Screening of pesticide distributions in foods of animal origin: a matrix-based approach for biotransfer factor modeling of grazing mammals†
Received
29th October 2021
, Accepted 13th March 2022
First published on 31st March 2022
Abstract
Pesticides are detected in a wide array of foods of animal origin, posing a threat to food safety and human health. Here, to facilitate the management of pesticide residues in livestock products, we proposed a screening model for simulating pesticide biotransfer factors (BTFs, ratio of steady-state pesticide concentration between animal tissues and feed) in mammalian bodies. The proposed model was developed based on simple matrix algebra using first-order kinetics. Simulation of over 700 pesticides in common food products derived from cattle and sheep indicated that pesticide biotransfer is a balancing process between uptake efficiency from the gut lumen and the uptake-elimination ratio (ratio of the overall uptake and elimination rate constants) in individual tissues. Furthermore, we parameterized the developed BTF model using the octanol–water partition coefficient. The simulated pesticide BTFs could be categorized into three lipophilicity phases, namely, the lipophilicity-boosting (log
KOW < 4), lipophilicity-balancing (4 ≤ log
KOW ≤ 8), and lipophilicity-limiting (log
KOW > 8) phases, and the simulated general trends of BTFs were consistent with the experimental data. Although the metabolic rate constants of pesticides in mammalian bodies warrant further evaluation, the model proposed here can assist in the risk assessment and regulatory management of pesticide residues in foods of animal origin.
Environmental significance
Globally, hundreds of plant protection products are registered and in use. After application, pesticide residues can remain in crops and plants that are used for feed production. As a result, different pesticide residues can enter and bioaccumulate in different animal tissues, including liver, meat, and milk, which are used for human food consumption. However, there is a lack of mechanism-based models, which are capable of high-throughput screening of residue levels in foods of animal origin in support of regulatory risk assessment. This work proposes a spreadsheet-based simulation tool that is flexible for dealing with different chemical species, grazing animals, and raw livestock products.
|
1. Introduction
Pesticides are fundamental to agricultural productivity; however, their residues transported through the agricultural food chain can cause health damage to both humans and animals.1–7 With that, pesticides contribute to the global animal and human health burden.8,9 To protect population health and promote commodity trade, international regulatory organizations, including the European Union (EU) and Codex Alimentarius Commission (Codex), have defined maximum residue levels (MRLs) as the legal limits for pesticides in foods. The EU and Codex have defined pesticide MRLs for nearly all food groups based on several regulatory approaches, such as market residue monitoring, precautionary principles, and analytical detection methods.10–12
In general, plant uptake models used in risk assessment and life cycle impact assessment of pesticides in crops for human consumption require information on plant physiology and structure (e.g., stems, leaves, and roots), because the edible parts (e.g., tubers and fruits) are typically unique to plants and homogeneous in terms of nutritional composition.13 Therefore, field measurements and maximum exposure levels via intake of pesticide residues in food or fodder crops can be directly used to set and compare with MRLs in crops.14 In contrast, the nutritional composition of edible products (e.g., muscle, fat, milk, and liver) derived from livestock varies greatly, particularly in terms of lipid and water contents, resulting in markedly different degrees of pesticide bioaccumulation in different body parts of animals biotransferred from the feed for lipophilic or hydrophilic compounds.1,15 In addition, the fate and distribution of pesticides in animal bodies are more complex than those in plants because of the more sophisticated physiological characteristics. More importantly, particularly for lipophilic pesticides, indirect consumption via eating animal parts after they have eaten fodder contaminated crops over months or even years is an important pathway.16,17 Also, even under low exposure doses (e.g., low residue levels in feed materials), lipophilic and persistent pesticides (e.g., lindane) can have a high degree of bioaccumulation in the livestock with a long life cycle (e.g., cattle and sheep), as compared to some short-life-cycle grazing animals such as chicken and duck.18 Therefore, understanding the quantitative relationships associations of pesticide concentrations in different edible parts of livestock from animal feed is essential for health risk and impact assessments, and MRL determination for foods of animal origin, which can also be a complementary component to “direct human consumption of crops” as current basis of evaluating and management pesticide residues in crops.19,20 For instance, according to the European Commission,11 pesticide MRLs should be defined by taking into account the distribution and depletion kinetics of residues in edible animal tissues. In addition, elucidating pesticide concentration distribution in edible animal tissues can help regulate pesticide levels in feed in animal husbandry (e.g., animal health and residues in raw livestock products, among others).21 Therefore, understanding the fate and distribution of pesticides in animal bodies is crucial for the risk management of livestock products.
Experimental efforts have been made to evaluate the distribution kinetics of pesticides in animal bodies, which have significantly improved our understanding of the toxicokinetics and toxicodynamics of pesticides, providing valid benchmark data for risk assessment.22,23 As there are hundreds of pesticides registered and used today, practical models simulating the fate of pesticides in animal bodies and estimating pesticide levels in edible tissues are required, particularly at the screening level. Some modeling approaches have been proposed to estimate pesticide concentrations in edible animal tissues. For instance, a complete physiologically based kinetic (PBK) model24,25 was introduced to simulate the fate of chemicals in common livestock. The PBK model includes 13 compartments and can be solved by a group of ordinary differential equations. This model predicts chemical concentration as a function of time following oral ingestion or intravenous injection. Further, using parameterization approaches based on simulating system dynamics, practical models estimating long-term average pesticide concentrations in meat and milk based on specific key physicochemical variables, such as the octanol–water partition coefficient (KOW), have been developed.26,27 Additionally, simplified partitioning rules can predict general pesticide distribution trends in mammal bodies; however, certain processes, such as metabolic rates in the liver, are often omitted as part of conservative assumptions.28 These modeling approaches, in addition to other bioaccumulation and PBK models,29–38 have generally advanced our understanding of the fate of pesticides in animal bodies.
To have more realistic approaches that follow strict mass balances (instead of short-cutting some processes for conservative reasons) and can be used for screening-level comparisons of many pesticide–crop–animal tissue combination, a suitable model should possess the following characteristics: (1) it should be sufficiently simple rather than running a group of complex differential equations; (2) it should consider all relevant toxicokinetic processes of pesticides in animal bodies (e.g., absorption, metabolism, convection, bioaccumulation, and elimination); and (3) it should predict pesticide concentrations in all common edible tissues (e.g., milk, muscle, fat, liver, and kidney) and perform high-throughput screening of thousands or more combinations of pesticides and (edible) animal tissues, which could help better determine MRLs. To resolve these challenges and promote regulatory risk assessment of pesticides in foods of animal origin, in the present study, we proposed a model based on matrix algebra, which can conveniently predict pesticide distributions in animal bodies and thereby calculate BTFs that connect pesticide concentrations in edible tissues of livestock and those in animal feed.
2. Methods and materials
2.1 General matrix algebra
Fig. 1 shows a conceptual diagram of the general model for pesticide transport and distribution among multiple compartments. The multiple-compartment system possesses the following characteristics: (1) the pesticide is transferred between every two compartments via diffusion or advection, mimicking the transfer process between two organs via diffusion across concentration gradient or convection via blood transport; (2) the pesticide undergoes degradation in each compartment, mimicking pesticide biodegradation or biotransformation in organs or tissues; (3) the pesticide entering each compartment is continuously taken in at a constant rate, mimicking the pesticide uptake via lumen absorption (i.e., food consumption); and (4) the pesticide leaving each compartment is continuously eliminated, mimicking several excretory processes, such as fecal, urinary, and biliary excretion and exhalation.
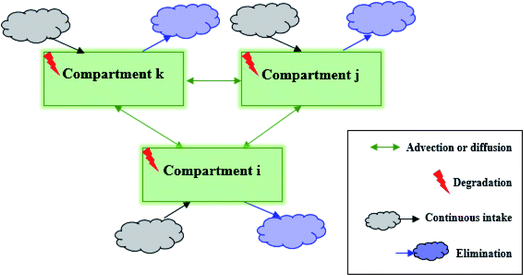 |
| Fig. 1 Conceptual diagram of the general compartment model of a pesticide being transported and distributed in a multiple-compartment system. | |
All processes including intake, advection (or diffusion), degradation, and elimination can be approximated as first-order kinetics. In other words, the reaction rate (mass change) is linearly proportional to pesticide concentration. We note that when the continuous mass intake only happens in specific compartments and there is no “mass transferred” through inter-compartmental processes, first-order kinetics rate constants can be set as zero. Then, in compartment i, the fate of the pesticide can be expressed as follows:
|  | (1) |
where
mi(
t) (mg) and
mj(
t) (mg) are the pesticide mass as a function of time in compartments
i and
j, respectively;
Fi (mg d
−1) is the flow rate of the pesticide (
i.e., the continuous mass intake) in compartment
i;
k+j→i (d
−1) and
k−i→j (d
−1) are the specific transfer rates (advection or diffusion) from compartment
j to
i and from compartment
i to
j, respectively; the term “
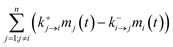
” denotes the net mass of the pesticide transferred from (
n − 1) compartments to compartment
i;
kd,i (d
−1) is the degradation (or biotransformation) rate of the pesticide in compartment
i; and
ke,i (d
−1) is the excretion rate of the pesticide in compartment
i.
Then, the fate and distribution of the pesticide in this system with n compartments can be expressed using linear equations, where the steady-state mass of the pesticide in the compartments can be solved using matrix algebra. Then, the matrix algebra of eqn (1) for the given system with n compartments can be expressed as follows:
| 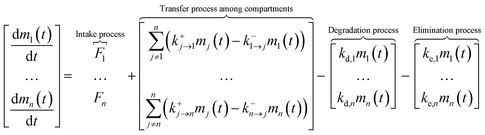 | (2) |
In the steady state, eqn (2) can be solved as follows:
| 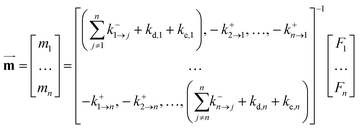 | (3) |
where

(mg) denotes the vector of the steady-state mass of the pesticide in compartments, and thereby the corresponding vector of the steady-state concentration of the pesticide (

, mg kg
−1) (
e.g.,

) can be expressed as follows:
| 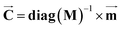 | (4) |
where
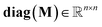
(kg) denotes the diagonal matrix of the mass (fresh) of compartments.
2.2 Specific matrix algebra for livestock
The specific matrix algebra for modeling the fate and distribution of pesticides in livestock bodies was adjusted from the general matrix algebra based on the complete ordinary differential equations introduced by Lautz et al.25 In their study, a generic PBK model, including 13 compartments (i.e., gut lumen, gut tissue, liver, heart, brain, adipose tissue, muscle, bone, lung, kidney, milk, arterial blood and venous blood), was developed to predict chemical concentrations in animal bodies using ordinary differential equations for describing the fate of chemicals in each organ (or tissue) compartment. In the present study, we simplified the pesticide fate processes in animal tissues and converted all processes into first-order kinetics, because the rate of the mass change is linearly proportional to pesticide concentration. We note that the biotransformation of the pesticide in the liver should follow the Michaelis–Menten kinetics, which can be approximated as the first-order kinetics when the pesticide concentration is low, particularly for the long-term exposure scenario.39 The developed model comprises seven tissue compartments [i.e., liver, kidney, lung, fat (adipose tissue), muscle, mammary gland, and blood]. In this model, the gut lumen is directly connected with the liver compartments considering the first pass effect (Fig. 2) and pesticide absorption from food in the gut lumen and its transfer to the liver are characterized using the uptake efficiency model.15 In addition, pesticide uptake into animal bodies was assumed to be a continuous process, that is, food stuff always exists in the gut lumen, such that the steady-state condition of the pesticide distribution in animal tissues can be reached. In addition, the blood system was treated as a well-mixed compartment, that is, pesticide concentration was assumed to be equal in venous and arterial blood; these assumptions have been widely applied in PBK modeling studies.39 The complete equations of our simplified model are provided in the Appendix.† Then, the specific matrix algebra can be adjusted from the general matrix algebra using the following rules (corresponding to the four characteristics of the general compartment model: (1) the pesticide can only be transported to organs via blood flux, resulting in zero transfer rate constants among organs; (2) the pesticide is metabolized (degradation or biotransformation) only in the liver compartment; (3) the pesticide is continuously taken in via feed consumption (i.e., inhalation or dermal route is not considered) only in the gut lumen compartment; and (4) the pesticide is eliminated in the gut lumen, kidney, liver, and mammary gland compartments via fecal, urinary, biliary, and milk excretion, respectively. In the present study, the physiological characteristics of mature animals were applied (ESI†); therefore, the elimination rate constant for animal growth (i.e., the dilution effect) was not considered.24,25 By adjusting the general matrix algebra in eqn (3), the specific matrix algebra for evaluating pesticide distribution in animal bodies in the steady state can be expressed as follows: |  | (5) |
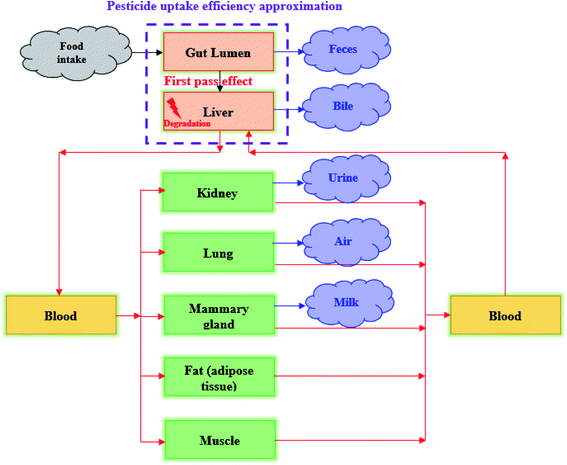 |
| Fig. 2 Conceptual diagram of the specific compartment model of a pesticide being transported and distributed in livestock bodies. | |
The variables are defined in Table 1, and their derivations are detailed in the ESI.† In this study, we considered the first pass effect (i.e., pesticides are transferred from the gut lumen to the liver) and thereby the mass intake of the pesticide in the liver (FLiver, mg d−1) can be approximately as follows:
| FLiver = EUptakeIRFoodCFood | (6) |
where
EUptake (unitless) is the uptake efficiency of pesticides
via oral ingestion (S2 of the ESI
†); IR
Food (kg d
−1) is the intake rate of food; and
CFood (dry mass, mg kg
−1) is the pesticide concentration in food. As we only considered the exposure pathway
via food consumption, the continuous mass intake in other organs or tissues are not considered in this study,
i.e., the mass flow rate is zero.
Table 1 Equations of the rate constant (d−1) for the mass of the pesticide in animal tissuesa
Rate constant |
Equation |
For rate constants (k, d−1), superscriptions “−” and “+” denote the elimination and uptake processes of the pesticide in tissues, respectively; and subscription “→” denotes the one-direction transfer (i.e., only from left to right). For blood flows (Q, kg d−1), subscription “⇔” denotes the double-direction flow (i.e., the left-to-right flow is equal to the right-to-left flow); and the expressions are provided in S1 of the ESI. For partition coefficients (K, unitless), subscription “numerator/denominator” denotes the numerator-to-denominator partition coefficient of the pesticide; and the expressions are provided in S4 of the ESI. For excretion rates (ER, kg d−1), the expressions are provided in S1 of the ESI.
|
Liver-to-blood elimination |
|
Metabolic biotransformation in the liver |
Estimated from biotransformation half-lives in fish (S3 of the ESI).40,41 |
Elimination via biliary excretion |
|
Blood-to-liver uptake |
|
Kidney-to-blood elimination |
|
Elimination via urinary excretion |
|
Blood-to-kidney uptake |
|
Lung-to-blood elimination |
|
Elimination via exhalation |
|
Blood-to-lung uptake |
|
Fat-to-blood elimination |
|
Blood-to-fat uptake |
|
Muscle-to-blood elimination |
|
Blood-to-muscle uptake |
|
Mammary gland-to-blood elimination |
|
Elimination via milk excretion |
|
Blood-to-mammary gland uptake |
|
Liver-to-blood uptake |
|
Kidney-to-blood uptake |
|
Lung-to-blood uptake |
|
Mammary gland-to-blood uptake |
|
Fat-to-blood uptake |
|
Muscle-to-blood uptake |
|
Blood-to-tissue i elimination |
|
2.3 Biotransfer factors (BTFs)
The BTF of a pesticide is defined as the steady-state concentration ratio of the pesticide in the animal tissues to the concentration in animal feed and its vector
can be expressed as follows: |  | (7) |
Therefore, the biotransfer factor of the pesticide in tissue i (BTFi) can be expressed as ‘
’, where Ci (mg kg−1) is the steady-state concentration of the pesticide in tissue i. The pesticide BTF should be differentiated for different food or fodder sources due to different nutritional compositions (i.e., lipid and water contents) that affect the EUptake value; here, we applied generic values of the nutritional compositions of fodder (S2 of the ESI†), which can be adjusted according to real situations. According to eqn (7), BTFi is equal to the numerical result of Ci when the CFood value is set at 1.0 mg kg−1.
2.4 Model parameterization and evaluation
We performed BTF simulations for 736 pesticides, of which the basic physicochemical parameters (e.g., log
KOW, biotransformation half-lives) were available from the current databases.42–44 Two common livestock animals, namely cattle and sheep, were selected to perform BTF simulations for foods of animal origin (raw). A significant portion of foods of animal origin are derived from cattle and sheep, and these animals are often exposed to pesticide residues when grazing in grasslands.45 All simulated data are provided in the ESI† database. Finally, we parameterized the BTF model using common physicochemical variables (e.g., KOW) and evaluated model performance by comparing the simulated results with reported experimental findings.
2.5 Regulatory applications
To help regulatory agencies derive pesticide MRLs, we proposed a relative potency approach considering both human health and pesticide distributions in raw livestock products (i.e., BTFs). As there was a strong linear relationship (R2 > 0.99) among the BTFs of pesticides in cattle and sheep products (S9 of the ESI†), one of the livestock products (e.g., the liver and kidney) can be used as the surrogate food product to express the BTFs of other food products. Then, the quantitative relationships of the pesticide BTFs of raw food products from livestock can serve as the starting point for calculating recommended MRLs (based on human health), even before detailed information on depletion and food processing is available, as follows: |  | (8) |
where CRi (kg d−1) is the consumption rate of livestock product i; DFi (unitless) and PFi (unitless) are the dissipation factor (the depletion process after slaughtering) and the food processing factor (the reduction process during storage, manufacturing, or cooking) of pesticide in livestock product i; ADI (mg kg−1 d−1) is the acceptable daily intake of the pesticide; BWHuman (kg) is the human body weight; and AF is the allocation factor that accounts for other exposure pathways. As the information of DFi and PFi for many pesticides is missing, we assumed that they are both 1.0 for conservative considerations and a realistic screening among hundreds of pesticides; and such factors in case regulatory agencies want to apply more realistic factors can be directly incorporated in eqn (8). To comply with the ratios of the theoretical steady-state concentrations of pesticides in livestock products, we selected the pesticide MRL in the liver (MRLLiver, mg kg−1) as the surrogate MRL. Then, eqn (8) can be expressed as follows: | 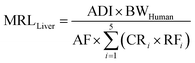 | (9a) |
| 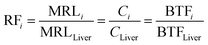 | (9b) |
where RFi is the relative factor of livestock product i, which is the ratio of the steady-state concentration of the pesticide in livestock product i to that in the liver. Thus, the derived MRLs in eqn (9a) and (b) consider both human health and pesticide distributions in foods of animal origin.
2.6 Uncertainty analysis
The physiological condition can vary substantially among animal species as well as individuals, which could lead to a considerable variation in the simulated BTFs of pesticides in raw livestock products. We provided a spreadsheet-based approach to generate uncertainty intervals of BTFs (ESI† database); with that, users can customize model inputs (e.g., milk production rates, animal body weights) to simulate the corresponding BTFs. Rossow et al.46 evaluated the impact of enzyme-treated feed on the production rate and nutrition composition (e.g., fat and protein contents) of milk for dairy cows. We took these data collected as model inputs to evaluate the variation in simulated BTFs of pesticides in milk. Details about the data and calculation are provided in S12 of the ESI.†
3. Results and discussion
3.1 Distribution of pesticide BTFs and model parameterizations
Fig. 3 shows the general distribution and central tendencies of simulated BTFs for 736 pesticides in five raw products derived from cattle and sheep, including liver, kidney, fat, muscle, and milk. Overall, the fat achieved the largest pesticide BTF among the selected raw products. For example, the median pesticide BTF of cattle fat was 1.63 which was over 15 and 18 times higher than that of the liver and other products, respectively. This result can be explained by the highest lipid content of fat (i.e., 80% applied in this study). Moreover, all selected pesticides in the present study were organic compounds with a median log
KOW of 3; thus, in the steady state, more lipophilic pesticide residues could be absorbed in the fat tissue than in the other tissues of cattle. The lipid content of the remaining four products was similar (i.e., around 3–4%). In general, however, pesticide BTF of the cattle liver was higher than that of the kidney, muscle, and milk. These results can be explained by the fact that after oral exposure to pesticides via food consumption, their residues are first transported from the gut lumen to the liver (i.e., the first pass effect), which acts as the major source of pesticide distribution within animal bodies. Meanwhile, in other tissues, blood flux is assumed to be the only source of pesticide biotransfer in our model, and blood pesticide concentration may be significantly reduced as a result of metabolic processes in the liver. Thus, at the steady state, pesticide residues are more kinetically favorable to be absorbed in the liver than in the kidney, muscle, and milk. However, in general, the simulated pesticide BTF of the sheep milk was higher than that of the liver, kidney, and muscle, which is due to the relatively high lipid content of the sheep milk (i.e., 7%).
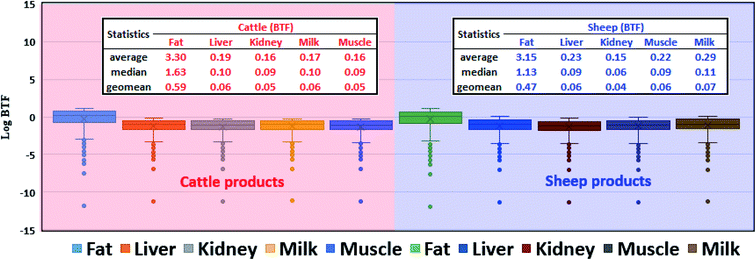 |
| Fig. 3 Box and whisker plots of the logarithmic values of the biotransfer factors (BTFs) of 736 pesticides in common cattle and sheep products (liver, kidney, fat, muscle, and milk). Average (arithmetic), median, and geomean values of BTFs of 736 pesticides in common cattle and sheep products are provided. | |
To further explore the thermodynamic and kinetic factors affecting pesticide BTFs of cattle products, we evaluated the associations of pesticide BTFs with key model input variables. Fig. 4A shows the simulated log
BTF values of the 736 pesticides in common food products derived from cattle plotted against the log
KOW values. The log
BTF values for cattle and sheep products were parametrized using log
KOW values (S8 of the ESI†). Overall, the association between the log
BTF and log
KOW values could be categorized into three phases. When the log
KOW value was below 4 (i.e., the lipophilicity-boosting phase), the log
BTF value increased with increasing log
KOW value. During this phase, the log
BTF value of hydrophilic pesticides in cattle products is extremely low, because these pesticides exhibit low uptake efficiency from the gut lumen into the blood system due to the low affinity of the bilayer structure (e.g., lipid layer) of the intestinal membrane (Fig. A1†), which limits the uptake of these pesticides.15 Of note, the log
BTF values of pesticides in the cattle products continued to increase until the log
KOW value reached 4, even though the simulated uptake efficiency reached the maximum value of 1 when the log
KOW value was increased to −0.6 (Fig. A1†). This is because although the kinetic condition (i.e., uptake efficiency) reaches its limit, the increase in log
KOW value renders the pesticide thermodynamically favorable to be absorbed in the lipid part of the cattle products. Thus, the higher the lipid content of the tissue (e.g., adipose tissue), the higher the log
BTF value of the pesticide in that tissue (Fig. A1†). When the log
KOW value exceeded 4 but remained below 8 (i.e., the lipophilicity-balancing phase), the log
BTF values of all cattle products reached their maximum values and remained unchanged with further increase in log
KOW. This is because the uptake–elimination ratio of the pesticide (i.e., the rate constant ratio of uptake to elimination; definitions and equations are provided in S7 of the ESI†) in cattle products reached its limit when the log
KOW value exceeded 4 (Fig. 4C). When the log
KOW value exceeded 8 (i.e., the lipophilicity-limiting phase), the log
BTF value decreased with increase in log
KOW. This is because the uptake efficiency of very highly lipophilic pesticides is low, due to the steric hindrance effect or the low concentration in the aqueous layer that limits the transport process (e.g., membrane penetration) of the pesticide from the gut lumen to the blood.15,47,48Fig. 4B shows the association between the simulated log
BTF values and the log half-life values of biotransformation in fish (the transformed km,Liver values in mammalian livers based on fish data are provided in S3 of the ESI†). Overall, longer half-lives produced higher log
BTF values. However, when the half-life exceeded 1 day, the log
BTF values reached the limit, and when the half-life exceeded 1000 day, the log
BTF values decreased. This can be explained by the positive association between the log half-life and log
KOW values of pesticides (Fig. A2†).
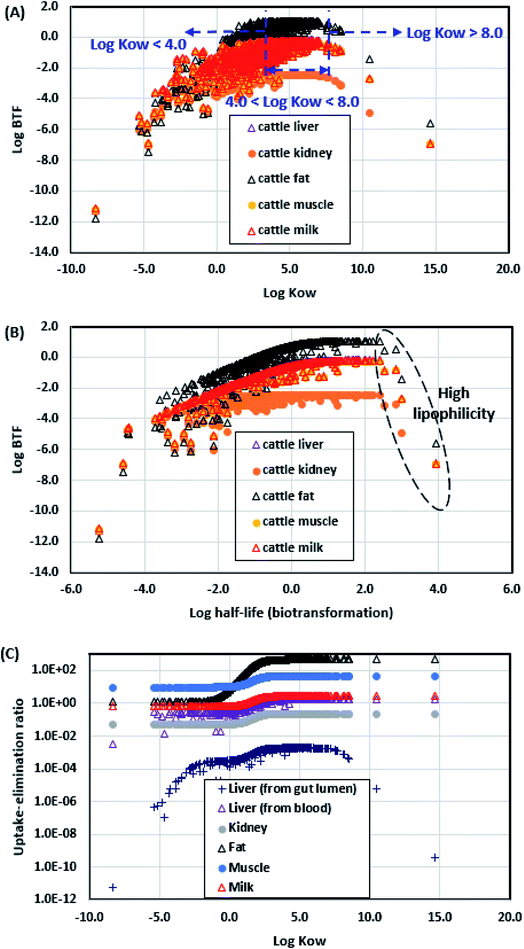 |
| Fig. 4 (A) Simulated logarithmic values of the biotransfer factors (log BTFs) of 736 pesticides in common cattle products (liver, kidney, fat, muscle, and milk) plotted against their log KOW values. (B) Simulated log BTF values of 736 pesticides in common cattle products plotted against the log half-life values of pesticides during biotransformation in fish (the transformed metabolic rate constants of pesticides in mammal livers are provided in S3 of the ESI†). (C) Simulated rate constant ratios of uptake to elimination (uptake-elimination ratio) for 736 pesticides in common cattle products. | |
3.2 Regulatory applications
To help derive MRLs based on human health and quantitative relationships of pesticide concentrations among livestock products, we simulated MRL factors of the 736 pesticides according to eqn (9a) and (b) based on a default value (0.01 mg kg−1 d−1) of the ADI (Fig. 5) for common cattle and sheep products, which can be used to derive MRLs by adjusting the ADI values of pesticides. The MRL factor of a pesticide is the allocation potential of the legal limit among the selected livestock products: a large MRL factor indicates that a higher MRL should be allocated to the livestock product. For lipophilic pesticides (i.e., high log
KOW values), the MRL factors of the fat are much larger than those of other livestock products, because lipophilic pesticides have higher potentials to be absorbed in the fat. Therefore, higher MRLs of lipophilic pesticides should be allocated to the fat compared to other livestock products; otherwise, the MRL of the fat could be too conservative to grant the pesticide concentrations that will not cause the adverse human health effect.
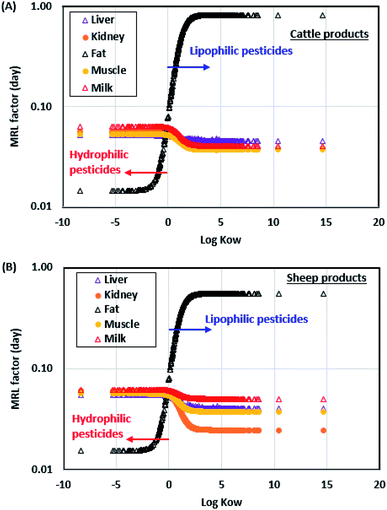 |
| Fig. 5 Simulated maximum residue level (MRL) factors of 736 pesticides for cattle (A) and sheep (B) products based on a default value (0.01 mg kg−1 d−1) of the acceptable daily intake (ADI) plotted against log KOW values. | |
To avoid the incompatibility of pesticide concentrations between the real-world (e.g., higher residue levels in fat than those in liver) and regulatory (e.g., lower MRLs in fat than those in kidney) scenarios, the proposed standard values (pesticide MRLs) should comply with the steady-state concentration ratios of the pesticide in livestock products. In the population, the consumption rate of the liver may be higher than that of the fat, which should lead to lower MRLs allocated to the liver if only the consumption rate of foods is considered. However, if the MRL of a lipophilic pesticide in the liver is much lower than that in the fat, the regulatory management of pesticide residues in the liver could challenge the legal limit of residues in the fat against the real residue levels. This is because theoretically, the residue levels of lipophilic pesticides in the fat are much higher than those in the liver after slaughtering. In addition to regulatory compatibility, the steady-state concentration ratios can serve as the initial indicators to further optimize MRLs in foods of animal origin considering the depletion and dissipation processes of the pesticide before human consumption.
Fig. 6 illustrates the recommended MRLs of chlorothalonil, ethephon, and glyphosate in five food products from cattle and sheep compared with the current EU MRLs [Reg. (EU) 2016/67].49 These three pesticides were selected because of its widespread use in agriculture,50–52 and they have large differences in lipophilicity (i.e., log
KOW). The recommended MRLs for chlorothalonil, ethephon, and glyphosate were calculated using eqn (9a) and (b) based on the ADI values49 of 0.015, 0.03, and 0.5 mg kg−1 d−1 and the CRi values from the standard food basket for mammals.11 In general, the EU defines the highest MRL in the kidney and the lowest MRL in fat for both cattle (bovine) and sheep. This is because the EU used the population consumption rates (i.e., exposure data) or field measurements (i.e., monitoring data) of mammal products to derived MRLs when the distribution and depletion process of pesticides cannot be determined,53 considering that fat has a high potential for pesticide absorption; therefore, the EU has allocated a lower MRL in fat than in other mammalian products.11 Although population health can be effectively protected using the human exposure risk model, low MRLs in fat could challenge the regulatory management of lipophilic pesticides (e.g., chlorothalonil). For instance, according to animal husbandry management, pesticide residues in animal feed must be controlled such that the residue levels in edible parts of the animal are lower than the MRLs.21 If the management of residue levels in animal feed can ensure that the residue levels in the muscle, liver, kidney, and milk are lower than the corresponding MRLs, the residue level in the fat is likely to exceed the corresponding MRL, particularly for lipophilic pesticides.22
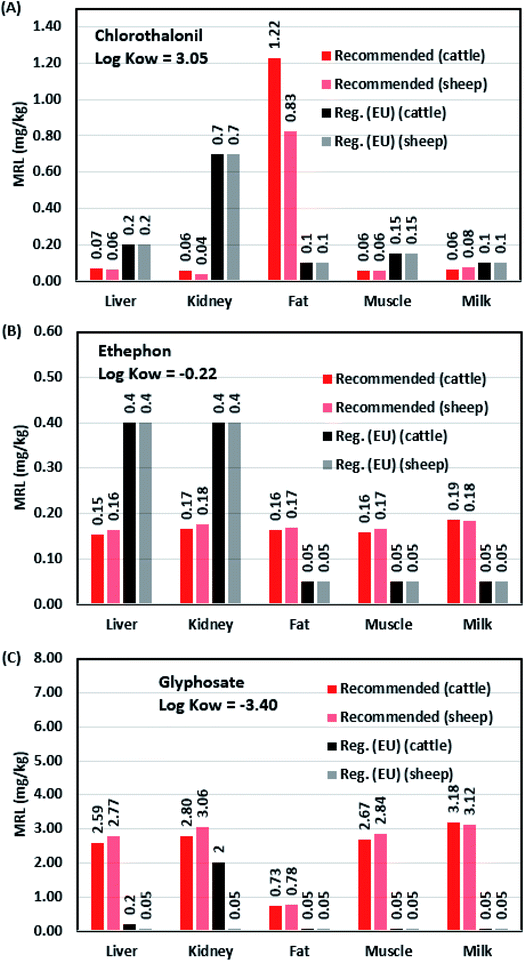 |
| Fig. 6 Recommended maximum residue levels (MRLs) of chlorothalonil (A), ethephon (B), and glyphosate (C) in common food products from cattle (bovine) and sheep compared with the current European Commission MRLs [Reg. (EU) 2016/67)]. | |
On the other hand, for hydrophilic or systemic pesticides (e.g., glyphosate and ethephon), higher MRLs are recommended to be allocated to other products (i.e., liver, kidney, muscle and milk), because they are not favorable to be absorbed in the fat but have high biotransfer potentials in other organ and tissues transported via blood flow throughout the body (i.e., large uptake-elimination ratios). Fig. 6B shows that the recommended MRLs of ethephon are similar among the selected products, which is due to its log
KOW close to zero. For glyphosate (Fig. 6C), EU defines the highest MRL in cattle kidney and the overall MRLs in cattle and sheep products are too conservative. This is because most MRLs are not defined in a risk- or science-based manner (e.g., detection limits or generic uncertainty factors for residues);54 and sometimes unnecessarily conservative MRLs could create the trade barrier, even though famers follow product labels and instructions to use pesticides for agricultural practice.55 Therefore, we suggest that regulatory agencies should consider applying the PBK model when defining MRLs, considering the theoretical steady-state concentration ratios of pesticide residues in animal products as the reference data.
3.3 Model evaluation
To evaluate the developed model, we compared the simulated BTFs with experimental data in the literature. Through extensive literature review, Rosenbaum et al.26 collected and categorized the daily intake dose-based BTFs of pollutants (i.e., ratio of steady-state pesticide concentration in food products to the daily intake dose of the residue) in cattle meat and milk. Thus, before comparing our results with the experimental data collected by Rosenbaum et al.,26 we adjusted the simulated BTFs according to the definition by Rosenbaum et al. and some current studies26,56 as follows: | 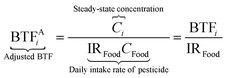 | (11a) |
|  | (11b) |
where BTFAi (mg kg−1 of pesticide in product i per mg d−1 of the pesticide daily intake, or mg kg−1 (mg d−1)−1) is the adjusted BTF in product i and BTFAMeat (mg kg−1 (mg d−1)−1) is the adjusted BTF of meat (lipid content, ∼0.25 g g−1), estimated using the mass-weighted adjusted BTFs of fat (lipid content, ∼0.8 g g−1) and muscle (lipid content, ∼0.028 g g−1) (Table A1†). Then, we evaluated the model by comparing the simulated BTFAi values of meat, muscle and milk with the experimental data collected by Rosenbaum et al.26
As shown in Fig. 7, the overall trends of the modeled and experimental log
BTFA values for meat, muscle, and milk are consistent in the lipophilicity-boosting (log
KOW < 4), lipophilicity-balancing (4 < log
KOW < 8), and lipophilicity-limiting phase (log
KOW > 8) phases. When the log
KOW is lower than 4, the simulated log
BTFA values are higher than the measured values. This is because our model was developed for predicting the steady-state concentrations of pesticides in animal tissues, whereas the previous studies were conducted under different experimental conditions. When the distribution of a compound in the cattle body does not reach a steady state, the measured concentration of that compound in meat and milk would be lower than that its steady-state concentration. For instance, the experimental length for collecting the BTFA data in milk ranged from 21 to 365 days. The measured log
BTFA values of DDT in milk were approximately −2.7 and −2.3 for the experimental length of 35 days and 365 days, respectively.26 In addition, different doses of compounds were administered to cattle during the experiment, which may affect the toxicokinetics of the compound in the cattle body.57 If the administered dose via the oral route is very large such that it exceeds the uptake capacity, the calculated log
BTFA values would be diluted. For instance, the measured concentration of chlordane in meat at 60 days was 0.1, 0.3, and 1 at the dosage rates of 16, 160, and 1600 mg d−1, respectively.58 When the log
KOW value exceeded 4 although the simulated log
BTFA values were within the ranges of the measured values, however, some simulated values of pesticides in meat are higher than some experimental result, which could be due to the reason that the steady state of chemicals in cattle bodies was not reached for some experiments. Also, lipid contents in the raw milk could vary among cattle breeds, which can have a high impact on the simulated results (Fig. A6†). In addition, our model applied biotransformation half-lives of pesticides in fish to estimate the metabolic rates in mammalian livers due to the information limitation, which could underestimate the metabolic rates for organic pollutants with relatively lower log
KOW values. We further evaluated the proposed model by comparing the means of the modeled log
BTFA values for pesticides in each log
KOW interval to those of the measured values (S10 of the ESI†), which showed the R2 values of over 0.8 and mean absolute errors less than 1 for meat, muscle, and milk. Therefore, comparison with the experimental data indicated that our simulation results presented similar trends as the measured values, and the variation among simulated and experimental values could be resolved by improving the metabolic rates of pesticides in mammal livers.
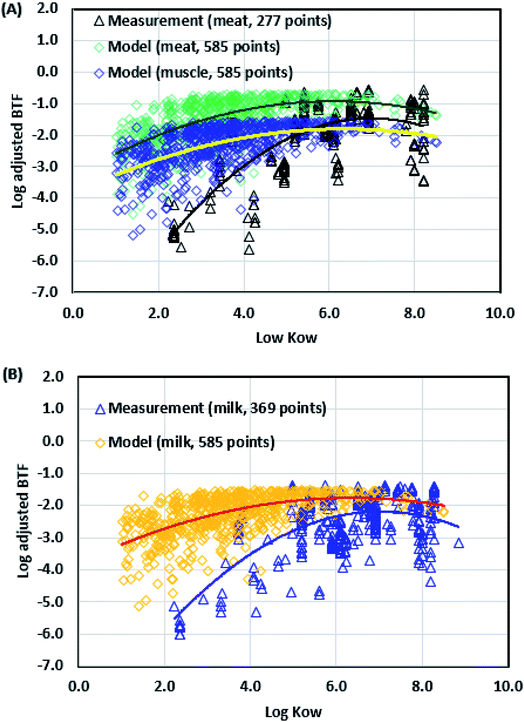 |
| Fig. 7 (A) Adjusted biotransfer factors (BTFs) of cattle meat (BTFAMeat) and muscle (BTFAMuscle) using the simple matrix algebra compared with the measurement data. (B) Adjusted BTFs of milk (BTFAMilk) compared with the measurement data. For cattle meat, the simulated BTFAMeat and BTFAMuscle data are plotted against log KOW values ranging from 1.02 to 8.51 and the measured compounds have log KOW values ranging from 2.23 to 8.21. For milk, the simulated BTFAMilk data are plotted against log KOW values ranging from 1.02 to 8.51 and the measured compounds have log KOW values ranging from 2.23 to 8.85. | |
3.4 Model limitations and recommendations
In the present study, we proposed a simple PBK model to simulate the steady-state BTFs of pesticides in raw foods of animal origin. Although the developed model may aid the screening-level risk assessment and regulatory management of pesticide residues in animal products after slaughter, we recommend some measures to improve consistency with the experimental data.
3.4.1 Improving matrix-based PBK model.
In this study, the proposed model applies the continuous pesticide intake rate, which assumes that there is always food stuff in the gut lumen of livestock. Although this continuous-process approach can simplify the simulation, the model should consider how much animal feed remains in the gut lumen and whether there is insufficient food to support the continuous process of pesticide uptake into the circulation system when the animal is sleeping. The physiological conditions of mammals (e.g., milk, biliary, and urine excretion) when sleeping differ from those in the daytime. Thus, we recommend considering a time-integrated approach to simulate the average concentrations of pesticides in raw foods of animal origin. The time-integrated approach had been successfully implemented in estimating fate factors of pesticides (i.e., average residue masses in environmental compartments) for the pulse-emission scenario.59 Once feeding and digestion patterns of livestock are known, the intermittent exposure to pesticides via food consumption can be equivalent to a pulse-like intake process, facilitating the BTF estimate using the matrix-based PBK approach.
In addition, the matrix-based PBK model is proposed to evaluate pesticide distributions in mammal bodies, whereas other domestic animals such as poultry and fish have different physiological systems from mammals. For example, mammals have mammary glands producing milk, so the secretion of milk is considered one of the elimination routes of pesticides in the PBK model. However, poultry (e.g., chickens and ducks) lay eggs, and some birds feed their young offspring by partially-digested food via regurgitation. By contrast, fish live underwater and breathe via gills, such that potential intake of pesticides by fish is dominated by the filter-feeding process, distinct from the digestion system of mammals.31,60 Therefore, different modeling structures from mammals are expected for poultry and fish to evaluate pesticide distributions inside the body.60,61 However, our proposed matrix-based approach can be flexibly coupled with PBK models for poultry and fish as long as the relevant processes (e.g., the egg-forming and filter-feeding processes for poultry and fish, respectively) are described using the first-order kinetics, and thereby performing high-throughput simulations of residue levels in poultry and fish products. Furthermore, the proposed model used blood transport as the only advection pathway to distribute pesticides in livestock bodies (tissues and organs). However, some chemical compounds (e.g., hydrophilic pesticides with high surface tension) are found to be also transported in a considerable manner via lymphatic or nervous systems,62,63 which could lead to underestimation of residue levels in lymphoid organs. Therefore, additional advective transport pathways via lymphatic or nervous systems are needed to be considered in the PBK model for hydrophilic compounds, which can be achieved by adding respective dimensions in the proposed matrix.
3.4.2 Metabolisms of pesticides in mammalian livers.
Due to data limitations, the metabolic rate constants of pesticides in the mammalian livers were approximated based on biotransformation half-lives in fish, which may be another reason the simulated BTF values were higher than the experimental data at low log
KOW values. Many weakly lipophilic pesticides (e.g., systemic pesticides) present much higher degradation rates in the environment than lipophilic pesticides, and they may present much higher metabolic rates in livestock bodies, including organs and tissues other than the liver. Also, the metabolites created in the liver should be evaluated, because this metabolic process can sometimes lead to more toxic chemicals (metabolites) before distribution in the rest of the animal. Although the distribution of the toxic metabolites in animal bodies can be simulated by incorporating the first-order kinetics of the generation process, information about generation rates and toxicities of metabolites should be determined in future studies. Thus, we recommend that once detailed information on the biotransformation process of pesticides in mammalian livers and other tissues is available, the metabolic rate constants as well as the metabolites of pesticides be incorporated to the corresponding compartments of our matrix approach.
3.4.3 Physiological variations of animals.
The proposed PBK model requires many physiological variables of livestock, which can vary substantially among species, varieties, and individuals, leading to variations in simulated BTFs. For example, Rossow et al.46 evaluated the impact of enzyme-treated feed on the production and nutrition composition of milk for dairy cows. We took the relevant physiological data measured by Rossow et al.46 to evaluate the uncertainty of the simulated BTFMilk values of pesticides (Table A6 and Fig. A7†). The results indicated that hydrophilic compounds (i.e., log
KOW < 0) had small variability of the simulated BTFMilk values among the selected dairy cows, whereas pesticides with moderate lipophilicity (i.e., log
KOW of ∼2.5) have large variability. This is due to solubility of pesticides with moderate lipophilicity in milk being more sensitive to the milk's nutrition composition (i.e., fat and water contents) as compared to hydrophilic pesticides, which makes milk production (i.e., elimination route of pesticides via milk excretion) have a greater impact on the simulated BTFMilk of the pesticides with moderate lipophilicity. Notably, other physiological variables such as organ mass and blood flow rate could also have considerable variations among animal individuals, leading to large uncertainty in the simulated BTFs. Pesticide properties (e.g., lipophilicity) and tissues vary widely. With our proposed approach, we enable the evaluation of a wide range of pesticide–animal tissue combinations, to which additional pathways and tissues can be conveniently added when related data become available.
4. Conclusions
In the present study, a screening-level model was proposed for simulating the BTFs of pesticides in foods of animal origin with a focus on mammals. The proposed model is based on matrix algebra using first-order kinetics. The simulated BTFs of pesticides in common livestock products (cattle and sheep) were parameterized and categorized according to log
KOW values into three lipophilicity phases. Comparison with experimental data in the literature indicated that the proposed model overestimated the BTFs of pesticides with relatively low log
KOW values, which can be attributed to several reasons, such as varying experimental conditions (e.g., experiment length and administrated pesticide doses) and limited information on metabolic rate constants. Nevertheless, the simulation results agreed with the experimental data on the general trends of pesticide BTFs over the log
KOW span. Our results indicate that the proposed model may serve as a tool for use in risk and life cycle impact assessment, in support of risk reduction for pesticides in food products as part of the global sustainability agenda for chemicals in consumer goods.64
Author contributions
Zijian Li: conceptualization, methodology, data curation, formal analysis, writing – original draft, writing – review & editing, resources, funding acquisition, project administration, supervision, read and approved the final version of the article. Jie Xiong: methodology, discussion, data curation, formal analysis. Peter Fantke: conceptualization, methodology, discussion, writing – review & editing, funding acquisition, supervision, read and approved the final version of the article.
Conflicts of interest
There are no conflicts to declare.
Acknowledgements
This study was financially supported the Sun Yat-sen University (grants 58000–18841211 and 58000-18841290), as well as by the SPRINT project (grant agreement no. 862568) funded under the European Union's Horizon 2020 Research and Innovation program, and by the “Safe and Efficient Chemistry by Design (SafeChem)” project funded by the Swedish Foundation for Strategic Environmental Research (grant no. DIA 2018/11).
References
- W. J. Doucette, C. Shunthirasingham, E. M. Dettenmaier, R. T. Zaleski, P. Fantke and J. A. Arnot, A review of measured bioaccumulation data on terrestrial plants for organic chemicals: metrics, variability, and the need for standardized measurement protocols, Environ. Toxicol. Chem., 2018, 37, 21–33 CrossRef CAS PubMed.
- M. S. McLachlan, Bioaccumulation of Hydrophobic Chemicals in Agricultural Food Chains, Environ. Sci. Technol., 1995, 30, 252–259 CrossRef.
- M. S. McLachlan, G. Czub, M. MacLeod and J. A. Arnot, Bioaccumulation of Organic Contaminants in Humans: A Multimedia Perspective and the Importance of Biotransformation, Environ. Sci. Technol., 2011, 45, 197–202 CrossRef CAS PubMed.
- M. Bagheri, K. Al-jabery, D. Wunsch and J. G. Burken, Examining plant uptake and translocation of emerging contaminants using machine learning: Implications to food security, Sci. Total Environ., 2020, 698, 133999 CrossRef CAS PubMed.
- C. Regrain, F. A. Zeman, M. Guedda, K. Chardon, V. Bach, C. Brochot, R. Bonnard, F. Tognet, L. Malherbe, L. Létinois, E. Boulvert, F. Marlière, F. Lestremau and J. Caudeville, Spatio-temporal assessment of pregnant women exposure to chlorpyrifos at a regional scale, J. Exposure Sci. Environ. Epidemiol., 2022, 32, 156–168 CrossRef CAS PubMed.
- P. Fantke, J. A. Arnot and W. J. Doucette, Improving plant bioaccumulation science through consistent reporting of experimental data, J. Environ. Manage., 2016, 181, 374–384 CrossRef PubMed.
- L. Luo, L. Dong, Q. Huang, S. Ma, P. Fantke, J. Li, J. Jiang, M. Fitzgerald, J. Yang, Z. Jia, J. Zhang, H. Wang, Y. Dai, G. Zhu, Z. Xing, Y. Liang, M. Li, G. Wei, J. Song, J. Wei, C. Peng, H. Zhang, W. Zhang, S. Wang, K. Mizuno, A. A. G. Marco, L. Wu, J. Xu, C. Xiong and S. Chen, Detection and risk assessments of multi-pesticides in 1771 cultivated herbal medicines by LC/MS-MS and GC/MS-MS, Chemosphere, 2021, 262, 127477 CrossRef CAS PubMed.
- L. Persson, B. M. Carney Almroth, C. D. Collins, S. Cornell, C. A. de Wit, M. L. Diamond, P. Fantke, M. Hassellöv, M. MacLeod, M. W. Ryberg, P. Søgaard Jørgensen, P. Villarrubia-Gómez, Z. Wang and M. Z. Hauschild, Outside the Safe Operating Space of the Planetary Boundary for Novel Entities, Environ. Sci. Technol., 2022, 56, 1510–1521 CrossRef PubMed.
- M. Kosnik, M. Z. Hauschild and P. Fantke, Toward assessing absolute environmental sustainability of chemical pollution, Environ. Sci. Technol., 2022 DOI:10.0.3.253/acs.est.1c06098.
- European Commission, EU – Pesticides database, https://ec.europa.eu/food/plant/pesticides/eu-pesticides-database/public/?event=homepage%26language=EN, accessed October 11, 2020.
-
European Commission,Volume 8: Notice to applicants and note for guidance: Establishment of maximum residue limits (MRLs) for residues of veterinary medicinal products in foodstuffs of animal origin. Notice of the European Community, Brussels, Belgium, 2003 Search PubMed.
-
FAO/WHO, Joint FAO/WHO Food Standards Programme Codex Committee on Pesticide Residues, Fortaleza, Brazil, 2006 Search PubMed.
- C. N. Legind, C. M. Kennedy, A. Rein, N. Snyder and S. Trapp, Dynamic plant uptake model applied for drip irrigation of an insecticide to pepper fruit plants, Pest Manage. Sci., 2011, 67, 521–527 CrossRef CAS PubMed.
- Y. Guo and Z. Li, A lognormal model for evaluating maximum residue levels of pesticides in crops, Environ. Pollut., 2021, 278, 116832 CrossRef CAS PubMed.
- I. A. O'Connor, M. A. J. Huijbregts, A. M. J. Ragas and A. J. Hendriks, Predicting the oral uptake efficiency of chemicals in mammals: Combining the hydrophilic and lipophilic range, Toxicol. Appl. Pharmacol., 2013, 266, 150–156 CrossRef PubMed.
- A. M. Taiwo, O. P. Talabi, A. A. Akintola, E. T. Babatunde, M. O. Olanrewaju, B. H. Adegbaju, S. L. Odebo, A. A. Bello, O. F. Matti, A. A. Adesanya and S. M. Ahmad, Evaluating the potential health risk of organochlorine pesticides in selected protein foods from Abeokuta southwestern Nigeria, Environ. Pollut. Bioavailability, 2020, 32, 131–145 CrossRef CAS.
- R. Weber, L. Bell, A. Watson, J. Petrlik, M. C. Paun and J. Vijgen, Assessment of pops contaminated sites and the need for stringent soil standards for food safety for the protection of human health, Environ. Pollut., 2019, 249, 703–715 CrossRef CAS PubMed.
-
European Commission, Directive 2002/32/EC of the European Parliament and of the Council of 7 May 2002 on undesirable substances in animal feed – Council statement, 2002 Search PubMed.
- P. Fantke, R. Friedrich and O. Jolliet, Health impact and damage cost assessment of pesticides in Europe, Environ. Int., 2012, 49, 9–17 CrossRef CAS PubMed.
- P. Fantke, W. A. Chiu, L. Aylward, R. Judson, L. Huang, S. Jang, T. Gouin, L. Rhomberg, N. Aurisano, T. McKone and O. Jolliet, Exposure and toxicity characterization of chemical emissions and chemicals in products: global recommendations and implementation in USEtoxInt, J. Life Cycle Assess., 2021, 26, 899–915 CrossRef CAS PubMed.
-
European Medicines Agency, Guideline on risk characterisation and assessment of maximum residue limits (MRL) for biocides, 2015 Search PubMed.
- W. R. Leeman, K. J. Van Den Berg and G. F. Houben, Transfer of chemicals from feed to animal products: The use of transfer factors in risk assessment, Food Addit. Contam., 2007, 24, 1–13 CrossRef CAS PubMed.
- F. Amutova, M. Delannoy, A. Baubekova, G. Konuspayeva and S. Jurjanz, Transfer of persistent organic pollutants in food of animal origin - Meta-analysis of published data, Chemosphere, 2021, 262, 128351 CrossRef CAS PubMed.
- L. S. Lautz, J. L. C. M. Dorne, R. Oldenkamp, A. J. Hendriks and A. M. J. Ragas, Generic physiologically based kinetic modelling for farm animals: Part I. Data collection of physiological parameters in swine, cattle and sheep, Toxicol. Lett., 2020, 319, 95–101 CrossRef CAS PubMed.
- L. S. Lautz, S. Hoeks, R. Oldenkamp, A. J. Hendriks, J. L. C. M. Dorne and A. M. J. Ragas, Generic physiologically based kinetic modelling for farm animals: Part II. Predicting tissue concentrations of chemicals in swine, cattle, and sheep, Toxicol. Lett., 2020, 318, 50–56 CrossRef CAS PubMed.
- R. K. Rosenbaum, T. E. McKone and O. Jolliet, CKow: A Dynamic Model for Chemical Transfer to Meat and Milk, Environ. Sci. Technol., 2009, 43, 8191–8198 CrossRef CAS PubMed.
- M. S. McLachlan, Model of the fate of hydrophobic contaminants in cows, Environ. Sci. Technol., 1994, 28, 2407–2414 CrossRef CAS PubMed.
- Z. Li, Spatiotemporal pattern models for bioaccumulation of pesticides in herbivores: an approximation theory for North American white-tailed deer, Sci. Total Environ., 2020, 737, 140271 CrossRef CAS PubMed.
- B. C. Kelly and F. A. P. C. Gobas, An arctic terrestrial food-chain bioaccumulation model for persistent organic pollutants, Environ. Sci. Technol., 2003, 37, 2966–2974 CrossRef CAS PubMed.
- F. A. P. C. Gobas, L. P. Burkhard, W. J. Doucette, K. G. Sappington, E. M. J. Verbruggen, B. K. Hope, M. A. Bonnell, J. A. Arnot and J. V. Tarazona, Review of existing terrestrial bioaccumulation models and terrestrial bioaccumulation modeling needs for organic chemicals, Integr. Environ. Assess. Manage., 2016, 12, 123–134 CrossRef CAS PubMed.
- F. A. P. C. Gobas and J. A. Arnot, Food web bioaccumulation model for polychlorinated biphenyls in San Francisco Bay, California, USA, Environ. Toxicol. Chem., 2010, 29, 1385–1395 CAS.
- P. H. Howard and D. C. G. Muir, Identifying New Persistent and Bioaccumulative Organics Among Chemicals in Commerce. III: Byproducts, Impurities, and Transformation Products, Environ. Sci. Technol., 2013, 47, 5259–5266 CrossRef CAS PubMed.
- D. Mackay, A. K. D. Celsie, D. E. Powell and J. M. Parnis, Bioconcentration, bioaccumulation, biomagnification and trophic magnification: a modelling perspective, Environ. Sci.: Processes Impacts, 2018, 20, 72–85 RSC.
- A. Celsie, D. Mackay, J. M. Parnis and J. A. Arnot, A fugacity-based toxicokinetic model for narcotic organic chemicals in fish, Environ. Toxicol. Chem., 2016, 35, 1257–1267 CrossRef CAS PubMed.
-
D. Li, Physiologically Based Pharmacokinetic Modeling for Exposure and Risk Assessment, in Encyclopaedia of Environmental Health, 2nd edn, 2019, pp. 233–238 Search PubMed.
- D. Li, G. Johanson, C. Emond, U. Carlander, M. Philbert and O. Jolliet, Physiologically based pharmacokinetic modeling of polyethylene glycol-coated polyacrylamide nanoparticles in rats, Nanotoxicology, 2014, 8, 128–137 CrossRef CAS PubMed.
- Z. Dong, X. Fan, W. Fan, H. Chang, Y. Xu, T. Li and M. Su, Pkweb: An online application for pharmacokinetic data analysis, Environ. Technol. Innovation, 2020, 19, 100838 CrossRef.
- Z. Dong, C. Liu, Y. Liu, K. Yan, K. T. Semple and R. Naidu, Using publicly available data, a physiologically-based pharmacokinetic model and Bayesian simulation to improve arsenic non-cancer dose-response, Environ. Int., 2016, 92, 239–246 CrossRef PubMed.
- Z. Dong, T. Li, Y. Wan, Y. Sun and J. Hu, Physiologically Based Pharmacokinetic Modeling for Chlorinated Paraffins in Rats and Humans: Importance of Biliary Excretion, Environ. Sci. Technol., 2019, 54, 938–946 CrossRef PubMed.
-
P. Ciffroy and A. Radomyski, The MERLIN-Expo Mammal model V1.1, 2021 Search PubMed.
- J. A. Arnot, W. Meylan, J. Tunkel, P. H. Howard, D. Mackay, M. Bonnell and R. S. Boethling, A quantitative structure-activity relationship for predicting metabolic biotransformation rates for organic chemicals in fish, Environ. Toxicol. Chem., 2009, 28, 1168–1177 CrossRef CAS PubMed.
-
P. Fantke, M. Huijbregts, M. Margni, M. Hauschild, O. Jolliet, T. McKone, R. Resenbaum and D. van de Meent, USEtox 2.0 User Manual (v2), 2015 Search PubMed.
- K. A. Lewis, J. Tzilivakis, D. J. Warner and A. Green, An international database for pesticide risk assessments and management, Hum. Ecol. Risk Assess., 2016, 22, 1050–1064 CrossRef CAS.
- A. J. Williams, C. M. Grulke, J. Edwards, A. D. McEachran, K. Mansouri, N. C. Baker, G. Patlewicz, I. Shah, J. F. Wambaugh, R. S. Judson and A. M. Richard, The CompTox Chemistry Dashboard: a community data resource for environmental chemistry, J. Cheminf., 2017, 9, 1–27 Search PubMed.
- Z. Li, Approximate Modeling of the Uptake of Pesticides by Grass for Grazing Risk Assessment and Pasture Management, ACS Agric. Sci. Technol., 2021, 1, 338–346 CrossRef CAS.
- H. A. Rossow, H. M. Golder and I. J. Lean, Variation in milk production, fat, protein, and lactose responses to exogenous feed enzymes in dairy cows, Applied Animal Science, 2020, 36, 292–307 CrossRef.
- F. A. P. C. Gobas, D. C. G. Muir and D. Mackay, Dynamics of dietary bioaccumulation and faecal elimination of hydrophobic organic chemicals in fish, Chemosphere, 1988, 17, 943–962 CrossRef CAS.
- S. D. Dimitrov, N. C. Dimitrova, J. D. Walker, G. D. Veith and O. G. Mekenyan, Predicting bioconcentration factors of highly hydrophobic chemicals. Effects of molecular size, Pure Appl. Chem., 2002, 74, 1823–1830 CrossRef CAS.
- U Pesticides database, https://ec.europa.eu/food/plant/pesticides/eu-pesticides-database/mrls/?event=search.pr, accessed July 28, 2021.
- A. Santovito, C. Gendusa, F. Ferraro, I. Musso, M. Costanzo, S. Ruberto and P. Cervella, Genomic damage induced by the widely used fungicide chlorothalonil in peripheral human lymphocytes, Ecotoxicol. Environ. Saf., 2018, 161, 578–583 CrossRef CAS PubMed.
- L. Li, W. Gu, S. Zuo, Y. Meng, C. Li, W. Li, Z. Zhang and S. Wei, Effects of thidiazuron and ethephon on the grain filling and dehydration characteristics of maize in Northeast China, Arch. Agron. Soil Sci., 2020, 1–17 Search PubMed.
- L. Lupi, F. Bedmar, M. Puricelli, D. Marino, V. C. Aparicio, D. Wunderlin and K. S. B. Miglioranza, Glyphosate runoff and its occurrence in rainwater and subsurface soil in the nearby area of agricultural fields in Argentina, Chemosphere, 2019, 225, 906–914 CrossRef CAS.
- European Commission, Regulation (EC) No 470/2009 of the European parliament and of the council of 6 May 2009 laying down Community procedures for the establishment of residue limits of pharmacologically active substances in foodstuffs of animal origin, repealing Council Regulation (EEC) No 2377/90 and amending Directive 2001/82/EC of the European Parliament and of the Council and Regulation (EC) No 726/2004 of the European Parliament and of the Council, https://eur-lex.europa.eu/LexUriServ/LexUriServ.do?uri=OJ, L:2009:152:0011:0022:en:PDF, accessed August 7, 2021.
- A. Brancato, D. Brocca, L. Carrasco Cabrera, C. De Lentdecker, Z. Erdos, L. Ferreira, L. Greco, S. Jarrah, D. Kardassi, R. Leuschner, A. Lostia, C. Lythgo, P. Medina, I. Miron, T. Molnar, R. Pedersen, H. Reich, A. Sacchi, M. Santos, A. Stanek, J. Sturma, J. Tarazona, A. Theobald, B. Vagenende and L. Villamar-Bouza, Review of the existing maximum residue levels for fenbuconazole according to Article 12 of Regulation (EC) No 396/2005, EFSA J., 2020, 18, e06059 Search PubMed.
-
M. T. Yeung, W. A. Kerr, B. Coomber, M. Lantz and A. McConnell, Declining International Cooperation on Pesticide Regulation, Springer International Publishing, Cham, 2017 Search PubMed.
- M. Rose, A. Fernandes, C. Foxall and A. Dowding, Transfer and uptake of polychlorinated dibenzo-p-dioxins and furans (PCDD/Fs) and polychlorinated biphenyls (PCBs) into meat and organs of indoor and outdoor reared pigs, Food Addit. Contam., Part A, 2012, 29, 431–448 CAS.
-
R. C. Gupta, Veterinary Toxicology: Basic and Clinical Principles, 3rd edn, 2018 Search PubMed.
- H. W. Dorough and R. W. Hemken, Chlordane residues in milk and fat of cows fed HCS 3260 (high purity chlordane) in the diet, Bull. Environ. Contam. Toxicol., 1973, 10(4), 208–216 CrossRef CAS PubMed.
- R. K. Rosenbaum, M. Margni and O. Jolliet, A flexible matrix algebra framework for the multimedia multipathway modeling of emission to impacts, Environ. Int., 2007, 33(5), 624–634 CrossRef PubMed.
- J. A. Arnot and F. A. P. C. Gobas, A Food Web Bioaccumulation Model For Organic Chemicals In Aquatic Ecosystems, Environ. Toxicol. Chem., 2004, 23, 2343 CrossRef CAS PubMed.
- L. S. Lautz, C. Nebbia, S. Hoeks, R. Oldenkamp, A. J. Hendriks, A. M. J. Ragas and J. L. C. M. Dorne, An open source physiologically based kinetic model for the chicken (Gallus gallus domesticus): Calibration and validation for the prediction residues in tissues and eggs, Environ. Int., 2020, 136, 105488 CrossRef CAS PubMed.
- J. C. Turner and V. Shanks, Absorption of some organochlorine compounds by the rat small intestine-In vivo, Bull. Environ. Contam. Toxicol., 1973, 10, 208–216 CrossRef PubMed.
-
R. E. Baynes and J. E. Riviere, in Hayes' Handbook of Pesticide Toxicology, Elsevier, 2010, pp. 877–892 Search PubMed.
- P. Fantke and N. Illner, Goods that are good enough: Introducing an absolute sustainability perspective for managing chemicals in consumer products, Curr. Opin. Green Sustain. Chem., 2019, 15, 91–97 CrossRef.
Footnote |
† Electronic supplementary information (ESI) available. See DOI: 10.1039/d1em00454a |
|
This journal is © The Royal Society of Chemistry 2022 |