The influence of hardness at varying pH on zinc toxicity and lability to a freshwater microalga, Chlorella sp.†
Received
16th February 2022
, Accepted 10th April 2022
First published on 12th April 2022
Abstract
Zinc is an essential element for aquatic organisms, however, activities such as mining and refining, as well as zinc's ubiquitous role in modern society can contribute to elevated environmental concentrations of zinc. Water hardness is widely accepted as an important toxicity modifying factor for metals in aquatic systems, though other factors such as pH are also important. This study investigated the influence of increasing water hardness, at three different pH values (6.7, 7.6 and 8.3), on the chronic toxicity of zinc to the growth rate of a microalgae, Chlorella sp. Zinc toxicity decreased with increasing hardness from 5 to 93 mg CaCO3 L−1 at all three pH values tested. The 72 h growth rate inhibition EC50 values ranged from 6.2 μg Zn L−1 (at 5 mg CaCO3 L−1, pH 8.3) to 184 μg Zn L−1 (at 92 mg CaCO3 L−1, pH 6.7). Increases in hardness from 93 to 402 mg CaCO3 L−1 generally resulted in no significant (p > 0.05) reduction in zinc toxicity. DGT-labile zinc measurements did not correspond with the observed changes in zinc toxicity as hardness was varied within a pH treatment. This suggests that cationic competition from increased hardness is decreasing zinc toxicity, rather than changes in metal lability. This study highlighted that current hardness algorithms used in water quality guidelines may not be sufficiently protective of sensitive species, such as Chlorella sp., in high hardness waters.
Environmental significance
The current Australian and New Zealand freshwater guideline values for zinc have a hardness correction algorithm which allows guidelines to be modified depending on the water hardness of the ecosystem. The algorithm was developed largely from North American fish toxicity data not controlled for constant alkalinity and pH which may not be appropriate for protecting more sensitive species, such as microalgae. In this work, we apply microalgae toxicity tests to demonstrate that such algorithms may not be protective across environmentally relevant hardness ranges.
|
Introduction
Metal toxicity to an organism is directly related to the bioavailability of that metal to the organism. Bioavailability is significantly influenced by in situ water chemistry, such as pH, hardness (measured as Ca and Mg), alkalinity, and dissolved organic matter (DOM).1 This occurs via several mechanisms, including: metal speciation changes (e.g. pH and alkalinity), cation competition for binding sites at the biotic ligand (e.g. pH and hardness) and metal complexation to organic ligands (e.g. DOM).2
Increased understanding of the importance of metal bioavailability in aquatic environments has led to the development of methods that measure different metal fractions using kinetic approaches.3 The diffusive gradients in thin films (DGT) sampling technique uses diffusion-based sampling and provides in situ kinetic measurements of the average labile metal concentration across the time of deployment.4 A chelating resin that selectively binds cations is overlaid by a diffusive gel and a 0.45 μm filter membrane that restrict mass transport based on molecular diffusion.3 In contrast, bioavailability models (such as the biotic ligand model) assume a thermodynamic equilibrium exists in bulk solution, which is not always the case.1 Here, kinetic approaches (as measured by DGT) may be more useful. DGT measurements can be used to check if all dissolved metal is labile; this can be of particular importance in toxicity tests using algae, which, when present at high cell density, can release exudates that complex metal and greatly reduce lability and hence bioavailability.5 DGT measurements can be compared to speciation estimates (determined with the Windermere Humic Aqueous Model [WHAMVII]), to help explain changes in toxicity under various water chemistry conditions.
The concepts of bioavailability and lability are similar, so it can be hypothesized that measures of lability will be correlated to observable changes in bioavailability and toxicity over varying water chemistry conditions. However, the relationship between DGT lability and metal toxicity under varying water chemistry is not well established. Macoustra et al. found that DGT-labile copper concentrations were correlated to microalgae toxicity with changing DOM concentration.6 Price et al. assessed the influence of pH on the relationship between DGT-labile zinc and microalgal toxicity finding lability was not correlated to toxicity with changing pH.7 Paller et al. found hardness had a small influence on copper and zinc lability, relative to the influence of DOM.8 These studies suggested that DGT measurements combined with bioavailability models may be useful as a tool for assessing metal toxicity over a range of water chemistries. However, there is still limited information on how DGT measurements can be directly linked to observed toxicity.
Numerous empirical and mechanistic models have been developed to explain relationships between toxicity and water chemistry, including hardness algorithms,9 multiple linear regressions (MLRs)10,11 and biotic ligand models (BLMs).1,12 These have subsequently been used to develop water quality guidelines. The US EPA first incorporated a bioavailability term through a hardness-dependent algorithm.9 This algorithm was then incorporated into other water quality regulatory frameworks, such as the Australian and New Zealand water quality guidelines.13 Since the development of these hardness algorithms, the role of other toxicity modifying factors (TMF) (pH, major ions, dissolved organic carbon [DOC]) have been further investigated.2 In the case of copper, rigorous testing of the dependence of copper toxicity on hardness highlighted that the hardness algorithms were not appropriate, resulting in removal of the hardness algorithm for copper guideline values in Australia and New Zealand.14,15 Markich et al. explained that a key reason for the discrepancies between the original results used to derive the copper hardness-algorithms and the more recent studies disputing the algorithm, was the covariation of hardness with alkalinity and pH.14 Hardness and dissolved inorganic carbon (DIC) (as measured by alkalinity and pH) influence metal bioavailability in different ways. Hardness affects bioavailability through calcium and magnesium competition at the biotic ligand, while DIC affects metal speciation in solution through complexation with carbonates, so it is important to separate these effects and assess them individually.14
Such rigorous assessment of the hardness algorithms has not occurred for other metals, such as zinc. The current Australian and New Zealand water quality guideline values for zinc use the original 1985 hardness-dependent algorithm, as shown in eqn (1).16
|  | (1) |
where GV is the guideline value in μg L
−1 normalised at a hardness of 30 mg CaCO
3 L
−1, and
H is the measured hardness (mg CaCO
3 L
−1).
17
This zinc hardness algorithm was derived largely from North American fish acute toxicity data and was not verified for freshwater microalgae due to the lack of high-quality data. Consequently, it is uncertain if the algorithm is protective of zinc toxicity to microalgae. Despite hardness being one of the most widely studied TMFs, there are limited studies on the effect of true water hardness (decoupled from alkalinity and pH) on zinc toxicity to freshwater microalgae and of those studies, most have been done on the green freshwater microalga Raphidocelis subcapitata.18–20
This study therefore had three main objectives: (1) to assess the influence of increasing hardness, at three different pH values, on the toxicity of zinc to a tropical freshwater microalga Chlorella sp. using environmentally relevant hardness values (5 to 400 mg CaCO3 L−1) and pH range (6.7 to 8.3); (2) to determine whether DGT-labile zinc measurements correlate with zinc toxicity at different water hardness; and (3) to compare the influence of hardness on zinc toxicity against the current hardness algorithms applied to the US and Australian and New Zealand freshwater guidelines to assess the protectiveness of the algorithm to microalgae.
Methods
General laboratory techniques and reagents
All general glassware and plasticware were cleaned in a dishwasher (Smeg GW4060, Gallay Scientific) using a detergent rinse cycle (Gallay clean A powder detergent, Gallay scientific), acid rinse cycle (2% HNO3, Merck), and finished with an ultrapure water (UPW) rinse cycle (18 MΩ cm, Milli-Q®, Millipore). All glassware and polypropylene sample vials and lids (Technoplas) used in testing and analysis were soaked (>24 h) in 10% (v/v) HNO3 (Merck) and thoroughly rinsed with UPW before testing.
Organism cultures
Algal growth inhibition bioassays were conducted using the tropical freshwater green microalga, Chlorella sp. isolated from Lake Aesake, Strickland River, Papua New Guinea.21 Algae were cultured in Jaworski's medium at 2/5 strength at 27 ± 1 °C on a 12
:
12 light/dark cycle (75 μmol photons m−2 s−1).22 Algae were transferred weekly into new media, and 5 to 7 day old cultures were used for test initiation to ensure continual exponential growth throughout the testing period.
Toxicity testing
All toxicity tests were conducted using modified synthetic water based on the USEPA recipe and the test protocol is based on Organisation for Economic Co-operation and Development test guideline 201 with modifications described in Franklin et al.23–25 To ensure that only true water hardness was varied and carbonate/bicarbonate alkalinity remained constant across all test solutions, NaHCO3 and KCl salts were kept constant at 96 mg L−1 and 4 mg L−1, respectively, based on the ‘moderately hard’ (90 mg CaCO3 L−1) recipe.23 Concentrations of CaSO4·2H2O and MgSO4·7H2O were modified to prepare water of varying hardness (nominally 5–400 mg CaCO3 L−1) and kept at a constant ratio (2
:
1) for all treatments. Concentrations of salts used to prepare test solutions are presented in the supplementary information Table S1.† All test solutions were adjusted to the required pH with dilute HCl or KOH, with pH being maintained using MOPS (3-N-morpholinopropanesulfonic acid) buffer (free acid form, Merck) to give a final MOPS concentration of 0.5 g L−1 (2.4 mM) in each treatment. MOPS has previously been shown to be non-toxic to microalgae and to not influence toxicity at 0.5 g L−1.7,26
The 72 h growth inhibition bioassays were conducted in 250 mL conical flasks coated in a silanizing solution (Coatasil, Thermofisher Scientific) with 75 mL of test solution. Each flask was spiked with 75 μL of 1.5 g NO3− L−1 (NaNO3) and 0.15 g PO43− L−1 (KH2PO4) stocks giving final concentrations of 1.5 mg NO3− L−1 (NaNO3) and 0.15 mg PO43− L−1 (KH2PO4) to sustain exponential growth over the 72 h test. Stock solutions (5, 20 and 1000 mg L−1) of zinc were prepared using analytical grade zinc chloride (Sigma-Aldrich) and appropriate volumes were spiked into test flasks. Zinc concentration series (of at least 10 concentrations and controls (in triplicate) ranging from 0–5000 μg Zn L−1) were tested at four different hardness concentrations (5, 31, 93 and 402 mg CaCO3 L−1) and at three different pH values (pH 6.7, 7.6 and 8.3) at each hardness. This pH and hardness ranges were chosen as it covers the 10th–90th percentile range of Australian and New Zealand natural water values27 and allows all tests to be conducted using a single buffering agent. EC50 values at 93 mg CaCO3 L−1 hardness were taken from Price et al. and included in the analysis of the present study.7
A 25 mL aliquot of test solution was taken from each flask for chemical analyses prior to algal inoculation but after a 24 h pre-equilibration period at the test conditions. Algal cells in culture media were centrifuged (1048 g, 7 min, rotor radius 15 cm; Spintron GT-175BR, 25 ± 1 °C) and washed with test solution. Centrifugation and washing were repeated three times to ensure removal of culture medium. Algal concentrate was spiked into test flasks to give a final cell density of 2–4 × 103 cells per mL.5 Tests were conducted in incubator cabinets (LABEC) under constant conditions 27 ± 1 °C, 12
:
12 photoperiod, and light intensity of 140 ± 20 μmol photons m−2 s−1 for 72 h. Tests of all pH and hardness treatments were repeated at least once to account for inter-test variability.
Algal cell densities in test solutions were determined using flow cytometry (FACSVerse, BD Biosciences) at 0, 24, 48 and 72 h. Cell densities were obtained by plotting side angle light scatter (SSC) versus chlorophyll a fluorescence intensity (FLB3) with manual gating. A threshold of 200 (arbitrary units) was set to exclude background noise from non-algal particles and gating allowed for the exclusion of dead cells as described in detail by Stone et al.28 Cell densities were used to calculate population growth rates.21 Population growth rates were normalised as a percentage of the test's control response to account for inter-test variability and allow data to be pooled.
A copper reference toxicant test was run concurrently with each hardness series test. Tests were conducted in ‘moderately hard’ modified synthetic water (90 mg CaCO3 L−1, pH adjusted to 7.5).23 The test was considered acceptable if algal growth rates in reference toxicant tests were 1.7 ± 0.4 doublings per day (mean ± standard deviation [SD], n = 20) and the copper reference median effect concentration (EC50) was within internal database limits of 2.6 ± 1.1 μg Cu L−1 (mean ± SD, n = 20). Additionally, controls in the hardness series tests needed to have a growth factor of at least 16 (specific growth rate of 0.92 doublings per day) within the 72 h test period.24 Test pH variability was required to be within ±0.1 pH unit of the average test pH for the 72 h test.
Chemical analyses
Subsamples (<0.45 μm) were collected from each test flask at the start (0 h) and end (72 h) of each test for dissolved metal analysis. Subsamples were filtered through acid-rinsed (flushed with 30 mL of both 10% HNO3 and ultrapure water) 0.45 μm syringe filters (polyethersulfone membrane, Sartorius). All metal samples were acidified to 0.2% (v/v) HNO3 (Tracepur, Merck) and stored below 4 °C until analysis. Metals samples were analysed by inductively coupled plasma-atomic emission spectrometry (ICP-AES, Agilent 730ES) with a minimum instrument detection limit of 0.16 μg Zn L−1. Matrix-matched calibration standards, blanks and drift-standards were used for quality assurance.
Samples were collected from the bulk test solution for DOC and alkalinity analysis prior to the addition of MOPS. Test solutions were filtered through acid-rinsed (flushed with 60 mL of both 10% HNO3 and ultrapure water) 0.45 μm filters (polyethersulfone, Sartorius), DOC samples were acidified with concentrated sulfuric acid (H2SO4) in glass amber vials and alkalinity samples were collected in high-density polyethylene containers and stored below 4 °C until analysis. DOC was determined by the non-purgeable organic carbon method (TOC-L series, Shimadzu) and alkalinity was determined using automated titration.
Physicochemical measurements including conductivity (model 30/10 FT, YSI) and dissolved oxygen (Oximeter 330, WTW) were made from each treatment at the start and end of each test, and measurements for pH (probe ROSS 815600, Thermo Fischer) were collected every 24 h throughout the test. All instruments were calibrated before use in accordance with manufacturer instructions.
Zinc speciation and lability
Two methods were used to investigate zinc speciation and lability: the Windermere Humic Acid Model (WHAM VII) and the diffusive gradients in thin-films (DGT) technique.
WHAM was used to estimate zinc speciation in each test solution. Input parameters included dissolved Zn, pH, temperature, major ions (Mg2+, Ca2+, K+, Na+, Cl−, SO42−, CO32−, NO3− and PO43−), and DOC. An open atmosphere assumption (pCO2 = 0.00038 atm) was applied to all speciation calculations as per DeForest and Van Genderen.29 Nominal concentrations for major anions and cations (except Mg2+ and Ca2+) were used (input data provided in Table S2†).
DGT-labile zinc was measured in six zinc concentrations between 0 and 400 μg L−1 at hardness concentrations of 31 and 402 mg CaCO3 L−1, with DGT-labile data for 93 mg CaCO3 L−1 taken from Price et al.7 The Chelex-100-based chelating resin (Na form, 100–200 wet mesh) and polyacrylamide diffusive gel were synthesized and assembled into DGT pistons following procedures by Amato et al.30 DGT pistons were deployed in acid-washed polycarbonate containers with test solution and inoculated with algal cell densities equivalent to initial densities used in the toxicity tests. DGT samplers were deployed in separate vessels to the toxicity tests as they did not fit inside a 250 mL conical flask. Deployed DGT samplers were placed on an orbital shaker (90–100 rpm) to ensure that the diffusive boundary layer was negligible. The orbital shaker was placed in an incubator cabinet (LABEC) under the same conditions as the toxicity tests. DGT samplers were retrieved following 72 h deployment, binding gels were eluted in 1 M HNO3 for >24 h, and then diluted 10-fold for ICP-AES analysis. DGT-labile zinc concentrations were calculated in accordance with equations detailed by Zhang and Davison.31 DGT experiments were only conducted at pH 6.7, as previous studies had shown that pH within the tested range (of 6.7 to 8.3) did not significantly influence DGT-labile zinc concentrations.7
Statistical analysis and modelling
Statistical analyses were performed using the R studio environment (version 4.0.2, R Core Team 2016) with the extension package drc.32 Figures were produced using the extension packages ggplot2 and ggpubr.33,34 Growth rate inhibition normalised to a percent of the respective control growth rate of that test was used as the response variable to derive all toxicity estimates. Effect concentrations for 10, 20 and 50 percent growth inhibition relative to controls (EC10, EC20 and EC50) were calculated using 4-parameter log-logistic or Weibull models. Akaike's information criterion (AIC) and model residual standard error were used for model selection via the mselect function within drc (model parameters are listed in ESI, Table S3†). As response data were normalised to a percentage of control, all models had upper limit parameters fixed to 100. When full effect responses (i.e. EC100) were observed, the lower limit parameter was fixed to 0, meaning only two parameters were estimated (the slope and inflection). When this was not the case (i.e., lower asymptote >0), the lower asymptote was not fixed.35 Note in scenarios where the lower asymptote was >0, the ED function in drc will calculate effect concentrations between the upper and lower limits of the model (i.e., an EC50 will represent the mid-point between upper and lower asymptotes rather than the 50% response relative to controls at 100% response). To ensure that EC values were based on response relative to controls, re-scaling of the input values in the ED function was required.
Ratio tests via the comped function within drc were used for significance testing of EC values among tests.36 Stepwise linear regression was used to test for significant interaction between hardness and pH as TMFs. The stepAIC function from the MASS package was used following approaches used by Brix et al.10 DGT-labile zinc results were compared using ANOVA and post hoc Tukey multiple pairwise-comparisons. All metal concentrations in concentration–response models and results were measured concentrations. Standard deviation (SD) was used to specify variability.
Results and discussion
Quality assurance and control
All tests met the test acceptability criteria. The pH variability within tests was no greater than ±0.1 units of the average pH in each test treatment. Dissolved organic carbon concentrations were kept low, with less than 1 mg C L−1 reported in test solutions for all tests on day 0 in the absence of MOPS. Hardness concentrations (measured as Ca and Mg) in each test remained consistent, with no differences between tests of the same hardness concentration (Table S4†). Alkalinity varied slightly across all hardness concentrations (Table S4†); however, relative to the large concentration range of the hardness treatments, the changes in alkalinity were small.
Control growth rates were acceptable in all tests (>1.2 doublings per day) (Table 1). Copper reference toxicant tests run concurrently had a mean EC50 of 2.0 (±0.4) μg L−1, indicating that the microalgal cultures had repeatable and comparable sensitivity across tests. Dissolved metal subsamples collected on day 0 and day 3 in toxicity tests had average losses of measured zinc across the test duration of <10%, except for very low zinc treatments (<10 μg Zn L−1), where losses were between 0 and 7.6 μg Zn L−1. The mean of day 0 and day 3 metal concentrations were used in all toxicity modelling.
Table 1 The 72 h effect concentrations (EC10/EC50) for population growth inhibition of Chlorella sp. exposed to zinc under different hardness treatments at 3 different pH conditions. Effect concentrations were calculated using pooled test data (n = 2). 95% confidence intervals are shown in parentheses. Free ion EC values represent the WHAM calculated free ion concentration at the dissolved EC values. Control growth rate is shown as doublings per day. EC20 data is provided in ESI, Table S5†
Hardness (mg CaCO3 L−1) |
pH |
Control growth rate |
Dissolved Zn (μg L−1) |
Zn2+ (μg L−1) |
EC10 |
EC50 |
EC10 |
EC50 |
5 |
6.7 |
1.7 |
1.5 (1.0–2.1) |
8.7 (7.1–10) |
1.0 (0.68–1.4) |
5.9 (4.9–7.0) |
|
7.6 |
1.2 |
1.8 (1.0–2.7) |
17 (12–21) |
1.0 (0.57–1.5) |
9.5 (7.0–12) |
|
8.3 |
1.7 |
0.85 (0.62–1.1) |
6.2 (5.3–7.2) |
0.29 (0.21–0.37) |
2.1 (1.8–2.4) |
31 |
6.7 |
1.5 |
3.3 (2.1–4.5) |
31 (22–40) |
2.2 (1.4–3.0) |
20 (15–27) |
|
7.6 |
1.4 |
2.1 (1.3–2.9) |
32 (24–40) |
1.2 (0.74–1.6) |
18 (14–23) |
|
8.3 |
1.9 |
1.3 (1.0–1.7) |
13 (11–15) |
0.47 (0.34–0.59) |
4.3 (3.6–5.1) |
93 |
6.7 |
1.8 |
4.5 (2.8–6.3) |
184 (138–231) |
3.0 (1.8–4.2) |
122 (92–153) |
|
7.6 |
1.8 |
0.79 (0.49–1.1) |
120 (104–135) |
0.45 (0.3–0.6) |
68 (60–77) |
|
8.3 |
2.0 |
3.2 (2.6–3.8) |
53 (49–56) |
1.1 (0.9–1.3) |
18 (17–19) |
402 |
6.7 |
1.8 |
5.3 (4.3–6.3) |
96 (66–125) |
3.2 (2.6–3.8) |
59 (41–77) |
|
7.6 |
1.2 |
4.4 (1.8–7.0) |
159 (107–211) |
2.4 (0.96–3.8) |
6.9 (3.8–10) |
|
8.3 |
1.7 |
3.9 (2.4–5.3) |
59 (51–66) |
1.4 (0.87–2.0) |
22 (19–25) |
The effect of hardness at different pH values on zinc toxicity to Chlorella sp.
Test hardness had an inverse correlation with zinc toxicity at all pH values tested (Fig. 1 and Table 1), with increasing hardness corresponding with decreasing toxicity. Exceptions to these trends included a significant (p = 5.3 × 10−7, Z = 5.01) decrease in EC50 values in the pH 6.7 test series from 184 μg L−1 at 93 mg CaCO3 L−1 to 96 μg L−1 at 402 mg CaCO3 L−1 and a significant (p = 1.9 × 10−4, Z = 3.73) decrease in EC10 in the pH 7.6 test series from 2.1 μg L−1 at 31 mg CaCO3 L−1 to 0.8 μg L−1 at 93 mg CaCO3 L−1 (Table 1).
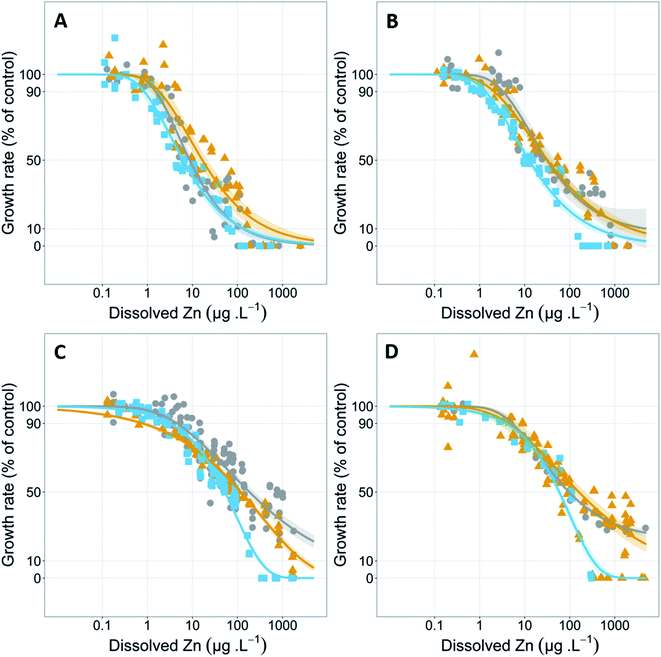 |
| Fig. 1 Concentration–response curves indicating the effect of pH and hardness on the growth rate of Chlorella sp. when exposed to dissolved zinc. Hardness concentrations tested were 5 (A), 31 (B), 93 (C) and 402 (D) mg CaCO3 L−1. Each hardness concentration was tested at pH 6.7 (grey circles), 7.6 (yellow triangles) and 8.3 (blue squares). Shaded ribbons represent the 95% confidence intervals. Each datapoint represents an individual replicate response and a corresponding measured zinc concentration. Data are pooled from separate experiments. Replicate responses were normalised to their respective controls for inter-test pooling. | |
Increasing water hardness is often found to reduce zinc toxicity.37 Hardness (and/or individual Ca2+ and Mg2+ concentrations) is a widely studied TMF for zinc; however, there are limited studies on direct effects of total hardness on algal species with most studies focusing on invertebrates and fish.38 One study using microalgae by Heijerick et al. investigated the individual influence of Ca2+ and Mg2+ rather than total hardness on zinc toxicity to R. subcapitata.18 Similar to the present study, the researchers reported significant decreases in toxicity with increasing concentrations for both calcium and magnesium, with a 1.7-fold increase in zinc EC50 values when calcium increased from 10 to 80 mg L−1 and a 6.5-fold increase when magnesium increased from 6 to 60 mg L−1. Similar ameliorative effects of increasing hardness on zinc toxicity have been shown for other freshwater organisms including acute and chronic toxicity to cladocerans and acute toxicity to fish.8,39–41 These trends are also apparent among other metals including nickel42 and uranium,43 but with a notable exception of copper, for which chronic toxicity has been shown to be minimally influenced by hardness.14,15,44 However, there are conflicting results around the influence of hardness on copper toxicity with recent toxicity models developed by Brix et al. showing hardness had a significant influence on both acute and chronic copper toxicity to a range of freshwater species.45 The authors also noted that hardness had a larger effect on acute toxicity models compared to chronic toxicity models.
Several mechanisms have been proposed to explain the observed ameliorative influence of hardness on zinc toxicity. Decreases in toxicity are generally attributed to competition between cations for binding sites at the biotic ligand on the organism. For rainbow trout, calcium has been shown to have a much greater ability to inhibit zinc uptake compared to magnesium, likely due to Ca2+ competing with Zn2+ for gill surface adsorption and uptake into the cell. In comparison, Mg2+ likely only competes with Zn2+ for cellular adsorption sites.46 This has been attributed to Ca2+ and Zn2+ sharing the same apical transport channel.47 These observations in fish may not directly translate to microalgae, with Heijerick et al. finding the opposite trend was true for R. subcapitata, where Mg2+ had a greater toxicity-reducing capacity compared to Ca2+.18 The calculated biotic ligand stability constants for Ca2+ were similar between microalgae and daphnids, but Mg2+ stability constants were more than 0.5
log units greater for microalgae. This suggests that magnesium has a higher binding affinity to the microalgal biotic ligand than for the daphnid biotic ligand, therefore providing greater toxicity amelioration. Additionally, zinc uptake mechanism studies by Reid et al. using the alga Chara corallina demonstrated that zinc influx into the algal cell was unlikely to be via the Ca2+ channels as zinc influx was unaffected by blockage of these channels by lanthanum (La3+).48
Increases in hardness from 5 to 93 mg CaCO3 L−1 resulted in a linear decrease in toxicity at all pH values and effect levels with strong linear correlation between EC50 values and hardness (R2 = 0.99 (pH 6.7), = 0.98 (pH 7.6), = 0.97 (pH 8.3)). Despite a 4.3-fold increase in hardness from 93 to 402 mg CaCO3 L−1, above 93 mg CaCO3 L−1 there was no continued significant decrease (p > 0.05) in toxicity at all pH values and effect levels, except for the EC10 (p = 0.005, Z = 2.83) at pH 7.6. Other studies have found similar plateauing in the protective effects at high hardness (and/or Ca2+ and Mg2+ ion concentrations). Rai et al. found that calcium concentrations above 20 mg L−1 resulted in reduced protective effects against zinc toxicity to Chlorella vulgaris.49 Similarly, in the current study the plateauing of protective effects occurred between 15 and 71 mg Ca L−1. Heijerick et al. also found higher concentrations of calcium and magnesium resulted in a plateau in the cations' ameliorative effect, with the authors noting that the biotic ligand model developed within that study would not be suitable for predicting zinc toxicity in higher hardness waters.40 It is important to note that, in natural waters, increased hardness is strongly correlated with increased pH and, these water chemistry conditions of 402 mg CaCO3 L−1 and pH 6.7 are unlikely. Analysis of water quality data in the United States by Brix et al. found that this combination of water chemistry fell outside 99% of the data collected.50 However, the authors also highlighted the importance of assessing toxicity in unlikely water chemistry conditions as metal-impacted sites may have unnatural associated water chemistries.50
Interactions of hardness and pH
Changes in pH do not appear to influence the protective effects of hardness, with relative changes in toxicity with changes in hardness remaining consistent between the pH treatments (i.e., compare the hardness treatment at different pH values in Fig. 2). At pH 6.7, an increase in hardness from 5 to 402 mg CaCO3 L−1 resulted in a 3.5-fold increase in EC10 values (1.5 to 5.3 μg L−1) and an 11-fold increase in EC50 values (8.7 to 96 μg L−1). Similar increases occurred at higher pH. At pH 7.6, a 2.4- and 9.4-fold increase in EC10 and EC50 values, respectively, were observed. At pH 8.3, a 4.5- and 9.5-fold increase in EC10 and EC50 values, respectively, were observed (Table 1). Use of stepwise linear regression confirmed this observed lack of interaction, which found interactive terms were not retained between hardness and pH for EC10 values (p = 0.829, t = 0.208) and EC50 values (p = 0.511, t = −0.680). Interestingly, the analysis also did not retain a pH term, with hardness being the only retained parameter (analysis output is provided in Table S6†). Limited interactions between the two water quality parameters was also found by Hyne et al., who reported a 2.5 and 2.3-fold decrease in acute zinc toxicity for C. dubia when hardness was increased from 44 to 374 mg CaCO3 L−1 at pH 7.5 and 8.4, respectively.44 Similar absences of interactions have also been observed for chronic nickel toxicity to R. subcapitata and acute copper toxicity to D. magna.42,51
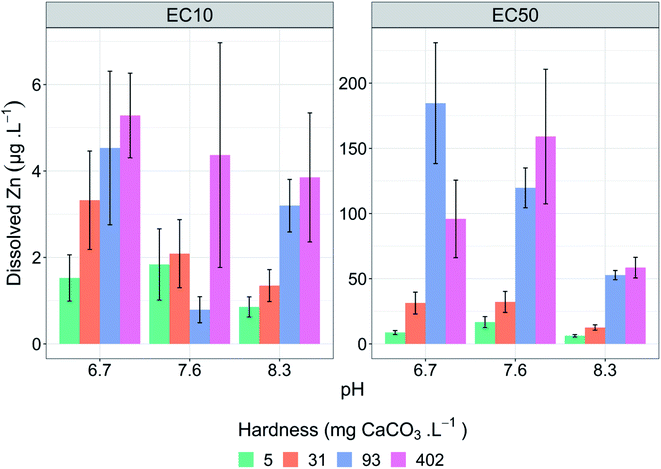 |
| Fig. 2 Comparison of EC10 and EC50 values for zinc as a function of hardness at 5 (green), 31 (orange), 93 (purple) and 402 (pink) mg CaCO3 L−1 at three different pH values. Effect concentrations at 10% (LHS panel) and 50% (RHS panel) growth rate inhibition after 72 h exposure. Error bars indicate the calculated lower and upper 95% confidences intervals. Note variable y-axis. | |
Zinc lability and speciation
DGT-labile zinc concentrations were measured in six dissolved zinc treatments (nominally 0–400 μg L−1), using the same composition as the 72 h algal growth-inhibition tests. DGT-labile zinc was less than dissolved zinc for all water conditions tested. The relationship between dissolved zinc and DGT-labile zinc followed strong linear relationships at each hardness tested (Fig. 3). For greater environmental relevance, the relationship between DGT-labile and dissolved zinc between 0 and 100 μg L−1, will be discussed. The linear relationship for all dissolved zinc concentrations tested are displayed in the inset plots in Fig. 3. DGT lability did not follow the same inverse relationship between hardness and zinc toxicity; no significant difference was detected (p = 0.0766, F = 2.929) in DGT-labile zinc concentrations relative to dissolved zinc concentrations (Fig. 3). This suggests zinc lability is unchanged across the hardness range tested (31–402 mg CaCO3 L−1) at pH 6.7. The lack of significant difference in DGT-labile zinc between hardness treatments suggests that the changes in bioavailability evident in the toxicity results of the present study are due to competition effects specific to the biotic ligand of the microalgae, rather than changes to zinc speciation or lability. Results of WHAM speciation modelling support the DGT results that changes in zinc speciation and lability are not the cause of the changes in toxicity with changes in hardness. Free zinc ion (Zn2+) was the major species present in solution under all test conditions. Zn2+ concentrations primarily varied as a function of pH (average change = 31%, SD = 5.4%), with only small changes due to hardness (average change = 4.1%, SD = 2.4%). Increased pH (6.7 to 8.3) resulted in a decrease in Zn2+ at all hardness concentrations, decreasing from an average of 69 to 34% and 61 to 37% for 5 and 402 mg CaCO3 L−1 hardness, respectively. Although Zn2+ decreased with increasing pH, toxicity increased with increasing pH for all hardness concentrations, suggesting that Zn2+ concentration is not the main factor influencing toxicity change in these conditions. A full summary of zinc speciation across all test conditions is provided in ESI, Table S7.†
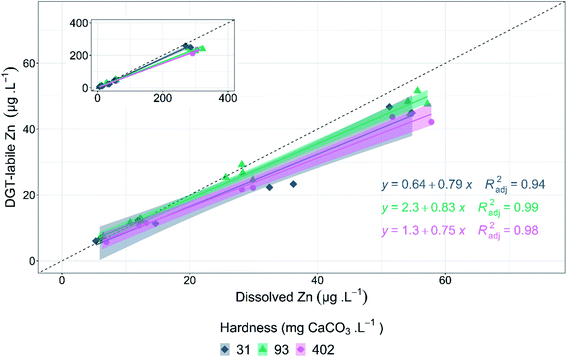 |
| Fig. 3 Dissolved zinc compared to DGT-labile zinc concentrations measured in 31 (grey diamonds), 93 (green triangles) and 402 (pink circles) mg CaCO3 L−1 at pH 6.7. Main plot displays data for zinc concentrations between 0 and 100 μg L−1. Inset plot displays all zinc concentrations determined for DGT-labile zinc (0–400 μg L−1). Dashed line represents a 1 : 1 relationship. | |
DGT results for the current study are similar to those of Paller et al., who measured DGT-labile zinc in low (13 mg CaCO3 L−1) and moderate (80–100 mg CaCO3 L−1) hardness waters in the absence of DOC.8 The study reported that differences in DGT-labile zinc did not correspond with the observed changes in acute zinc toxicity to C. dubia as hardness was varied. Philipps et al. assessed the influence of hardness on copper DGT lability and reported no significant changes in labile copper when hardness varied from 40–48 to 160–180 mg CaCO3 L−1.52 The study also found that while DGT lability remained unchanged with hardness, DGT-labile copper correlated with copper bioaccumulation in P. promelas, but not for copper bioaccumulation in the freshwater mussel, L. cariosa.
Several studies have compared biological responses to DGT lability changes under varying water chemistries including pH, hardness, and DOC.6–8,52,53 Consensus from these studies highlights that DGT lability is not a good predictor of bioavailability change when the primary cause of bioavailability change is via cation competition (H+, Ca2+, Mg2+). However, based on the studies with DOC, DGT may provide useful information on bioavailability in the presence of DOC, as changes in bioavailability and toxicity are associated with DOC–metal complexation.
Implications for water quality guideline values
Hardness was the first TMF investigated and as such hardness-dependent algorithms are incorporated into the water quality guidelines of several freshwater regulatory frameworks.16,54 The findings of the present study along with others have important implications for hardness-dependent guideline values for zinc.40,49 The collective results indicate that environmentally relevant high hardness conditions may not provide protective effects to all freshwater organisms from zinc toxicity.
Using eqn (1) there is a 9-fold difference in the zinc 95% species protection guideline value over the hardness range of 31–402 mg CaCO3 L−1 from 8 to 72 μg L−1, and a 3.6-fold difference over a range of 93–402 mg CaCO3 L−1 from 21 to 72 μg L−1. The use of this algorithm may provide appropriate increases in guideline values up until 93 mg CaCO3 L−1 but based on the results of the current study, precaution is needed at higher hardness. Moreover, the use of the algorithm with the ANZG16 framework does not allow for modifying the guideline value for hardness concentrations below 30 mg CaCO3 L−1, potentially providing insufficient protection in very soft waters. Australia has both very soft (<10 mg CaCO3 L−1)55 and hard waters (>200 mg CaCO3 L−1)56 and therefore may be subject to under protection using the current hardness-correction algorithms. While use of BLMs and MLRs is not endorsed by the current Australian and New Zealand framework, there is scope within the framework to justify the use of such models where appropriate. Based on the results of the current study, it may be most appropriate to use established bioavailability models (such as BLMs and MLRs) to adjust default zinc guideline values where hardness is very soft or hard. However, models developed with non-algal species (such as fish and daphnids) may not be appropriate and algal specific models may be required. Such species specific models are currently being developed and applied in order to revise zinc guideline values in Australia and New Zealand.
Conclusions
This study showed that increasing hardness has a protective effect on zinc toxicity to Chlorella sp. up to 93 mg CaCO3 L−1 across an environmentally relevant pH range of 6.7 to 8.3, with further increases in hardness not offering greater protection, with no difference in zinc toxicity from 93 to 402 mg CaCO3 L−1. It was also demonstrated that DGT-labile zinc did not change over the hardness range of 31 to 402 mg CaCO3 L−1 and did not correspond to observed changes in Chlorella sp. response across the same hardness range, this likely means that hardness affects Chlorella sp. through competition rather than speciation. DGT results were supported by WHAM speciation modelling, where only small changes in zinc speciation were due to changes in hardness. The results of this study also showed that current zinc hardness-algorithms used in water quality guidelines may not be appropriate to use for high hardness waters. More flexible and robust approaches, such as MLR models and/or BLMs are currently being considered in future revisions of Australian and New Zealand water quality guidelines, which could greatly improve the ability of regulators and industry to derive site-specific zinc guideline values for protecting sensitive aquatic biota, such as Chlorella sp.
Author contributions
G. Price: methodology, software, investigation, formal analysis, data curation, writing–original draft, and visualization. J. Stauber: conceptualization, methodology, resources, funding acquisition, project administration, writing–review and editing, and supervision. A. Holland: methodology, investigation, software, writing–review and editing, and supervision. D. Koppel: software, writing–review and editing, and supervision. E. Van Genderen: conceptualization, and writing–review and editing. A. Ryan: software and writing–review and editing. D. Jolley: conceptualization, methodology, resources, writing–reviewing and editing, and supervision.
Conflicts of interest
There are no conflicts to declare.
Acknowledgements
The present study was funded by the International Zinc Association and an Australian Government Research Training Program Scholarship for G. Price. We thank S. Stone and K. McKnight for providing technical advice during method development and manuscript preparation. We also thank G. Batley and D. Spadaro for providing comments which improved the manuscript.
References
- D. M. Di Toro, H. E. Allen, H. L. Bergman, J. S. Meyer, P. R. Paquin and R. C. Santore, Biotic Ligand Model of the Acute Toxicity of Metals. 1. Technical Basis, Environ. Toxicol. Chem., 2001, 20, 2383 CrossRef CAS PubMed.
- W. J. Adams, R. Blust, R. Dwyer, D. R. Mount, E. Nordheim, P. H. Rodriguez and D. Spry, Bioavailability Assessment of Metals in Freshwater Environments: A Historical Review, Environ. Toxicol. Chem., 2020, 39, 48–59 CrossRef CAS PubMed.
- W. Davison and H. Zhang,
In situ speciation measurements of trace components in natural waters using thin-film gels, Nature, 1994, 367, 546–548 CrossRef CAS.
- H. Zhang and W. Davison, Use of diffusive gradients in thin-films for studies of chemical speciation and bioavailability, Environ. Chem., 2015, 12, 85–101 CrossRef CAS.
- N. M. Franklin, J. L. Stauber, S. C. Apte and R. P. Lim, Effect of initial cell density on the bioavailability and toxicity of copper in microalgal bioassays, Environ. Toxicol. Chem., 2002, 21, 742–751 CrossRef CAS PubMed.
- G. K. Macoustra, A. Holland, J. L. Stauber and D. F. Jolley, Effect of Various Natural Dissolved Organic Carbon on Copper Lability and Toxicity to the Tropical Freshwater Microalga Chlorella sp., Environ. Sci. Technol., 2019, 53, 2768–2777 CrossRef CAS PubMed.
- G. A. V. Price, J. L. Stauber, A. Holland, D. J. Koppel, E. J. Van Genderen, A. C. Ryan and D. F. Jolley, The Influence of pH on Zinc Lability and Toxicity to a Tropical Freshwater Microalga, Environ. Toxicol. Chem., 2021, 40, 2836–2845 CrossRef CAS PubMed.
- M. H. Paller, S. M. Harmon, A. S. Knox, W. W. Kuhne and N. V. Halverson, Assessing effects of dissolved organic carbon and water hardness on metal toxicity to Ceriodaphnia dubia using diffusive gradients in thin films (DGT), Sci. Total Environ., 2019, 697, 134107 CrossRef CAS PubMed.
-
USEPA, Guidelines for Deriving Numerical National Water Quality Criteria for the Protection of Aquatic Organisms and Their Uses, Duluth, MN, 1985 Search PubMed.
- K. V. Brix, D. K. Deforest, L. M. Tear, M. Grosell and W. J. Adams, Use of Multiple Linear Regression Models for Setting Water Quality Criteria for Copper: A Complementary Approach to the Biotic Ligand Model, Environ. Sci. Technol., 2017, 51, 5182–5192 CrossRef CAS PubMed.
- J. L. Stauber, L. A. Golding, A. Peters, G. Merrington, M. S. Adams, M. Binet, G. E. Batley, F. Gissi, K. McKnight, E. R. Garman, E. T. Middleton, J. Gadd and C. Schlekat, Application of Bioavailability Models to Derive Chronic Guideline Values for Nickel in Freshwaters of Australia and New Zealand, Environ. Toxicol. Chem., 2021, 40, 100–112 CrossRef CAS PubMed.
- K. A. C. De Schamphelaere and C. R. Janssen, A biotic ligand model predicting acute copper toxicity for Daphnia magna: the effects of calcium, magnesium, sodium, potassium, and pH, Environ. Sci. Technol., 2002, 36, 48–54 CrossRef CAS PubMed.
-
ANZECC and ARMCANZ, Australian and New Zealand Guidelines for Fresh and Marine Water Quality, Natl. Water Qual. Manag. Strateg, 2000 Search PubMed.
- S. J. Markich, G. E. Batley, J. L. Stauber, N. J. Rogers, S. C. Apte, R. V. Hyne, K. C. Bowles, K. L. Wilde and N. M. Creighton, Hardness corrections for copper are inappropriate for protecting sensitive freshwater biota, Chemosphere, 2005, 60, 1–8 CrossRef CAS PubMed.
- S. J. Markich, A. R. King and S. P. Wilson, Non-effect of water hardness on the accumulation and toxicity of copper in a freshwater macrophyte (Ceratophyllum demersum): how useful are hardness-modified copper guidelines for protecting freshwater biota?, Chemosphere, 2006, 65, 1791–1800 CrossRef CAS PubMed.
-
Australian and New Zealand Governments, Australian and New Zealand guidelines for fresh and marine water quality, http://www.waterquality.gov.au/anz-guidelines, accessed 14 July 2021 Search PubMed.
-
M. Warne, G. E. Batley, R. A. Van Dam, J. Chapman, D. R. Fox, C. W. Hickey and J. L. Stauber, Revised Method for Deriving Australian and New Zealand Water Qaulity Guideline Values for Toxicants – Update of 2015 Version. Prepared for the Revision of the Australian and New Zealand Guidelines for Fresh and Marine Water Quality, Canberra, Australia, 2018 Search PubMed.
- D. G. Heijerick, K. A. C. De Schamphelaere and C. R. Janssen, Biotic ligand model development predicting Zn toxicity to the alga Pseudokirchneriella subcapitata: possibilities and limitations, Comp. Biochem. Physiol., Part C: Toxicol. Pharmacol., 2002, 133, 207–218 CAS.
- K. A. C. De Schamphelaere, S. Lofts and C. R. Janssen, Bioavailability Models for Predicting Acute and Chronic Toxicity of Zinc to Algae, Daphnids, and Fish in Natural Surface Waters, Environ. Toxicol. Chem., 2005, 24, 1190–1197 CrossRef CAS PubMed.
- T. Van Regenmortel, O. Berteloot, C. R. Janssen and K. A. C. De Schamphelaere, Analyzing the capacity of the Daphnia magna and Pseudokirchneriella subcapitata bioavailability models to predict chronic zinc toxicity at high pH and low calcium concentrations and formulation of a generalized bioavailability model for D. magna, Environ. Toxicol. Chem., 2017, 36, 2781–2798 CrossRef CAS PubMed.
- N. M. Franklin, J. L. Stauber and R. P. Lim, Development of flow cytometry-based algal bioassays for assessing toxicity of copper in natural waters, Environ. Toxicol. Chem., 2001, 20, 160–170 CrossRef CAS PubMed.
-
A. S. Thompson, J. C. Rhodes and I. Pettman, Culture Collection of Algae and Protozoa: Catalogue of Strains, Freshwater Biological Association, 1988 Search PubMed.
-
USEPA, Methods for Measuring the Acute Toxicity of Effluents and Receiving Waters to Freshwater and Marine Organisms, USEPA, Washington, DC, 2002 Search PubMed.
-
OECD, in OECD Guidelines for the Testing of Chemicals., OECD Publishing, 2011 Search PubMed.
-
N. M. Franklin, J. L. Stauber and M. S. Adams, in Techniques in Aquatic Toxicology, Taylor and Francis, Boca Raton, Fl, USA, 2005, vol. 2, pp. 735–756 Search PubMed.
- K. A. C. De Schamphelaere, D. G. Heijerick and C. R. Janssen, Comparison of the effect of different pH buffering techniques on the toxicity of copper and zinc to Daphnia magna and Pseudokirchneriella subcapitata, Ecotoxicology, 2004, 13, 697–705 CrossRef CAS PubMed.
-
J. L. Stauber, G. A. V. Price, A. Evans, J. Gadd, A. Holland, G. E. Batley, M. T. Binet, L. A. Golding, C. W. Hickey, A. J. Harford, D. F. Jolley, D. J. Koppel, K. McKnight, L. Morais, A. C. Ryan, K. Thompson, E. J. Van Genderen, R. A. Van Dam and M. S. J. Warne, Towards Bioavailability-Based Guideline Values for Zinc for Australian and New Zealand Freshwaters, Australia, 2021 Search PubMed.
- S. Stone, M. S. Adams, J. L. Stauber, D. F. Jolley and M. Warne, Development and application of a multispecies toxicity test with tropical freshwater microalgae, Environ. Pollut., 2019, 250, 97–106 CrossRef CAS PubMed.
- D. K. Deforest and E. J. Van Genderen, Application of U.S. EPA guidelines in a bioavailability-based assessment of ambient water quality criteria for zinc in freshwater, Environ. Toxicol. Chem., 2012, 31, 1264–1272 CrossRef CAS PubMed.
- E. D. Amato, T. Bolam and M. J. Belzunce-Segarra, Guidelines for the use of Diffusive Gradients in Thin Films for measuring metal fluxes in sediment, ICES Tech. Mar. Environ. Sci., 2019, 62, 19 Search PubMed.
- H. Zhang and W. Davison, Performance Characteristics of Diffusion Gradients in Thin Films for the in situ Measurement of Trace Metals in Aqueous Solution, Anal. Chem., 1995, 67, 3391–3400 CrossRef CAS.
- C. Ritz, F. Baty, J. C. Streibig and D. Gerhard, Dose-response analysis using R, PLoS One, 2015, 10, 1–13 CrossRef PubMed.
-
H. Wickham, Elegant Graphics for Data Analysis, Springer-Verlag, New York, USA, 2016 Search PubMed.
-
A. Kassambara, Package ggpubr; ‘ggplot2’ Based Publication Ready Plots, 2018 Search PubMed.
- C. Ritz, Toward a unified approach to dose-response modeling in ecotoxicology, Environ. Toxicol. Chem., 2010, 29, 220–229 CrossRef CAS PubMed.
- M. W. Wheeler, R. M. Park and A. J. Bailer, Comparing median lethal concentration values using confidence interval overlap or ratio tests, Environ. Toxicol. Chem., 2006, 25, 1441–1444 CrossRef CAS PubMed.
-
Canadian Council of Ministers of the Environment (CCME), Canadian water quality guidelines for the protection of aquatic life: zinc, in Canadian Environmental Quality guidelines, 1999, Canadian Council of Ministers of the Environment, Winnipeg, MB, 2018, pp. 1–13 Search PubMed.
-
J. S. Meyer, S. J. Clearwater, T. A. Doser, M. J. Rogaczewski and J. A. Hansen, Effects of Water Chemistry on the Bioavailability and Toxicity of Waterborne Cadmium, Copper, Nickel, Lead and Zinc to Freshwater Organisms, Society of Environmental Toxicology and Chemistry, Pensacola, FL, 2007 Search PubMed.
- M. G. Barron and S. Albeke, Calcium control of zinc uptake in rainbow trout, Aquat. Toxicol., 2000, 50, 257–264 CrossRef CAS PubMed.
- D. G. Heijerick, K. A. C. De Schamphelaere and C. R. Janssen, Predicting Acute Zinc Toxicity for Daphnia magna As a Function of Key Water Chemistry Characteristics: Development and Validation of a Biotic Ligand Model, Environ. Toxicol. Chem., 2002, 21, 1309 CrossRef CAS PubMed.
- D. G. Heijerick, K. A. C. De Schamphelaere, P. A. Van Sprang and C. R. Janssen, Development of a chronic zinc biotic ligand model for Daphnia magna, Ecotoxicol. Environ. Saf., 2005, 62, 1–10 CrossRef CAS PubMed.
- N. M. E. Deleebeeck, K. A. C. De Schamphelaere and C. R. Janssen, Effects of Mg2+ and H+ on the toxicity of Ni2+ to the unicellular green alga Pseudokirchneriella subcapitata: model development and validation with surface waters, Sci. Total Environ., 2009, 407, 1901–1914 CrossRef CAS PubMed.
- A. L. Charles, S. J. Markich, J. L. Stauber and L. F. De Filippis, The effect of water hardness on the toxicity of uranium to a tropical freshwater alga (Chlorella sp.), Aquat. Toxicol., 2002, 60, 61–73 CrossRef CAS PubMed.
- R. V. Hyne, F. Pablo, M. Julli and S. J. Markich, Influence of water chemistry on the acute toxicity of copper and zinc to the cladoceran ceriodaphnia CF dubia, Environ. Toxicol. Chem., 2005, 24, 1667–1675 CrossRef CAS PubMed.
- K. V. Brix, L. M. Tear, R. C. Santore, K. Croteau and D. K. DeForest, Comparative Performance of Multiple Linear Regression and Biotic Ligand Models for Estimating the Bioavailability of Copper in Freshwater, Environ. Toxicol. Chem., 2021, 40, 1649–1661 CrossRef CAS PubMed.
- D. H. Alsop and C. M. Wood, Influence of waterborne cations on zinc uptake and toxicity in rainbow trout, Oncorhynchus mykiss, Can. J. Fish. Aquat. Sci., 1999, 56, 2112–2119 CrossRef CAS.
- C. Hogstrand, P. M. Verbost, S. E. Wendelaar Bonga and C. M. Wood, Mechanisms of zinc uptake in gills of freshwater rainbow trout: Interplay with calcium transport, Am. J. Physiol.: Regul., Integr. Comp. Physiol, 1996, 270(5), 1141–1147 CrossRef PubMed.
- R. J. Reid, J. D. Brookes, M. A. Tester and F. A. Smith, The mechanism of zinc uptake in plants characterisation of the low-affinity system, Planta, 1996, 198, 5290 CrossRef.
- L. C. Rai, J. P. Gaur and H. D. Kumar, Protective effects of certain environmental factors on the toxicity of zinc, mercury, and methylmercury to Chlorella vulgaris, Environ. Res., 1981, 25, 250–259 CrossRef CAS PubMed.
- K. V. Brix, D. K. DeForest, L. M. Tear, W. Peijnenburg, A. Peters, E. T. Middleton and R. Erickson, Development of Empirical Bioavailability Models for Metals, Environ. Toxicol. Chem., 2020, 39, 85–100 CrossRef CAS PubMed.
- K. E. Long, E. J. Van Genderen and S. J. Klaine, The Effects of Low Hardness and pH on Copper Toxicity to Daphnia magna, Environ. Toxicol., 2004, 23, 72–75 CrossRef CAS PubMed.
- R. R. Philipps, X. Xu, G. L. Mills and R. B. Bringolf, Impact of natural organic matter and increased water hardness on DGT prediction of copper bioaccumulation by yellow lampmussel (Lampsilis cariosa) and fathead minnow (Pimephales promelas), Environ. Pollut., 2018, 241, 451–458 CrossRef CAS PubMed.
- G. K. Macoustra, D. F. Jolley, J. L. Stauber, D. J. Koppel and A. Holland, Speciation of nickel and its toxicity to Chlorella sp. in the presence of three distinct dissolved organic matter (DOM), Chemosphere, 2021, 273, 128454 CrossRef CAS PubMed.
-
USEPA, Water Quality Criteria Documents for the Protection of Aquatic Life in Ambient Water: 1995 Updates, 1995 Search PubMed.
- A. Peters, G. Merrington, J. Stauber, L. A. Golding, G. E. Batley, F. Gissi, M. S. Adams, M. Binet, K. McKnight, C. E. Schlekat, E. R. Garman and E. T. Middleton, Empirical Bioavailability Corrections for Nickel in Freshwaters for Australia and New Zealand Water Quality Guideline Development, Environ. Toxicol. Chem., 2021, 40, 113–126 CrossRef CAS PubMed.
-
G. E. Batley, R. A. Van Dam, M. Warne, J. Chapman, D. R. Fox, C. W. Hickey and J. L. Stauber, Technical Rationale for Changes to the Method for Deriving Australian and New Zealand Water Quality Guideline Values for Toxicants – Update of 2014 version. Prepared for the Revision of the Australian and New Zealand Guidelines for Fresh and Marine Water, Canberra, Australia, 2018 Search PubMed.
|
This journal is © The Royal Society of Chemistry 2022 |