Investigating the dynamics of methylmercury bioaccumulation in the Beaufort Sea shelf food web: a modeling perspective†
Received
13th March 2022
, Accepted 9th May 2022
First published on 24th June 2022
Abstract
High levels of methylmercury (MeHg) have been reported in Arctic marine biota, posing health risks to wildlife and human beings. Although MeHg concentrations of some Arctic species have been monitored for decades, the key environmental and ecological factors driving temporal trends of MeHg are largely unclear. We develop an ecosystem-based MeHg bioaccumulation model for the Beaufort Sea shelf (BSS) using the Ecotracer module of Ecopath with Ecosim, and apply the model to explore how MeHg toxicokinetics and food web trophodynamics affect bioaccumulation in the BSS food web. We show that a food web model with complex trophodynamics and relatively simple MeHg model parametrization can capture the observed biomagnification pattern of the BSS. While both benthic and pelagic production are important for transferring MeHg to fish and marine mammals, simulations suggest that benthic organisms are primarily responsible for driving the high trophic magnification factor in the BSS. We illustrate ways of combining empirical observations and modelling experiments to generate hypotheses about factors affecting food web bioaccumulation, including the MeHg elimination rate, trophodynamics, and species migration behavior. The results indicate that population dynamics rather than MeHg elimination may determine population-wide concentrations for fish and lower trophic level organisms, and cause large differences in concentrations between species at similar trophic levels. This research presents a new tool and lays the groundwork for future research to assess the pathways of global environmental changes in MeHg bioaccumulation in Arctic ecosystems in the past and the future.
Environmental significance
High levels of toxic methylmercury (MeHg) have been found in Arctic marine biota. Many environmental and ecological drivers can affect MeHg levels and trends in biota, and their relative influences are difficult to disentangle through monitoring data alone. We develop and evaluate an ecosystem-based bioaccumulation model for the Beaufort Sea shelf, and apply it to explore how toxicokinetics and food web trophodynamics affect MeHg bioaccumulation. The model is able to capture the observed biomagnification pattern of the BSS, and illustrates the key roles of population dynamics in determining concentrations in biota and benthic organisms in elevating the biomagnification efficiency in the BSS. Future research can apply this model to assess the impact of different global environmental changes on bioaccumulation in Arctic ecosystems.
|
1. Introduction
Human activities have greatly perturbed the natural biogeochemical cycle of mercury (Hg).1,2 Monomethylmercury (MeHg), an organic form of Hg and a highly potent neurotoxicant, can biomagnify in aquatic food webs, resulting in concentrations that are at least a million times higher in predatory fish and mammals than in seawater.3 Mercury enters the Arctic Ocean through a number of different pathways, including river discharge, atmospheric deposition, snow and ice melt, and coastal erosion (ranked from the most important to the least important, though there remains substantial uncertainty).4–6 Although remote and far away from major anthropogenic sources, the Arctic has been impacted by global anthropogenic emissions of Hg due to long-range transport and global distillation.7,8 Previous studies estimated that 70 to 95% of the present-day Hg in Arctic marine mammals comes from anthropogenic emissions.9,10 Some Arctic apex predators have the highest MeHg levels in the world, with measured concentrations exceeding the threshold for neurocognitive impairment and liver diseases.7 Many of these animals, such as beluga whales and ringed seals, are also traditional foods that are nutritionally, culturally, spiritually, and economically significant to Arctic Indigenous populations.11
The seawater MeHg concentration is one of the key components responsible for the spatial and temporal variability of MeHg in marine biota. The fivefold spatial difference observed in the MeHg level of skipjack tuna across the Pacific Ocean can be largely explained by the peak the MeHg concentration in the water column.12 Prior studies indicated that the decline of seawater MeHg in the North Atlantic Ocean between 1990 and 2012, due to reduced anthropogenic Hg emissions and releases, led to a decrease in MeHg levels of Atlantic bluefin tuna from the 1980s to the 2010s.13,14 In the Arctic, in situ methylation of inorganic Hg is considered to be the dominant source of MeHg.15 High MeHg concentrations are observed in Arctic marine waters, driven by active methylation and reduced demethylation due to lower solar radiation and colder temperatures.16 Factors controlling the seawater MeHg concentration, such as availability of inorganic Hg, microbial activity, solar radiation, and temperature,5,15,16 are all highly sensitive to climate change and/or human activities. Hence, the rapid warming in the Arctic and ongoing global environmental policies, including those that address climate change and anthropogenic pollution, will likely have a large impact on MeHg concentrations in seawater.
Recent work suggests that the most important pathways for climate impacts may not be through contaminant loading or biogeochemistry, but through food web dynamics. Schartup et al. (2019) (ref. 13) suggested that ocean warming has altered the bioenergetics and food web structure in the Gulf of Maine, driving the trend of increasing MeHg concentrations in Atlantic bluefin tuna since the 2010s. This is likely also the case in the Arctic, which is experiencing a rapid increase in sea surface temperature and dramatic sea ice reduction.17–19 Increasing seawater temperatures could change a number of bioenergetic parameters relevant to MeHg bioaccumulation in individual organisms, including food ingestion, respiration, growth, and elimination rates.20 At the population level, rising seawater temperature has led to the northward expansion of subarctic species into the Arctic and the recession of cold pools essential for Arctic resident species, causing structural change in Arctic marine food webs.21 A number of studies have linked the temporal trends of Hg in some Arctic species (e.g., polar bears, ringed seals, beluga, and seabirds) to rapid sea-ice reduction, which has altered the timing, intensity, and composition of plankton production and induced ecosystem shift from an ice-associated food web to a pelagic one.22–27
Measured concentrations of MeHg in Arctic species over time represent the net effect of environmental factors governing Hg loading, methylation and demethylation rates, and ecological characteristics such as trophic interactions and bioenergetics;28–30 thus, it is often challenging to use these empirical data alone to identify the driving factors of changes in Hg levels in biota. To elucidate the pathways of global environmental change on MeHg bioaccumulation in Arctic ecosystems, an effective tool that connects environmental factors (e.g., sea ice, temperature, and contaminant loading) with food-web dynamics is urgently needed. Here we develop an ecosystem-based bioaccumulation model for the Beaufort Sea shelf ecosystem and provide a holistic analysis of how the Hg burden of marine biota responds to changes in environmental and ecological factors relevant to Hg bioaccumulation. We aim to (1) test whether a food web model with complex trophodynamics and relatively simple MeHg model parametrization can capture observed patterns of MeHg bioaccumulation at each trophic level; and (2) generate hypotheses about the most influential environmental and toxicokinetic factors driving the variability of MeHg concentrations in fish and marine mammals in the Beaufort Sea shelf ecosystem. This research provides useful information for further assessing the pathways of global environmental changes on MeHg bioaccumulation in Arctic ecosystems in the past and the future.
2. Materials and methods
2.1. Study area
Our model area is the Canadian Beaufort Sea shelf (hereafter referred to as the BSS, Fig. 1), the largest North American shelf in the Arctic. The majority of the BSS is shallower than 200 m, and north of the BSS is the Canada Basin, which extends roughly 1130 km north and reaches a depth of 3600 m. This region has experienced a range of climate change impacts, including increased air and water temperature, decreased sea-ice extent, a longer open water season, and more frequent and extreme storms.31,32 The BSS, which provides habitat for many resident and migratory marine mammals and fish species, is part of the homeland of the Inuvialuit people. Several studies have reported Hg concentrations of environmental and biological samples collected in this region, including seawater,15 plankton,33–36 benthos,33,37,38 and various fish species and ringed seals.27,33 In addition, as a sentinel species for ecosystem-based monitoring of contaminant cycling and climate change, the Eastern Beaufort Sea Beluga stock (“Beaufort beluga” hereafter) and its Hg burden have been monitored for almost four decades in Canada by a community-based biomonitoring program led by the Fisheries Joint Management Committee (FJMC), a co-management body with members appointed by both the Inuvialuit Game Council and the Government of Canada.39 In addition to Hg data, Inuvialuit harvesters have rich knowledge of the ecosystem and the species inhabiting it (Fig. 1).
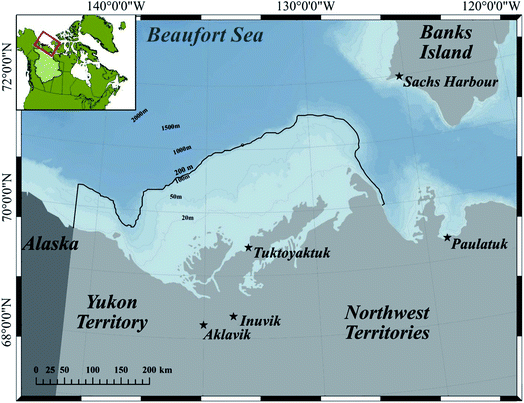 |
| Fig. 1 Map of the Canadian Beaufort Sea shelf and surrounding communities (the stars). The model area, Beaufort Sea shelf including Mackenzie estuary, is defined by the 200 m contour (outlined in black) along the shelf-break in Canadian waters (map reproduced from Hoover et al. 2021 (ref. 40) with permission). | |
2.2. Food web
The trophodynamics of the BSS marine ecosystem across different functional groups, ranging from primary producers to top predators, for the period 1970–2012, have been previously constructed using the Ecopath with Ecosim open-source modeling software suite (EwE) and trophic structured in agreement with stable isotope analysis.40,41 For each functional group, the EwE model setup simulates the whole population that encompasses different sexes, ages, and body sizes. The detailed characteristics of specific populations in the BSS food web have been described in prior studies.40,41 EwE is a widely used trophodynamic ecosystem approach for simulating the flow of mass and energy across the food web using information including the biomass, feeding, production, and mortality rate of components of a variety of functional groups.42 In this study, we use the BSS food web model built in Ecopath that reflects the average food web structure and dynamics between 2008 and 2012. It contains 31 functional groups, ranging from primary producers and detritus to top predators (i.e., beluga whales) (Table 1), and represents a mass-balanced ecosystem structure in which biomass flows into a group via reproduction and immigration, and equally, flows out of the group through predation, harvest, and natural mortality.
Table 1 Model input in Ecotracer for simulating MeHg dynamics in the Beaufort Sea food web (see detailed description of each group in ESI Table S1)
Group namea |
Initial conc. (t t−1) |
Direct absorption rateb (km2 per ton per year) |
Proportion of contaminant assimilatedc |
Elimination ratee (per year) |
Detailed description of the species composition in each group can be found in Table S1.
Only applies to benthos, zooplankton, and primary producers. See ESI 1.1 for calculations of each group.
Adopted the average assimilation efficiencies in the literature for zooplankton,43 bivalves and mollusks,13 worms,13 and fish.13 Arthropods, echinoderms, other benthos, and jellies were assumed to have the same assimilation efficiency as macro-zooplankton. Marine mammals were assumed to have the same assimilation efficiency as fish.
Set as zero for the low elimination rate scenario.
The elimination rates of pilot whale, baleen whale, and seal from Booth and Zeller 2005 (ref. 44) are used for beluga, bowhead, and ringed and bearded seals here. The average elimination rate of bivalves from Pan and Wang 2011 are used for all benthos. The rate for other lower trophic level organisms is calculated and details can be found in ESI 1.2.
|
Beluga |
0 |
0 |
0.85 |
0.100 |
Bowhead |
0 |
0 |
0.85 |
0.200 |
Ringed Seal |
0 |
0 |
0.85 |
0.250 |
Bearded Seal |
0 |
0 |
0.85 |
0.250 |
Char & Dolly Varden |
0 |
0 |
0.85 |
0.328 d |
Ciscos & Whitefish |
0 |
0 |
0.85 |
0.367 d |
Salmonids |
0 |
0 |
0.85 |
0.363 d |
Small Nearshore Forage Fish |
0 |
0 |
0.85 |
0.415 d |
Arctic & Polar Cods |
0 |
0 |
0.85 |
0.450 d |
Capelin |
0 |
0 |
0.85 |
0.636 d |
Flounder & Benthic Cods |
0 |
0 |
0.85 |
0.231 d |
Small Benthic Marine Fish |
0 |
0 |
0.85 |
0.388 d |
Other Fish |
0 |
0 |
0.85 |
0.388 d |
Arthropods |
0 |
0.001 |
0.85 |
1.825 d |
Bivalves |
0 |
0.00027 |
0.65 |
1.825 d |
Echinoderms |
0 |
0.00027 |
0.65 |
1.825 d |
Mollusks |
0 |
0.00027 |
0.65 |
1.825 d |
Worms |
0 |
0.00027 |
0.65 |
1.825 d |
Other Benthos |
0 |
0.00027 |
0.85 |
1.825 d |
Jellyfishes |
0 |
0.0000158 |
0.85 |
1.825 d |
Macro-Zooplankton |
0 |
0.0000158 |
0.85 |
1.241 d |
Medium Copepods |
0 |
0.000378 |
0.6 |
3.468 d |
Large Copepods |
0 |
0.00011 |
0.85 |
2.190 d |
Other Meso-Zooplankton |
0 |
0.0002 |
0.6 |
7.410 d |
Micro-Zooplankton |
0 |
0.00134 |
0.6 |
11.607 d |
Large Pelagic Producers |
0 |
0.00024 |
0 |
0 |
Small Pelagic Producers |
0 |
0.0024 |
0 |
0 |
Ice Algae |
0 |
0.0006 |
0 |
0 |
Benthic Plants |
0 |
0.0001182 |
0 |
0 |
Pelagic Detritus |
0 |
0 |
0 |
0 |
Benthic Detritus |
0 |
0 |
0 |
0 |
2.3. Ecotracer simulation
We use Ecotracer, an EwE module, to simulate the bioaccumulation of MeHg in the BSS ecosystem by tracking the gains and losses of MeHg in all functional groups. The total amount of MeHg in each functional group is calculated based on five processes: predator–prey interactions, direct uptake from seawater, internal metabolism, internal decay, and harvest. Walters and Christensen (2018)45 provided a detailed description of the Ecotracer module, and this tool has been applied to simulate the dynamics of various contaminants in marine ecosystems, including Hg,44,46,47 persistent organic pollutants,46–48 microplastics,49 and radioisotopes.45,50,51
The intake amounts of MeHg for functional group i come from the uptake of MeHg from either water (i.e., μiBiCo in eqn (1)) or food (i.e.,
in eqn (1)). The losses of MeHg for group i are attributed to predation
, harvest (Hi), natural mortality (MOi), and elimination of MeHg (Ei), including direct excretion and metabolic transformation (namely demethylation) (eqn (2)). The sum of predation, harvest, and natural mortality is the total mortality (i.e., the ratio between production and biomass P/B), an indicator for the population turnover rate. The P/B ratio for each group is included in Table S1.† Our simulation generates the steady-state MeHg concentration for each group (i.e., when intake = loss):
|  | (1) |
where
Co represents the seawater MeHg concentration (ton per km
2); for group i,
Bi is the biomass (ton),
μi is the direct absorption rate of MeHg from water (km
2 per ton per year), and AE
i is the assimilation efficiency.
Qji is the consumption rate (ton per year) of prey j by predator i, and
Cj is the MeHg concentration in prey j (ton of MeHg per ton of biomass).
|  | (2) |
where
Qik is the rate of consumption (ton per year) of group i due to predation by k and

is the fraction of group i consumed by predator k,
Hi is the mortality rate due to harvests (per year), MO
i (per year) is the natural or other mortality rate,
Ei (per year) is the elimination rate, and
Ci is the MeHg concentration in predator i (ton of MeHg per ton of biomass).
All initial concentrations of MeHg are set to zero for all functional groups and seawater. MeHg is released into the modeled environment through a base inflow rate and is lost through biological uptake and base volume exchange. No information is available for the net input of MeHg into the BSS system, so we set the simulated BSS seawater MeHg concentration to match the observed peak concentration (0.224 pM) of a shelf-break station in the Beaufort Sea (71° N 04.933′, 133° W 39.072′),15 through a constant abiotic flow rate through a system of 1.05 × 10−5 ton MeHg per km2 per year. Limited seawater MeHg data are available for the BSS. Because coastal shelves generally have higher MeHg concentrations than the adjacent continental slope and open ocean, as a result of direct MeHg inputs from rivers and wetlands and elevated MeHg production in benthic sediment and water,8,52–54 we use the observed peak MeHg concentration between the shelf and slope to represent the average seawater MeHg level on the BSS. We run a 100 year simulation and the MeHg concentrations of all functional groups reach a steady state between 40 and 50 years.
The trophic interactions in the BSS food web have been characterized in Ecopath through the literature and stable isotope data in previous studies, which account for temperature and the individual body size (e.g., fish length and weight) when calculating the mortality and consumption rate for each functional group.40,41 Other parameters required for characterizing MeHg dynamics in Ecotracer include the direct absorption rate, assimilation efficiency of MeHg from food, and elimination rate. We estimated these parameters using values or equations generated in prior studies (Table 1, see the detailed methodology for parameterization in the ESI†). We applied direct absorption of MeHg from water to low-trophic level organisms (i.e., benthic plants, phytoplankton, zooplankton and benthos), as this is one of the dominant pathways for their MeHg accumulation.55–57 Since MeHg accumulated in higher trophic level groups predominantly comes from dietary intake,58–60 we assume no direct uptake of MeHg from water in these groups. The direct absorption rate in phytoplankton is calculated based on the phytoplankton size classes and the DOC concentrations in the seawater collected from the Beaufort Sea shelf (∼120 μM C61).43 The direct absorption rate in zooplankton is a function of its mass and temperature, as described by Schartup et al. (2018).43 To estimate the direct absorption rate of Arctic benthos, we first derive a linear relationship between the MeHg absorption rate and filtration rate based on the experimental results of various bivalve species at room temperature.57 We then calculate the MeHg absorption rate of Arctic bivalves based on the filtration rate of Arctic clams62 and account for temperature effects on the MeHg uptake rate in cold waters.63 We describe the calculations of the direct absorption rate for each group in detail in ESI 1.1.†
We assume that elimination of MeHg occurs in all consumers and not in producers. The elimination rates of MeHg in zooplankton and fish are calculated based on the body mass and temperature using previously published equations (Schartup, 2018 (ref. 43) and Trudel, 1997 (ref. 91)) (see ESI 1.2†). Prior studies that used the Ecotracer module to simulate the Hg dynamics in marine or freshwater ecosystems have all set elimination rates of MeHg in fish and lower trophic level organisms as zero due to slow excretion and inefficient internal demethylation.44,46,47 We therefore run and compare the simulations with and without empirical elimination rates of MeHg for fish and lower trophic levels. In vivo demethylation of MeHg occurs widely in marine mammals, which transforms MeHg into labile inorganic Hg and HgSe nanoparticles.64–69 No field or lab data are available for estimating the elimination or demethylation rate of MeHg for marine mammals (e.g., seals and whales). Here we use the literature values for similar marine mammals previously estimated by ecosystem modeling approaches to represent the elimination rate (i.e., excretion and demethylation) of MeHg in these organisms44 (Table 1).
2.4. Data analysis
2.4.1. Comparing the model output with observations.
We calculate the average of observed mean concentrations across each empirical study as the overall observed mean MeHg concentration. To assess the model bias for each functional group, we calculate the ratio between modeled (M) and observed MeHg concentrations (O) (eqn (3)) and normalized mean bias (eqn (4)). The results are shown in Table S1.† | 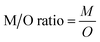 | (3) |
| 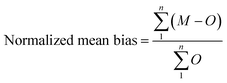 | (4) |
where n is the number of empirical studies that generate observed mean MeHg values.
2.4.2. Estimating trophic magnification of MeHg.
The trophic magnification factor (TMF) has been widely used as a tool for assessing chemical bioaccumulation in different ecosystems.70 For a given chemical, many factors, such as ecological and ecosystem characteristics, data treatment, and study design can lead to variability and uncertainty in estimated TMFs.70 Here we derive the model predicted TMF for the BSS food web and assess its conformity to empirical studies as a tool to evaluate our model performance and gain mechanistic understanding of BSS food web biomagnification, rather than accurately quantifying the degree of MeHg bioaccumulation in this food web.
We run a simple linear regression of modeled MeHg concentrations across the entire BSS food web against their respective trophic levels previously determined by Hoover et al. (2021)40 (eqn (5)). We then calculate the TMF as the antilog of the regression slope (eqn (6)). As beluga and bowhead whales migrate between the Beaufort, Chukchi, and Bering Seas annually, they are transient species that may not exclusively fit in the BSS food web. To reflect the extent of biomagnification in the BSS ecosystem, we produced the TMF of resident organisms in the BSS by excluding beluga and bowhead whales. Prior studies reveal substantial differences between the TMFs of organic pollutants in the piscivorous and marine mammalian food webs from the same Arctic ecosystem.71 We also calculated the TMF of the piscivorous food web (i.e., predatory fish as apex predators) for comparing TMFs between piscivorous and marine mammalian food webs in the BSS:
where [MeHg] and TL are the MeHg concentration and trophic level of organisms in the BSS food web, respectively.
2.4.3. Sensitivity analysis.
To investigate the most influential parameters responsible for MeHg bioaccumulation in the BSS food web (as represented in our model), we conduct sensitivity analyses of seawater and benthic detritus MeHg concentrations and the toxicokinetic parameters regarding the uptake, biotransformation, and elimination of MeHg in each broad biota category (i.e., marine mammals, fish, benthos, zooplankton, producers). We perturb each parameter by ±10% of the original amount, and the sensitivity is calculated using eqn (7): | 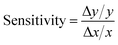 | (7) |
where x is a specific parameter and y is the simulated MeHg concentration of each organism. Δy is the change in the MeHg concentration (y) because of the change (Δx) of the input parameter. Δx/x is fixed as 10% in our sensitivity analysis.
3. Results and discussion
3.1. Model performance and evaluation
Given the EwE model setup, our simulation produces average MeHg concentrations for each functional group population. We found that the simulated population-wide MeHg concentrations of most BSS functional groups are comparable to their respective published values (i.e., within ±SE) without MeHg elimination in fish and lower trophic level organisms, as shown in Fig. 2a. In contrast, the application of empirical elimination rates of MeHg in fish and lower trophic levels leads to apparent underestimation of MeHg concentrations in most groups and we discuss the underlying reasons in Section 3.4. Herein, we only evaluate the model output from the runs with no elimination of MeHg applied in fish and lower trophic level organisms.
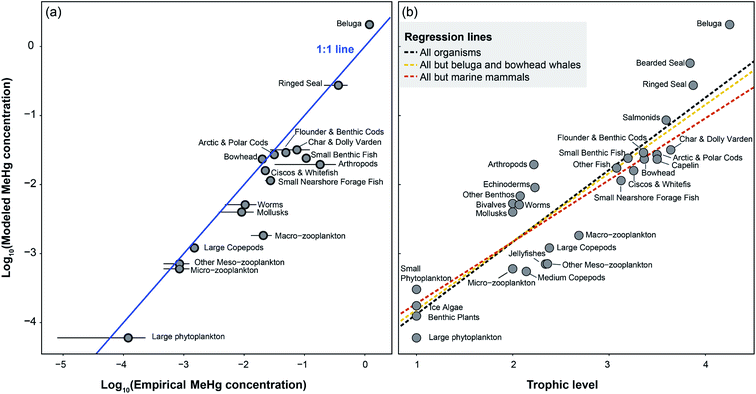 |
| Fig. 2 (a) Comparison between log transformed modeled and empirical MeHg concentrations (μg g−1 wet weight) of Beaufort Sea shelf food web. The blue line signifies the 1 : 1 ratio. The compiled dataset of empirical concentrations and standard errors (SE) indicated as error bars can be found in the ESI.† (b) Methylmercury biomagnification in the Beaufort Sea shelf food web. The dotted lines represent regression of MeHg concentrations of all or subsets of organisms in the food web. The regression slopes for black, yellow, and red lines are 1.05 ± 0.10 (SE), 0.99 ± 0.10, and 0.89 ± 0.11, corresponding to TMFs of 11.1, 9.8, and 7.8. | |
The challenges of using Ecotracer to simulate MeHg bioaccumulation in a food web have been noted in prior studies, indicated by large model bias (M/O ratio = 0.016 to 0.056)46 or lack of overlap between predicted and observed ranges.47 Compared to these previous studies, our model simulation shows significant improvement (M/O ratio = 0.088 to 0.86; normalized mean bias = −0.91 to −0.14, across functional groups) and we attribute the better performance to the well-characterized nature of the BSS food web and detailed parameterization of the direct absorption rate of lower trophic level organisms based on the body size and temperature. Compared with available MeHg observations of BSS biota, our model tends to underestimate MeHg concentrations except for two highly migratory marine mammals – Beaufort beluga (M/O ratio = 1.74; normalized mean bias = 0.74) and bowhead whales (M/O ratio = 1.17; normalized mean bias =0.17) (Fig. 2a and Table S1†). This may result from underestimating the seawater MeHg concentration and/or the uptake of MeHg at the base of the BSS food web given the great influence of these factors on the MeHg concentration in all BSS biota (see Section 3.2).
The estimated MeHg concentrations in the pelagic food web (e.g., phytoplankton, zooplankton, and bowhead) match the observed values better than organisms from the benthic food web (e.g., worms, mollusks, arthropods, and benthic fish) (Fig. 2a). Marine sediment is the major MeHg production source in contaminated coastal regions,72–77 while active methylation in marine waters is considered the dominant source of MeHg in the (sub)Arctic.15,54,78 Our results suggest that the BSS sediment could still play an appreciable role in contributing to MeHg accumulated in benthic organisms and, consequently, their predators (e.g., fish and marine mammals). The inability to capture legacy Hg contributions and in situ methylation in sediment in the current Ecotracer module setup may drive the overall underestimation performance. To better represent food-web MeHg bioaccumulation in many shallow coastal ecosystems, including the BSS, we suggest adding functions that take into account the direct loading of MeHg to the benthic ecosystem by the deposition of river-born particles and the methylation of legacy and present inorganic Hg in the benthic environment in Ecotracer.74,79 In addition, MeHg concentrations in the water column vary by depth and organisms foraging at different depths could receive different Hg burdens,80–82 which is currently not accounted for in this model due to lack of information on the vertical MeHg profile in the BSS. Future work generating data of MeHg variability by depth and incorporating this information into another EwE module, Ecospace, could provide more accurate simulation of MeHg bioaccumulation in each functional group.
Large differences between simulated and observed MeHg concentrations (M/O ratio = 0.088 to 0.22) appear in groups that encompass a wide range of species or genera, such as macro-zooplankton, arthropods, and small benthic fish (Fig. 2a). The highly heterogeneous species composition of these functional groups leads to the large variability in MeHg concentrations observed in field measurements. For example, the macro-zooplankton group of the BSS food web includes krill, shrimp, mysids, and amphipods40 and the simulated MeHg concentration for this group is 1.8 ng g−1 wet weight. The MeHg concentrations of field collected macro-zooplankton vary from 3.2 ± 1.8 (mean ± SD) ng g−1 wet weight in omnivorous krill (Thysanoessa spp.) to 65 ± 10 ng g−1 wet weight in carnivorous circumpolar shrimp (Eualus gaimardii),36,38 with amphipods and mysids falling in that range (Table S1†). The simulated MeHg concentration of each functional group represents its average MeHg level, thus it may not match the empirical data of any single species, particularly non-dominant species of that group.
The Hg burden of Beaufort beluga whales has been monitored for almost four decades and a previous study showed that the average MeHg concentrations in muscle tissues of this population varied within a relatively small range (0.97–1.4 μg g−1 wet weight) between 2005 and 2012.39 Our simulated beluga MeHg concentration (2.1 μg g−1 wet weight) is higher than the empirical range, and we attribute the difference to their migratory foraging behavior, which is currently not accounted for in the EwE representation of the BSS, as elaborated in Section 3.5.
3.2. Sensitivity analyses of environmental and toxicokinetic factors
3.2.1. Seawater and benthic detritus MeHg.
The modeled MeHg concentration in all functional groups is highly sensitive to the seawater MeHg levels (sensitivity ≈ 1), indicating that simulated changes in seawater MeHg will result in proportional changes in the biota MeHg burden. The MeHg concentrations of all groups respond little to the change in the initial MeHg concentration of benthic detritus (sensitivity close to zero). This is because in our Ecotracer representation of the system, the benthic detritus in the BSS is largely driven by sinking particles containing mostly ice algae and to a lesser extent phytoplankton, which ultimately obtain MeHg from seawater. Variation in the initial MeHg level of benthic detritus alone only leads to a transient change of MeHg in benthic organisms, and the seawater MeHg concentration is the factor that dictates the steady-state MeHg concentration of these organisms in the model.
Climate change likely influences MeHg concentrations in BSS seawater in multiple ways. Leitch et al. (2007)53 showed that the Mackenzie River input is the dominant Hg source in the Beaufort Sea. The warming of the Mackenzie Basin is likely to increase both inorganic Hg and MeHg riverine fluxes to the Beaufort Sea due to permafrost melt, increases in freshwater discharge, and more frequent extreme events (flooding, storms, and forest fires).52,53 Riverine MeHg bound to the terrestrial dissolved organic matter (DOM) can be resistant to degradation and is biologically available.83 With the rise in temperature, inorganic Hg inputs, terrestrial DOM discharge, and the microbial methylation of inorganic Hg in the BSS water column would likely be enhanced.52,53,84 In the meantime, the dramatic reduction in sea ice and longer open-water periods lead to increased light penetration in ice-free seawater thereby fostering photodegradation of MeHg. To date, little quantitative data exist to infer the net effect of these environmental variabilities on MeHg production and degradation in the Arctic. Given the high sensitivity of the MeHg burden in biota to seawater MeHg levels, an accurate assessment of climatic impacts on the net MeHg production (or loss) in the Arctic marine ecosystems should be among the top research priorities for addressing the impacts of global environmental changes on MeHg bioaccumulation in Arctic food webs.
3.2.2. Low trophic level organisms.
All producers (benthic plants, phytoplankton, and ice algae) obtain MeHg from the water via passive absorption,55 hence their MeHg concentrations are only controlled by their direct absorption rate (Fig. 3; Table S2†). Zooplankton, which obtain MeHg from both water and food uptake, are sensitive to direct absorption rates of phytoplankton and zooplankton, and the assimilation efficiency of MeHg in zooplankton. Benthic organisms, such as bivalves and arthropods, are pivotal for transferring MeHg to fish and marine mammals in the BSS marine ecosystem. Pan and Wang (2011) (ref. 57) illustrated the strong ability of bivalves to obtain MeHg from both dissolved and dietary phases. Detrital materials, which are ultimately derived from ice algae and phytoplankton, are the major dietary sources of benthic organisms in the BSS. Our sensitivity analysis suggests that the MeHg burden in benthic organisms is controlled by three factors: direct absorption rate and assimilation efficiency of MeHg in benthos, and the direct absorption rate in producers, with the dissolved MeHg direct uptake rate playing a dominant role (Fig. 3; Table S2†). We hypothesize that this is partially due to the underestimation of MeHg intake from benthic detritus for the reasons elaborated in Section 3.1. In addition, the direct absorption rate of Beaufort Sea benthic organisms was estimated based on the relationship between MeHg uptake and filtration rates derived in laboratory experiments on subtropical bivalves, as this information is unavailable for other types of benthos. The high uncertainty of this parameter, combined with its large influence in upper trophic level MeHg concentrations, underscores the need to characterize the biodynamics of the MeHg accumulation in non-bivalve benthos and in benthic organisms living in cold marine environments.
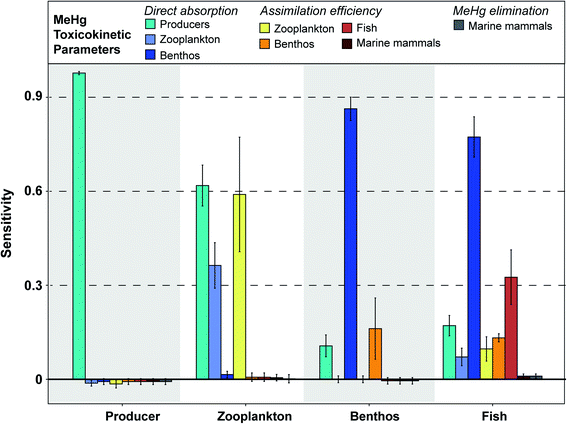 |
| Fig. 3 Sensitivity of simulated MeHg concentrations in primary producers, zooplankton, benthos, and fish to each input parameter, as measured through the sensitivity coefficient. The further the sensitivity coefficient is from 0, the more sensitive the simulated MeHg concentration is to changes in the input parameter. Sensitivity coefficients are generated by decreasing or increasing each parameter by 10% of the baseline. Coefficients were largely symmetrical between increases and decreases, so the plot only shows the data generated by increasing the input parameter by 10%. Full details of these sensitivity coefficients for each parameter can be found in Table S2.† | |
Our model assumes that all MeHg uptake by fish species comes from ingested food. Fish species in the BSS consume a variety of lower-trophic level organisms, which explains that factors related to the MeHg concentrations in producers, zooplankton and benthos all have an impact on fish MeHg concentrations. Although dietary preferences differ across these fish groups, a general pattern of sensitivity coefficients is found: direct absorption rate of MeHg in benthos (sensitivity: 0.70 to 0.87) and assimilation efficiency in fish (sensitivity: 0.18 to 0.43) are the most influential factors associated with fish MeHg concentrations (Fig. 3). This highlights the importance of the benthic pathway for transferring MeHg to fish, as discussed in detail earlier.
3.2.3. Marine mammals.
The sensitivity of MeHg concentrations in mammals to different toxicokinetic parameters of lower trophic level organisms reflects their feeding strategies (Fig. 4). The most influential factor for varying MeHg burden in piscivorous marine mammals, including beluga, ringed seals, and beard seals, is the direct absorption rate of MeHg in benthos, the same as the most sensitive parameter for marine mammals' diet – fish. In contrast, bowhead whales, a filter-feeding marine mammal species that feed primarily on zooplankton, are highly sensitive to the direct absorption rate of phytoplankton and zooplankton and the assimilation efficiency of MeHg in zooplankton, the factors controlling the MeHg content of zooplankton (Fig. 4).
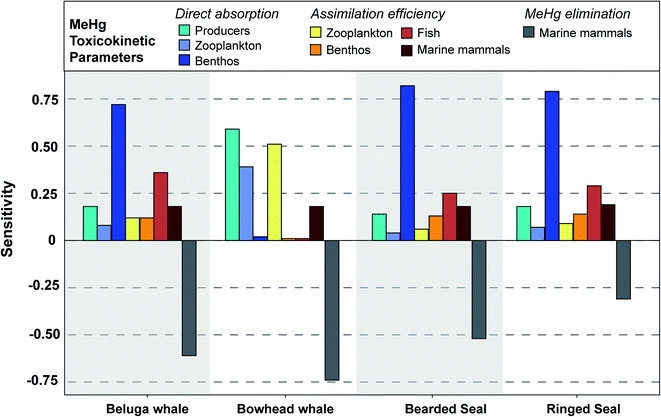 |
| Fig. 4 Sensitivity of simulated MeHg concentrations in four types of marine mammals to each input parameter, as measured through the sensitivity coefficient. The further the sensitivity coefficient is from 0, the more sensitive the simulated MeHg concentration is to changes in the input parameter. Negative coefficients indicate opposing directions of change (i.e., an increase in MeHg elimination results in a decrease in the concentration). Sensitivity coefficients are generated by decreasing or increasing each parameter by 10% of the baseline. Coefficients were largely symmetrical between increases and decreases, so the plot only shows the data generated by increasing the input parameter by 10%. Full details of these sensitivity coefficients for each parameter can be found in Table S2.† | |
In vivo demethylation of MeHg is widely observed across marine mammals and is considered the main mechanism for MeHg elimination; therefore, the MeHg concentrations of beluga, bowhead whales, and bearded seals are highly sensitive to their demethylation rates (sensitivity: −0.52 to –0.74) (Fig. 4). Compared with other marine mammals, ringed seals are less sensitive to the demethylation rate (sensitivity: −0.31) (Fig. 4). This results from their rapid population turnover (i.e., high mortality rate P/B) in the BSS ecosystem due to hunting activities by polar bears and humans. As mortality and MeHg elimination are the two main mechanisms for MeHg loss in each functional group (see eqn (2)), the higher mortality rate means greater importance of population turnover relative to MeHg elimination in determining MeHg concentrations in any given group. Ringed seals are the major diet of polar bears in the ecosystem and are harvested by Inuvialuit communities.85 The predation and hunting mortality rates of ringed seals are 10 and 6 times higher than those of bearded seals, which largely explains the simulated difference in MeHg concentrations and sensitivity to the MeHg elimination rate between these two species. Prior studies found that age classes, feeding strategies, and trophic level can play roles in determining the MeHg concentrations of phocid seals (i.e., ringed, bearded, spotted, and harbor seals) in the Alaskan and Canadian Arctic.86,87 Our model simulation demonstrates that food web trophodynamics, particularly top-down interactions including predation and fishing activities, could also have a large impact on population average MeHg concentrations of these species, thus stressing the importance of the food web context when interpreting the Hg burden.
3.3. MeHg biomagnification in the BSS food web
Polar systems are known to biomagnify MeHg more efficiently than ecosystems at lower latitudes due to longer food chains, limited biomass dilution, and slower excretion of MeHg at colder temperatures.88 We estimate that the TMF of the entire BSS food web is 11.1, similar to the empirical TMF value (10.1) of the Beaufort Sea estuarine and shelf food web with beluga whales as an apex predator.33 The simulated and observed TMF values of the BSS food web are among the highest TMFs of MeHg reported for other polar marine ecosystems (range: 3.0 to 11.3), including Baffin Bay, Svalbard, West Greenland, Melville Sound, and Gulf of Amundsen.88 Although the TMF is a relatively simple metric given complex real-world food webs, it has utility as a screening metric to highlight important areas for further comparative research between ecosystems.88 Our results suggest that there may be highly efficient biomagnification of MeHg throughout the BSS food web relative to other polar systems, and highlight the potential vulnerability of elevated MeHg exposure in the BSS fish and marine mammals, and the Inuvialuit communities who rely on these wildlife as nutritionally and culturally important subsistence food.
The estimated TMFs of the BSS food web without migratory species (i.e., beluga and bowhead whales) and without marine mammals are 9.8 and 7.8, respectively, slightly lower but statistically indifferent from the TMF of the entire BSS food web at the α =0.05 level. This is consistent with the finding that there is no significant effect of the food web composition on the TMF in other marine ecosystems as summarized by Lavoie et al. (2013),88 but contrary to the recent observation in Antarctic marine ecosystems where the TMF is higher when endotherms are included.89 As many endotherms like marine mammals and birds are migratory, we suggest that the influence of the food web composition on the TMF observed in some studies may originate from their MeHg uptake via foraging outside of the studied food web. We illustrate how Beaufort beluga MeHg concentrations vary depending on the extent of foraging in different feeding grounds in Section 3.5.
Similar to many other coastal shelf ecosystems, the BSS has a highly coupled benthic and pelagic food web.40Fig. 2b illustrates that both benthic and pelagic biomass production are important contributors for transferring MeHg to higher trophic level organisms like fish. The heavy reliance on benthic organisms that contain higher levels of MeHg than pelagic ones leads to the high TMF in the BSS food web (Fig. 2b). Although at similar trophic levels, both simulated and literature MeHg concentrations in benthos are almost one order of magnitude higher than those in zooplankton. The major dietary component of benthic organisms is benthic detritus, largely composed of sinking particles on the sea floor, while phytoplankton are the dominant food source for zooplankton. Benthic detritus and phytoplankton have comparable simulated MeHg concentrations (benthic detritus: 0.26 ng g−1 wet weight; phytoplankton: 0.06 to 0.30 ng g−1 wet weight) (Table S1†). Thus, the higher MeHg concentrations in benthos than zooplankton result from the greater biomagnification step between benthic detritus and benthos (BMF: 15–75) than the one between phytoplankton and herbivorous/omnivorous zooplankton (BMF: 2–20) (Fig. 2b). We attribute the elevated biomagnification at the base of the BSS benthic food web to population dynamics because turnover in benthos is on an average 15 times slower than that of zooplankton communities. In other words, benthos generally have a much longer lifetime to accumulate MeHg than zooplankton. These findings emphasize the necessity of incorporating population turnover or biomass dilution in addition into trophic levels for predicting MeHg concentrations of marine organisms.
In addition, Pomerleau et al. (2016)36 reported higher total Hg and MeHg concentrations in marine zooplankton collected in the BSS than those from five other Arctic regions, including the Laptev Sea, Chukchi Sea, Canadian Arctic Archipelago, Hudson Bay, and northern Baffin Bay. Hence, it is possible that the BSS pelagic food web also has elevated bioconcentration between water and phytoplankton and/or greater biomagnification between phytoplankton and zooplankton relative to other Arctic ecosystems. Further research effort on MeHg bioaccumulation at the base of Arctic marine food webs is required to fully elucidate the reasons for elevated MeHg burden in the BSS marine biota.
3.4. Population-wide MeHg elimination rate
If empirical elimination rates of MeHg in fish and lower trophic levels are applied, the simulated MeHg concentrations in benthos, fishes, and piscivorous marine mammals are on an average 9 times lower than the observed values. The discrepancy between simulated and observed values indicates that the empirical MeHg elimination rates, often derived from controlled laboratory experiments on a small number of individuals, do not accurately reflect the MeHg toxicokinetics in fish and lower trophic levels in the natural environment. Following other studies,13,90 we used the chronic MeHg exposure equation based on the fish body size and water temperature to estimate the MeHg elimination rates in free-ranging fish in the field.91 The data used for deriving this equation are largely from studies in which fish were exposed to MeHg in artificial ways (e.g., concentrated MeHg solution and food spiked with MeHg). Recent evidence from both field and laboratory studies showed that fish fed with naturally contaminated prey have 2.4 to 5.5 times lower MeHg elimination rates than those estimated by using the chronic exposure equation.92 Future work incorporating factors that can cause differences in MeHg elimination rates in fish and macroinvertebrates, such as age, sex, and species,20,57,92,93 may improve the applicability of the laboratory derived MeHg elimination rates in field studies.
In addition to MeHg elimination from fish bodies,91,92 mortality is the other route of MeHg loss in any given functional group (eqn (2)). In EwE, when the biomass is at a steady state, the mortality rate is calculated as the ratio between production and biomass and reflects how fast the population turns over. In the field, relatively fast reproduction, growth, predation, and mortality often occurs in low-trophic level organisms like plankton, benthos, and fish. Population dynamics are therefore directly related to the total MeHg burden in a functional group, and thereby the average MeHg concentration of the population. For example, the recruitment of new fish adds new MeHg into the fish population and mortality due to predation, fishing, and other reasons removes the MeHg in deceased fish out of the group. The simulations with no MeHg elimination in fish and lower trophic level organisms yield the MeHg concentrations comparable to observed levels, indicating that population turnover may be the dominant factor for explaining the MeHg loss in each group.
A recent study based on a 15 year whole-ecosystem experiment showed little to no loss of Hg in northern pike in 6 to 8 years after the cessation of Hg spike addition to a boreal lake.94 The authors hypothesized that population turnover, rather than the slow MeHg elimination, drives the change in the MeHg concentration of the fish population in the field.94 Our study further corroborates this proposition by using a modeling approach to illustrate that a food web model with well-defined population dynamics is adequate to predict population-wide MeHg concentrations in lower trophic level organisms; therefore, it is appropriate to assume a negligible amount of MeHg eliminated by these functional groups throughout their lifetime. Nevertheless, it is worth mentioning that MeHg elimination is an important route of Hg loss in species with slow population turnover in the field and/or with enhanced demethylation capacity, such as marine mammals.
3.5. Bering Sea vs. Beaufort Sea foraging
We find that the simulated MeHg concentration of Beaufort beluga whales (2.1 μg g−1 wet weight) is 1.8 times higher than the observed mean Hg concentrations (∼1.2 μg g−1 wet weight), which implies that we may have overestimated the MeHg intake from food. The model simulates the beluga MeHg concentration assuming that they exclusively forage in the BSS year-round. However, Beaufort beluga whales only spend summer in the eastern Beaufort Sea and Mackenzie Delta region, and they migrate through the Chukchi Sea to the Bering Sea where they spend winter,39,95,96 with the feeding contribution of the Beaufort Sea unknown. The consistency between simulated and observed MeHg concentrations in major dietary items of beluga (e.g., Arctic cod, cisco & whitefish) suggests that belugas' dietary exposure of MeHg in the Beaufort Sea is not overestimated. Therefore, we postulate that the lower-than-simulated MeHg concentrations for this beluga stock mainly results from consuming fishes with lower MeHg content in the Bering and Chukchi Seas compared to those in the BSS.
The Beaufort Sea shelf is known to have elevated Hg concentration in the water column and marine food chain compared to other Arctic regions. Seawater in the BSS was found to have much higher MeHg concentrations (0.134 pM at Chla max, and 0.227 pM at oxycline),8,15 in comparison to less than the detection limit (<0.020 pM) throughout the water columns across six stations in the Bering Sea.97 The marine zooplankton collected in the BSS exhibited higher MeHg concentrations relative to other Arctic regions such as the Laptev Sea, Chukchi Sea, Canadian Arctic Archipelago, Hudson Bay and northern Baffin Bay.36 We compared the published Hg values of common prey items of Beaufort beluga whales, such as Arctic cod, Pacific herring, and other species. The MeHg concentrations of the same prey species are 2.0 to 2.7 times lower in the Bering Sea than the ones collected in the Beaufort Sea (Table S3†), supporting our hypothesis. Given the relatively high reporting limit of some fish Hg data collected in the Bering Sea98 and that this beluga stock frequently consumes lower-trophic level organisms in the Bering Sea (e.g., shrimp and octopus),99 we anticipate that the ratio (R) of average MeHg concentrations in the belugas' prey intake from the Beaufort vs. Bering Sea may be greater than that observed.
Here we explore how Beaufort beluga MeHg concentrations vary depending on the extent of feeding in the Bering Sea vs. Beaufort Sea, under two scenarios of the concentration ratio (R = 2 or 4) between the two Arctic regions (Fig. 5). The observed MeHg concentration of beluga came from subsistence harvests in the Mackenzie Delta,39 where about 90% of the landed catch of Beaufort beluga in the past occurred in July, shortly after their spring migration from Bering Sea.96,100 Given belugas' foraging behavior in the Chukchi and Bering Seas prior to summering in the BSS and the long half-life time of MeHg in other mammals (51 days in ringed seals,68 50–80 days in human populations101,102), the MeHg uptake in the Chukchi and Bering seas should account for a significant fraction of the MeHg burden in these beluga whales, even after they forage in Beaufort for 1–2 months prior to the harvest. We estimate that only 16 to 44% of MeHg in Beaufort beluga whales comes from their food uptake in the Beaufort Sea (Fig. 5), revealing the necessity of monitoring Hg contamination in other feeding grounds (i.e., Chukchi and Bering Seas) for further understanding the levels and trends of the Hg burden in Beaufort belugas.
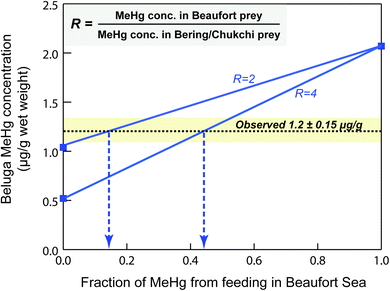 |
| Fig. 5 The fraction of MeHg in Beaufort beluga whales from feeding in the Beaufort Sea calculated based on different ratios (R) between the MeHg concentration of beluga diet in the Beaufort Sea and in the Bering Sea. The horizontal dashed line and the yellow shade indicate the observed beluga MeHg concentrations (mean ± standard error; wet weight based) across 2005–2012 from subsistence harvests in the BSS. The arrows point to the calculated fractions of MeHg in Beaufort beluga whales from foraging in the Beaufort Sea. | |
A range of tools can be applied to provide insight into the dietary composition of top predators, such as fatty acid signatures and stable isotope ratios.33,103,104 However, it is often difficult to assess the apportionment of the food or contaminant uptake across regions for highly migratory species foraging a wide range of prey at various geographic locations. Here we illustrate a method of estimating the MeHg contribution between two foraging grounds for Beaufort beluga using a combination of empirical data from field studies and ecosystem modeling that simulates bounding case MeHg concentrations (i.e. assuming full feeding in each location: one for the Beaufort Sea and one for the Bering Sea). This method is applicable to other migratory species for assessing their exposure sources of various bioaccumulative pollutants.
Like Beaufort beluga whales, Bering–Chukchi–Beaufort bowhead whales summering in the BSS region spend fall in the Chukchi Sea and winter in the Bering Sea.105 In contrast to beluga whales, the model-simulated concentration of bowhead whales (0.023 μg g−1) based on the assumption of feeding solely in the Beaufort Sea is very close to the literature value (0.020 μg g−1). The observed bowhead Hg data come from the subsistence hunt in Barrow,106 which occurs during both spring and fall as whales migrate between the Bering and Beaufort Seas.105,107 We postulate that the similarity between the literature value and model simulation is due to (1) the large fraction of samples from the fall hunt which captures the bowhead whales after feeding in the Beaufort Sea for the whole summer,107 and/or (2) a similar MeHg concentration in their diet (i.e., small to moderate sized crustaceans, such as euphausiids and copepods) between the Bering, Chukchi, and Beaufort Seas. We cannot exclude the possibility that the relatively small sample size of the observations (N = 33) may not reflect the average MeHg concentrations of this population. Additional information on MeHg concentrations of this bowhead stock, and crustaceans across Bering–Chukchi–Beaufort feeding grounds, will enable a better estimate of the MeHg contribution for bowhead whales across different Arctic regions.
4. Conclusions
Here we developed an ecosystem-based MeHg bioaccumulation model that has a detailed representation of the BSS trophodynamics, and relatively simple representation of MeHg toxicokinetics. The model is able to capture the highly efficient biomagnification in the BSS food web and simulated MeHg concentrations of most BSS functional groups are comparable to their respective published values. The results suggest that the heavy reliance on benthic organisms with higher levels of MeHg, compared to pelagic ones, leads to the high biomagnification efficiency in the BSS food web. Future development of the Ecotracer module to account for the methylation of inorganic Hg reservoirs in the benthic environment can improve the representation of food-web MeHg bioaccumulation in many shallow coastal ecosystems. Prior studies have not been able to attribute the observed temporal trend of beluga Hg burdens to individual anthropogenic, environmental, or ecological factors.22,39 Our model integrates environmental factors and food-web dynamics, thus providing a tool that can be further applied to holistically examine how ecological and environmental change drivers interact and which contributes the most to the observed temporal evolution of Hg concentrations in this beluga stock.
While incorporating the MeHg elimination rate in marine mammals is essential for simulating the MeHg burden in these animals, we find that the application of the experimentally derived MeHg elimination rate in fish, invertebrates, and plankton largely underestimates the MeHg concentrations in these groups. The results indicate that population turnover, rather than MeHg elimination, plays a dominant role in removing MeHg from populations and determines the population-wide MeHg concentration in each functional group. Our results suggest that the direct elimination of MeHg from fish and lower trophic level organisms is likely negligible at a population-level due to fast population turnover in the field and inefficient demethylation. This finding is consistent with the recent results from a whole-ecosystem experiment, and to the best of our knowledge, this study is the first explicit illustration of this effect using an ecosystem modeling approach.
At present, the interactions between climate change and Hg cycling in the Arctic is poorly understood, which limits our ability to assess climate change impacts on MeHg in the Arctic marine food web and any implications for human exposure.108 The results of the sensitivity analyses highlight that the seawater MeHg concentration and direct absorption rate of dissolved MeHg in benthos and plankton are among the most influential environmental and toxicokinetic factors driving the variability of MeHg concentrations in fish and marine mammals in the Beaufort Sea shelf ecosystem. However, these variables are also amongst the most poorly constrained. Further research to better quantify these important parameters, and their likely evolution with global environmental change (such as increased human activity, and global warming) will be critical for estimating future MeHg impacts in this sensitive ecosystem.
Conflicts of interest
All authors declared no conflicts of interest.
Acknowledgements
We gratefully acknowledge input and feedback from the Inuvialuit Game Council, and the contribution of beluga whale harvesters in the Inuvialuit Settlement Region to the long-standing biomonitoring project and published observational data from which was used in this study. We also thank all other investigators that contributed to the rich environmental and biotic monitoring data sets of the Beaufort Sea shelf. We thank Dr Juan Jose Alava for his helpful advice on Ecotracer parameterization. This project was funded by the Northern Contaminants Program of Canada (M-45; AG, ML, CH, LL), a Natural Sciences and Engineering Research Council of Canada Discovery Grant (RGPIN-2018-04893; AG, ML, EG), and a Natural Sciences and Engineering Research Council Canada Graduate Scholarship Master's level (to EG). C. Hoover and L. Loseto would like to acknowledge the Fisheries Joint Management Committee, Fisheries and Oceans Canada, Manitoba Centres of Excellence Fund, and ArcticNet for funding contributions to the Ecopath with Ecosim model.
References
- D. G. Streets, M. K. Devane, Z. Lu, T. C. Bond, E. M. Sunderland and D. J. Jacob, All-Time Releases of Mercury to the Atmosphere from Human Activities, Environ. Sci. Technol., 2011, 45(24), 10485–10491 CrossRef CAS PubMed.
- H. M. Amos, D. J. Jacob, D. G. Streets and E. M. Sunderland, Legacy Impacts of All-Time Anthropogenic Emissions on the Global Mercury Cycle, Global Biogeochem. Cycles, 2013, 27(2), 410–421 CrossRef CAS.
- K. Kidd, M. Clayden and T. Jardine, Bioaccumulation and Biomagnification of Mercury through Food Webs, Environ. Chem. Toxicol. Mercury, 2011, 453–499, DOI:10.1002/9781118146644.ch14.
- J. A. Fisher, D. J. Jacob, A. L. Soerensen, H. M. Amos, A. Steffen and E. M. Sunderland, Riverine Source of Arctic Ocean Mercury Inferred from Atmospheric Observations, Nat. Geosci., 2012, 5(7), 499–504, DOI:10.1038/ngeo1478.
- A. L. Soerensen, D. J. Jacob, A. T. Schartup, J. A. Fisher, I. Lehnherr, V. L. St Louis, L. E. Heimbürger, J. E. Sonke, D. P. Krabbenhoft and E. M. Sunderland, A Mass Budget for Mercury and Methylmercury in the Arctic Ocean, Global Biogeochem. Cycles, 2016, 30(4), 560–575, DOI:10.1002/2015GB005280.
- A. Dastoor, H. Angot, J. Bieser, J. H. Christensen, T. A. Douglas, L. E. Heimbürger-Boavida, M. Jiskra, R. P. Mason, D. S. McLagan, D. Obrist, P. M. Outridge, M. V. Petrova, A. Ryjkov, K. A. St. Pierre, A. T. Schartup, A. L. Soerensen, K. Toyota, O. Travnikov, S. J. Wilson and C. Zdanowicz, Arctic Mercury Cycling, Nat. Rev. Earth Environ., 2022, 3(4), 270–286, DOI:10.1038/s43017-022-00269-w.
-
AMAP, AMAP Assessment 2011: Mercury in the Arctic, Arct. Monit. Assess. Program. (AMAP), Oslo, Norw. 2011, p. 193 Search PubMed.
- J. L. Kirk, I. Lehnherr, M. Andersson, B. M. Braune, L. Chan, A. P. Dastoor, D. Durnford, A. L. Gleason, L. L. Loseto and A. Steffen, Mercury in Arctic Marine Ecosystems: Sources, Pathways, and Exposure, Environ. Res., 2012, 119, 64–87 CrossRef CAS PubMed.
- R. Dietz, P. M. Outridge and K. A. Hobson, Anthropogenic Contributions to Mercury Levels in Present-Day Arctic Animals—a Review, Sci. Total Environ., 2009, 407(24), 6120–6131 CrossRef CAS PubMed.
- J.-P. Desforges, P. Outridge, K. A. Hobson, M. P. Heide-Jørgensen and R. Dietz, Anthropogenic and Climatic Drivers of Long-Term Changes of Mercury and Feeding Ecology in Arctic Beluga (Delphinapterus Leucas) Populations, Environ. Sci. Technol., 2022, 56(1), 271–281, DOI:10.1021/ACS.EST.1C05389.
- A. Kendrick, Canadian Inuit Sustainable Use and Management of Arctic Species, Int. J. Environ. Stud., 2013, 70(3), 414–428 CrossRef.
- A. Médieu, D. Point, T. Itai, H. El Ene Angot, P. J. Buchanan, V. Erie Allain, L. Fuller, S. Griffiths, D. P. Gillikin, J. E. Sonke, L.-E. Heimb€ Urger-Boavida, M.-M. E. Desgranges, C. E. Menkes, D. J. Madigan, P. Brosset, O. Gauthier, A. Tagliabue, L. Bopp, A. Verheyden and A. Lorrain, Evidence That Pacific Tuna Mercury Levels Are Driven by Marine Methylmercury Production and Anthropogenic Inputs, Proc. Natl. Acad. Sci. U. S. A., 2022, 119(2), e2113032119, DOI:10.1073/PNAS.2113032119.
- A. T. Schartup, C. P. Thackray, A. Qureshi, C. Dassuncao, K. Gillespie, A. Hanke and E.
M. Sunderland, Climate Change and Overfishing Increase Neurotoxicant in Marine Predators, Nature, 2019, 572(7771), 648–650, DOI:10.1038/s41586-019-1468-9.
- C. S. Lee, M. E. Lutcavage, E. Chandler, D. J. Madigan, R. M. Cerrato and N. S. Fisher, Declining Mercury Concentrations in Bluefin Tuna Reflect Reduced Emissions to the North Atlantic Ocean, Environ. Sci. Technol., 2016, 50(23), 12825–12830, DOI:10.1021/ACS.EST.6B04328/SUPPL_FILE/ES6B04328_SI_001.PDF.
- I. Lehnherr, V. L. S. Louis, H. Hintelmann, J. L. Kirk, V. L. St Louis, H. Hintelmann and J. L. Kirk, Methylation of Inorganic Mercury in Polar Marine Waters, Nat. Geosci., 2011, 4(5), 298–302, DOI:10.1038/ngeo1134.
- Y. Zhang, A. L. Soerensen, A. T. Schartup and E. M. Sunderland, A Global Model for Methylmercury Formation and Uptake at the Base of Marine Food Webs, Global Biogeochem. Cycles, 2020, 34(2), e2019GB006348, DOI:10.1029/2019GB006348.
- M. C. Serreze and J. Stroeve, Arctic Sea Ice Trends, Variability and Implications for Seasonal Ice Forecasting, Philos. Trans. R. Soc., A, 2015, 373(2045), 20140159 CrossRef PubMed.
- J. Stroeve, M. Serreze, S. Drobot, S. Gearheard, M. Holland, J. Maslanik, W. Meier and T. Scambos, Arctic Sea Ice Extent Plummets in 2007, EOS, Trans., Am. Geophys. Union, 2008, 89(2), 13–14 CrossRef.
- J. E. Overland and M. Wang, When Will the Summer Arctic Be Nearly Sea Ice Free?, Geophys. Res. Lett., 2013, 40(10), 2097–2101 CrossRef.
- M. Trudel and J. B. Rasmussen, Bioenergetics and Mercury Dynamics in Fish: A Modelling Perspective, Can. J. Fish. Aquat. Sci., 2006, 63(8), 1890–1902, DOI:10.1139/F06-081.
- M. Fossheim, R. Primicerio, E. Johannesen, R. B. Ingvaldsen, M. M. Aschan and A. V. Dolgov, Recent Warming Leads to a Rapid Borealization of Fish Communities in the Arctic, Nat. Clim. Change, 2015, 5(7), 673–677, DOI:10.1038/nclimate2647.
-
A. Gaden and G. A. Stern, Temporal Trends in Beluga, Narwhal and Walrus Mercury Levels: Links to Climate Change, in A Little Less Arctic, Springer, 2010, pp. 197–216 Search PubMed.
- A. Gaden, S. H. Ferguson, L. Harwood, H. Melling and G. A. Stern, Mercury Trends in Ringed Seals (Phoca Hispida) from the Western Canadian Arctic since 1973: Associations with Length of Ice-Free Season, Environ. Sci. Technol., 2009, 43(10), 3646–3651 CrossRef CAS PubMed.
- M. A. Mckinney, S. Pedro, R. Dietz, C. Sonne, A. T. Fisk, D. Roy, B. M. Jenssen and R. J. Letcher, A Review of Ecological Impacts of Global Climate Change on Persistent Organic Pollutant and Mercury Pathways and Exposures in Arctic Marine Ecosystems, Curr. Zool., 2015, 61(4), 617–628, DOI:10.1093/CZOOLO/61.4.617.
- B. M. Braune, A. J. Gaston, K. A. Hobson, H. G. Gilchrist and M. L. Mallory, Changes in Food Web Structure Alter Trends of Mercury Uptake at Two Seabird Colonies in the Canadian Arctic, Environ. Sci. Technol., 2014, 48(22), 13246–13252, DOI:10.1021/ES5036249.
- D. J. Yurkowski, E. S. Richardson, N. J. Lunn, D. C. G. Muir, A. C. Johnson, A. E. Derocher, A. D. Ehrman, M. Houde, B. G. Young, C. D. Debets, L. Sciullo, G. W. Thiemann and S. H. Ferguson, Contrasting Temporal Patterns of Mercury, Niche Dynamics, and Body Fat Indices of Polar Bears and Ringed Seals in a Melting Icescape, Environ. Sci. Technol., 2020, 54(5), 2780–2789, DOI:10.1021/ACS.EST.9B06656/SUPPL_FILE/ES9B06656_SI_001.PDF.
- M. Houde, Z. E. Taranu, X. Wang, B. Young, P. Gagnon, S. H. Ferguson, M. Kwan and D. C. G. Muir, Mercury in Ringed Seals (Pusa Hispida) from the Canadian Arctic in Relation to Time and Climate Parameters, Environ. Toxicol. Chem., 2020, 39(12), 2462–2474, DOI:10.1002/ETC.4865.
- D. G. Buck, D. C. Evers, E. Adams, J. DiGangi, B. Beeler, J. Samánek, J. Petrlik, M. A. Turnquist, O. Speranskaya, K. Regan and S. Johnson, A Global-Scale Assessment of Fish Mercury Concentrations and the Identification of Biological Hotspots, Sci. Total Environ., 2019, 687, 956–966, DOI:10.1016/J.SCITOTENV.2019.06.159.
- C. A. Eagles-Smith, J. G. Wiener, C. S. Eckley, J. J. Willacker, D. C. Evers, M. Marvin-DiPasquale, D. Obrist, J. A. Fleck, G. R. Aiken, J. M. Lepak, A. K. Jackson, J. P. Webster, A. R. Stewart, J. A. Davis, C. N. Alpers and J. T. Ackerman, Mercury in Western North America: A Synthesis of Environmental Contamination, Fluxes, Bioaccumulation, and Risk to Fish and Wildlife, Sci. Total Environ., 2016, 568, 1213–1226, DOI:10.1016/J.SCITOTENV.2016.05.094.
- C. A. Eagles-Smith, E. K. Silbergeld, N. Basu, P. Bustamante, F. Diaz-Barriga, W. A. Hopkins, K. A. Kidd and J. F. Nyland, Modulators of Mercury Risk to Wildlife and Humans in the Context of Rapid Global Change, Ambio, 2018, 47(2), 170–197, DOI:10.1007/S13280-017-1011-X.
- G. K. Manson and S. M. Solomon, Past and Future Forcing of Beaufort Sea Coastal Change, Atmos.-Ocean, 2007, 45(2), 107–122, DOI:10.3137/ao.450204.
- K. R. Wood, J. E. Overland, S. A. Salo, N. A. Bond, W. J. Williams and X. Dong, Is There a “New Normal”Climate in the Beaufort Sea?, Polar Res., 2013, 32, 19552, DOI:10.3402/polar.v32i0.19552.
- L. L. Loseto, G. A. Stern, D. Deibel, T. L. Connelly, A. Prokopowicz, D. R. S. Lean, L. Fortier and S. H. Ferguson, Linking Mercury Exposure to Habitat and Feeding Behaviour in Beaufort Sea Beluga Whales, J. Mar. Syst., 2008, 74(3), 1012–1024 CrossRef.
-
C. M. Semmler, Sources, Cycling, and Fate of Arsenic and Mercury in the Coastal Beaufort Sea, Citeseer, Alaska, 2006 Search PubMed.
-
A. E. Burt, Mercury Uptake and Dynamics in Sea Ice Algae, Phytoplankton and Grazing Copepods from a Beaufort Sea Arctic Marine Food Web, University of Manitoba, Canada, 2012 Search PubMed.
- C. Pomerleau, G. A. Stern, M. Pućko, K. L. Foster, R. W. Macdonald and L. Fortier, Pan-Arctic Concentrations of Mercury and Stable Isotope Ratios of Carbon (Δ13C) and Nitrogen (Δ15N) in Marine Zooplankton, Sci. Total Environ., 2016, 551–552, 92–100, DOI:10.1016/J.SCITOTENV.2016.01.172.
- M. Pućko, A. Burt, W. Walkusz, F. Wang, R. W. Macdonald, S. Rysgaard, D. G. Barber, J. É. Tremblay and G. A. Stern, Transformation of Mercury at the Bottom of the Arctic Food Web: An Overlooked Puzzle in the Mercury Exposure Narrative, Environ. Sci. Technol., 2014, 48(13), 7280–7288, DOI:10.1021/ES404851B/SUPPL_FILE/ES404851B_SI_001.PDF.
- A. Loria, P. Archambault, A. Burt, A. Ehrman, C. Grant, M. Power and G. A. Stern, Mercury and Stable Isotope (Δ13C and Δ15N) Trends in Decapods of the Beaufort Sea, Polar Biol., 2020, 43(5), 443–456, DOI:10.1007/s00300-020-02646-x.
- L. L. Loseto, G. A. Stern and R. W. Macdonald, Distant Drivers or Local Signals: Where Do Mercury Trends in Western Arctic Belugas Originate?, Sci. Total Environ., 2015, 509, 226–236 CrossRef PubMed.
- C. Hoover, C. Giraldo, A. Ehrman, K. D. Suchy, S. A. MacPhee, J. D. Brewster, J. D. Reist, M. Power, H. Swanson and L. L. Loseto, The Canadian Beaufort Shelf Trophic Structure: Evaluating an Ecosystem Modelling Approach by Comparison with Observed Stable Isotopic Structure, Arct. Sci., 2021, 1–21, DOI:10.1139/as-2020-0035.
-
C. A. Hoover, W. Walkusz, S. MacPhee, A. Nieme, A. Majewski and L. Loweto, Canadian Beaufort Sea Shelf Food Web Structure and Changes from 1970-2012, 2021 Search PubMed.
- V. Christensen and C. J. Walters, Ecopath with Ecosim: Methods, Capabilities and Limitations, Ecol. Modell., 2004, 172(2–4), 109–139, DOI:10.1016/J.ECOLMODEL.2003.09.003.
- A. T. Schartup, A. Qureshi, C. Dassuncao, C. P. Thackray, G. Harding and E. M. Sunderland, A Model for Methylmercury Uptake and Trophic Transfer by Marine Plankton, Environ. Sci. Technol., 2018, 52(2), 654–662 CrossRef CAS PubMed.
- S. Booth and D. Zeller, Mercury, Food Webs, and Marine Mammals: Implications of Diet and Climate Change for Human Health, Environ. Health Perspect., 2005, 113(5), 521–526, DOI:10.1289/ehp.7603.
- W. J. Walters and V. Christensen, Ecotracer: Analyzing Concentration of Contaminants and Radioisotopes in an Aquatic Spatial-Dynamic Food Web Model, J. Environ. Radioact., 2018, 181, 118–127, DOI:10.1016/J.JENVRAD.2017.11.008.
- J. J. Alava, A. M. Cisneros-Montemayor, U. R. Sumaila and W. W. L. Cheung, Projected Amplification of Food Web Bioaccumulation of MeHg and PCBs under Climate Change in the Northeastern Pacific, Sci. Rep., 2018, 8(1), 13460 CrossRef PubMed.
- L. M. McGill, B. S. Gerig, D. T. Chaloner and G. A. Lamberti, An Ecosystem Model for Evaluating the Effects of Introduced Pacific Salmon on Contaminant Burdens of Stream-Resident Fish, Ecol. Modell., 2017, 355, 39–48, DOI:10.1016/J.ECOLMODEL.2017.03.027.
- L. H. Larsen, K. Sagerup and S. Ramsvatn, The Mussel Path – Using the Contaminant Tracer, Ecotracer, in Ecopath to Model the Spread of Pollutants in an Arctic Marine Food Web, Ecol. Modell., 2016, 331, 77–85, DOI:10.1016/J.ECOLMODEL.2015.10.011.
- J. Boyer, K. Rubalcava, S. Booth and H. Townsend, Proof-of-Concept Model for Exploring the Impacts of Microplastics Accumulation in the Maryland Coastal Bays Ecosystem, Ecol. Modell., 2022, 464, 109849, DOI:10.1016/J.ECOLMODEL.2021.109849.
- K. M. Tierney, J. J. Heymans, G. K. P. Muir, G. T. Cook, J. Buszowski, J. Steenbeek, W. J. Walters, V. Christensen, G. MacKinnon, J. A. Howe and S. Xu, Modelling Marine Trophic Transfer of Radiocarbon (14C) from a Nuclear Facility, Environ. Model. Software, 2018, 102, 138–154, DOI:10.1016/J.ENVSOFT.2018.01.013.
- S. Booth, W. J. Walters, J. Steenbeek, V. Christensen and S. Charmasson, An Ecopath with Ecosim Model for the Pacific Coast of Eastern Japan: Describing the Marine Environment and Its Fisheries Prior to the Great East Japan Earthquake, Ecol. Modell., 2020, 428, 109087, DOI:10.1016/J.ECOLMODEL.2020.109087.
- P. F. Schuster, R. G. Striegl, G. R. Aiken, D. P. Krabbenhoft, J. F. Dewild, K. Butler, B. Kamark and M. Dornblaser, Mercury Export from the Yukon River Basin and Potential Response to a Changing Climate, Environ. Sci. Technol., 2011, 45(21), 9262–9267, DOI:10.1021/es202068b.
- D. R. Leitch, J. Carrie, D. Lean, R. W. Macdonald, G. A. Stern and F. Wang, The Delivery of Mercury to the Beaufort Sea of the Arctic Ocean by the Mackenzie River, Sci. Total Environ., 2007, 373(1), 178–195 CrossRef CAS PubMed.
- A. T. Schartup, P. H. Balcom, A. L. Soerensen, K. J. Gosnell, R. S. D. Calder, R. P. Mason and E. M. Sunderland, Freshwater Discharges Drive High Levels of Methylmercury in Arctic Marine Biota, Proc. Natl. Acad. Sci. U. S. A., 2015, 112(38), 11789–11794 CrossRef CAS PubMed.
- R. P. Mason, J. R. Reinfelder and F. M. M. Morel, Uptake, Toxicity, and Trophic Transfer of Mercury in a Coastal Diatom, Environ. Sci. Technol., 1996, 30(6), 1835–1845 CrossRef CAS.
- M. Canli and R. W. Furness, Mercury and Cadmium Uptake from Seawater and from Food by the Norway Lobster Nephrops Norvegicus, Environ. Toxicol. Chem., 1995, 14(5), 819–828, DOI:10.1002/ETC.5620140512.
- K. Pan and W. X. Wang, Mercury Accumulation in Marine Bivalves: Influences of Biodynamics and Feeding Niche, Environ. Pollut., 2011, 159(10), 2500–2506, DOI:10.1016/J.ENVPOL.2011.06.029.
-
D. W. Rodgers, You Are What You Eat and a Little Bit More: Bioenergetics-Based Models of Methylmercury Accumulation in Fish Revisited, in Mercury Pollution: Integration and Synthesis, ed. C. Watras and J. Huckabee, Lewis Publications, Boca Raton, 1994, pp. 427–439 Search PubMed.
- B. D. Hall, R. A. Bodaly, R. J. P. Fudge, J. W. M. Rudd and D. M. Rosenberg, Food as the Dominant Pathway of Methylmercury Uptake by Fish, Water, Air, Soil Pollut., 1997, 100(1–2), 13–24, DOI:10.1023/A:1018071406537.
- L. E. Hrenchuk, P. J. Blanchfield, M. J. Paterson and H. H. Hintelmann, Dietary and Waterborne Mercury Accumulation by Yellow Perch: A Field Experiment, Environ. Sci. Technol., 2011, 46(1), 509–516, DOI:10.1021/ES202759Q.
- C. L. Osburn, L. Retamal and W. F. Vincent, Photoreactivity of Chromophoric Dissolved Organic Matter Transported by the Mackenzie River to the Beaufort Sea, Mar. Chem., 2009, 115(1–2), 10–20, DOI:10.1016/j.marchem.2009.05.003.
- J. K. Petersen, M. K. Sejr and J. E. N. Larsen, Clearance Rates in the Arctic Bivalves Hiatella Arctica and Mya Sp, Polar Biol., 2003, 26(5), 334–341, DOI:10.1007/s00300-003-0483-2.
- M. T. K. Tsui and W. X. Wang, Temperature Influences on the Accumulation and Elimination of Mercury in a Freshwater Cladoceran, Daphnia Magna, Aquat. Toxicol., 2004, 70(3), 245–256, DOI:10.1016/j.aquatox.2004.09.006.
- E. Nakazawa, T. Ikemoto, A. Hokura, Y. Terada, T. Kunito, S. Tanabe and I. Nakai, The Presence of Mercury Selenide in Various Tissues of the Striped Dolphin: Evidence from Mu-XRF-XRD and XAFS Analyses, Metallomics, 2011, 3(7), 719–725, 10.1039/c0mt00106f.
- F. E. Huggins, S. A. Raverty, O. S. Nielsen, N. E. Sharp, J. D. Robertson and N. V. C. Ralston, An XAFS Investigation of Mercury and Selenium in Beluga Whale Tissues, Environ. Bioindic., 2009, 4(4), 291–302 CrossRef CAS.
- Z. Gajdosechova, M. M. Lawan, D. S. Urgast, A. Raab, K. G. Scheckel, E. Lombi, P. M. Kopittke, K. Loeschner, E. H. Larsen and G. Woods,
In Vivo Formation of Natural HgSe Nanoparticles in the Liver and Brain of Pilot Whales, Sci. Rep., 2016, 6, 34361 CrossRef CAS PubMed.
- M. Li, C. A. Juang, J. D. Ewald, R. Yin, B. Mikkelsen, D. P. Krabbenhoft, P. H. Balcom, C. Dassuncao and E. M. Sunderland, Selenium and Stable Mercury Isotopes Provide New Insights into Mercury Toxicokinetics in Pilot Whales, Sci. Total Environ., 2020, 710, 136325, DOI:10.1016/j.scitotenv.2019.136325.
- J. D. Ewald, J. L. Kirk, M. Li and E. M. Sunderland, Organ-Specific Differences in Mercury Speciation and Accumulation across Ringed Seal (Phoca Hispida) Life Stages, Sci. Total Environ., 2019, 650, 2013–2020, DOI:10.1016/j.scitotenv.2018.09.299.
- E. Bolea-Fernandez, A. Rua-Ibarz, E. M. Krupp, J. Feldmann and F. Vanhaecke, High-Precision Isotopic Analysis Sheds New Light on Mercury Metabolism in Long-Finned Pilot Whales (Globicephala Melas), Sci. Rep., 2019, 9(1), 1–10, DOI:10.1038/s41598-019-43825-z.
- K. Borgå, K. A. Kidd, D. C. G. Muir, O. Berglund, J. M. Conder, F. A. P. C. Gobas, J. Kucklick, O. Malm and D. E. Powell, Trophic Magnification Factors: Considerations of Ecology, Ecosystems, and Study Design, Integr. Environ. Assess. Manage., 2012, 8(1), 64–84, DOI:10.1002/ieam.244.
- B. C. Kelly, M. G. Ikonomou, J. D. Blair, B. Surridge, D. Hoover, R. Grace and F. A. P. C. Gobas, Perfluoroalkyl Contaminants in an Arctic Marine Food Web: Trophic Magnification and Wildlife Exposure, Environ. Sci. Technol., 2009, 43(11), 4037–4043, DOI:10.1021/es9003894.
- C. R. Hammerschmidt, W. F. Fitzgerald, C. H. Lamborg, P. H. Balcom and P. T. Visscher, Biogeochemistry of Methylmercury in Sediments of Long Island Sound, Mar. Chem., 2004, 90, 31–52, DOI:10.1016/j.marchem.2004.02.024.
- C. C. Gilmour and G. S. Riedel, Measurement of Hg Methylation in Sediments Using High Specific-Activity203Hg and Ambient Incubation, Water, Air, Soil Pollut., 1995, 80(1–4), 747–756, DOI:10.1007/BF01189726.
- E. M. Sunderland, F. A. P. C. Gobas, B. A. Branfireun and A. Heyes, Environmental Controls on the Speciation and Distribution of Mercury in Coastal Sediments, Mar. Chem., 2006, 102(1–2), 111–123, DOI:10.1016/J.MARCHEM.2005.09.019.
- S. Jung, S. Y. Kwon, M.-L. Li, R. Yin and J. Park, Elucidating Sources of Mercury in the West Coast of Korea and the Chinese Marginal Seas Using Mercury Stable Isotopes, Sci. Total Environ., 2021, 152598, DOI:10.1016/J.SCITOTENV.2021.152598.
- S. Y. Kwon, J. D. Blum, C. Y. Chen, D. E. Meattey and R. P. Mason, Mercury Isotope Study of Sources and Exposure Pathways of Methylmercury in Estuarine Food Webs in the Northeastern US, Environ. Sci. Technol., 2014, 48(17), 10089–10097 CrossRef CAS PubMed.
- G. E. Gehrke, J. D. Blum, D. G. Slotton and B. K. Greenfield, Mercury Isotopes Link Mercury in San Francisco Bay Forage Fish to Surface Sediments, Environ. Sci. Technol., 2011, 45(4), 1264–1270 CrossRef CAS PubMed.
- J. L. Kirk, V. L. St. Louis, H. Hintelmann, I. Lehnherr, B. Else and L. Poissant, Methylated Mercury Species in Marine Waters of the Canadian High and Sub Arctic, Environ. Sci. Technol., 2008, 42(22), 8367–8373 CrossRef CAS PubMed.
- K. A. Merritt and A. Amirbahman, Mercury Methylation Dynamics in Estuarine and Coastal Marine Environments — A Critical Review, Earth-Sci. Rev., 2009, 96(1–2), 54–66, DOI:10.1016/J.EARSCIREV.2009.06.002.
- J. D. Blum, B. N. Popp, J. C. Drazen, C. Anela Choy, M. W. Johnson, C. A. Choy and M. W. Johnson, Methylmercury Production below the Mixed Layer in the North Pacific Ocean, Nat. Geosci., 2013, 6(10), 879–884, DOI:10.1038/ngeo1918.
- D. J. Madigan, M. Li, R. Yin, H. Baumann, O. E. Snodgrass, H. Dewar, D. P. Krabbenhoft, Z. Baumann, N. S. Fisher, P. Balcom and E. M. Sunderland, Mercury Stable Isotopes Reveal Influence of Foraging Depth on Mercury Concentrations and Growth in Pacific Bluefin Tuna, Environ. Sci. Technol., 2018, 52(11), 6256–6264, DOI:10.1021/acs.est.7b06429.
- E. M. Sunderland, D. P. Krabbenhoft, J. W. Moreau, S. A. Strode and W. M. Landing, Mercury Sources, Distribution, and Bioavailability in the North Pacific Ocean: Insights from Data and Models, Global Biogeochem. Cycles, 2009, 23(2), GB2010, DOI:10.1029/2008GB003425.
- S. Jonsson, U. Skyllberg, M. B. Nilsson, E. Lundberg, A. Andersson and E. Björn, Differentiated Availability of Geochemical Mercury Pools Controls Methylmercury Levels in Estuarine Sediment and Biota, Nat. Commun., 2014, 5(1), 1–10, DOI:10.1038/ncomms5624.
- R. W. Macdonald and L. L. Loseto, Are Arctic Ocean Ecosystems Exceptionally Vulnerable to Global Emissions of Mercury? A Call for Emphasised Research on Methylation and the Consequences of Climate Change, Environ. Chem., 2010, 7(2), 133–138 CrossRef CAS.
- K. R. N. Florko, G. W. Thiemann and J. F. Bromaghin, Drivers and Consequences of Apex Predator Diet Composition in the Canadian Beaufort Sea, Oecologia, 2020, 194(1–2), 51–63, DOI:10.1007/S00442-020-04747-0/FIGURES/7.
- L.-A. Dehn, G. G. Sheffield, E. H. Follmann, L. K. Duffy, D. L. Thomas, G. R. Bratton, R. J. Taylor and T. M. O’hara, Trace Elements in Tissues of Phocid Seals Harvested in the Alaskan and Canadian Arctic: Influence of Age and Feeding Ecology, cdnsciencepub.com, 2005, 83(5), 726–746, DOI:10.1139/Z05-053.
- B. G. Young, L. L. Loseto and S. H. Ferguson, Diet Differences among Age Classes of Arctic Seals: Evidence from Stable Isotope and Mercury Biomarkers, Polar Biol., 2010, 33(2), 153–162, DOI:10.1007/S00300-009-0693-3/TABLES/4.
- R. A. Lavoie, T. D. Jardine, M. M. Chumchal, K. A. Kidd and L. M. Campbell, Biomagnification of Mercury in Aquatic Food Webs: A Worldwide Meta-Analysis, Environ. Sci. Technol., 2013, 47(23), 13385–13394 CrossRef CAS PubMed.
- G. Chiang, K. A. Kidd, M. Díaz-Jaramillo, W. Espejo, P. Bahamonde, N. J. O'Driscoll and K. R. Munkittrick, Methylmercury Biomagnification in Coastal Aquatic Food Webs from Western Patagonia and Western Antarctic Peninsula, Chemosphere, 2021, 262, 128360, DOI:10.1016/J.CHEMOSPHERE.2020.128360.
- B. E. Ferriss and T. E. Essington, Can Fish Consumption Rate Estimates Be Improved by Linking Bioenergetics and Mercury Mass Balance Models? Application to Tunas, Ecol. Modell., 2014, 272, 232–241, DOI:10.1016/J.ECOLMODEL.2013.10.010.
- M. Trudel and J. B. Rasmussen, Modeling the Elimination of Mercury by Fish, Environ. Sci. Technol., 1997, 31(6), 1716–1722 CrossRef CAS.
- C. P. Madenjian, S. R. Chipps and P. J. Blanchfield, Time to Refine Mercury Mass Balance Models for Fish, Facets, 2021, 6(1), 272–286, DOI:10.1139/FACETS-2020-0034.
- J. A. Arnot and F. A. P. C. Gobas, A Food Web Bioaccumulation Model for Organic Chemicals in Aquatic Ecosystems, Environ. Toxicol. Chem., 2004, 23(10), 2343–2355 CrossRef CAS PubMed.
- P. J. Blanchfield, J. W. M. Rudd, L. E. Hrenchuk, M. Amyot, C. L. Babiarz, K. G. Beaty, R. A. D. Bodaly, B. A. Branfireun, C. C. Gilmour, J. A. Graydon, B. D. Hall, R. C. Harris, A. Heyes, H. Hintelmann, J. P. Hurley, C. A. Kelly, D. P. Krabbenhoft, S. E. Lindberg, R. P. Mason, M. J. Paterson, C. L. Podemski, K. A. Sandilands, G. R. Southworth, V. L. St Louis, L. S. Tate and M. T. Tate, Experimental Evidence for Recovery of Mercury-Contaminated Fish Populations, Nature, 2021, 601(7891), 74–78, DOI:10.1038/s41586-021-04222-7.
- D. D. W. Hauser, K. L. Laidre, R. S. Suydam and P. R. Richard, Population-Specific Home Ranges and Migration Timing of Pacific Arctic Beluga Whales (Delphinapterus Leucas), Polar Biol., 2014, 37(8), 1171–1183, DOI:10.1007/s00300-014-1510-1.
- L. Storrie, N. E. Hussey, S. A. MacPhee, G. O'Corry-Crowe, J. Iacozza, D. G. Barber, A. Nunes and L. L. Loseto, Year-Round Dive Characteristics of Male Beluga Whales From the Eastern Beaufort Sea Population Indicate Seasonal Shifts in Foraging Strategies, Front. Mar. Sci., 2022, 8, 1–22, DOI:10.3389/fmars.2021.715412.
- A. M. Agather, K. L. Bowman, C. H. Lamborg and C. R. Hammerschmidt, Distribution of Mercury Species in the Western Arctic Ocean (U.S. GEOTRACES GN01), Mar. Chem., 2019, 216, 103686, DOI:10.1016/j.marchem.2019.103686.
-
Alaska Department of Environmental Conservation, Total Mercury in Fish and Shellfish Caught in Alaskan Waters, 2021 Search PubMed.
- L. T. Quakenbush, R. S. Suydam, A. L. Bryan, L. F. Lowry, K. J. Frost and B. A. Mahoney, Diet of Beluga Whales, Delphinapterus Leucas, in Alaska from Stomach Contents, March-November, Mar. Fish. Rev., 2015, 77(1), 70–84, DOI:10.7755/MFR.77.1.7.
-
L. Harwood, M. Kingsley and F. Pokiak, Monitoring Beluga Harvests in the Mackenzie Delta and Near Paulatuk, NT, Canada: Harvest Efficiency and Trend, Size and Sex of Landed Whales, and Reproduction, 1970-2009; 2015. https://doi.org/ DOI:10.13140/RG.2.1.2133.4644.
- S. Jo, H. D. Woo, H.-J. J. Kwon, S.-Y. Y. Oh, J.-D. D. Park, Y.-S. S. Hong, H. Pyo, K. S. Park, M. Ha, H. Kim, S. J. Sohn, Y. M. Kim, J. A. Lim, S. A. Lee, S. Y. Eom, B. G. Kim, K. M. Lee, J. H. Lee, M. S. Hwang and J. Kim, Estimation of the Biological Half-Life of Methylmercury Using a Population Toxicokinetic Model, Int. J. Environ. Res. Public Health, 2015, 12(8), 9054–9067, DOI:10.3390/IJERPH120809054.
-
CDC, Biomonitoring Summary, CDC National Biomonitoring Program, 2017 Search PubMed.
- E. Choy, C. Giraldo, B. Rosenberg, J. Roth, A. Ehrman, A. Majewski, H. Swanson, M. Power, J. Reist and L. Loseto, Variation in the Diet of Beluga Whales in Response to Changes in Prey Availability: Insights on Changes in the Beaufort Sea Ecosystem, Mar. Ecol.: Prog. Ser., 2020, 647, 195–210, DOI:10.3354/meps13413.
- A. Ehrman, C. Hoover, C. Giraldo, S. A. MacPhee, J. Brewster, C. Michel, J. D. Reist, M. Power, H. Swanson, A. Niemi, W. Walkusz and L. Loseto, A Meta-Collection of Nitrogen Stable Isotope Data Measured in Arctic Marine Organisms from the Canadian Beaufort Sea, 1983–2013, BMC Res. Notes, 2021, 14(1), 1–3, DOI:10.1186/s13104-021-05743-0.
-
The North Slope Borough, Bowhead Whale Subsistence Harvest Research, https://www.north-slope.org/departments/wildlife-management/studies-and-research-projects/bowhead-whales/bowhead-whale-subsistence-harvest-research#pubs, accessed Jan 8, 2022 Search PubMed.
- T. M. O'Hara, C. Hanns, G. Bratton, R. Taylor and V. M. Woshner, Essential and Non-Essential Elements in Eight Tissue Types from Subsistence-Hunted Bowhead Whale: Nutritional and Toxicological Assessment, Int. J. Circumpolar Health, 2006, 65(3), 228–242, DOI:10.3402/IJCH.V65I3.18108.
-
R. S. Suydam and J. C. George, Subsistence Harvest of Bowhead Whales (Balaena Mysticetus) by Alaskan Eskimos, 1974 to 2003, 2004 Search PubMed.
- K. Sundseth, J. M. Pacyna, A. Banel, E. G. Pacyna and A. Rautio, Climate Change Impacts on Environmental and Human Exposure to Mercury in the Arctic, Int. J. Environ. Res. Public Health, 2015, 12(4), 3579–3599, DOI:10.3390/IJERPH120403579.
|
This journal is © The Royal Society of Chemistry 2022 |
Click here to see how this site uses Cookies. View our privacy policy here.