DOI:
10.1039/D2CS00339B
(Review Article)
Chem. Soc. Rev., 2022,
51, 6210-6221
The importance of cellular degradation kinetics for understanding mechanisms in targeted protein degradation
Received
29th April 2022
First published on 6th July 2022
Abstract
Targeted protein degradation has exploded over the past several years due to preclinical and early clinical therapeutic success of numerous compounds, and the emergence of new degradation modalities, which has broadened the definition of what a degrader is. The most characterized and well-studied small molecule degraders are molecular glues and proteolysis targeting chimeras (PROTACs). These degraders induce a ternary complex between a target protein, degrader, and E3 ligase component, resulting in ubiquitination and subsequent degradation of the target protein via the ubiquitin proteasomal system (UPS). This event-driven process requires success at all steps through a complex cascade of events. As more systems, degraders, and targets are tested, it has become increasingly clear that achieving degradation is only the first critical milestone in a degrader development program. Rather highly efficacious degraders require a combination of multiple optimized parameters: rapid degradation, high potency, high maximal degradation (Dmax), and sustained loss of target without re-dosing. Success to meet these more rigorous goals depends upon the ability to characterize and understand the dynamic cellular degradation profiles and relate them to the underlying mechanism for any given target treated with a specific concentration of degrader. From this starting point, optimization and fine tuning of multiple kinetic parameters such as how fast degradation occurs (the rate), how much of the target is degraded (the extent), and how long the target remains degraded (the duration) can be performed. In this review we explore the diversity of cellular kinetic degradation profiles which can arise after molecular glue and PROTAC treatment and the potential implications of these varying responses. As the overall degradation kinetics are a sum of individual mechanistic steps, each with their own kinetic contributions, we discuss the ways in which changes at any one of these steps could potentially influence the resultant kinetic degradation profiles. Looking forward, we address the importance in characterizing the kinetics of target protein loss in the early stages of degrader design and how this will enable more rapid discovery of therapeutic agents to elicit desired phenotypic outcomes.
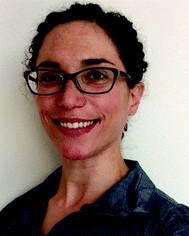
Kristin M. Riching
| Kristin M. Riching, PhD, is a senior scientist and group leader at Promega. She manages a team dedicated to developing innovative technologies in the area of targeted protein degradation and induced proximity. Her early work in building a platform of live cellular assays for TPD enabled pioneering discoveries in degrader kinetics and mechanism of action. She is currently focused on expanding these approaches to gain further insights into degrader activity, advancing capabilities for degrader screening and optimization, and investigating new modalities for TPD and induced proximity. Kristin received her PhD in Biomedical Engineering from the University of Wisconsin – Madison studying invasion and metastasis in breast cancer, and she joined Promega in 2014. |
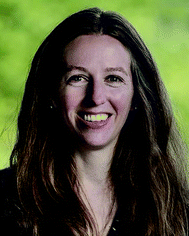
Elizabeth A. Caine
| Elizabeth A. Caine is a Senior Scientist at Promega Corporation developing approaches to monitor the functional mechanisms of targeted protein degradation in live cells. She received her PhD in Comparative Biomedical Sciences from the University of Wisconsin-Madison where she characterized vaccines and studied the pathogenesis of human enteroviruses that cause hand, foot, and mouth disease. Elizabeth completed her postdoctoral training at Washington University in St. Louis researching the immune response in the female reproductive tract after Zika virus infection. |
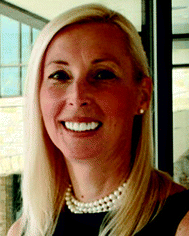
Marjeta Urh
| Marjeta Urh, PhD, is a Senior Director in R&D at Promega Corporation. She manages technical and strategic development of innovative products and technologies for areas of protein and nucleic acid analysis. She works with cross-functional teams on identifying company-wide initiatives to drive Promega business and long-term success. She provides overall scientific leadership to enable increase of footprint in emerging marketers and enter novel areas of science, most recently championing development of technologies to monitor cellular kinetics and mechanism of targeted protein degradation. Prior to joining Promega, Marjeta worked in the Department of Anti-Infectives, Research and Development at GlaxoSmithKline. She received her PhD in Genetics at University of Wisconsin-Madison and completed her postdoctoral studies at Genetique Microbienne, INRA, Paris, France. |
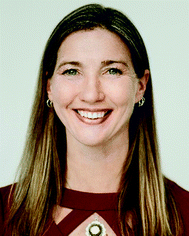
Danette L. Daniels
| Danette L. Daniels, PhD, is a Vice President of the Protein Degrader Platform at Foghorn Therapeutics developing therapeutic degraders in the areas of chromatin regulation and oncology. She was an early leader in the field of targeted protein degradation, pioneering approaches to monitor cellular kinetics of degradation, understand mechanism of action, and most recently, co-developing a new PROTAC modality during her previous position as a R&D Group Leader at Promega Corporation. She received her PhD in Biophyiscs at Yale University and completed a postdoctoral fellowship at Stanford University studying Wnt signaling. |
Introduction
The emergence and success of targeted protein degradation as a therapeutic modality has opened new possibilities in terms of targets, including those previously thought to be intractable or undruggable, and expanded available treatments.1–10 Degrader compounds co-opt endogenous cellular machinery and degradation pathways to remove disease-causing target proteins from the cell.3,5,11 The remarkable success of immunomodulatory drug (IMiD) degraders as powerful anti-cancer agents has paved the way for entry into the clinic of the largest class of degraders, heterobifunctional proteolysis targeted chimeras (PROTACs), which are in development for numerous indications within oncology, immunology, and neurodegenerative disease.2,4,6,7,12–14 IMiDs, also known as CRBN-based molecular glues, and PROTACs work very similarly by functioning as a chemical bridge to induce proximity between a target protein and E3 ligase or E3 component, forming a ternary complex3,5,9–11,15–20 (Fig. 1). This non-native complex positions the target protein into an active E3 ligase complex where it is then ubiquitinated and subsequently degraded through the ubiquitin proteasomal system (UPS)3,5,10,11 (Fig. 1). Highly optimized degraders that very efficiently co-opt the UPS induce rapid and sustained target loss, which disrupts a target's native protein homeostasis and outcompetes protein synthesis and any compensatory regulatory mechanisms by continually driving target loss for extended periods of time (Fig. 1).
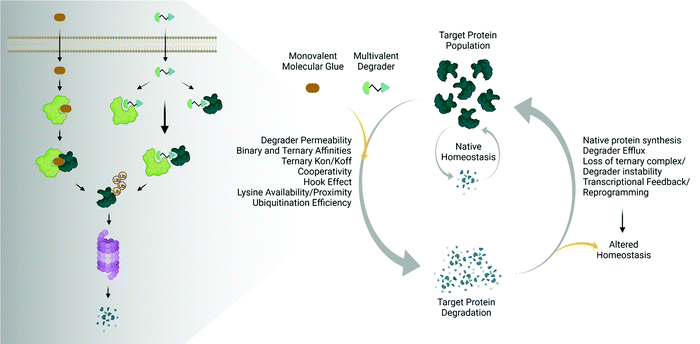 |
| Fig. 1 Degrader mechanisms and impact on native protein homeostasis. Traditional UPS-driven targeted protein degraders consist of monovalent molecular glues or multivalent (bivalent or trivalent) compounds. The efficiency of degradation beyond endogenous degradation rates is influenced by many factors including cellular permeability, the induced binary and ternary interactions, as well as ubiquitination. The sustained impact on target protein levels is further influenced not only by the degrader itself, but also the presence of any feedback on native regulatory mechanisms, leading to potentially altered homeostatic responses. Image created with BioRender.com. | |
While the first examples of targeted protein degradation were driven through the UPS, many other approaches have now been described utilizing a similar induced proximity concept but differ in that they drive degradation via lysosomal, autophagy, and/or chaperone recruitment.1,2 As degradation via the UPS is limited to intracellular targets with particular localization,21 these alternative degradation pathways significantly expand the number of targets, including aggregated, extracellular, or multi-pass membrane proteins and even beyond proteins to nucleic acids, which can now be considered for therapeutic degradation.1,2,22,23 In the expansion of degradation pathways, degraders themselves have moved beyond small molecules, and depending on the pathway of degradation employed, have shown success as antibodies, antibody-conjugates, or nucleic-acid-drug conjugates.1,2 Given the exploding pace of research in the areas of induced proximity and targeted protein degradation, it is highly likely that both the choice of pathways to degradation as well as composition of degraders will continue to evolve, in some cases together, resulting in increased options of targets that can be considered.
The various types of degraders all function through a complex, multistep event-driven pathway,1,2 differentiating significantly from classical occupancy-driven mechanisms employed by activating or blocking agents, such as inhibitors.24 These numerous steps required for degradation have their individual dynamics and collectively impinge upon targeted protein degradation outcomes (Fig. 1), contrasting again to small molecule inhibitors or activators whose potency relies primarily on robust cellular permeability and high affinity binding to their target. Though event-driven pharmacology can be difficult to optimize or deconvolute, it offers advantages as it provides multiple points within the pathway that could be enhanced either alone or in combination to improve compound efficacy.
The steps in event-driven degradation are not only dynamic, but they also each are competitive with other cellular processes that would hinder successful degradation induced by PROTACs or molecular glues (Fig. 1). Therefore, tipping the balance at any one event can positively or negatively impact the overall potency of compounds and the degradation outcome (Fig. 1). As a result of this interplay of events, we can observe that degradation of the target can assume different trajectories as function of time. For any given target, this can be captured as a kinetic degradation profile (Fig. 2a), where target protein level is graphed as a function of time at a given degrader concentration.25 At a high level, the kinetic degradation profile is the target's cellular response signature to a degrader and follows the target protein levels in real-time from its native homeostatic expression to its maximal removal (Fig. 2a), though in reality most degraders do not result in complete target protein degradation unless they have been extensively optimized. The time that which the target protein will remain degraded will vary extensively, but in general it will eventually be followed by post-degradation recovery, which can restore native protein expression or result in altered homeostasis via feedback or transcriptional reprogramming (Fig. 2a).
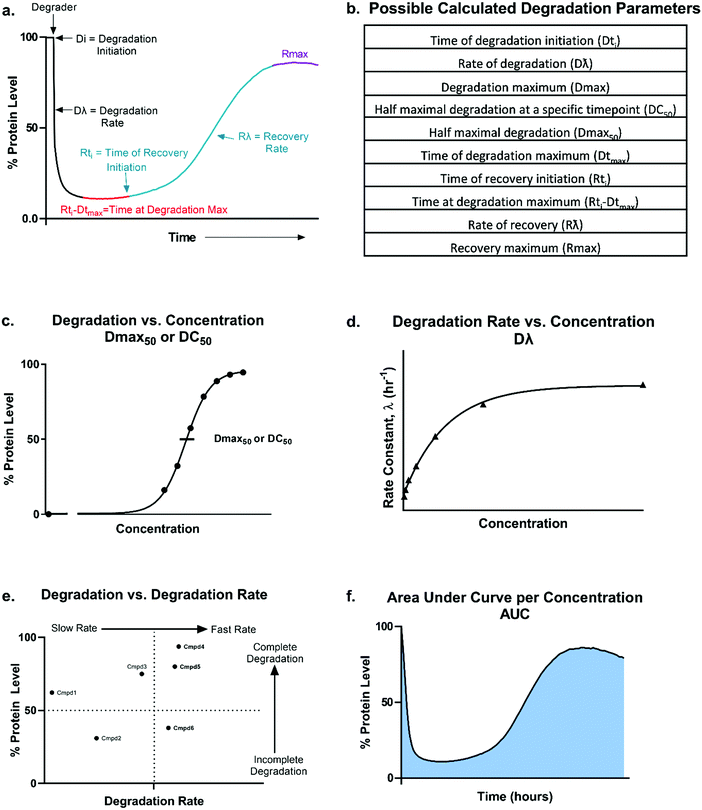 |
| Fig. 2 Overview of degradation profile and quantitative parameters. Model (a) and table (b) outlining the possible parameters which could be generated using the degradation profile. Dmax50 or DC50 values (c), degradation rate (d), correlation between percent degradation to degradation rate (e), and area under the curve per concentration (f) can all be calculated or determined from the kinetic degradation profile. | |
From these kinetic degradation profiles, a core set of kinetic parameters can be defined and quantitated as described in Fig. 2a and b. Historically DC50 values of degradation compounds have been calculated by determining the apparent degradation maximum (Dmax) across a concentration series at a single time point and plotted this against concentration to yield a DC50 at that time (Fig. 2c). This however is only a single snapshot of the entire degradation process. As shown in Fig. 2a, Dmax changes over time and the degradation profile will be different for any given concentration. Each concentration therefore will reach their Dmax at different times. To capture the kinetic nature of the process, the absolute Dmax achieved at an individual concentration, irrespective of the time at of which it occurs, can be plotted against concentration and a Dmax50 can be determined (Fig. 2c).25 There are many other factors in addition to the kinetic Dmax which will vary for different targets and degraders, and these can be as critical or possibly more impactful than Dmax alone for achieving the desired phenotypic outcome (Fig. 2a and b). For example, even if compounds have the same Dmax, many core kinetic parameters could differ such as the time required to initiate degradation, the rate of degradation, the time at which Dmax was first achieved, how long the target remained at Dmax, and the time at which recovery of the target begins to occur. These differences can further be analysed by plotting non-traditional degradation parameters against each other25–27 (Fig. 2d–f) For example, degradation rate versus concentration can yield insight as to where the degradation rate will plateau and most clearly show when the hook effect will begin to occur (Fig. 2d). Plotting Dmaxversus degradation rate will allow for separation of compounds which quickly and completely degrade from those which are slower and/or incomplete26 (Fig. 2e). Lastly, at any given concentration, the area under the degradation curve (AUC) can be calculated and used to differentiate compounds which may have similar rates and Dmax, yet maintain Dmax for very different lengths of time (Fig. 2f).
Analysis in this extended fashion can shed light upon potential clustering of compounds within a series that have similar kinetic strengths or weaknesses and will also reveal the highly differentiating compounds which have multiple favourable kinetic parameters (Fig. 2c–f).
These analyses are essential for fully understanding a degrader's kinetic potency and efficacy within the cell to facilitate more informed compound ranking. Additionally, they yield critical insight into degrader mechanism of action as particular steps in the degradation pathway can be correlated with changes in specific parameters. Inspired by numerous previous studies showing diverse degradation responses with pan-PROTACs as well as larger degrader chemical series studies,25,27–45 here we present an in-depth exploration of kinetic target degradation profiles. We present how drastically different they can be when responding to a given degrader, though despite these differences, some general profile patterns that are emerging, the nuances between them, and the information that can be gleaned from each. As degradation is a sum of multiple kinetic steps, we additionally discuss how individual mechanistic steps can impact the overall kinetic profiles and how this understanding can aid optimization and design of degraders to more rapidly advance programs.
Kinetic degradation profiles and their diversity
Given the number of kinetic parameters (Fig. 2) and dynamic processes (Fig. 1) required for successful targeted protein degradation, it is not surprising kinetic profiles can differ drastically even for the same target treated with two very chemically similar degraders. Interestingly though, some general trends in the profiles are emerging, some of which have been correlated to distinct mechanistic aspects such as cellular permeability, ternary complex formation, productive ubiquitination, and recovery mechanisms (Fig. 1). The general profiles can be grouped in 5 broad sections: “The Classic +/− Hook Effect”, “The Partial”, “The Linear”, “The Delayed” and “The Rapid Recovery” as shown in Fig. 3. In these next sections we step through each profile and discuss the understandings and mechanistic clues each of these profiles hold.
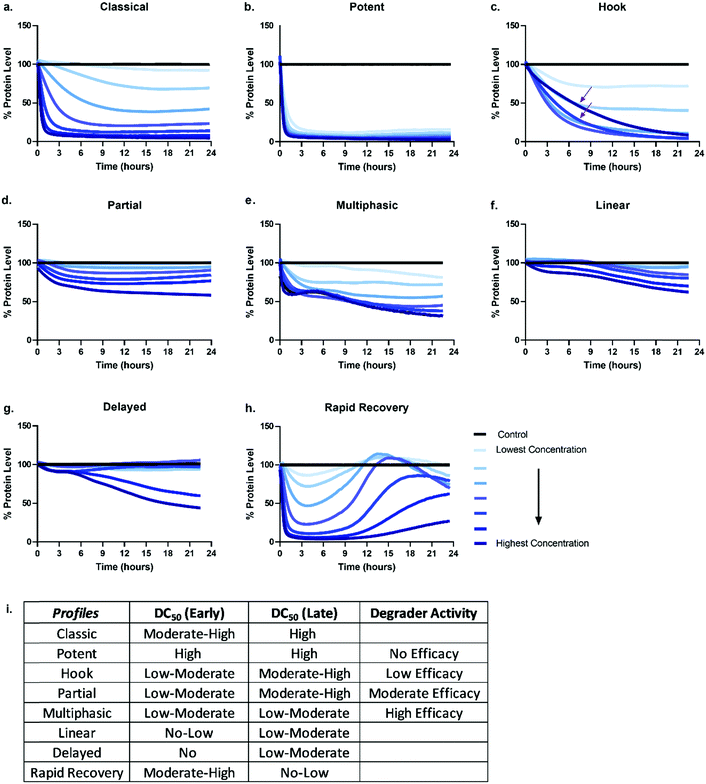 |
| Fig. 3 General degradation profile trends. Possible kinetic degradation profiles are depicted by plotting percent protein level over time after the addition of a degrader compound. Each line represents degradation at a specific degrader concentration over time. The classical (a) and potent (b) profiles shows rapid dose dependent and sustained degradation of the target protein. Profiles for hook (c), partial (d), multiphasic (e), linear (f), delayed (g), and rapid recovery (h) are also represented. The red arrows in the hook (c) profile highlight the slowing of the degradation rate when higher concentrations of degrader are used. (i) Predicted differences in measured degradation efficacy if degradation and DC50 values are measured and determined at a single early timepoint (4–6 hours), and/or single late timepoint (18–24 hours) for each of the profiles (a–h). | |
The classic +/− hook effect
The Classic degradation profile is the goal of any molecular glue or PROTAC program. The hallmarks of a Classic profile are the immediate initiation of degradation, a rapid and steep drop, i.e. rapid degradation rate, to complete Dmax within a matter of a few hours post-compound treatment (Fig. 3a). Initiation of degradation, degradation rate, and Dmax show significant dependency upon compound concentration, wherein lower concentrations demonstrate slower degradation rates and typically take longer to reach their partial Dmax (Fig. 3a). As the concentration of degrader increases, onset and rate of degradation improves and reaches a maximum value, λmax, while Dmax will move to completion and be sustained. Potent compounds show high maximal rates combined with high maximal degradation at very low concentrations and often across a wider concentration range (Fig. 3b). Both classic and potent profiles have been observed with the varying generations of BET bromodomain and BRD7/9 PROTACs,25,27,42,46 IMiD molecular glues,46 kinases35 and fusion-tag PROTACs.47
Molecular glues and PROTACs diverge in the Classic category with respect to the hook effect. As PROTACs have more than one binding ligand, at high concentrations, saturation binding within their respective binary complexes can become competitive with ternary complex formation (Fig. 1) slowing down or halting the PROTAC-mediated target degradation (Fig. 3c). This results in the hook effect, which is manifested first by a slowing of the rate of degradation, leading to a delay in reaching Dmax (Fig. 3c), which has previously been observed kinetically for several targets.25,35,46 As the concentration of PROTAC increases beyond this saturation, the rate is slowed so significantly, the degradation curves begin to mirror those of the lowest concentrations of degrader in terms of rate and potentially also Dmax. This window of PROTAC degrader efficacy, which has an upper boundary set by the hook effect is correlated with cellular cooperativity and/or avidity of the PROTAC ternary complex.16,25,27,30,41,42,48 If the PROTAC ternary complex is favourable, the window of PROTAC maximal degrader efficacy will occur across a broader concentration range, looking like the highly potent profiles shown in Fig. 3 and has notably been measured for a PROTAC which has both combined avidity and cooperativity in the ternary complex.27 However, if the PROTAC ternary complex is not favourable, this window will be narrow, and the maximal degradation rate will not hold a plateau. While orthogonal biochemical, biophysical, and cellular measurements are always recommended to confirm ternary complex cooperativity, the Classic kinetic degradation profile can help guide compound design and optimization for improving ternary complex formation.
The partial
Partial degradation is defined by degradation reaching and sustaining a Dmax that is not complete, nor can reach completion despite increasing compound concentrations or extended periods of time and is likely the most common degradation profile observed at early stages on any degradation project wherein compounds are not yet well optimized for any of the processes. Partial degradation, like Classic profiles, can have fast or slow rates of degradation, as well as show the impact of the hook effect at high concentrations with examples shown for several targets.27,35 Partial degradation could be due to a variety of factors; selective degradation of a sub-population of the target, or the competing effects of new protein synthesis or target deubiquitination which result in a new equilibrium steady state of target protein levels (Fig. 3d). As targets involved in various protein complexes can have different structural conformation and potentially be localized to different compartments within the cell, it is highly plausible that the degrader compound is not equally efficient at degrading the target in all these various contexts. Indeed, it is possible that the entire cellular population of target, if available for PROTAC binding, may not in some instances be accessible for ternary complex formation or productive ubiquitination leading to sub-population degradation.49 If partial degradation is due to sub-population degradation of the target, degradation profiles can look like those of The Potent (Fig. 3b) with fast rates of degradation and many concentrations overlapping. It will differ however as it will not plateau at 90–100% Dmax values, rather much lower values. It is possible that sub-population degradation can be overcome by changing either binding ligand to the target or choice of E3 ligase recruiter.
Another scenario which could lead to partial degradation is preferential degradation of specific target isoform populations, again which may be involved in different interactions or even lack the binding domain of the degrader.49 In these cases, one isoform may show a Classic and complete degradation profiles while the other isoform does not degrade, but this composite results in an overall Partial profile. Ability to separately detect and measure the isoforms will enable understanding in these scenarios. Additionally, partial degradation could be due to amino acid sequence heterogeneity of targets which frequently mutate, particularly in disease and oncology.49 Determining how these mutations as compared to the wild-type protein impact degradation is an important consideration and understanding of the kinetics could better allow for development of specificity.
Degradation can also be observed as multi-phasic with several degradation rates and appear to step down in phases (Fig. 3e). The multi-phasic nature may or may not be observed across all concentrations within a dose response curves. These profiles are very complicated and challenging to quantitate with respect to the parameters in Fig. 2. Multi-phasic profiles indicate that target protein loss is driven by more than one process or pathway and has been observed with pan-active degraders, most notably with a broad spectrum kinase inhibitor converted to a degrader.35 If observed for PROTACs, it is important to understand how the target binder alone can impact target protein levels, either positively or negatively. If the inhibitor alone does modulate the target protein level, this effect will be additive or divergent with the PROTAC-mediated degradation, yielding multiphasic behaviour until one pathway or the other dominates the degradation rate or Dmax. As discussed above with other partial degradation profiles, an alternative situation which could lead to multiphasic behaviour would be differential degradation of targets within different complexes or locations degraded at different rates. For example, a target free in the cytoplasm could have a fast initial rate of loss, but the population of that same target tethered to the plasma membrane or encompassed in multi protein complex, could follow more slowly. This would yield multiphasic degradation, with separate rates and trajectories towards Dmax.
The linear
Linear degradation profiles show a slow degradation rate and linear loss of protein levels (Fig. 3f). This is also concentration dependent, but often the differences between concentrations are shallower than observed in the Classic or Partial degradation profiles (Fig. 3f). A linear profile can be reflective of either very poor targeted protein degradation mechanisms, or general protein loss not due to degrader mechanism, but rather due to loss of cellular viability or other aspects of the molecule which may globally impact transcription or translation. If the former, slow linear loss indicates very poor efficiency of degradation, which unfortunately could be due to problems at any or all the upstream mechanistic steps of compound permeability, ternary complex formation, or productive ubiquitination. When these profiles are observed, testing for cellular permeability of compounds is the most rapid way to diagnose the problem. If they are poorly permeable, traditional medicinal chemistry approaches can be applied to improve the series. If the degraders are readily permeable, then changes to linkers and/or E3 ligase handles would likely be needed to improve upon ternary complex and ubiquitination efficiency steps. If the compound is showing target loss due to loss in cell viability or toxicity, the same linear kinetic profile would be found with a non-specific protein target, and loss would also be seen in a multiplex cell viability assay.
The delayed
The Delayed degradation profile can show the shape of any of the previous profiles, Classic, Partial, or Linear, but is defined by a significant time lag post-compound treatment prior to any initiation of degradation (Fig. 3g). As with some of the profiles, this signature profile could be a result of various scenarios and can arise with both molecular glues and PROTACs. The first scenario is that the target is not the primary protein target(s) of the degrader, rather it is a secondary target that only begins degradation after the primary target(s) is degraded. Again this has been observed when a pan-active inhibitor is used for conversion to degrader,35 but could arise even for a selective target binder PROTAC if it preferentially binds an off-target protein. As degraders can be catalytic, this initial loss of primary target tips the balance towards secondary targets which might be less efficient for degradation, yet no longer are in competition with the primary targets for degrader binding and E3 recruitment. In this scenario, global proteomics can be done at the early time points within the lag to determine which targets are the primary targets. The second scenario could be that target protein loss is delayed because it is not driven by the degrader itself, but by other transcriptional or translation regulatory pathways which impact the protein levels. This can be determined using specific controls such as proteasomal inhibitors or competitive inhibitors that block ternary complex formation to determine whether protein loss still results. The third scenario could be that the compound has very poor cellular permeability and needs to reach sufficient concentration prior to initiating detectable degradation. Orthogonal studies can be done to determine if this is the case and as mentioned above, compounds could then be improved accordingly.
The rapid recovery
Unless cell death is triggered by sustained target protein loss, all profiles above will have a recovery phase and the target will return to some level of protein homeostasis (Fig. 3h). Monitoring the rate and the extent of target recovery, and the time and concentrations at which this will occur is incredibly important for understanding the complete efficacy window of any targeted protein degradation compound. As levels of any given protein are often exquisitely regulated, it is also important to be aware of any feedback loops or transcriptional pathways which may be in place to sense loss of the target protein and may be compensatively and rapidly activated after treatment with degrader (Fig. 3h). An interesting example of rapid recovery was observed for a BET family member, BRD2, after treatment with a pan-BET degrader.25 Interesting this protein was shown to be upregulated with treatment of the BET inhibitor alone indicating its levels were sensitive to both inhibition and degradation.25 Additionally, different cell types, which will vary in endogenous expression of target as well as all the E3 ligase machinery50 needed for degradation likely have different kinetic recovery profiles. Other factors which can greatly influence recovery are compound stability and/or rate of compound efflux.
As full kinetic profiles cannot always be determined or are technically too challenging in certain scenarios, the diversity of these profiles begs the question at which times would be best to measure degradation to roughly understand what category a degrader would fall. As shown in Fig. 3i are the predicted differences in measured degradation efficacy upon measuring a DC50 at an early time point (between 4–6 hours) and late time point (between 18–24 hours) for each of the types of profiles. Based upon this it would be recommended to minimally monitor degradation at both an early timepoint as well as late time point to gain some insight into the degrader profile. The early timepoint also helps to deconvolute whether loss observed at the later timepoint could potentially be a cause of cell toxicity or off-target mechanisms and not target specific degradation.
Mechanistic steps which influence overall degradation profiles
Ternary complex formation
Medicinal chemistry optimization of small molecules has historically relied on understanding of how chemical properties directly relate to cellular entry and the pharmacology of binding to target proteins. Compounds that elicit their intended function downstream of binding to the target present greater challenges in establishing robust SAR, as the activity of binding is often uncoupled from their functional outcome24,51 (Fig. 1). Even with degraders that can act catalytically, potent binary engagement to the target and E3 ligase as well as ternary complex formation do not always confer efficient and potent degradation.25–45 Still, given the relative ease in measuring binary and ternary complex binding affinities with availability of biochemical, cell-based, and biophysical tools, significant emphasis is placed on identifying properties of ternary complex formation such as cooperativity, stability, kD, and most recently residence time, all parameters which can drive degradation efficacy.16,19,20,27,30,43,45,48,52–57 Unlike occupancy-driven small molecule inhibitors, bifunctional degraders are further challenged by competition between ternary complexes and individual binary complexes, which can become saturated at high degrader concentrations, resulting in a hook effect (Fig. 1 and 3c). In contrast, molecular glues do not show a hook effect as they are traditionally monovalent, only binding to one substrate initially before facilitating neosubstrate protein interactions. Most PROTACs exhibit a bell-shaped ternary binding profile with concentration, which can appear to result in complete inhibition of ternary complexes as the concentration of binary complexes dominates at high PROTAC concentrations.16,41,53 Depending upon the time of degradation measured at those high concentrations, a corresponding dramatic reduction in Dmax can be observed. If the PROTACs are poorly soluble this shifts the concentration range where the bell shape will occur towards the higher end concentration range and therefore the hook effect might not be measurable as the concentration required to fully characterize it would be too high.
Attempts to overcome the hook effect lie in the design of degraders that can form highly cooperative and/or avid complexes in order to broaden the ternary bell curve by delaying the point at which binary complexes compete.16,25,27,48,53–57 While cooperative ternary complexes are a highly sought feature of degraders given the broader range of maximal efficacy they will have, there is not a direct correlation between ternary complex cooperativity and the key degradation parameters; degradation potency, Dmax, or degradation rate. Rather, the correlations are more with ubiquitination efficiency. Therefore, an unfavourable ternary complex that results in optimal positioning of target lysines only needs to be stable long enough to allow for ubiquitination to achieve robust degradation. Thus, optimizing degrader design to achieve the desired Classic, potent degradation profiles as shown in Fig. 3a and b is not only dependent upon the kinetics of ternary complex formation and stability, but also target ubiquitination (Fig. 1 and 4), as ubiquitination is both highly correlated with degradation rate and is a strong predictor of degradation efficacy.25–27,42 As the penultimate event that precedes proteasomal docking and degradation, it is perhaps not surprising that such strong correlations are observed.
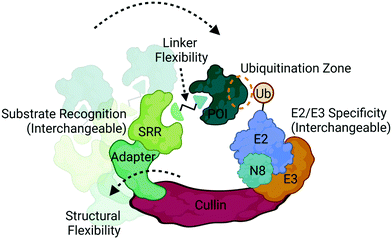 |
| Fig. 4 Structural and compositional dynamics of CRLs that influence ubiquitination. CRLs are modular multi-subunit complexes that contain several dynamically interchangeable components, such as the substrate recognition receptors (SRRs), E2/E3 ligase components, and neddylation factor (N8). CRLs also display structural conformational flexibility that influences positioning of the target protein of interest (POI) with respect to the ubiquitin-loaded E2. Proximity can also be optimized via linker design in multivalent degraders in achieve successful ubiquitination. Image created with BioRender.com. | |
Ubiquitination
Ubiquitination is a covalent post-translational modification to target lysine residues that is carried out by the ATP-dependent sequential activation of E1 (ubiquitin-activating), E2 (ubiquitin-conjugating) and E3 (ubiquitin ligase) enzymes.58 Over 600 E3 ligases are expressed in the human genome which confer substrate specificity, cellular specificity, and regulation of diverse protein functions and homeostasis.9,58 Within this large family of enzymes exists multiple subclasses of E3 ligases that are categorized based on the presence of certain domains and their mechanism of ubiquitin transfer.9,58 Those which have been most successfully and extensively used in targeted protein degradation are the Cullin RING ligases (CRL) complexes CRL2VHL and CRL4CRBN (Fig. 4), which target proteins for degradation via the UPS.2,3,8,12,20
CRLs are highly dynamic complexes in terms of their composition and conformation59 (Fig. 4), both of which could influence degradation kinetics. VHL and CRBN are substrate recognition receptors (SRRs) that dynamically associate with either CRL2 or CRL4 complexes, respectively. Other SRRs have also been successfully co-opted with a degrader can associate into these same complexes,60 potentially in a competitive fashion. The compositional dynamics of SRRs as well as the potential for non-CRL associated proteins to be recruited as substrate anchors underscores the inherent modularity of CRLs. Furthermore, other components of CRLs such as the E2 are also not necessarily static (Fig. 4), and studies have shown that different E2s may be responsible for the kinetics of different ubiquitination events such as mono-ubiquitination vs chain extension.59 In addition to composition, CRLs are highly dynamic in conformation. The cullin scaffold anchors and positions the catalytic core of the complex containing the E3 ligase and ubiquitin-loaded E2 relative to the SRR2,3,8,12,20,59,61,62 (Fig. 4).
While the geometry and activation of CRLs is tightly regulated through neddylation, target ubiquitination can only occur when lysines present on the target surface are in sufficient proximity to the ubiquitin-loaded E2.61,62 Many factors can influence this proximity including the high degree of structural dynamics that lead to many complex conformations61–63 (Fig. 4). Indeed, the flexibility of DDB1, the adaptor in CRL4 complexes depicted in Fig. 4, has been shown to allow for a wide range of spatial conformations.63 In addition to the intrinsic factors within the complex that influence target positioning, the length, flexibility, and composition of the linker in bifunctional degraders also play a pivotal role in orienting the target (Fig. 4). Compounds that only differ by subtle changes in linker can have dramatically different effects on target lysine positioning or accessibility,29,40 which both complicates and presents opportunities for bivalent degrader design. In contrast to bifunctional PROTAC degraders, molecular glues may exhibit fewer opportunities to optimize target positioning given the more rigid substrate receptor:target binding interface, and instead may be restricted to the positioning conferred by intrinsic properties of the CRL.12,64–69 Recent chemoproteomics studies using a photolenalidome probe demonstrated that IMiDs may facilitate binding of a broader array of targets to CRBN than previously thought but that ultimately may not be degraded, perhaps in part due to poor lysine proximity.70
Despite these complexities, positioning of target lysine residues coupled with sufficiently stable ternary complexes that provide enough time for ubiquitination to occur are likely the key drivers that determine the rate of ubiquitination for both bifunctional degraders and molecular glues. Measurements of cellular ubiquitination kinetics have revealed striking correlation with the degradation rate.25,27,42 While native protein degradation has been shown to primarily exhibit first-order kinetics, from the profiles shown in Fig. 3, degrader-induced kinetics may or may not always show first-order behaviour due to competing and/or differential cellular processes, as well as inability to target all populations of protein equally. For profiles which do not show first-order degradation kinetics, ubiquitination kinetics are often slow, and can sometimes mimic the multiphasic nature of the degradation profiles themselves, suggesting differential rates of ternary complex formation and/or ubiquitination with respect to specific target pools. While the activity of dubiquitinases (DUBs) has been suspected to play a role in antagonizing degrader mechanisms by deubiquitinating target proteins. Their specific involvement and impact on degrader efficacy has been limited, but an excellent example of USP15 counteracting CRBN molecular glue activity on particular neosubstrates has been shown.71 The diversity of DUB:target specificities, and the inability to selectively inhibit DUBs makes them difficult to study in this context. In cases where degraders fail to efficiently ubiquitinate their targets, it is possible that the rate of deubiquitination by DUBs predominates (Fig. 1), rather than resulting from poor target positioning within the ternary complex, ultimately leading to slow or partial profiles. Whether or not DUB activity is present, a bias in the kinetics of ubiquitination over deubiquitination is necessary for target degradation to occur.
Recent studies have demonstrated the utility of computational modelling approaches to simulate degradation kinetics from a mechanistic model of ternary complex and ubiquitination reaction kinetics45 or predict successful ubiquitination through modelling of ternary complex conformational dynamics.72–75 These conformational models include molecular dynamics simulations and ternary complex docking and ensemble clustering that either take advantage of available structures for protein:ligand complexes or incorporate biophysical techniques such as HDX-MS to inform on protein:protein interfaces.72–75 Many of these methods have resulted in accurate alignment of ternary ensembles with solved x-ray crystal structures. One which took into consideration the components of the entire CRL, identified potential target lysines in a “ubiquitination zone” relative to the ubiquitin-charged E2 on the target (Fig. 4), several of which were validated as ubiquitination sites in cellular studies.72
Conclusions
Determining cellular degradation kinetics is critical for complete characterization of degraders and profiles will be influenced by numerous factors; target:E3 combination and mechanisms, cell line, target expression level, E2/E3 machinery expression, and compound permeability and efflux (Fig. 5). Kinetic degradation analysis also yields insight into mechanism of action of event-driven degrader compounds, which will vary dependent upon the modality of degradation and therefore important to understand in each application. Kinetic analyses across a concentration series capture the cellular dynamics and efficacy of the degrader over a time, providing the broader picture which cannot be fully understood when assessing degradation at single times point or concentrations. Degradation profiles comprise numerous key parameters which can be measured and quantitated for comparison beyond standard Dmax and concentration plots. The multi-dimensional analysis of degraders which is possible with kinetic analysis allows for rank ordering of compounds across diverse set of measurements and offers insights in which mechanistic aspects are optimal and those that should be further optimized. For therapeutic degrader development, characterizing different cell type kinetic profiles will be very important for dosing, choice of indications or disease models, and understanding PK/PD relationships. Lessons learned with degraders will be foundational for future induced proximity compounds that have event-driven mechanisms of actions yet may have different functional outcomes. While event-driven pharmacology poses more challenges than occupancy-driven, this review highlights the advantages wherein individual steps in the process may be sub-optimal, but in can be compensated for with enhanced kinetics at downstream steps to yield highly efficacious compounds.
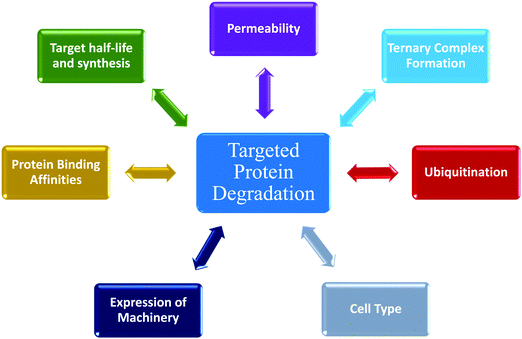 |
| Fig. 5 Numerous dynamic processes impacting targeted protein degradation via ubiquitin proteasome pathway (UPS). Targeted protein degradation is an event-driven process composed of multiple steps. This schematic summarizes many of the factors that impact degradation, each which have their own kinetic activities that influence the overall degradation profile. | |
Conflicts of interest
The authors declare no conflict of interest.
Notes and references
- S. Alabi and C. Crews, J. Biol. Chem., 2021, 100647, DOI:10.1016/j.jbc.2021.100647.
- M. Bekes, D. R. Langley and C. M. Crews, Nat. Rev. Drug Discovery, 2022, 21, 181–200 CrossRef CAS PubMed.
- G. M. Burslem and C. M. Crews, Cell, 2020, 181, 102–114 CrossRef CAS PubMed.
- P. P. Chamberlain and L. G. Hamann, Nat. Chem. Biol., 2019, 15, 937–944 CrossRef CAS PubMed.
- A. Ciulli and W. Farnaby, Drug Discovery Today Technol., 2019, 31, 1–3 CrossRef PubMed.
- R. J. Deshaies, Nat. Chem. Biol., 2015, 11, 634–635 CrossRef CAS PubMed.
- A. Mullard, Nat. Rev. Drug Discovery, 2021, 20, 247–250 CrossRef CAS PubMed.
- T. K. Neklesa, J. D. Winkler and C. M. Crews, Pharmacol. Ther., 2017, 174, 138–144 CrossRef CAS PubMed.
- M. Schapira, M. F. Calabrese, A. N. Bullock and C. M. Crews, Nat. Rev. Drug Discovery, 2019, 18, 949–963 CrossRef CAS PubMed.
- L. M. Luh, U. Scheib, K. Juenemann, L. Wortmann, M. Brands and P. M. Cromm, Angew. Chem., Int. Ed. Engl., 2020, 59, 15448–15466 CrossRef CAS PubMed.
- D. L. Daniels, K. M. Riching and M. Urh, Drug Discovery Today Technol., 2019, 31, 61–68 CrossRef PubMed.
- P. P. Chamberlain, L. A. D'Agostino, J. M. Ellis, J. D. Hansen, M. E. Matyskiela, J. J. McDonald, J. R. Riggs and L. G. Hamann, ACS Med. Chem. Lett., 2019, 10, 1592–1602 CrossRef CAS PubMed.
- S. L. Schreiber, Cell, 2021, 184, 3–9 CrossRef CAS PubMed.
- C. C. Bjorklund, J. Kang, M. Amatangelo, A. Polonskaia, M. Katz, H. Chiu, S. Couto, M. Wang, Y. Ren, M. Ortiz, F. Towfic, J. E. Flynt, W. Pierceall and A. Thakurta, Leukemia, 2020, 34, 1197–1201 CrossRef PubMed.
- E. Bulatov and A. Ciulli, Biochem. J., 2015, 467, 365–386 CrossRef CAS PubMed.
- S. J. Hughes and A. Ciulli, Essays Biochem., 2017, 61, 505–516 CrossRef PubMed.
- T. Ishida and A. Ciulli, SLAS Discovery, 2021, 26, 484–502 CrossRef CAS PubMed.
- A. Kannt and I. Dikic, Cell Chem. Biol., 2021, 28, 1014–1031 CrossRef CAS PubMed.
- P. Ottis, M. Toure, P. M. Cromm, E. Ko, J. L. Gustafson and C. M. Crews, ACS Chem. Biol., 2017, 12, 2570–2578 CrossRef CAS PubMed.
- S. Ramachandran and A. Ciulli, Curr. Opin. Struct. Biol., 2021, 67, 110–119 CrossRef CAS PubMed.
- M. Schneider, C. J. Radoux, A. Hercules, D. Ochoa, I. Dunham, L. P. Zalmas, G. Hessler, S. Ruf, V. Shanmugasundaram, M. M. Hann, P. J. Thomas, M. A. Queisser, A. B. Benowitz, K. Brown and A. R. Leach, Nat. Rev. Drug Discovery, 2021, 20, 789–797 CrossRef CAS PubMed.
- M. G. Costales, B. Suresh, K. Vishnu and M. D. Disney, Cell Chem. Biol., 2019, 26, 1180–1186 e1185 CrossRef CAS PubMed.
- S. K. Dey and S. R. Jaffrey, Cell Chem. Biol., 2019, 26, 1047–1049 CrossRef CAS PubMed.
- M. Pettersson and C. M. Crews, Drug Discovery
Today Technol., 2019, 31, 15–27 CrossRef PubMed.
- K. M. Riching, S. Mahan, C. R. Corona, M. McDougall, J. D. Vasta, M. B. Robers, M. Urh and D. L. Daniels, ACS Chem. Biol., 2018, 13, 2758–2770 CrossRef CAS PubMed.
- K. M. Riching, J. D. Vasta, S. J. Hughes, V. Zoppi, C. Maniaci, A. Testa, M. Urh, A. Ciulli and D. L. Daniels, Curr. Res. Chem. Bio., 2021, 1, 1 Search PubMed.
- S. Imaide, K. M. Riching, N. Makukhin, V. Vetma, C. Whitworth, S. J. Hughes, N. Trainor, S. D. Mahan, N. Murphy, A. D. Cowan, K. H. Chan, C. Craigon, A. Testa, C. Maniaci, M. Urh, D. L. Daniels and A. Ciulli, Nat. Chem. Biol., 2021, 17, 1157–1167 CrossRef CAS PubMed.
- D. P. Bondeson, B. E. Smith, G. M. Burslem, A. D. Buhimschi, J. Hines, S. Jaime-Figueroa, J. Wang, B. D. Hamman, A. Ishchenko and C. M. Crews, Cell Chem. Biol., 2018, 25, 78–87 e75 CrossRef CAS PubMed.
- K. H. Chan, M. Zengerle, A. Testa and A. Ciulli, J. Med. Chem., 2018, 61, 504–513 CrossRef CAS PubMed.
- M. S. Gadd, A. Testa, X. Lucas, K. H. Chan, W. Chen, D. J. Lamont, M. Zengerle and A. Ciulli, Nat. Chem. Biol., 2017, 13, 514–521 CrossRef CAS PubMed.
- H. T. Huang, D. Dobrovolsky, J. Paulk, G. Yang, E. L. Weisberg, Z. M. Doctor, D. L. Buckley, J. H. Cho, E. Ko, J. Jang, K. Shi, H. G. Choi, J. D. Griffin, Y. Li, S. P. Treon, E. S. Fischer, J. E. Bradner, L. Tan and N. S. Gray, Cell Chem. Biol., 2018, 25, 88–99 e86 CrossRef CAS PubMed.
- B. Jiang, Y. Gao, J. Che, W. Lu, I. H. Kaltheuner, R. Dries, M. Kalocsay, M. J. Berberich, J. Jiang, I. You, N. Kwiatkowski, K. M. Riching, D. L. Daniels, P. K. Sorger, M. Geyer, T. Zhang and N. S. Gray, Nat. Chem. Biol., 2021, 17, 675–683 CrossRef CAS PubMed.
- B. Jiang, E. S. Wang, K. A. Donovan, Y. Liang, E. S. Fischer, T. Zhang and N. S. Gray, Angew. Chem., Int. Ed. Engl., 2019, 58, 6321–6326 CrossRef CAS PubMed.
- M. P. Muller and D. Rauh, Cell Chem. Biol., 2018, 25, 4–6 CrossRef PubMed.
- K. M. Riching, M. K. Schwinn, J. D. Vasta, M. B. Robers, T. Machleidt, M. Urh and D. L. Daniels, SLAS Discovery, 2021, 26, 560–569 CrossRef CAS PubMed.
- C. M. Robb, J. I. Contreras, S. Kour, M. A. Taylor, M. Abid, Y. A. Sonawane, M. Zahid, D. J. Murry, A. Natarajan and S. Rana, Chem. Commun., 2017, 53, 7577–7580 RSC.
- M. Teng, J. Jiang, Z. He, N. P. Kwiatkowski, K. A. Donovan, C. E. Mills, C. Victor, J. M. Hatcher, E. S. Fischer, P. K. Sorger, T. Zhang and N. S. Gray, Angew. Chem., Int. Ed. Engl., 2020, 59, 13865–13870 CrossRef CAS PubMed.
- T. C. Ting, M. Goralski, K. Klein, B. Wang, J. Kim, Y. Xie and D. Nijhawan, Cell Rep., 2019, 29, 1499–1510 e1496 CrossRef CAS PubMed.
- G. E. Winter, D. L. Buckley, J. Paulk, J. M. Roberts, A. Souza, S. Dhe-Paganon and J. E. Bradner, Science, 2015, 348, 1376–1381 CrossRef CAS PubMed.
- G. E. Winter, A. Mayer, D. L. Buckley, M. A. Erb, J. E. Roderick, S. Vittori, J. M. Reyes, J. di Iulio, A. Souza, C. J. Ott, J. M. Roberts, R. Zeid, T. G. Scott, J. Paulk, K. Lachance, C. M. Olson, S. Dastjerdi, S. Bauer, C. Y. Lin, N. S. Gray, M. A. Kelliher, L. S. Churchman and J. E. Bradner, Mol. Cell, 2017, 67, 5–18 e19 CrossRef CAS PubMed.
- M. Zengerle, K. H. Chan and A. Ciulli, ACS Chem. Biol., 2015, 10, 1770–1777 CrossRef CAS PubMed.
- V. Zoppi, S. J. Hughes, C. Maniaci, A. Testa, T. Gmaschitz, C. Wieshofer, M. Koegl, K. M. Riching, D. L. Daniels, A. Spallarossa and A. Ciulli, J. Med. Chem., 2019, 62, 699–726 CrossRef CAS PubMed.
- R. P. Nowak, S. L. DeAngelo, D. Buckley, Z. He, K. A. Donovan, J. An, N. Safaee, M. P. Jedrychowski, C. M. Ponthier, M. Ishoey, T. Zhang, J. D. Mancias, N. S. Gray, J. E. Bradner and E. S. Fischer, Nat. Chem. Biol., 2018, 14, 706–714 CrossRef CAS PubMed.
- K. A. Donovan, F. M. Ferguson, J. W. Bushman, N. A. Eleuteri, D. Bhunia, S. Ryu, L. Tan, K. Shi, H. Yue, X. Liu, D. Dobrovolsky, B. Jiang, J. Wang, M. Hao, I. You, M. Teng, Y. Liang, J. Hatcher, Z. Li, T. D. Manz, B. Groendyke, W. Hu, Y. Nam, S. Sengupta, H. Cho, I. Shin, M. P. Agius, I. M. Ghobrial, M. W. Ma, J. Che, S. J. Buhrlage, T. Sim, N. S. Gray and E. S. Fischer, Cell, 2020, 183, 1714–1731 e1710 CrossRef CAS PubMed.
- D. W. Bartlett and A. M. Gilbert, J. Pharmacokinet. Pharmacodyn., 2021, 48, 149–163 CrossRef CAS PubMed.
- K. M. Riching, S. D. Mahan, M. Urh and D. L. Daniels, J. Vis. Exp., 2020, 165 DOI:10.3791/61787.
- E. A. Caine, S. D. Mahan, R. L. Johnson, A. N. Nieman, N. Lam, C. R. Warren, K. M. Riching, M. Urh and D. L. Daniels, Curr. Protoc. Pharmacol., 2020, 91, e81 CAS.
- W. Farnaby, M. Koegl, M. J. Roy, C. Whitworth, E. Diers, N. Trainor, D. Zollman, S. Steurer, J. Karolyi-Oezguer, C. Riedmueller, T. Gmaschitz, J. Wachter, C. Dank, M. Galant, B. Sharps, K. Rumpel, E. Traxler, T. Gerstberger, R. Schnitzer, O. Petermann, P. Greb, H. Weinstabl, G. Bader, A. Zoephel, A. Weiss-Puxbaum, K. Ehrenhofer-Wolfer, S. Wohrle, G. Boehmelt, J. Rinnenthal, H. Arnhof, N. Wiechens, M. Y. Wu, T. Owen-Hughes, P. Ettmayer, M. Pearson, D. B. McConnell and A. Ciulli, Nat. Chem. Biol., 2019, 15, 672–680 CrossRef CAS PubMed.
- A. J. Tao, G. E. Gadbois, S. A. Buczynski and F. M. Ferguson, Curr. Opin. Chem. Biol., 2022, 67, 102114 CrossRef CAS PubMed.
- X. Luo, I. Archibeque, K. Dellamaggiore, K. Smither, O. Homann, J. R. Lipford and D. Mohl, iScience, 2022, 25, 103985 CrossRef CAS PubMed.
- G. M. Burslem, B. E. Smith, A. C. Lai, S. Jaime-Figueroa, D. C. McQuaid, D. P. Bondeson, M. Toure, H. Dong, Y. Qian, J. Wang, A. P. Crew, J. Hines and C. M. Crews, Cell Chem. Biol., 2018, 25, 67–77 e63 CrossRef CAS PubMed.
- S. D. Mahan, K. M. Riching, M. Urh and D. L. Daniels, Methods Mol. Biol., 2021, 2365, 151–171 CrossRef PubMed.
- M. J. Roy, S. Winkler, S. J. Hughes, C. Whitworth, M. Galant, W. Farnaby, K. Rumpel and A. Ciulli, ACS Chem. Biol., 2019, 14, 361–368 CrossRef CAS PubMed.
- P. S. Dragovich, T. H. Pillow, R. A. Blake, J. D. Sadowsky, E. Adaligil, P. Adhikari, S. Bhakta, N. Blaquiere, J. Chen, J. Dela Cruz-Chuh, K. E. Gascoigne, S. J. Hartman, M. He, S. Kaufman, T. Kleinheinz, K. R. Kozak, L. Liu, L. Liu, Q. Liu, Y. Lu, F. Meng, M. M. Mulvihill, A. O'Donohue, R. K. Rowntree, L. R. Staben, S. T. Staben, J. Wai, J. Wang, B. Wei, C. Wilson, J. Xin, Z. Xu, H. Yao, D. Zhang, H. Zhang, H. Zhou and X. Zhu, J. Med. Chem., 2021, 64, 2534–2575 CrossRef CAS PubMed.
- P. S. Dragovich, T. H. Pillow, R. A. Blake, J. D. Sadowsky, E. Adaligil, P. Adhikari, J. Chen, N. Corr, J. Dela Cruz-Chuh, G. Del Rosario, A. Fullerton, S. J. Hartman, F. Jiang, S. Kaufman, T. Kleinheinz, K. R. Kozak, L. Liu, Y. Lu, M. M. Mulvihill, J. M. Murray, A. O'Donohue, R. K. Rowntree, W. S. Sawyer, L. R. Staben, J. Wai, J. Wang, B. Wei, W. Wei, Z. Xu, H. Yao, S. F. Yu, D. Zhang, H. Zhang, S. Zhang, Y. Zhao, H. Zhou and X. Zhu, J. Med. Chem., 2021, 64, 2576–2607 CrossRef CAS PubMed.
- T. H. Pillow, P. Adhikari, R. A. Blake, J. Chen, G. Del Rosario, G. Deshmukh, I. Figueroa, K. E. Gascoigne, A. V. Kamath, S. Kaufman, T. Kleinheinz, K. R. Kozak, B. Latifi, D. D. Leipold, C. Sing Li, R. Li, M. M. Mulvihill, A. O'Donohue, R. K. Rowntree, J. D. Sadowsky, J. Wai, X. Wang, C. Wu, Z. Xu, H. Yao, S. F. Yu, D. Zhang, R. Zang, H. Zhang, H. Zhou, X. Zhu and P. S. Dragovich, ChemMedChem, 2020, 15, 17–25 CrossRef CAS PubMed.
- R. P. Law, J. Nunes, C. W. Chung, M. Bantscheff, K. Buda, H. Dai, J. P. Evans, A. Flinders, D. Klimaszewska, A. J. Lewis, M. Muelbaier, P. Scott-Stevens, P. Stacey, C. J. Tame, G. F. Watt, N. Zinn, M. A. Queisser, J. D. Harling and A. B. Benowitz, Angew. Chem., Int. Ed. Engl., 2021, 60, 23327–23334 CrossRef CAS PubMed.
- L. T. Henneberg and B. A. Schulman, Cell Chem. Biol., 2021, 28, 889–902 CrossRef CAS PubMed.
- N. Zheng and N. Shabek, Annu. Rev. Biochem., 2017, 86, 129–157 CrossRef CAS PubMed.
- S. L. Paiva and C. M. Crews, Curr. Opin. Chem. Biol., 2019, 50, 111–119 CrossRef CAS PubMed.
- D. Horn-Ghetko, D. T. Krist, J. R. Prabu, K. Baek, M. P. C. Mulder, M. Klugel, D. C. Scott, H. Ovaa, G. Kleiger and B. A. Schulman, Nature, 2021, 590, 671–676 CrossRef CAS PubMed.
- K. Baek, D. C. Scott and B. A. Schulman, Curr. Opin. Struct. Biol., 2021, 67, 101–109 CrossRef CAS PubMed.
- J. Lee and P. Zhou, Mol. Cell, 2007, 26, 775–780 CrossRef CAS PubMed.
- M. Slabicki, Z. Kozicka, G. Petzold, Y. D. Li, M. Manojkumar, R. D. Bunker, K. A. Donovan, Q. L. Sievers, J. Koeppel, D. Suchyta, A. S. Sperling, E. C. Fink, J. A. Gasser, L. R. Wang, S. M. Corsello, R. S. Sellar, M. Jan, D. Gillingham, C. Scholl, S. Frohling, T. R. Golub, E. S. Fischer, N. H. Thoma and B. L. Ebert, Nature, 2020, 585, 293–297 CrossRef CAS PubMed.
- C. Mayor-Ruiz, S. Bauer, M. Brand, Z. Kozicka, M. Siklos, H. Imrichova, I. H. Kaltheuner, E. Hahn, K. Seiler, A. Koren, G. Petzold, M. Fellner, C. Bock, A. C. Muller, J. Zuber, M. Geyer, N. H. Thoma, S. Kubicek and G. E. Winter, Nat. Chem. Biol., 2020, 16, 1199–1207 CrossRef CAS PubMed.
- K. Baek and B. A. Schulman, Nat. Chem. Biol., 2020, 16, 2–3 CrossRef CAS PubMed.
- X. Du, O. A. Volkov, R. M. Czerwinski, H. Tan, C. Huerta, E. R. Morton, J. P. Rizzi, P. M. Wehn, R. Xu, D. Nijhawan and E. M. Wallace, Structure, 2019, 27, 1625–1633 e1623 CrossRef CAS PubMed.
- Z. Kozicka and N. H. Thoma, Cell Chem. Biol., 2021, 28, 1032–1047 CrossRef CAS PubMed.
- T. Han, M. Goralski, N. Gaskill, E. Capota, J. Kim, T. C. Ting, Y. Xie, N. S. Williams and D. Nijhawan, Science, 2017, 356, eaal3755 CrossRef PubMed.
- Z. Lin, Y. Amako, F. Kabir, H. A. Flaxman, B. Budnik and C. M. Woo, J. Am. Chem. Soc., 2022, 144, 606–614 CrossRef CAS PubMed.
- T. V. Nguyen, Proc. Natl. Acad. Sci. U. S. A., 2021, 118, e2111391118 CrossRef PubMed.
- N. Bai, K. M. Riching, A. Makaju, H. Wu, T. M. Acker, S. C. Ou, Y. Zhang, X. Shen, D. Bulloch, H. Rui, B. Gibson, D. L. Daniels, M. Urh, B. Rock and S. C. Humphreys, J. Biol. Chem., 2022, 101653, DOI:10.1016/j.jbc.2022.101653.
- M. L. Drummond, A. Henry, H. Li and C. I. Williams, J. Chem. Inf. Model., 2020, 60, 5234–5254 CrossRef CAS PubMed.
- M. L. Drummond and C. I. Williams, J. Chem. Inf. Model., 2019, 59, 1634–1644 CrossRef CAS PubMed.
- G. Weng, D. Li, Y. Kang and T. Hou, J. Med. Chem., 2021, 64, 16271–16281 CrossRef CAS PubMed.
Footnote |
† Current address: Foghorn Therapeutics, 500 Technology Square Suite 700, Cambridge, MA USA 02139. |
|
This journal is © The Royal Society of Chemistry 2022 |
Click here to see how this site uses Cookies. View our privacy policy here.