DOI:
10.1039/D2FO00552B
(Paper)
Food Funct., 2022,
13, 6306-6316
Urolithins: potential biomarkers of gut dysbiosis and disease stage in Parkinson's patients†
Received
23rd February 2022
, Accepted 23rd April 2022
First published on 28th April 2022
Abstract
Gut microbiota alteration (gut dysbiosis) occurs during the onset and progression of Parkinson's disease. Gut dysbiosis biomarkers could be relevant to prodromal disease. Urolithins, anti-inflammatory metabolites produced from some dietary polyphenols by specific gut microbial ecologies (urolithin metabotypes), have been proposed as biomarkers of gut microbiota composition and functionality. However, this has not been explored in Parkinson's disease patients. The current study aimed to assess associations between urolithin metabotypes, gut dysbiosis and disease severity in Parkinson's disease patients. Participants (52 patients and 117 healthy controls) provided stool samples for microbiota sequencing and urine samples for urolithin profiling before and after consuming 30 g of walnuts for three days. Data on demographics, medication, disease duration and Hoehn and Yahr disease stage were collected. We observed a significant gradual increase of urolithin non-producers (metabotype-0) as the disease severity increased. The gut microbiome of metabotype-0 patients and patients with the greatest severity was characterized by a more altered bacterial composition, i.e., increased pro-inflammatory Enterobacteriaceae and reduced protective bacteria against autoimmune and inflammatory processes, including butyrate and urolithin-producing bacteria (Lachnospiraceae members and Gordonibacter). Besides, their microbiome was characterized by predictive functions of lipopolysaccharide biosynthesis and metabolism of glutathione, cysteine and methionine that could indirectly reflect the gut pro-inflammatory status. Urolithin detection in urine is a feasible, non-invasive and fast approach that can reflect gut microbiome dysbiosis and intestinal inflammation in Parkinson's disease patients. Our current study could provide novel strategies for improving diagnostics, and for preventing and treating disease progression in microbiota-based interventions.
Introduction
The diagnosis of Parkinson's Disease (PD) is based on clinical observations.1 As motor impairment symptoms occur only late in the course of the disease, the interventions are generally targeted at alleviating symptoms rather than preventing disease progression. Consequently, there is a need to know better the pathogenesis of PD in its early stages.2 Clinical and experimental evidence has shown gut microbiota alteration (gut dysbiosis) during the onset and progression of PD. Therefore, gut dysbiosis biomarkers could be relevant to identify prodromal PD patients.2–9 Gut dysbiosis has been associated with an alteration of microbial metabolites and neurotoxins in PD, highlighting the need to investigate microbial metabolomes as potential biomarkers that predict PD and possibly reflect its progression.10–14
Gut microbiome biomarkers are being postulated as potential functional markers in health and disease states. The ability or not to transform some polyphenols in the diet (for example ellagitannins from walnuts, pomegranate, strawberries, etc. into urolithins) has been proposed as a biomarker of the state of the intestinal microbiota.15–19 The gut microbial ecologies associated with this specific polyphenol metabolism are known as urolithin metabotypes (UMs). In the Western and Eastern populations, three UMs have been identified depending on the final urolithins produced, i.e., metabotype A (UM-A, only urolithin A producers), metabotype B (UM-B, urolithin A, isourolithin A and urolithin B producers), and metabotype 0 (UM-0, urolithin non-producers).20,21 The percentage of individuals with UM-0 (10%) remains approximately constant in the population from 5 to 90 years,22 although this proportion can be affected in transient or permanent gut dysbiosis states.23,24
Based on the gut dysbiosis involvement in the onset and progression of PD and the potential to stratify individuals in UMs as a tool to predict functionality and health status of gut microbiota, we speculated that UM distribution (%) could be altered in PD patients. We hypothesized that urolithin metabotyping could be used as a biomarker of the gut microbiota state in PD, which could correlate with disease severity and prognosis. Therefore, we aim here to assess associations between UMs, gut dysbiosis and severity stage in Parkinson's disease patients.
Materials and methods
Participant recruitment and data collection
The study was approved by the ethics committee of the University of Murcia (Spain), and PD patients (n = 52) gave informed consent. The study was conducted following the guidelines established in the Declaration of Helsinki (1975) and its amendments. The Hoehn and Yahr (H&Y) stage was assessed.25 Disease duration was the difference between age at study and age at onset. All other metadata were collected using questionnaires that the participants completed. For comparative purposes, samples from healthy controls (n = 117) obtained in a previous trial15 were also used (Table 1).
Table 1 Demographic and clinical characteristics of participants
|
PD patients (n = 52) |
Healthy controls (n = 117) |
P
|
n/a not applicable; NS, not significant; COMT, catechol-ortho-methyl transferase; MAO, monoamine oxidase; UMs, urolithin metabotypes; UM-A, metabotype A (only urolithin A producers); UM-B, metabotype B (urolithin A, isourolithin A and urolithin B producers); UM-0, urolithin non-producers. |
Demographics
|
Sex (females, %) |
40.4% |
41.2% |
NS |
Mean age (years) and range |
68 ± 8 (44–88) |
60 ± 6 (44–72) |
≤0.001 |
Body mass index (kg m−2) |
26.7 ± 3.4 |
27.2 ± 4.7 |
NS |
Disease duration
|
Mean age (years) and range |
9.7 ± 6.0 (2–24) |
|
n/a |
<10 years (%) |
52% |
0% |
n/a |
>10 years (%) |
48% |
0% |
n/a |
Hoehn and Yahr disease stage (%)
|
H&Y score (mean ± SD) |
2.9 ± 0.9 |
|
|
Stage I (%) |
6% |
0% |
n/a |
Stage II (%) |
33% |
0% |
n/a |
Stage III (%) |
22% |
0% |
n/a |
Stage IV (%) |
39% |
0% |
n/a |
Medication (%)
|
Carbidopa/levodopa |
91% |
0% |
n/a |
COMT inhibitor |
51% |
0% |
n/a |
MAO inhibitor |
51% |
0% |
n/a |
Dopamine agonist |
40% |
0% |
n/a |
Amantadine |
9% |
0% |
n/a |
UMs (%)
|
|
|
0.015 |
UM-A |
45% |
57% |
|
UM-B |
27.5% |
34% |
|
UM-0 |
27.5% |
9% |
|
Sampling procedure and determinations
Stool samples were collected for the gut microbiota analysis at baseline, while urine samples were collected for the urolithin analysis after consuming 30 g of walnuts for three days, a short polyphenol-rich intervention in which participants can produce urolithins.20 Urolithins were determined in urine samples by ultra performance liquid chromatography–electro spray ionization–quadrupole time of flight–mass spectrometry (UPLC-ESI-qToF-MS) as previously described.22,26
Faecal microbiota analysis of PD patients and healthy controls was performed by 16S ribosomal RNA gene sequencing in a MiSeq-Illumina® platform as described previously.15 Relative abundances were calculated. Linear discriminant (LDA) and partial least squares discriminant (PLS-DA) analyses were used to identify taxonomic and functional differences in the gut microbiota of PD patients depending on the urolithin production capacity and PD severity. Patients with H&Y stages ranging from 1 to 2.5 were included in the mild PD (MPD) group, while patients with H&Y stages ranging from 3 to 5 were included in the severe PD (SPD) group as previously described.27 The LDA effect size (LEfSe) algorithm was assayed with the online interface Galaxy (https://huttenhower.sph.harvard.edu/galaxy/root). Only variables with log LDA scores of >2.0 were considered. PLS-DA was performed using the R package mixOmics (https://www.mixOmics.org). The variable importance for the projection (VIP) scores was used to rank the ability of different taxa to discriminate between different groups. Volunteers were grouped depending on health status, urolithin production and PD stage using principal component analysis (PCA) and hierarchical clustering analysis (HCA) via R commander using ‘ggbiplot’ graphics.15 The Wilcoxon signed-rank test was performed to detect significant differences. When more than two groups were compared, analyses of variance (ANOVA) and Bonferroni t-test or the Kruskal–Wallis test followed by Dunn's test were used for normally and non-normally distributed data, respectively. We applied a multinomial logit model to evaluate changes in UM distribution in volunteers depending on the health status and PD stage.
Potential microbial functions were identified by PICRUst v0.9.0 (https://picrust.github.io/picrust/). Following PICRUst analysis, the potential microbial functions associated with the health status, urolithin production and PD stage were identified by LEfSe. Additionally, microbial and potential microbial functions were associated by Spearman's correlation using the SPSS software, version 23.0 (SPSS Inc., Chicago, IL, USA).
Results
Participant characteristics
Participant characteristics are summarized in Table 1. Sex (%) and body mass index were similar between the PD and healthy control (HC) groups. In contrast, the mean age was higher in the group with PD (68 years) than in the HC group (60 years) (P ≤ 0.001); therefore, analyses comparing the PD and HC groups were adjusted for age. PD patients had an average disease duration of 9.75 ± 6.0 years with a distribution of 52% (<10 years) and 48% (>10 years). The mean H&Y stage was 2.94 ± 0.9. All PD patients were using antiparkinsonian medication, whereas HC subjects were not taking any medication at the time of sample collection.
Alterations of gut microbiota composition and UM distribution in PD patients
UM distribution showed differences between PD and HC volunteers (P = 0.015) (Table 1). UM-A individuals (producers of only urolithin A) were prevalent in both groups (PD and HC) but reduced in PD (45%) compared to HC (57%). UM-B individuals (urolithins A and B and isourolithin A producers) were also slightly reduced in PD (27%) versus HC (34%). In contrast to urolithin producers (either UM-A or UM-B), urolithin non-producers (UM-0) were abnormally increased in PD (27%) with respect to HC (9%). Thus, in the PD group, the abundance of urolithin non-producers (UM-0) was significantly higher than in the HC group (P = 0.004) (Table 1).
Faecal microbiota differences between PD and HC were also analysed to ensure concordance with previous studies. No difference in alpha diversity (Shannon and Chao1 indexes) was observed between PD and HC groups (data not shown). However, LDA (Fig. 1A) and the cladogram (Fig. 1B) generated from LEfSe analysis confirmed the differences between PD and HC groups in the abundance of several bacterial groups. In the LEfSe analysis, health status (HC vs. PD) was used as class and age as a subclass to find biomarkers for health status consistent also at the age level. The abundance of Firmicutes was higher in the HC group at the phylum level, whereas Proteobacteria and Cyanobacteria were higher in PD (Fig. 1A and B). PD patients had a higher relative abundance of Enterobacteriaceae, Desulfovibrionaceae, Lactobacillaceae, Enterococcaceae, Actinomycetaceae, and their corresponding genera Escherichia-Shigella, Klebsiella, Desulfovibrio, Bilophila, Lactobacillus, Enterococcus, and Actinomyces than the HC group. Conversely, PD patients had a lower abundance of Clostridia, their families Ruminococcaceae (now Oscillospiraceae) and Lachnospiraceae, and their genera Faecalibacterium, Anaerostipes, Clostridium XVIII cluster and Lachnospiraceae incertae sedis than the HC group (Fig. 1A and B). PCA plot of the gut microbiome illustrated differences between the PD and HC groups (P ≤ 0.001), but only in the second principal component (PC2), where the main drivers were Proteobacteria, bacilli and Lactobacillales versus the Firmicutes phylum, Clostridia class, Clostridiales order (now Eubacteriales), as well as their families Lachnospiraceae and Ruminococcaceae (Fig. 1C).
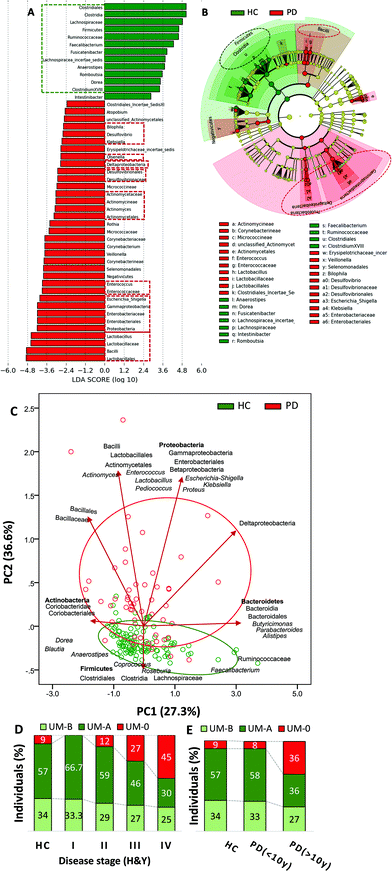 |
| Fig. 1 Differences in the faecal microbiome between healthy control (HC) and Parkinson's disease (PD) volunteers. (A) LDA score (log 10) and (B) cladogram generated by LEfSe analysis show potential microbial biomarkers of health status vs. PD. The green and red dashed lines highlight the biomarkers discussed in the text. (C) Principal component analysis (PCA) with clustering analysis shows differences in the faecal microbiome between HC and PD. (D) Distribution of urolithin metabotypes UM-A (metabotype A: producers of only urolithin A), UM-B (metabotype B: urolithin A, isourolithin A and urolithin B producers), and UM-0 (urolithins non-producers) in HC volunteers and PD patients grouped by disease severity based on the Hoehn & Yahr stage. (E) Distribution of urolithin metabotypes in HC volunteers and PD patients grouped by disease duration (< or >10 years). | |
Associations of disease severity with gut microbiome composition and UM distribution in PD patients
LDA (Fig. 2A) and the cladogram (Fig. 2B) generated from LEfSe analysis showed the differences between MPD (H&Y stage ranging from 1 to 2.5) and SPD (H&Y stage ranging from 3 to 5) groups in several bacterial groups. Most microbial biomarkers for health status (HC vs. PD) mentioned in the previous section (Fig. 1A and B) were higher in MPD than in SPD (Fig. 2A and B). Besides, Gordonibacter was also higher in MPD than in SPD. Conversely, SPD patients had a higher relative abundance of biomarkers mentioned before for disease status (PD vs. HC) (Fig. 1A and B) than MPD (Fig. 2A and B). The PCA plot of the gut microbiome illustrated differences between the MPD and SPD groups (P ≤ 0.001), but only in PC2 as shown between HC and PD (Fig. 2C). In summary, PD patients were grouped by PD severity (MPD and SPD), the group with the greatest severity of PD (SPD) being more distant from the HC group. Gradual differences in PC2 were observed when PD patients were clustered by health status (HC, MPD and SPD) (P ≤ 0.001) (Fig. 2D) but also by disease duration (more or less than 10 years) (P ≤ 0.001) (Fig. 2E). Spearman correlation analysis confirmed positive correlations between PC2 and both H&Y stages (r = 0.572; P ≤ 0.001) and PD disease duration in years (r = 0.553; P ≤ 0.001). Interestingly, differences depending on PD medication were also observed in the case of COMT inhibitors in the second principal component (PC2) (P ≤ 0.013).
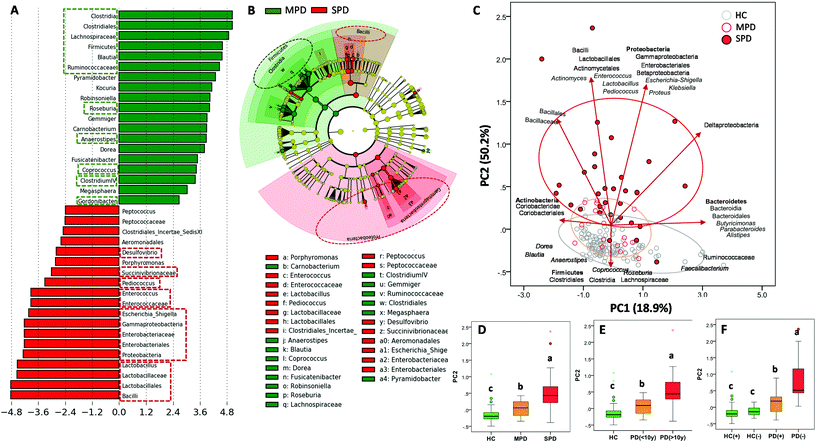 |
| Fig. 2 Differences in the faecal microbiome between mild Parkinson's disease (MPD: 1–2.5) and severe Parkinson's disease (SPD: 3–5) patients based on the Hoehn and Yahr stage. (A) LDA score (log 10) and (B) cladogram generated by LEfSe analysis shows potential microbial biomarkers of PD severity. (C) Principal component analysis (PCA) with clustering analysis shows differences in the faecal microbiome between HC, Mild PD (MPD) and severe PD (SPD). (D) Box plots of second axes of the PCA (PC2) grouped by PD status: MPD (1–2.5) vs. SPD (3–5) based on the Hoehn and Yahr stage. (E) Box plots of PC2 grouped by PD duration (< or >10 years). (F) Box plots of second axes of the PCA (PC2) grouped by production (+, i.e., urolithin producers either UM-A or UM-B) or not (−, i.e., UM-0) of urolithins in healthy control (HC(+) vs. HC(−)) and Parkinson's disease (PD(+) vs. PD(−)). Groups with different letters were significantly different (P < 0.05). The green and red dashed lines highlight the biomarkers discussed in the text. | |
Concerning UM distribution, we observed a gradual and significant increase of UM-0 (percentage of urolithin non-producers) as the disease severity increased (P = 0.041) (Fig. 1D). Thus, UM-0 abundance in the H&Y stage I was 10%, similar to that in the HC group, but increased up to 45% in the H&Y stage IV, whereas UM-A abundance was especially reduced (Fig. 1D). Also, UM-0 abundance increased up to 36% when PD duration was higher than 10 years fundamentally at the expense of the reduction in UM-A (Fig. 1E). In contrast, UM-0 was only slightly superior in COMT inhibitor consumers (26.1%) than in non-consumers (22.7%). The PCA of the gut microbiome illustrated differences between urolithin-producing (PD(+)) and non-producing (PD(−)) patients, but only in PC2 (P = 0.003) (Fig. 2F) as shown between HC, MPD and SPD (Fig. 2D). PD(−) differed from PD(+), either UM-A (P = 0.007) or UM-B (P = 0.015), in PC2 without differences between them. When HC volunteers were clustered into producers (HC+) and non-producers (HC−) of urolithins, differences in PC2 were not significant (Fig. 2F). Indeed, PC2 did not differ among UMs in the HC group.
In the PD group, LDA generated from LEfSe analysis showed the differences between PD patient producers (PD+) and non-producers (PD−) of urolithins (Fig. 3A). Interestingly, most microbial biomarkers for health-disease status (HC vs. PD) (Fig. 1A and B) and PD disease severity (MPD vs. SPD) (Fig. 2A and B) also differed between PD(+) and PD(−) (Fig. 3A–C). The PLS-DA model and the VIP score confirmed it (Fig. S1A and S1B†). PD(+) had higher levels of urolithin-producing bacteria (Gordonibacter and Ellagibacter) but also higher levels of other butyrate-producing bacteria associated with less PD severity (MPD), such as Blautia, Roseburia, and Anaerostipes, all from the Lachnospiraceae family (Fig. 3A and B). The heatmap of UMs, firstly differed in two groups, non-producers (PD(–)) and producers (PD(+)), and secondly subdivided producers (PD+), into two more similar groups (urolithin producers from UM-A and UM-B) (Fig. 3D).
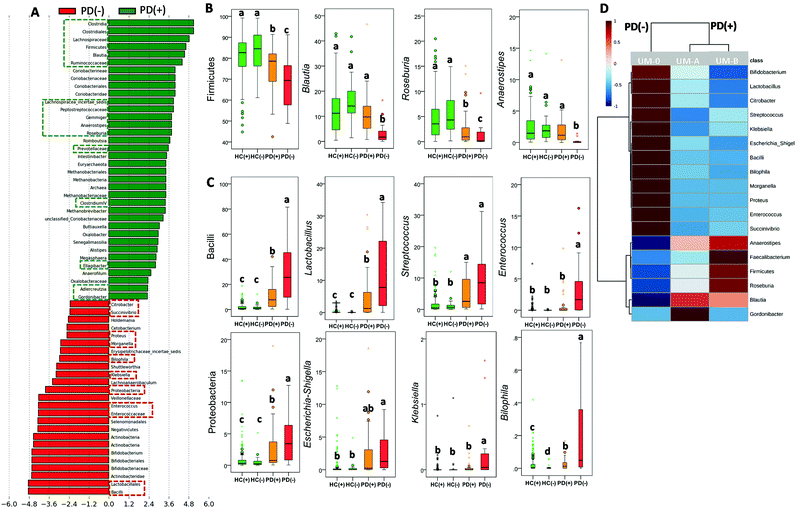 |
| Fig. 3 Differences in the faecal microbiome between urolithin producers (+) and urolithin non-producers (−) in Parkinson's disease (PD). (A) LDA score (log 10) generated by LEfSe analysis shows potential microbial biomarkers of urolithin production (PD(+): producers from UM-A & UM-B) vs. non-production (PD(−): UM-0) in PD patients. The green and red dashed lines highlight the biomarkers discussed in the text. Relative abundance (%) of main bacterial biomarkers of (B) health status, and (C) PD and (D) heat map shows the relative abundance differences. Groups with different letters were significantly different (P < 0.05). | |
The abundance of other taxa, mainly Coriobacteriia (class level), Coriobacteriaceae, Prevotellaceae and Methanobacteriaceae (family level) and some of their genera (i.e., Adlercreutzia, Senegalimassilia and Methanobrevibacter) were also potential markers of PD(+) (Fig. 3A). According to these findings, microbial richness (Chao1 index) was significantly higher (P = 0.034) in PD(+) (1911 ± 613) than in PD(−) (1680 ± 594). Similarly, in the HC group, a significant difference (P = 0.011) in the Chao1 index between urolithin producers (HC(+), 1900 ± 585) and non-producers (HC(−), 1514 ± 634) was also observed. However, differences between HC(+) and HC(−) in the microbial composition affected a minimal number of bacteria (Fig. S2†).
Functional alterations of gut microbiome in PD and correlations with disease severity and UMs
We used PICRUSt to evaluate potential functional alterations in the microbiome of PD patients. Pathways including LPS biosynthesis, metabolism of some amino acids (tyrosine, cysteine and methionine, and a few other amino acids including glutathione and selenocompounds) and xenobiotic biodegradation and metabolism (degradation of naphthalene, benzoate, aminobenzoate and toluene) were more abundant in SPD and PD(+) than in MPD (Fig. S1C†) and PD(−) (Fig. 4A), respectively. Conversely, biosynthetic pathways of amino acids such as phenylalanine, tyrosine and tryptophan biosynthesis, as well as arginine and proline metabolism, had more genes in MPD and PD(+), relative to SPD and PD(−) patients, respectively (Fig. 4A and Fig. S1C†). Fig. 4B shows significant correlations between some predicted microbial functions and microbial groups, whereas correlations among microbial groups are shown in Fig. 4C. Interestingly, phenylalanine, tyrosine and tryptophan biosynthesis and arginine and proline metabolism, which were increased in PD(+) and MPD, were positively associated with several microbial groups increased in PD(+) and MPD, such as Blautia, Roseburia, Anaerostipes, Faecalibacterium and Gordonibacter. Several bacterial groups, augmented in PD(−) and SPD (Proteobacteria and bacilli members), positively correlated with many of the microbial functions enriched in PD(−) and SPD (Fig. 4B).
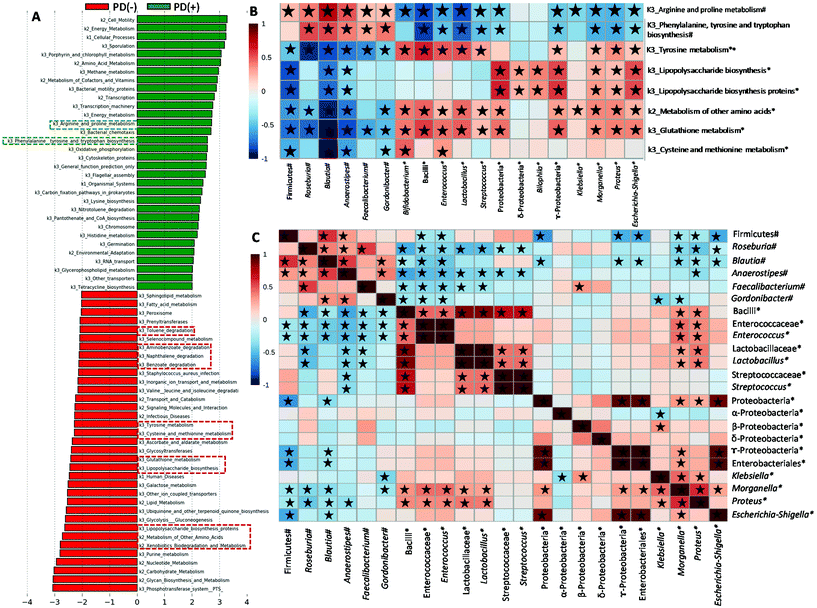 |
| Fig. 4 Functional predictions for the faecal microbiome of PD patients. (A) LDA score (log 10) generated by LEfSe analysis shows potential microbial biomarkers of urolithin production (PD(+): producers from UM-A & UM-B) vs. non-production (PD(−): UM-0) in PD patients. (B) Spearman's correlation heatmap of microbial abundances with microbial metabolic functions in PD patients. (C) Spearman's correlation heatmap of microbial abundances. #Higher abundance in urolithin producers (PD(+): producers from UM-A & UM-B) and mild PD (MPD: 1–2.5); *higher abundance in urolithin non-producers (PD(−): UM-0) and severe PD (SPD: 3–5) based on the Hoehn and Yahr stage. ★Spearman's correlation values with P < 0.05. | |
Discussion
We investigated the possible associations between clinical phenotypes of PD patients, their gut microbiome and their ability to produce urolithins. Our results confirmed associations between PD and gut microbiome, including reduced levels of some bacterial genera, such as Faecalibacterium and Anaerostipes, associated with anti-inflammatory properties and preservation of the gut mucosa barrier through the synthesis of SCFA or mucin. This agrees with several studies reporting that a decrease in butyrate-producing bacteria characterizes intestinal dysbiosis in PD patients.2,4–8,27,28 We also confirmed elevated levels of bacterial genera associated with pro-inflammatory properties due to LPS bacterial endotoxin such as Escherichia-Shigella and Klebsiella (family Enterobacteriaceae).2,4–8,27 The family Desulfovibrionaceae, which can obtain energy from the sulphate released from intestinal mucins by cross-feeding of bacteria, was also significantly increased in the PD group. Overall, these findings would suggest an aberrant breakdown of the intestinal mucous layer in PD as previously described.3 Interestingly, Olsenella, a p-cresol producer, was also augmented in PD versus HC. In this regard, Cirstea et al. showed that this microbial metabolite produced from tyrosine degradation was elevated in the serum of PD patients, and it correlated with Olsenella, firmer stool consistency and constipation but not with PD severity.3
When the gut microbiota composition was evaluated depending on the H&Y stage of PD patients, we observed that most microbial biomarkers for health-disease status (HC vs. PD) also discriminated between SPD (where postural instability exists) and MPD. This could suggest that these microbial biomarkers are primarily related to the composition of the dysbiotic gut microbiome rather than being alterations intrinsically associated with PD per se. The gut microbiome PCA plot illustrated that the PD data showed greater dispersion than HC. Therefore, the gut microbiota may reflect different pathophysiological mechanisms behind the clinical heterogeneity of PD. A higher distance of SPD than MPD from the centroid of the HC group suggests a more altered bacterial composition (deeper dysbiotic state) in SPD versus MPD. This aligns with previous studies supporting the idea that gut dysbiosis is associated with the development and progression of PD.28,29 In addition to microbial biomarkers of health/disease status (HC vs. PD), a reduction of other bacteria associated with preserving the intestinal mucosa barrier such as Blautia, Roseburia and Coprococcus was observed in SPD versus MPD. Our findings agree with a recent report in which the reduction in several butyrate-producing bacteria such as Blautia, Coprococcus, and Lachnospira was associated with dysbiosis, non-tremor-dominant (non-TD) phenotypes and a more severe α-synucleinopathy.12 In the same line, another study showed that Enterobacteriaceae was more abundant in PD with postural instability and gait difficulty (PIGD phenotype; a non-TD form) than in tremor-dominant (TD) patients.30 In our current study, Olsenella did not differ between the MPD and SPD groups, confirming the lack of correlation between this bacterium and motor/neurological characteristics as previously reported.3 Interestingly, Gordonibacter abundance did not distinguish between HC and PD but discriminated SPD from MPD. This bacterium could be potentially associated with anti-inflammatory properties and gut mucosa barrier preservation because of urolithin production.31,32 Indeed, the anti-inflammatory and neuroprotective effects of urolithins have been shown in different in vitro and animal studies.33–36 We observed a gradual increase in urolithin non-producers as the PD severity augmented. The PCA diagram of the gut microbiome illustrated the more distant group (PD(−)) characterized by a more altered bacterial composition and less diversity. The main discriminant taxa between MPD and SPD (butyrate, LPS and urolithin producers) also discriminated between PD(+) and PD(−). The aberrant abundance of these 3 bacterial groups was also observed in other diseases such as active Crohn's disease, presenting intestinal inflammation.37 Therefore, that alteration of butyrate, LPS and urolithin producing bacteria may have a specificity of inflammatory status applicable to different diseases, including PD. This agrees with a previous study in PD, which reported a reduction in Blautia, Coprococcus, Roseburia and Faecalibacterium, which potentially shifts the microbial balance within the colon to a more inflammatory phenotype.2
An imbalance between regulation and inflammation could be underlying the pathogeny of PD, as suggested before.38 Thus, a decrease in levels of anti-inflammatory regulatory T (Treg) cells could play an important role in the progression of PD where reduced levels of Tregs are unable to control the pro-inflammatory response associated with PD progression.39 In fact, both the levels of cytokines and immune cells have been related to symptom worsening, as reflected in clinical scales like the H&Y scale and the Unified Parkinson's Disease Rating Scale (UPDRS). Consistent with this, in SPD and PD(−), we observed the deepest reduction in Lachnospiraceae members and Gordonibacter, which are recognized as Treg-inducing bacteria40 and inflammatory T helper 17 (TH17) cell-inhibitory bacteria,41 respectively. SCFA and secondary bile acids produced by these bacteria are involved in modulating the differentiation and function of T cells, including inflammatory T helper 17 (TH17) cells and Treg cells.40,41 Furthermore, the deeper reduction of these bacteria in SPD and PD(−) might lead to a decrease in the synthesis of butyrate and mucin, which can result in increased gut permeability, as reviewed before.38 This may, in turn, promote more translocation, from the intestine to the systemic circulation, of LPS-producing Enterobacteriaceae such as Escherichia-Shigella, also overgrown in PD(−) and SPD groups, probably favoured by greater intraluminal oxygen because of a disruption of the epithelium. LPS circulation activates innate immunity characterized by pro-inflammatory cytokine production, which can cross the brain–blood barrier resulting in neuroinflammation and aggravating PD.38 Together, this suggests that the gut microbiota of PD(−) and SPD reflect the pro-inflammatory status of these patients.
We confirmed correlations of some members of these 3 bacterial groups (butyrate, LPS and urolithin producers) with predictive functions of the gut microbiome in PD. Pathways of LPS biosynthesis were augmented in PD but especially in SPD and PD(−) and correlated to Enterobacteriaceae members. It has been proposed that microbial components (LPS, proteins etc.) or metabolites may influence neurodegeneration by promoting amyloid formation by human proteins or enhancing inflammatory responses to endogenous neuronal amyloids.42 We also found that predictive functional pathways of some amino acids' metabolism were augmented in PD and especially in SPD and PD(−). A recent study also showed that the microbiota of PD versus HC was characterized by increased proteolytic fermentation and the production of harmful metabolites from amino acids such as tyrosine.3 The pathway of tyrosine metabolism, mainly increased in SPD and PD(−), correlated with Enterococcus abundance in PD patients. According to this, a recent study has identified a conserved tyrosine decarboxylase (TyrDC) in Enterococcus faecalis able to metabolize levodopa (L-dopa; the primary medication used to treat PD) to dopamine, which cannot cross the blood–brain barrier and causes unwanted side effects.43 Glutathione metabolism, which was also mainly increased in PD(−) and SPD and correlated to Proteobacteria members (Proteus, Morganella and Escherichia-Shigella) and Bacilli members (Streptococcus, Lactobacillus and Enterococcus), was one of the five most predictive pathways in paediatric Crohn's disease with more severe dysbiosis.37 Interestingly, glutathione, synthesized by Proteobacteria and some Enterococci and Streptococci, protects these bacteria from oxidative stress and allows them to keep their homeostasis.44 Glutathione, which is a cysteine-containing tripeptide, can be used as a sulfur source by different bacteria. The metabolism of sulfur-containing amino acids (cysteine and methionine) was also increased in PD(−) patients and SPD and correlated to Bifidobacterium, which increased in PD(−) patients, as well. Consistent with this, bifidobacteria have long been known to be cysteine auxotrophs, although various bifidobacteria strains can also use methionine when it is the only available sulphur source.45 A previous study with a colitis rat model also showed increased cysteine and methionine metabolism.46 In the present study, the increased levels of cysteine and methionine metabolism, as well as glutathione metabolism in PD(−) and SPD, could result in the biosynthesis of advantageous compounds for bacteria against oxidative stress, a feature of the inflammatory environment. Accordingly, the increases in those microbial products from amino acid metabolism could indirectly reflect the gut pro-inflammatory status in PD(−) and SPD patients. In contrast, PD(+) and MPD patients were predicted to have enriched arginine and proline metabolism functions. Polyamines, such as putrescine and spermidine, are gut microbial metabolites derived from arginine. They protect intestinal mucosa cells from oxidative damage maintaining the intestinal barrier and influencing the immune system.47 Therefore, the higher abundance of arginine and proline metabolism in the gut microbiome of MPD and PD(+) could indirectly reflect a healthier status of the gut barrier than that of the SPD and PD(−) patients. Xenobiotic biodegradation and metabolism were also increased in PD but mainly in SPD and PD(−) versus MPD and PD(+). A previous study hypothesized the role of xenobiotics in initiating the dysbiosis of the gut microbiome and whether recent or continued exposure to PD-associated xenobiotics (i.e., herbicide or insect repellent such as naphthalene) may contribute to the progression of neurodegeneration.8 Therefore, the increases in xenobiotic biodegradation and metabolism pathway could indirectly reflect gut dysbiosis associated with xenobiotic exposition of PD patients, especially in PD(−) and SPD.
Indicators of gut microbiota dysbiosis include low microbial diversity, decreased butyrate-producing bacteria and an increase in several genera of LPS-producing Enterobacteriaceae.48 However, identifying each disease-specific dysbiotic microbial signature has proven to be challenging. Furthermore, gut dysbiosis severity in PD and other diseases is difficult to quantify, and this depends on many microbial groups, as indicated above. A recent study has identified metabolites of potential bacterial origin that are altered in PD, such as p-cresol and phenylacetylglutamine, but they are not related to motor/neurological features or inflammation status.3 In the current study, for the first time, we identified that urolithin non-production in PD is associated with more severe PD. Besides, the gut microbiota of these UM-0 patients was enriched in pro-inflammatory Enterobacteriaceae and reduced in protective bacteria, such as Lachnospiraceae members and Gordonibacter, against autoimmune and inflammatory disorders and potentially a more severe α-synucleinopathy in the enteric nervous system. However, more research is needed to confirm this.
Conclusions
Although the causal role of the gut microbiota in PD pathogenesis has been previously reported,49,50 it is unknown whether host signals in PD could promote gut dysbiosis or a specific gut microbiota played a possible role in the onset and progression of PD. Therefore, we cannot establish whether being UM-0 or having a high abundance of pro-inflammatory microbiota is the cause or the consequence of the severity in PD. Nevertheless, the statistically significant associations found in the present study establish a potential connection between urolithin production, gut dysbiosis and PD severity. In this regard, urolithin detection in urine samples is a feasible, fast, and non-invasive approach that may reflect gut microbiome dysbiosis and gut inflammation in PD patients. Our study could pave the way to implement novel strategies for improving the diagnostics, and preventing and treating disease progression in microbiota-targeted interventions, including drugs, antibiotics, probiotics, and faecal microbiota transplants.
Author contributions
Conceptualization and design: M. V. S and M. T. H.; volunteer recruitment: M. T. H.; execution and characterization of volunteers: E. F. V., A. L. G. M., L. C. B. and M. T. H.; methodology and software: M. R. V. and M. V. S.; validation: M. T. H. and M. V. S.; formal analysis: M. R. V. and M. V. S.; writing: M. V. S.; critical review of the manuscript for important intellectual content: J. C. E., M. T. H. and M. V. S. All authors have read and approved the final manuscript.
Conflicts of interest
There are no conflicts to declare.
Acknowledgements
This research has been supported by the projects AGL2015-64124-R and PID2019-103914RB-I00 (Spanish Ministry of Science and Innovation), PIE-201570I005 (Spanish National Research Council) and FS/20880/PI/18 (Fundación Séneca, Spain). The authors are grateful to the Murcia Federation of Parkinson's disease, Victoria López, Marian Martín, Genoveva Órtiz, and Consuelo Sánchez Rodrigo for their help and support during the study.
References
- E. Tolosa, A. Garrido, S. W. Scholz and W. Poewe, Challenges in the diagnosis of Parkinson's disease, Lancet Neurol., 2021, 20, 385–397 CrossRef CAS PubMed.
- A. Keshavarzian, S. J. Green, P. A. Engen, R. M. Voigt, A. Naqib, C. B. Forsyth, E. Mutlu and K. M. Shannon, Colonic bacterial composition in Parkinson's disease, Mov. Disord., 2015, 30, 1351–1360 CrossRef CAS PubMed.
- M. S. Cirstea, A. C. Yu, E. Golz, K. Sundvick, D. Kliger, N. Radisavljevic, L. H. Foulger, M. Mackenzie, T. Huan, B. B. Finlay and S. Appel-Cresswell, Microbiota Composition and Metabolism Are Associated With Gut Function in Parkinson's Disease, Mov. Disord., 2020, 35, 1208–1217 CrossRef CAS PubMed.
- M. F. Sun and Y. Q. Shen, Dysbiosis of gut microbiota and microbial metabolites in Parkinson's Disease, Ageing Res. Rev., 2018, 45, 53–61 CrossRef CAS PubMed.
- S. P. Van Kessel and S. El Aidy, Bacterial Metabolites Mirror Altered Gut Microbiota Composition in Patients with Parkinson's Disease, J. Parkinson's Dis., 2019, 9, S359–S370 CAS.
- H.-L. Chiang and C.-H. Lin, Altered Gut Microbiome and Intestinal Pathology in Parkinson's Disease, J. Mov. Disord., 2019, 12, 67–83 CrossRef.
- S. Gerhardt and M. H. Mohajeri, Changes of colonic bacterial composition in parkinson's disease and other neurodegenerative diseases, Nutrients, 2018, 10, 708 CrossRef PubMed.
- E. M. Hill-Burns, J. W. Debelius, J. T. Morton, W. T. Wissemann, M. R. Lewis, Z. D. Wallen, S. D. Peddada, S. A. Factor, E. Molho, C. P. Zabetian, R. Knight and H. Payami, Parkinson's disease and Parkinson's disease medications have distinct signatures of the gut microbiome, Mov. Disord., 2017, 32, 739–749 CrossRef CAS PubMed.
- S. M. Aquilonius and K. Fuxe, Parkinsonism and Related Disorders: Introduction, Parkinsonism Relat. Disord., 2004, 10, 257–258 CrossRef PubMed.
-
V. L. Peipei Li, B. A. Killinger, I. Beddows, E. Ensink, A. Yilmaz, N. Lubben, J. Lamp, M. Schilthuis, I. E. Vega, M. Britschgi, J. Andrew Pospisilik, P. Brundin, L. Brundin and S. Graham, Gut microbiome dysbiosis is associated with elevated toxic bile acids in Parkinson's disease, bioRxiv, 2020, 09.26.2798, 1–63.
- Y. Hasuike, T. Endo, M. Koroyasu, M. Matsui and C. Mori, Bile acid abnormality induced by intestinal dysbiosis might explain lipid metabolism in Parkinson's disease, Med. Hypotheses, 2020, 134, 109436 CrossRef CAS PubMed.
- S. Vascellari, M. Melis, V. Palmas, S. Pisanu, A. Serra, D. Perra, M. L. Santoru, V. Oppo, R. Cusano, P. Uva, L. Atzori, M. Morelli, G. Cossu and A. Manzin, Clinical phenotypes of Parkinson's disease associate with distinct gut microbiota and metabolome enterotypes, Biomolecules, 2021, 11, 1–16 CrossRef PubMed.
- A. E. Hill, R. Wade-Martins and P. W. J. Burnet, What Is Our Understanding of the Influence of Gut Microbiota on the Pathophysiology of Parkinson's Disease?, Front. Neurosci., 2021, 15, 1–9 Search PubMed.
- V. Metta, V. Leta, K. R. Mrudula, L. K. Prashanth, V. Goyal, R. Borgohain, G. Chung-Faye and K. R. Chaudhuri, Gastrointestinal dysfunction in Parkinson's disease: molecular pathology and implications of gut microbiome, probiotics, and fecal microbiota transplantation, J. Neurol., 2022, 269, 1154–1163 CrossRef PubMed.
- M. Romo-Vaquero, A. Cortés-Martín, V. Loria-Kohen, A. Ramírez-de-Molina, I. García-Mantrana, M. C. Collado, J. C. Espín and M. V. Selma, Deciphering the Human Gut Microbiome of Urolithin Metabotypes: Association with Enterotypes and Potential Cardiometabolic Health Implications, Mol. Nutr. Food Res., 2019, 63, 1800958 CrossRef PubMed.
- F. A. Tomas-Barberan, M. V. Selma and J. C. Espín, Polyphenols’ Gut Microbiota Metabolites: Bioactives or Biomarkers?, J. Agric. Food Chem., 2018, 66, 3593–3594 CrossRef CAS PubMed.
- A. Cortés-Martín, G. Colmenarejo, M. V. Selma and J. C. Espín, Genetic Polymorphisms, Mediterranean Diet and Microbiota-Associated Urolithin Metabotypes can Predict Obesity in childhood-Adolescence, Sci. Rep., 2020, 10, 7850 CrossRef PubMed.
- M. V. Selma, A. González-Sarrías, J. Salas-Salvadó, C. Andrés-Lacueva, C. Alasalvar, A. Örem, F. A. Tomás-Barberán and J. C. Espín, The gut microbiota metabolism of pomegranate or walnut ellagitannins yields two urolithin-metabotypes that correlate with cardiometabolic risk biomarkers: Comparison between normoweight, overweight-obesity and metabolic syndrome, Clin. Nutr., 2018, 37, 897–905 CrossRef CAS PubMed.
- A. González-Sarrías, R. García-Villalba, M. Romo-Vaquero, C. Alasalvar, A. Örem, P. Zafrilla, F. A. Tomás-Barberán, M. V. Selma and J. C. Espín, Clustering according to urolithin metabotype explains the interindividual variability in the improvement of cardiovascular risk biomarkers in overweight-obese individuals consuming pomegranate: A randomized clinical trial, Mol. Nutr. Food Res., 2017, 61, 1600830 CrossRef PubMed.
- F. A. Tomás-Barberán, R. García-Villalba, A. González-Sarrías, M. V. Selma and J. C. Espín, Ellagic acid metabolism by human gut microbiota: consistent observation of three urolithin phenotypes in intervention trials, independent of food source, age, and health status, J. Agric. Food Chem., 2014, 62, 6535–6538 CrossRef PubMed.
- W. Xian, S. Yang, Y. Deng, Y. Yang, C. Chen, W. Li and R. Yang, Distribution of Urolithins Metabotypes in Healthy Chinese Youth: Difference in Gut Microbiota and Predicted Metabolic Pathways, J. Agric. Food Chem., 2021, 69, 13055–13065 CrossRef CAS PubMed.
- A. Cortés-Martín, R. García-Villalba, A. González-Sarrías, M. Romo-Vaquero, V. Loria-Kohen, A. Ramírez-De-Molina, F. A. Tomás-Barberán, M. V. Selma and J. C. Espín, The gut microbiota urolithin metabotypes revisited: the human metabolism of ellagic acid is mainly determined by aging, Food Funct., 2018, 9, 4100–4106 RSC.
- A. Cortés-Martín, M. Romo-Vaquero, I. García-Mantrana, A. Rodríguez-Varela, M. C. Collado, J. C. Espín and M. V. Selma, Urolithin Metabotypes can Anticipate the Different Restoration of the Gut Microbiota and Anthropometric Profiles during the First Year Postpartum, Nutrients, 2019, 11, 2079 CrossRef PubMed.
- A. Cortés-Martín, C. E. Iglesias-Aguirre, A. Meoro, M. V. Selma and J. C. Espín, Pharmacological Therapy Determines the Gut Microbiota Modulation by a Pomegranate Extract Nutraceutical in Metabolic Syndrome: A Randomized Clinical Trial, Mol. Nutr. Food Res., 2021, 6, 2001048 CrossRef PubMed.
- C. G. Goetz, W. Poewe, O. Rascol, C. Sampaio, G. T. Stebbins, C. Counsell, N. Giladi, R. G. Holloway, C. G. Moore, G. K. Wenning, M. D. Yahr and L. Seidl, Movement Disorder Society Task Force Report on the Hoehn and Yahr Staging Scale: Status and Recommendations, Mov. Disord., 2004, 19, 1020–1028 CrossRef PubMed.
- R. García-Villalba, J. C. Espín and F. A. Tomás-Barberán, Chromatographic and spectroscopic characterization of urolithins for their determination in biological samples after the intake of foods containing ellagitannins and ellagic acid., J. Chromatogr. A, 2016, 1428, 162–175 CrossRef PubMed.
- W. Li, X. Wu, X. Hu, T. Wang, S. Liang, Y. Duan, F. Jin and B. Qin, Structural changes of gut microbiota in Parkinson's disease and its correlation with clinical features, Sci. China Life Sci., 2017, 60, 1223–1233 CrossRef PubMed.
- T. Minato, T. Maeda, Y. Fujisawa, H. Tsuji, K. Nomoto, K. Ohno and M. Hirayama, Progression of Parkinson's disease is associated with gut dysbiosis: Two-year follow-up study, PLoS One, 2017, 12, 1–14 CrossRef PubMed.
- V. T. E. Aho, P. A. B. Pereira, S. Voutilainen, L. Paulin, E. Pekkonen, P. Auvinen and F. Scheperjans, Gut microbiota in Parkinson's disease: Temporal stability and relations to disease progression, EBioMedicine, 2019, 44, 691–707 CrossRef PubMed.
- F. Scheperjans, V. Aho, P. A. B. Pereira, K. Koskinen, L. Paulin, E. Pekkonen, E. Haapaniemi, S. Kaakkola, J. Eerola-Rautio, M. Pohja, E. Kinnunen, K. Murros and P. Auvinen, Gut microbiota are related to Parkinson's disease and clinical phenotype, Mov. Disord., 2015, 30, 350–358 CrossRef PubMed.
- M. V. Selma, D. Beltrán, R. García-Villalba, J. C. Espín and F. A. Tomás-Barberán, Description of urolithin production capacity from ellagic acid of two human intestinal Gordonibacter species., Food Funct., 2014, 5, 1779–1784 RSC.
- M. V. Selma, F. A. Tomás-Barberán, D. Beltrán, R. García-Villalba and J. C. Espín, Gordonibacter urolithinfaciens sp. nov., a urolithin-producing bacterium isolated from the human gut, Int. J. Syst. Evol. Microbiol., 2014, 64, 2346–2352 CrossRef CAS PubMed.
- Z. Gong, J. Huang, B. Xu, Z. Ou, L. Zhang, X. Lin, X. Ye, X. Kong, D. Long, X. Sun, X. He, L. Xu, Q. Li and A. Xuan, Urolithin A attenuates memory impairment and neuroinflammation in APP/PS1 mice, J. Neuroinflammation, 2019, 16, 1–13 CrossRef PubMed.
- J. Xu, C. Yuan, G. Wang, J. Luo, H. Ma, L. Xu, Y. Mu, Y. Li, N. P. Seeram, X. Huang and L. Li, Urolithins Attenuate LPS-Induced Neuroinflammation in BV2Microglia via MAPK, Akt, and NF-κB Signaling Pathways, J. Agric. Food Chem., 2018, 66, 571–580 CrossRef CAS PubMed.
- M. Kujawska and J. Jodynis-Liebert, Polyphenols in parkinson's disease: A systematic review of in vivo studies, Nutrients, 2018, 10, 642 CrossRef PubMed.
- M. Larrosa, A. González-Sarrías, M. J. Yáñez-Gascón, M. V. Selma, M. Azorín-Ortuño, S. Toti, F. Tomás-Barberán, P. Dolara and J. C. Espín, Anti-inflammatory properties of a pomegranate extract and its metabolite urolithin-A in a colitis rat model and the effect of colon inflammation on phenolic metabolism., J. Nutr. Biochem., 2010, 21, 717–725 CrossRef CAS PubMed.
- J. D. Lewis, E. Z. Chen, R. N. Baldassano, A. R. Otley, A. M. Griffiths, D. Lee, K. Bittinger, A. Bailey, E. S. Friedman, C. Hoffmann, L. Albenberg, R. Sinha, C. Compher, E. Gilroy, L. Nessel, A. Grant, C. Chehoud, H. Li, G. D. Wu and F. D. Bushman, Inflammation, Antibiotics, and Diet as Environmental Stressors of the Gut Microbiome in Pediatric Crohn's Disease, Cell Host Microbe, 2015, 18, 489–500 CrossRef CAS PubMed.
- M. F. Sun and Y. Q. Shen, Dysbiosis of gut microbiota and microbial metabolites in Parkinson's Disease, Ageing Res. Rev., 2018, 45, 53–61 CrossRef CAS PubMed.
- D. D. Álvarez-luquín, A. Arce-sillas, J. Leyva-hernández, E. Sevilla-reyes, M. C. Boll, E. Montes-moratilla, V. Vivas-almazán, C. Pérez-correa, U. Rodríguez-ortiz, R. Espinoza-cárdenas, G. Fragoso, E. Sciutto and L. Adalid-peralta, Regulatory impairment in untreated Parkinson's disease is not restricted to Tregs : other regulatory populations are also involved, J. Neuroinflammation, 2019, 16, 212 CrossRef PubMed.
- S. Narushima, Y. Sugiura, K. Oshima, K. Atarashi, M. Hattori, M. Suematsu and K. Honda, Characterization of the 17 strains of regulatory T cell-inducing human-derived Clostridia, Gut Microbes, 2014, 5, 333–339 CrossRef PubMed.
-
D. Paik, L. Yao, Y. Zhang, S. Bae and G. D. D. Agostino, Human gut bacteria produce TH17-modulating bile acid metabolites, bioRxiv, 2021, 01.08.4259, 1–55.
- R. P. Friedland and M. R. Chapman, The role of microbial amyloid in neurodegeneration, PLoS Pathog., 2017, 13, e1006654 CrossRef PubMed.
- V. M. Rekdal, E. N. Bess, J. E. Bisanz, P. J. Turnbaugh and E. P. Balskus, Discovery and inhibition of an interspecies gut bacterial pathway for Levodopa metabolism, Science, 2019, 364, eaau6323 CrossRef PubMed.
- G. V. Smirnova and O. N. Oktyabrsky, Glutathione in bacteria, Biochemistry, 2005, 70, 1199–1211 CAS.
- M. Wada, S. Fukiya, A. Suzuki, N. Matsumoto, M. Matsuo and A. Yokota, Methionine utilization by bifidobacteria: possible existence of a reverse transsulfuration pathway, Biosci. Microbiota, Food Health, 2021, 40, 80–83 CrossRef CAS PubMed.
- H. Zheng, M. Chen, Y. Li, Y. Wang, L. Wei, Z. Liao, M. Wang, F. Ma, Q. Liao and Z. Xie, Modulation of gut microbiome composition and function in experimental colitis treated with sulfasalazine, Front. Microbiol., 2017, 8, 1–15 Search PubMed.
- J. Singh, R. Metrani, S. R. Shivanagoudra, G. K. Jayaprakasha and B. S. Patil, Review on Bile Acids: E ff ects of the Gut Microbiome, Interactions with Dietary Fiber, and Alterations in the Bioaccessibility of Bioactive Compounds, J. Agric. Food Chem., 2019, 67, 9124–9138 CrossRef CAS PubMed.
- A. K. Degruttola, D. Low, A. Mizoguchi and E. Mizoguchi, Current understanding of dysbiosis in disease in human and animal models, Inflamm. Bowel Dis., 2016, 22, 1137–1150 CrossRef PubMed.
- H. Huang, H. Xu, Q. Luo, J. He, M. Li, H. Chen, W. Tang, Y. Nie and Y. Zhou, Fecal microbiota transplantation to treat Parkinson's disease with constipation: A case report, Medicine, 2019, 98, e16163 CrossRef PubMed.
- X. Y. Kuai, X. H. Yao, L. J. Xu, Y. Q. Zhou, L. P. Zhang, Y. Liu, S. F. Pei and C. L. Zhou, Evaluation of fecal microbiota transplantation in Parkinson's disease patients with constipation, Microb. Cell Fact., 2021, 20, 98 CrossRef CAS PubMed.
|
This journal is © The Royal Society of Chemistry 2022 |
Click here to see how this site uses Cookies. View our privacy policy here.