The mechanism for acetate formation in electrochemical CO(2) reduction on Cu: selectivity with potential, pH, and nanostructuring†
Received
8th May 2022
, Accepted 1st August 2022
First published on 3rd August 2022
Abstract
Nanostructured Cu catalysts have increased the selectivities and geometric activities for high value C–C coupled (C2) products (ethylene, ethanol, and acetate) in the electrochemical CO(2) reduction reaction (CO(2)RR). The selectivity among the high-value C2 products is also altered, where for instance the yield of acetate increases with alkalinity and is dependent on the catalyst morphology. The reaction mechanisms behind the selectivity towards acetate vs. other C2 products remain controversial. In this work, we elucidate the reaction mechanism for acetate formation by using ab initio simulations, a coupled kinetic-transport model, and loading dependent experiments. We find that trends in acetate selectivity can be rationalized from variations in electrolyte pH and the local mass transport properties of the catalyst and not from changes in Cu's intrinsic activity. The selectivity mechanism originates from the transport of ketene, a stable (closed shell) intermediate, away from the catalyst surface into solution where it reacts to form acetate. While this type of mechanism has not yet been discussed in the CO(2)RR, variants of it may explain similar selectivity fluctuations observed for other stable intermediates like CO and acetaldehyde. Our proposed mechanism suggests that acetate selectivity increases with increasing pH, decreasing catalyst roughness and significantly varies with the applied potential.
Broader context
The electrochemical reduction of CO2 enables the storage of renewable energy in carbon neutral fuels while simultaneously reducing greenhouse gas emissions. Recent developments have led to industrially relevant performances exploiting nanostructured Cu catalysts and gas-diffusion electrodes, which produce high value C2 products at high current densities. The selectivity between these C2 products, which mainly consist of ethylene, ethanol and acetate, still poses a challenge to maximize cost efficiency. In this joint experimental–theoretical work, we elucidate the selectivity mechanism towards acetate vs. ethylene and ethanol using a multi-scale modelling approach with excellent agreement to experiments. We determine that the transport properties at the catalyst surface are pivotal in steering the selectivity between acetate and other C2 products. While our insights deduce design rules for an acetate rich or poor CO2 reduction process important for a pure liquid product stream, they can be generalized to understand selectivity changes with catalyst roughness of other products and processes.
|
1 Introduction
The electrochemical reduction of carbon dioxide (CO2RR) or carbon monoxide (CORR) has the potential to enable the storage of renewable energy via the production of carbon neutral commodity chemicals. Cu electrocatalysts have thereby been extensively explored for the CO(2)RR, since Cu is the only material able to produce high value C–C coupled (C2) products (ethylene, ethanol and acetate).1 Improvements in activity and selectivity of these catalysts are urgently needed to enable the commercialization and cost-effective operation of CO(2)RR electrolyzers.2,3 To this end, two key developments have been made over the last decade. First, gas diffusion electrodes (GDE) in combination with flow cell setups achieve significantly higher current densities towards CO(2)RR products over traditional H-cell setups, which suffer from mass transport limitations.4–6 Second, various means of nanostructuring Cu have been explored in order to increase the active surface area and to tune the selectivity towards high value products.1,7–10 For instance, nanostructured catalysts in a GDE/flow-cell setup have led to significantly increased selectivities towards acetate under alkaline conditions.11,12 Originally considered a minor product, under these specific conditions, acetate has Faradaic efficiencies comparable to those of ethylene and ethanol, the two major C2 products produced by Cu under neutral and mildly alkaline conditions.4,11,13 To date, there is no consensus on how nanostructuring or mass transport impacts the intrinsic activity and selectivity of Cu towards the possible C2 products.9,10
In this paper, we suggest that nanostructuring of Cu changes the selectivity for acetate vs. other C2 products through altering the mass transport of products away from the electrode, and not through changes in intrinsic activity via new active sites.12 We suggest that acetate forms via a solution reaction of ketene with OH−, which is heavily affected by the transport and electrolyte pH. Our insight results from a comprehensive examination of the reported experimental activities of Cu catalysts normalized to the active surface area, an ab initio derived microkinetic model coupled to mass transport, and through activity measurements on different Cu catalysts in a range of pH and loadings.
Previous computational studies have attempted to rationalize the acetate activity and selectivity through reaction thermodynamics and barriers.11,12,14 However, the dependence of acetate selectivity on pH and potential remained an open question, and performance differences in various Cu samples were assumed to arise from different active sites. We suggest that such mechanisms are inconsistent with the electrochemically active surface area (ECSA)-normalized activity of all reported Cu catalysts and the potential dependence of the observed selectivity. In contrast, our computational model shows that the interplay of kinetics and mass transport determines the acetate selectivity vs. other C2 products. The model agrees with the experimentally observed trends in the dependence of acetate selectivity on potential, electrolyte pH, and catalyst roughness. Previously, transport limitations have already been suggested to alter the selectivity behavior between CO(2)RR products and H2 by affecting the reactant concentrations and local pH at the catalyst surface.6,15–20 This work showcases how mass transport also controls the competition between the desorption and the further reduction of stable intermediates with a corresponding impact on selectivity. This concept has been similarly proposed for stable intermediates in the oxygen reduction reaction (ORR) and methanol oxidation.21 This principle is, therefore, generalizable to controlling the selectivity of other stable intermediates in the CO(2)RR like CO and acetaldehyde. Overall, our combined analysis of experiments and simulations suggests that acetate selectivity against other C2 products is optimized by a high interfacial and bulk pH and a low, microscopic surface roughness of the Cu catalyst, which should be considered in the design of CO(2)RR electrolyzers.
2 Results & discussion
2.1 ECSA normalized current densities suggest no change in intrinsic activity toward C2 products with nanostructuring
We compare ECSA-normalized current densities, jECSA, to evaluate CO(2)RR activities and selectivities, in Fig. 1. jECSA is the best available estimate for the intrinsic activity in the absence of mass transport limitations, as turnover frequencies (TOF), which reflect the true intrinsic activity per active site, are generally not accessible in electrochemical measurements.1,10,22 Within the variations in active site densities amongst the catalysts considered, jECSA is proportional to the turnover frequency.23
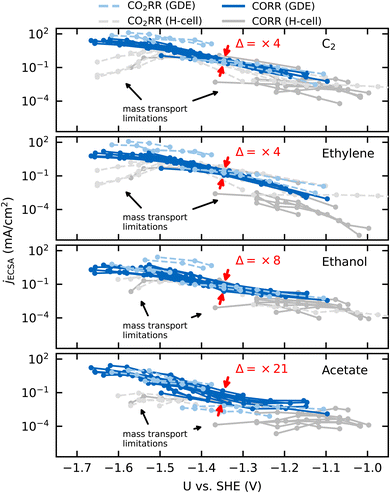 |
| Fig. 1 ECSA normalized partial current densities against USHE for C2 (top), ethylene (upper center), ethanol (lower center), and acetate (bottom) adapted from different CO2RR (dashed lines) and CORR (solid lines) studies conducted in a GDE/flow-cell setup (light-blue/blue) or a conventional H-cell setup (light-gray/gray). Each line represents an individual data set of the same reaction conditions. The GDE data is adapted from ref. 4, 11 and 25–29 and the H-cell data from ref. 7, 8, 30, 32 and 33. The red arrows indicate the (multiplicative) factor in variation of jECSA(Δ) at −1.35VSHE. | |
Fig. 1 shows jECSA for various Cu catalysts in both GDE and H-cell setups towards the sum of all C2 products in CO(2)RR and specifically toward ethylene, ethanol, and acetate. Even under a wide range of reaction conditions (CO and CO2 as the reactant, different electrolytes with pH ranging from 6.8 to 14.3, wide potential range) and catalyst morphologies (from Cu single crystals and foils to deliberately roughened oxide-derived (OD) Cu, nanowires and nanoparticles), we find the currents to overlap within an order of magnitude for data sets without obvious transport limitations (blue lines in Fig. 1, which all correspond to GDE setups). H-cell setups, where transport limitations are present at lower overpotentials (gray lines in Fig. 1), show larger deviations.
Recent reviews have pointed out the similar intrinsic activities amongst different types of Cu catalysts towards all CO(2)RR products.1,10 We confirm this observation in Fig. 1, and, in addition, we highlight the similar activity of different Cu catalysts towards the individual major C2 products. The minor variation in partial current densities in Fig. 1 suggests a uniform intrinsic activity towards the different products and therefore the same active site on the different catalysts (when following typical preparation procedures24). If the order-of-magnitude change in partial current densities was to arise instead from a change in active site, the difference in the activation energies towards a given product would be around ≤0.05–0.10 eV, assuming similar active site densities (and following the Arrhenius law). The expected electronic effects of changing an active site are, however, usually between 0.3 and 1.0 eV23 and would result in significantly larger variation (Δ) of 5 to 15 orders of magnitude in the TOF and corresponding partial current densities. Such large variations stand in contrast to the minor variations (Δ) of factor 4 to 21 as indicated in Fig. 1. Therefore, we hypothesize that possible changes in selectivity (contained in minor variations in activity between different C2 products) arise only due to changes in active site densities (or respectively, the uncertainty in the determined ECSA), electrolyte pH or transport limitations.
Fig. 1 also shows a subtly stronger variation of the acetate currents in comparison to ethylene and ethanol although all C2 products follow a similar potential behavior (or Tafel slope). While the former is a function of the electrolyte pH and catalyst morphology (see below), the latter indicates the same rate determining step for these C2 products. In what follows, we derive a comprehensive mechanism for acetate formation which rationalizes the dependence of its activity on pH and catalyst morphology.
2.2
Ab initio calculations suggest a solution phase reaction of ketene and OH− towards acetate
Fig. 2(a) shows our proposed mechanism of the selectivity determining steps (SDS) towards acetate vs. other major C2 products, determined on the basis of DFT simulations. Central to this mechanism is the ketene intermediate as suggested previously.8,11,14 However, we emphasize its importance in both its desorbed (H2CCO(aq)) and adsorbed (H2CCO*) state. As shown in Fig. 2(a and b), a proton-coupled electron transfer (PCET) to HCCO* leads to the formation of the stable ketene molecule, desorbed from the surface. This reaction step is pivotal because past its formation, H2CCO(aq) can either react with OH− to acetate in solution (a well-known reaction34) or readsorb and get further reduced to other C2 products. Either pathway is differently influenced by pH, (ketene) transport, and potential. The elementary reactions making up this branching point evolve around two SDS as indicated in Fig. 2(a):
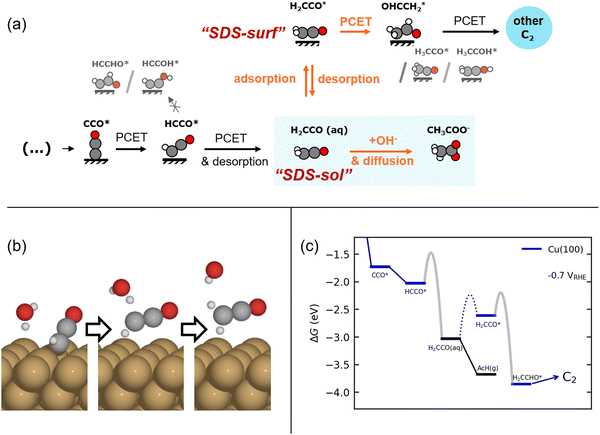 |
| Fig. 2 (a) Schematic of the ketene pathway. We show the mechanism we investigated in our model, i.e. after the RDS and including the two selectivity-determining steps, “SDS-sol” and “SDS-surf”' towards acetate via a solution reaction or the further reduction of the adsorbed ketene (H2CCO*) to form other C2 products on the catalyst surface. We also depict the excluded alternative reduction steps. (b) Illustration of the simultaneous desorption and reduction of HCCO* → H2CCO(aq) on Cu(100) as obtained from CI-NEB simulations. (c) Free energy diagram for the suggested ketene pathway on Cu(100) at −0.7 V vs. RHE (pH = 14). Depicted is the considered division into the pathways including reduction of H2CCO* (blue) and the desorption of ketene and its hydrolysis to acetate (black), respectively. The adsorption barrier of ketene (dotted) and the approximated electrochemical barriers (gray, see Methods) are shown. Free energy diagrams for Cu(111), Cu(110), and Cu(211) are shown in Fig. S12 (ESI†). | |
1. (SDS-surf, only low overpotentials) The PCET reaction of H2CCO* towards other C2 products vs. the desorption of re-adsorbed ketene.
2. (SDS-sol) The solution reaction vs. re-adsorption of desorbed ketene.
Firstly, we note that SDS-sol is dependent on the concentration of H2CCO(aq) and OH− at the catalyst surface which depends strongly on mass transport. Secondly, we note that SDS-sol involves the competition between two chemical steps while SDS-surf between a chemical and an electrochemical step. Acetate selectivity of SDS-sol is therefore not (directly) potential dependent in contrast to SDS-surf. The concentration and potential (in)dependence have an important impact on the selectivity, as we detail below.
We derive and validate this mechanism using density functional theory (DFT) calculations. In our derivation, we assume the same rate determining step (RDS) for all C2 products based on previous experimental and theoretical studies, which has been suggested to either be a CO* dimerization35–37 or the protonation of the OCCO* intermediate with water as the proton donor.38 The same RDS is consistent with the range of similar Tafel slopes and the distinguishing lack of an inverse pH-activity relationship shared among the considered C2 products (ethylene, ethanol, acetate)11 (see Fig. 1 above and elaboration of the pH effect in the ESI,† Section S6). Such a shared and early RDS means that the energetics of the following intermediates have no effect on the activity towards the sum of all C2 products, and only affect the selectivity amongst them (i.e.
, with x being a specific product of the SDS).39 We evaluate our suggested reaction mechanism on representative facets: Cu(111) and Cu(100) as terraces and Cu(110) and Cu(211) as steps. We choose intermediates prior to HCCO* (and thus ketene) based on their thermodynamic or kinetic stability which is in line with established trends.40 On all facets considered, the free energies of all steps are mostly downhill and any uphill step is well below 0.75 eV as shown in Fig. 2(c) and Fig. S12 (ESI†) which makes the mechanism feasible at room temperature.23 We also evaluated the chemical and PCET barriers at a constant potential41 (see Methods and Section S3 in the ESI†) around SDS-sol and SDS-surf which are also low enough to lead to measurable rates at room temperature (see Fig. 2(c) and Fig. S12, Table S6 in the ESI†). An only exception is an insurmountable re-adsorption barrier for ketene on Cu(111) and Cu(110), which makes these facets inactive for the proposed mechanism to other C2 products (see Fig. S12 and Section S5.6 in the ESI†). We emphasize that the direct HCCO* → H2CCO(aq) step was an explicit result from the transition state search and occurs on all investigated facets.
2.3 Acetate selectivity is determined by the interplay of kinetics, mass transport, and electrode roughness
To investigate the selectivity behavior we couple our DFT-based energetics with both a microkinetic and a mass transport model. The microkinetic model describes the surface reactions of our proposed reaction mechanism to acetate and other C2 products (see Fig. 2 and the ESI,† Section S5.1). The mass transport model describes the transport according to the conditions of CORR operation in a GDE/flow cell setup (with corresponding pH, reactants, and diffusion lengths), where the highest selectivity for acetate is observed.4,8,11,25,30 We only consider the dominant pathway for the acetate formation (i.e. CO(2)RR at cathode) in this study, although acetate could also be formed through a partial oxidation of ethanol (the product of CO(2)RR) at the anode in certain cell configurations due to its cross-over through membranes.25,42
In our microkinetic model we fit the common RDS for the C2 products to the ECSA normalized activity found in experiments (see the ESI,† Section S5.1). Furthermore, we apply our DFT-derived energies for Cu(100) in the microkinetic model, whereby the quantitative behavior of the model is only sensitive to the re-adsorption, desorption, and reduction barrier of the ketene intermediate and insensitive to any other facet dependent energy (see the ESI,† Section S5.6). We note that we fitted the free energy of H2CCO(aq) at the interface to reproduce the experimental selectivity, as it is poorly represented by the static electrode–solvent interface applied in our calculations. This fit leads to an energy correction of 0.29 eV which is within DFT uncertainty on the one hand but also reproduces the adsorption barrier height on Cu(211) on the other hand, and is therefore a reasonable assumption (see the ESI,† Section S5.6.1 and Fig. S18).
For the description of the mass transport, we use a 1D-mass transport model where we account for the diffusion of CO, OH−, and H2CCO as well as the solution reaction of H2CCO and OH− to acetate,19,43 as illustrated by Fig. 3 and Fig. S14 (ESI†). We also include a Sherwood relation to account for the convective mass transport encountered in a flow cell. It describes the diffusion of species from the bulk of the flowing electrolyte to the electrode surface.44–46 All constants and coefficients are taken from the literature, for details refer to Methods and the ESI,† Section S5. Within our 1D approximation, the effect of the nanostructuring of Cu is captured only by a single roughness factor ρ, and we assume a constant active site density. The roughness ρ generally describes the ratio of active area and geometric area (AECSA/Ageo). This ratio can change microscopically with local curvature and porosity of the nanostructured Cu surface or mesoscopically with the loading of Cu particles on the GDE. As discussed in detail later, the short diffusion length scales in the presence of the rapid solution reaction treated here, effectively define ρ to be a microscopic roughness.
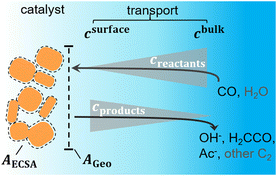 |
| Fig. 3 Schematic of the mass transport considered in our model. CO, OH−, H2CCO, and Acetate (Ac−) (black color) are considered for transport while H2O and other C2 are neglected (grey color). Typical concentration gradients are depicted and it is indicated how the transport scales with geometric area AGeo while processes on the catalyst surface scale with AECSA. | |
The included mass transport limitations give rise to changes in the microscopic reaction environment at the catalyst surface through changes in the surface concentrations of individual species (in our case of CO, OH−, and H2CCO). Their local concentrations decisively affect the coupled reaction kinetics. For example, increasing flux and current densities at higher overpotentials give rise to increased concentration gradients, such that the surface concentrations of reactants (CO) decrease while those of the electrochemical products (OH− and H2CCO) increase (compare Fig. 3). The concentration profiles are further affected by pH and ρ as discussed in detail in the ESI,† Section S5.5 which importantly influence acetate selectivity trends. These relationships finally enable the simulation of the C2 current and selectivity dependent on potential, bulk pH and catalyst roughness ρ as depicted in Fig. 4(a).
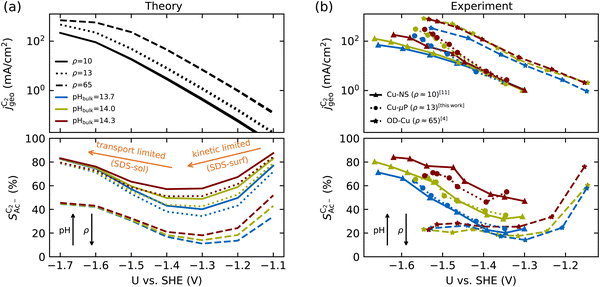 |
| Fig. 4 Geometric current densities towards all C2 products ( , top) and acetate selectivity among them ( , bottom) against USHE as (a) simulated by our transport coupled microkinetic model and (b) experimentally measured. Each line represents an individual data set with the same reaction conditions. The different colors represent varying electrolyte pH and the solid, dotted, and dashed lines experiments and simulations with Cu-NS or ρ = 10, Cu-μP or ρ = 13, and OD-Cu or ρ = 65, respectively. The experimental data has been measured in this work or is adapted from ref. 4 and 11. Note that the simulated is invariant to pH as it follows the pH independent RDS of the C2 products and competition to C1 products like methane and the hydrogen evolution reaction or cation effects47 are not included in our model, the simulated is therefore depicted in black. The simulated for ρ = 10 was scaled by ½ according to the lower loading used for the Cu-NS of 0.5 mg cm−2 compared to 1.0 mg cm−2.4,11 The dominating selectivity mechanisms including kinetic and transport competition are indicated (see the text). | |
The simulated geometric current densities of C2 products (
) are shown in the top panel of Fig. 4(a). The maximum
reached at high overpotentials (more negative U) reflects the CO diffusion limited current density (see Fig. S17a, showing the depletion of CO close to the surface, ESI†). At lower overpotentials, the linear dependence of
on the catalyst ρ reflects the linear dependence of
on AECSA.
The bottom panel in Fig. 4(a) shows the computed selectivity of acetate vs. the total C2 products
. We emphasize that the shown selectivities, being molar ratios relating between C2 products, differ from Faradaic efficiencies (FE) which are weighted by transferred electrons and therefore ambiguous for mechanistic analysis. In general, we distinguish the critical variables affecting
as the roughness ρ and the (interfacial) pH. These variables depend on catalyst morphology and on electrolysis conditions. The resulting trends arise from the competition between the transport/solution reaction and re-adsorption of H2CCO(aq) in SDS-sol (see Fig. 2(a)):
• An increasing roughness ρ lowers
since an increased catalyst surface area (AECSA) favors H2CCO(aq) adsorption. Note that the area which affects the transport and solution reaction towards acetate (Ageo) remains unchanged.
• An increasing concentration [OH−] increases
since OH− catalyzes the solution reaction of ketene to acetate. This selectivity behavior confirms a first order dependence of acetate formation with OH−. The interfacial pH (which scales with the bulk pH) is decisive because the conversion of ketene occurs close to the catalyst surface (as elaborated below).
scales stronger with the roughness and weaker with pH (compare Fig. 4) following the relationship
| 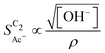 | (1) |
as we derive in the ESI,
† Section S7. The U-shaped selectivity curve
vs. potential can be explained by the interplay of SDS-sol and SDS-surf.
1. At low overpotentials: the selectivity towards other C2 products is limited by the potential dependent protonation of H2CCO* (in SDS-surf) while the step towards acetate is potential independent. Therefore, as the overpotential increases, the selectivity towards acetate decreases.
2. At high overpotentials: the protonation of H2CCO* is facile. The selectivity is then determined by H2CCO(aq) readsorption vs. the solution phase reaction of H2CCO(aq) with OH− (in SDS-sol). The build-up of local OH− concentration at increasing overpotentials (local pH, see the concentration profile in Fig. S17b, ESI†) favors the latter reaction and leads to an uptick in acetate selectivity.
The complex selectivity behavior of our simulations is consistent with experiments as shown in Fig. 4(b). We compare it to experimental data measured in this work and data adapted from ref. 4 and 11 which is based on conditions equivalent to our simulations (CORR in a GDE/flow-cell setup). Different Cu-catalyst morphologies are included in this data with nanosheets (Cu-NS), micron sized particles (Cu-μP), and oxide-derived Cu (OD-Cu). The roughness of these catalysts was determined to be 10, 13, and 65, respectively, via capacitance measurements and after normalization by loading (see the ESI,† Table S5).4,11 At comparable catalyst loadings for these samples, the differences in the roughness should arise for the most part from microscopic variations amongst the catalysts' morphology. We therefore apply these roughness values to be the microscopic roughness ρ in our model. We find
to align between theory and experiment regarding the limiting behavior of CO diffusion and the scaling with respect to ρ, which is expected as a direct result of the fitted RDS. Importantly, as a consequence of the proposed mechanism, the simulated acetate selectivity
shows very good agreement with the experiment. We observe the same trends in roughness and electrolyte pH for the acetate selectivity
, i.e. increasing
with decreasing roughness and increasing pH. Also, the complex potential dependent U-shape is found in both simulated and measured data. We note that this U-shape is not only observed in the acetate selectivity among C2 products
shown here but can also be seen in acetate's Faradaic efficiency (see the ESI,† Fig. S1).
We note that at higher overpotentials, there is a pH dependence of activities (partial current densities
) in the experimental data in the upper panel of Fig. 4(b). We did not include the competing pH dependent C1 pathway or ion concentration effects1,47 in our simulations which could reproduce such an apparent pH dependence. Other/smaller quantitative deviation may originate in uncertainty of the measured catalyst roughness, of assumed flow-rates and of the charge-neutral approximation or neglected size effects in our Nernst-Planck formulation in the transport model.
2.4 Short diffusion lengths of ketene suggest that micro-, not macro-scopic roughness determines acetate selectivity
As we show in Fig. 5(a), the diffusion length Ld for H2CCO(aq) is within 200 nm of the catalyst surface due to the rapid solution reaction between H2CCO and OH−. This length scale is not only well within a diameter of a single pore of a GDE electrode6,17,45 but on the same order of magnitude as the diameter of the catalyst particles (dP) used for the experiments in Fig. 4(b) (see the ESI,† Fig. S2 and ref. 4, 11). Thus, in such an electrode arrangement the diffusion spheres around the nanoparticles do not overlap as we show in Fig. 5(c). Instead, the length scale of roughness features relevant for the competition between kinetics and mass transport in our selectivity mechanism are smaller than dP. We therefore define ρ in our model as a microscopic roughness Areal/Asmooth, where Asmooth corresponds to the area of an ideal, smooth nanoparticle. This definition of the roughness fulfills its general definition AECSA/Ageo, but not vice versa. Thus, changes in catalyst loading (which alter AECSA/Ageo) do not alter ρ and should therefore lead to no changes in the acetate selectivity. This is true unless the diffusion spheres of adjacent particles could overlap significantly which would be the case for very small nanoparticles (dP ≪ 100 nm) (compare Fig. 5(c)).
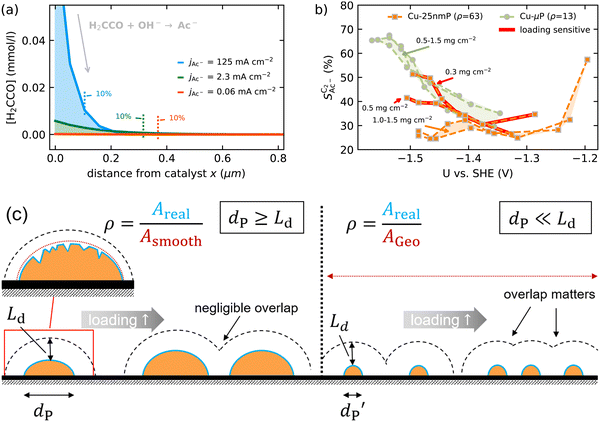 |
| Fig. 5 (a) Concentration profile of ketene against distance from the catalyst indicating the length scales of its conversion to acetate as given by our mass transport model at different partial current densities for acetate. The vertical lines indicate the distance where 90% of ketene has reacted to acetate. (b) Acetate selectivity among all C2 products ( ) against USHE for experiments in a GDE with different loadings of Cu-μP and Cu-25nmP. Each line represents an individual data set of the same reaction conditions. The shaded regions show the loading-independent selectivity ranges. The red highlighted lines are the loading dependent data found for the Cu-25nmP with small dP. (c) Illustration of the effect of the diffusion length Ld in comparison to the particle diameter dP, loading and the roughness ρ relevant to transport effects. At short Ld the overlap of diffusion spheres is negligible and only the local roughness matters (compared to a smooth particle surface). At long Ld diffusion spheres overlap and loading affects selectivity. | |
We evaluate this hypothesis through our measurements of CO reduction in 1 M KOH on Cu catalysts of different morphology and at different loadings. Specifically, we compare micron-sized (Cu-μP, same as above) and 25 nm-sized (Cu-25nmP) particles which we characterize with a mass normalized roughness ρ of 13 and 63, respectively (see the ESI,† Section S1.5). Note that by changing only the loading of Cu particles, the morphology of the catalyst and its microscopic surface roughness ρ remain the same. With higher loadings, the geometric current densities increase (which necessitates measurements at different potential ranges for matching current densities), but the current densities coincide after mass normalization (see Fig. S5, ESI†). This alignment with normalization suggests that the higher current densities at higher loadings are due to the increased active area with the same intrinsic activity. As shown in Fig. 5(b), the same selectivity of acetate among the C2 products (
) is found within the range of 0.5–1.5 mg cm−2 and 1.0–1.5 mg cm−2 for Cu-μP and Cu-25nmP, respectively. While
is independent of these loadings, it still differs according to the mass normalized roughness ρ and hence according to the catalyst morphology, in line with our computational model (compare Fig. 4(a) and Fig. S4, ESI†). These trends in
confirm that it is not the loading-dependent mesoscopic roughness but the morphology dependent microscopic roughness (ρ) that affects the selectivity towards the solution reaction. In correspondence to our predicted length scales in Fig. 5(a), we do, however, see a loading dependence at very low loadings for the very small Cu-25nmP. Decreasing the loading progressively to 0.5 and 0.3 mg cm−2 we find an increasing
. We hypothesize that, at such low loadings the overlap of diffusion lengths (Ld) between particles decreases with loading since each particle has statistically fewer neighbors. This in turn noticeably lowers the effective surface area at the length scales relevant to the solution reaction, i.e. ketene has less (local) surface to re-adsorb, subsequently increasing
. At higher loadings the amount of neighbor particles saturates similar to a packing limit (leading to uniform selectivity with increasing loading). This particle size effect is very sensitive because we do not find any noticeable loading dependency for the slightly larger 40–60 nm sized Cu particles (Cu-50nmP) which are additionally shown in the ESI† (Section S1.4 and S1.5). From these particle sizes onward, the local surface area gained by particle overlap is negligible compared to the particles area at the solution reaction length scales (see Fig. 5(c)).
While our findings highlight the importance of the local, microscopic variations in the structure of the Cu catalysts, we emphasize that this relevance arises from the rapid solution reaction which leads to a very short diffusion length (compare Fig. 5). For intermediates that do not react in solution it has been shown in the ORR (for H2O2) and methanol oxidation (for formaldehyde and formic acid) that the mesoscopic roughness (hence the loading) also influences their degree of re-adsorption and further reduction or oxidation, respectively.21 This difference is due to the larger diffusion lengths expected for pure diffusion processes.
2.5 Considerations of alternative pathways
Since our mechanism in Fig. 2 is derived from DFT simulations, there may be inherent errors in the predicted energetics48 and barriers and therefore uncertainties in the resultant pathways. Supporting our suggested mechanism, we discuss here why previously proposed11,12,14 and other alternative pathways are unlikely from a theoretical analysis of the experimentally observed pH and potential dependence in the activity.
2.5.1 Acetate selectivity cannot be determined by a PCET.
The pH dependence of acetate in comparison to other C2 products is only possible with OH− as a reactant in contrast to a recent study12 which proposed an early PCET reaction as the SDS towards acetate. On a potential vs. SHE scale, PCET reactions in alkaline conditions, where water is a proton donor, are either pH independent49 (where the RDS is the first PCET) or show a decrease in activity as pH increases (where the RDS is the 2nd or later PCET)38 (also see the ESI,† Section S6). In contrast, as shown in Fig. 4, the activity for acetate increases with pH in relation to other C2 products. Since the C2 products do not depend on pH on an SHE scale1,38,50 (or have even an apparent increasing activity with pH as visible in the upper panel of Fig. 4(b)) it follows that the SDS of acetate must in turn have an intrinsic pH dependence (which is greater than the apparent C2 one). This is only possible if OH− is a reactant in the SDS, since the involvement of OH− would lead to the necessary intrinsic pH dependence when involved in any reaction step prior to, coinciding with, or after the RDS.
2.5.2 Acetate does not have an RDS separate from other major C2 products.
Our assumption of a shared RDS between acetate and other C2 products is the most likely scenario and has also been assumed in previous work.11,12,14 As Fig. 1 shows, the total C2's, ethylene, ethanol, and acetate follow the same experimental trends: They share similar Tafel slopes (see ref. 11 and Fig. 1) and do not follow an otherwise distinguishing inverse pH-activity relationship (see the ESI,† Section S6).38 Furthermore, an alternative separate RDS of acetate would need to be a potential dependent reaction involving OH− on the catalyst surface to yield the pH and potential dependence in acetate activity. This reaction step would, however, correspond to an oxidation reaction since OH− would need to discharge.
2.5.3 The SDS for acetate does not involve OH− in a surface reaction.
Alternative to our suggested solution reaction, a hydrolysis on the catalyst surface has been suggested.11,14 This reaction would imply a nucleophilic attack of OH− occurring on the negatively charged surface onto H2CCO* (or another early intermediate). This step appears highly improbable due to electrostatic repulsion, the oxidative nature of the process and limited steric accessibility of the surface bound carbon atoms in those intermediates. Simulating this reaction for H2CCO*, we indeed find it associated with a high reaction barrier of
and potential dependence which is inconsistent with the experimental behavior (see the ESI,† Section S3.2).
2.5.4 The selectivity determining step(s) for acetate do not involve competing PCET vs. chemical reaction steps at high overpotentials.
Ref. 12 and 14 have, on the basis of reaction thermodynamics and barriers, suggested the selectivity towards acetate to be solely determined by the competition between a chemical surface reaction towards acetate vs. a PCET towards other C2 products. However, this mechanism is unlikely because the PCET step towards other C2 products would increase exponentially with increasing potential, which would result in negligible amounts of acetate, whose TOF would be potentially independent (compare potential dependence SDS-surf). In contrast, our proposed SDS-sol includes competition between H2CCO re-adsorption and a solution reaction, where neither of these steps are PCET. This non-PCET dependence in SDS-sol compensates the potential dependence of the consecutive SDS-surf. The corresponding coupled transport-kinetic model (Fig. 4) shows significant selectivities for both acetate and C2's over a wide potential range consistent with experiments.
2.5.5 A Cannizzaro-type reaction is less likely than ketene hydrolysis in solution phase.
The Cannizzaro reaction has been suggested as a possible source for acetate formation.51–53 Since this solution-based disproportionation reaction of acetaldehyde to acetate and ethanol is also OH− catalyzed, it would yield the same trends with pH and roughness as ketene hydrolysis. It would, however, also yield a 50
:
50 ratio of ethanol and acetate which is never found experimentally. Even if a substantial part of the additional ethanol would be oxidized to acetate at the anode,25,42 a slightly increased ethanol yield with pH and roughness should still be expected. Experimentally, the opposite trend is found,11,12 which renders this mechanism unlikely.
2.5.6 Ketene hydrolysis in solution best explains the experimental observations.
The proposed solution reaction of ketene is in line with C18O4 and H182O52 isotope labeling experiments and a recent study where amines are co-fed to CORR.14 For the latter, a competing solution reaction of ketene with OH− or amines explains the studies selectivity trends with potential, pH, and amine identity.
2.5.7 The proposed mechanism is not specific to the reaction conditions.
Our proposed mechanism and its implications are valid for both CORR and CO2RR. However, the latter usually contains a reduced local pH due to the equilibrium of CO2 with bicarbonate4 which gives rise to low acetate yields. A notable exception is highly alkaline electrolytes, e.g. 10 M KOH (pH ≈ 15) where high acetate yields are observed for the CO2RR.13 Similarly, high yields of acetate at high overpotentials are less often seen in H-cell experiments, since high current densities are not achievable which would lead to the necessarily high local pH values (pH ≥ 14–15, comparison in the ESI,† Fig. S16).
2.5.8 The ketene pathway is most likely the main C2 pathway.
We assume in our computational model that all C2 products go through the ketene mechanism. This assumption is supported by acetate selectivities >80% and direct FE competition between acetate and all other C2 products in the experiments of ref. 4 and 11 as shown in Fig. 4(b). Contrary to these observations, recent experiments at very negative potentials of <−1.9 VSHE showed that acetate can be obtained as the sole liquid product, out-competing nearly all ethanol, while ethylene is less affected.12 Our DFT simulations can also not provide a clear picture in that matter. An early bifurcation from the ketene pathway via the reduction of HCCO* to HCCOH* instead of H2CCO(aq) at very high overpotentials may be likely, but within the typical DFT error very uncertain (see the ESI,† Section S3.1). While most available data at low overpotentials or/and highly alkaline conditions4,8,11,14,31,42 suggest that the ketene pathway is the main C2 pathway, we cannot exclude other pathways. However, the uncertainty about additional mechanisms does not affect the preceding arguments about selectivity since the contribution of any prior bifurcation must be small, given the overall high acetate yields.
3 Conclusion
In summary, we have presented a novel mechanism towards acetate in the CO(2)RR based on the solution reaction of ketene which elucidates the dependence of its selectivity on potential, pH, and microscopic roughness. We gained this insight by analysis of the available GDE/flow cell and H-cell data, an experimental investigation of the effect of catalyst loading, and with the development of a multiscale model that predicts trends in selectivity vs. potential, pH, and roughness consistent with experiments. Our proposed mechanism dictates that acetate selectivity is determined by the transport and subsequent solution reaction of ketene vs. its (re-)adsorption and further reduction at the catalyst surface.
We emphasize that we have not invoked any special active site(s) towards acetate specific to the catalysts' morphology in our model; the effect of nanostructuring is captured by a single roughness parameter relevant at nm length scales consistent with experiments. The effect of roughness arises from its influence on the interplay between the transport and kinetics. Our examination of available ECSA-normalized data on nanostructured Cu suggests that active site(s) remained to be the same across all types of Cu investigated thus far.
Based on our insight from the elaborated mechanism, we propose the following strategies to maximize or minimize acetate yields: Generally, a high pH, a low catalyst roughness, and very high (≥−1.2 VSHE) or very low potentials (≤−1.5 VSHE) maximize the acetate yield. For the highest acetate yield, the reaction should occur at low potentials and/or under very alkaline CORR conditions, similar to those exploited in recent work.11,12,42 In contrast, a low acetate selectivity follows an operation of a very rough catalyst under neutral conditions using a buffer and intermediate potentials (−1.6 to −1.3 VSHE).
The presented mechanism rationalizes the trends in acetate selectivity based on the transport of stable intermediates/products. We note that a similar transport related process may also be relevant for other stable intermediates in the CO(2)RR like CO and acetaldehyde as also suggested by operando mass spectrometry experiments.53,54 This work highlights the importance of a rigorous evaluation of intrinsic catalyst activities and the potential impact of the interplay of reaction energetics, solution phase reactions, and mass transport in mechanistic studies of the CO(2)RR and beyond. From a simulation perspective, the combination of these effects on multiple length scales requires rigorous multiscale models beyond usual approaches focusing only on the reaction energetics on the catalyst surface.
4 Methods
4.1 Electrode preparation
The experiments were conducted using commercial micron-sized Cu particles (Cu-μP, Alfa Aesar) and 40–60 nm Cu particles (Cu-50nmP, Sigma-Aldrich) and 25 nm Cu particles (Cu-25nmP, Sigma-Aldrich) as a cathode with NiFeOx as an anode. To prepare the cathode catalyst ink, 35 mg of copper powder was dispersed in 3 ml of isopropanol and 50 μl of Nafion ionomer (5 wt% in H2O, Fuel Cell Store). The ink was drop-casted onto Sigracet 39 BB GDL (Fuel Cell Store) to a loading of 1.0 mg cm−2 for the electrolyte pH test. For the loading test, the loading was varied from 0.3 to 1.5 mg cm−2. The anode was prepared via an electrodeposition method on the cleaned nickel foam following a previous report.55 The nickel and iron ions were deposited onto the cleaned nickel foam at a potential of −1.0 V vs. Ag/AgCl for 5 minutes in a mixture solution of 3 mM Ni(NO3)2·6H2O (Sigma-Aldrich) and Fe(NO3)3·9H2O (Sigma-Aldrich). A platinum wire was used as a counter electrode in this preparation.
4.2 CO electrolysis
All CO electrolysis was performed in a three-channel flow cell. The dimensions of the channel were 2 cm × 0.5 cm × 0.15 cm with 1 cm2 geometric area of the electrode exposed to the electrolyte flow. The cathode and anode were separated by an anion exchange membrane (FAA-3-50, Fumatech) activated in 1 M KOH (99.98% metal basis, Alfa Aesar) overnight prior to the experiment. The CO was fed into the electrolyzer at a 10 sccm flow rate controlled using a mass flow controller. Aqueous KOH solution was fed into each anolyte and catholyte chamber. The catholyte flow rate was set to 0.6 ml min−1 without circulation while the anolyte flow rate was set to 1 ml min−1 to facilitate oxygen flow generated from the oxygen evolution reaction at the anode. Each electrode had direct contact with the electrolyte as the electrolyte flowed. The cathode potential was measured using an external Hg/HgO reference electrode (4.24 M KOH, Koslow Scientific) and converted to the standard hydrogen electrode (SHE) scale with 100% iR correction E(vs. SHE) = E(vs. Hg/HgO) + 0.098 V − ηiR. During the electrolysis, the gas products (i.e. hydrogen, ethylene and methane) were transferred through HaySep D and Molsieve 5 Å columns and identified/quantified with a thermal conductivity detector and a flame ionization detector on a Multiple Gas Analyzer no. 5 gas chromatography (SRI Instruments). The liquid products (i.e. acetate, ethanol and n-propanol) were collected from the outlet of the catholyte for 5 minutes at each potential and quantified by 1H NMR spectroscopy (Bruker AVIII 600 MHz NMR spectrometer) using 25 ppm dimethyl sulfoxide (Alfa Aesar) in D2O (Sigma-Aldrich) as an internal standard.
4.3 Material characterization
The surface structure of the cathode catalysts was characterized using a Zeiss Auriga-60 scanning electron microscope (see Fig. S2, ESI†).
The roughness factors (Rf) of different Cu catalysts were calculated by normalizing the double-layer capacitance (Cdl) of each catalyst deposited onto carbon paper to that of reference Cu (Cu foil). Cu foil was polished with sandpaper then cleaned in 1 M HCl solution prior to measurement for removing surface oxide layers. Toray carbon paper (Fuel Cell Store) was used to deposit the catalyst layer in order to minimize the contribution of the microporous layer capacitance of Sigracet 39 BB GDL during the measurement. 0.1 mg cm−2 of Cu particles were loaded on Toray carbon paper. The measurement was performed in a 0.1 M HClO4-filled H-cell separated by a Nafion membrane with constant inert gas flowing. Multiple scans of cyclic voltammetry at various scan rates (50 to 200 mV s−1) were performed by scanning a non-Faradaic potential window of Cu (−0.2 to −0.1 V vs. Ag/AgCl) using a platinum wire as a counter electrode and Ag/AgCl (Pine Research) reference electrode. Then, Cdl was obtained from the slope of the geometric current density measured vs. the scan rate. The roughness factor Rf was normalized with its loading giving
f.
|  | (2) |
4.4 DFT calculations
DFT calculations were conducted using the BEEF-vdW56 exchange-correlation functional. All thermodynamics were computed with GPAW57,58 where an electrochemical environment was mimicked using a hybrid explicit/implicit solvation scheme including a static water layer.41 Our GPAW results compared well with the results obtained in QuantumEspresso59 in combination with the self-consistent continuum solvation (SCCS) model.60 Electrochemical reaction thermodynamics were referenced via the computational hydrogen electrode (CHE).61 We applied the corrections by Christensen et al. to mitigate systematic DFT errors.48 Free energies were obtained following the ideal gas law for gas phase species and the harmonic oscillator model for adsorbates, respectively. All possible surface adsorbates were thoroughly sampled for their most stable adsorption site on each Cu facet using the package CatKit package.62 From this, sampling intermediates prior to HCCO* were selected for each reduction stage according to their thermodynamic stability which is also in line with established kinetic trends40 (see the final path in Fig. S12, ESI†). The grand canonical PCET barriers where computed in alkaline conditions (H2O as the proton donor) using the Solvated Jellium Model (SJM)41,63 including an explicit water layer. We note that the calculations of electrochemical barriers still bear some uncertainties,64,65 so we cautiously take these results as tentative. However, we note that the calculation of alkaline PCET barriers in this work was only possible via the use of this grand canonical framework, due to spontaneous coadsorption of OH− in conventional transition state searches. For handling of atomic structures, geometry optimizations, vibration calculations and climbing-image nudged-elastic-band (CI-NEB)66 calculations, the Atomic Simulation Environment (ASE) package67 was used. For further details of DFT calculations, slab models and convergence criteria see the ESI,† Section S2.
4.5 Coupled microkinetic and transport model
The microkinetic model was solved for its steady-state using an ODE solver available in the SciPy distribution.68 Lateral interactions were ignored as they appear insensitive to the investigated mechanism (see the ESI,† Section S5.6.3). The mass transport followed a hierarchical multiscale model where the transport within a short diffusion length from the catalyst surface is solved via a 1-D model employing the electro-neutral Nernst–Planck equation43,69 and outside of this diffusion length described by effective mass transport relations.44–46 The transport of CO was assumed solely from the gaseous side and solved analytically with a short diffusion layer of 150 nm, necessary to achieve the high current densities (see Fig. 4). On the gaseous side the partial pressure of CO was assumed with 1 atm and it's aqueous solubility by the Henry constant.70 The transport of OH− and H2CCO and their solution reaction was numerically solved for a diffusion length of 1 μm. Based on available experimentally determined constants employed in the model (see the ESI,† Section S5.2), the solution reaction is completed within this diffusion length (see Fig. 5(a)). Beyond the diffusion layer of 1 μm conditions equivalent to the electrode/electrolyte interface are assumed where the OH− concentration is determined through a Sherwood relation describing the flow perpendicular to the catalyst surface.45,46 The microkinetic and transport models are iteratively solved for the catalyst surface concentrations. More details about the transport coupled microkinetic model can be found in the ESI,† Section 5.
Code & data availability
The code for the coupled microkinetic and transport model with all input data used in this work as well as the digitized data from Fig. 1 is available under the MIT License (https://opensource.org/licenses/MIT) and CC BY 4.0 (https://creativecommons.org/licenses/by/4.0/), respectively, in Zenodo (https://doi.org/10.5281/zenodo.5013854).
Conflicts of interest
There are no conflicts of interest to declare.
Acknowledgements
The research leading to these results has received funding from the European Unions Horizon 2020 research and innovation programme under grant agreement no. 851441, SELECTCO2. Financial support was provided by V-Sustain: The VILLUM Centre for the Science of Sustainable Fuels and Chemicals (#9455) from VILLUM FONDEN. The authors acknowledge PRACE for awarding them access to the JUWELS supercomputer at GCS@FZJ in Germany through project 2020235596. The authors at the University of Delaware thank the National Science Foundation for the financial support (Award No. CBET-1904966 and CBET-1803200). Open Access funding provided by the Max Planck Society.
References
- S. Nitopi, E. Bertheussen, S. B. Scott, X. Liu, A. K. Engstfeld, S. Horch, B. Seger, I. E. Stephens, K. Chan and C. Hahn,
et al., Progress and perspectives of electrochemical CO2 reduction on copper in aqueous electrolyte, Chem. Rev., 2019, 119, 7610–7672 CrossRef CAS PubMed.
- M. Jouny, W. Luc and F. Jiao, General techno-economic analysis of CO2 electrolysis systems, Ind. Eng. Chem. Res., 2018, 57, 2165–2177 CrossRef CAS.
- P. De Luna, C. Hahn, D. Higgins, S. A. Jaffer, T. F. Jaramillo and E. H. Sargent, What would it take for renewably powered electrosynthesis to displace petrochemical processes?, Science, 2019, 364, eaav3506 CrossRef CAS PubMed.
- M. Jouny, W. Luc and F. Jiao, High-rate electroreduction of carbon monoxide to multi-carbon products, Nat. Catal., 2018, 1, 748–755 CrossRef CAS.
- C.-T. Dinh, T. Burdyny, M. G. Kibria, A. Seifitokaldani, C. M. Gabardo, F. P. G. De Arquer, A. Kiani, J. P. Edwards, P. De Luna and O. S. Bushuyev,
et al., CO2 electroreduction to ethylene via hydroxide-mediated copper catalysis at an abrupt interface, Science, 2018, 360, 783–787 CrossRef CAS PubMed.
- T. Burdyny and W. A. Smith, CO2 reduction on gas-diffusion electrodes and why catalytic performance must be assessed at commercially-relevant conditions, Energy Environ. Sci., 2019, 12, 1442–1453 RSC.
- C. W. Li and M. W. Kanan, CO2 reduction at low overpotential on Cu electrodes resulting from the reduction of thick Cu2O films, J. Am. Chem. Soc., 2012, 134, 7231–7234 CrossRef CAS PubMed.
- C. W. Li, J. Ciston and M. W. Kanan, Electroreduction of carbon monoxide to liquid fuel on oxide-derived nanocrystalline copper, Nature, 2014, 508, 504–507 CrossRef CAS PubMed.
- R. Kas, K. Yang, D. Bohra, R. Kortlever, T. Burdyny and W. A. Smith, Electrochemical CO2 reduction on nanostructured metal electrodes: Fact or defect?, Chem. Sci., 2020, 11, 1738–1749 RSC.
- J. Resasco and A. T. Bell, Electrocatalytic CO2 reduction to fuels: Progress and opportunities, Trends Chem., 2020, 2, 825–836 CrossRef CAS.
- W. Luc, X. Fu, J. Shi, J.-J. Lv, M. Jouny, B. H. Ko, Y. Xu, Q. Tu, X. Hu and J. Wu,
et al., Two-dimensional copper nanosheets for electrochemical reduction of carbon monoxide to acetate, Nat. Catal., 2019, 2, 423–430 CrossRef CAS.
- P. Zhu, C. Xia, C.-Y. Liu, K. Jiang, G. Gao, X. Zhang, Y. Xia, Y. Lei, H. N. Alshareef and T. P. Senftle,
et al., Direct and continuous generation of pure acetic acid solutions via electrocatalytic carbon monoxide reduction, Proc. Natl. Acad. Sci. U. S. A., 2020, 118, e2010868118 CrossRef PubMed.
- Y. Wang, H. Shen, K. J. Livi, D. Raciti, H. Zong, J. Gregg, M. Onadeko, Y. Wan, A. Watson and C. Wang, Copper nanocubes for CO2 reduction in gas diffusion electrodes, Nano Lett., 2019, 19, 8461–8468 CrossRef CAS PubMed.
- M. Jouny, J.-J. Lv, T. Cheng, B. H. Ko, J.-J. Zhu, W. A. Goddard and F. Jiao, Formation of carbon-nitrogen bonds in carbon monoxide electrolysis, Nat. Chem., 2019, 11, 846–851 CrossRef CAS PubMed.
- A. S. Hall, Y. Yoon, A. Wuttig and Y. Surendranath, Mesostructure-induced selectivity in CO2 reduction catalysis, J. Am. Chem. Soc., 2015, 137, 14834–14837 CrossRef CAS PubMed.
- L.-C. Weng, A. T. Bell and A. Z. Weber, Modeling gas-diffusion electrodes for CO2 reduction, Phys. Chem. Chem. Phys., 2018, 20, 16973–16984 RSC.
- S. Suter and S. Haussener, Optimizing mesostructured silver catalysts for selective carbon dioxide conversion into fuels, Energy Environ. Sci., 2019, 12, 1668–1678 RSC.
- D. Raciti, M. Mao and C. Wang, Mass transport modelling for the electroreduction of CO2 on Cu nanowires, Nanotechnology, 2017, 29, 044001 CrossRef PubMed.
- D. Bohra, J. H. Chaudhry, T. Burdyny, E. A. Pidko and W. A. Smith, Modeling the electrical double layer to understand the reaction environment in a CO2 electrocatalytic system, Energy Environ. Sci., 2019, 12, 3380–3389 RSC.
- J. Jang, M. Rüscher, M. Winzely and C. G. Morales-Guio, Gastight rotating cylinder electrode: Towards decoupling mass transport and intrinsic kinetics in electrocatalysis, AIChE J., 2021, e17605 Search PubMed.
- Y. Seidel, A. Schneider, Z. Jusys, B. Wickman, B. Kasemo and R. Behm, Mesoscopic mass transport effects in electrocatalytic processes, Faraday Discuss., 2009, 140, 167–184 RSC.
- E. L. Clark, J. Resasco, A. Landers, J. Lin, L.-T. Chung, A. Walton, C. Hahn, T. F. Jaramillo and A. T. Bell, Standards and protocols for data acquisition and reporting for studies of the electrochemical reduction of carbon dioxide, ACS Catal., 2018, 8, 6560–6570 CrossRef CAS.
-
J. K. Nørskov, F. Studt, F. Abild-Pedersen and T. Bligaard, Fundamental Concepts in Heterogeneous Catalysis, John Wiley & Sons, Inc., 2014 Search PubMed.
- F. Scholten, K.-L. C. Nguyen, J. P. Bruce, M. Heyde and B. Roldan Cuenya, Identifying structure-selectivity correlations in the electrochemical reduction of CO2: A comparison of well-ordered atomically clean and chemically etched copper single-crystal surfaces, Angew. Chem., 2021, 133, 19318–19324 CrossRef.
- D. S. Ripatti, T. R. Veltman and M. W. Kanan, Carbon monoxide gas diffusion electrolysis that produces concentrated C2 products with high single-pass conversion, Joule, 2019, 3, 240–256 CrossRef CAS.
- J. Zhang, W. Luo and A. Züttel, Self-supported copper-based gas diffusion electrodes for CO2 electrochemical reduction, J. Mater. Chem. A, 2019, 7, 26285–26292 RSC.
- M. Ma, E. L. Clark, K. T. Therkildsen, S. Dalsgaard, I. Chorkendorff and B. Seger, Insights into the carbon balance for CO2 electroreduction on Cu using gas diffusion electrode reactor designs, Energy Environ. Sci., 2020, 13, 977–985 RSC.
- G. L. De Gregorio, T. Burdyny, A. Loiudice, P. Iyengar, W. A. Smith and R. Buonsanti, Facet-dependent selectivity of Cu catalysts in electrochemical CO2 reduction at commercially viable current densities, ACS Catal., 2020, 10, 4854–4862 CrossRef CAS PubMed.
- X. Wang, Z. Wang, F. P. G. de Arquer, C.-T. Dinh, A. Ozden, Y. C. Li, D.-H. Nam, J. Li, Y.-S. Liu and J. Wicks,
et al., Efficient electrically powered CO2-to-ethanol via suppression of deoxygenation, Nat. Energy, 2020, 5, 478–486 CrossRef CAS.
-
(a) E. Bertheussen, A. Verdaguer-Casadevall, D. Ravasio, J. H. Montoya, D. B. Trimarco, C. Roy, S. Meier, J. Wendland, J. K. Nørskov and I. E. Stephens,
et al., Acetaldehyde as an intermediate in the electroreduction of carbon monoxide to ethanol on oxide-derived copper, Angew. Chem., Int. Ed., 2016, 128, 1472–1476 CrossRef;
(b) E. Bertheussen, T. V. Hogg, Y. Abghoui, A. K. Engstfeld, I. Chorkendorff and I. E. Stephens, Electroreduction of CO on polycrystalline copper at low overpotentials, ACS Energy Lett., 2018, 3, 634–640 CrossRef CAS;
(c) L. Wang, S. A. Nitopi, E. Bertheussen, M. Orazov, C. G. Morales-Guio, X. Liu, D. C. Higgins, K. Chan, J. K. Nørskov and C. Hahn,
et al., Electrochemical carbon monoxide reduction on polycrystalline copper: Effects of potential, pressure, and pH on selectivity toward multicarbon and oxygenated products, ACS Catal., 2018, 8, 7445–7454 CrossRef CAS.
-
(a) L. Wang, S. Nitopi, A. B. Wong, J. L. Snider, A. C. Nielander, C. G. Morales-Guio, M. Orazov, D. C. Higgins, C. Hahn and T. F. Jaramillo, Electrochemically converting carbon monoxide to liquid fuels by directing selectivity with electrode surface area, Nat. Catal., 2019, 2, 702–708 CrossRef CAS;
(b) D. Raciti, L. Cao, K. J. Livi, P. F. Rottmann, X. Tang, C. Li, Z. Hicks, K. H. Bowen, K. J. Hemker and T. Mueller,
et al., Low-overpotential electroreduction of carbon monoxide using copper nanowires, ACS Catal., 2017, 7, 4467–4472 CrossRef CAS.
- K. P. Kuhl, E. R. Cave, D. N. Abram and T. F. Jaramillo, New insights into the electrochemical reduction of carbon dioxide on metallic copper surfaces, Energy Environ. Sci., 2012, 5, 7050–7059 RSC.
- Y. Huang, A. D. Handoko, P. Hirunsit and B. S. Yeo, Electrochemical reduction of CO2 using copper single-crystal surfaces: effects of CO* coverage on the selective formation of ethylene, ACS Catal., 2017, 7, 1749–1756 CrossRef CAS.
- J. Andraos and A. J. Kresge, Correlation of rates of uncatalyzed and hydroxide-ion catalyzed ketene hydration. A mechanistic application and solvent isotope effects on the uncatalyzed reaction, Can. J. Chem., 2000, 78, 508–515 CAS.
- K. J. P. Schouten, E. Pérez Gallent and M. T. Koper, Structure sensitivity of the electrochemical reduction of carbon monoxide on copper single crystals, ACS Catal., 2013, 3, 1292–1295 CrossRef CAS.
- J. H. Montoya, C. Shi, K. Chan and J. K. Nørskov, Theoretical insights into a CO dimerization mechanism in CO2 electroreduction, J. Phys. Chem. Lett., 2015, 6, 2032–2037 CrossRef CAS PubMed.
- G. Kastlunger, L. Wang, N. Govindarajan, H. H. Heenen, S. Ringe, T. Jaramillo, C. Hahn and K. Chan, Using pH dependence to understand mechanisms in electrochemical CO reduction, ACS Catal., 2022, 12, 4344–4357 CrossRef CAS.
- X. Liu, P. Schlexer, J. Xiao, Y. Ji, L. Wang, R. B. Sandberg, M. Tang, K. S. Brown, H. Peng and S. Ringe,
et al., pH effects on the electrochemical reduction of CO(2) towards C2 products on stepped copper, Nat. Commun., 2019, 10, 1949 CrossRef PubMed.
- N. Govindarajan, G. Kastlunger, H. H. Heenen and K. Chan, Improving the intrinsic activity of electrocatalysts for sustainable energy conversion: where are we and where can we go?, Chem. Sci., 2022, 13, 14–26 RSC.
- A. M. Patel, S. Vijay, G. Kastlunger, J. K. Nørskov and K. Chan, Generalizable trends in electrochemical protonation barriers, J. Phys. Chem. Lett., 2021, 12, 5193–5200 CrossRef CAS PubMed.
- G. Kastlunger, P. Lindgren and A. A. Peterson, Controlled-potential simulation of elementary electrochemical reactions: Proton discharge on metal surfaces, J. Phys. Chem. C, 2018, 122, 12771–12781 CrossRef CAS.
-
S. Overa, B. Crandall, B. Shrimant, D. Tian, B. H. Ko, H. Shin, C. Bae and F. Jiao Enhancing acetate selectivity by coupling anodic oxidation in carbon monoxide electroreduction. 2022.
- A. Subramaniam, J. Chen, T. Jang, N. R. Geise, R. M. Kasse, M. F. Toney and V. R. Subramanian, Analysis and simulation of one-dimensional transport models for lithium symmetric cells, J. Electrochem. Soc., 2019, 166, A3806 CrossRef CAS.
-
D. Bohra, J. Chaudhry, T. Burdyny, E. Pidko and W. Smith Mass Transport in Catalytic Pores of GDE-Based CO2 Electroreduction Systems. 2020.
- M. A. Modestino, S. M. H. Hashemi and S. Haussener, Mass transport aspects of electrochemical solar-hydrogen generation, Energy Environ. Sci., 2016, 9, 1533–1551 RSC.
-
E. L. Cussler and E. L. Cussler, Diffusion: mass transfer in fluid systems, Cambridge University Press, 2009 Search PubMed.
- J. Resasco, L. D. Chen, E. Clark, C. Tsai, C. Hahn, T. F. Jaramillo, K. Chan and A. T. Bell, Promoter effects of alkali metal cations on the electrochemical reduction of carbon dioxide, J. Am. Chem. Soc., 2017, 139, 11277–11287 CrossRef CAS PubMed.
- R. Christensen, H. A. Hansen and T. Vegge, Identifying systematic DFT errors in catalytic reactions, Catal. Sci. Technol., 2015, 5, 4946–4949 RSC.
- D. Strmcnik, M. Uchimura, C. Wang, R. Subbaraman, N. Danilovic, D. Van Der Vliet, A. P. Paulikas, V. R. Stamenkovic and N. M. Markovic, Improving the hydrogen oxidation reaction rate by promotion of hydroxyl adsorption, Nat. Chem., 2013, 5, 300 CrossRef CAS PubMed.
-
Y. i Hori, Modern aspects of electrochemistry, Springer, 2008, pp 89–189 Search PubMed.
- Y. Y. Birdja and M. T. Koper, The importance of Cannizzaro-type reactions during electrocatalytic reduction of carbon dioxide, J. Am. Chem. Soc., 2017, 139, 2030–2034 CrossRef CAS PubMed.
- Y. Lum, T. Cheng, W. A. Goddard III and J. W. Ager, Electrochemical CO reduction builds solvent water into oxygenate products, J. Am. Chem. Soc., 2018, 140, 9337–9340 CrossRef CAS PubMed.
- E. L. Clark, J. Wong, A. J. Garza, Z. Lin, M. Head-Gordon and A. T. Bell, Explaining the incorporation of oxygen derived from solvent water into the oxygenated products of CO reduction over Cu, J. Am. Chem. Soc., 2019, 141, 4191–4193 CrossRef CAS PubMed.
- B. Hasa, M. Jouny, B. H. Ko, B. Xu and F. Jiao, Flow electrolyzer mass spectrometry with a gas-diffusion electrode design, Angew. Chem., Int. Ed., 2020, 133, 3314–3319 CrossRef.
- X. Lu and C. Zhao, Electrodeposition of hierarchically structured three-dimensional nickel–iron electrodes for efficient oxygen evolution at high current densities, Nat. Commun., 2015, 6, 6616 CrossRef CAS PubMed.
- J. Wellendorff, K. T. Lundgaard, A. Møgelhøj, V. Petzold, D. D. Landis, J. K. Nørskov, T. Bligaard and K. W. Jacobsen, Density functionals for surface science: Exchange-correlation model development with Bayesian error estimation, Phys. Rev. B: Condens. Matter Mater. Phys., 2012, 85, 235149 CrossRef.
- J. J. Mortensen, L. B. Hansen and K. W. Jacobsen, Real-space grid implementation of the projector augmented wave method, Phys. Rev. B: Condens. Matter Mater. Phys., 2005, 71, 035109 CrossRef.
- J. Enkovaara, C. Rostgaard, J. J. Mortensen, J. Chen, M. Dułak, L. Ferrighi, J. Gavnholt, C. Glinsvad, V. Haikola and H. A. Hansen,
et al., Electronic structure calculations with GPAW: A real-space implementation of the projector augmented-wave method, J. Phys.: Condens. Matter, 2010, 22, 253202 CrossRef CAS PubMed.
- P. Giannozzi, S. Baroni, N. Bonini, M. Calandra, R. Car, C. Cavazzoni, D. Ceresoli, G. L. Chiarotti, M. Cococcioni and I. Dabo,
et al., QUANTUM ESPRESSO: a modular and open-source software project for quantum simulations of materials, J. Phys.: Condens. Matter, 2009, 21, 395502 CrossRef PubMed.
- O. Andreussi, I. Dabo and N. Marzari, Revised self-consistent continuum solvation
in electronic-structure calculations, J. Chem. Phys., 2012, 136, 064102 CrossRef PubMed.
- J. Nørskov, J. Rossmeisl, A. Logadottir, L. Lindqvist, J. Kitchin, T. Bligaard and H. Jónsson, Origin of the overpotential for oxygen reduction at a fuel-cell cathode, J. Phys. Chem. B, 2004, 108, 17886–17892 CrossRef.
- J. R. Boes, O. Mamun, K. Winther and T. Bligaard, Graph theory approach to high-throughput surface adsorption structure generation, J. Phys. Chem. A, 2019, 123, 2281–2285 CrossRef CAS PubMed.
- P. Lindgren, G. Kastlunger and A. A. Peterson, A Challenge to the G 0 interpretation of hydrogen evolution, ACS Catal., 2019, 10, 121–128 CrossRef.
- J. A. Gauthier, S. Ringe, C. F. Dickens, A. J. Garza, A. T. Bell, M. Head-Gordon, J. K. Nørskov and K. Chan, Challenges in modeling electrochemical reaction energetics with polarizable continuum models, ACS Catal., 2018, 9, 920–931 CrossRef.
- J. A. Gauthier, C. F. Dickens, H. H. Heenen, S. Vijay, S. Ringe and K. Chan, Unified approach to implicit and explicit solvent simulations of electrochemical reaction energetics, J. Chem. Theory Comput., 2019, 15, 6895–6906 CrossRef CAS PubMed.
- P. Lindgren, G. Kastlunger and A. A. Peterson, Scaled and dynamic optimizations of nudged elastic bands, J. Chem. Theory Comput., 2019, 15, 5787–5793 CrossRef CAS PubMed.
- A. H. Larsen, J. J. Mortensen, J. Blomqvist, I. E. Castelli, R. Christensen, M. Dułak, J. Friis, M. N. Groves, B. Hammer and C. Hargus,
et al., The atomic simulation environment—a Python library for working with atoms, J. Phys.: Condens. Matter, 2017, 29, 273002 CrossRef PubMed.
- P. Virtanen, R. Gommers, T. E. Oliphant, M. Haberland, T. Reddy, D. Cournapeau, E. Burovski, P. Peterson, W. Weckesser and J. Bright,
et al., SciPy 1.0: fundamental algorithms for scientific computing in Python, Nat. Methods, 2020, 17, 261–272 CrossRef CAS PubMed.
- H. H. Heenen, J. Voss, C. Scheurer, K. Reuter and A. C. Luntz, Multi-ion conduction in Li3OCl glass electrolytes, J. Phys. Chem. Lett., 2019, 10, 2264–2269 CrossRef CAS PubMed.
- R. Sander, Compilation of Henry's law constants (version 4.0) for water as solvent, Atmos. Chem. Phys., 2015, 15, 4399–4981 CrossRef CAS.
Footnotes |
† Electronic supplementary information (ESI) available. See DOI: https://doi.org/10.1039/d2ee01485h |
‡ These authors contributed equally. |
§ Present address: Department of Chemical Engineering, Texas Tech University, Lubbock, TX 79409, USA. |
|
This journal is © The Royal Society of Chemistry 2022 |
Click here to see how this site uses Cookies. View our privacy policy here.