DOI:
10.1039/D1LC00603G
(Critical Review)
Lab Chip, 2022,
22, 1068-1092
Oxygen control: the often overlooked but essential piece to create better in vitro systems
Received
9th July 2021
, Accepted 20th January 2022
First published on 21st January 2022
Abstract
Variations in oxygen levels play key roles in numerous physiological and pathological processes, but are often not properly controlled in in vitro models, introducing a significant bias in experimental outcomes. Recent developments in microfluidic technology have introduced a paradigm shift by providing new opportunities to better mimic physiological and pathological conditions, which is achieved by both regulating and monitoring oxygen levels at the micrometre scale in miniaturized devices. In this review, we first introduce the nature and relevance of oxygen-dependent pathways in both physiological and pathological contexts. Subsequently, we discuss strategies to control oxygen in microfluidic devices, distinguishing between engineering approaches that operate at the device level during its fabrication and chemical approaches that involve the active perfusion of fluids oxygenated at a precise level or supplemented with oxygen-producing or oxygen-scavenging materials. In addition, we discuss readout approaches for monitoring oxygen levels at the cellular and tissue levels, focusing on electrochemical and optical detection schemes for high-resolution measurements directly on-chip. An overview of different applications in which microfluidic devices have been utilized to answer biological research questions is then provided. In the final section, we provide our vision for further technological refinements of oxygen-controlling devices and discuss how these devices can be employed to generate new fundamental insights regarding key scientific problems that call for emulating oxygen levels as encountered in vivo. We conclude by making the case that ultimately emulating physiological or pathological oxygen levels should become a standard feature in all in vitro cell, tissue, and organ models.
Introduction
The tissue concentration of oxygen plays an important role in a variety of cellular processes in both normal and pathophysiological conditions, ranging from embryonic development to cancer as prototypical examples. Yet, in in vitro studies involving cells and tissues in classical systems, this role is often insufficiently taken into account, if not entirely neglected.1 This leads to in vitro studies performed under non-reproducible, non-physiological conditions with well-documented unwanted effects on experimental outcomes.1,2 Therefore, it is essential to create and employ in vitro models that include active and accurate control of the oxygen tension. In recent years, engineers have been addressing this challenge and designed numerous systems to regulate the oxygen tension in advanced 2D and 3D in vitro tissue models, with excellent potential for answering biological research questions. There is indeed increasing recognition of the importance of hypoxia in a wide variety of diseases and disorders as well as with respect to the action of many therapeutic agents. Examples range from the well-studied role of hypoxia in the progression of cancer3 to onset of fibrotic diseases4 and transient ischemic attacks (TIAs)5 or myocardial infarction,6 and from (cancer) immunotherapy7 to hypoxia-targeted drug delivery approaches.8 Furthermore, essentially all organs in the human body are exposed to different oxygen tensions and some to very low oxygen levels, and to gradients of oxygen across tissues. Hence, also non-pathological biological processes are impacted by low oxygen tension, including embryonic development,9 articular cartilage function,10 wound healing11 and intestinal homeostasis,12 all of which must be taken into account to create proper and fully functional in vitro models for human organs or organ functions.
Despite the immense potential of advanced engineered systems, researchers in life sciences are continuing to rely to a large extent on classical methods for studying hypoxia such as large incubators with mostly non-physiologically high oxygen percentage, a variety of hypoxic chambers, and various animal models. Yet, all these systems, while they have proven value, also exhibit well-identified limitations and flaws. In 2D cultures growing in hypoxic chambers, the natural 3D environment of cells is not present, leading to many molecular and cellular adaptations and alterations.13 Furthermore, oxygen gradients cannot be incorporated in such systems. Hence, simple models in hypoxic chambers do not accurately or even properly mimic physiological processes happening in vivo. Crucially, the oxygen tension is not regulated at the micrometre scale, or in other words at the scale of the cellular model, but rather at the level of the system, risking the introduction of significant variations within the system. Animal models, on the contrary, are more complex and incorporate these physiological differences in oxygen tension and gradients thereof, but they tend to be expensive, suffer from a low throughput, are difficult to control or monitor at the molecular or cellular level and are generally not compatible with the study of dynamic biological processes in real-time. In addition, they are ethically problematic, subjected to strict regulation, like the 3 Rs legislation in the European Union and similar regulations in the US.14–16 Finally, there are significant worries regarding the extrapolation of results from animal models to the human situation due to essential physiological differences and the poor ability of rodents to model human diseases.17 Importantly, these differences include variations with respect to oxygen levels.18,19
The advent of microfluidic technology is causing a paradigm shift. The inherent flaws of conventional 2D cellular and animal models can be overcome by exploiting novel technological advances for the (bio)engineering and application of microfabricated and microfluidic in vitro models. Those miniaturized models, that also encompass organ-on-chip devices,20 offer new opportunities to engineer and control the cell microenvironment,21 which is notably brought about by the fact that surface phenomena dominate over bulk ones at the micrometre scale and that flows are laminar.22 The cell culture environment can be tuned at the micrometre scale, including a variety of physical and chemical parameters, such as gas tensions and temperatures. Similarly, stable gradients of those parameters can be created to better reproduce tissue-specific in vivo conditions.23,24
The purpose of this review is to help build the bridge between engineers and life science researchers with respect to the use of advanced in vitro approaches with oxygen levels as found in physiological or pathological conditions. By informing about the advantages over alternative approaches and discussing main opportunities and key challenges for translational research, the potential of these systems for all-human disease models will be elucidated to life scientists and engineers alike. In this review, we will highlight several of the many roles of oxygen in health and disease(s), at the molecular, cellular, organ and systemic levels, and discuss methods to control oxygen in microfluidic devices. We will then review studies that have implemented these methods and critically evaluate currently employed readout approaches. Finally, we share our vision for the future and report on main opportunities and key challenges that we have identified in the field.
Importance of oxygen in biological processes: from the molecular to the tissue level
Pathways involving hypoxia inducible factors (HIFs)
At the cellular level, several signalling pathways are involved in oxygen sensing, which differ in their sensitivity to the severity and duration of oxygen deprivation.25 The most well-known pathway involves the hypoxia-inducible factors (HIFs), which are an evolutionarily conserved class of transcription factors and key mediators of the cellular response to hypoxia (Fig. 1).26 There exist three HIF factors, which are transcriptional heterodimer complexes composed of a conditionally available α subunit (HIF-1α, HIF-2α, and HIF-3α) and an always present β subunit, the aryl hydrocarbon receptor nuclear translocator (ARNT). All α subunits contain an oxygen-dependent degradation domain (ODD). Under normoxic conditions, α subunits are hydroxylated at proline residues in their ODD by enzymes called prolyl hydroxylase domain (PHD) proteins, leading to their recognition by the von Hippel–Lindau (VHL) E3 ubiquitin ligase. VHL attaches a chain of ubiquitin proteins to the HIF α subunits (ubiquitination), which leads to recognition and degradation by the proteasome.27 Low oxygen levels lead to a graded decrease in the enzymatic activity of PHD proteins (half-maximum at 15–20 μM O2, or around 2% O2), thereby stabilizing HIF protein complexes.28 As a result, they accumulate and translocate to the nucleus, where they execute their transcriptional activities by binding to HIF-responsive elements (HREs), subsequently increasing the expression of a large set of HIF-responsive genes that ensure proper adaptation to the detected hypoxic conditions.26,29 The distinct HIF transcriptional complexes have both overlapping and unique gene targets, and also exhibit distinct functions in vivo.30 HIF-1α is associated with upregulation of genes needed for cell survival in low-oxygen environments, while HIF-2α promotes the transcription of genes related to stem cell behavior, such as octamer-binding transcription factor 4 (OCT4), NANOG and c-MYC.30 HIF-3α is much less studied, though it also acts as a transcription factor and is upregulated in various pathological situations, as for instance in heart failure.31 In certain cell types and contexts HIF-1α drives the initial response to acute hypoxia, while HIF-2α takes over during chronic hypoxia due to the activity of hypoxia-associated factor,32 a ubiquitin ligase that binds and adds a ubiquitin protein chain to HIF-1α, leading to its degradation. HIF-1α affects the transcription of genes in many different cellular processes. By increasing the transcription of glucose transporters such as glucose transporter 1 (GLUT1) and glycolytic enzymes such as hexokinase 1 (HK1), aldolase A (ALDOA) and pyruvate kinase (PKM), HIF-1α is responsible for switching energy production in hypoxic cells from an oxygen-dependent oxidative phosphorylation to an oxygen-independent glycolysis, which in the context of cancer leads to an enhanced aggressiveness.33 Additionally, by regulating the transcription of vascular endothelial growth factor (VEGF), HIF-1α and HIF-2α signalling also stimulates angiogenesis.3 Other physiological processes affected by HIF signalling include, for instance, immune suppression and cell migration.34–36
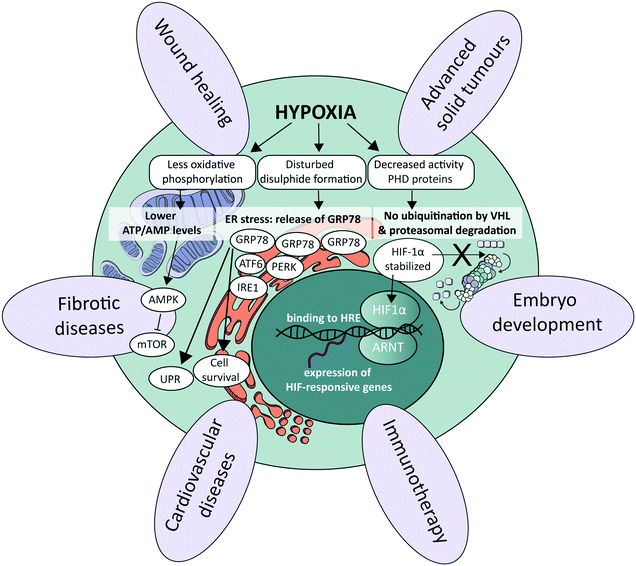 |
| Fig. 1 Importance of hypoxia in physiological and pathological processes. Schematic representation of the most important signalling pathways controlled by oxygen (middle), petals around indicating a few examples of essential physiological and pathophysiological processes that are significantly influenced by oxygen levels. ATF6, activating transcription factor 6; ARNT, aryl hydrocarbon receptor nuclear translocator; ER, endoplasmic reticulum; HIF1, hypoxia-inducible factor 1; HRE, hypoxia response element; IRE1, inositol-requiring enzyme 1; mTOR, mammalian target of rapamycin; PERK, protein kinase R (PKR)-like endoplasmic reticulum kinase; PHD, prolyl hydroxylase domain; UPR, unfolded protein response; VHL, von Hippel–Lindau. | |
Alternative oxygen sensing pathways
Other pathways that respond to low oxygen levels are the unfolded protein response (UPR) and the mammalian target of rapamycin (mTOR) pathway. The UPR pathway is a transcriptional induction pathway activated in cells undergoing endoplasmic reticulum (ER) stress (Fig. 1).37 Under hypoxic conditions, unfolded and misfolded proteins accumulate in the ER, which can be explained by the oxidizing potential of oxygen that supports disulphide bond formation in post-translational folding.38 In non-stressed cells, the UPR mediating stress-responsive proteins activating transcription factor 6 (ATF6), inositol-requiring enzyme 1 (IRE1) and protein kinase R (PKR)-like endoplasmic reticulum kinase (PERK) are maintained in an inactive state through interaction with glucose regulated protein 78 (GRP78), also referred to as binding immunoglobulin protein (BiP).39 Upon the accumulation of unfolded or misfolded proteins and ensuing ER stress, these stress-responsive proteins are released and trigger the UPR pathway to enhance the transcription of genes involved in cell survival40–42 and ER capacity.43,44 mTOR is a central nutrient sensor that regulates growth, metabolism, cell survival and autophagy.45 mTOR signalling is sensitive to oxygen levels through various independent signalling pathways.25 One energy-dependent pathway, for instance, involves sensing of reduced ATP production by oxidative phosphorylation due to limited oxygen availability, which activates AMP-activated protein kinase (AMPK). AMPK in turn phosphorylates the tuberous sclerosis protein 1 (TSC1)–TSC2 complex,46 which represses mTOR through acting as a GTPase-activating protein toward the mTOR-activating small GTPase Rheb.47
Role of oxygen at the tissue level in healthy conditions
Tissue oxygenation is one of the most crucial processes in human physiology as it is closely related to (energy) metabolism and cellular survival, and it involves a multitude of intracellular signalling cascades, some being introduced above. From inhalation of air to oxygen diffusion across alveolar membranes, transport on haemoglobin in red blood cells and diffusion into tissues, oxygen availability is very tightly regulated in vivo at the entire body level. The oxygen concentration drops from 20.9% in the troposphere to approximately 12% in arterial blood, and decreases further to ∼5% in venous blood due to oxygen consumption throughout the body.48 Physiological oxygen concentrations vary greatly between and within tissues in the human body, and oxygen gradients in certain tissues often play important (patho)physiological roles (Fig. 1). Methods to determine oxygen concentrations in tissues in vivo vary considerably, and different approaches often yield somewhat distinct values for the same tissues.3,48 Nevertheless, bulk measurements have revealed that organs with a relatively high average oxygen concentration include ovaries (∼11.5%) and the renal cortex (∼9.5%), while tissues with lower average oxygen concentrations include muscle fibres (3.8%) and the brain (4–6%) (Fig. 2).3,48 Despite the comparatively low oxygen pressure in the brain, neurons are extremely sensitive to hypoxia, causing rapid and irreversible brain damage after oxygen deprivation.49 Noteworthy, controlling oxygen tension is also a major challenge in the formation of brain organoids,50 which as soon as they reach a certain size, are likely to present a hypoxic core.
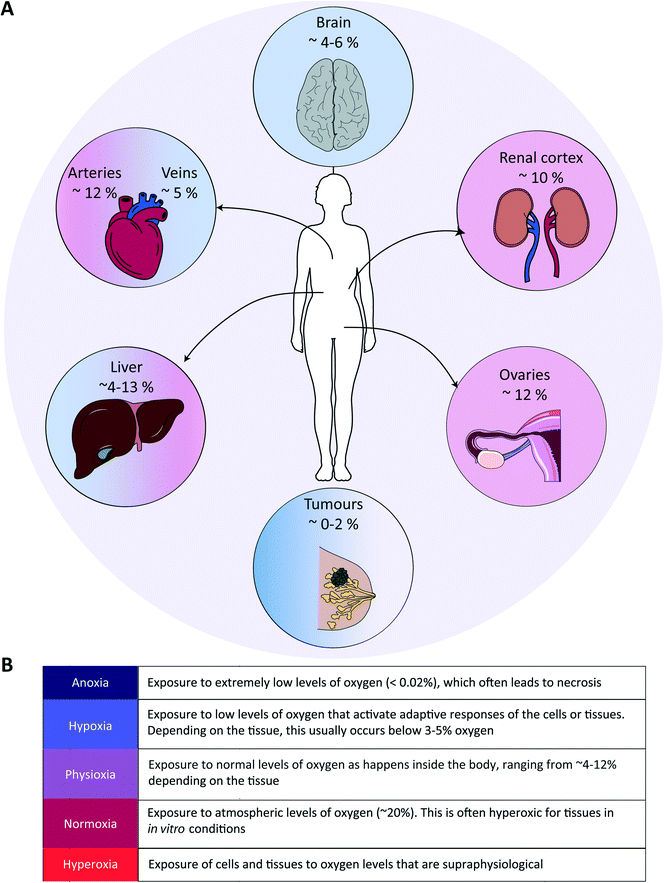 |
| Fig. 2 Examples of oxygen levels in several tissues in the human body. (A) Various organs are depicted with their respective tissue oxygen levels, emphasizing that oxygen levels are widely different in tissues, which is usually not taken into account in in vitro cell or tissue models. (B) Glossary of terms to describe exposure to various oxygen levels. | |
In the liver, oxygen concentrations vary significantly (from ∼4 to 13%) and are directly linked to so-called zonation. Three zones can be distinguished in a liver sinusoid between the central vein and the space where the blood flows coming from the portal vein and hepatic artery are mixed. The oxygen content decreases going from the latter region to the former, and each of the three zones is characterized by a different O2 percentage which impacts both the cell metabolic capacities and their cytokine secretion profile.51–53 As such, liver zonation with these distinct oxygen levels should be incorporated for toxicity screening, liver disease models and metabolic studies. Noteworthy, normal early embryo development happens in a hypoxic environment, estimated to be at 1.5–2% O2 in the uterus of rhesus monkeys.18 The low oxygen tension plays a crucial role in regulating stem cell fate and developmental morphogenesis.9 In line with this observation, growing embryos for in vitro fertilization (IVF) at 5% O2 instead of atmospheric conditions of 20% O2 benefits their quality and has been reported to result in more live births per embryo transfer.54,55 Hypoxia also plays a dual role in wound healing. In the initial stages, it is beneficial and sets in motion a wide array of processes, including the upregulation of genes that enhance healing processes through HIF-1α stabilization.56 Prolonged hypoxia is however detrimental: it delays the entire wound healing process, and can even contribute to the generation of non-healing ulcers.11
Importance of oxygen levels in disease
As alluded to before, the importance of hypoxia has been established in a wide range of pathologies (Fig. 1). In the case of cardiovascular diseases, tissues are often exposed to hypoxia and even anoxia after a stroke, and intermittent hypoxia is linked to high blood pressure and myocardial ischemia due to sleep apnoea.57 Fibrotic diseases are also strongly associated to the pro-inflammatory, angiogenic, and fibrogenic effects of chronic hypoxia.58,59 Further examples are provided in the last section of this review (Table 3). Yet, the prime example of the importance of hypoxia in a pathological situation is in advanced solid tumours, which we discuss in more detail in the following paragraph.
In solid tumours, oxygen concentrations are usually very low, due to the limited penetration and diffusion of oxygen into these dense and relatively poorly vascularized tissues. Distances between vessels are typically greater than the average oxygen diffusion length of a few hundreds of microns.60 On average, oxygen concentrations in tumour tissues range from 1 to 2%, but can be as low as 0.3% in the case of pancreatic tumours.3 Such a low oxygen concentration leads to both direct and indirect metabolic adaptations: e.g., directly with lower ATP generation by oxidative phosphorylation and the inhibition of fatty acid desaturation, and indirectly by mediating hypoxia-induced changes in gene expression levels,61 causing a reduced pH and the release of angiogenic factors.62 Hypoxia is also associated with increased reactive oxygen species (ROS) levels,63 enhanced probability for cancer metastases through increased inflammation and enhancing the epithelial-to-mesenchymal transition (EMT),64 and therapy resistance,65 notably due to the fact that cells switch metabolism profiles, which facilitates entry into a quiescent state. Hypoxia furthermore leads to immune evasion, thereby negatively affecting the efficacy of immunotherapies.66 As tumours are heterogeneously oxygenated, regions in tumours that are relatively distant from the nearest functional blood vessel can become fully anoxic, meaning oxygen levels below 0.02%.67 When this happens, cells undergo necrosis, leading to the formation of a necrotic core. Next to the degree of hypoxia, the temporal nature of exposure to hypoxic conditions is an important factor with a significant impact on tumour cell behaviour. Tumour cells can be exposed to chronic hypoxia, which occurs in cells located too far away from a blood vessel and in an environment with a high cell density. Alternatively, acute hypoxia is a sudden (irreversible) establishment of hypoxia, for example due to sudden closure of a blood vessel induced by the pressure exerted by the increased tumour mass.68 Finally, cyclic or intermittent hypoxia is a state where cycles of hypoxia followed by reoxygenation occur due to transient shutdowns of the poor-quality tumour vasculature.68,69
Controlling the oxygen tension in a microfluidic device
Different approaches have been developed to accurately control the oxygen tension in microfluidic devices in combination with both 2D and 3D cell culture models (Fig. 3), with the underlying aim to precisely model the gas concentrations found in physiological or pathological conditions in specific tissues or organs. Concrete aims are usually to generate hypoxic conditions, either in a chronic or in a cyclic manner, or to create an oxygen gradient across a cell culture. These approaches can broadly be classified in two main categories: on one hand, engineering approaches implemented at the device level during its fabrication, e.g., through proper choice of materials employed to build the microfluidic device (Fig. 3A and B), and, on the other hand, chemical approaches that involve the active perfusion of fluids oxygenated at a precise level, or fluids supplemented with oxygen-producing or oxygen-scavenging chemicals (Fig. 3C). Noteworthy, a few reports combine these two approaches (Fig. 3D). Finally, open-well configurations have also been proposed either with membranes separating gas channels from a cell culture area in an open well (Fig. 3E, top) or in devices assembled in blocks of oxygen-permeable or impermeable materials (Fig. 3E, bottom). Since other reviews have extensively described the microfabrication process as well as the set up and validation of oxygen control in such devices,70–72 here, only a brief overview of all approaches is provided.
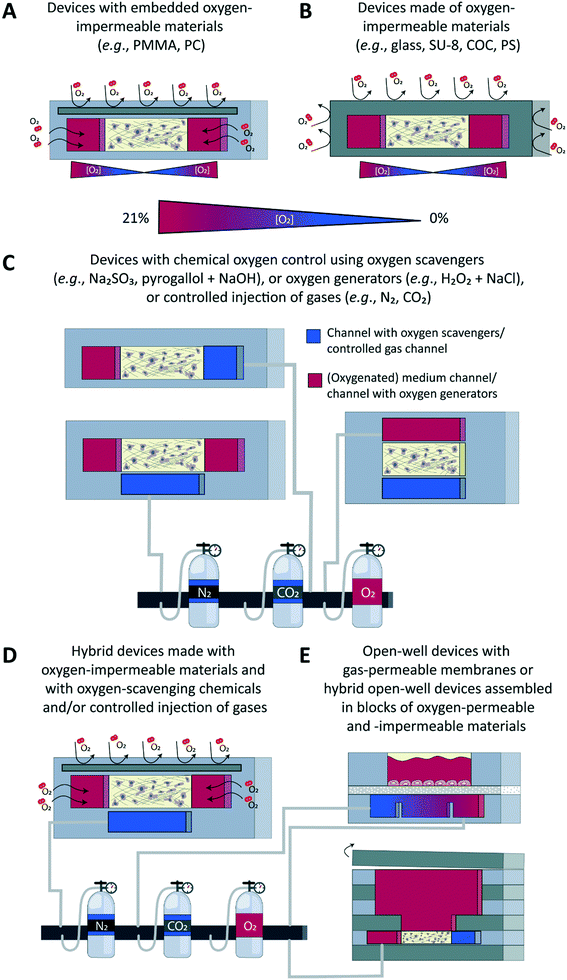 |
| Fig. 3 Approaches to control oxygen in microfluidic devices. An overview and schematic representation of various approaches to control the oxygen tension in microfluidic devices is given, showing the cross-sectional view of the device in which a 3D cell model is created in a hydrogel matrix. (A) Devices with embedded oxygen-impermeable substrates. (B) Devices made entirely from oxygen-impermeable substrates. (C) Devices in which oxygen control is set using oxygen-scavenging or oxygen-generating molecules or controlled gas injection. (D) Hybrid devices combining multiple approaches simultaneously to control oxygen. (E) Open-well devices with a gas-permeable membrane between gas channels and a cell culture area in an open well (top) or hybrid open-well devices assembled in blocks combining layers of oxygen-permeable and -impermeable material, and with an open reservoir for addition of medium (bottom). COC, cyclic olefin copolymer; H2O2, hydrogen peroxide; Na2SO3, sodium sulphite; PC, polycarbonate; PMMA, polymethyl methacrylate; PS, polystyrene. | |
Engineering approaches to control the oxygen tension in a microfluidic device
Microfluidic devices meant for cell culture experiments and to create organ models are mostly fabricated from the elastomeric material PDMS, or polydimethylsiloxane, which is gas permeable, its permeability to oxygen being 4.1 × 10−5 cm2 s−1.73 This property, which was initially identified as an advantage to ensure cells in a device would be supplied with adequate amounts of oxygen and carbon dioxide,22 can actually turn out into a major limitation when wanting to tune the oxygen tension and/or create physioxic or hypoxic culture conditions to emulate the in vivo situation. Therefore, a first obvious alternative is to not use PDMS, but a gas-impermeable material such as glass,74 SU-875,76 or a thermoplastic like polystyrene (PS)77,78 or cyclic olefin copolymer (COC) (Fig. 3B),78 or even a combination of a thermoplastic with another material to increase the barrier function of the device.78 Alternatively, still with the aim of blocking free oxygen diffusion into the cell culture area, a gas-impermeable material can be embedded in a PDMS-based device, which has notably been achieved using polymethylmethacrylate (PMMA)79,80 or polycarbonate (PC) substrates (Fig. 3A).81,82 The main advantage of this engineering approach is that the oxygen tension in the device and the cell microenvironment is set by the cells themselves, which are actively consuming oxygen. This scenario is in theory analogous to the in vivo situation in which the metabolic activity of cells, together with the delivery of oxygen through the circulation, are decisive for the local oxygen tension in a tissue and the possible presence of gradients thereof. Still, for this ideal physiological scenario to be achieved, culture parameters must be adequately scaled, like the cell-liquid ratio for instance, to ensure cell metabolism and oxygen delivery are matching the in vivo situation.
Chemical approaches to control the oxygen tension in a microfluidic device
The second approach is of a chemical nature and relies on active perfusion of fluids (liquids or gases) conditioned at a given oxygen concentration or supplemented with chemicals to scavenge or generate oxygen. This chemical approach provides more flexibility in the experimental design to possibly alter dynamically and on-demand the oxygen concentration in the device and the tissue microenvironment, e.g., to create intermittent or chronic hypoxia, which is important to model an ischemic shock, for instance.83–85
In a first example, medium containing an appropriate oxygen concentration was directly perfused on a multi-layer cellular model of the liver sinusoid, in the view of modelling the oxygen zonation found in this tissue.86 The flow rate and the channel height were both adjusted to not introduce any bias due to the permeation of oxygen through the PDMS; a shallow oxygen gradient was found both experimentally and through modelling, along the channel, but only in its top part, and with no impact on the cellular model. Most of the time the pre-conditioned fluids are not perfused directly onto the cell culture, but in additional channels or structures in the device, leveraging once more the high gas permeability of the PDMS material. These additional channels are typically located above83,84,87 or below88 the cell culture area, with gas permeation occurring though a thin PDMS membrane placed between the culture chamber and the control channels. When using one broad control channel covering the entire cell culture area, the latter is homogeneously exposed to the same oxygen tension, which can however be changed over time. Gradients of oxygen have also been successfully created across a cell culture, by using two control channels placed on the two sides of the culture area and perfused with air and nitrogen.88,89 By changing the distance between the two control channels and the composition of the gas perfused therein, the gradient characteristics such as the range and shape/slope can easily be adjusted.88 Yet another approach to control oxygen levels made use of silicone hydrogel synthetic vessels for the controlled delivery of oxygen to a 3D cancer cell culture.90
Instead of employing pre-conditioned fluids, solutions can be supplemented with chemicals to actively and in situ alter the dissolved oxygen concentration, through its consumption by oxygen-scavenging species such as sodium sulphite80,85,91,92 and pyrogallol/NaOH93,94 or its production by oxygen-producing species such as a combination of hydrogen peroxide and NaCl.93 In a first example, Bulutoglu et al. directly perfused a solution of sodium sulphite and cobalt sulphate onto cellular models;92 specifically, thanks to a gradient generator located upstream to an array of culture chambers, they exposed hepatocyte monolayers to medium oxygenated to specific levels, as found in the different zones in the liver, and containing various concentrations of free fatty acids, to create a non-alcoholic fatty liver disease (NAFLD) model. To prevent direct contact between cells and oxygen-scavenging and oxygen-producing chemicals, these are like the pre-conditioned fluids described above also most often perfused in channels adjacent to the cell culture area93 or located below it.91 A thin PDMS membrane separates the culture chambers from these control fluidic lines, through which gases can diffuse easily, which is analogous to having oxygen diffusing across alveolar membranes in the lung.
To create gradients of oxygen across a cell culture area, the latter can be flanked on one side only by one channel, perfused with oxygen-scavenging chemicals, acting as a sink,85,94 or by two channels perfused respectively with oxygen-scavenging and oxygen-generating chemicals,93 acting, respectively, as a sink and a source.
Hybrid engineering/chemical approaches to control the oxygen tension in a microfluidic device
Finally, a handful of reports have combined engineering and chemical approaches to add a dynamic component to the system or to ensure the system remains at a given oxygen concentration. In all cases, a gas-impermeable substrate was introduced in a PDMS fluidic layer, while actively perfusing in dedicated side channels either sodium sulphite to scavenge oxygen on one side of a cell culture chamber80 to generate an oxygen gradient across it, or gas mixtures on both sides to create hypoxic, normoxic conditions, or an oxygen gradient, and to be able to switch between these different scenarios on-demand.81,82,95–97
Readout – sensors
As a first readout, oxygen sensors can be integrated in the microfluidic device, with the primary aim to experimentally characterize the devices when the oxygen tension is actively controlled or to correlate the oxygen tension with other biological, physical, or cellular parameters. While some sensors have been developed to evaluate the oxygen consumption and/or metabolism of (individual) cells, tissues, or embryos,98,99 they can equally be employed to monitor the oxygen tension in miniaturized devices. Two approaches dominate for on-chip oxygen sensing, using either electrochemical100 or optical detection,101 which are both discussed in more detail in the following. It is worth noticing that other approaches are also widely employed to evaluate the impact of hypoxia on cells and tissues and to examine their biological response. These include the detection of metabolic markers with various microscopy techniques or collection of cells and investigation of protein and/or gene expression levels. Since these techniques by themselves belong to the standard biochemical toolbox of molecular and microscopy analysis and are not specific for the study of hypoxia, we will not discuss them in depth in the text, but they are summarized in Table 1.
Table 1 Techniques and/or readout approaches for oxygen levels in microfluidic devices
Level |
Technique/readout |
Purpose/application |
Time/location |
Advantages |
Limitations/risks |
ELISA, enzyme-linked immunosorbent assay; LC-MS, liquid chromatography mass spectrometry; OoC, organ-on-a-chip; PTM, post-translational modifications; ROS, reactive oxygen species; RT-qPCR, reverse transcription quantitative polymerase chain reaction.
|
Device level |
Electrochemical sensing |
Spatio-temporal mapping of oxygen concentration |
Real-time |
High sensitivity |
Integration of electrodes |
|
Correlation between O2 concentration and biological response |
In situ
|
Fast response time |
Toxicity of the sensor materials |
|
Ease of miniaturization |
Planar (2D) information |
|
Multi-parametric sensors |
Sensor biofouling |
|
Interference with other species in solution |
|
Active consumption of O2 |
|
Optical sensing/bioluminescence |
Real-time |
No O2 consumption |
Toxicity of the probes |
|
In situ
|
High sensitivity |
Generation of ROS |
|
Fast response time |
Planar (2D) information if planar sensor and not embedded in a 3D hydrogel matrix |
|
Two measurement schemes (time-resolved and intensity) |
Need for bulky optical instrumentation |
|
Microscopes ubiquitous in life sciences labs |
|
Fluorescence |
O2 level and gradients thereof |
Real-time |
Microscopes ubiquitous in laboratories |
Loss of fluorescence over time/bleaching |
|
In situ
|
|
Cellular & tissue levels |
Fluorescence microscopy |
Hypoxia imaging (hypoxia-sensitive fluorescent probes) |
Live cell imaging |
Well-established protocols |
Altered behaviour of fluorescently labelled species (molecules, cells) |
|
In situ
|
Non-invasive |
|
(Fluorescence) microscopy |
Cell metabolism (fluorescent glucose analogue 2-NBDG) |
Live cell imaging |
Continuous measurements |
Cell transfection/engineering in some cases – for specific marker of interest (signalling pathways) or cell tracking using fluorescence microscopy |
|
Cell migration, proliferation, quiescence |
In situ
|
Possibly real-time information |
|
|
Apoptosis, cell viability (drug response) |
Little to no (photo)toxicity |
|
|
Tissue size and variations thereof depending on the O2 tension (swelling) |
3D information |
|
|
ROS production (ROS-sensitive fluorescent probes) |
Measurements possibly inside cell culture incubators |
|
Signaling pathway activation (e.g., HIF, UPR) |
Immunoassays (ELISA, Luminex) |
Inflammation |
Off-line |
Well-established protocols |
Dependent on antibody availability |
|
|
|
Intercellular signalling |
Suitable for continuous measurements and on-line analysis |
Labour intensive |
|
|
Metabolism |
Expensive to multiplex |
|
Tissue function |
|
Immunostaining and fluorescence microscopy |
OoC characterization |
Endpoint |
Well-established protocols |
No continuous monitoring |
|
Protein expression (levels) |
In situ or off-line |
Microscopes ubiquitous in laboratories |
Sample sacrifice |
|
|
Signaling pathway activation |
Difficult to implement with 3D cellular models and on-chip when using multi-material devices |
|
|
Triggering of biological processes |
Dependent on antibody availability |
|
|
RT-qPCR |
Gene expression levels for specific processes/pathways |
Endpoint |
Well-established protocols |
Limited number of samples: pooling of multiple devices |
|
Off-line |
Easy design of primers |
Lack of spatial information |
|
Suitable for all transcripts, including miRNA |
|
RNA Seq |
Gene expression profiles |
Endpoint |
Downscalable to the single cell level |
Limited number of samples: pooling of multiple devices |
|
Off-line |
Comprehensive gene expression analysis |
Lack of spatial information unless implemented at the single cell level in combination with regioselective cell extraction |
|
High cost per sample |
|
LC-MS/MS |
Protein expression profiles (proteomics) |
Endpoint |
Comprehensive or targeted analysis |
Limited number of samples: pooling of multiple devices |
|
Off-line |
Sub-proteomics analysis (PTM profiling) |
Lack of spatial information |
|
High cost per sample |
|
Secretomics |
Real-time (possibly) |
Suitable for continuous measurements and on-line analysis |
Need for dedicated sample preparation if online analysis |
|
|
Metabolism |
In situ or off-line |
High cost per sample |
Electrochemical sensing
Electrochemical sensors mostly consist of miniaturized electrodes, which are fabricated as part of the substrates of the device. They come as miniaturized Clark electrodes,102 ultra-microelectrodes (UMEs) – and mostly arrays thereof,98,103,104 with different geometries and are based on various metal and oxide materials. Yet, they all rely on the same sensing principle: the reduction of the oxygen in solution, which generates a current directly proportional to the dissolved oxygen concentration. As summarized in Table 1, the main advantages of these electrochemical sensors are: (a) their sensitivity; (b) ease of miniaturization and integration in a microfluidic device; (c) suitability to record at different surface locations in the device and image oxygen gradients simply by adapting the sensor geometry; and (d) the possibility to correlate oxygen concentration with other (metabolic) parameters, measured using the same electrode or another sensor. At the same time, limitations associated with electrochemical sensing are: (a) the possible toxicity of the electrode materials; (b) the impossibility to access three-dimensional information, which is particularly important when working with 3D cellular constructs; (c) the possible interference of other species in solution also reduced at the same potential; (d) electrode biofouling through uncontrolled adsorption of proteins such as albumin on the electrode surface, a widely encountered issue that leads to a progressive reduction in the active sensing area, and; (e) more importantly, the active consumption of oxygen through the measurements which may deplete oxygen in the direct vicinity of the cells, and give rise locally to oxygen levels that are significantly lower than initially targeted. This can easily become critical when working with microliter to sub-microliter volumes and small oxygen amounts, as is the case in microfluidic devices.
Readers interested in such microsensors for on-chip monitoring of oxygen as well as other parameters of the cell metabolism, are referred to a recent review by Kieninger et al.100 Of particular interest is recent work in which the same RuOx-based sensor was employed in a Transwell system to dually monitor and thereby correlate two essential parameters in the cellular microenvironment, namely the oxygen tension and acidification rate, to characterize the metabolic activity of cardiomyocytes supplied with either glucose- or galactose-supplemented medium.105 To address one major limitation of electrochemical sensing, which is the consumption of oxygen by the measurements, van Rossem et al. proposed an innovative approach combining an UME array and millisecond time scale recordings, reducing significantly the magnitude the oxygen consumption while still supporting real-time and continuous measurements.104
Optical sensing
Optical sensors employ oxygen-sensitive luminescent probes, in the form of coordination metal complexes based on Pt, Pd or Ru. Their luminescence is quenched in the presence of oxygen, and the higher the measured luminescence intensity, the lower the oxygen tension.106 Alternatively, time-resolved measurements are employed, which consider the lifetime of the luminescence probe.101,106 This latter detection scheme is less sensitive to background noise due to the autofluorescence of other species in the sample, and photobleaching.101,106 For oxygen sensing, these luminescent probes can be introduced in different manners in a microfluidic device. First, they can be added to the culture medium, either freely in solution provided they are water-soluble86,94,107,108 or after embedding in microbeads,109 which limits direct interactions of the probe with the living biological sample and reduces toxicity concerns. Alternatively, they are encapsulated in a solid material and embedded in a continuous or patterned polymer thin film110 placed at the bottom or top of the device, or in prefabricated trenches in the PDMS roof,111 approaches which equally limit interactions of the probes with the biological sample.
Fluorescence microscopes are ubiquitous in biological labs, which makes this optical sensing approach very attractive compared to electrochemical measurements. Furthermore, it does not alter the cell microenvironment by consuming oxygen. However, optical measurements mostly require bulky instrumentation, which makes them less suitable for full integration and miniaturization. Furthermore, fluorescent chemical probes can be toxic to cells directly exposed to them107 or, indirectly, through the photo-induced production of radical species.112 Finally, thin-film optical sensors, as their electrochemical counterparts, only reveal 2D information on the oxygen concentration in the vicinity of the sensors, while 3D cellular models, which are more relevant, exhibit an inhomogeneous oxygen distribution in all three dimensions, and often have little to no oxygen in their core. To address this last limitation, Ru-based oxygen-sensitive probes have been covalently attached to a collagen hydrogel, which was subsequently employed as a matrix to create a 3D cellular construct in a microfluidic device, possibly allowing 3D mapping of the oxygen distribution in the resulting tissue.113
Biological applications of hypoxia in microfluidic devices
Although there have been major efforts in developing methods to control and measure the oxygen tension in microfluidic devices, as discussed in the previous sections, usually these devices have only been validated by confirming well-known biological or pathological processes affected by oxygen levels, as an understandable first proof-of-concept step. Nevertheless, several studies have already provided interesting insights, e.g., with respect to the relation between oxygen levels and cell migration,82,87,93–97,114–116 ROS production,74,117–119 activity of HIFs88,120,121 and metabolic changes.77,79,122,123 Other work has focused on intermittent vs. chronic hypoxia81,83,87,124 or investigated targeting of hypoxia by hypoxia-responsive drugs.93,125,126 An overview of these studies is presented in Table 2 and is discussed in this section.
Table 2 Overview of biological applications of microfluidic devices with oxygen control
Biological process |
Method of oxygen control |
Cell type |
Techniques |
Readouts |
Ref. |
Human breast adenocarcinoma.
Human pancreatic ductal cell carcinoma.
Human umbilical vein endothelial cells.
Madin–Darby canine kidney cells.
Chinese hamster ovary cells.
Human alveolar basal epithelial adenocarcinoma cells.
Non-tumorigenic mammary epithelial cells.
Human breast adenocarcinoma.
Human brain microvascular endothelial cells.
Human cerebral microvasculature endothelial cells.
Human bone marrow derived MSCs.
Human glioblastoma astrocytoma cells.
Human breast invasive ductal carcinoma.
Endothelial colony forming cell-derived endothelial cells.
Normal human lung fibroblasts.
Human colorectal adenocarcinoma cells.
Human cervical carcinoma cells.
Human epithelial ovarian cancer cells.
Peripheral blood mononuclear cells.
Chimeric antigen receptor T-cells.
|
Cell migration |
Hybrid: oxygen impermeable material and chemical oxygen control in gas channel (Fig. 3D) |
MDA-MB-231a |
Real-time microscopy |
Cell migration trajectory |
82
|
|
Chemical control of oxygen in gas channel (Fig. 3C) |
PANC-1b |
Phase contrast microscopy |
Cell migration along oxygen gradient in a collagen matrix |
87
|
|
Chemical control of oxygen in gas channel (Fig. 3C) |
MDA-MB-231a |
Real-time microscopy |
Cell net displacement, trajectory, directionality. Cell viability |
96
|
|
Chemical control of oxygen in leaching channel with oxygen scavenging-chemicals (Fig. 3C) |
HUVECc |
Real-time microscopy |
Speed of collective cell migration |
116
|
|
ROS production and ER stress |
Open-well device with gas-permeable membrane and chemical oxygen control in gas channels (Fig. 3E top) |
MDCKd |
Real-time microscopy |
Intracellular fluorescence of ROS-sensitive dyes |
119
|
|
Open-well device with gas-permeable membrane and chemical control of oxygen in gas channels (Fig. 3E top) |
CHOe cells stably expressing 6 U-HIF-binding repeats (HBR) as a reporter of HIF-α stabilization |
Fluorescence microscopy |
Changes in fluorescence intensity of the HBR reporter and redox probe in the mitochondrial membrane |
117
|
|
A549f cells expressing a redox-sensitive fluorescent biosensor in the mitochondria |
|
Hybrid: chemical control of oxygen in gas channel and gas-impermeable materials (Fig. 3D) |
MCF-12Ag and MDA-MB-468h |
Fluorescence microscopy |
Fluorescent ER stress indicator thioflavin T |
118
|
|
HIFα stabilization and hypoxia-triggered metabolic changes |
Chemical control of oxygen in gas channel (Fig. 3C) |
hCMEC/D3i |
Fluorescence microscopy |
Immunofluorescence using anti-HIF-1α and anti-HIF-2α antibodies |
88
|
|
Hybrid: chemical control of oxygen in gas channels and layers of oxygen-impermeable materials stacked as a block (Fig. 3E bottom) |
MDA-MB-231a cell line transfected with a 5× hypoxia response element (5HRE)/eGFP gene construct |
Fluorescence microscopy |
Expression of eGFP under hypoxia |
120
|
|
Open-well device with gas permeable membrane and chemical control of oxygen in gas channels (Fig. 3E top) |
HLMVECj and MSCsk |
Fluorescence microscopy |
Immunofluorescence with anti-HIF-1α antibody |
122
|
|
Western blot |
Analysis of LDHA expression |
|
RT-qPCR |
Quantification of gene expression |
|
Oxygen-impermeable material embedded in PDMS (Fig. 3A) |
U-251-MGl |
Fluorescence microscopy |
Imaging of the fluorescent hypoxia sensor Image-iT, the fluorescent glucose analogue 2-NBDG uptake and the pH-sensitive TR/fluo-labelled dextran |
79
|
|
Oxygen-impermeable materials in the form of a cap and pillars (Fig. 3A) |
MCF-7m |
Fluorescence microscopy |
Immunofluorescence of GLUT-1 |
132
|
|
RT-qPCR |
Gene expression analysis after laser microdissection of different regions of the device |
|
Intermittent hypoxia and co-cultures with hypoxic cells |
Hybrid: oxygen-impermeable material embedded in PDMS and chemical oxygen control in gas channels (Fig. 3D) |
MDA-MB-231a |
Real-time microscopy |
Cell migration speed. Cell distribution and proliferation under different oxygen conditions |
81
|
|
Chemical control of oxygen in gas channel (Fig. 3C) |
Tumour spheroids from MCF-7 cellsm |
Brightfield, fluorescence microscopy, and two-photon microscopy |
Monitoring of global spheroid size |
83
|
|
Volumetric changes of single cells |
|
Chemical control of oxygen in leaching channel with oxygen scavengers (Fig. 3C) |
ECFC-ECsn made constitutively fluorescent and NHLFo |
Real-time microscopy |
Angiogenesis tracking of fluorescent endothelial cells |
85
|
|
Chemical control of oxygen in gas channel (Fig. 3C) |
C57BL/6 mice-derived pancreatic islets |
Off-chip insulin ELISA |
Insulin production |
124
|
|
Fluorescence microscopy |
Rhodamine 123 fluorescence to observe changes in mitochondrial membrane potential |
|
Barriers in microfluidic devices |
Chemical control of oxygen in gas channel (Fig. 3C) |
CaCo-2p and primary HC2978 colon organoids from a patient |
Fluorescence microscopy |
Immunofluorescence of hypoxia markers |
140
|
|
Obligate anaerobic bacteria: F. prausnitzii, Eubacterium rectale, and B. thetaiotaomicron, with different degrees of oxygen sensitivity |
RT-qPCR |
Quantification of gene expression |
|
LC-MS/MS |
Analysis of metabolites produced by bacteria |
|
Oxygen-impermeable materials (Fig. 3B) |
CaCo-2p |
Fluorescence microscopy |
Imaging of intracellular fluorescent hypoxia sensors, actin cytoskeleton and tight junctions, or GFP expression in bacteria under hypoxic conditions |
141
|
|
Facultative anaerobic bacteria Escherichia coli and obligate anaerobic Bifidobacterium adolescentis |
|
Hypoxia-targeted therapies |
Chemical control of oxygen in leaching channel with oxygen scavengers (Fig. 3C) |
A549f |
Brightfield and fluorescence microscopy |
Live/dead cell ratios using calcein AM and ethidium homodimer-1 after drug treatment |
93, 126 |
|
Chemical control of oxygen in leaching channel with oxygen scavengers (Fig. 3C) |
A549f and HeLaq cells |
Fluorescence microscopy |
Cell viability staining using acridine orange and propidium iodide (AO/PI) to assess oxygen–drug interactions |
93, 126 |
Oxygen-impermeable materials (Fig. 3B) |
SKOV3r |
Fluorescence microscopy |
T-cell toxicity using calcein AM/propidium iodide (live dead assay) |
125
|
|
|
T-cells isolated from PBMCs,s CAR-Tt cells containing scFv 4D5 (anti-HER2) |
Immunofluorescence of GLUT-1 and PD-L1 in tumour cells, and CD45 for T-cell infiltration |
Cell migration along oxygen gradients
As a first application, the migratory behaviour of cells has been investigated along oxygen gradients in 2D and 3D cellular models. In particular, the motility of PANC-1 pancreatic cancer cells through a collagen gel was found to increase under hypoxic conditions, with marked directionality towards higher oxygen tensions.87 MDA-MB 231 breast cancer cells seeded as a 3D model in collagen or fibrin hydrogels showed a similar behaviour, with enhanced motility and directionality towards higher oxygen concentrations, as imaged using confocal microscopy.82,96 Interestingly, aggressive MDA-MB-231 breast cancer cells and cancer stem cells, both cultured as a monolayer, were found to migrate towards hypoxic areas,80 showing an opposite migratory behaviour. The authors hypothesized that this different behaviour could be explained by the fact that their model only included an oxygen gradient, unlike other devices where other gradients, e.g., glucose gradients, co-occurred with the oxygen gradient. Still using the same aggressive breast cancer cell line, cultured in 3D in collagen, molecular analysis was performed, confirming the activation of the HIF-1 signalling pathway under hypoxic conditions.82
In a device fabricated from SU-8, hypoxia-driven cell migration was observed with the formation of pseudopalisades,127 which are tumour regions with high cell density where tumour cells are hypoxic and overexpress HIF-1α. Pseudopalisades are typical structures of glioblastoma and are linked to an enhanced migration due to an epithelial–mesenchymal transition induced by HIF-1 activity.115 In another device flanked by an oxygen-depleting channel on one side and an oxygen-generating channel on the opposite side, gradients were reported to impact A549 cell migration, which was linked to an increased secretion of transforming growth factor β (TGF-β) under hypoxic conditions.93 The interactions between fibroblasts and cancer cells,94 as well as the response of cancer cells to various anti-cancer drugs, were also affected by this oxygen gradient.93
Healthy endothelial cells, on the contrary, migrated towards lower oxygen concentrations when grown as a 2D model, corroborating the importance that oxygen gradients play in guiding and regulating angiogenesis.116 Funamoto et al. evaluated the impact of hypoxic conditions on the integrity of an endothelium lining; barrier function was significantly impacted by the hypoxic conditions and adhesion molecules were internalized by the cells, as revealed by on-chip immunostaining.95 Endothelial cell migration was also imaged in the same platform, under hypoxic vs. normoxic exposure,97 using particle imaging velocimetry (PIV). As was the case for cancer cells, migration was enhanced under hypoxic conditions, which was associated with a decrease in VE-cadherin expression, and stabilization and nuclear translocation of HIF-1α, which confirmed the active sensing and cellular response activation to the presence of hypoxia.
ROS production and ER stress in response to changes in oxygen tension
Cells grown under hypoxic conditions are known to produce increased levels of ROS, although the exact mechanisms for this remain unknown.128–130 Using microfluidic devices with a hypoxic microenvironment, ROS-related features have been investigated and correlated to the presence or absence of hypoxia in the device. For instance, Lo et al.119 used an open-well device equipped with an array of underlying channels perfused with gas at different oxygen concentrations (similar to Fig. 3E, top). A thin PDMS membrane separated the two structures, allowing the delivery of an oxygen gradient to Madin–Darby canine kidney (MDCK) cells seeded in the well. MDCK cells presented increased ROS levels under both hypoxic and hyperoxic conditions, which could be alleviated by an overnight incubation with vitamin C. ROS production by Chinese hamster ovary (CHO) cells was also quantified in a similar configuration, in an open-well chip with gas channels underneath.117 CHO cells were modified to express a mitochondrially-localized redox probe, whose fluorescence correlates with oxidation of the glutathione pool in the mitochondrial matrix. A decreased fluorescence was observed in response to the production of ROS under hypoxia, indicating a reduction in oxidative stress. This result was counterintuitive since ROS-induced oxidative stress would be expected to increase the oxidation of the (mitochondrial) glutathione pool and is indicative of subcellular differences in the (adaptive) response to ROS. Finally, using a hybrid device (similar to Fig. 3D) with chemical control of oxygen using a gas channel and a glass coating on the inner channel walls, ER stress was reported to increase along the oxygen gradient towards the lower oxygen areas, which was previously found to correlate with UPR activation,131 though this was not further investigated in that study.118
Activity of HIFs and hypoxia-triggered metabolic changes
As explained before, HIF-1 and HIF-2 complexes play major roles in the activation of cellular pathways in response to hypoxia following stabilization of the complex subunits HIF-1α and HIF-2α. Studies with microfluidic devices have shed new light on the role of these transcription factor complexes in hypoxia. For instance, PANC-1 cells grown in 2D were exposed to oxygen gradients created by perfusing oxygen and nitrogen compressed gases through two microchannels flanking the cell culture area (as depicted in Fig. 3C), and presented significant activation of HIF-1α below 2.5% oxygen, while remarkably, HIF-2α was activated along the entire gradient, even in the areas at normoxic conditions.88 This finding led to the conclusion that the stabilization of HIF-2α in normoxic cells is somehow mediated by the proximity of hypoxic cells. The underlying mechanism is not yet known but the authors hypothesized that it could include paracrine signals such as the secretion of exosomes, metabolites, or growth factors. Differences in cellular responses along an oxygen gradient were analysed using an open-well device assembled as a block with layers of silicone and acrylic in combination with oxygen control in gas channels (as depicted in Fig. 3E bottom).120 MDA-MB-231 breast cancer cells which were stably transfected with a reporter that expressed enhanced green fluorescent protein (eGFP) controlled by hypoxia-response elements (5HRE/eGFP),121 which would thus be triggered by HIF1 or HIF2 activation, were cultured in a gel on the bottom of the well. As expected, the eGFP expression directly correlated with the oxygen tension. Specifically, the device was stratified in four areas based on the oxygen tension, and in areas of the device where the oxygen tension was below 27 mmHg O2 (∼3.5% O2) a higher oxidative stress was found that was associated with a greater hypoxic response, which was not the case in devices not exposed to an oxygen gradient, where the eGFP intensity did not change significantly.120 Rexius-Hall et al. investigated the cellular response of human lung microvascular endothelial cells to oxygen tension in real-time using an open-well configuration equipped with two meandering gas channels below (device depicted in Fig. 3E top).122 This configuration allowed the creation simultaneously of both normoxic and hypoxic conditions having a small area in between with a steep oxygen gradient (0–21% O2). Translocation of HIF-1α to the nucleus was observed in these cells under hypoxic conditions, as well as upregulation of lactate dehydrogenase A (LDHA) expression, a downstream target of HIF-1. Upregulation of LDHA as well as other downstream target genes associated with metabolic switching towards glycolysis was also observed in human mesenchymal stem cells (MSCs), in the same device.
Several studies have focused on investigating the physiological effects of hypoxia on different cell markers and pathways related to cancer development, metastasis, and therapy resistance. One common response that tumour cells exhibit in a hypoxic microenvironment is the HIF-1-mediated increased reliance on glycolysis over oxidative phosphorylation.33 For instance, Ayuso et al. demonstrated that natural oxygen gradients, as found in tumour tissues, could successfully be emulated in a PS-based oxygen-impermeable device, together with an associated biochemical gradient of glucose (device as depicted in Fig. 3B).77 In recent work, we showed metabolic switching of U-251 glioblastoma cells in a microfluidic device with controlled hypoxia (device as depicted in Fig. 3A).79 The gradient in oxygen towards the middle of the tumour cell-containing a 3D collagen gel, was associated with a decrease in pH, as well as an increase in the uptake by the cells of a glucose analogue. These observations indicate that the cells increase their reliance on glycolysis for an oxygen independent energy production, which is likely a consequence of enhanced HIF-1 transcriptional activities; yet this was not verified. The reproduction of these acidic conditions in a 3D model is essential since it is also associated with drug resistance. Finally, another study that used a circular cell culture area with a monolayer focussed on analysing the expression of genes involved in proliferation, apoptosis, glycolysis, and migration in MCF-7 breast cancer cells isolated from specific normoxic or hypoxic regions of the device.132 Downregulation of one proliferation gene (MKI67) as well as upregulation of genes involved in apoptosis, glycolysis and migration occurred in the most hypoxic region of the device, in comparison to the normoxic region.
Effects of intermittent hypoxia and co-cultures with hypoxic cells
Intermittent hypoxia has been found to influence cell migration, metabolic adaptation, and angiogenesis.68,69 Leveraging the capability of microfluidic devices to provide spatiotemporal control on the oxygen tension, the response of tumour cells to this condition was examined.81 That study revealed an increased cell motility upon intermittent hypoxia, with an optimum motility in the region of the device with 5% oxygen. As discussed in a previous section, tumour cells and endothelial cells grown in 3D preferentially migrated towards slightly hypoxic regions (∼5% oxygen). This was tested in a device with a hybrid method of oxygen control (as depicted in Fig. 3D) that used a PC embedded film and 8 h cycles of oxygen flow at different concentrations. An intermittent oxygen gradient was created towards the centre of the device by perfusing the oxygen at different concentrations through side channels next to the medium channels. The migratory behaviour was linked to the presence of metabolic or chemical gradients caused by the exposure to intermittent hypoxia. Interestingly, after cells were exposed to 8 h cycles of hypoxia, changes in migration speed occurred after approximately 4 h, even though the oxygen gradient in the device stabilized after 15 min. This observation could be explained by the time the α-subunits of HIF transcriptional complexes require after stabilization to translocate to the nucleus and upregulate the transcription of migration-related genes. Intermittent hypoxia was also applied in a device incorporating spheroids in collagen scaffolds, using a device with chemical oxygen control in side channels (as in Fig. 3C).83 Surprisingly, spheroids displayed a dynamic swelling-and-shrinking behaviour following this cyclic hypoxia condition, which had a clear impact on drug delivery. This oxygen-dependent swelling seemed not to be caused by changes in cell–cell interactions but rather by cytoplasmic swelling of individual cells.83
Both chronic and intermittent hypoxia also impact angiogenesis, which was studied using a co-culture of fibroblasts and endothelial cells in a fibrin hydrogel, leading to the formation of a 3D vascular network.85 After formation of this network, fibroblasts alone in fibrin hydrogel were introduced in outer culture chambers to drive the expansion of the vasculature towards these chambers. To control the oxygen across the devices, two other channels were perfused with oxygen-scavenging chemicals and/or normal medium, continuously, or intermittently, allowing the formation of uniform hypoxic conditions, an oxygen gradient, or intermittent hypoxia. Both during chronic and intermittent hypoxia, angiogenesis was directed towards the side of the chamber with the lowest oxygen concentrations. This effect was also stronger during intermittent hypoxia than during chronic hypoxia, which is in good agreement with the fact that fibroblasts secrete VEGF under intermittent hypoxia, and that secreted amounts of VEGF depend on the frequency of the oxygen cycles.133 Intermittent hypoxia has also been applied to investigate pancreatic islet physiology, in the view of improving their transplantation. For this, spheroidal aggregates of pancreatic endocrine cells were exposed to controllable levels of oxygen and glucose,124 revealing that pre-conditioning of the pancreatic islets to intermittent hypoxia increased their steady-state cytoplasmic calcium levels while inhibiting the triggered calcium pulse response that induces insulin secretion upon glucose stimulation.
Physiological barriers and oxygen in organ-on-chips
A subclass of organ-on-chip devices aim to replicate the physiological barriers of the human body, such as the blood–alveolar barrier,134,135 the blood–brain barrier,136 the intestinal barrier,137etc., typically using a compartmentalized approach with an apical and a basal chamber.138 Health-associated bacteria grow under hypoxic conditions, and in fact contribute to them by producing short-chain fatty acids (SCFAs) that stimulate oxidative phosphorylation in gut epithelial cells, whereas disease-associated bacteria do not produce SCFAs and are typically facultative anaerobe, meaning they can also grow under aerobic conditions. A first example, while not being microfluidic, made use of 50 mL culture tubes to establish a co-culture system for investigating the symbiotic relationship between oxygen-requiring intestinal Caco-2 cells and the super oxygen-sensitive health-associated Faecalibacterium prausnitzii (F. prausnitzii). By varying the oxygen supply through partly opening or fully closing the cap, Sadabad et al. observed large differences through analyses of metabolites; Caco-2 cells did no yet show signs of hypoxia after 24 h, indicating there was still sufficient oxygen in the medium.139
Recently, a gut microbiome (GuMI) device was developed where the crosstalk between the mucosal barrier of the colon and F. prausnitzii could be studied in a long-term culture.140 The researchers were able to stably establish completely anoxic conditions in the apical side of the GuMI device and aerobic conditions in the basal side. This dual oxygen settings promoted the colon epithelial cells to create a tight barrier and towards a more differentiated state than under static conditions, with a global activation of hypoxia-responsive genes and pathways, despite the aerobic conditions at the basal side (∼17% O2). The presence of flow in the device was crucial for the establishment of the distinct oxygen levels in the two compartments, and creating associated metabolic effects, both in bacteria and colon epithelial cells cultured in the apical compartment. Additionally, the presence of F. prausnitzii in the apical side of the device gave rise to anti-inflammatory effects by producing metabolites that help downregulate toll-like receptors 3 and 4 and histone deacetylases, similar to what has been reported in vivo. The gut oxygen conditions were also emulated in a two-compartment device separated by a porous membrane.141 Caco-2 cells were grown on the membrane with their basal side facing O2 flow and the facultative anaerobic bacteria Escherichia coli and the anaerobic Bifidobacterium adolescentis were added on the apical side. On the top of the device a thick 5 mm extra layer of PDMS was added as a cap to slow down oxygen diffusion. This extra layer, combined with the fast consumption of the oxygen in the medium by the cell monolayer, was shown to be sufficient to create an anaerobic environment in the side of the device corresponding to the apical side of the Caco-2 cells. As expected, facultative anaerobic bacteria grew at slower rates in the device supplemented with the thick PDMS layer and Caco-2 cell activation of hypoxia markers correlated with a lower oxygen tension in the device. All these examples illustrate the capability and relevance of compartmental control of oxygen conditions in microfluidic devices to study the effect of distinct oxygen levels on the gut microbiome.
Investigation of hypoxia-targeted therapies
As a last example, hypoxia-targeted therapies have been investigated in microfluidic devices with controlled oxygen tension, in the context of cancer. A commonly used chemotherapeutic drug to target cancer cells in a hypoxic microenvironment is tirapazamine (TPZ). TPZ is known to become activated and thus toxic after being reduced in low oxygen environments, which leads to the formation of free radicals and, in turn, DNA damage.142 This hypoxia-dependent toxicity of TPZ was investigated in an oxygen gradient, revealing an increased sensitivity to TPZ when the oxygen tension diminished.93,126 In contrast, a decreased toxicity under low oxygen tension of another, oxygen-dependent chemotherapeutic, bleomycin, was found.
CAR-T cell immunotherapies were influenced by oxygen gradients, with enhanced activity of those CAR-T cells in the normoxic areas of the device and lower cell cytotoxicity in the hypoxic areas.125 The decreased activity of the CAR-T cells could be explained by an increase in surface expression of the immunosuppressor molecule PD-L1 by tumour cells linked to HIF-1α stabilization under hypoxic conditions, or by the competition for glucose specifically under hypoxic conditions. The reduced activity observed in hypoxic regions could help explain why CAR-T cell therapies are more effective in liquid tumours than in solid tumours, which often present large hypoxic regions.
Oxygen control in microfluidic devices: our vision for the future
Over the last years, and as discussed in this review, significant technological/engineering advancements have enhanced our ability to accurately control oxygen levels in in vitro models of healthy and/or diseased tissues, which have altogether created a plethora of opportunities for novel or better-designed biological and (bio)medical studies. Reproducing the (patho-)physiological oxygen levels as observed in tissues in vivo is always recommended over the commonly used in vitro hyperoxic conditions. Cells growing under such conditions may in some instances present oxygen toxicity,2 and in other cases reduced cell death.123 It may also simply fail to reflect physiological conditions.1 Still, given the immense importance of oxygen control, the uptake of experimental approaches that combine physiologically relevant in vitro cellular models with engineered systems that have controllable oxygen conditions is low. In the following, we elaborate on reasons for this and obstacles to be lifted, and we offer our vision of the future, combining both technological and biological aspects, as schematically summarized in Fig. 4.
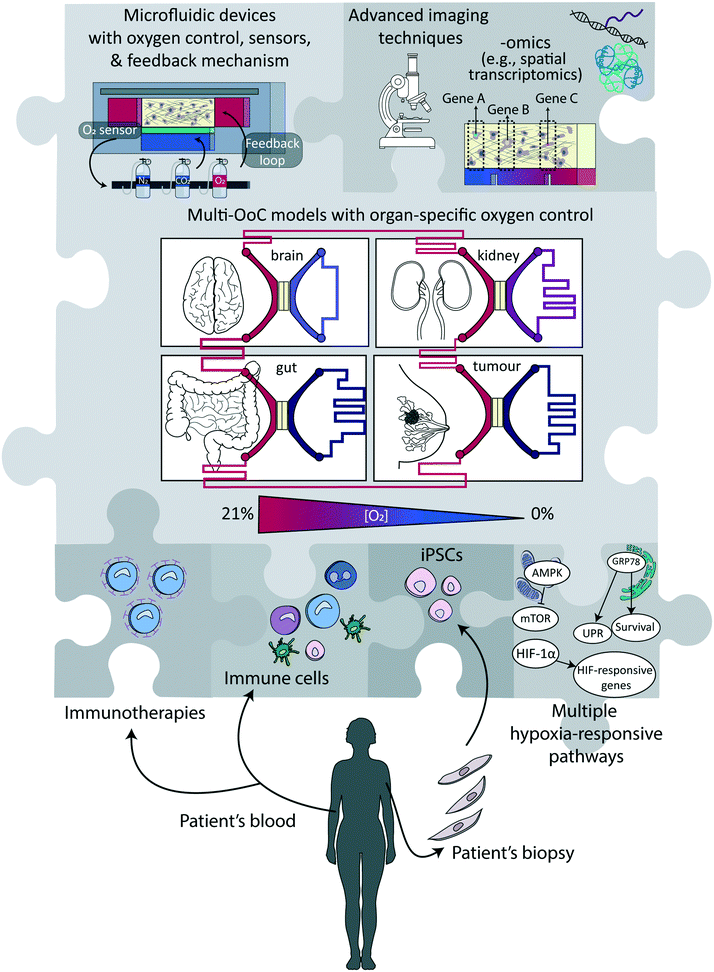 |
| Fig. 4 Potential elements in next-generation (multi)-organ-on-a-chip devices involving oxygen control. To create next-generation (multi)-organ-on-a-chip devices with an improved ability to mimic physiological conditions in humans, various elements indicated as ‘pieces of a puzzle’ can be combined in ways that are most suitable to address the research question at hand. | |
Technological advances
Arguably, one of the biggest challenges that remain to be addressed is to design technologies and approaches which are more user-friendly, and/or at least easy to implement in non-specialized laboratories. For now, most approaches discussed in this review require both access to dedicated equipment and building complex set-ups, which can represent an obstacle for their massive use by life science or medical researchers. Similarly, engineering challenges concomitantly need to be overcome to make these devices compatible with high-throughput microscopy, which can be achieved by parallelization, automation and increasing modularity. Modularity is of prime importance to create multi-organ-on-a-chip models for studying processes or diseases involving multiple organs or a systemic dimension.143 A preferred approach to that end would be to link different platforms representing distinct tissues and not to pursue a body-on-a-chip approach with uniform oxygen levels,144 since the different models are likely to be maintained at different oxygen levels, as happens in the human body (Fig. 2 and 4). Beyond that, an important next step is the standardization of protocols and devices to support ease of use and wide adoption.145 Especially for implementation in pharmaceutical settings, massive parallelization and reduction in cost-per-unit is essential. Noteworthy, this challenge is shared with most of the new developments in the fields of microfluidics and organ-on-a-chip technology.145–148
So far, the two engineering aspects of controlling and sensing oxygen levels have been mostly performed separately, or sensing was solely employed as a readout, to notably to calibrate the devices. Yet, coupling these two essential functions, with the incorporation of a feedback loop mechanism to also regulate the oxygen tension in the device could bring another experimental dimension. For instance, as discussed previously, one significant limitation of electrochemical sensors is that they actively consume oxygen, with the risk that the oxygen level in the device progressively deviates from the set value, especially if measurements are continuous. This limitation could be lifted with the implementation of an active regulation mechanism to ensure the oxygen level in the device would remain constant at the desired value, through continuous supplementation or removal of oxygen, e.g., by changing the oxygenation level either of the perfused medium or in the control layers. More importantly, the availability of active regulation would lighten the constraints on the materials used to fabricate the microfluidic devices. If oxygen would diffuse through PDMS, for instance, or any other gas-permeable material, adequate supply of medium with more or less oxygen, depending on the evolution of the system, would compensate once more for any deviation from the initially set oxygen level value. Next-generation devices combining continuous regulation and real-time sensing can equally benefit biological applications to help unravel possible correlations between oxygen level, changes therein due to cellular respiration, and disease progression or onset, while possibly maintaining the oxygen level in the device or altering it on-demand.
We focus here on oxygen, as an essential parameter of the cell microenvironment, which is present at different concentrations in different tissues and disease conditions. Yet, the engineering toolbox we discuss in this review could be extended to other important parameters. These include for instance nitrogen, carbon dioxide, and methane, which are all essential for the microbial ecosystem in the human gut microbiome.149 The pH of the solution represents another key candidate, as acidity, which is tightly linked to the CO2 level, differs strongly across tissues and between subcellular compartments. Both pH and CO2 play crucial roles in physiological and pathophysiological situations, including but not limited to lysosomal activities, brain function,150 tumour tissues,151 cardiovascular diseases as well as serious electrolyte disorders such as metabolic acidosis.152
Specific directions that might take hypoxia studies in microfluidic systems to the next level include the incorporation of cells derived from induced pluripotent stem cells (iPSCs) in order to overcome genetic differences between cells (Fig. 4),153 and to engineer them to be compatible with novel imaging techniques of 3D tissues like, e.g., light sheet microscopy,154 optical coherence tomography155 or wavefront shaping.156 Especially microscopy techniques that combine real-time information with direct visualisation of the hypoxia response, e.g., using fluorescent fusion proteins with HRE elements in their promoters, would provide more in-depth insights into signalling pathways at the single cell level. Furthermore, analyses have thus far focused mainly on HIF-1 as the main hypoxia-responsive pathway, while there are several more signalling pathways that play crucial roles in determining cellular function, notably involving mTOR and the UPR (Fig. 1 and 4). Molecular analyses of the cells, at the mRNA, protein or secretome levels, would provide complementary information to imaging techniques. Multichannel (cyclical) single molecule FISH (fluorescence in situ hybridization) techniques could be used for transcriptome level measurements while retaining the spatial resolution, which is key to correlate gene expression profile and local oxygen abundance.157 Other approaches that have been developed for off-chip analyses include the determination of the cytokine secretion profile (secretomics)158 and their metabolic activity using collected medium (metabolomics),159 or their protein expression profile (proteomics),160 as well as gene expression analysis or single-cell transcriptomic studies, notably single cell RNA sequencing, after recovery of the cellular materials.161
Innovative applications
The important roles of oxygen and related hypoxia in organ function and disease have now been firmly established. Yet, the existence of many unanswered questions warrants a bright future for innovations in the field of microfluidics and organ-on-a-chip with respect to better oxygen control. In Table 3, we highlight medical and scientific problems that would greatly benefit from the application of devices with precise oxygen control and possible tight regulation, to generate new fundamental knowledge and provide more appropriate tissue and disease models. Examples include the need for investigations of the interrelationship between autophagy, radiotherapy resistance and hypoxia, the need to better understand the oxygen-dependence of liver metabolism and how drug detoxification is affected by it in the three zones of the liver sinusoid. Furthermore, the replication of the oxygen concentration at different stages of embryo development at the microscale level has the potential to further improve IVF treatments, and investigations on the exposure to intermittent hypoxia in pancreatic islets could provide valuable information regarding the suitability of pancreatic islets for transplantation.
Table 3 Opportunities for devices with advanced oxygen control and regulation to address scientific and/or medical problems
Disease/organ/application |
What we know |
What we do not know |
Solid tumours |
Immunotherapy resistance |
Immune cells exhibit reduced tumour cell-killing activity under hypoxic conditions |
What is the (quantitative) relationship between the oxygenation level of tumour tissues and the activity of anti-cancer immunotherapies? |
|
Radiotherapy resistance |
Hypoxic conditions impact the ROS production and thereby therapy effectiveness, and autophagy supports cancer cell survival |
How autophagy blockade under hypoxic conditions can be exploited to increase the effectiveness of radiotherapy? |
|
Metastasis formation |
Hypoxia increases the migratory and invasive properties of tumour cells through enhanced EMT |
Can hypoxia-targeted anti-tumour drugs reduce EMT and thereby metastasis potential? |
|
Liver |
Liver diseases |
In non-alcoholic fatty liver disease (NAFLD) there is a differential accumulation of lipids in the different zones of the liver in an oxygen-dependent manner.51–53 |
Can the recapitulation of the oxygen gradients together with liver zonation in an in vitro model help to better understand NAFLD (or other liver metabolic diseases) and facilitate the development of new therapies? |
|
Drug metabolism in liver |
(Detoxifying) enzymes are differentially expressed in the three zones of the liver |
What is the effect of an oxygen gradient on drug metabolism in the liver? |
|
Brain |
In vitro models for neurobiology studies |
Brain organoids stop growing when they reach a size of ∼4 mm because of poor oxygen diffusion to the core |
To what extent is oxygen diffusion a limiting factor in those in vitro models of brain development and function and how could the incorporation of a vasculature-aided oxygen control improve brain organoid size and functionality? |
|
Stroke |
Neurons die when patients suffer from a stroke. Our understanding of the relative contribution of oxygen deprivation and reperfusion injury to neuron toxicity is limited in the context of stroke. Glial cells migrate towards the brain lesion after a stroke, forming a glial scar |
Can an in vitro brain-on-a-chip model that simulates the oxygen deprivation and reperfusion help understand the role of oxygen in neuronal death following a stroke? |
|
What is the role of oxygen gradients in glial cell migration induced upon a stroke? |
|
In vitro fertilization |
Embryo culture for in vitro fertilization |
In the oviduct and afterwards in the uterus, embryos grow under hypoxic conditions during their first stages of (pre-implantation) development, but this is not yet standardized in in vitro procedures |
Can embryo quality be improved by better emulating the in vivo situation with tight regulation of the oxygen tension during this pre-implantation period? |
|
Fibrosis |
|
Hypoxia has been linked to a variety of fibrotic diseases (e.g., systemic sclerosis, endometriosis) |
Does hypoxia have a causal role in the development of fibrotic diseases or is it merely a consequence? |
|
Diabetes |
Pancreatic islets transplantation |
Exposure to intermittent hypoxia occurs in vivo in pancreatic islets and plays a role in physiology |
Does the exposure to microscale-regulated intermittent hypoxia in vitro affect the suitability of pancreatic islets for transplantation? |
|
Gut |
Gut microbiome |
The composition of the gut microbiome is affected by hypoxia and the presence of oxygen gradients in the gut |
How does the oxygen-dependent composition of the gut microbiome affect cancer immunotherapies and inflammation-associated gut disorders? |
As an illustrative example to highlight where a wider implementation of devices with oxygen control is very likely to benefit research, we will discuss in depth the field of immunotherapy (1st example in Table 3), where new approaches against cancer or infectious diseases are being developed. It has become increasingly evident that the activity of many types of immune cells is profoundly affected by hypoxia. For instance, macrophages massively infiltrate hypoxic regions in a tumour tissue, and remain trapped there, exerting several pro-tumoural functions as tumour-associated macrophages (TAM), including increasing invasion of cancer cells, metastasis and angiogenesis, a multifaceted immunosuppression and resistance to chemotherapy.162 Hypoxia furthermore attracts neutrophils and promotes their survival by inhibiting apoptosis and inducing glycolytic enzymes in a HIF-1-dependent manner.163 There is a striking feed-forward mechanism: neutrophils can further promote hypoxia, which in turn enhances their pro-tumorigenic profile. By relieving hypoxia in tumours, it was shown that neutrophils alter their profile, and become tumouricidal, which altogether leads to a massive reduction in tumour burden.164 Arguably, these potent effects of oxygen and its regulation have long been underappreciated due to the lack of suitable models at the right oxygen level to study immune cell function. Myeloid-derived suppressor cells (MDSCs) are a further suppressive immune cell type that often accumulates after therapeutic interventions in hypoxic tumour regions; once more, mitigation of hypoxia was reported to block tumour infiltration of MDSCs.165 Hypoxia also increases the expression of the innate immune checkpoint CD47166 and the adaptive immune checkpoint PD-L1,167,168 which contributes to a reduced potential of immune cells or immune cell-activating therapies to control tumours. While many of these effects are directly mediated by HIF signalling pathways and are therefore likely oxygen-dependent, it is often unclear to what extent the co-occurring acidity in hypoxic tissues could also play a role, as many immunosuppressive effects have also been associated with extracellular acidosis in infections and in solid tumours.169 Furthermore, mTOR signalling is oxygen-dependent and has profound and context-dependent effects on immune function, as illustrated also by the immunosuppressive170 or immunostimulatory171 effects of the mTOR inhibitor rapamycin at various concentrations in distinct settings. The role and oxygen-dependent activity of mTOR should therefore also be considered when studying immune cell function.
Advanced 3D in vitro models can have control over cell density, interstitial flow, and matrix composition as well as the ability to independently control and monitor oxygen levels and acidity. When combined with the visualisation of cellular processes with a high resolution in real time, this will radically change the way studies are conducted on therapeutic immune cell-metabolic reprogramming approaches as well as (combination) therapies aimed at alleviating the local immunosuppression. Such models have particular promises for the study of nanoparticle-based approaches for targeting hypoxic areas in tumours, which often rely on HIF-1 inhibition or hypoxia-activated prodrugs.172 Alternatively, these models can be leveraged to investigate the impact of inhibiting the enzymatic activity of carbonic anhydrase IX (CAIX), a HIF-1-inducible protein, which has the potential to reverse extracellular acidosis under hypoxic conditions.173 Since CAIX is highly overexpressed on the surface of tumour cells in hypoxic regions and internalizes very fast by endocytosis,174 it is an excellent marker and target for the specific delivery of therapeutic or diagnostic agents, e.g., via antibodies, nanobodies, or affibodies.175–177 Ideally, cellular models that are being exploited for the testing of hypoxia-targeted therapies should have built-in normoxic (tumour) regions in order to properly investigate the selectivity of targeting, the true role of the hypoxia in the observed effect, and to potentially separate these effects from those caused by acidosis to understand if these two parameters could act in a synergistic manner.
Conclusion
Major strides have been made in the design of novel miniaturized devices that support relevant in vitro cell culture conditions with physiological or, where this is applicable, pathological oxygen levels. While so far studies have mainly focused on the mere validation of technologies, the field is now ripe for a much broader uptake of advanced cellular models that include oxygen levels as seen in healthy or diseased human tissues, with easy-to-use solutions for non-specialized laboratories being the major hurdle to overcome. Given the multitude of cellular processes that are significantly affected by oxygen levels, our opinion is that down the line, generating appropriate oxygen levels should become a standard feature in essentially all in vitro cell and tissue culture studies.
Abbreviations and glossary
ALDOA | Aldolase A – enzyme involved in glucose metabolism (glycolysis) |
AMP | Adenosine monophosphate – organic compound that is produced during hydrolysis of high-energy phosphate bonds, notably ATP |
AMPK | Adenosine monophosphate-activated protein kinase – central energy sensor that stimulates ATP-producing pathways, and senses AMP |
n/a | Angiogenic factors – proteins that stimulate new blood vessel formation (angiogenesis) |
ATF6 | Activating transcription factor 6 – stress-responsive protein that mediates the UPR |
ATP | Adenosine triphosphate – the cell's ‘energy currency’: organic compound that provides energy to drive cellular processes |
ARNT | Aryl hydrocarbon nuclear translocator – constitutively expressed β-subunit of the HIF-1 transcription factor |
BiP | Binding immunoglobulin protein – another name for GRP78 |
CAIX | Carbonic anhydrase IX – downstream target of HIF-1 that catalyzes the interconversion between carbon dioxide and water and the dissociated ions of carbonic acid (bicarbonate and hydrogen ions) |
CAR-T cell | Chimeric antigen receptor T cell – genetically engineered T cell for use in immunotherapy |
c-MYC | MYC proto-oncogene – proto-oncogene involved in cell division, differentiation, and death |
EMT | Epithelial-to-mesenchymal transition – a transcription factor-driven process by which epithelial (tumour) cells lose their cell polarity and cell–cell adhesion properties and become more migratory and invasive |
ER | Endoplasmic reticulum – type of cellular organelle composed of a network of tubular membranes that is involved in the transport of molecules |
GLUT1 | Glucose transporter 1 – protein that facilitates cellular glucose import |
n/a | Glutathione – tripeptide antioxidant that prevents damage caused by reactive oxygen species (ROS) |
GRP78 | Glucose-regulated protein 78 – protein located in the ER involved in proper protein folding and stability |
GTPase | Enzyme class that hydrolyses the nucleotide guanosine triphosphate (GTP) to guanosine diphosphate (GDP) |
n/a | GTPase Rheb – GTPase that activates mTOR |
HIFs | Hypoxia inducible factors – transcription factors involved in the cellular response to hypoxia |
HK1 | Hexokinase 1 – enzyme involved in glucose metabolism (glycolysis) |
HREs | HIF-responsive elements – binding sites for HIFs in the DNA that activate HIF-responsive genes |
n/a | Immune evasion – a strategy used by tumours or pathogens to avoid recognition, attack and/or removal by the host's immune system |
IRE1 | Inositol-requiring enzyme 1 – stress-responsive protein that mediates the UPR |
n/a | Liver sinusoid – type of capillary where oxygen-rich blood is mixed with nutrient-rich blood |
LDHA | Lactate dehydrogenase A – downstream target of HIF-1 that is involved in energy metabolism |
MDSC | Myeloid-derived suppressor cells – immune cell type that contributes to the resistance to cancer (immuno) therapies |
n/a |
MKI67 – gene encoding for protein Ki-67, one of the most used cell proliferation markers |
mTOR | Mammalian target of rapamycin – central nutrient sensor that stimulates protein synthesis, growth, and survival |
NANOG | Homeobox protein NANOG – transcriptional factor involved in stem cell behaviour. Name derives from the Irish “Tír na nÓg” – land of the young |
OCT4 | Octamer-binding transcription factor 4 – transcription factor involved in stem cell behaviour |
ODD | Oxygen-dependent degradation domain – domain of HIFs that is hydroxylated (addition of an –OH group) at a proline residue and that regulates HIF degradation |
PD-L1 | Programmed death-ligand 1 – protein involved in suppressing the adaptive arm of the immune system and a target for cancer immunotherapies |
PERK | Protein kinase R (PKR)-like endoplasmic reticulum kinase – stress-responsive protein that mediates the UPR |
PHD | Prolyl hydroxylase domain protein – protein that hydroxylate (adds an –OH group) HIFs at their ODD |
PKM | Pyruvate kinase muscle isozyme – enzyme involved in glucose metabolism (glycolysis) |
PTM | Post-translational modification – addition of chemical moieties to proteins after their biosynthesis at the ribosomes |
n/a | Proteasome – a waste-disposal nanomachine present inside every cell |
ROS | Reactive oxygen species – highly reactive molecules that are involved in cellular signalling but that can cause macromolecular damage when produced in excess |
TIA | Transient ischemic attack – temporary neurological dysfunction caused by impeded blood flow without ensuing cell death |
TAM | Tumour-associated macrophage – macrophage subtype that is associated with tumours and has a pro-tumorigenic character |
UPR | Unfolded protein response – metabolic pathway activated upon endoplasmic reticulum (ER) stress to promote cell survival |
TSC1–TSC2 | Tuberous sclerosis complex 1–2 – key negative regulator of mTOR, which thereby reduces cellular growth |
VE-cadherin | Vascular endothelial cadherin – protein responsible for endothelial cell adhesion |
VEGF | Vascular endothelial growth factor – signalling protein that stimulates the growth of new blood vessels |
VHL E3 | Von Hippel–Lindau E3 – ubiquitin ligase – enzyme that attaches ubiquitin (small regulatory protein) to proteins to prime them for degradation |
Conflicts of interest
There are no conflicts to declare.
Acknowledgements
V. P.-C. was supported through an internal funding programme at the Radboud University Medical Center awarded to W. P. R. V. W. P. R. V. and S. L. G. acknowledge financial support from a TURBO (Twente University – RadBOudUMC) grant. S. L. G. acknowledges financial support from the CHIP-ME project (Cross-organ Human In Vitro Platforms for Metastatic Environments) funded by Health Holland (project TKI-LSH LSHM19012). We would like to thank Dr. Bastien Venzac for carefully reading the manuscript and providing valuable feedback.
References
- A. Al-Ani, D. Toms, D. Kondro, J. Thundathil, Y. Yu and M. Ungrin, PLoS One, 2018, 13, e0204269 CrossRef PubMed
.
- D. Lloyd and C. F. Williams, Adv. Microb. Physiol., 2015, 67, 293–314 CrossRef CAS PubMed
.
- B. Muz, P. de la Puente, F. Azab and A. K. Azab, Hypoxia, 2015, 3, 83–92 CrossRef PubMed
.
- C. Beyer, G. Schett, S. Gay, O. Distler and J. H. Distler, Arthritis Res. Ther., 2009, 11, 220 CrossRef PubMed
.
- M. Takasawa, J. S. Beech, T. D. Fryer, Y. T. Hong, J. L. Hughes, K. Igase, P. S. Jones, R. Smith, F. I. Aigbirhio, D. K. Menon, J. C. Clark and J. C. Baron, J. Cereb. Blood Flow Metab., 2007, 27, 679–689 CrossRef PubMed
.
- A. Valencia and J. H. Burgess, Circulation, 1969, 40, 641–652 CrossRef CAS PubMed
.
- S. Chouaib, M. Z. Noman, K. Kosmatopoulos and M. A. Curran, Oncogene, 2017, 36, 439–445 CrossRef CAS PubMed
.
- A. Sharma, J. F. Arambula, S. Koo, R. Kumar, H. Singh, J. L. Sessler and J. S. Kim, Chem. Soc. Rev., 2019, 48, 771–813 RSC
.
- S. L. Dunwoodie, Dev. Cell, 2009, 17, 755–773 CrossRef CAS PubMed
.
- J. E. Lafont, Int. J. Exp. Pathol., 2010, 91, 99–106 CrossRef CAS PubMed
.
- W. X. Hong, M. S. Hu, M. Esquivel, G. Y. Liang, R. C. Rennert, A. McArdle, K. J. Paik, D. Duscher, G. C. Gurtner, H. P. Lorenz and M. T. Longaker, Adv. Wound Care, 2014, 3, 390–399 CrossRef PubMed
.
- T. Kumar, R. Pandey and N. S. Chauhan, Front. Cell. Infect. Microbiol., 2020, 10, 227 CrossRef CAS PubMed
.
- C. Jensen and Y. Teng, Front. Mol. Biosci., 2020, 7, 33 CrossRef CAS PubMed
.
-
W. M. S. Russell and R. L. Burch, The principles of humane experimental technique, Methuen, London, 1959 Search PubMed
.
- R. C. Hubrecht and E. Carter, Animals, 2019, 9, 754 CrossRef PubMed
.
- N. P. Fenwick and D. Fraser, Anim. Welfare, 2005, 14, 367–377 CAS
.
- D. G. Hackam and D. A. Redelmeier, JAMA, 2006, 296, 1731–1732 CAS
.
- B. Fischer and B. D. Bavister, J. Reprod. Fertil., 1993, 99, 673–679 CrossRef CAS PubMed
.
- L. H. Gray and J. M. Steadman, J. Physiol., 1964, 175, 161–171 CrossRef CAS PubMed
.
- S. N. Bhatia and D. E. Ingber, Nat. Biotechnol., 2014, 32, 760–772 CrossRef CAS PubMed
.
- E. W. Young and D. J. Beebe, Chem. Soc. Rev., 2010, 39, 1036–1048 RSC
.
- G. M. Whitesides, Nature, 2006, 442, 368–373 CrossRef CAS PubMed
.
- X. Wang, Z. M. Liu and Y. Pang, RSC Adv., 2017, 7, 29966–29984 RSC
.
- E. K. Sackmann, A. L. Fulton and D. J. Beebe, Nature, 2014, 507, 181–189 CrossRef CAS PubMed
.
- B. G. Wouters and M. Koritzinsky, Nat. Rev. Cancer, 2008, 8, 851–864 CrossRef CAS PubMed
.
- M. Miranda-Galvis and Y. Teng, Int. J. Mol. Sci., 2020, 21, 5487 CrossRef CAS PubMed
.
- P. H. Maxwell, M. S. Wiesener, G. W. Chang, S. C. Clifford, E. C. Vaux, M. E. Cockman, C. C. Wykoff, C. W. Pugh, E. R. Maher and P. J. Ratcliffe, Nature, 1999, 399, 271–275 CrossRef CAS PubMed
.
- J. Fandrey, T. A. Gorr and M. Gassmann, Cardiovasc. Res., 2006, 71, 642–651 CrossRef CAS PubMed
.
- J. Pouyssegur, F. Dayan and N. M. Mazure, Nature, 2006, 441, 437–443 CrossRef CAS PubMed
.
- J. P. Schöning, M. Monteiro and W. Gu, Clin. Exp. Pharmacol. Physiol., 2017, 44, 153–161 CrossRef PubMed
.
- S. L. Yang, C. Wu, Z. F. Xiong and X. Fang, Mol. Med. Rep., 2015, 12, 2411–2416 CrossRef CAS PubMed
.
- M. Y. Koh and G. Powis, Trends Biochem. Sci., 2012, 37, 364–372 CrossRef CAS PubMed
.
- G. L. Semenza, Curr. Opin. Genet. Dev., 2010, 20, 51–56 CrossRef CAS PubMed
.
- J. Jiang, Y. L. Tang and X. H. Liang, Cancer Biol. Ther., 2011, 11, 714–723 CrossRef CAS PubMed
.
- H. S. Leong and A. F. Chambers, Proc. Natl. Acad. Sci. U. S. A., 2014, 111, 887–888 CrossRef CAS PubMed
.
- P. Vaupel and G. Multhoff, Adv. Exp. Med. Biol., 2018, 1072, 171–175 CrossRef CAS PubMed
.
- S. Chipurupalli, E. Kannan, V. Tergaonkar, R. D’Andrea and N. Robinson, Int. J. Mol. Sci., 2019, 20, 749 CrossRef CAS PubMed
.
- M. Koritzinsky, F. Levitin, T. van den Beucken, R. A. Rumantir, N. J. Harding, K. C. Chu, P. C. Boutros, I. Braakman and B. G. Wouters, J. Cell Biol., 2013, 203, 615–627 CrossRef CAS PubMed
.
- A. S. Lee, Methods, 2005, 35, 373–381 CrossRef CAS PubMed
.
- K. Lee, W. Tirasophon, X. Shen, M. Michalak, R. Prywes, T. Okada, H. Yoshida, K. Mori and R. J. Kaufman, Genes Dev., 2002, 16, 452–466 CrossRef CAS PubMed
.
- L. Romero-Ramirez, H. Cao, D. Nelson, E. Hammond, A. H. Lee, H. Yoshida, K. Mori, L. H. Glimcher, N. C. Denko, A. J. Giaccia, Q. T. Le and A. C. Koong, Cancer Res., 2004, 64, 5943–5947 CrossRef CAS PubMed
.
- H. P. Harding, I. Novoa, Y. Zhang, H. Zeng, R. Wek, M. Schapira and D. Ron, Mol. Cell, 2000, 6, 1099–1108 CrossRef CAS PubMed
.
- J. Wu, D. T. Rutkowski, M. Dubois, J. Swathirajan, T. Saunders, J. Wang, B. Song, G. D. Yau and R. J. Kaufman, Dev. Cell, 2007, 13, 351–364 CrossRef CAS PubMed
.
- H. Yoshida, T. Matsui, A. Yamamoto, T. Okada and K. Mori, Cell, 2001, 107, 881–891 CrossRef CAS PubMed
.
- D. M. Sabatini, Proc. Natl. Acad. Sci. U. S. A., 2017, 114, 11818–11825 CrossRef CAS PubMed
.
- L. Liu, T. P. Cash, R. G. Jones, B. Keith, C. B. Thompson and M. C. Simon, Mol. Cell, 2006, 21, 521–531 CrossRef CAS PubMed
.
- Y. Li, K. Inoki and K. L. Guan, Mol. Cell. Biol., 2004, 24, 7965–7975 CrossRef CAS PubMed
.
- E. Ortiz-Prado, J. F. Dunn, J. Vasconez, D. Castillo and G. Viscor, Am. J. Blood Res., 2019, 9, 1–14 CAS
.
- J. Cervos-Navarro and N. H. Diemer, Crit. Rev. Neurobiol., 1991, 6, 149–182 CAS
.
- X. Qian, H. Song and G. L. Ming, Development, 2019, 146, 166074 CrossRef PubMed
.
- C. Fang, K. O. Lindros, T. M. Badger, M. J. Ronis and M. Ingelman-Sundberg, Hepatology, 1998, 27, 1304–1310 CrossRef CAS PubMed
.
- K. Jungermann and T. Kietzmann, Hepatology, 2000, 31, 255–260 CrossRef CAS PubMed
.
- T. Kietzmann, Redox Biol., 2017, 11, 622–630 CrossRef CAS PubMed
.
- E. Kasterstein, D. Strassburger, D. Komarovsky, O. Bern, A. Komsky, A. Raziel, S. Friedler and R. Ron-El, J. Assist. Reprod. Genet., 2013, 30, 1073–1079 CrossRef PubMed
.
- J. C. Dumoulin, C. J. Meijers, M. Bras, E. Coonen, J. P. Geraedts and J. L. Evers, Hum. Reprod., 1999, 14, 465–469 CrossRef CAS PubMed
.
- I. R. Botusan, V. G. Sunkari, O. Savu, A. I. Catrina, J. Grunler, S. Lindberg, T. Pereira, S. Yla-Herttuala, L. Poellinger, K. Brismar and S. B. Catrina, Proc. Natl. Acad. Sci. U. S. A., 2008, 105, 19426–19431 CrossRef CAS PubMed
.
- J. Morand, C. Arnaud, J. L. Pepin and D. Godin-Ribuot, Sci. Rep., 2018, 8, 2997 CrossRef PubMed
.
- E. A. Triantafyllou, I. Mylonis, G. Simos and E. Paraskeva, Hypoxia, 2019, 7, 87–91 CrossRef PubMed
.
- Y. Romero and A. Aquino-Galvez, Int. J. Mol. Sci., 2021, 22, 8335 CrossRef CAS PubMed
.
- G. Mehta, A. Y. Hsiao, M. Ingram, G. D. Luker and S. Takayama, J. Controlled Release, 2012, 164, 192–204 CrossRef CAS PubMed
.
- K. L. Eales, K. E. Hollinshead and D. A. Tennant, Oncogenesis, 2016, 5, e190 CrossRef CAS PubMed
.
- G. L. Semenza, Crit. Rev. Biochem. Mol. Biol., 2000, 35, 71–103 CrossRef CAS PubMed
.
- T. L. Clanton, J. Appl. Physiol., 2007, 102, 2379–2388 CrossRef CAS PubMed
.
- J. W. DiGiacomo and D. M. Gilkes, Target. Oncol., 2018, 13, 157–173 CrossRef PubMed
.
- W. Luo and Y. Wang, Adv. Exp. Med. Biol., 2019, 1136, 1–18 CrossRef CAS PubMed
.
- S. Terry, A. S. T. Engelsen, S. Buart, W. S. Elsayed, G. H. Venkatesh and S. Chouaib, Cancer Lett., 2020, 492, 1–10 CrossRef CAS PubMed
.
- M. Hockel and P. Vaupel, J. Natl. Cancer Inst., 2001, 93, 266–276 CrossRef CAS PubMed
.
- K. Saxena and M. K. Jolly, Biomolecules, 2019, 9, 339 CrossRef CAS PubMed
.
- C. Michiels, C. Tellier and O. Feron, Biochim. Biophys. Acta, 2016, 1866, 76–86 CAS
.
- M. D. Brennan, M. L. Rexius-Hall, L. J. Elgass and D. T. Eddington, Lab Chip, 2014, 14, 4305–4318 RSC
.
- P. E. Oomen, M. D. Skolimowski and E. Verpoorte, Lab Chip, 2016, 16, 3394–3414 RSC
.
- K. R. Rivera, M. A. Yokus, P. D. Erb, V. A. Pozdin and M. Daniele, Analyst, 2019, 144, 3190–3215 RSC
.
- S. G. Charati and S. A. Stern, Macromolecules, 1998, 31, 5529–5535 CrossRef CAS
.
- B. Harink, S. Le Gac, D. Barata, C. van Blitterswijk and P. Habibovic, Lab Chip, 2014, 14, 1816–1820 RSC
.
- J. M. Ayuso, R. Mongel, G. A. Llamazares, M. Moreno, M. Agirregabiria, J. Bergamo, M. Doblare, L. Ochoa and L. J. Fernandez, Front. Mater., 2015, 2, 37 Search PubMed
.
- M. Virumbrales-Munoz, J. M. Ayuso, M. Olave, R. Monge, D. de Miguel, L. Martinez-Lostao, S. Le Gac, M. Doblare, I. Ochoa and L. J. Fernandez, Sci. Rep., 2017, 7, 11998 CrossRef PubMed
.
- J. M. Ayuso, M. Virumbrales-Munoz, A. Lacueva, P. M. Lanuza, E. Checa-Chavarria, P. Botella, E. Fernandez, M. Doblare, S. J. Allison, R. M. Phillips, J. Pardo, L. J. Fernandez and I. Ochoa, Sci. Rep., 2016, 6, 36086 CrossRef CAS PubMed
.
- G. Mehta, J. Lee, W. Cha, Y. C. Tung, J. J. Linderman and S. Takayama, Anal. Chem., 2009, 81, 3714–3722 CrossRef CAS PubMed
.
- V. Palacio-Castañeda, L. Kooijman, B. Venzac, W. P. R. Verdurmen and S. Le Gac, Micromachines, 2020, 11, 382 CrossRef PubMed
.
- J. J. F. Sleeboom, J. Toonder and C. M. Sahlgren, Int. J. Mol. Sci., 2018, 19, 3047 CrossRef PubMed
.
- R. Koens, Y. Tabata, J. C. Serrano, S. Aratake, D. Yoshino, R. D. Kamm and K. Funamoto, APL Bioeng., 2020, 4, 016106 CrossRef CAS PubMed
.
- K. Funamoto, I. K. Zervantonakis, Y. Liu, C. J. Ochs, C. Kim and R. D. Kamm, Lab Chip, 2012, 12, 4855–4863 RSC
.
- S. M. Grist, S. S. Nasseri, L. Laplatine, J. C. Schmok, D. Yao, J. Hua, L. Chrostowski and K. C. Cheung, Sci. Rep., 2019, 9, 17782 CrossRef PubMed
.
- S. M. Grist, J. C. Schmok, M. C. Liu, L. Chrostowski and K. C. Cheung, Sensors, 2015, 15, 20030–20052 CrossRef CAS PubMed
.
- S. F. Lam, V. S. Shirure, Y. E. Chu, A. G. Soetikno and S. C. George, PLoS One, 2018, 13, e0209574 CrossRef CAS PubMed
.
- F. T. Lee-Montiel, S. M. George, A. H. Gough, A. D. Sharma, J. Wu, R. DeBiasio, L. A. Vernetti and D. L. Taylor, Exp. Biol. Med., 2017, 242, 1617–1632 CrossRef CAS PubMed
.
- M. A. Acosta, X. Jiang, P. K. Huang, K. B. Cutler, C. S. Grant, G. M. Walker and M. P. Gamcsik, Biomicrofluidics, 2014, 8, 054117 CrossRef PubMed
.
- M. L. Rexius-Hall, J. Rehman and D. T. Eddington, Integr. Biol., 2017, 9, 742–750 CrossRef CAS PubMed
.
- M. A. Acosta, X. Jiang, P. K. Huang, K. B. Cutler, C. S. Grant, G. M. Walker and M. P. Gamcsik, Biomicrofluidics, 2014, 8, 054117 CrossRef PubMed
.
- A. A. Jaeger, C. K. Das, N. Y. Morgan, R. H. Pursley, P. G. McQueen, M. D. Hall, T. J. Pohida and M. M. Gottesman, Biomaterials, 2013, 34, 8301–8313 CrossRef CAS PubMed
.
- S. Barmaki, V. Jokinen, D. Obermaier, D. Blokhina, M. Korhonen, R. H. A. Ras, J. Vuola, S. Franssila and E. Kankuri, Acta Biomater., 2018, 73, 167–179 CrossRef CAS PubMed
.
- B. Bulutoglu, C. Rey-Bedon, Y. B. A. Kang, S. Mert, M. L. Yarmush and O. B. Usta, Lab Chip, 2019, 19, 3022–3031 RSC
.
- Y. A. Chen, A. D. King, H. C. Shih, C. C. Peng, C. Y. Wu, W. H. Liao and Y. C. Tung, Lab Chip, 2011, 11, 3626–3633 RSC
.
- W. Sun, Y. Chen, Y. Wang, P. Luo, M. Zhang, H. Zhang and P. Hu, Analyst, 2018, 143, 5431–5437 RSC
.
- K. Funamoto, D. Yoshino, K. Matsubara, I. K. Zervantonakis, K. Funamoto, M. Nakayama, J. Masamune, Y. Kimura and R. D. Kamm, Integr. Biol., 2017, 9, 529–538 CrossRef CAS PubMed
.
- H. Nam, K. Funamoto and J. S. Jeon, Biomicrofluidics, 2020, 14, 044107 CrossRef CAS PubMed
.
- Y. Tabata, D. Yoshino, K. Funamoto, R. Koens, R. D. Kamm and K. Funamoto, Integr. Biol., 2019, 11, 26–35 CrossRef PubMed
.
- C. C. Wu, T. Saito, T. Yasukawa, H. Shiku, H. Abe, H. Hoshi and T. Matsue, Sens. Actuators, B, 2007, 125, 680–687 CrossRef CAS
.
- Y. Date, S. Takano, H. Shiku, K. Ino, T. Ito-Sasaki, M. Yokoo, H. Abe and T. Matsue, Biosens. Bioelectron., 2011, 30, 100–106 CrossRef CAS PubMed
.
- J. Kieninger, A. Weltin, H. Flamm and G. A. Urban, Lab Chip, 2018, 18, 1274–1291 RSC
.
- S. M. Grist, L. Chrostowski and K. C. Cheung, Sensors, 2010, 10, 9286–9316 CrossRef CAS PubMed
.
- C. C. Wu, T. Yasukawa, H. Shiku and T. Matsue, Sens. Actuators, B, 2005, 110, 342–349 CrossRef CAS
.
- K. Hiramoto, M. Yasumi, H. Ushio, A. Shunori, K. Ino, H. Shiku and T. Matsue, Anal. Chem., 2017, 89, 10303–10310 CrossRef CAS PubMed
.
- F. van Rossem, J. G. Bomer, H. L. de Boer, Y. Abbas, E. de Weerd, A. van den Berg and S. Le Gac, Sens. Actuators, B, 2017, 238, 1008–1016 CrossRef CAS
.
- E. Tanumihardja, R. H. Slaats, A. D. van der Meer, R. Passier, W. Olthuis and A. van den Berg, ACS Sens., 2021, 6, 267–274 CrossRef CAS PubMed
.
- A. Ruggi, F. W. B. van Leeuwen and A. H. Velders, Coord. Chem. Rev., 2011, 255, 2542–2554 CrossRef CAS
.
- G. Mehta, K. Mehta, D. Sud, J. W. Song, T. Bersano-Begey, N. Futai, Y. S. Heo, M. A. Mycek, J. J. Linderman and S. Takayama, Biomed. Microdevices, 2007, 9, 123–134 CrossRef CAS PubMed
.
- C. O'Donovan, E. Twomey, J. Alderman, T. Moore and D. Papkovsky, Lab Chip, 2006, 6, 1438–1444 RSC
.
- K. Q. Jiang, P. C. Thomas, S. P. Forry, D. L. DeVoe and S. R. Raghavan, Soft Matter, 2012, 8, 923–926 RSC
.
- S. M. Grist, N. Oyunerdene, J. Flueckiger, J. Kim, P. C. Wong, L. Chrostowski and K. C. Cheung, Analyst, 2014, 139, 5718–5727 RSC
.
- Z. Lin, T. Cherng-Wen, P. Roy and D. Trau, Lab Chip, 2009, 9, 257–262 RSC
.
- E. C. Jensen, Anat. Rec., 2012, 295, 2031–2036 CrossRef CAS PubMed
.
-
A. S. M. Virumbrales-Muñoz, R. Monge, J.-M. Ayuso, G. A. Llamazares, I. Ochoa, A. Ruggi, L. Fernández and S. Le Gac, MicroTAS conference 2016, Dublin (Ireland), 2016 Search PubMed.
- D. Yahara, T. Yoshida, Y. Enokida and E. Takahashi, Adv. Exp. Med. Biol., 2016, 923, 129–134 CrossRef CAS PubMed
.
- J. V. Joseph, S. Conroy, K. Pavlov, P. Sontakke, T. Tomar, E. Eggens-Meijer, V. Balasubramaniyan, M. Wagemakers, W. F. den Dunnen and F. A. Kruyt, Cancer Lett., 2015, 359, 107–116 CrossRef CAS PubMed
.
- H. C. Shih, T. A. Lee, H. M. Wu, P. L. Ko, W. H. Liao and Y. C. Tung, Sci. Rep., 2019, 9, 8234 CrossRef PubMed
.
- M. B. Byrne, M. T. Leslie, H. S. Patel, H. R. Gaskins and P. J. A. Kenis, Biomicrofluidics, 2017, 11, 054116 CrossRef PubMed
.
- D. H. Khan, S. A. Roberts, J. R. Cressman and N. Agrawal, Sci. Rep., 2017, 7, 13487 CrossRef PubMed
.
- J. F. Lo, E. Sinkala and D. T. Eddington, Lab Chip, 2010, 10, 2394–2401 RSC
.
- M. W. Boyce, W. C. Simke, R. M. Kenney and M. R. Lockett, Anal. Methods, 2020, 12, 18–24 RSC
.
- A. S. Truong and M. R. Lockett, Analyst, 2016, 141, 3874–3882 RSC
.
- M. L. Rexius-Hall, G. Mauleon, A. B. Malik, J. Rehman and D. T. Eddington, Lab Chip, 2014, 14, 4688–4695 RSC
.
- L. M. Tiede, E. A. Cook, B. Morsey and H. S. Fox, Cell Death Dis., 2011, 2, e246 CrossRef CAS PubMed
.
- J. F. Lo, Y. Wang, A. Blake, G. Yu, T. A. Harvat, H. Jeon, J. Oberholzer and D. T. Eddington, Anal. Chem., 2012, 84, 1987–1993 CrossRef CAS PubMed
.
- Y. Ando, E. L. Siegler, H. P. Ta, G. E. Cinay, H. Zhou, K. A. Gorrell, H. Au, B. M. Jarvis, P. Wang and K. Shen, Adv. Healthcare Mater., 2019, 8, e1900001 CrossRef PubMed
.
- L. Wang, W. Liu, Y. Wang, J. C. Wang, Q. Tu, R. Liu and J. Wang, Lab Chip, 2013, 13, 695–705 RSC
.
- J. M. Ayuso, R. Monge, A. Martinez-Gonzalez, M. Virumbrales-Munoz, G. A. Llamazares, J. Berganzo, A. Hernandez-Lain, J. Santolaria, M. Doblare, C. Hubert, J. N. Rich, P. Sanchez-Gomez, V. M. Perez-Garcia, I. Ochoa and L. J. Fernandez, Neuro-Oncology, 2017, 19, 503–513 CAS
.
- A. Gorlach, E. Y. Dimova, A. Petry, A. Martinez-Ruiz, P. Hernansanz-Agustin, A. P. Rolo, C. M. Palmeira and T. Kietzmann, Redox Biol., 2015, 6, 372–385 CrossRef PubMed
.
- R. L. Chen, U. H. Lai, L. L. Zhu, A. Singh, M. Ahmed and N. R. Forsyth, Front. Cell Dev. Biol., 2018, 6, 132 CrossRef PubMed
.
- M. Tafani, L. Sansone, F. Limana, T. Arcangeli, E. De Santis, M. Polese, M. Fini and M. A. Russo, Oxid. Med. Cell. Longevity, 2016, 2016, 3907147 Search PubMed
.
- D. R. Beriault and G. H. Werstuck, Biochim. Biophys. Acta, Mol. Cell Res., 2013, 1833, 2293–2301 CrossRef CAS PubMed
.
- Y. Ando, H. P. Ta, D. P. Yen, S. S. Lee, S. Raola and K. Shen, Sci. Rep., 2017, 7, 15233 CrossRef PubMed
.
- S. M. Ehsan and S. C. George, J. Biosci. Bioeng., 2015, 120, 347–350 CrossRef CAS PubMed
.
- D. Huh, B. D. Matthews, A. Mammoto, M. Montoya-Zavala, H. Y. Hsin and D. E. Ingber, Science, 2010, 328, 1662–1668 CrossRef CAS PubMed
.
- P. Zamprogno, S. Wuthrich, S. Achenbach, G. Thoma, J. D. Stucki, N. Hobi, N. Schneider-Daum, C. M. Lehr, H. Huwer, T. Geiser, R. A. Schmid and O. T. Guenat, Commun. Biol., 2021, 4, 168 CrossRef CAS PubMed
.
- A. Oddo, B. Peng, Z. Tong, Y. Wei, W. Y. Tong, H. Thissen and N. H. Voelcker, Trends Biotechnol., 2019, 37, 1295–1314 CrossRef CAS PubMed
.
- A. Bein, W. Shin, S. Jalili-Firoozinezhad, M. H. Park, A. Sontheimer-Phelps, A. Tovaglieri, A. Chalkiadaki, H. J. Kim and D. E. Ingber, Cell. Mol. Gastroenterol. Hepatol., 2018, 5, 659–668 CrossRef PubMed
.
- C. Moraes, G. Mehta, S. C. Lesher-Perez and S. Takayama, Ann. Biomed. Eng., 2012, 40, 1211–1227 CrossRef PubMed
.
- M. S. Sadabad, J. Z. H. von Martels, M. T. Khan, T. Blokzijl, G. Paglia, G. Dijkstra, H. J. M. Harmsen and K. N. Faber, Sci. Rep., 2015, 5, 17906 CrossRef PubMed
.
- J. Zhang, Y. J. Huang, J. Y. Yoon, J. Kemmitt, C. Wright, K. Schneider, P. Sphabmixay, V. Hernandez-Gordillo, S. J. Holcomb, B. Bhushan, G. Rohatgi, K. Benton, D. Carpenter, J. C. Kester, G. Eng, D. T. Breault, O. Yilmaz, M. Taketani, C. A. Voigt, R. L. Carrier, D. L. Trumper and L. G. Griffith, Med, 2021, 2, 74–98 e79 CrossRef PubMed
.
- C. Wang, T. Dang, J. Baste, A. Anil Joshi and A. Bhushan, FASEB J., 2021, 35, e21291 CAS
.
- K. B. Peters and J. M. Brown, Cancer Res., 2002, 62, 5248–5253 CAS
.
- N. Picollet-D’hahan, A. Zuchowska, I. Lemeunier and S. Le Gac, Trends Biotechnol., 2021, 39, 788–810 CrossRef PubMed
.
- J. H. Sung, Y. I. Wang, N. Narasimhan Sriram, M. Jackson, C. Long, J. J. Hickman and M. L. Shuler, Anal. Chem., 2019, 91, 330–351 CrossRef CAS PubMed
.
- Q. Ramadan and M. Zourob, Biomicrofluidics, 2020, 14, 041501 CrossRef CAS PubMed
.
- D. E. Watson, R. Hunziker and J. P. Wikswo, Exp. Biol. Med., 2017, 242, 1559–1572 CrossRef CAS PubMed
.
- Q. R. Wu, J. F. Liu, X. H. Wang, L. Y. Feng, J. B. Wu, X. L. Zhu, W. J. Wen and X. Q. Gong, Biomed. Eng. Online, 2020, 19, 9 CrossRef PubMed
.
- L. A. Low, C. Mummery, B. R. Berridge, C. P. Austin and D. A. Tagle, Nat. Rev. Drug Discovery, 2021, 20, 345–361 CrossRef CAS PubMed
.
- P. B. Hylemon, S. C. Harris and J. M. Ridlon, FEBS Lett., 2018, 592, 2070–2082 CrossRef CAS PubMed
.
- M. Obara, M. Szeliga and J. Albrecht, Neurochem. Int., 2008, 52, 905–919 CrossRef CAS PubMed
.
- X. Zhang, Y. Lin and R. J. Gillies, J. Nucl. Med., 2010, 51, 1167–1170 CrossRef CAS PubMed
.
- W. Aoi and Y. Marunaka, BioMed Res. Int., 2014, 2014, 598986 Search PubMed
.
- Y. Shi, H. Inoue, J. C. Wu and S. Yamanaka, Nat. Rev. Drug Discovery, 2017, 16, 115–130 CrossRef CAS PubMed
.
- I. Albert-Smet, A. Marcos-Vidal, J. J. Vaquero, M. Desco, A. Munoz-Barrutia and J. Ripoll, Front. Neuroanat., 2019, 13, 1 CrossRef CAS PubMed
.
- J. C. McIntosh, L. Yang, T. Wang, H. Zhou, M. R. Lockett and A. L. Oldenburg, Biomed. Opt. Express, 2020, 11, 3181–3194 CrossRef CAS PubMed
.
- A. Thendiyammal, G. Osnabrugge, T. Knop and I. M. Vellekoop, Opt. Lett., 2020, 45, 5101–5104 CrossRef PubMed
.
- C. L. Xia, J. Fan, G. Emanuel, J. J. Hao and X. W. Zhuang, Proc. Natl. Acad. Sci. U. S. A., 2019, 116, 19490–19499 CrossRef CAS PubMed
.
- Y. L. Deng, Y. Zhang, S. Sun, Z. H. Wang, M. J. Wang, B. Q. Yu, D. M. Czajkowsky, B. Y. Liu, Y. Li, W. Wei and Q. H. Shi, Sci. Rep., 2014, 4, 7499 CrossRef CAS PubMed
.
- D. A. Ouattara, J. M. Prot, A. Bunescu, M. E. Dumas, B. Elena-Herrmann, E. Leclerc and C. Brochot, Mol. BioSyst., 2012, 8, 1908–1920 RSC
.
- I. M. Lazar, N. S. Gulakowski and A. C. Lazar, Anal. Chem., 2020, 92, 169–182 CrossRef CAS PubMed
.
- R. J. Kimmerling, G. Lee Szeto, J. W. Li, A. S. Genshaft, S. W. Kazer, K. R. Payer, J. de Riba Borrajo, P. C. Blainey, D. J. Irvine, A. K. Shalek and S. R. Manalis, Nat. Commun., 2016, 7, 10220 CrossRef CAS PubMed
.
- A. T. Henze and M. Mazzone, J. Clin. Invest., 2016, 126, 3672–3679 CrossRef PubMed
.
- P. Sadiku and S. R. Walmsley, EMBO Rep., 2019, 20, e47388 CrossRef PubMed
.
- K. Mahiddine, A. Blaisdell, S. Ma, A. Crequer-Grandhomme, C. A. Lowell and A. Erlebacher, J. Clin. Invest., 2020, 130, 389–403 CrossRef CAS PubMed
.
- C. Zhang, D. L. Xia, J. H. Liu, D. Huo, X. Q. Jiang and Y. Hu, Adv. Funct. Mater., 2020, 30, 2000189 CrossRef CAS
.
- H. Zhang, H. Lu, L. Xiang, J. W. Bullen, C. Zhang, D. Samanta, D. M. Gilkes, J. He and G. L. Semenza, Proc. Natl. Acad. Sci. U. S. A., 2015, 112, E6215–E6223 CrossRef CAS PubMed
.
- Y. Messai, S. Gad, M. Z. Noman, G. Le Teuff, S. Couve, B. Janji, S. F. Kammerer, N. Rioux-Leclerc, M. Hasmim, S. Ferlicot, V. Baud, A. Mejean, D. R. Mole, S. Richard, A. M. M. Eggermont, L. Albiges, F. Mami-Chouaib, B. Escudier and S. Chouaib, Eur. Urol., 2016, 70, 623–632 CrossRef CAS PubMed
.
- I. B. Barsoum, C. A. Smallwood, D. R. Siemens and C. H. Graham, Cancer Res., 2014, 74, 665–674 CrossRef CAS PubMed
.
- F. E. Diaz, E. Dantas and J. Geffner, Mediators Inflammation, 2018, 2018, 1218297 Search PubMed
.
- R. N. Saunders, M. S. Metcalfe and M. L. Nicholson, Kidney Int., 2001, 59, 3–16 CrossRef CAS PubMed
.
- J. B. Mannick, M. Morris, H. U. P. Hockey, G. Roma, M. Beibel, K. Kulmatycki, M. Watkins, T. Shavlakadze, W. H. Zhou, D. Quinn, D. J. Glass and L. B. Klickstein, Sci. Transl. Med., 2018, 10, eaaq1564 CrossRef PubMed
.
- V. L. Silva and W. T. Al-Jamal, J. Controlled Release, 2017, 253, 82–96 CrossRef CAS PubMed
.
- Y. Lou, P. C. McDonald, A. Oloumi, S. Chia, C. Ostlund, A. Ahmadi, A. Kyle, U. Auf dem Keller, S. Leung, D. Huntsman, B. Clarke, B. W. Sutherland, D. Waterhouse, M. Bally, C. Roskelley, C. M. Overall, A. Minchinton, F. Pacchiano, F. Carta, A. Scozzafava, N. Touisni, J. Y. Winum, C. T. Supuran and S. Dedhar, Cancer Res., 2011, 71, 3364–3376 CrossRef CAS PubMed
.
- E. Bourseau-Guilmain, J. A. Menard, E. Lindqvist, V. Indira Chandran, H. C. Christianson, M. Cerezo Magana, J. Lidfeldt, G. Marko-Varga, C. Welinder and M. Belting, Nat. Commun., 2016, 7, 11371 CrossRef CAS PubMed
.
- F. J. Huizing, J. Garousi, J. Lok, G. Franssen, B. A. W. Hoeben, F. Y. Frejd, O. C. Boerman, J. Bussink, V. Tolmachev and S. Heskamp, Sci. Rep., 2019, 9, 18898 CrossRef CAS PubMed
.
- H. Honarvar, J. Garousi, E. Gunneriusson, I. Hoiden-Guthenberg, M. Altai, C. Widstrom, V. Tolmachev and F. Y. Frejd, Int. J. Oncol., 2015, 46, 513–520 CrossRef CAS PubMed
.
- A. S. van Brussel, A. Adams, S. Oliveira, B. Dorresteijn, M. El Khattabi, J. F. Vermeulen, E. van der Wall, W. P. Mali, P. W. Derksen, P. J. van Diest and P. M. van Bergen En Henegouwen, Mol. Imaging Biol., 2016, 18, 535–544 CrossRef CAS PubMed
.
|
This journal is © The Royal Society of Chemistry 2022 |