Single cell-asymmetrical flow field-flow fractionation/ICP-time of flight-mass spectrometry (sc-AF4/ICP-ToF-MS): an efficient alternative for the cleaning and multielemental analysis of individual cells†
Received
29th July 2022
, Accepted 8th November 2022
First published on 11th November 2022
Abstract
One of the greatest challenges in the analysis of single cells, quite often is the severe ionic background their samples exhibit. With that in mind, Asymmetrical Flow Field-Flow Fractionation (AF4), as a cleaning technique, was combined on-line with the multielemental analytical capabilities of an Inductively Coupled Plasma-Time of Flight-Mass Spectrometer (ICP-ToF-MS). In that manner, the heavy ionic matrix effect of untreated cells' samples can be significantly reduced. As a proof of concept, commercial baker's yeast cells were analysed. Their metal content can be determined on a single cell level, for multiple elements at a time. The combination yielded promising results, with the system exhibiting the ability to effectively limit the effect of matrices as severe as 3 × 105 mg per L sodium chloride (NaCl) or 50 mg per L ionic phosphorus (P). In the meantime, no hampering in the analysis of the cells was observed, due to clogging or otherwise equipment related issues. Furthermore, cells grown in heavy metal medium (lead, Pb, 0.1–10 mg L−1) were also analysed without prior cleaning, highlighting the ability for fast analysis without previous manual and/or laborious cleaning procedures. That fact, coupled with the ability of the ToF to detect the single cells' content for both the event related element (P) and the spiked element (Pb), on the same event, highlighted the potential of the further development and optimization of this fast, robust, and effective coupling and its future applicability to numerous life-science related applications. In addition, new and versatile data treatment tools were developed, and their performance was tested against established methods.
1. Introduction
Determining the metal content of single cells is a vital aspect of cellular analysis. It allows for the determination of different characteristics in cell populations, without falsely assuming homogeneity, since variability among distinct cells is taken into account.1 From the earliest stages of single cell analytical approaches,2 a significant amount of research has led to great progress in the field.3,4 Apart from unveiling secrets of nature,5 which often happens by studying heterogeneity among cell populations,6,7 many other critical aspects of everyday life and wellbeing rely on the further development or improvement of single cell analytical techniques. Studying the metal content, proteins and toxins at a single cell level can elevate the quality control protocols and results of the food industry to a higher and, nowadays, necessary level of competence.4,8–10 The rising need for theragnostic methods has also opened new possibilities for the integration of single cell analytical processes in the study of diseases, or their prevention,6,10–12 ultimately leading to an improved way of life.
Inductively Coupled Plasma-Mass Spectrometry (ICP-MS) has efficiently been incorporated in the analysis of individual cells,13,14 their behaviour in the ionization source,15 or the determination of their biological response to external stimuli.16 During the last years, mostly single and triple quadrupole or sector field mass spectrometers have been used. The development of Time-of-Flight Mass Analysers (ToF), exhibited the superiority of the multielemental analysis in such cases.17,18
However, there are inherent challenges in the analysis of cell samples (especially in high-throughput procedures), quite often stemming from the high complexity of their matrices.19,20 Despite single moieties, like particles and cells, being detected using shorter time frames (dwell time), higher ionic background leads to worse signal to noise ratios. That occasionally leads to difficulties in the distinction between the ionic and the particulate fraction of the samples.
A wide assortment of resources has been incorporated in assessing and trying to cope with matrix effects of any nature.21–23 (Gradient-/) centrifugation24,25 and (ultra-/micro-/) filtration26–28 are some of the most commonly used methods for separating cells and their constituents, or moieties within their size range, from their supernatant. They too, however, suffer from a number of limitations. Those include endangering the integrity of the cells, lack of automatization options, and low sample throughput, which is an essential hurdle in a potential theragnostic approach. While a lot of work has been done around introduction systems,29,30 and steps have been taken towards automatized procedures,31,32 a lot of ground is yet to be covered.
In this study, Asymmetrical Flow Field-Flow Fractionation (AF4) is used to efficiently reduce the effects of highly ionic matrices. AF4 is one of the most widely used variations of the Field Flow Fractionation (FFF) analytical techniques group.33 Due to the applied field being a liquid flow dispersed over a large surface, the technique is characterized by very low stress on the analytes. In addition, having the option of being connected to an autosampler, it can be used for a large number of samples, while the whole procedure can be fully automated. Furthermore, the ionic strength of the carrier phase can be modified, so that it will be suitable both for the elution of the analytes, and for the protection of the cells from osmotic shock.
To this day, there are multiple applications of AF4 being reported, in various fields, like biology34–36 or (nano-) medicine.37,38 Of particular interest to this study, were the numerous applications concerning nano- and microparticle fractionation and characterization,39–43 and even more so, the ones incorporating the AF4 in (single) particle analysis.44,45 Developing a protocol for nanoparticle analysis using AF4 can be a very complicated procedure, as there are multiple aspects that need to be optimized, and issues to be addressed.46–49 Despite being traditionally coupled with non-destructive detectors, like MALS, DLS etc.,41,50 the coupling of a mass spectrometer has also been reported, and is nowadays quite usual.36,44,51 Very recently, the benefits of the ToF mass analyser have been combined with the advantages of the AF4 technique, providing a very efficient tool for the multielemental analysis of individual particles.52 However, while FFF techniques have previously been incorporated in separation procedures of different cell populations,53–55 there is, to this day and to the authors' best knowledge, no mention of the AF4 being used in the field of single cell analysis.
Towards this goal, the incorporation of the AF4 technique, coupled on-line with a sc-ICP-ToF-MS instrument, is presented and discussed, with the purpose of developing an easy, on-line and all-round cell cleaning alternative for the multielemental analysis of single cells' metal content – in this application, yeast. In addition, the relevant data processing is addressed, with the development of targeted python-based coding scripts. By taking advantage of the immense capabilities of a programming language (like python),56 not only could the whole aforementioned procedure be controlled by a computer, but the same program could also evaluate the data in real-time. In this scenario, this complete procedure could indicate which of the samples should be further considered, e.g., for a specific treatment, at the push of a button.
2. Materials and methods
2.1. Yeast samples
For the preparation of the initial yeast samples, 0.1 g of dried baker's yeast and 0.1 g of crystalline sugar were suspended in 10 mL of ultrapure water (milliQ System, Merck KGaA, Germany). For the preparation of the Pb incubated cells, the appropriate Pb amount (CertiPUR®, lead ICP standard, Pb(NO3)2, in HNO3, 2–3% (v/v), 1000 mg L−1 Merck KGaA) was also added at this point. Following the initial suspension, the samples were periodically, lightly stirred and flipped, for the next 30 minutes. After that, they were deemed fit for analysis, and were kept in the fridge (4 °C) for a maximum of 4 days (no significant difference was observed during their analysis within that timeframe), except for the Pb incubated cells, which were only used during the next couple of hours. Any consequent dilutions, either in water or in heavy matrix solutions, were performed right before the injections, to minimise the cells' interactions with matrix components or the effect of ionic strength change. Sodium chloride (Suprapur®, Merck KGaA) was used for the sodium chloride matrix test, while phosphorus ICP standard (CertiPUR®, H3PO4 in H2O, 1000 mg L−1 P, Merck KGaA) was used for the phosphorus matrix test.
2.2. AF4/ICP-ToF-MS
An Asymmetrical Flow Field-Flow Fractionation system (AF2000 Focus, Postnova Analytics, GmbH, Germany), utilizing two solvent delivery systems (Postnova PN1122) and a vacuum degasser (Postnova PN7505) was coupled on-line to a laser light scattering detector (LLC) (Postnova PN3000), which was followed by an ICP-ToF-mass spectrometer (ICP-ToF-MS, 2R, TOFWERK, Switzerland). The ICP-ToF-MS was operated in single cell mode: the averaged spectrum acquisition rate was 100.2 Hz, corresponding to averaged mass spectra composed of data summed from 217 full mass spectra. The introduction system consisted of a quartz cyclonic spray chamber (Thermo Scientific, USA), and an SC-175 (Burgener Research Inc., Canada) nebulizer. The operating conditions for the ICP-ToF-MS are summarized in Table 1.
Table 1 ICP-ToF-MS operating conditions
Plasma power [W] |
1550 |
Plasma gas flow rate [L min−1] |
14 |
Auxiliary gas flow rate [L min−1] |
0.8 |
Nebulizer gas flow rate [L min−1] |
0.92 |
Integration time [ms] |
9.982 |
The AF4 channel was equipped with a 250 μm spacer (Postnova), and 10 kDa PES membrane (NADIR® UP010, MICRODYN-NADIR GmbH, Germany) on top of a ceramic frit. The carrier phase consisted of 100 mg L−1 SDS (PanPeac AppliChem, ITW Reagent) and 50 mg L−1 NaN3 (Sigma-Aldrich Chemie GmbH, Germany) in ultrapure water.
The membranes were regularly changed (every ∼30 runs), and their performance was tested and verified using a mix of latex particles of different sizes (Latex – LS – Mix, Postnova).
3. Results and discussion
3.1. Cells' and membranes' integrity testing
Flow cytometry was incorporated (BD Accuri C6 Cytometer, BD biosciences, Florida, USA) for the determination of the cell population in the samples, and the following determination of the technique's overall detection efficiency (0.102% ± 0.006%, measured 3 times in different samples, same conditions). The efficiency refers to the number of cells initially inserted to the channel versus the number of cell events detected by the ToF instrument. The currently low efficiency of the system was to be expected, e.g., taking into account the expected sample loss within the channel, and the introduction system not being optimal for cell analysis. However, the overall setup was sufficient for proving the concept.
Potential limits for memory effects were tested by obtaining acquisitions both before and after the samples. The upper concentration limit of the technique, e.g., followed by no significant memory effect (membrane staining), was determined using a dilution factor of 400 of the original samples (∼407
500 cells per mL), while the lower limit, i.e., still obtaining statistically significant numbers of detected events, was determined at a dilution factor of 1000 (∼163
000 cells per mL). The viable range of number concentrations for the sample is currently narrow but was deemed sufficient to prove the concept. The integrity of the cells after the AF4 channel, and before entering the plasma, was verified by collecting the relevant fragments from the tip of the nebulizer and examining them using a scanning electron microscope. Fig. 1 showcases the relevant results.
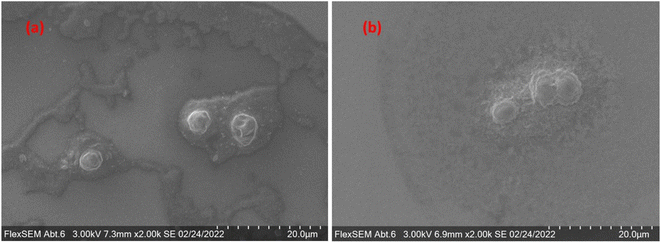 |
| Fig. 1 Microscopy (FlexSEM) images of (a) the sample before its entry to the system and (b) the sample after it exits the nebulizer. | |
3.2. Optimized measurement procedure
Developing a protocol for the analysis of single cells, incorporating an AF4 unit, understandably shares a lot of steps with the development of a protocol for nanoparticle samples.46 In addition, due to the fact that cells are a lot more fragile and stickier than most particles, additional restrictions must be taken into consideration. During the process of their cleaning, cells are prone to osmotic shock due to changes in ionic strength. Ergo, both the mobile phase's ionic strength, and the time of the overall analysis, can only vary within specific limits, so as not to damage the cells' population more than necessary. Both of those parameters, however, are also interlinked with other aspects of the procedure, like the sample adequately achieving equilibrium during the focusing step, and the entirely of it exiting the channel by the end of the rinsing step. That also includes any potential aggregation and agglomeration phenomena, as well as parts of the sample irreversible sticking to the membrane. All of the abovementioned considerations were taken into account while determining the composition of the mobile phase (see Section 2.2) through the trial-and-error process. The detector flow was kept constant at 1 mL min−1 throughout the procedure, while the pressure was also stabilized by a backpressure regulator, optimized at 9.1 ± 0.1 bar for this specific flow. The profiles of the various flows and the pressure in the channel for the duration of the method are depicted in Fig. 2.
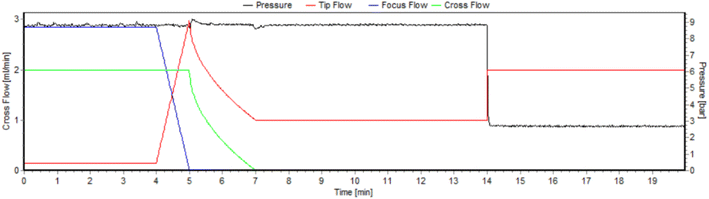 |
| Fig. 2 Profile of the tip (red), focus (blue) and cross (green) flow for the duration of the method. The right y-axis corresponds to the pressure within the channel. | |
3.3. Data processing
The result of the developed measurement procedure for this work, monitoring P for cell detection, is depicted in Fig. 3a, where the Laser Light Scattering (LLS) data (orange) match the corresponding ToF raw data (blue). The LLS data are briefly found out of the detector's range (∼7.5–9.5 min), due to the recording program's limitations. Two different approaches were tested for the processing of the resulting single-cell data: first, they were processed utilizing the particle processing module that is embedded in the operating software of the 2R ICP-ToF-MS instrument by TOFWERK. This processing is explained thoroughly by Meili-Borovinskaya et al.52 In order to obtain more control over the handling of the data, and the ability to access every individual step of the data processing and being able to monitor and altering it as well, a python-based script was developed. This was deemed necessary by the special nature of the data, due to the separation of the steps and the concentration of majority of the sample within a subsection of the dataset. The normalized cell histograms produced by the 2R software and the newly developed python script, with their corresponding Gaussian fits, are depicted in Fig. 3b and c respectively.
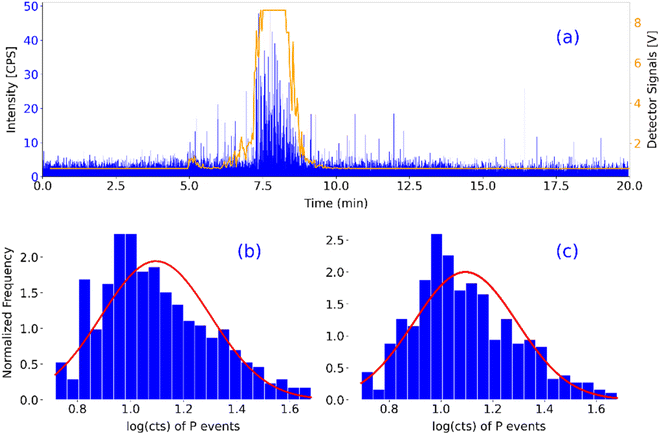 |
| Fig. 3 (a) Orange: online LLS detector's response, blue: ToF-MS response, of a single measurement. (b and c) Normalized histograms of the detected cells, as determined by (b) the instrument's own software and (c) the developed python script respectively. | |
The 2R software detected 393 cell related phosphorus events, with a mean value ± standard deviation of 1.10 ± 0.21 or 12.46 ± 1.60 cts. The in-house developed script detected 403 cell related phosphorus events, with a mean value of 1.10 ± 0.20 or 12.44 ± 1.58 cts. In terms of comparison, the two approaches are performing similarly, which means that the development of the script was successful. That fact was also validated for the rest of the presented results, as they were evaluated with both approaches. Ergo, only the results from the newly developed approach will be presented.
The basic schematic algorithm of the python script is depicted in Fig. 4. A more detailed, step by step description of the algorithm can be found in the ESI.† In essence, the code imports the raw data and segments the dataset into 1000-datapoints long pieces. Then, it applies to each of them a repetitive process, as described by Pace et al.,57 with the purpose of identifying particle signals, according to a given threshold value (in this work, Th = mean + 2.72 + 3.29 × SD).58 This approach is derived from the work of Meili-Borovinskaya et al.52 As a result, the original dataset is split into two datasets, one containing the cell-related pulses, and one containing everything else. Those datasets can then be accessed for the extraction of any useful information presented in this work, and potentially more. As mentioned before, each individual step can be altered to the user's discretion.
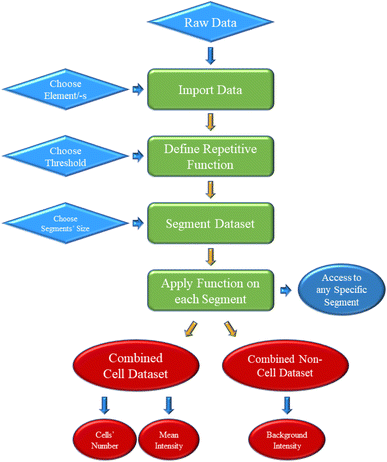 |
| Fig. 4 Developed python script's basic schematic algorithm. | |
The multielemental analytical capabilities of the ToF mass analyser allows for the simultaneous determination of more than one element, within the same pulse. From the perspective of single cell analysis, that can be an advantage, as the metal content of cell populations can be directly determined and correlated with each individual cell's size. Therefore, it is imperative for newly developed approaches to be able to distinguish which pulses contain the elements of interest, i.e., cell-related signals overlapped by metal related signals. Towards that goal, a second coding part was also developed, that is able to identify such overlapping signals, and therefore enable the safer study of crucial aspects of environmental research, eliminating the possibility of falsely identified signals. The same code allows for the calculation of the ratio of elements of interest for each individual cell.
The individual treatment of the segments was especially useful in this work, for two main reasons: the change in the flow and therefore the background level during the rinsing step, and the concentration of most of the sample in a 3 minute elution window. By exploiting the segmentation feature of the script (which is included in the 2R software as well), the cell dataset is mathematically separated from the background, even in cases that the separation is not clear from the histogram of the original dataset. In this manner, cells can be detected in sections of lower background levels, unaffected by higher background level in other sections of the dataset. This feature is depicted in Fig. 5, where the cell (blue) and non-cell (red) dataset splits of an original dataset are shown.
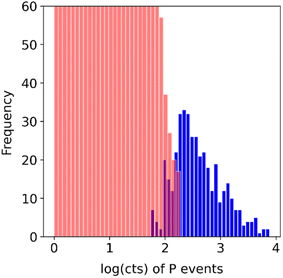 |
| Fig. 5 Cell (blue) and non-cell (red) dataset splits of a whole recorded dataset. | |
The apparent overlap between the separated background dataset and the separated cell dataset is a result of the difference in the background of different sections of the whole dataset. An example of this would be the obvious drop around the 14 minutes mark. Due to this, cells whose phosphorus content would not make them detectable in sections of the dataset with slightly elevated background, are being detected when found in sections with slightly lower background. Those events constitute part of the leftmost section of the cell dataset's histogram (blue).
3.4. Sodium chloride matrix tackling
Sodium chloride (NaCl) is one of the most commonly problematic matrix components, usually during the analysis of environmental samples (seawater salinity: ∼3.5% (w/w)).59 In the interest of pushing the boundaries of the technique and taking into account the solubility of NaCl in water (3.6 × 105 mg L−1), samples diluted by a factor of 1000 – in terms of cells, compared to the originally made samples – were made in 105 mg L−1, 2 × 105 mg L−1, and 3 × 105 mg L−1 NaCl. The matrix in this case is knowingly more severe than the usual needs of any analysis, as the purpose is proving the concept of matrix effect reduction. The dilution was performed right before the introduction to the instrument, to minimize the sample's exposure to the matrix, while the dilution factor matched the lower limit of the technique, as explained in Section 3.1. The results of the test are depicted in Fig. 6. The relevant histograms can be found in Fig. S1 in the ESI.†
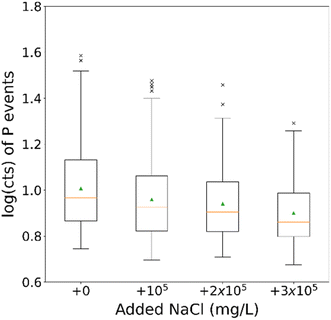 |
| Fig. 6 Boxplot of P events for a dilution factor of 1000 for the different added NaCl concentrations. The green arrows indicate the distributions' mean values. | |
Due to the way each dataset is processed (in 1000 datapoints-long segments) there are multiple values for the determined threshold, i.e., the critical value for the event determination, each one corresponding to one of the segments. The robust statistics (median, median absolute deviation [MAD] and interquartile range [IQR], all converted to cts) of the above-shown datasets and the thresholds of said datasets are summarised in Table 2.
Table 2 Summary of the robust statistics for the control sample, and the samples were 105, 2 × 105 and 3 × 105 mg L−1 of NaCl have been added
Sample |
Control |
105 mg L−1 NaCl |
2 × 105 mg L−1 NaCl |
3 × 105 mg L−1 NaCl |
Median absolute deviation.
Interquartile range.
|
No. of events |
128 |
153 |
94 |
86 |
Median |
9.23 |
8.44 |
8.01 |
7.25 |
MADa |
1.30 |
1.31 |
1.24 |
1.20 |
IQRb |
1.89 |
1.74 |
1.64 |
1.55 |
Thres. median |
7.64 |
6.47 |
6.42 |
6.48 |
Thres. MADa |
0.44 |
0.27 |
0.28 |
0.19 |
Thres. IQRb |
1.44 |
0.97 |
0.95 |
0.99 |
Regarding the apparent decrease in median values, it is likely that it is a result of the NaCl matrix harming the cells prior to their introduction to the AF4 channel.60 The number of detected events however does not appear to follow a trend with increasing NaCl concentration. The same is true for the threshold values of the respective datasets, indicating that the addition of increasing NaCl concentration did not hamper the analysis of the sample, f.i. by increasing the background. Additionally, no sign of the NaCl hampering the analysis was observed while conducting it, as opposed to when much lower salt concentrations are used with more conventional sc-ICP-MS methods. Considering the above-mentioned observations, the technique seems to be effectively reducing the matrix effect on the analysis of the untreated cells samples, since even the exhibited differences can be considered a result of the matrix on the cells before the cleaning procedure could take effect. The conclusion is furthermore strengthened by the fact that the analysis of a sample of such severe matrix is practically impossible without previous treatment, in a case when the AF4 or some other cleaning approach is not incorporated.
3.5. Phosphorus matrix tackling
For the sake of proving the technique's matrix elimination capabilities, the second element chosen to be added in ionic form was phosphorus, since it is the one being monitored for the determination of cell events. Similarly, to the NaCl test mentioned above, a dilution factor of 400 was performed, again right before the introduction to the instrument, to minimize the sample's exposure to the matrix. The dilution factor chosen matched the highest tolerable limit of the technique, as explained in Section 3.1. The results for 0, 10 and 50 mg L−1 of ionic P added to the samples' matrix are depicted in Fig. 7. The relevant histograms can be found in Fig. S2 in the ESI.† The P concentrations were limited by the chemical composition of the standard solution used (phosphoric acid) and the changes it could cause to the sample's pH value.
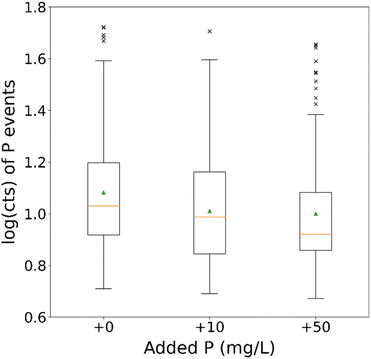 |
| Fig. 7 Boxplots of P events for a dilution factor of 400 for the different added ionic P concentrations. The green arrows indicate the distributions' mean values. | |
The robust statistics of the above-shown datasets and the thresholds of said datasets are summarized in Table 3.
Table 3 Summary of the robust statistics for the control sample, and the samples where 10 and 50 mg L−1 of ionic P have been added
Sample |
Control |
10 mg L−1 ionic P |
50 mg L−1 ionic P |
Median absolute deviation.
Interquartile range.
|
No. of events |
155 |
132 |
147 |
Median |
10.70 |
9.71 |
8.33 |
MADa |
0.13 |
0.15 |
0.10 |
IQRb |
0.28 |
0.32 |
0.22 |
Thres. median |
7.26 |
6.90 |
6.91 |
Thres. MADa |
0.24 |
0.36 |
0.84 |
Thres. IQRb |
1.30 |
1.31 |
1.59 |
There is once again an apparent decrease in the median values of the datasets, potentially as a result of the matrix (standard solution was based on phosphoric acid) affecting the cells before they enter the channel. All three samples, however, appear to contain a similar number of cell-related events, with no obvious trends, regardless of the difference in the added P in their matrix. In addition, the threshold values once again indicate that the critical values for the events' determination do not increase with increasing P concentration, which would be the case in the ionic fraction of the sample was not eliminated (that would lead to an increase of the background, and therefore the threshold). Considering the above-mentioned observations, it appears once again that the addition of ionic matrix did not hamper the analysis of the cells' samples. It did, however, make it possible without previous treatment of the sample, despite the high ionic matrix. Ergo, their cleaning has effectively reduced the matrix effect and the on-line sc-AF4/ICP-ToF-MS is sufficiently working.
3.6. Lead grown cells
Ecotoxicological studies, among others, necessitate the monitoring of more than one element in the same cell. To prove the technique's applicability in such a case, yeast cells were grown in Pb enriched environment, instead of ultrapure water. More specifically, 0.1, 1 and 10 mg L−1 of Pb were added during the culture of the cells, and the resulting samples were diluted by a factor of 400 and analysed after 30 minutes.
The detected P events in each case were as follows: 205 for the normally cultured sample, 258 for the one cultured in 0.1 mg L−1 of Pb, 179 for 1 mg L−1, and 215 for 10 mg L−1. The Pb events were 2 for the normally cultured sample, 6 for the one cultured in 0.1 mg L−1 of Pb, 35 for 1 mg L−1, and 305 for 10 mg L−1. Exploiting the potential of the second python script, the events of P signals that also contained Pb signals, i.e., cells that have accumulated Pb during their growth, were also identified. Therefore, the ratio of determined cells that have accumulated Pb could also be calculated, as well as the Pb counts to P counts ratio for each individual detected cell (used to compare the amount of Pb accumulated with increasing Pb concentration in the growth medium). The numbers of overlapping signals and their respective ratios in each case are: 0 for the normally cultured sample (0% overlap), 1 for the sample cultured in 0.1 mg L−1 of Pb (∼0.4% overlap), 21 for 1 mg L−1 (∼11.7% overlap), and 163 for 10 mg L−1 (∼75.8% overlap). Regarding the Pb to P ratio of individual cells, the median of the sample cultured in 0.1 mg L−1 was 0.37 with 0 MAD (there is only a single datapoint identified to have both elements). For the sample cultured in 1 mg L−1, median was 0.39 with 0.08 MAD and 0.18 IQR, and for the sample cultured in 10 mg L−1, the median was 0.80 with 0.27 MAD and 0.57 IQR. The percentages of the overlapping signals (ergo, P events also containing Pb events) and the boxplot of the Pb/P count ratios for each individual cell for the different Pb concentrations in culture medium are depicted in Fig. 8.
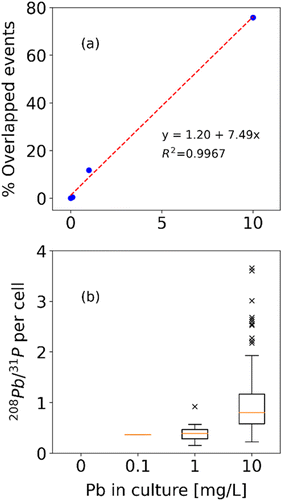 |
| Fig. 8 (a) Percentage of identified P events overlapped by Pb events and (b) boxplot of Pb to P per cell ratio for every detected cell for 0.1, 1 and 10 mg L−1 of Pb added during cell culturing. | |
The P signals detected do not exhibit a trend related to the added ionic Pb concentration, while the Pb signals understandably increase with increasing Pb concentration. The percentage of detected P signals that also correspond to a detected Pb signals, however, is exhibiting a clear and relative – almost linear – increase with increasing added ionic Pb concentration. That would be a clear indication, that when more Pb becomes available during the suspension culture of the cells, more cells are accumulating Pb at the same time, under the same conditions. Regarding the per cell accumulated Pb (Pb to P ratio) the trend is also clear and relative to the increasing Pb concentration. That would be a clear indication, that when more Pb becomes available during the suspension culture of the cells, individual cells accumulate higher amounts of Pb, on average. Both of those being the logical results of adding more Pb to the growth solution, it indicates the technique's capability to identify more than one element on the same cell event as well as its applicability for e.g., ecotoxicological studies. Moreover, the script's ability to identify which events contain both elements severely limit the possibility of false positive signals, and therefore false results. That constitutes another step towards a full automatization of the procedure.
4. Conclusions
The on-line coupling of the AF4 unit to the ICP-ToF-MS yielded promising results for the easy matrix cleaning and multi-elemental analysis of cell-related samples on a single-cell level. The mildness of the AF4 makes it an ideal purification tool, due to the cells fragility and tendency to agglomerate. In the meantime, the connection of an autosampler increases the possibility of automatization, which is highly needed in the field of single cell analysis. This can save time and resources, while the automatization can be further pushed in means of a data handling and process control, which is enabled by the newly developed python scripts. Taking the code's ability to identify overlapping signals into consideration as well, steps have been taken towards the full automatization of the procedure, from sample injection to results interpretation. By case-optimizing this high-throughput, automatized procedure, and tailoring it to the needs of the analysis, it can be a useful tool for ecotoxicological studies, as well as clinical trials on a single cell basis.
Conflicts of interest
The authors declare no competing financial interest.
Acknowledgements
The authors would like to thank Dr Steffen Weidner (Federal Institute for Materials Research and Testing, Division 6.3 – Structure Analysis) for his advice and his technical support regarding AF4 as well as the technical support team of Postnova Analytics GmbH, for their help with the AF2000 instrument that was used in this work. The authors also acknowledge the help of Mr Charlie Tobias (Federal Institute for Materials Research and Testing, Division 1.1 – Inorganic Trace Analysis) for the cytometry measurements, and of Ms. Olivia Netzband and Ms. Michaela Lagleder (Federal Institute for Materials Research and Testing, Division 6.1 – Surface Analysis and Interfacial Chemistry) for the microscopy images. Finally, the authors want to thank the German Federal Ministry for Economic Affairs and Climate Action (BMWK) for the financial support.
References
- S. P. Couvillion, Y. Zhu, G. Nagy, J. N. Adkins, C. Ansong and R. S. Renslow,
et al., New mass spectrometry technologies contributing towards comprehensive and high throughput omics analyses of single cells, Analyst, 2019, 144(3), 794–807 RSC.
- G. T. Matioli and H. B. Niewisch, Electrophoresis of hemoglobin in single erythrocytes, Science, 1965, 150(3705), 1824–1826 CrossRef CAS PubMed.
- T. Nomizu, S. Kaneco, T. Tanaka, D. Ito, H. Kawaguchi and B. T. Vallee, Determination of Calcium Content in Individual Biological Cells by Inductively Coupled Plasma Atomic Emission Spectrometry, Anal. Chem., 1994, 66(19), 3000–3004 CrossRef CAS.
- J. Sun, L. Gao, L. Wang and X. Sun, Recent advances in single-cell analysis: Encapsulation materials, analysis methods and integrative platform for microfluidic technology, Talanta, 2021, 234, 122671 CrossRef CAS PubMed.
- A. Regev, S. A. Teichmann, E. S. Lander, I. Amit, C. Benoist and E. Birney,
et al., The Human Cell Atlas, eLife, 2017, 6, e27041 CrossRef PubMed.
- I. Monga, K. Kaur and S. K. Dhanda, Revisiting hematopoiesis: applications of the bulk and single-cell transcriptomics dissecting transcriptional heterogeneity in hematopoietic stem cells, Briefings Funct. Genomics, 2022, 21(3), 159–176 CrossRef PubMed.
-
N. S. Barteneva and I. A. Vorobjev, Heterogeneity of Metazoan Cells and Beyond: To Integrative Analysis of Cellular Populations at Single-Cell Level, in Cellular Heterogeneity: Methods and Protocols, ed. N. S. Barteneva and I. A. Vorobjev, Springer, New York, 2018, pp. 3–23 Search PubMed.
- M. Marcinkowska and D. Baralkiewicz, Multielemental speciation analysis by advanced hyphenated technique - HPLC/ICP-MS: A review, Talanta, 2016, 161, 177–204 CrossRef CAS PubMed.
- K. Kleparnik, Recent advances in combination of capillary electrophoresis with mass spectrometry: Methodology and theory, Electrophoresis, 2015, 36(1), 159–178 CrossRef CAS PubMed.
- A. Taylor, N. Barlow, M. P. Day, S. Hill, N. Martin and M. Patriarca, Atomic Spectrometry Update: review of advances in the analysis of clinical and biological materials, foods and beverages, J. Anal. At. Spectrom., 2019, 34(3), 426–459 RSC.
- C. Lombard-Banek and J. E. Schiel, Mass Spectrometry Advances and Perspectives for the Characterization of Emerging Adoptive Cell Therapies, Molecules, 2020, 25(6), 1396 CrossRef CAS PubMed.
-
K. H. M. Sturgess, F. J. Calero-Nieto, B. Gottgens and N. K. Wilson, Single-cell analysis of hematopoietic stem cells, in BONE MARROW ENVIRONMENT: Methods and Protocols Methods in Molecular Biology, 2021, vol. 2038, pp. 301–337 Search PubMed.
- K.-S. Ho and W.-T. Chan, Time-resolved ICP-MS measurement for single-cell analysis and on-line cytometry, J. Anal. At. Spectrom., 2010, 25(7), 1114–1122 RSC.
- S.-i Miyashita, A. S. Groombridge, S.-I. Fujii, A. Minoda, A. Takatsu and A. Hioki,
et al., Highly efficient single-cell analysis of microbial cells by time-resolved inductively coupled plasma mass spectrometry, J. Anal. At. Spectrom., 2014, 29(9), 1598–1606 RSC.
- F. Li, D. W. Armstrong and R. S. Houk, Behavior of Bacteria in the Inductively Coupled Plasma: Atomization and Production of Atomic Ions for Mass Spectrometry, Anal. Chem., 2005, 77(5), 1407–1413 CrossRef CAS PubMed.
- W.-Y. Lau, K.-H. Chun and W.-T. Chan, Correlation of single-cell ICP-MS intensity distributions for the study of heterogeneous cellular responses to environmental stresses, J. Anal. At. Spectrom., 2017, 32(4), 807–815 RSC.
- D. R. Bandura, V. I. Baranov, O. I. Ornatsky, A. Antonov, R. Kinach and X. Lou,
et al., Mass Cytometry: Technique for Real Time Single Cell Multitarget Immunoassay Based on Inductively Coupled Plasma Time-of-Flight Mass Spectrometry, Anal. Chem., 2009, 81(16), 6813–6822 CrossRef CAS PubMed.
- L. Hendriks, A. Gundlach-Graham, B. Hattendorf and D. Günther, Characterization
of a new ICP-TOFMS instrument with continuous and discrete introduction of solutions, J. Anal. At. Spectrom., 2017, 32(3), 548–561 RSC.
- E. S. F. Berman, S. L. Fortson, K. D. Checchi, L. Wu, J. S. Felton and K. J. J. Wu,
et al., Preparation of Single Cells for Imaging/Profiling Mass Spectrometry, J. Am. Soc. Mass Spectrom., 2008, 19(8), 1230–1236 CrossRef CAS PubMed.
- M. Chiu, W. Lawi, S. Snyder, P.-K. Wong, J. Liao and V. Gau, Matrix Effect - A Challenge Toward Automation of Molecular Analysis, JALA, 2010, 15, 233–242 CAS.
- E. Chambers, D. M. Wagrowski-Diehl, Z. Lu and J. R. Mazzeo, Systematic and comprehensive strategy for reducing matrix effects in LC/MS/MS analyses, J. Chromatogr. B: Anal. Technol. Biomed. Life Sci., 2007, 852(1–2), 22–34 CrossRef CAS PubMed.
- C. Henderson, P. Lythgoe and K. Theis, A Pragmatic Approach to Coping with Matrix Effects during ICP-MS Analysis of Trace Elements in Silicate Rocks and Calibration of REE Interferences, J. Geosci. Environ. Prot., 2019, 07, 82–125 Search PubMed.
- B. K. Matuszewski, M. L. Constanzer and C. M. Chavez-Eng, Strategies for the assessment of matrix effect in quantitative bioanalytical methods based on HPLC-MS/MS, Anal. Chem., 2003, 75(13), 3019–3030 CrossRef CAS PubMed.
- A. S. Rathore, S. E. Sobacke, T. J. Kocot, D. R. Morgan, R. L. Dufield and N. M. Mozier, Analysis for residual host cell proteins and DNA in process streams of a recombinant protein product expressed in Escherichia coli cells, J. Pharm. Biomed. Anal., 2003, 32(6), 1199–1211 CrossRef CAS PubMed.
- X. G. Li, S. Li and G. Kellermann, An integrated liquid chromatography-tandem mass spectrometry approach for the ultra-sensitive determination of catecholamines in human peripheral blood mononuclear cells to assess neural-immune communication, J. Chromatogr. A, 2016, 1449, 54–61 CrossRef CAS PubMed.
- T. Mairinger, J. Trondle, M. Hanscho and S. Hann, On-line clean-up and LC-MS analysis of primary metabolites in cell culture supernatants, Anal. Methods, 2017, 9(38), 5703–5710 RSC.
- A. Mihalcea, C. Ungureanu, A. A. Chirvase and A. Onu, Separation by Microfiltration of Rhodotorula Rubra Cells from the Culture Broth, Rev. Chim., 2012, 63(5), 536–539 CAS.
- M. Gryta and W. Tomczak, Microfiltration of post-fermentation broth with backflushing membrane cleaning, Chem. Pap., 2015, 69(4), 544–552 CAS.
- M. Corte-Rodríguez, R. Álvarez-Fernández, P. García-Cancela, M. Montes-Bayón and J. Bettmer, Single cell ICP-MS using on line sample introduction systems: Current developments and remaining challenges, TrAC, Trends Anal. Chem., 2020, 132, 116042 CrossRef.
- C. Toncelli, K. Mylona, M. Tsapakis and S. A. Pergantis, Flow injection with on-line dilution and single particle inductively coupled plasma – mass spectrometry for monitoring silver nanoparticles in seawater and in marine microorganisms, J. Anal. At. Spectrom., 2016, 31(7), 1430–1439 RSC.
- M. Au, M. Schwinn, K. Kuhlmeier, C. Büchel and B. Meermann, Development of an automated on-line purification HPLC single cell-ICP-MS approach for fast diatom analysis, Anal. Chim. Acta, 2019, 1077, 87–94 CrossRef CAS PubMed.
- R. P. Lamsal, G. Jerkiewicz and D. Beauchemin, Flow Injection Single Particle Inductively Coupled Plasma Mass Spectrometry: An Original Simple Approach for the Characterization of Metal-Based Nanoparticles, Anal. Chem., 2016, 88(21), 10552–10558 CrossRef CAS PubMed.
-
M. Schimpf, K. Caldwell and J. C. Giddings, Field Flow Fractionation Handbook, Wiley-Interscience, New York, 2000 Search PubMed.
- H. Zhang, D. Freitas, H. S. Kim, K. Fabijanic, Z. Li and H. Chen,
et al., Identification of distinct nanoparticles and subsets of extracellular vesicles by asymmetric flow field-flow fractionation, Nat. Cell Biol., 2018, 20(3), 332–343 CrossRef CAS PubMed.
- H. Zhang and D. Lyden, Asymmetric-flow field-flow fractionation technology for exomere and small extracellular
vesicle separation and characterization, Nat. Protoc., 2019, 14(4), 1027–1053 CrossRef CAS PubMed.
- S. H. Kim, J. S. Yang, J. C. Lee, J. Y. Lee, J. Y. Lee and E. Kim,
et al., Lipidomic alterations in lipoproteins of patients with mild cognitive impairment and Alzheimer's disease by asymmetrical flow field-flow fractionation and nanoflow ultrahigh performance liquid chromatography-tandem mass spectrometry, J. Chromatogr. A, 2018, 1568, 91–100 CrossRef CAS PubMed.
- M. Wagner, S. Holzschuh, A. Traeger, A. Fahr and U. S. Schubert, Asymmetric flow field-flow fractionation in the field of nanomedicine, Anal. Chem., 2014, 86(11), 5201–5210 CrossRef CAS PubMed.
- F. Quattrini, G. Berrecoso, J. Crecente-Campo and M. J. Alonso, Asymmetric flow field-flow fractionation as a multifunctional technique for the characterization of polymeric nanocarriers, Drug Delivery Transl. Res., 2021, 11(2), 373–395 CrossRef CAS PubMed.
- P. Fedotov, N. Vanifatova, V. Shkinev and B. Spivakov, Fractionation and characterization of nano- and microparticles in liquid media, Anal. Bioanal. Chem., 2011, 400, 1787–1804 CrossRef CAS PubMed.
- A. I. Ivaneev, M. S. Ermolin and P. S. Fedotov, Separation, Characterization, and Analysis of Environmental Nano- and Microparticles: State-of-the-Art Methods and Approaches, J. Anal. Chem., 2021, 76(4), 413–429 CrossRef CAS.
- M. E. Collins, E. Soto-Cantu, R. Cueto and P. S. Russo, Separation and Characterization of Poly(tetrafluoroethylene) Latex Particles by Asymmetric Flow Field Flow Fractionation with Light-Scattering Detection, Langmuir, 2014, 30(12), 3373–3380 CrossRef CAS PubMed.
- B. Meermann, A.-L. Fabricius, L. Duester, F. Vanhaecke and T. Ternes, Fraction-related quantification of silver nanoparticles via on-line species-unspecific post-channel isotope dilution in combination with asymmetric flow-field-flow fractionation (AF4)/sector field ICP-mass spectrometry (ICP-SF-MS), J. Anal. At. Spectrom., 2014, 29(2), 287–296 RSC.
- B. Meermann, K. Wichmann, F. Lauer, F. Vanhaecke and T. A. Ternes, Application of stable isotopes and AF4/ICP-SFMS for simultaneous tracing and quantification of iron oxide nanoparticles in a sediment–slurry matrix, J. Anal. At. Spectrom., 2016, 31(4), 890–901 RSC.
- K. A. Huynh, E. Siska, E. Heithmar, S. Tadjiki and S. A. Pergantis, Detection and Quantification of Silver Nanoparticles at Environmentally Relevant Concentrations Using Asymmetric Flow Field-Flow Fractionation Online with Single Particle Inductively Coupled Plasma Mass Spectrometry, Anal. Chem., 2016, 88(9), 4909–4916 CrossRef CAS PubMed.
- D. M. Mitrano, A. Barber, A. Bednar, P. Westerhoff, C. P. Higgins and J. F. Ranville, Silver nanoparticle characterization using single particle ICP-MS (SP-ICP-MS) and asymmetrical flow field flow fractionation ICP-MS (AF4-ICP-MS), J. Anal. At. Spectrom., 2012, 27(7), 1131–1142 RSC.
- J. Gigault, J. M. Pettibone, C. Schmitt and V. A. Hackley, Rational strategy for characterization of nanoscale particles by asymmetric-flow field flow fractionation: A tutorial, Anal. Chim. Acta, 2014, 809, 9–24 CrossRef CAS PubMed.
- J. S. Yang and M. H. Moon, Flow optimisations with increased channel thickness in asymmetrical flow field-flow fractionation, J. Chromatogr. A, 2018, 1581–1582, 100–104 CrossRef CAS PubMed.
- K. G. Wahlund and A. Zattoni, Size separation of supermicrometer particles in asymmetrical flow field-flow fractionation. Flow conditions for rapid elution, Anal. Chem., 2002, 74(21), 5621–5628 CrossRef CAS PubMed.
- M. H. Moon, P. S. Williams, D. Kang and I. Hwang, Field and flow programming in frit-inlet asymmetrical flow field-flow fractionation, J. Chromatogr. A, 2002, 955(2), 263–272 CrossRef PubMed.
- T. J. Cho and V. A. Hackley, Fractionation and characterization of gold nanoparticles in aqueous solution: asymmetric-flow field flow fractionation with MALS, DLS, and UV–Vis detection, Anal. Bioanal. Chem., 2010, 398(5), 2003–2018 CrossRef CAS PubMed.
- J. S. Yang, J. Y. Kim, J. C. Lee and M. H. Moon, Investigation of lipidomic perturbations in oxidatively stressed subcellular organelles and exosomes by asymmetrical flow field–flow fractionation and nanoflow ultrahigh performance liquid chromatography–tandem mass spectrometry, Anal. Chim. Acta, 2019, 1073, 79–89 CrossRef CAS PubMed.
- O. Meili-Borovinskaya, F. Meier, R. Drexel, M. Baalousha, L. Flamigni and A. Hegetschweiler,
et al., Analysis of complex particle mixtures by asymmetrical flow field-flow fractionation coupled to inductively coupled plasma time-of-flight mass spectrometry, J. Chromatogr. A, 2021, 1641, 461981 CrossRef CAS PubMed.
- K. D. Caldwell, Z. Q. Cheng, P. Hradecky and J. C. Giddings, Separation of human and animal cells by steric field-flow fractionation, Cell Biophys., 1984, 6(4), 233–251 CrossRef CAS PubMed.
- J. C. Bigelow, J. C. Giddings, Y. Nabeshima, T. Tsuruta, K. Kataoka and T. Okano,
et al., Separation of B and T lymphocytes by a hybrid field-flow fractionation/adhesion chromatography technique, J. Immunol. Methods, 1989, 117(2), 289–293 CrossRef CAS PubMed.
-
D. S. Kompala and P. Todd, Industrial ACSDo, Chemistry E, Technology ACSDoB, Meeting ACS. Cell Separation Science and Technology, American Chemical Society, 1991 Search PubMed.
- T. E. Lockwood, R. Gonzalez de Vega and D. Clases, An interactive Python-based data processing platform for single particle and single cell ICP-MS, J. Anal. At. Spectrom., 2021, 36(11), 2536–2544 RSC.
- H. E. Pace, N. J. Rogers, C. Jarolimek, V. A. Coleman, C. P. Higgins and J. F. Ranville, Determining Transport Efficiency for the Purpose of Counting and Sizing Nanoparticles via Single Particle Inductively Coupled Plasma Mass Spectrometry, Anal. Chem., 2011, 83(24), 9361–9369 CrossRef CAS PubMed.
- M. Tanner, Shorter signals for improved signal to noise ratio, the influence of Poisson distribution, J. Anal. At. Spectrom., 2010, 25(3), 405–407 RSC.
- M. Reig, S. Casas, C. Aladjem, C. Valderrama, O. Gibert and F. Valero,
et al., Concentration of NaCl from seawater reverse osmosis brines for the chlor-alkali industry by electrodialysis, Desalination, 2014, 342, 107–117 CrossRef CAS.
- Y. K. Chae, S. H. Kim, J. E. Ellinger and J. L. Markley, Dosage Effects of Salt and pH Stresses on Saccharomyces cerevisiae as Monitored via Metabolites by Using Two Dimensional NMR Spectroscopy, Bull. Korean Chem. Soc., 2013, 34(12), 3602–3608 CrossRef CAS PubMed.
|
This journal is © The Royal Society of Chemistry 2022 |