Prospects and challenges for autonomous catalyst discovery viewed from an experimental perspective
Received
10th February 2022
, Accepted 23rd April 2022
First published on 25th April 2022
Abstract
The urgency with which fundamental questions of energy conversion and the sustainable use of raw materials must be solved today requires new approaches in catalysis research. One way is to couple high-throughput experiments with machine learning methods in autonomous catalyst development. The fact that the active form of a catalyst is only created under working conditions and that the catalytic function is always in a very complex relationship with a number of physical and chemical properties of the material makes it essential to integrate operando experiments into systems of autonomous catalyst development. The analysis of the current state of the art and knowledge revealed a lack of integration of the numerous, technically very different unit operations in catalyst discovery and a great need for new developments in online and in situ analytics, especially in catalyst synthesis. To pave the way for autonomous processing of work packages by robots, it is proposed to advance the automation of single unit operations currently performed by human researchers by introducing standard operating procedures described in handbooks. The work according to rigorous protocols produces, on the one hand, reliable data that can be evaluated using artificial intelligence and facilitates on the other hand the automation. Special attention should be paid to the acquisition and real-time evaluation of analytical data in in situ and operando experiments as well as the automatic storage of data and metadata in databases.
1. Introduction
The challenges of climate change impose on catalysis research the task of rapidly developing new solutions that are robust, affordable and scalable to large dimensions for the conversion of energy, hydrogen supply, and the energy- and resource-saving manufacture of chemical products.1–5 Heterogeneous catalysis at the solid–gas or solid–liquid interface plays an essential role in this respect. Developing a new heterogeneous catalyst using traditional methodology is a time-consuming and cost-intensive process that may need years or decades from the initial idea to industrial implementation. Thus, it took 20 years from the first experiments to reduce carbon monoxide with hydrogen by Franz Fischer and Hans Tropsch in 1925 to the industrial introduction of Fischer–Tropsch synthesis with a capacity of 600
000 t per year in 1945.6 Parallelized methods of catalyst preparation and testing were therefore applied early on to speed up the search for new catalysis technologies.7,8 However, the development of a chemical production process in industry is not only associated with the search for a new catalyst, but simultaneously involves the engineering of appropriately adapted process parameters and reactor technologies, as was the case with the introduction of the large-scale process for ammonia synthesis. The sophisticated material chemistry and design of the reactor was developed by chemical engineers under the leadership of Carl Bosch, while more than 2500 catalysts were tested by Alwin Mittasch and his co-workers at BASF in over 6500 experiments in the years 1909–1912 to find the iron-based ammonia synthesis catalyst that is still used today.8 The technical implementation of the Haber–Bosch process led to an innovative change in the chemical industry of that era and changed our world.9 Such pioneering inventions are commonly brought about by social constraints, such as the fight against hunger and the demand for explosives for war purposes in the first half of the last century.
It is open to debate why the widespread application of experimental and computer-based high-throughput methods in industrial and academic laboratories at the turn of the millennium and in the 2000s10–17 did not lead to any truly ground-breaking discoveries in catalysis.16 Currently, the field is rapidly evolving towards enhanced integration of high-throughput experimentation and data-driven science.18–21 The autonomous discovery of new catalysts, inorganic compounds and functional materials by robots without the intervention of the scientist is seen as an exciting prospect for advancing catalysis research and materials science.20,22–31 The beneficial use of a toolkit like this requires full integration of unit operations in catalyst development such as automated synthesis of catalyst libraries, (high-throughput) characterization and testing, data analysis with machine learning algorithms,18,20,22,32–34 and design of new experiments by active learning and automated reasoning20 in closed feedback loops. The integration of artificial intelligence and robotics provides the key to the practical implementation of such a concept.26 The advantage of unbiased synthesis design lies in the possibility of leaving the predesigned paths that science tends to follow based on experience and thus opening up completely new phase spaces.20,23,25,26 The automation of experiments, their parallelisation and miniaturisation save time, ultimately reduce costs and improve reproducibility. On the other hand, it must be taken into account that the performance of highly active real catalysts producing products on a large scale is always influenced by transport phenomena, which leads to problems in scaling up miniaturised and high-throughput experiments.
In recent years, artificial intelligence and in particular machine learning techniques have been used with success in many fields including materials research29,35 and catalysis.20,22,26,34,36–41 The multifaceted structure of the data from theory, and various synthesis and characterisation experiments poses a challenging task for data governance.42 More and more databases are being developed and shared to make data available and promote catalyst discovery through data science.42–54
This paper analyses from an experimental point of view how far catalysis research still is from autonomous catalyst development, which prerequisites have to be created for this and whether these efforts are worthwhile given the nature of a catalyst as a metastable functional material. Directions that can be taken to accelerate the discovery of new catalysts are discussed.
2. How to optimize a kinetic phenomenon
In the development of a solid heterogeneous catalyst, it is not a single physical or chemical property that is optimized, but the complex interplay between the bulk and surface properties of the catalyst and its chemical interaction with the reacting phase.55 This interaction, reflected in a polarization of the adsorbed molecules and corresponding adsorption equilibria, leads to a specific coverage of the catalyst surface with the reacting molecules, intermediates, products and solvating species under reaction conditions. The degree of coverage in turn determines the overall reaction rates. A heterogeneously catalyzed reaction is never an elementary reaction, as the process is at least composed of three steps: adsorption, surface reaction and desorption.56 The target variable in catalysis is therefore the optimal ratio of various rates in a complex interfacial reaction network that maximizes the formation of the desired product.57 In addition, intrinsic and transport kinetics always overlap, but particularly under high performance conditions. The intricate interplay between all these factors, which are multidimensional in terms of space and time, leads to the fact that descriptors in catalysis are generally complex mathematical relationships (see Fig. 1). Furthermore, there are some peculiarities to take into account in the search for new catalysts, which will be briefly explained below.
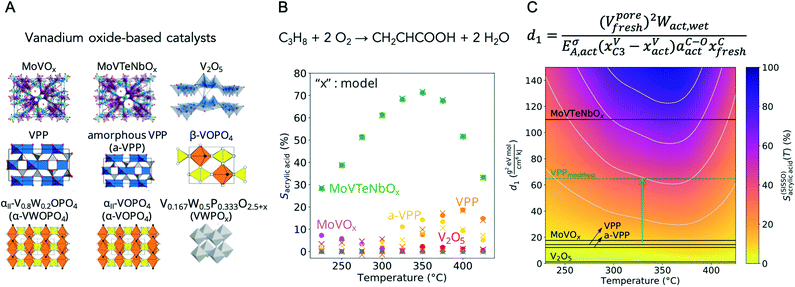 |
| Fig. 1 (A) Vanadium oxide-based catalysts synthesized, analysed by ex situ, in situ and operando techniques after synthesis (fresh), rapid activation in the reaction feed (act) and catalysis, and investigated in the selective oxidation of propane to acrylic acid;69 (B) dependency of the selectivity to acrylic acid S (circles) on the reaction temperature T, showing that acrylic acid, as an intermediate product, is only formed at intermediate temperatures; other products formed are mainly propylene at low temperatures (low propane conversions) and CO and CO2 at high temperatures (high propane conversions); the values modelled by a machine learning algorithm (SISSO) are indicated as crosses; (C) map of catalysts given by the SISSO model for the acrylic acid selectivity as a function of the temperature S(SISSO)acrylic acid(T) represented by a colour code indicating the regions of the materials space corresponding to high selectivity (in blue) and low selectivity (in yellow). The catalysts used for deriving the descriptor are indicated by the black lines. The descriptor d1 consists of a complex function incorporating ex situ measured data such as the pore volume V and the carbon content xC on the surface of the fresh catalysts determined by XPS, but mainly in situ and operando data such as the work function W measured by near-ambient pressure XPS (NAP-XPS) in a reaction feed to which water vapor has been added, the vanadium content xV at the surface of the activated catalysts and the catalysts working in propane-rich feed, the activation energy of the charge carrier transport EA measured by a contactless microwave-based method and the concentration of adsorbates containing C–O bonds aC–O at the surface of the activated catalysts measured by XPS; the green dashed line indicates a hypothetical vanadyl pyrophosphate (VPP) catalyst proposed for future syntheses; the new catalyst could be obtained by increasing the pore volume of VPP by 50%, e.g. by using additives in the synthesis, and by reducing the activation energy of the charge carrier transport by 50%, e.g. by adding promoters. | |
Catalyst kinetics
Entanglement of reaction and solid-state kinetics.
According to our current understanding the breaking and making of chemical bonds at atomic scale takes place at active sites58 that are subject to structural changes either within one catalytic cycle or through the fluctuating emergence and disappearance of sites, which, due to their large number, on average provide stationary performance during the needed long-term stable runtime of the catalyst.59,60 Reaction kinetics and kinetics of catalyst transformations are chemically closely correlated. The link is established by the local chemical potential and its variation in time. Harsh reaction conditions accelerate solid-state chemical conversions of the catalyst leading in the worst case to irreversible transformations and catalyst deactivation.
Present catalyst synthesis concepts are generally aimed at creating the active site by synthetic means as a result of polycondensation or grafting processes using molecular starting compounds and the toolkit of advanced inorganic, metalorganic or organic chemistry. Targets are set based on chemical intuition, crystallographic considerations,61 model concepts from surface science,62 or by analogy with molecular compounds.63 Computational chemistry is mainly employed to support these assumptions energetically.64,65 This strategy is in conflict with catalyst dynamics and the metastable nature of active sites. Although it is generally accepted that the product of catalyst synthesis is a catalyst precursor and that the generally unknown structure of the active phase is only formed in contact with the reacting molecules under working conditions, this insight is nevertheless hardly taken into account in the paradigm of current catalysis research. Since the active site cannot be synthesized, the chemistry leading from the catalyst precursor to the active form must therefore be included in the design concept. Furthermore, reaction conditions that are optimal for one precursor may well be disadvantageous for another precursor. This considerably expands the parameter space to be explored. Another important consequence of reversible or irreversible dynamic adaptations of a catalyst under process conditions is that sufficient long times on stream must be kept in catalyst testing in order to capture formation and deactivation phenomena.66 Temporal trends or fluctuations are, therefore, important indicators.
Derived demands on the experiments.
Automation makes it possible to better meet these requirements, as it can speed up the process, but catalysis must be carried out under realistic reaction conditions. A high degree of integration of all unit operation in catalyst development and recording of all metadata and their consideration in the data analysis is required, which is technically demanding. Alternatively, it remains to be investigated whether certain material properties of catalyst precursors, such as specific surface area, crystal structure, band gap or elemental composition, are predestined for the evolution of particular performance under reaction conditions. The selection of the target parameters could then be based on those of the catalyst precursor of a known benchmark catalyst. However, if an established material is taken as a basis, it is most likely that only a local, but not the global maximum of the catalytic performance will be found. And even with this approach, extensive high-throughput characterization of catalyst precursor libraries cannot be avoided.
In an analysis of propane oxidation data by machine learning using a compressed-sensing method (SISSO),67,68 complex but interpretable correlations were found for the discussion and prediction of the catalytic performance of vanadium oxide-based catalysts involving both certain physicochemical properties of the catalyst precursors and certain parameters measured under operating conditions, highlighting the importance of dynamic processes in the identification of descriptors.69 Nine polycrystalline oxides were synthesized, characterized and tested in propane oxidation according to rigorous protocols described in a handbook.70 The bulk and surface properties of both fresh and activated catalyst samples were extensively characterized by using X-ray diffraction, N2 adsorption, X-ray fluorescence, laboratory X-ray photoelectron spectroscopy and temperature-programmed reduction/oxidation. In addition, the chemical composition and the electronic surface and bulk properties, respectively, of the activated catalysts were measured under various reaction conditions of propane oxidation using near ambient pressure photoelectron spectroscopy (NAP-XPS) and the microwave cavity perturbation technique (MCPT). The complex function obtained by machine learning that describes and predicts the performance of the catalysts includes key features measured for both the precursor and the activated catalysts, such as the pore volume and the concentration of certain adsorbates on the surface, as well as parameters measured in operando, such as the activation energy of charge carrier transport, the vanadium concentration and the oxidation state of vanadium under different reaction conditions. These “catalyst genes” were used to map the catalytic properties and to derive new synthesis strategies for improved catalysts on this basis (Fig. 1). This study clearly shows that the measurement of key parameters in operando experiments on the working catalyst is crucial for descriptor discovery as it reflects the dynamic response of the catalyst to the chemical potential under reaction conditions, i.e. the entanglement of solid state and reaction kinetics.
Multiscale nature of functional properties
Heterogeneous catalysts are hierarchical systems.55 The dimensions of the structured catalyst span orders of magnitudes from the mm large pellets to the nm small active sites. Synthesis strategies are generally designed to optimize the interaction of the catalyst components across all scales. Only then control is executed over the local chemical potential at the active site. Bulk and surface properties are fine-tuned in view of phenomena like solvation, heat transport, and mass transfer. A variety of properties such as the concentration of hydrophobic or hydrophilic surface functional groups, the type and concentration of defects, the mixing of catalyst and reacting phase in terms of leaching or (sub)surface enrichment of reacting molecules, the heat capacity, thermal conductivity, particle size or porosity are important parameters, to name but a few. Particularly difficult to control and define are crucial factors such as the interaction between the active phase and the stabilizing matrix or the perimeter between these two components. Solids of the same composition, crystal structure, similar particle size distribution and crystallinity can, for example, have completely different catalytic properties if the primary particles have different morphologies.71 The autonomous search for catalysts must therefore take into account a large number of parameters, which must be determined by comprehensively characterizing the materials using a broad variety of methods in different states of the catalyst. In terms of an unbiased approach, it is not possible to predict a priori which of the properties is particularly important for the material's function as a catalyst.
When used in industrial reactors or other technical applications, the catalyst precursors are formed into a structured body with the help of binders and additives, for example into pellets or extrudates, or an electrode is fabricated.72–75 These substances and the shaping procedure will modify the activity, selectivity, and stability of the catalyst by influencing the transport kinetics for mass and energy,76 but also by the presence of additional and possibly different active sites.77 The development of formulation recipes therefore involves a similar amount of effort as the identification of the suitable active phase itself. These additional steps in the workflow for catalyst development currently receive little attention in academic research, even within the conventional approach.78 But they must be taken into account if new disruptive technologies are ultimately to be actually introduced into practice.
Scalability
Miniaturization of the batch size is advantageous for expensive materials and enables rapid screening of a large number of samples in high-throughput experiments, but with the limitations discussed above in terms of transport kinetics.11,17,21,23,26 Furthermore, the density of the sample libraries can be increased in this way, i.e., more samples can be prepared and thus the step size can be reduced when varying a parameter.21 However, scaling up synthesis approaches is difficult and not successful in every case, so that some hits from high-throughput studies will never have a chance of technical implementation. So, these experiments and the corresponding investments in materials and working time will not pay off. In addition, several characterization methods can reach the limits of their sensitivity if the sample quantities are too small. For example, determining the specific surface area of 2D samples on an array using conventional gas adsorption methods is practically impossible. Alternative measurement methods, for example the indirect determination of the surface area via the particle size measured by XRD, can offer a solution here, but not in every case. Thus, when integrating catalyst synthesis, characterization and testing for autonomous catalyst design, the advantages of miniaturization can be counterbalanced by the disadvantages in terms of the yield of knowledge in physicochemical analysis and the reduced prospects for scalability.
Experimental diversity and complexity
The synthesis methods used for the preparation of heterogeneous catalysts are very diverse and generally multi-stage, with the different stages requiring very different experimental conditions, ranging, for example, from condensation in aqueous medium or other solvents at room temperature or lower temperatures to thermal treatment at high temperatures in a gas stream in rotating furnaces or in a plasma. Moreover, catalysis research is an interdisciplinary field in which a wide variety of methods are used for characterization of freshly prepared catalyst precursors and for analysis of catalysts in operation. In operando experiments, the functional properties of a catalyst are measured by analytical methods, such as gas chromatography or mass spectrometry, while spectroscopic experiment, a diffraction method or microscopy is carried out at the same time.79 Therefore, the data and metadata of operando experiments are particularly extensive and generally also very complex due to the time-based coupling of at least two methods that are carried out with very different equipment. Equally diverse are the chemical reactions that are catalyzed and for which very different process conditions and reactor technologies are applied. For these reasons, there can be no universal robot for autonomous catalyst development. Nevertheless, automated building blocks could be used in modular systems to find solutions to specific problems, just as different modules have been used in the past for different synthesis stages and work steps in characterization and testing.
3. The handbook-concept
The challenges in optimizing a catalyst arise from its basic function, which is to activate inert molecules by interaction at the interface so that a reaction takes place under milder conditions than in the homogeneous phase. The target parameter is a kinetic value. The measured value may depend on the workflow of the catalyst test due to the complicated, but function-inherent interplay between solid-state kinetics and reaction kinetics explained above. Depending on the catalyst pretreatment and testing workflow, experiments on the same catalyst can lead to different local maxima in catalyst performance, resulting in unclear structure–reactivity relationships.70 This is the case even if all experiments are conducted according to the guidelines of best experimental practice and under the same reaction conditions in the steady state in terms of temperature, contact time and feed composition. To explain this further, a catalyst can adopt different steady states depending on its previous operating history, e.g., due to differences in the degree of reduction/oxidation in the conditioning phase of the catalyst, which can be carried out under different gas compositions or when the catalyst was tested before this particular measurement point at high or low conversion. Similarly, the particle size or the perimeter between active phase and matrix may differ due to different sintering behavior if the temperature variation in a catalyst test was started at either high or low temperatures. The concentration and type of acid–base sites may also vary depending on the conditions under which the steady state was achieved. In order to arrive at unambiguous structure–function relationships in an unknown phase space, catalysis experiments must be carried out according to strict experimental protocols,80–84 which can be described in handbooks (Fig. 2).70 Standard operating procedures are also necessary because one cannot measure all the properties of the catalyst under reaction conditions, not only because that would be too time-consuming or technically difficult to realize, but also because one cannot necessarily know which properties all need to be measured.
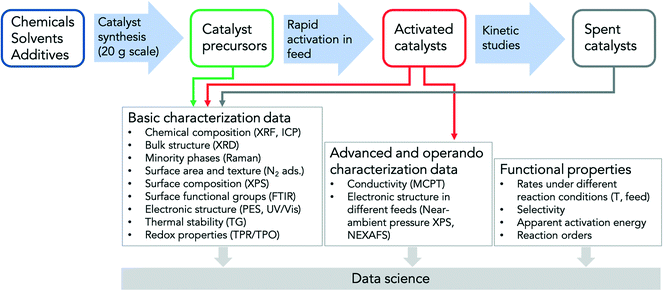 |
| Fig. 2 General workflow designed for a project on the selective oxidation of short-chain alkanes on a number of known active, inactive, selective and non-selective oxidation catalysts in which two laboratories and a theory group were involved;70 the aim was to study whether the data obtained are suitable for evaluation with artificial intelligence methods; the synthesis of the fresh catalysts in sufficient quantity for a comprehensive analysis, their rapid activation in the reaction feed and all steps of parameter variation (T, GHSV, feed composition) in testing of the catalysts are described in detail. Rapid activation means testing the catalysts for 48 h under highest possible conversion (80% with respect to alkane and/or oxygen) or highest possible temperature (450 °C) if this conversion is not reached. The aim of the treatment is to quickly bring the catalysts into a steady-state for the kinetic and operando investigations or to eliminate rapidly deactivating catalysts from the beginning. Furthermore, the handbook specifies in which stages and with which methods and how the catalysts have to be analyzed. Thus, freshly prepared catalysts as well as activated and used catalysts are characterized ex situ, while operando investigations are only carried out on the activated catalysts. | |
The approach of the handbook also has the advantage that the results obtained in different laboratories can be compared directly, even if the experiments are carried out manually. This is not only important when the data is made available in open databases and thus becomes generally accessible for evaluation with artificial intelligence methods.
The work according to rigorous protocols also enables a direct ranking of materials in a literature comparison. For many reactions, the identification of interesting materials classes is not possible because the published data are not comparable due to very different activation and reaction conditions. Oxidation reactions,85 the production of hydrogen by ammonia decomposition,86 or electrochemical synthesis of ammonia80 should be mentioned here as examples. The direct comparison of catalysts is achievable also when the individual laboratories have different equipment, because it is possible to agree on an absolutely necessary standard for the experiments that all laboratories can meet. There are, of course, no restrictions on conducting additional experiments. In addition, technical errors can be minimized by accurately describing the test and characterization methods in standard operating procedures and by measuring benchmark catalysts.66,87–90
Automation supports the work according to a handbook and increases reproducibility. On the other hand, it requires the specification of the unit operations to be carried out, which in turn can be defined in a handbook. However, this implies that an ontology in catalysis research must be developed that allows the prosaic description of catalysis experiments in handbooks to be translated into a generally applicable machine-readable language.91–93
4. Automation of unit operations
In the past decades, a lot of effort has been invested in the automation of synthesis, characterization and screening steps, with combinatorial research and high-throughput catalysis in particular being at the forefront. Progress in this field has been summarized in numerous review articles.10–13,16,17,19,21,94–99 Libraries have been built with a large number of catalysts for different reactions using both high-speed array approaches and classical parallel synthesis and testing strategies with catalyst masses in mg or g scale. Initially, methods from combinatorial drug discovery were adapted for the design of the starting library, such as the split & pool method, which involves the combinatorial permutation of parameters and thus enables the generation of arbitrary combinations of variables in large parameter spaces.17,100 The widely used DoE (Design of Experiment) tools, which are based on linear regression algorithms, allow experiments to be conducted with high throughput and maximum efficiency by identifying precisely those parameters for further screening rounds that have a particular impact on the target variable. Machine learning-based techniques such as genetic algorithms (GA) and artificial neural networks (ANN) have also been used for a long time to gain insights from prior high-throughput experiments for designing the next generations.101–104 Great potential is seen in the better integration of high-throughput experiments and data science.19,35 The progressive development of machine learning and robotics could fundamentally change basic research and significantly accelerate progress.
Machine learning is intended to generate knowledge from data and is thus an important tool for automating intelligent behaviour through artificial intelligence. However, the recent and rapidly increasing interest in data driven catalysis research has a strong emphasis on computational chemistry.22,38,40,41,53,57,105–111 Examples are the bottom-up prediction of crystal structures,112 new mixed oxides,113 or alloys and intermetallic compounds114 from first principles, establishing scaling relationships between the adsorption energies of reactants and activation reaction energies (Brønsted–Evans–Polanyi (BEP) relationships),115,116 the fast exploration of potential energy surfaces,117 and addressing complex reaction networks.57 In addition, literature data have also been used for data mining and knowledge extraction,41,118–123 although this approach is problematic as often only “good” catalyst data are published,124 and the dataset thus consists of many similar results, which are sometimes incomplete and poorly documented. The combination of advanced machine learning methods with high-throughput experimentation is an important step towards robotic autonomous catalyst development.19,26,125,126 However, the technical realisation of the necessary fully integrated systems is still in its infancy, which is due to the diversity and complexity of catalysis experiments. The related experimental challenges are briefly discussed in the following. In general, the testing of catalysts is largely automated in many laboratories or commercial solutions are available.13,21 Therefore, the focus in the next section will be on developments for automated synthesis and characterization of catalysts. A shortcoming of many high-throughput screening experiments for functional analysis of catalysts, however, is that the reaction parameter space is not sufficiently large and its structure not diverse enough. Consequently, the screening of process conditions in a wide field127,128 is an important task and should be implemented in all future investigations.
Integration of automated catalyst synthesis, characterization and functional analysis
A wide variety of methods of inorganic and organic synthetic chemistry as well as physical methods are used for the preparation of heterogeneous catalysts in the form of amorphous or polycrystalline powders (3D materials) as well as 2D materials. The methods include precipitation reactions under normal pressure or solvothermal conditions, sol–gel techniques, solid-state syntheses, methods for surface modification, such as impregnation or grafting of organometallic complexes, atomic layer deposition, electrochemical techniques or sputtering to mention just a few examples. The vast majority of published experiments are currently conducted largely manually. Efforts already made to automate catalyst synthesis are not limited to high-throughput experiments, but have been particularly advanced here in order to be able to synthesize large catalyst precursor libraries in a reasonable time.
Automated bulk syntheses.
One of the early examples of combinatorial catalyst preparation is the hydrothermal synthesis of zeolites on a scale of 1–10 g in a Teflon block with 100 reaction chambers. By screening the system TMA2O–Li2O–Cs2ONa2O–Al2O3–SiO2 (TMA = trimethylammonium) it could be shown that even with parallelized synthesis the zeolite phases and approximate stability ranges expected under the synthesis conditions can be obtained.129 These experiments were carried out entirely manually, but have motivated automation in the field of high-throughput hydrothermal synthesis. Initially, pipetting robots were used to produce the synthesis gels.130–132 Subsequently, in addition to more integrated self-built systems,133,134 commercial synthesis robots from companies such as Zinsser, Chemspeed, or the former company Symyx135 were used. Advances in the automated hydrothermal preparation of zeolites and metal organic frameworks (MOF's) have been summarized in recent reviews.99,136 Other early, but already technically advanced examples exist for the synthesis of multi-metal mixed oxides. High surface area Sn, In, Co, Ru, Ni, Fe, Mn, Y, Ce and Rare Earth oxides and their mixtures were produced in a Symyx robot for precipitation reactions using coupled co-precipitation and combustion synthesis.137 The precipitation robot consisted of eight parallel precipitation channels equipped with pH probe, temperature probe, three probes for liquid addition, and magnetic stirrer. Precipitation could therefore be carried out as liquid addition, as titration or as constant pH precipitation.137 Sol–gel methods were also performed using synthesis robots.138,139 For example, an extensive library of Mo-V-Sb catalysts was optimized in terms of chemical composition for the oxidation of isobutane to methacrolein.139 Magnesium ethoxide samples as support materials for Ziegler–Natta catalysts were prepared in parallel and automated with excellent reproducibility and morphological control.140 These few selected examples for the synthesis of bulk 3D catalysts clearly show that it was possible to parallelize and automate classic aqueous synthesis processes or sol–gel routes in batch mode.
Automated surface modification.
Similarly, for the surface modification by impregnations, the catalytic performance of materials produced with a synthesis robot has been shown to be comparable to manually produced materials.14 This is even more remarkable since a multitude of factors influence the processes involved in impregnation and this is again specific to the support and the different elements to be deposited in a combinatorial approach.141,142 Sixty alumina-supported multi-component catalysts were prepared by sequential impregnation with various aqueous metal salt solutions (Ag, Ca, Co, Cr, Cu, Fe, Ga, La, Mg, Mn, Ni and Zr) and other components dosed as acids, and dried in a robot at 100 °C. However, the subsequent calcination at 500 °C was then carried out manually in individual crucibles. Except in the oxidative dehydrogenation of ethane to ethylene as catalytic test reaction, the materials were not characterized. Wafer-based catalyst libraries were also used to study impregnated catalysts.143 For this purpose, quartz wafers were bead-blasted through steel masks to create 16–16 arrays of wells. Metal oxide supports were inserted into these wells using a robot. The supports were then impregnated with premixed aqueous metal precursor solutions. Each well contained about 250 μg of catalyst, resulting in a catalyst film about 10 μm thick and 3 mm in diameter. The catalytic tests for CO oxidation/volatile organic compound (VOC) removal and water-gas shift (WGS) reaction were performed directly on the wafers, using mass spectrometry and IR thermography for analytics.
High throughput pulsed laser ablation (PLA) is another technique that was used to synthesize metal nanoparticles of Cu, Ag, Pd, Cr, Mn, Ru, Sn and Ir on the outer surface of Al2O3, CeO2, SiO2, TiO2 and ZrO2 pellets (0.4 cm diameter and 0.1 cm thick cylinders). Again, apart from electron microscopy, essentially only an analysis of catalytic performance in the partial oxidation of propylene was studied.144
State of development in catalyst synthesis.
The examples outlined above are quite representative of the (high-throughput) synthesis of bulk and supported catalysts and show that, generally, only individual synthesis steps of the mostly multi-stage processes have been automated. Samples have to be manually transferred from step to step, e.g., from hydrothermal synthesis or impregnation to drying and then to calcination. There were some early attempts to integrate different wet chemical steps, such as hydrothermal synthesis and the subsequent washing and filtration steps.133
It is also striking that there has been no significant technical progress in this area over the last 5–10 years. Neither has automation been increasingly introduced, nor has there been significant progress in the better integration of synthesis steps in catalyst synthesis or the integration of synthesis, characterization and functional analysis. Parallelized zeolite synthesis, for example in the laboratory of Avelino Corma at the Instituto de Tecnología Química (ITQ) in Valencia, Spain, has led to the discovery of a whole range of new zeolites with great potential for technical applications.145–147 However, the overall progress in catalysis research in general was apparently not sufficient to justify the considerable instrumental and programming efforts and investments required for fully automated synthesis in a closed loop without manual intervention, from the preparation of the starting solutions to the activation of the catalyst precursors in the reaction gas. Thus, the technical development seems to have stagnated in the last decade. The development of fully integrated systems in individual academic research groups working in the field of interfacial catalysis is particularly challenging due to the need for expertise in very different areas in such projects. For professional solutions, it therefore seems worthwhile to bundle knowledge and experience in natural science, engineering and computer technology fields in competence centers.
Integration and automation of characterization.
Autonomy can be implemented in research areas where methods are available that can provide sensory input. The combinatorial hydrothermal synthesis was coupled with direct characterization of the synthesis product by automated microdiffraction using the example of zeolite TS-1 in model experiments already carried out long ago.103,132,133,148,149 On the basis of commercial software, methods for the automatic decoding of XRD patterns were developed, enabling crystallographic phases to be identified in phase mixtures quickly and reliably.150 Optical methods are also suitable for the automated characterization of solids.149,151–153 Polycrystalline perovskite samples were synthesized in a synthesis robot and the optical band gap was directly determined by means of photoluminescence (PL) and absorption.154 But even if individual methods such as XRD, Raman spectroscopy, EDX and UV/vis spectroscopy are automated, sample transfer from synthesis to analytical instruments and from instrument to instrument is generally required, as was the case, for example, in the synthesis and optimization of multicomponent oxides and high-entropy materials.28,155
Synthesis control through feedback loops.
Sensor-technologies can also be used during the synthesis of inorganic catalyst materials, as the typical parameters of a condensation reaction, e.g., in the aqueous phase, such as temperature, pressure, pH value or conductivity, or information about the dosage of starting substances can be easily recorded and controlled. However, in parallelized experiments, online analytics could represent a significant cost factor. Co-precipitation156,157 and hydrothermal synthesis158–160 have been performed in single automated synthesis reactors equipped with a variety of probes. Automation has proved to be a major advantage here, as the reproducibility of catalyst syntheses in a batch size, which is required for comprehensive material analysis and functional testing, has been significantly improved compared to experiments carried out manually.161 In addition, the complete and uninterrupted recording of synthesis parameters and spectroscopic or other physicochemical data during synthesis provides fundamental insights that can be used for the targeted control of the polycondensation reactions. The changes in temperature, pH and turbidity measured during co-precipitation and ageing of Cu–Zn hydroxy carbonates in an automated laboratory reactor (LabMax, Mettler-Toledo) are exemplarily shown in Fig. 3. The double-walled glass reactor with an effective volume of 2.5 L heated with oil was equipped with a stirrer, two balances and two membrane pumps for dosing the metal salt solutions and the precipitating agent, and probes for measuring temperature, pH, conductivity, and turbidity. The thermostatic control guarantees excellent temperature regulation and isothermal conditions when needed. All data from the sensors are permanently measured, processed by a computer and used to control the precipitation reaction in feedback loops. For example, in the experiment shown in Fig. 3 the pH was kept constant at 6.5 during simultaneous addition of the mixed metal nitrate solution and the dissolved precipitating agent Na2CO3. The transformation of the amorphous precipitate formed initially into a crystalline phase mixture of malachite (Cu,Zn)2(OH)2CO3 and aurichalcite (Zn,Cu)5(OH)6(CO3)2, already visible due to the color change, occurs after about 60 minutes of ageing at 65 °C as it is indicated by a temporarily drop in the pH and an increase in turbidity of the mixture. Information on phase composition and particle size distribution was obtained in this experiment by manual sampling and ex situ XRD and SEM analyses. Based on these data, the previously in the industry empirically determined optimal pH and temperature regimes of the co-precipitation has been rationalized162 and a highly reproducible synthesis was developed on laboratory scale for the preparation of a high-performance benchmark catalyst for methanol synthesis.161 Due to the proven reproducibility of the syntheses and the recording of all data and metadata, such fully automated instruments, which are also available in smaller batch sizes for screening studies (e.g., OptiMax, Mettler-Toledo) (Fig. 3B),163,164 are routinely and exclusively used for all aqueous precipitation syntheses in our laboratory. However, the proprietary data format of the manufacturer makes it difficult to automatically upload the synthesis data into a database and link this data to the database entry of the precipitate. A Python-based routine is currently used for this step.
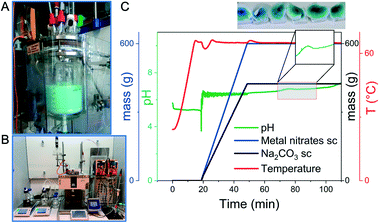 |
| Fig. 3 (A) Automated precipitation reactor (2.5 L) for larger scale synthesis and (B) for screening experiments (1 L); (C) synthesis protocol for the precipitation of a Cu–Zn hydroxycarbonate as precursor for a methanol synthesis catalyst; in this experiment the temperature, the masses of added metal nitrates and sodium carbonate as precipitating agent and the pH were recorded. The photos of the filtered samples illustrate the color change during ageing due to phase transitions. | |
In situ investigation of catalyst synthesis.
In addition to the standard reaction parameters, spectroscopic, diffraction or scattering signals could also be used as analytical input. Nucleation and growth have a major influence on the material and functional properties of solid catalysts, and these processes can be influenced by numerous process parameters during solid state formation. Analytical tracking of nucleation is a major challenge due to the small size of the nuclei and limitations regarding the sensitivity of methods. Instead, by combining spectroscopy and diffraction techniques, both the chemical reactions of molecular precursors and intermediates and the formation of long-range order can be followed before and after nucleation. However, solid formation reactions, i.e., the evolution of long-range order, are predominantly detectable with advanced analytical techniques that are not routinely available for the study of suspensions and cannot be easily coupled with synthesis reactors in a normal laboratory. These are, for example, methods such as neutron or X-ray diffraction, which in combination with the analysis of pair distribution functions (PDF) can also provide information about the density and distribution of atoms in disordered amorphous structures in early stages of particle growth.165 To study the formation of solids in realistic environments, e.g. under hydrothermal conditions, synchrotron sources with high brilliance are often used for techniques such as small-angle X-ray scattering (SAXS), wide-angle X-ray scattering (WAXS), energy dispersive X-ray diffraction (EDXRD) and X-ray absorption spectroscopy (XAS) in single and combined experiments.166–172 The use of synchrotron radiation excludes routine use in the synthesis laboratory. Similarly, this is the case with X-ray spectroscopy, e.g., quick-scanning extended X-ray absorption fine-structure spectroscopy (QEXAFS), which provides access to information about changes in local coordination in molecular precursors and during solid state formation.172–174
Early stages of solid formation can also be investigated with high-resolution transmission electron microscopy, primarily at low temperatures on samples that have been quenched and extracted from the synthesis vessel.175 Due to the formation of reactive radicals by electron beam radiolysis of the solvent, however, the method is less suitable for in situ investigations.176 In addition, the local information obtained by electron microscopy is highly complex and the measurement, even if user-friendly routines such as those developed for the ChemiTEM are used,177 is time-consuming. Therefore, electron microscopy is less suitable for providing a simple sensory signal to guide a synthesis in direct feedback loops.
Multinuclear magic-angle-spinning solid-state nuclear magnetic resonance (MAS ssNMR) spectroscopy and NMR spectroscopy in the liquid phase are also methods that can be applied to withdrawn samples.178–180 NMR spectroscopy may also be done in operando, but relies on sealed rotors or tubes. Although cells have been developed that can be used even at high temperatures and pressures,181 this only allows syntheses to be followed in the batch size of these rotors and in this specific environment. In contrast, online NMR182 but also FTIR spectroscopy183,184 are common methods used for monitoring and controlling organic reactions in the homogeneous phase in real time in flow processes.
Cluster ions from solution can be detected using electrospray ionization (ESI) combined with mass spectrometry (MS).185,186 Samples must be extracted and transferred to the gas phase. However, only highly diluted solutions can be examined, which are generally not representative of the ion concentration under synthesis conditions. In addition, structural changes can occur during the transfer to the gas phase, as the solvate shell is removed.187
More suitable for laboratory applications are light scattering techniques using optical fibres, which can be applied to track the change in particle size distribution with time.188
Similarly, UV/vis, FTIR and Raman spectroscopy (Fig. 4) are suitable for structural investigations of molecular precursors, intermediates, structuring agents and additives, secondary units and amorphous as well as crystalline phases under synthesis conditions.158,159,189 These investigations can be carried out directly in synthesis reactors using probes. Complementary attenuated total reflection (ATR) Fourier transform infrared spectroscopy (FTIR) can be used to investigate dissolution and re-precipitation processes at the solid–liquid interface.190–193 ATR-FTIR is also applied to track the progress of the catalysed reaction in the homogeneous phase online.184
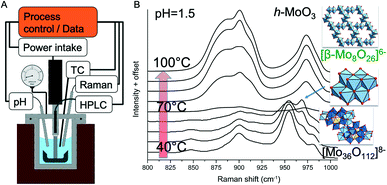 |
| Fig. 4 (A) Schematic of an automated, analytical autoclave for hydrothermal synthesis; temperature, pressure, stirring rate, power input of the stirrer, pumping rate of two HPLC pumps, pH and Raman spectra can be measured and used for experiment control; sampling is possible with a dip tube; (B) the example shows Raman spectra recorded with increasing temperature at pH 1.5 during precipitation of molybdenum oxide in the closed autoclave starting from a supramolecular precursor via octamolybdate as an intermediate. | |
The layout of a reactor for hydrothermal synthesis combined with Raman spectroscopy is shown in Fig. 4. The reactor was used to investigate the conversion of polyoxometalates and their condensation into oxide nanoparticles by Raman spectroscopy. The simultaneous determination of acid–base equilibria under hydrothermal conditions provided insights into the molecular processes of self-assembly and precursor formation of highly complex metastable phases. With this knowledge, polycrystalline MoV(Te,Nb) mixed oxides with orthorhombic structure were prepared in sequences of reactions in which different secondary building units were successively assembled to form the target phase.158 The study of the condensation reactions of polyoxometalates under hydrothermal conditions provided the direct experimental evidence that the speciation is very different from that under normal conditions, which has important implications for hydrothermal synthesis strategies.159,160 In these experiments, however, there was no direct feedback from the spectroscopic signal to the control variables of the synthesis. The evaluation of the data was only carried out by the researcher who also drew the conclusions for further experimental planning and performed the modified synthesis experiments.
Flow syntheses.
Continuous flow processes offer the potential for controlled and reproducible synthesis of nanomaterials with the advantage that they can be performed both on a large scale and as a high-throughput experiment.194–196 Also, the number of components in a flow device is often smaller and the flow scheme is similar to the scheme of equipment used for testing catalysts in thermal catalysis, which could facilitate automation and integration. In continuous precipitation reactions, variable supersaturation rates due to local inhomogeneities in pH and metal ion concentrations at the drop-in point can be avoided and also residence times can be better and in a wider range controlled than in a batch reactor. This results in more homogeneous products with tunable particle size and morphology. Different (micro)reactor technologies for mixing solutions have been investigated and tested.194,197 Precipitation can also be carried out under supercritical conditions by mixing a stream of aqueous metal salt solutions with a stream of supercritical water, where the molecular precursors are rapidly converted into oxide nanoparticles by simultaneous hydrolysis and dehydration.195,198 Parallelization of continuous flow processes is possible, but not necessarily required, as syntheses can be carried out sequentially in a short time by varying the relative concentrations of the metal salt precursors used while carefully avoiding cross-contaminations. Thus, the phase spaces of materials can be explored in a wide range with high information density by means of comparatively low investment costs (Fig. 5).196,199 In supercritical hydrothermal synthesis, the otherwise necessary thermal pretreatment of catalysts could also be omitted, since the critical temperature of water, for example, at 373.95 °C, is above the typical reaction temperature of at least some thermally catalyzed reactions and the operation temperature of electrodes in electrocatalytic conversions. Washing and ageing steps, which are important for precipitation at normal pressure, have not yet been considered in continuous operations, which poses a challenge for integrated processes to be developed in the future.194
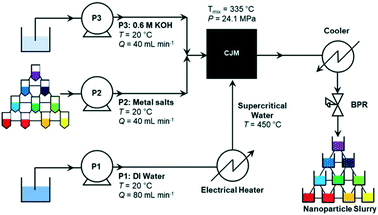 |
| Fig. 5 Flow chart for the synthesis of a BaxSryCazTiO3 sample nanoparticle library of candidate ORR catalysts composed of 66 materials. P1 is pump 1, P2 is pump 2, and P3 is pump 3. BPR stands for back-pressure regulator and CJM stands for confined jet mixer. Reprinted with permission from A. R. Groves, T. E. Ashton, J. A. Darr, ACS Combinatorial Science, 2020, 22, 750–756. Copyright 2022 American Chemical Society. | |
Classical solid-state synthesis techniques have also been applied to synthesize ceramic materials and catalysts for high-temperature applications by automated parallelized ball milling and consecutive heat treatment.200–202 Again, continuous methods such as aerosol techniques can be automated and integrated with less effort, contributing to the generation of catalyst libraries for high-temperature processes through automated sequential syntheses.203 Aerosol technologies including spray drying or flame spray pyrolysis are well established and are now routinely used to produce a wide range of materials, such as fumed silica, zinc oxide or titania, but also more complex oxides and other compounds, such as high entropy alloy nanoparticles,204 in powder form. Larger scale fabrication is also possible with these methods.
Microfluidic techniques that can be used to vary the composition and morphology of (multi)metallic catalyst nanoparticles should also be mentioned as another interesting high-throughput method, although here the scale is very small in terms of the amount of synthesis product.205–207 Possibilities for upscaling such syntheses have already been investigated.208 The synthesis can also be coupled with plasma treatment.209
2D catalysts.
Thin film materials in 2D geometry have long been studied in catalysis, primarily as model systems62,210–214 or in high-throughput experiments.215,216 In interfacial catalysis, thin-film technologies are important for electrocatalytic or photocatalytic applications.217,218 But they are also receiving considerable attention in the introduction of alternative micro-structured reactor technologies.219 This is partly because completely different reactivities can be expected in thin films than in their bulk analogues due to geometric constraints in the film and electronic effects caused by the specific interaction with the substrate or a stacked configuration of different layers. In addition, the 2D arrangement enables the development of new forms of energy input, such as resistance heating or inductive heating.220,221
Widely used methods of synthesizing thin-film catalysts include solvent-based processes such as dip coating, drop casting, spin coating, inkjet printing, electrochemical deposition and combinations thereof.222–225 Films can also be grown under solvothermal conditions.226 Mixed metal oxide libraries for V–Al–Nb and Cr–Al–Nb based oxidation catalysts were prepared using sol–gel techniques by applying alkoxide solutions to quartz wafers with automatic dispensing robots.216 More recently, multicomponent mesoporous metal oxides were fabricated using inkjet printing.227 During printing, the inks are applied drop by drop, the drops flow together and layers are formed after the solvent evaporates. In this way, three-dimensional systems can also be created layer by layer.228 However, in catalysis research, filter papers are often printed which burn during subsequent calcination to yield conventional 3D catalysts, such as in a study of LiMgMnOx–La2O3 catalysts for the oxidative coupling of methane.229 Rather, inkjet printing was used here to produce thousands of different formulas precisely and quickly in a combinatorial approach. The method was also applied for example to deposit metal nanoparticles on nanostructured carbon substrates for the electrochemical oxygen reduction reaction (ORR).230
For deposition from the gas phase methods such as pulsed laser deposition, radiofrequency magnetron sputtering, spray pyrolysis, and chemical or physical vapor deposition are used. Rh–Pt–Cu and Rh–Pd–Cu libraries for the oxidation of CO and NO were for example synthesized by radiofrequency (RF) sputtering through masks onto a quartz wafer in a combinatorial study. The phase diagrams of 3 metals were mapped in this way, where each catalyst had a diameter of 1.5 mm and a thickness of approximately 100 nm.215 Physical vapor deposition (PVD) has been applied to the synthesis of a variety of thin film catalysts. Examples include the synthesis of mixed oxides, such as La–Mn–Ni perovskites as thin film catalysts for the oxygen reduction/evolution reaction,231 or the synthesis of CuW binary alloy thin films tested in the hydrogen evolution reaction.232
A semi-automatic, continuous, laboratory-scale chemical vapor deposition (CVD) furnace system (Robofurnace) was developed for the development of a rapid CVD formulation for the growth of a carbon nanotubes (CNTs) forest on a silicon substrate, with a 10-fold increase in CNT mass density compared to a reference formulation using a manual tube furnace.233
Pulsed laser deposition (PLD) can be used to rapidly deposit various materials on flat substrates. Sub-monolayers, thickness gradients or complex layered composites of chemically variable compounds such as mixed oxides234 or metal nanoparticles can be deposited.144
Flame spray pyrolysis is suitable for the fabrication of layers, which is important for the preparation of sensors, electrodes or 2D catalysts for thermal catalysis.235 With this method, crack-free coatings can be achieved. Cracks occur in conventional solvent-based methods of coating (drop coating, dip coating, spin coating) due to capillary forces occurring during the removal of solvents.
The examples show that both the variety of materials and the preparation methods available for 2D catalysts are similarly diverse as for powder catalysts. In the case of wet chemical methods in particular, it has already been shown in the context of combinatorial catalysis research that at least the wet chemical synthesis steps can be automated using dispensing robots and inkjet printers. Thermal post-treatment was carried out in separate steps in other set-ups, and there was generally no integration of the different synthesis steps.138,139,143,227 Since, apart from very simple methods such as dip coating, thin films are largely synthesized in dedicated and advanced equipment, there is a good basis for recording all synthesis parameters and developing automated syntheses controlled by feedback from analytical data as has been already demonstrated for example for autonomous fabrication of Nb-doped TiO2 thin films with minimized electrical resistance by reactive magnetron sputter deposition.236
Closed-loop systems
Research projects in catalysis usually start with (i) the development of a catalyst synthesis concept based on the analysis of the present knowledge, followed by (ii) catalyst synthesis including the formation of the active phase and (iii) comprehensive analysis of the materials and functional properties. The results are (iv) evaluated, interpreted and (v) the conclusions are implemented into an improved synthesis concept or into adapted formation or reaction conditions by human researchers (Fig. 6, left). These feedback loops are time-consuming, so progress is slow even if individual steps are automated or parallelised. The whole process could be carried out autonomously by robots, saving time and resources and exploring a larger parameter space more effectively. However, this would require the complete automation of all unit operations, the online acquisition of spectroscopic and other analytical data and their evaluation in real time in order to be incorporated into further experimental design by machine learning algorithms. All data generated could then be automatically stored in databases (Fig. 6, right) and made available for own or independent data analysis and future research in the same or even completely different research fields.
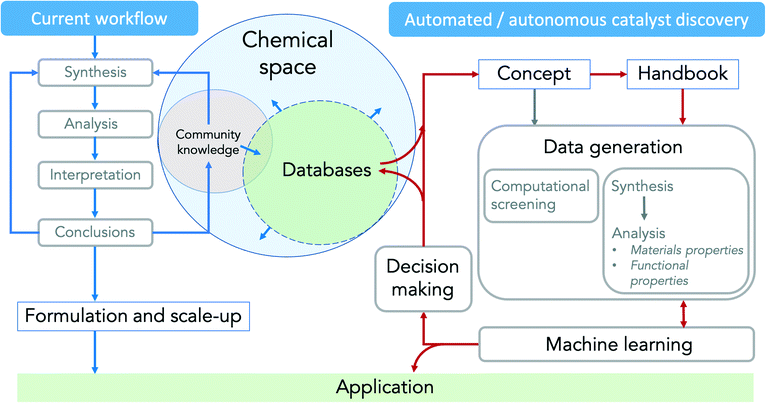 |
| Fig. 6 Feedback loops in current (left, blue arrows) and automated/autonomous (right, red arrows) catalyst discovery. | |
However, examples of pioneering work towards a truly autonomous development of a heterogeneous catalyst are still lacking. One reason could be the enormous complexity of the experiments in all stages of the respective workflow. As shown above, the synthesis of catalyst precursors is a multi-step process, and the process parameters and properties of the intermediates are generally not analytically detected or are recorded poorly because the corresponding methods are either particularly expensive and specialized or have not yet been developed. The material analysis of a catalyst in all its life phases from the precursor via the activated and working material to the used or deactivated catalyst requires the application of a multitude of physical and chemical methods in usually interdisciplinary research (see for example Fig. 2). Operando experiments in particular are multidimensional and involve the linking of advanced spectroscopic, diffraction or other analytical experiments, often performed at synchrotron light sources where the light source alone is an extremely complex instrument, with simultaneous product analysis. This means that not only the automation and integration of technically very different and intricate workflows has to be solved by elaborate engineering, but also an enormously large number of different analytical data has to be collected and processed simultaneously. Besides these technical and data challenges, there is an even more fundamental problem. The entanglement of solid-state and reaction kinetics explained in chapter 2 is the reason why in catalysis generally no simple correlations between material properties and catalytic function are found69,237 and why it is important to collect a large amount of diverse analytical data preferably on the working catalyst in operando experiments.69 And this, in turn, is the reason why it will not be possible to create a simple demonstration example with reasonable effort in which a catalytic function, for example, a reaction rate or a selectivity is optimized one-dimensionally by varying only one key parameter by, for example, modifying the synthesis, formation, or reaction conditions to convincingly demonstrate the value and potential of autonomous catalyst development.
Autonomous syntheses of molecules.
In contrast, in the synthesis of organic molecules23,24,27,238–240 and to some extent in materials research,236,241 interesting case studies on autonomous synthesis have already been carried out, clearly showing the potential (Table 1). An organic synthesis robot was used to investigate the chemical reactivity of multi-component mixtures.239 Input was generated by online analysis using NMR, mass and ATR infrared spectroscopies. The data were evaluated with a machine learning algorithm to automatically categorize the reaction mixtures according to their reactivity. For this purpose, the model was trained on 72 reactive and non-reactive homogeneous mixtures. The spectra of the starting compounds were compared with those of the mixtures, and if there were differences, the corresponding measurement was recorded as a reactivity hit.
Table 1 Examples of autonomous molecule and material synthesis
Material |
System |
Synthesis method |
Analytical input |
Target function |
Ref. |
Scavenger (dyes) in combination with a conjugated polymer (P10) |
Suspension |
Mixing and suspending |
Hydrogen evolution [μmol] |
Hydrogen evolution rate in photocatalytic water splitting |
Burger 2020 (ref. 240) |
C81H68N4O8 (spiro-OMeTAD) |
Thin film of molecules on a glass substrate |
Spin coating and drying in hot (165 °C) air |
Dark-field photography |
Hole mobility represented by a calculated pseudomobility |
MacLeod 2020 (ref. 223) |
UV/vis/NIR |
Four-point probe conductivity |
Nb–TiO2 |
Thin film on glass substrate |
Reactive magnetron sputter deposition in presence of oxygen |
Electrical resistance |
— |
Shimizu 2020 (ref. 236) |
Organic molecules |
Homogeneous liquids |
Liquid dosing and mixing |
NMR |
— |
Granda 2019 (ref. 239) |
ATR |
Carbon nanotubes |
|
Cold-wall CVD |
Raman |
Growth rate [s−1] |
Nikolaev 2014, 2016 (ref. 241 and 245) |
In a photocatalytic study on water splitting using the conjugated polymer P10 as catalyst, biological hole scavengers such as the organic molecules methylene blue, acid red 87, or rhodamine B were searched for, which are comparable in their efficiency to petrochemical amines.240 Further optimization parameters were pH, ionic strength and the presence of surfactants. A mobile robot was used for the quest, which carried out autonomous experiments controlled by a Bayesian search algorithm.242 The target variable was the amount of hydrogen produced in photocatalytic water splitting over P10 in presence of hole scavengers and additives, which was determined using head space gas chromatography. This autonomous screening yielded photocatalyst mixtures that were six times more active than the original formulations.
In a final example for molecular systems, hole mobility was optimized in thin films of spiro-OMeTAD, an organic molecule with the molecular sum formula C81H68N4O8 used as hole transport material (HTM) in perovskite solar cells (PSCs), on microscope slide substrates.223 The hole mobility of spiro-OMeTAD is crucial for solar cell performance. However, it is very sensitive to the parameters of the spin coating process, such as dopants, additives, spin coating solvents and the post-processing conditions. The optimization of the film synthesis was carried out with the help of a robotic platform called “Ada”, with which solutions can be autonomously prepared and mixed, deposited as thin films on rigid substrates and annealed. The system also independently examines the morphology of the prepared layers and their optical and conductive properties. The hole mobility served as the target parameter, but this cannot be easily measured. Therefore, a method was developed that uses four-point probe conductivity measurements and UV-vis-NIR spectroscopy to determine a diagnostic parameter, called pseudo-mobility, which is proportional to the hole mobility. The data were transferred to the software package ChemOS,243 which uses the global Bayesian optimization algorithm Phoenics244 to design new experiments by active learning from the previously collected data to maximize hole mobility in this proof-of-principle study.
Autonomous syntheses of solids.
The autonomous synthesis of solids is even more demanding experimentally. One example with a strong link to heterogeneous catalysis is the development of an autonomous research system (ARES) for the synthesis of single-walled carbon nanotubes (CNTs) with an optimized growth rate as the target parameter.241,245 The system is able to learn how to grow CNTs through a chemical vapor deposition (CVD) process using an artificial intelligence planner that specifies the growth conditions – temperature, pressure and partial pressures of ethylene, hydrogen and water vapor – based on the evaluation of a Raman signal as feedback control. Interestingly, the ARES heating laser used to set the reaction temperature also served as the excitation source for Raman spectroscopy, allowing the intensity of the G-band in the CNT spectrum to be detected, and its increase over time was used to determine the CNT growth rate. Due to the autonomous feedback and the associated high iteration rate, the system was able to optimize several experimental parameters simultaneously and achieve convergence, which shows the potential for solving complex problems in materials research.
In another example, the electrical resistance of Nb-doped TiO2 thin films on a glass substrate was minimized by combining Bayesian optimization with robotics.236 The thin film was prepared by reactive magnetron sputter deposition. The system then employs the machine learning algorithm to predict the oxygen partial pressure during the sputtering process that leads to a minimum electrical resistance of the synthesis product, which is automatically measured in the integrated setup.
In the last two examples, where solids were synthesized, only one response variable was recorded as an input variable for the artificial intelligence in each case (intensity of a Raman band, electrical resistance). This will not be sufficient for optimizing a catalysis function, as descriptors are generally complex correlations (Fig. 1C).69 This means that autonomous systems for catalysis research must be much more complex in design and process significantly more data. And it also means that analytical methods have to be adapted or specially developed in order to be implemented online and evaluated in real time.
5. Digitalization of catalysis
Advances in high-throughput and combinatorial research have shown that robots can support the search for new catalysts by relieving researchers of routine tasks and producing and analyzing vast numbers of samples in a short time in separate and sequential operations. However, integrating all these steps into an artificial intelligence-driven system for autonomous catalyst discovery is technically challenging on its own due to the diversity of materials and the complexity of the workflows using a large number of different instruments and methods as outlined above. The dilemma in pushing this technical development forward is that due to the intricacy of the relationships between material and functional properties in catalysis, it will be extremely difficult to create a proof-of-principle example without immense engineering, programming and computing effort. Probably only national or international-wide efforts could establish a demonstration laboratory that can cope with the complexity of the problem.
A more pragmatic and short-term approach may be to apply artificial intelligence methods to highly reproducible, precisely measured and sufficiently diverse data sets to decipher the intrinsic physical properties of the materials responsible for the desired function, or the so-called “genes” of a catalyst.69,246
To ensure data quality, it is necessary to work according to rigorous procedures80,83,84,247 that are usefully documented in handbooks (Fig. 6).70 If there were a broad consensus in the research community on working according to standard operating procedures, the use of shared benchmarks for data verification and an open attitude towards making research data available in publicly accessible repositories, then sufficient reliable data would be available for analysis by data science. High-throughput experiments in local laboratories with their limitations in terms of batch size, realistic reaction conditions, lack of accuracy, analytical data and real-time analytical feedback would then no longer really be necessary.
When working according to handbooks, the catalyst synthesis must be precisely documented and provide a sufficiently large batch size so that all kinetic, characterization and operando experiments can be performed on only one batch. There is a great need for the development of new and the implementation of known online analytical techniques to monitor and document all data and metadata of the synthesis. This alone will greatly increase the reproducibility of catalyst synthesis. In functional analysis, the reaction conditions must be investigated in a broad and very diverse parameter space and the catalysis experiments must be performed for a sufficiently long time to be able to study formation and deactivation phenomena. Not only the freshly synthesized catalyst precursor should be comprehensively characterized, but also activated and used samples. If possible, the characterization should be carried out under reaction conditions with simultaneous analysis of the catalytic performance. This means that operando experiments must become the rule rather than the exception.
In addition, the theoretical understanding of elementary processes at the atomic level has gained enormously in importance in the past decades and the search for new catalysts is increasingly also being carried out on the basis of computational screening, which leads to a multiplication of potentially interesting catalysts. The feasibility of fully automated computational screening through the combination of machine learning and optimization has also already been demonstrated.248 Here, too, methodological challenges have to be solved in order to avoid chemically unrealistic and non-synthesizable proposals.249 The hits identified by theory in turn can then be verified in experiments according to handbooks.
Working according to rigorous protocols is facilitated by automating unit operations and, in turn, handbook instructions provide the necessary specifications for automation. Thus, these two ways of working are mutually supportive. Automated procedures should involve the recording and automatic uploading of all data and metadata into repositories (Fig. 6) and linking these data to all available information on the catalyst material under investigation.250
6. Conclusions
In summary, the autonomous development of catalysts requires a complete integration of the very complex and multifaceted experimental and theoretical steps in catalysis research, especially the integration of operando experiments under realistic conditions. Such an effort is costly, time-consuming and personnel-intensive. To this end, the integration of computational chemistry and robotics, which were not the subject of the present analysis, and experiment must be further advanced. To demonstrate the benefits of artificial intelligence and thus drive development, a step-by-step approach such as the following seems more feasible:
• Combining the handbook concept, including the analysis of benchmark catalysts, with the automation of experiments and calculations and the storage of data in databases will lead to more data that can be reasonably evaluated using machine learning methods. This will accelerate the discovery of new catalysts and processes, even if not all steps of catalysis research have yet been integrated into autonomously operating systems and at the same time lays the technical foundations for the autonomous processing of certain work packages by robots.
• In order to make standard operating procedures machine-readable, an ontology of catalysis research must be developed.
• The measurement of structural and electronic properties of the catalyst under realistic working conditions through operando investigations is particularly important because of the link between the chemistry of the catalyst and the reaction medium. Further technical developments are required for autonomous catalyst development, including the integration of operando techniques into automated workflows and real-time analysis of operando data.
• An important requirement in this context is that local data infrastructures must be adapted or established and their linkage with higher-level repositories must be ensured in order to make the data available to the community after publication in accordance with the FAIR principle.251 An evaluation of the data of different working groups with one question or the evaluation under completely different aspects can lead to ground-breaking new insights.
• Given the need to find robust solutions to challenging questions of energy conversion and ensuring sustainable material cycles, the target variable in both autonomous and human investigator-driven catalysis research must always be the macro-kinetic performance of the catalyst, which necessarily requires scaling up batch sizes and incorporating catalyst shaping already in fundamental and screening studies.
Conflicts of interest
There are no conflicts to declare.
Acknowledgements
My thanks go to Robert Schlögl for the constructive discussion on the manuscript, and to Clara Patricia Marshall for providing figures. The article was written as part of the authors' activities in the FAIR Data Infrastructure for Condensed-Matter Physics and the Chemical Physics of Solids (FAIRmat) consortium of the German Research-Data Infrastructure NFDI founded by the German Research Foundation. Open Access funding provided by the Max Planck Society.
Notes and references
- A. J. Martín, T. Shinagawa and J. Pérez-Ramírez, Chem, 2019, 5, 263–283 Search PubMed.
- G. Centi and J. Čejka, ChemSusChem, 2019, 12, 621–632 CrossRef CAS PubMed.
- J. Artz, T. E. Müller, K. Thenert, J. Kleinekorte, R. Meys, A. Sternberg, A. Bardow and W. Leitner, Chem. Rev., 2018, 118, 434–504 CrossRef CAS PubMed.
- J. G. Chen, R. M. Crooks, L. C. Seefeldt, K. L. Bren, R. M. Bullock, M. Y. Darensbourg, P. L. Holland, B. Hoffman, M. J. Janik, A. K. Jones, M. G. Kanatzidis, P. King, K. M. Lancaster, S. V. Lymar, P. Pfromm, W. F. Schneider and R. R. Schrock, Science, 2018, 360, eaar6611 CrossRef PubMed.
- R. Schlögl, Top. Catal., 2016, 59, 772–786 CrossRef.
- H. Schulz, Appl. Catal., A, 1999, 186, 3–12 CrossRef CAS.
-
A. Mittasch and W. Frankenburg, in Advances in Catalysis, ed. W. G. Frankenburg, V. I. Komarewsky and E. K. Rideal, Academic Press, 1950, vol. 2, pp. 81–104 Search PubMed.
-
R. Schlögl, in Handbook of Heterogeneous Catalysis, Wiley-VCH Verlag GmbH & Co. KGaA, 2008, DOI:10.1002/9783527610044.hetcat0129.
- J. W. Erisman, M. A. Sutton, J. Galloway, Z. Klimont and W. Winiwarter, Nat. Geosci., 2008, 1, 636–639 CrossRef CAS.
- J. Greeley, T. F. Jaramillo, J. Bonde, I. Chorkendorff and J. K. Nørskov, Nat. Mater., 2006, 5, 909–913 CrossRef CAS PubMed.
- B. Jandeleit, D. J. Schaefer, T. S. Powers, H. W. Turner and W. H. Weinberg, Angew. Chem., Int. Ed., 1999, 38, 2494–2532 CrossRef CAS.
- A. Hagemeyer, B. Jandeleit, Y. Liu, D. M. Poojary, H. W. Turner, A. F. Volpe and W. Henry Weinberg, Appl. Catal., A, 2001, 221, 23–43 CrossRef CAS.
- S. Senkan, Angew. Chem., Int. Ed., 2001, 40, 312–329 CrossRef CAS PubMed.
- I. Hahndorf, O. Buyevskaya, M. Langpape, G. Grubert, S. Kolf, E. Guillon and M. Baerns, Chem. Eng. J., 2002, 89, 119–125 CrossRef CAS.
- A. C. van Veen, D. Farrusseng, M. Rebeilleau, T. Decamp, A. Holzwarth, Y. Schuurman and C. Mirodatos, J. Catal., 2003, 216, 135–143 CrossRef CAS.
- W. F. Maier, ACS Comb. Sci., 2019, 21, 437–444 CrossRef CAS PubMed.
- D. Farrusseng, Surf. Sci. Rep., 2008, 63, 487–513 CrossRef CAS.
- B. Rohr, H. S. Stein, D. Guevarra, Y. Wang, J. A. Haber, M. Aykol, S. K. Suram and J. M. Gregoire, Chem. Sci., 2020, 11, 2696–2706 RSC.
- K. McCullough, T. Williams, K. Mingle, P. Jamshidi and J. Lauterbach, Phys. Chem. Chem. Phys., 2020, 22, 11174–11196 RSC.
- H. S. Stein and J. M. Gregoire, Chem. Sci., 2019, 10, 9640–9649 RSC.
- X. Liu, B. Liu, J. Ding, Y. Deng, X. Han, C. Zhong and W. Hu, Adv. Funct. Mater., 2022, 32, 2107862 CrossRef CAS.
- G. Pilania, Comput. Mater. Sci., 2021, 193, 13 CrossRef.
- C. W. Coley, N. S. Eyke and K. F. Jensen, Angew. Chem., Int. Ed., 2020, 59, 22858–22893 CrossRef CAS PubMed.
- C. W. Coley, N. S. Eyke and K. F. Jensen, Angew. Chem., Int. Ed., 2020, 59, 23414–23436 CrossRef CAS PubMed.
- T. Dimitrov, C. Kreisbeck, J. S. Becker, A. Aspuru-Guzik and S. K. Saikin, ACS Appl. Mater. Interfaces, 2019, 11, 24825–24836 CrossRef CAS PubMed.
- D. P. Tabor, L. M. Roch, S. K. Saikin, C. Kreisbeck, D. Sheberla, J. H. Montoya, S. Dwaraknath, M. Aykol, C. Ortiz, H. Tribukait, C. Amador-Bedolla, C. J. Brabec, B. Maruyama, K. A. Persson and A. Aspuru-Guzik, Nat. Rev. Mater., 2018, 3, 5–20 CrossRef CAS.
- P. S. Gromski, A. B. Henson, J. M. Granda and L. Cronin, Nat. Rev. Chem., 2019, 3, 119–128 CrossRef.
- Q. Wang, L. Velasco, B. Breitung and V. Presser, Adv. Energy Mater., 2021, 11, 2102355 CrossRef CAS.
- E. Stach, B. DeCost, A. G. Kusne, J. Hattrick-Simpers, K. A. Brown, K. G. Reyes, J. Schrier, S. Billinge, T. Buonassisi, I. Foster, C. P. Gomes, J. M. Gregoire, A. Mehta, J. Montoya, E. Olivetti, C. Park, E. Rotenberg, S. K. Saikin, S. Smullin, V. Stanev and B. Maruyama, Matter, 2021, 4, 2702–2726 CrossRef.
- E. J. Braham, R. D. Davidson, M. Al-Hashimi, R. Arróyave and S. Banerjee, Dalton Trans., 2020, 49, 11480–11488 RSC.
- N. J. Szymanski, Y. Zeng, H. Huo, C. J. Bartel, H. Kim and G. Ceder, Mater. Horiz., 2021, 8, 2169–2198 RSC.
- K. M. Jablonka, D. Ongari, S. M. Moosavi and B. Smit, Chem. Rev., 2020, 120, 8066–8129 CrossRef CAS PubMed.
- S. M. Moosavi, K. M. Jablonka and B. Smit, J. Am. Chem. Soc., 2020, 142, 20273–20287 CrossRef CAS PubMed.
- Y. N. Guan, D. Chaffart, G. H. Liu, Z. Y. Tan, D. S. Zhang, Y. J. Wang, J. D. Li and L. Ricardez-Sandoval, Chem. Eng. Sci., 2022, 248, 20 CrossRef.
- N. S. Eyke, B. A. Koscher and K. F. Jensen, Trends Chem., 2021, 3, 120–132 CrossRef CAS.
- C. Chen, Y. Zuo, W. Ye, X. Li, Z. Deng and S. P. Ong, Adv. Energy Mater., 2020, 10, 1903242 CrossRef CAS.
- W. Sha, Y. Guo, Q. Yuan, S. Tang, X. Zhang, S. Lu, X. Guo, Y.-C. Cao and S. Cheng, Adv. Intell. Syst., 2020, 2, 1900143 CrossRef.
- J. R. Kitchin, Nat. Catal., 2018, 1, 230–232 CrossRef.
- Z. Li, S. Wang and H. Xin, Nat. Catal., 2018, 1, 641–642 CrossRef.
- P. Schlexer Lamoureux, K. T. Winther, J. A. Garrido Torres, V. Streibel, M. Zhao, M. Bajdich, F. Abild-Pedersen and T. Bligaard, ChemCatChem, 2019, 11, 3581–3601 CrossRef CAS.
- K. Takahashi, L. Takahashi, I. Miyazato, J. Fujima, Y. Tanaka, T. Uno, H. Satoh, K. Ohno, M. Nishida, K. Hirai, J. Ohyama, T. N. Nguyen, S. Nishimura and T. Taniike, ChemCatChem, 2019, 11, 1146–1152 CrossRef CAS.
- P. S. F. Mendes, S. Siradze, L. Pirro and J. W. Thybaut, ChemCatChem, 2021, 13, 836–850 CrossRef CAS.
- E. Soedarmadji, H. S. Stein, S. K. Suram, D. Guevarra and J. M. Gregoire, npj Comput. Mater., 2019, 5, 79 CrossRef.
- C. Wulf, M. Beller, T. Boenisch, O. Deutschmann, S. Hanf, N. Kockmann, R. Kraehnert, M. Oezaslan, S. Palkovits, S. Schimmler, S. A. Schunk, K. Wagemann and D. Linke, ChemCatChem, 2021, 13, 3223–3236 CrossRef CAS.
- J. Fujima, Y. Tanaka, I. Miyazato, L. Takahashi and K. Takahashi, React. Chem. Eng., 2020, 5, 903–911 RSC.
- K. T. Winther, M. J. Hoffmann, J. R. Boes, O. Mamun, M. Bajdich and T. Bligaard, Sci. Data, 2019, 6, 75 CrossRef PubMed.
- L. Himanen, A. Geurts, A. S. Foster and P. Rinke, Adv. Sci., 2019, 6, 1900808 CrossRef PubMed.
- E. A. Pfeif and K. Kroenlein, APL Mater., 2016, 4, 053203 CrossRef.
- E. M. Hart, P. Barmby, D. LeBauer, F. Michonneau, S. Mount, P. Mulrooney, T. Poisot, K. H. Woo, N. B. Zimmerman and J. W. Hollister, PLoS Comput. Biol., 2016, 12, e1005097 CrossRef PubMed.
- A. Jain, S. P. Ong, G. Hautier, W. Chen, W. D. Richards, S. Dacek, S. Cholia, D. Gunter, D. Skinner, G. Ceder and K. A. Persson, APL Mater., 2013, 1, 011002 CrossRef.
-
A. J. Lawson, J. Swienty-Busch, T. Géoui and D. Evans, in The Future of the History of Chemical Information, American Chemical Society, 2014, ch. 8, vol. 1164, pp. 127–148 Search PubMed.
- C. Draxl and M. Scheffler, MRS Bull., 2018, 43, 676–682 CrossRef.
- S. Stocker, G. Csányi, K. Reuter and J. T. Margraf, Nat. Commun., 2020, 11, 5505 CrossRef CAS PubMed.
- C. A. Grambow, L. Pattanaik and W. H. Green, Sci. Data, 2020, 7, 137 CrossRef CAS PubMed.
- R. Schlögl, Angew. Chem., Int. Ed., 2015, 54, 3465–3520 CrossRef PubMed.
- G. Ertl, Angew. Chem., Int. Ed., 2008, 47, 3524–3535 CrossRef CAS PubMed.
- Z. W. Ulissi, A. J. Medford, T. Bligaard and J. K. Nørskov, Nat. Commun., 2017, 8, 14621 CrossRef PubMed.
- M. Boudart, Top. Catal., 2000, 13, 147 CrossRef CAS.
- M. Boudart, J. Am. Chem. Soc., 1952, 74, 1531–1535 CrossRef CAS.
- P. Mars and D. W. van Krevelen, Chem. Eng. Sci., 1954, 3(Supplement 1), 41–59 CrossRef CAS.
- R. K. Grasselli, D. J. Buttrey, P. DeSanto, J. D. Burrington, C. G. Lugmair, A. F. Volpe, Jr. and T. Weingand, Catal. Today, 2004, 91-92, 251–258 CrossRef CAS.
- J. Sauer and H.-J. Freund, Catal. Lett., 2015, 145, 109–125 CrossRef CAS.
- C. Copéret, Nat. Energy, 2019, 4, 1018–1024 CrossRef.
- C. Chizallet, Top. Catal., 2022, 65, 69–81 CrossRef CAS.
- B. W. J. Chen, L. Xu and M. Mavrikakis, Chem. Rev., 2021, 121, 1007–1048 CrossRef CAS PubMed.
- S. L. Scott, ACS Catal., 2018, 8, 8597–8599 CrossRef CAS.
- R. Ouyang, E. Ahmetcik, C. Carbogno, M. Scheffler and L. M. Ghiringhelli, JPhys Mater., 2019, 2, 024002 CrossRef.
- R. Ouyang, S. Curtarolo, E. Ahmetcik, M. Scheffler and L. M. Ghiringhelli, Phys. Rev. Mater., 2018, 2, 083802 CrossRef CAS.
- L. Foppa, L. M. Ghiringhelli, F. Girgsdies, M. Hashagen, P. Kube, M. Hävecker, S. J. Carey, A. Tarasov, P. Kraus, F. Rosowski, R. Schlögl, A. Trunschke and M. Scheffler, MRS Bull., 2021, 46, 1016–1026 CrossRef CAS PubMed.
- A. Trunschke, G. Bellini, M. Boniface, S. J. Carey, J. Dong, E. Erdem, L. Foppa, W. Frandsen, M. Geske, L. M. Ghiringhelli, F. Girgsdies, R. Hanna, M. Hashagen, M. Hävecker, G. Huff, A. Knop-Gericke, G. Koch, P. Kraus, J. Kröhnert, P. Kube, S. Lohr, T. Lunkenbein, L. Masliuk, R. Naumann d'Alnoncourt, T. Omojola, C. Pratsch, S. Richter, C. Rohner, F. Rosowski, F. Rüther, M. Scheffler, R. Schlögl, A. Tarasov, D. Teschner, O. Timpe, P. Trunschke, Y. Wang and S. Wrabetz, Top. Catal., 2020, 63, 1683–1699 CrossRef CAS.
- A. Trovarelli and J. Llorca, ACS Catal., 2017, 7, 4716–4735 CrossRef CAS.
-
F. Schüth and M. Hesse, in Handbook of Heterogeneous Catalysis, ed. G. Ertl, H. Knözinger, F. Schüth and J. Weitkamp, Wiley-VCH Verlag GmbH & Co. KGaA, Weinheim, 2008, pp. 676–699, DOI:10.1002/9783527610044.hetcat0034.
- T. Shoinkhorova, A. Dikhtiarenko, A. Ramirez, A. Dutta Chowdhury, M. Caglayan, J. Vittenet, A. Bendjeriou-Sedjerari, O. S. Ali, I. Morales-Osorio, W. Xu and J. Gascon, ACS Appl. Mater. Interfaces, 2019, 11, 44133–44143 CrossRef CAS PubMed.
-
E. T. C. Vogt, G. T. Whiting, A. D. Chowdhury and B. M. Weckhuysen, in Advances in Catalysis, ed. F. C. Jentoft, Elsevier Academic Press Inc, San Diego, 2015, vol. 58, pp. 143–314 Search PubMed.
- S. Mitchell, N.-L. Michels, K. Kunze and J. Pérez-Ramírez, Nat. Chem., 2012, 4, 825–831 CrossRef CAS PubMed.
- J. J. E. Maris, D. Fu, F. Meirer and B. M. Weckhuysen, Adsorption, 2021, 27, 423–452 CrossRef CAS.
- V. G. Baldovino-Medrano, M. T. Le, I. Van Driessche, E. Bruneel, C. Alcázar, M. T. Colomer, R. Moreno, A. Florencie, B. Farin and E. M. Gaigneaux, Catal. Today, 2015, 246, 81–91 CrossRef CAS.
- S. Mitchell, N.-L. Michels and J. Pérez-Ramírez, Chem. Soc. Rev., 2013, 42, 6094–6112 RSC.
- M. A. Bañares, Catal. Today, 2005, 100, 71–77 CrossRef.
- S. Z. Andersen, V. Čolić, S. Yang, J. A. Schwalbe, A. C. Nielander, J. M. McEnaney, K. Enemark-Rasmussen, J. G. Baker, A. R. Singh, B. A. Rohr, M. J. Statt, S. J. Blair, S. Mezzavilla, J. Kibsgaard, P. C. K. Vesborg, M. Cargnello, S. F. Bent, T. F. Jaramillo, I. E. L. Stephens, J. K. Nørskov and I. Chorkendorff, Nature, 2019, 570, 504–508 CrossRef CAS PubMed.
- J. J. Birtill, Ind. Eng. Chem. Res., 2007, 46, 2392–2398 CrossRef CAS.
- T. Bligaard, R. M. Bullock, C. T. Campbell, J. G. Chen, B. C. Gates, R. J. Gorte, C. W. Jones, W. D. Jones, J. R. Kitchin and S. L. Scott, ACS Catal., 2016, 6, 2590–2602 CrossRef CAS.
- I. Spanos, A. A. Auer, S. Neugebauer, X. Deng, H. Tüysüz and R. Schlögl, ACS Catal., 2017, 7, 3768–3778 CrossRef CAS.
- B. H. R. Suryanto, H.-L. Du, D. Wang, J. Chen, A. N. Simonov and D. R. MacFarlane, Nat. Catal., 2019, 2, 290–296 CrossRef CAS.
- L. M. Madeira, M. F. Portela and C. Mazzocchia, Journal, 2004, 46, 53–110 Search PubMed.
- K. E. Lamb, M. D. Dolan and D. F. Kennedy, Int. J. Hydrogen Energy, 2019, 44, 3580–3593 CrossRef CAS.
- Of best practice in catalysis, Nat. Catal., 2020, 3, 471–472 Search PubMed.
- J. G. Chen, C. W. Jones, S. Linic and V. R. Stamenkovic, ACS Catal., 2017, 7, 6392–6393 CrossRef CAS.
- U. I. Kramm, R. Marschall and M. Rose, ChemCatChem, 2019, 11, 2563–2574 CrossRef CAS.
- F. Schüth, M. D. Ward and J. M. Buriak, Chem. Mater., 2018, 30, 3599–3600 CrossRef.
- S. H. M. Mehr, M. Craven, A. I. Leonov, G. Keenan and L. Cronin, Science, 2020, 370, 101–108 CrossRef CAS PubMed.
- L. Wilbraham, S. H. M. Mehr and L. Cronin, Acc. Chem. Res., 2021, 54, 253–262 CrossRef CAS PubMed.
- L. Takahashi and K. Takahashi, J. Phys. Chem. Lett., 2019, 10, 7482–7491 CrossRef CAS PubMed.
- J. N. Cawse, Acc. Chem. Res., 2001, 34, 213–221 CrossRef CAS PubMed.
- F. Schuth, O. Busch, C. Hoffmann, T. Johann, C. Kiener, D. Demuth, J. Klein, S. Schunk, W. Strehlau and T. Zech, Top. Catal., 2002, 21, 55–66 CrossRef.
- K. Stöwe, C. Dogan, F. Welsch and W. F. Maier, Z. Phys. Chem., 2013, 227, 561–593 CrossRef.
- L. Lukashuk and K. Foettinger, Johnson Matthey Technol. Rev., 2018, 62, 316–331 CrossRef CAS.
- C. L. Allen, D. C. Leitch, M. S. Anson and M. A. Zajac, Nat. Catal., 2019, 2, 2–4 CrossRef.
- I. G. Clayson, D. Hewitt, M. Hutereau, T. Pope and B. Slater, Adv. Mater., 2020, 32, 2002780 CrossRef CAS PubMed.
- J. Klein, T. Zech, J. M. Newsam and S. A. Schunk, Appl. Catal., A, 2003, 254, 121–131 CrossRef CAS.
- D. Wolf, O. V. Buyevskaya and M. Baerns, Appl. Catal., A, 2000, 200, 63–77 CrossRef CAS.
- L. Baumes, D. Farrusseng, M. Lengliz and C. Mirodatos, QSAR Comb. Sci., 2004, 23, 767–778 CrossRef CAS.
- A. Corma, J. M. Serra, P. Serna and M. Moliner, J. Catal., 2005, 232, 335–341 CrossRef CAS.
- A. Tompos, M. Sanchez-Sanchez, L. Végvári, G. P. Szijjártó, J. L. Margitfalvi, A. Trunschke, R. Schlögl, K. Wanninger and G. Mestl, Catal. Today, 2021, 363, 45–54 CrossRef CAS.
- T. Toyao, Z. Maeno, S. Takakusagi, T. Kamachi, I. Takigawa and K.-i. Shimizu, ACS Catal., 2020, 10, 2260–2297 CrossRef CAS.
- W. Yang, T. T. Fidelis and W.-H. Sun, ACS Omega, 2020, 5, 83–88 CrossRef CAS PubMed.
- S. Ma and Z.-P. Liu, ACS Catal., 2020, 10, 13213–13226 CrossRef CAS.
- B. R. Goldsmith, J. Esterhuizen, J.-X. Liu, C. J. Bartel and C. Sutton, AIChE J., 2018, 64, 2311–2323 CrossRef CAS.
- J. G. Freeze, H. R. Kelly and V. S. Batista, Chem. Rev., 2019, 119, 6595–6612 CrossRef CAS PubMed.
- C. Fricke, B. Rajbanshi, E. A. Walker, G. Terejanu and A. Heyden, ACS Catal., 2022, 2487–2498, DOI:10.1021/acscatal.1c04844.
- M. Steiner and M. Reiher, Top. Catal., 2022, 65, 6–39 CrossRef CAS PubMed.
- J. Graser, S. K. Kauwe and T. D. Sparks, Chem. Mater., 2018, 30, 3601–3612 CrossRef CAS.
- G. Hautier, C. C. Fischer, A. Jain, T. Mueller and G. Ceder, Chem. Mater., 2010, 22, 3762–3767 CrossRef CAS.
- A. O. Oliynyk and A. Mar, Acc. Chem. Res., 2018, 51, 59–68 CrossRef CAS PubMed.
- Z. W. Ulissi, M. T. Tang, J. Xiao, X. Liu, D. A. Torelli, M. Karamad, K. Cummins, C. Hahn, N. S. Lewis, T. F. Jaramillo, K. Chan and J. K. Nørskov, ACS Catal., 2017, 7, 6600–6608 CrossRef CAS.
- M. Andersen, S. V. Levchenko, M. Scheffler and K. Reuter, ACS Catal., 2019, 9, 2752–2759 CrossRef CAS.
- J. Behler and M. Parrinello, Phys. Rev. Lett., 2007, 98, 146401 CrossRef PubMed.
- M. Baysal, M. E. Günay and R. Yıldırım, Int. J. Hydrogen Energy, 2017, 42, 243–254 CrossRef CAS.
- A. J. Medford, M. R. Kunz, S. M. Ewing, T. Borders and R. Fushimi, ACS Catal., 2018, 8, 7403–7429 CrossRef CAS.
- S. Nishimura, J. Ohyama, T. Kinoshita, S. Dinh Le and K. Takahashi, ChemCatChem, 2020, 12, 5888–5892 CrossRef CAS.
- R. Palkovits and S. Palkovits, ACS Catal., 2019, 9, 8383–8387 CrossRef CAS.
- K. Takahashi, I. Miyazato, S. Nishimura and J. Ohyama, ChemCatChem, 2018, 10, 3223–3228 CrossRef CAS.
- K. Takahashi, L. Takahashi, T. N. Nguyen, A. Thakur and T. Taniike, J. Phys. Chem. Lett., 2020, 11, 6819–6826 CrossRef CAS PubMed.
- P. Raccuglia, K. C. Elbert, P. D. F. Adler, C. Falk, M. B. Wenny, A. Mollo, M. Zeller, S. A. Friedler, J. Schrier and A. J. Norquist, Nature, 2016, 533, 73–76 CrossRef CAS PubMed.
- M. R. Karim, M. Ferrandon, S. Medina, E. Sture, N. Kariuki, D. J. Myers, E. F. Holby, P. Zelenay and T. Ahmed, ACS Appl. Energy Mater., 2020, 3, 9083–9088 CrossRef.
- T. Williams, K. McCullough and J. A. Lauterbach, Chem. Mater., 2020, 32, 157–165 CrossRef CAS.
- R. Naumann d'Alnoncourt, Y. V. Kolen'ko, R. Schlögl and A. Trunschke, Comb. Chem. High Throughput Screening, 2012, 15, 161–169 CrossRef PubMed.
- T. N. Nguyen, T. T. P. Nhat, K. Takimoto, A. Thakur, S. Nishimura, J. Ohyama, I. Miyazato, L. Takahashi, J. Fujima, K. Takahashi and T. Taniike, ACS Catal., 2020, 10, 921–932 CrossRef CAS.
- D. E. Akporiaye, I. M. Dahl, A. Karlsson and R. Wendelbo, Angew. Chem., Int. Ed., 1998, 37, 609–611 CrossRef CAS PubMed.
- P. P. Pescarmona, J. J. T. Rops, J. C. van der Waal, J. C. Jansen and T. Maschmeyer, J. Mol. Catal. A: Chem., 2002, 182-183, 319–325 CrossRef CAS.
- K. Choi, D. Gardner, N. Hilbrandt and T. Bein, Angew. Chem., Int. Ed., 1999, 38, 2891–2894 CrossRef CAS PubMed.
- Y. Song, J. Yu, G. Li, Y. Li, Y. Wang and R. Xu, Chem. Commun., 2002, 1720–1721, 10.1039/B204496J.
- T. P. Caremans, C. E. A. Kirschhock, P. Verlooy, J. S. Paul, P. A. Jacobs and J. A. Martens, Microporous Mesoporous Mater., 2006, 90, 62–68 CrossRef CAS.
- M. Moliner, J. M. Serra, A. Corma, E. Argente, S. Valero and V. Botti, Microporous Mesoporous Mater., 2005, 78, 73–81 CrossRef CAS.
- K. P. F. Janssen, J. S. Paul, B. F. Sels and P. A. Jacobs, Appl. Surf. Sci., 2007, 254, 699–703 CrossRef CAS.
- M. Moliner, Y. Román-Leshkov and A. Corma, Acc. Chem. Res., 2019, 52, 2971–2980 CrossRef CAS PubMed.
- C. Bluthardt, C. Fink, K. Flick, A. Hagemeyer, M. Schlichter and A. Volpe, Catal. Today, 2008, 137, 132–143 CrossRef CAS.
- D. K. Kim and W. F. Maier, J. Catal., 2006, 238, 142–152 CrossRef CAS.
- J. S. Paul, J. Urschey, P. A. Jacobs, W. F. Maier and F. Verpoort, J. Catal., 2003, 220, 136–145 CrossRef CAS.
- P. Chammingkwan, M. Terano and T. Taniike, ACS Comb. Sci., 2017, 19, 331–342 CrossRef CAS PubMed.
- P. Munnik, P. E. de Jongh and K. P. de Jong, Chem. Rev., 2015, 115, 6687–6718 CrossRef CAS PubMed.
- H.-R. Cho and J. R. Regalbuto, Catal. Today, 2015, 246, 143–153 CrossRef CAS.
- C. Brooks, S. Cypes, R. K. Grasselli, A. Hagemeyer, Z. Hogan, A. Lesik, G. Streukens, A. F. Volpe, H. W. Turner, W. H. Weinberg and K. Yaccato, Top. Catal., 2006, 38, 195–209 CrossRef CAS.
- M. Kahn, A. Seubsai, I. Onal and S. Senkan, Comb. Chem. High Throughput Screening, 2010, 13, 67–74 CrossRef CAS PubMed.
- A. Corma, M. J. Díaz-Cabañas, J. L. Jordá, C. Martínez and M. Moliner, Nature, 2006, 443, 842–845 CrossRef CAS PubMed.
- J. Li, A. Corma and J. Yu, Chem. Soc. Rev., 2015, 44, 7112–7127 RSC.
- J. Jiang, J. Yu and A. Corma, Angew. Chem., Int. Ed., 2010, 49, 3120–3145 CrossRef CAS PubMed.
- J. Klein, C. W. Lehmann, H.-W. Schmidt and W. F. Maier, Angew. Chem., Int. Ed., 1998, 37, 3369–3372 CrossRef CAS PubMed.
- E. M. Chan, C. Xu, A. W. Mao, G. Han, J. S. Owen, B. E. Cohen and D. J. Milliron, Nano Lett., 2010, 10, 1874–1885 CrossRef CAS PubMed.
- L. A. Baumes, M. Moliner and A. Corma, Chem. – Eur. J., 2009, 15, 4258–4269 CrossRef CAS PubMed.
- S. Mitrovic, E. W. Cornell, M. R. Marcin, R. J. R. Jones, P. F. Newhouse, S. K. Suram, J. Jin and J. M. Gregoire, Rev. Sci. Instrum., 2015, 86, 013904 CrossRef PubMed.
- M. Schwarting, S. Siol, K. Talley, A. Zakutayev and C. Phillips, Mater. Discov., 2017, 10, 43–52 CrossRef.
- J. Li, Y. Tu, R. Liu, Y. Lu and X. Zhu, Adv. Sci., 2020, 7, 1901957 CrossRef CAS PubMed.
- S. Chen, Y. Hou, H. Chen, X. Tang, S. Langner, N. Li, T. Stubhan, I. Levchuk, E. Gu, A. Osvet and C. J. Brabec, Adv. Energy Mater., 2018, 8, 1701543 CrossRef.
- L. Velasco, J. S. Castillo, M. V. Kante, J. J. Olaya, P. Friederich and H. Hahn, Adv. Mater., 2021, 33, 2102301 CrossRef CAS PubMed.
- M. Behrens, D. Brennecke, F. Girgsdies, S. Kissner, A. Trunschke, N. Nasrudin, S. Zakaria, N. F. Idris, S. B. A. Hamid, B. Kniep, R. Fischer, W. Busser, M. Muhler and R. Schlögl, Appl. Catal., A, 2011, 392, 93–102 CrossRef CAS.
- M. Behrens and R. Schlögl, Z. Anorg. Allg. Chem., 2013, 639, 2683–2695 CrossRef CAS.
- M. Sanchez Sanchez, F. Girgsdies, M. Jastak, P. Kube, R. Schlögl and A. Trunschke, Angew. Chem., Int. Ed., 2012, 51, 7194–7197 CrossRef CAS PubMed.
- J. Noack, F. Rosowski, R. Schlögl and A. Trunschke, Z. Anorg. Allg. Chem., 2014, 640, 2730–2736 CrossRef CAS.
- K. Wenderich, J. Noack, A. Kärgel, A. Trunschke and G. Mul, Eur. J. Inorg. Chem., 2018, 2018, 917–923 CrossRef CAS.
- J. Schumann, T. Lunkenbein, A. Tarasov, N. Thomas, R. Schlögl and M. Behrens, ChemCatChem, 2014, 6, 2889–2897 CrossRef CAS.
- M. Behrens, F. Studt, I. Kasatkin, S. Kühl, M. Hävecker, F. Abild-Pedersen, S. Zander, F. Girgsdies, P. Kurr, B.-L. Kniep, M. Tovar, R. W. Fischer, J. K. Nørskov and R. Schlögl, Science, 2012, 336, 893–897 CrossRef CAS PubMed.
- D. Rein, K. Friedel Ortega, C. Weidenthaler, E. Bill and M. Behrens, Appl. Catal., A, 2017, 548, 52–61 CrossRef CAS.
- K. F. Ortega, D. Rein, C. Lüttmann, J. Heese, F. Özcan, M. Heidelmann, J. Folke, K. Kähler, R. Schlögl and M. Behrens, ChemCatChem, 2017, 9, 659–671 CrossRef CAS.
- M. E. Potter, M. E. Light, D. J. M. Irving, A. E. Oakley, S. Chapman, P. Chater, G. Cutts, A. Watts, M. Wharmby, B. D. Vandegehuchte, M. W. Schreiber and R. Raja, Phys. Chem. Chem. Phys., 2020, 22, 18860–18867 RSC.
- M. G. O'Brien, A. M. Beale and B. M. Weckhuysen, Chem. Soc. Rev., 2010, 39, 4767–4782 RSC.
- A. M. Beale and G. Sankar, Chem. Mater., 2002, 15, 146–153 CrossRef.
- A. M. Beale and G. Sankar, Chem. Mater., 2005, 18, 263–272 CrossRef.
- P. Norby, Curr. Opin. Colloid Interface Sci., 2006, 11, 118–125 CrossRef CAS.
- A. Michailovski, R. Kiebach, W. Bensch, J.-D. Grunwaldt, A. Baiker, S. Komarneni and G. R. Patzke, Chem. Mater., 2006, 19, 185–197 CrossRef.
- R. Kiebach, N. Pienack, W. Bensch, J.-D. Grunwaldt, A. Michailovski, A. Baiker, T. Fox, Y. Zhou and G. R. Patzke, Chem. Mater., 2008, 20, 3022–3033 CrossRef CAS.
- M. Goesten, E. Stavitski, E. A. Pidko, C. Gücüyener, B. Boshuizen, S. N. Ehrlich, E. J. M. Hensen, F. Kapteijn and J. Gascon, Chem. – Eur. J., 2013, 19, 7809–7816 CrossRef CAS PubMed.
- A. Aerts, C. E. A. Kirschhock and J. A. Martens, Chem. Soc. Rev., 2010, 39, 4626–4642 RSC.
- S. Khalid, W. Caliebe, P. Siddons, I. So, B. Clay, T. Lenhard, J. Hanson, Q. Wang, A. I. Frenkel, N. Marinkovic, N. Hould, M. Ginder-Vogel, G. L. Landrot, D. L. Sparks and A. Ganjoo, Rev. Sci. Instrum., 2010, 81, 015105 CrossRef CAS PubMed.
- J. Baumgartner, A. Dey, P. H. H. Bomans, C. Le Coadou, P. Fratzl, N. A. J. M. Sommerdijk and D. Faivre, Nat. Mater., 2013, 12, 310–314 CrossRef CAS PubMed.
- T. J. Woehl, Chem. Mater., 2020, 32, 7569–7581 CrossRef CAS.
- W. Hetaba, R. Imlau, L. Duarte-Correa, M. Lamoth, S. Kujawa and T. Lunkenbein, Chemistry–Methods, 2021, 1, 401–407 CrossRef.
- I. I. Ivanova, Y. G. Kolyagin, I. A. Kasyanov, A. V. Yakimov, T. O. Bok and D. N. Zarubin, Angew. Chem., Int. Ed., 2017, 56, 15344–15347 CrossRef CAS PubMed.
- M. Haouas, F. Taulelle and C. Martineau, Prog. Nucl. Magn. Reson. Spectrosc., 2016, 94–95, 11–36 CrossRef CAS PubMed.
- R. I. Maksimovskaya, V. M. Bondareva and G. I. Aleshina, Eur. J. Inorg. Chem., 2008, 2008, 4906–4914 CrossRef.
- J. Z. Hu, M. Y. Hu, Z. Zhao, S. Xu, A. Vjunov, H. Shi, D. M. Camaioni, C. H. F. Peden and J. A. Lercher, Chem. Commun., 2015, 51, 13458–13461 RSC.
- V. Sans, L. Porwol, V. Dragone and L. Cronin, Chem. Sci., 2015, 6, 1258–1264 RSC.
- C. F. Carter, H. Lange, S. V. Ley, I. R. Baxendale, B. Wittkamp, J. G. Goode and N. L. Gaunt, Org. Process Res. Dev., 2010, 14, 393–404 CrossRef CAS.
- V. Dragone, V. Sans, A. B. Henson, J. M. Granda and L. Cronin, Nat. Commun., 2017, 8, 15733 CrossRef PubMed.
- S. A. Pelster, R. Kalamajka, W. Schrader and F. Schüth, Angew. Chem., Int. Ed., 2007, 46, 2299–2302 CrossRef CAS PubMed.
- E. F. Wilson, H. N. Miras, M. H. Rosnes and L. Cronin, Angew. Chem., Int. Ed., 2011, 50, 3720–3724 CrossRef CAS PubMed.
- M. Marianski, J. Seo, E. Mucha, D. A. Thomas, S. Jung, R. Schlögl, G. Meijer, A. Trunschke and G. von Helden, J. Phys. Chem. C, 2019, 123, 7845–7853 CrossRef CAS.
- J. Häne, D. Brühwiler, A. Ecker and R. Hass, Microporous Mesoporous Mater., 2019, 288, 109580 CrossRef.
- F. Fan, Z. Feng, G. Li, K. Sun, P. Ying and C. Li, Chem. – Eur. J., 2008, 14, 5125–5129 CrossRef CAS PubMed.
- F. Zaera, Chem. Rev., 2012, 112, 2920–2986 CrossRef CAS PubMed.
- J. C. Groen, G. M. Hamminga, J. A. Moulijn and J. Pérez-Ramírez, Phys. Chem. Chem. Phys., 2007, 9, 4822–4830 RSC.
- A. Patis, V. Dracopoulos and V. Nikolakis, J. Phys. Chem. C, 2007, 111, 17478–17484 CrossRef CAS.
- J. S. Falcone Jr, J. L. Bass, P. H. Krumrine, K. Brensinger and E. R. Schenk, J. Phys. Chem. A, 2010, 114, 2438–2446 CrossRef PubMed.
- D. Tichit, G. Layrac and C. Gérardin, Chem. Eng. J., 2019, 369, 302–332 CrossRef CAS.
- J. A. Darr, J. Zhang, N. M. Makwana and X. Weng, Chem. Rev., 2017, 117, 11125–11238 CrossRef CAS PubMed.
- A. R. Groves, T. E. Ashton and J. A. Darr, ACS Comb. Sci., 2020, 22, 750–756 CrossRef CAS PubMed.
- M. Schur, B. Bems, A. Dassenoy, I. Kassatkine, J. Urban, H. Wilmes, O. Hinrichsen, M. Muhler and R. Schlögl, Angew. Chem., Int. Ed., 2003, 42, 3815–3817 CrossRef CAS PubMed.
- P. Zielke, Y. Xu, S. B. Simonsen, P. Norby and R. Kiebach, J. Supercrit. Fluids, 2016, 117, 1–12 CrossRef CAS.
- X. Weng, J. K. Cockcroft, G. Hyett, M. Vickers, P. Boldrin, C. C. Tang, S. P. Thompson, J. E. Parker, J. C. Knowles, I. Rehman, I. Parkin, J. R. G. Evans and J. A. Darr, J. Comb. Chem., 2009, 11, 829–834 CrossRef CAS PubMed.
- B. Li, S. S. Kaye, C. Riley, D. Greenberg, D. Galang and M. S. Bailey, ACS Comb. Sci., 2012, 14, 352–358 CrossRef CAS PubMed.
- S. Shuang, H. Li, G. He, Y. Li, J. Li and X. Meng, Rev. Sci. Instrum., 2019, 90, 083904 CrossRef PubMed.
- T. A. Stegk, R. Janssen and G. A. Schneider, J. Comb. Chem., 2008, 10, 274–279 CrossRef CAS PubMed.
- D. P. Debecker, S. Le Bras, C. Boissière, A. Chaumonnot and C. Sanchez, Chem. Soc. Rev., 2018, 47, 4112–4155 RSC.
- Y. Yang, B. Song, X. Ke, F. Xu, K. N. Bozhilov, L. Hu, R. Shahbazian-Yassar and M. R. Zachariah, Langmuir, 2020, 36, 1985–1992 CrossRef CAS PubMed.
- C.-G. Yang, Z.-R. Xu, A. P. Lee and J.-H. Wang, Lab Chip, 2013, 13, 2815–2820 RSC.
- M. Thiele, A. Knauer, D. Malsch, A. Csáki, T. Henkel, J. M. Köhler and W. Fritzsche, Lab Chip, 2017, 17, 1487–1495 RSC.
- H. V. Nguyen, K. Y. Kim, H. Nam, S. Y. Lee, T. Yu and T. S. Seo, Lab Chip, 2020, 20, 3293–3301 RSC.
- S. E. Lohse, J. R. Eller, S. T. Sivapalan, M. R. Plews and C. J. Murphy, ACS Nano, 2013, 7, 4135–4150 CrossRef CAS PubMed.
- P. Maguire, D. Rutherford, M. Macias-Montero, C. Mahony, C. Kelsey, M. Tweedie, F. Pérez-Martin, H. McQuaid, D. Diver and D. Mariotti, Nano Lett., 2017, 17, 1336–1343 CrossRef CAS PubMed.
- H. Kuhlenbeck, S. Shaikhutdinov and H.-J. Freund, Chem. Rev., 2013, 113, 3986–4034 CrossRef CAS PubMed.
- Z.-j. Wang, Z. Yan, C.-j. Liu and D. W. Goodman, ChemCatChem, 2011, 3, 551–559 CrossRef CAS.
- G. A. Somorjai and J. Y. Park, Surf. Sci., 2009, 603, 1293–1300 CrossRef CAS.
- U. Diebold, S.-C. Li and M. Schmid, Annu. Rev. Phys. Chem., 2010, 61, 129–148 CrossRef CAS PubMed.
- O. Shekhah, J. Liu, R. A. Fischer and C. Wöll, Chem. Soc. Rev., 2011, 40, 1081–1106 RSC.
- P. Cong, R. D. Doolen, Q. Fan, D. M. Giaquinta, S. Guan, E. W. McFarland, D. M. Poojary, K. Self, H. W. Turner and W. H. Weinberg, Angew. Chem., Int. Ed., 1999, 38, 483–488 CrossRef PubMed.
- Y. Liu, P. Cong, R. D. Doolen, H. W. Turner and W. H. Weinberg, Catal. Today, 2000, 61, 87–92 CrossRef CAS.
- G. Zhao, K. Rui, S. X. Dou and W. Sun, Adv. Funct. Mater., 2018, 28, 1803291 CrossRef.
- H. Dau, C. Limberg, T. Reier, M. Risch, S. Roggan and P. Strasser, ChemCatChem, 2010, 2, 724–761 CrossRef CAS.
- S. Mehla, J. Das, D. Jampaiah, S. Periasamy, A. Nafady and S. K. Bhargava, Catal. Sci. Technol., 2019, 9, 3582–3602 RSC.
- W. Wang, G. Tuci, C. Duong-Viet, Y. Liu, A. Rossin, L. Luconi, J.-M. Nhut, L. Nguyen-Dinh, C. Pham-Huu and G. Giambastiani, ACS Catal., 2019, 9, 7921–7935 CrossRef CAS.
- M. J. Hülsey, C. W. Lim and N. Yan, Chem. Sci., 2020, 11, 1456–1468 RSC.
- J. He, K. E. Dettelbach, D. A. Salvatore, T. Li and C. P. Berlinguette, Angew. Chem., Int. Ed., 2017, 56, 6068–6072 CrossRef CAS PubMed.
- B. P. MacLeod, F. G. L. Parlane, T. D. Morrissey, F. Häse, L. M. Roch, K. E. Dettelbach, R. Moreira, L. P. E. Yunker, M. B. Rooney, J. R. Deeth, V. Lai, G. J. Ng, H. Situ, R. H. Zhang, M. S. Elliott, T. H. Haley, D. J. Dvorak, A. Aspuru-Guzik, J. E. Hein and C. P. Berlinguette, Sci. Adv., 2020, 6, eaaz8867 CrossRef CAS PubMed.
- B. Fousseret, M. Mougenot, F. Rossignol, J.-F. Baumard, B. Soulestin, C. Boissière, F. Ribot, D. Jalabert, C. Carrion, C. Sanchez and M. Lejeune, Chem. Mater., 2010, 22, 3875–3883 CrossRef CAS.
- M. Mougenot, M. Lejeune, J. F. Baumard, C. Boissiere, F. Ribot, D. Grosso, C. Sanchez and R. Noguera, J. Am. Ceram. Soc., 2006, 89, 1876–1882 CrossRef CAS.
- S. Sun, W. Wang and L. Zhang, J. Phys. Chem. C, 2012, 116, 19413–19418 CrossRef CAS.
- X. Liu, Y. Shen, R. Yang, S. Zou, X. Ji, L. Shi, Y. Zhang, D. Liu, L. Xiao, X. Zheng, S. Li, J. Fan and G. D. Stucky, Nano Lett., 2012, 12, 5733–5739 CrossRef CAS PubMed.
- L. J. Deiner and T. L. Reitz, Adv. Eng. Mater., 2017, 19, 1600878 CrossRef.
- Z. Li, L. He, S. Wang, W. Yi, S. Zou, L. Xiao and J. Fan, ACS Comb. Sci., 2017, 19, 15–24 CrossRef CAS PubMed.
- Y. Yao, Z. Huang, T. Li, H. Wang, Y. Liu, H. S. Stein, Y. Mao, J. Gao, M. Jiao, Q. Dong, J. Dai, P. Xie, H. Xie, S. D. Lacey, I. Takeuchi, J. M. Gregoire, R. Jiang, C. Wang, A. D. Taylor, R. Shahbazian-Yassar and L. Hu, roc. Natl. Acad. Sci. U. S. A., 2020, 117, 6316–6322 CrossRef CAS PubMed.
- K. Bradley, K. Giagloglou, B. E. Hayden, H. Jungius and C. Vian, Chem. Sci., 2019, 10, 4609–4617 RSC.
- A. Anastasopoulos, J. Blake and B. E. Hayden, J. Phys. Chem. C, 2011, 115, 19226–19230 CrossRef CAS.
- C. R. Oliver, W. Westrick, J. Koehler, A. Brieland-Shoultz, I. Anagnostopoulos-Politis, T. Cruz-Gonzalez and A. J. Hart, Rev. Sci. Instrum., 2013, 84, 115105 CrossRef PubMed.
- D. A. Keller, A. Ginsburg, H.-N. Barad, K. Shimanovich, Y. Bouhadana, E. Rosh-Hodesh, I. Takeuchi, H. Aviv, Y. R. Tischler, A. Y. Anderson and A. Zaban, ACS Comb. Sci., 2015, 17, 209–216 CrossRef CAS PubMed.
- S. Pokhrel and L. Mädler, Energy Fuels, 2020, 34, 13209–13224 CrossRef CAS PubMed.
- R. Shimizu, S. Kobayashi, Y. Watanabe, Y. Ando and T. Hitosugi, APL Mater., 2020, 8, 111110 CrossRef CAS.
- Y. Bai, L. Wilbraham, B. J. Slater, M. A. Zwijnenburg, R. S. Sprick and A. I. Cooper, J. Am. Chem. Soc., 2019, 141, 9063–9071 CrossRef CAS PubMed.
- C. Empel and R. M. Koenigs, Angew. Chem., Int. Ed., 2019, 58, 17114–17116 CrossRef CAS PubMed.
- J. M. Granda, L. Donina, V. Dragone, D.-L. Long and L. Cronin, Nature, 2018, 559, 377–381 CrossRef CAS PubMed.
- B. Burger, P. M. Maffettone, V. V. Gusev, C. M. Aitchison, Y. Bai, X. Wang, X. Li, B. M. Alston, B. Li, R. Clowes, N. Rankin, B. Harris, R. S. Sprick and A. I. Cooper, Nature, 2020, 583, 237–241 CrossRef CAS PubMed.
- P. Nikolaev, D. Hooper, F. Webber, R. Rao, K. Decker, M. Krein, J. Poleski, R. Barto and B. Maruyama, npj Comput. Mater., 2016, 2, 16031 CrossRef.
- B. Shahriari, K. Swersky, Z. Wang, R. P. Adams and N. d. Freitas, Proc. IEEE, 2016, 104, 148–175 Search PubMed.
- L. M. Roch, F. Häse, C. Kreisbeck, T. Tamayo-Mendoza, L. P. E. Yunker, J. E. Hein and A. Aspuru-Guzik, Sci. Robot., 2018, 3, eaat5559 CrossRef PubMed.
- F. Häse, L. M. Roch, C. Kreisbeck and A. Aspuru-Guzik, ACS Cent. Sci., 2018, 4, 1134–1145 CrossRef PubMed.
- P. Nikolaev, D. Hooper, N. Perea-López, M. Terrones and B. Maruyama, ACS Nano, 2014, 8, 10214–10222 CrossRef CAS PubMed.
- J. K. Nørskov and T. Bligaard, Angew. Chem., Int. Ed., 2013, 52, 776–777 CrossRef PubMed.
- S. Moniri, T. Van Cleve and S. Linic, J. Catal., 2017, 345, 1–10 CrossRef CAS.
- K. Tran and Z. W. Ulissi, Nat. Catal., 2018, 1, 696–703 CrossRef CAS.
-
J. T. Margraf, Z. Ulissi, Y. Jung and K. Reuter, ChemRxiv, Cambridge: Cambridge Open Engage, 2021, DOI:10.26434/chemrxiv-2021-bd6g6.
-
M. Wesemann, AC archive, https://github.com/fhimpg/archive).
- M. D. Wilkinson, M. Dumontier, I. J. Aalbersberg, G. Appleton, M. Axton, A. Baak, N. Blomberg, J.-W. Boiten, L. B. da Silva Santos, P. E. Bourne, J. Bouwman, A. J. Brookes, T. Clark, M. Crosas, I. Dillo, O. Dumon, S. Edmunds, C. T. Evelo, R. Finkers, A. Gonzalez-Beltran, A. J. G. Gray, P. Groth, C. Goble, J. S. Grethe, J. Heringa, P. A. C. 't Hoen, R. Hooft, T. Kuhn, R. Kok, J. Kok, S. J. Lusher, M. E. Martone, A. Mons, A. L. Packer, B. Persson, P. Rocca-Serra, M. Roos, R. van Schaik, S.-A. Sansone, E. Schultes, T. Sengstag, T. Slater, G. Strawn, M. A. Swertz, M. Thompson, J. van der Lei, E. van Mulligen, J. Velterop, A. Waagmeester, P. Wittenburg, K. Wolstencroft, J. Zhao and B. Mons, Sci. Data, 2016, 3, 160018 CrossRef PubMed.
|
This journal is © The Royal Society of Chemistry 2022 |
Click here to see how this site uses Cookies. View our privacy policy here.