CrossRef CAS PubMed
.
- O. Y. F. Henry, R. Villenave, M. J. Cronce, W. D. Leineweber, M. A. Benz and D. E. Ingber, Lab Chip, 2017, 17, 2264–2271 RSC
.
- B. M. Maoz, A. Herland, O. Y. F. Henry, W. D. Leineweber, M. Yadid, J. Doyle, R. Mannix, V. J. Kujala, E. A. Fitzgerald, K. K. Parker and D. E. Ingber, Lab Chip, 2017, 17, 2294–2302 RSC
.
- E. Naumovska, G. Aalderink, C. W. Valencia, K. Kosim, A. Nicolas, S. Brown, P. Vulto, K. S. Erdmann and D. Kurek, Int. J. Mol. Sci., 2020, 21, 4964 CrossRef CAS PubMed
.
- L. M. Griep, F. Wolbers, B. de Wagenaar, P. M. ter Braak, B. B. Weksler, I. A. Romero, P. O. Couraud, I. Vermes, A. D. van der Meer and A. van den Berg, Biomed. Microdevices, 2013, 15, 145–150 CrossRef CAS PubMed
.
- P. M. Misun, J. Rothe, Y. R. F. Schmid, A. Hierlemann and O. Frey, Microsyst. Nanoeng., 2016, 2, 16022 CrossRef CAS PubMed
.
- J. Kieninger, Y. Tamari, B. Enderle, G. Jobst, J. A. Sandvik, E. O. Pettersen and G. A. Urban, Biosensors, 2018, 8, 44 CrossRef PubMed
.
- S. A. M. van Stroe-Biezen, F. M. Everaerts, L. J. J. Janssen and R. A. Tacken, Anal. Chim. Acta, 1993, 273, 553–560 CrossRef CAS
.
- A. J. van Stroe and L. J. J. Janssen, Anal. Chim. Acta, 1993, 279, 213–219 CrossRef
.
- M. A. Nejad, P. Umstätter and H. M. Urbassek, J. Mol. Model., 2020, 26, 54 CrossRef CAS PubMed
.
- A. Colom, R. Galgoczy, I. Almendros, A. Xaubet, R. Farré and J. Alcaraz, J. Biomed. Mater. Res., Part A, 2014, 102, 2776–2784 CrossRef PubMed
.
-
J. Dornhof, J. Kieninger, J. Maurer, G. A. Urban and A. Weltin, in 20th International Conference on Solid-State Sensors, Actuators and Microsystems and Eurosensors XXXIII, (Transducers and Eurosensors XXXIII), 2019, pp. 1106–1108 Search PubMed
.
- H. Liu, Y. Wang, K. Cui, Y. Guo, X. Zhang and J. Qin, Adv. Mater., 2019, 31, 1902042 CrossRef CAS PubMed
.
- A. Weltin, J. Kieninger, B. Enderle, A. K. Gellner, B. Fritsch and G. A. Urban, Biosens. Bioelectron., 2014, 61, 192–199 CrossRef CAS PubMed
.
- B. Bohl, R. Steger, R. Zengerle and P. Koltay, J. Micromech. Microeng., 2005, 15, 1125–1130 CrossRef
.
- B. Rubehn, S. B. E. Wolff, P. Tovote, A. Lüthi and T. Stieglitz, Lab Chip, 2013, 13, 579–588 RSC
.
- Y. T. Kim, S. Bohjanen, N. Bhattacharjee and A. Folch, Lab Chip, 2019, 19, 3086–3093 RSC
.
- X. Li, J. Strietz, A. Bleilevens, E. Stickeler and J. Maurer, Int. J. Mol. Sci., 2020, 21, 404 CrossRef CAS PubMed
.
- J. Strietz, S. S. Stepputtis, M. Follo, P. Bronsert, E. Stickeler and J. Maurer, Int. J. Mol. Sci., 2021, 22, 1808 CrossRef CAS PubMed
.
- T. Reya, S. J. Morrison, M. F. Clarke and I. L. Weissman, Nat. Rev. Cancer, 2001, 414, 105–111 CAS
.
- K. Moshksayan, N. Kashaninejad, M. E. Warkiani, J. G. Lock, H. Moghadas, B. Firoozabadi, M. S. Saidi and N. T. Nguyen, Sens. Actuators, B, 2018, 263, 151–176 CrossRef CAS
.
- S. Perottoni, N. G. B. Neto, C. Di Nitto, R. I. Dmitriev, M. T. Raimondi and M. G. Monaghan, Lab Chip, 2021, 21, 1395–1408 RSC
.
- J. M. Ayuso, M. Virumbrales-Muñoz, A. Lacueva, P. M. Lanuza, E. Checa-Chavarria, P. Botella, E. Fernández, M. Doblare, S. J. Allison, R. M. Phillips, J. Pardo, L. J. Fernandez and I. Ochoa, Sci. Rep., 2016, 6, 36086 CrossRef CAS PubMed
.
- S. Tzung, K. M. Kim, G. Basañez, C. D. Giedt, J. Simon, J. Zimmerberg, K. Y. J. Zhang and D. M. Hockenbery, Nat. Cell Biol., 2001, 3, 183–191 CrossRef CAS PubMed
.
- K. Saxena and M. K. Jolly, Biomolecules, 2019, 9, 339 CrossRef CAS PubMed
.
- C. J. Lovitt, T. B. Shelper and V. M. Avery, BMC Cancer, 2018, 18, 41 CrossRef PubMed
.
- R. A. Gatenby and R. J. Gillies, Nat. Rev. Cancer, 2004, 4, 891–899 CrossRef CAS PubMed
.
Footnote |
† Electronic supplementary information (ESI) available: Describing the computational modelling of mass transport. Video S1: filling of cell compartment with standard laboratory pipette. Video S2: microscopic view of microstructure-guided filling of cell compartment with Matrigel. See DOI: 10.1039/d1lc00689d |
|
This journal is © The Royal Society of Chemistry 2022 |
Click here to see how this site uses Cookies. View our privacy policy here.