DOI:
10.1039/D1SM00703C
(Paper)
Soft Matter, 2021,
17, 6773-6786
Investigating lipid headgroup composition within epithelial membranes: a systematic review†
Received
12th May 2021
, Accepted 23rd June 2021
First published on 24th June 2021
Abstract
Membrane lipid composition is often quoted within the literature, but with very little insight into how or why these compositions vary when compared to other biological membranes. One prominent area that lacks understanding in terms of rationale for lipid variability is the human gastro-intestinal tract (GIT). We have carried out a comprehensive systematic literature search to ascertain the key lipid components of epithelial membranes, with a particular focus on addressing the human GIT and to use compositional data to understand structural aspects of biological membranes. Both bacterial outer membranes and the human erythrocyte membrane were used as a comparison for the mammalian [epithelial] membranes and to understand variations in lipid presence. We show that phosphatidylcholine (PC) lipid types tend to dominate (33%) with phosphatidylethanolamines (PE) and cholesterol having very similar abundances (25 and 23% respectively). This systematic review presents a detailed insight into lipid headgroup composition and roles in various membrane types, with a summary of the distinction between the major lipid bilayer forming lipids and how peripheral lipids regulate charge and fluidity. The variety of lipids present in biological membranes is discussed and rationalised in terms function as well as cellular position.
Introduction
Almost 50 years on from the proposal of the fluid mosaic model of cell membranes by Singer and Nicholson in 1972, the model is still relatively untouched and is accepted today as the foundation of our understanding of cell surfaces.1 Since then it has been amended and updated in order to agree with current understanding, forming a cornerstone of a field that is in constant change.2
The cell membrane is an extremely complex set of structure and functions. Because of this, being able to understand it at a fundamental level is challenging and requires a number of different techniques to be able to provide information. Historically biological mimetic (biomimetic) membranes were studied using monolayers at the air–water interface through techniques such as Langmuir–Blodgett deposition.3 Moving forwards, more complex analyses of increasingly advanced and accurate models have been developed, including using solid state NMR techniques to help understand bilayer dynamics, probing layers on a solid support through atomic force microscopy, or investigations of bilayers using a quartz crystal microbalance with dissipation monitoring, attenuated total reflection FTIR, or much more powerful techniques such as small angle X-ray scattering (SAXS), neutron reflectometry (NR), or small angle neutron scattering (SANS).4–10 For systems such as DSC, SANS, and NR bilayer deposition, micelle/vesicle sizes of 50–200 nm are common.11,12 In order to gain an in depth and accurate understanding of how the biological membrane functions, a number of these techniques are needed in tandem. Fig. 1 shows some examples of systems used as foundations for membrane modelling. It should be made clear that even the most complex biomimetic models do not yet reflect the cell in its entirety. It is common for each model to examine a small number of functions or components but given that biological membranes include all kinds of complex parts (ion channels, receptors, surface proteins, surface carbohydrates, etc.) it is a hard task to investigate all of these at once. Whilst modelling does not always completely imitate the real world state of the system, these methods are also valuable tools in understanding membrane interactions.13,14
 |
| Fig. 1 Schematics of some typical methods for modelling lipid systems and for observing their interactions; top left – micelles; typical of surfactants and other hydrophobic molecules when introduced to an aqueous environment. Top right – monolayers; very common set-up for Langmuir–Blodgett type experiments for measuring lipid surface pressure and tension. Bottom left – vesicles; these can adopt a large size regime and are frequently used to mimic simple bilayer interactions when formed with lipids. Bottom right – asymmetric bilayers; complex layer formation is taking centre stage in many surface sensitive techniques as they become more accurate and complex. | |
At present, the mammalian membrane environment is considered to be dominated by glycerophospholipids and supplemented by the presence of sphingolipids and sterols.15 This review sets out to bring together the understanding that surrounds membrane systems, in particular those that are relevant to epithelial surfaces and identify potential gaps in the literature as far as mammalian model systems are concerned. Further, we show that bacterial modelling is extremely popular as a method for examining the effectiveness of antimicrobial agents16 and compare the compositions of the mammalian epithelium and the human erythrocyte. Within the membrane environment, epithelial cells are polarised and are able to maintain separation between the apical, lumen facing interface, and the basolateral portion. Even within these adjacent domains of a single cell, there is localised variation in the distribution of species across the surface and that relative concentrations of various components can have large implications in the function of a surface.17,18 Given that there is such variation in lipid composition within the natural world, this invites questions concerning why there are so many kinds of lipids, and do differences in lipid species at a surface have an effect on function?19 While lipids form such a ubiquitous part of the natural world, the reason for their variation and breadth remains relatively poorly understood.20 Cells expend a lot of resources on the synthesis of the vast array of lipids within a membrane – but for what reason?21 It is prevalent throughout the biological world that structure is secondary to function; that is that there is an implicit reason for such a diverse range of lipid species.22 Bacterial membranes can also be built from the neat lipid components, or alternatively by using bacterial lipid extracts, both of which are suitable for investigation using the techniques outlined above.23 The large scope for variability in membrane composition and structure gives rise to a range of ways to observe and model such membranes. As epithelia differ in their specific composition from cell line models to bacterial to simple mammalian epithelial linings.20,24,25 With differing structure and function comes differing methods for probing the various aspects of a membrane.26 Quintessentially these reviews highlight that as techniques and technology progresses, so too does our understanding.
When modelling lipid systems, as with any model, there are a lot of components to consider. It is important to try and get the model to describe its real-world counterpart, although naturally as the accuracy becomes better the complexity often increases as a result. In this article, the aim is to understand the content of the scientific literature through a holistic approach in order to gain insight into what is known about lipid composition in general, as well as in more detail at a tissue/biological interface specific level in order to identify possible gaps or areas of need in the scientific knowledge. We set out to answer the question “What is the composition of epithelial membranes?” Throughout this article the kinds of species shown in Fig. 2 are referred to. Fig. 2 gives a breakdown of how the species are related, the chemical class they belong to, as well as a brief schematic representation.
 |
| Fig. 2 Depiction of lipid classes, along with categorical examples of each class and a simplified schematic representations. Chain lengths and degree of saturation varies within natural membranes, typically ranging from around C-14 to C-24. | |
From Fig. 2, triacylglycerides are a large component in mammalian fats, as well as present in the blood. In all their various guises glycerophospholipids, are the main components of biological membranes. There is evidence to show that the difference between phospholipids and sphingolipid chain packing is heavily involved in membrane domain formation, and the regulation of lipid rafts,27 although lipid rafts remain a controversial topic.28 Phospholipids have a higher degree of unsaturation resulting in a more disperse lipid packing in the membrane. Sphingolipids are lipids that contain a sphingoid base, and play important roles in cell recognition. Sphingolipids tend to have much longer and more saturated chains when compared to other membrane species (sterols, phospholipids, etc.) which results in a higher transition temperature as a result of tighter packing geometries.29 Glycolipids are a class of lipid that contain a glycosidic carbohydrate; like sphingolipids they also play a role in cell recognition, and they help to provide structural stability to the cell membrane.30–32 Glycolipids, both N-linked and O-linked, are thought to be involved in the apical signalling and sorting machinery.33
Structures of some major cellular lipid components are shown below in Fig. 3. Common to all of them is the glycerol-derived backbone along with the R-groups that denote the scope for varying hydrocarbon chains that differ by length and saturation which varies within natural membranes, typically ranging from around C-14 to C-24.30,34 Shown in blue are the head groups that are phosphate bound and vary between lipid classes. As well as chain length and saturation cholesterol plays a vital role in mammalian membrane structure to preserve integrity and the regulation of the fluidity of the bilayer through how it impacts the packing of lipid tails.35–37 It is understood that cholesterol is found preferentially in the plasma membrane when compared to intracellular membranes.38
 |
| Fig. 3 Structures of some membrane lipid head groups shown in blue that are phosphate bound. The glycerol-derivative backbone is also shown along with the positions of the R-groups that can vary in length and saturation. | |
Previously, it was thought that within any given membrane environment a single lipid species would dominate, and that other lipids were only present on much smaller scales and within local domains when required for specific functions. However, more recently it is understood that local environments contain a broader range of lipids that are derivatised and have more specific local functions.39,40 Phospholipids, once derivatised, can become distributed throughout the membrane differently which will contribute towards cell membrane functionality in some way.41
Epithelial cells are often characterised as having two major distinct portions which are functionally separate. The lumen facing portion of the cell is described as apical, while the bottom is described as basal. Tight junctions are responsible for connecting adjacent cells with a tight seal, with the seal acting to prevent movements around the outsides of the cell, as well as maintaining distinct membrane portions.42,43 Just as lipids are polarised between epithelial cell membrane portions, so too are proteins; it has been shown in cells that are tight junction deficient that polarity is maintained and the lipid and protein distribution does not differ from cells that contain tight junctions.44
It is has been understood for some time that lipids are not symmetrically distributed across the membrane leaflets; choline containing lipids tend towards the outer leaflet while anionic lipids are located preferentially on the inner leaflet, as well as other species such as PIP2.45–47 There are also reports of PE being more abundant in the inner leaflet, and this is covered in other reviews.48,49 These kinds of compositions, and their effects, have also been studied computationally. Simulations show the effects of composition, chain length, curvature, and thermodynamic behaviours.50
In this study, we use a comprehensive literature search to systematically investigate the lipid head group composition of mammalian epithelial cells, with a particular focus on those that are intestinal and from the human GIT. Rationale for understanding the lipid composition of the GIT stems from the importance of helping to understand how these biological surfaces are structured and how they behave. By using the compositional data to inform models, there is scope for this kind of information to develop how models behave during illness or disease, and even to help study the effects of pharmaceutical compounds on these kinds of biological surfaces. Further, the principles used can be taken and applied elsewhere, for example closely related surfaces such as other epithelia can be similarly modelled. This article aims to identify gaps within the literature where data relating to GIT lipid composition is concerned, and identify some open questions that can help shape research in this area moving forwards. First, the contents of epithelial membranes are studied, and then these are broken down into various subgroups depending on the source of the epithelium. These head group compositions are then compared to bacterial outer membranes and the epithelium of human erythrocytes. Similarities and differences between the various membrane types are considered all with the intention of understanding reasons for lipid variability within membranes.
Methodology
Systematic review of epithelial cell composition
The focus of this systematic review was to search terms for lipid membranes that linked to the lipid head group composition of these lipids, as this will provide crucial information that will serve as a basis for (i) providing insight into how the membrane operates as well as (ii) serving as a basis for model membrane synthesis. The composition of the epithelial membranes was investigated, along with bacterial outer membrane composition and that of the human erythrocyte for modes of comparison. Bacterial membranes were chosen because they are relatively well characterised and understood, having long since been used as membrane models. Human erythrocytes were chosen to allow comparison to another human epithelial membrane, especially for the case of comparing to human cell types. The first step in this study was to screen the literature for articles containing relevant information on cell membrane lipid composition. This was done through a systematic search using the database Web of Science®, searching all databases and screening by document type for ‘Articles’ Search terms were selected in order to remove unwanted hits and then secondary search terms were used in order to focus a broad result into a list of results that contained relevant information. The search was not filtered by year, and was from 1970–2020. Note – 1970 was chosen as the start year as this is the earliest year listed when going through the filtering step.
Articles were collected through two independent searches, and the results were combined. Each search was comprised of two lists shown in Fig. 4b: lists A and B were used together while lists 1 and 2 were also paired. During searches, search terms were combined using the Boolean operators OR between groups showing results that appeared through both. The two sets of lists were combined using the AND operator, which resulted in only articles featuring in both searches being displayed. Every possible combination of searches within the respective lists was used to generate results and the lists are shown below.
 |
| Fig. 4 (a) Flow diagram that summarises the method for the selection of articles. (b) Schematic showing how search terms were combined between lists using both AND and OR Boolean operators. | |
The literature search found studies that met the inclusion criteria; (a) lipid composition, (b) epithelial membranes, and (c) membrane structure for healthy cells.51–105 Any studies from the search that did not contain quantitative information were discarded from the results. Data from the remaining studies were collated and analysed in order to assess the lipid composition of biological species and are presented below. The selection criteria were chosen to allow thousands of publications to be narrowed through the title or abstract of the publication. They were selected in a way that allowed a multi-layered analysis of the state of epithelial composition in mammalian cells as a whole, ending in a very specific view of lipid composition of the human GIT. The search was conducted independently by 2 authors, and collation of the results was filtered and results collated by a single author examining abstracts and applying inclusion/exclusion criteria. The results include a range of mammalian animal types. From the 54 search results acquired there were 75 individual sets of data that related to lipid headgroup composition of one of the relevant tissues outlined above, that cover a very broad range of cell types. These can be divided down into subgroups for data processing based on cell type, location, those that are specific to humans, etc. These will be covered in more detail within the results section of the review and comparisons between such subsets of data are shown in Fig. 8.
As a final note, while the head group composition was the focus of this review, it is understood that tail length and level of unsaturation all have important roles, these are commented on in later parts of this review, although not investigated and scrutinised as intensely as the head group composition.106 Further, composition across leaflets is not quantitatively investigated, however it should be acknowledged that this phenomenon occurs in most biomembranes.49
Fig. 5 below shows a breakdown of all the results from the search based on how many lipid species were reported in their analysis, with some reporting up to 8 lipids and others as little as 3. 71% of all the publications found reported 5 or more lipids.
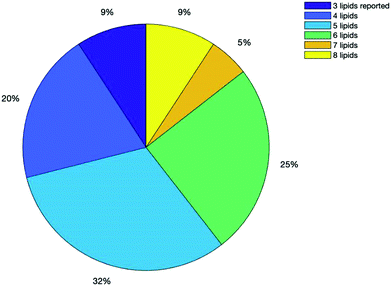 |
| Fig. 5 Chart showing number of lipids reported in the search results by percentage. | |
Bacterial outer membrane composition
Following the search for epithelial membranes, it was important to understand what makes the human epithelial layer unique and how it contrasts from other membranes that play similar roles. It should be understood that the searches for both bacterial outer membranes and the human erythrocyte were not comprehensive systematic searches. Instead they serve as markers for comparison. Since bacterial membranes are relatively well understood these were chosen as a point of reference. To get insight into bacterial membrane compositions, a cross section of the literature was found with three distinct search terms which were connected with the Boolean AND operator:
• Bacterial.
• Outer membrane.
• Phosphatidylethanolamine.
The search was conducted using Web of Science, within “All Databases”. The date was not limited and the native range is 1970–2020. When compared to bacterial composition, there are significant differences in the presence of lipids that are common to both membrane types. Search term results were narrowed down and selected by including studies that evaluated (outer) membrane composition of both Gram-positive and Gram-negative bacteria; n = 21.107–127
Human erythrocyte membrane composition
The human erythrocyte membrane was also used as a membrane comparison. Comparing and contrasting this with bacterial and human membranes provided insight into how differences have an impact on function, or instead, is lipid composition optimised according to function? To that end, a lipid composition search was carried out for human erythrocyte membranes. The search was designed from the outset to only include membranes from erythrocytes that were specifically human, and contained several lipid classes in their analysis. The search terms comprised of two pairs (a) membrane composition OR phospholipid composition AND (b) human erythrocyte OR human red blood cell; each pair being combined using a Boolean OR operator, and then a total AND combinator to yield 23 results, of which only 9 were appropriate for inclusion.128–136 One important note on the erythrocyte data; only 2 of the 9 compositions contained data that related to cholesterol content, and as such it may not be completely representative. However, where cholesterol contents were reported, the amounts of other lipids were reduced in all cases compared to where they were not reported. This may result in artificial inflation of the lipid values of the other membrane lipids. To ensure that the lack of cholesterol data was not erroneously inflating the remaining lipid data, the two values that were taken from the literature search (49.75 and 45.46%) were averaged and used for all missing cholesterol values. The averaged value of 47.6% is in agreement with the literature that describes human erythrocyte membrane composition.28,38,137–139 From the average cholesterol values, the remaining lipid compositions were adjusted. As a result of the majority of the data for cholesterol being the same average value this leads to smaller than usual standard deviations and error value; however, this does lead to a more representative measurement than having artificially large lipid head group data.
Publication rate for studies relating to ‘lipid composition’
One of the first things that was considered when conducting this study, is how the phospholipid composition has appeared in the literature over time. Fig. 6 shows the number of publications as a function of time that are discoverable under the search term “lipid composition” in the Web of Science database. The explosion in the number of publications in the early 1990s likely comes as result of the ‘-omics’ trend; the leaps in mass spectrometry and liquid chromatography technologies, along with developments in tandem methodologies, has enabled lipids to enter the omics realm behind genomics and proteomics.140 Within the figure, the blue bars are representative of the total number of publications for a given year where a topic was lipid composition. Within these, the other coloured bars represent categories by which the results can be sub-divided into “human”, “bacterial”, “mammalian”, or “intestine”, etc. The smaller coloured bars are included within the total “Lipid composition” count and form part of the blue bars.
 |
| Fig. 6 The number of publications for a given year as a result of the search term ‘lipid composition’. Colouring within the bars represents the total number of publications in a given filter term used to refine the overall ‘lipid composition’ search. The smaller coloured bars are included within the total count and form part of the blue bars. | |
Lipid compositional studies
Epithelial membrane composition
It should be noted that when seeking out lipid compositional and lipidomic studies there can be a large amount of variability in the lipids that are examined within these studies, which is described and visualised in more detail in the ESI.† Through searching the literature our search provided 54 papers that have defined lipid composition but from a variety of biological sources for epithelial cells. The data averaging lipid composition for all 54 is displayed in Fig. 7. While this hides any variability between different types of epithelial cells it does allow identification of the major lipid components seen within these systems. We report lipids as a relative percentage of total lipid content within each study. This serves to give a general overview of general lipid content within nature, and more specifically mammalian biology, which lipids tend to dominate.
 |
| Fig. 7 Values of overall mean lipid content taken from the studies found as a result of the initial literature search in Web of Science; n = 54. These percentages represent the proportion of lipids present in all membranes irrespective of origin or type. Numerical values for the mean are presented along with their standard deviation. Tabular results can be found in the ESI,† in Table S3. | |
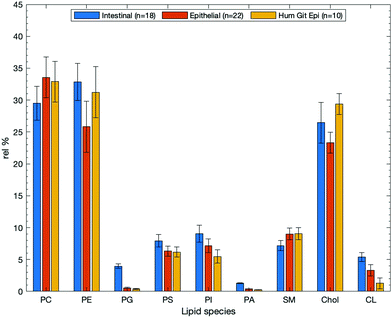 |
| Fig. 8 Mean compositional data for intestinal studies obtained from the systematic review search for articles that pertained to intestinal tissues (n = 18), epithelial samples (n = 22), and samples that related to the human GIT epithelium (n = 10). Values are plotted as relative means, and data are given as relative means SEM in Table 1. Individual plots with their associated error bars are given in the ESI.† | |
The 54 papers included in this study define lipid content from a variety of epithelial membrane sources. It is clear that within the majority of compositions, PC and PE lipids dominate, along with cholesterol, with average compositions of ca. 37, 26 and 25% respectively It has been reported that PC accounts for >50% of the lipids within eukaryotic membranes, although the results from this review show that this may be an over-estimation.22 No doubt, the variability within lipid composition is dependent on the tissue type in terms of its origin, structure and function. These factors will have an impact on the phospholipid content and this is something that this text seeks to explore further.
When lipid membranes’ composition are taken as an average this does not by necessity take into account the function of those membranes. In order to make judgements about different membrane types, a more focussed analysis must be conducted. Below comparisons are drawn from data obtained in the review and will show specific data grouped together by cell type or by environment to investigate how lipid compositional trends differ as a function of biological geography. One limitation of the literature search was that not all papers reported exclusively cell membrane composition. Where the membrane was not isolated, these data may reflect more the whole cell lipid isolate. As such, these data should be handled with care and later are separated out into various surface types to reduce the effects of non-specific lipid extraction.
Studies that contained lipid compositional data for intestinal samples (n = 18) showed that the three most dominant lipids are PE, PC, and Chol have relative percentages of 32.9 ± 2.9, 29.5 ± 2.5, and 26.5 ± 3.5% respectively (see Table 1). This is different when compared with epithelial or human GIT epithelial membranes which show PC as the most abundant lipid. Interestingly, SM is around 3% lower from the mean data (Fig. 7); a decrease that could be explained by the increased prevalence of other bilayer lipids such as PS, PI, and PA, each with their own structural roles. Intestinal data, which is not strictly from epithelial samples, shows elevated amounts of the anionic lipids. This may suggest that anionic lipids do not play as much of a role in epithelial and surface cells as they might in basal or non-lumen facing cell portions- shown in Fig. 8. Note that while not all these data are from human intestinal samples, they are still deemed relevant in that they perform the same function: acting as a barrier for absorption of nutrients from the GIT. Conversely, cholesterol is the most abundant membrane species in human erythrocytes, going against the trend of being PC or PE dominant.
Table 1 Comparison of the lipid compositions of samples taken from the literature study conducted as outlined above. Data below is from studies taken from the total 54 articles found, and divided into subsets related to intestinal (n = 18), epithelial (n = 22), and human GIT epithelial samples (n = 10). Values are given as rel% and quoted alongside are the SEM and standard deviations of the mean
Lipid |
Epithelia (n = 18) |
SEM |
Std dev. |
Intestinal (n = 22) |
SEM |
Std dev. |
hum. GIT epi. (n = 10) |
SEM |
Std dev. |
PC |
37.0 |
1.7 |
15.1 |
29.5 |
2.6 |
12.1 |
33.6 |
3.1 |
13.1 |
PE |
26.1 |
1.5 |
13.2 |
32.9 |
2.9 |
13.4 |
25.8 |
3.9 |
16.5 |
PG |
1.8 |
0.4 |
1.8 |
4.0 |
1.3 |
2.3 |
0.6 |
0.2 |
0.3 |
PS |
7.9 |
0.5 |
3.6 |
7.9 |
0.9 |
4.0 |
6.3 |
0.9 |
3.2 |
PI |
7.9 |
1.1 |
7.9 |
8.5 |
1.4 |
5.7 |
7.2 |
1.1 |
4.3 |
PA |
1.4 |
0.4 |
1.6 |
1.3 |
0.0 |
0.0 |
0.4 |
0.1 |
0.1 |
SM |
10.1 |
0.9 |
7.1 |
7.2 |
0.7 |
3.4 |
9.0 |
1.0 |
3.8 |
Chol |
24.5 |
1.7 |
9.1 |
26.4 |
3.5 |
10.4 |
23.3 |
2.1 |
6.7 |
CL |
4.9 |
0.8 |
3.0 |
5.4 |
1.1 |
3.1 |
3.3 |
1.4 |
3.4 |
There is evidence that cholesterol and sphingomyelin composition have an impact on each other. Cholesterol has been shown to be associated with SM in preference of other membrane species.141 The effect of SM presence within a bilayer on cholesterol was demonstrated to be more important than cholesterol's interactions with the layer lipids.142 Cholesterol and SM have both been linked to the production of laterally organised microdomains (rafts) within membranes, and these topics have been reviewed elsewhere.29,143,144
Where epithelial surfaces are concerned (n = 22, although not necessarily exclusive to the GIT) PC, PE, and Chol are still the dominant lipids with amounts of 33.6, 25.8, and 23.3% respectively. However, unlike for intestinal samples, PC is the most abundant lipid here. The relatively low proportion of cholesterol in epithelial surfaces is likely related to their function, as all interfaces that are lumen facing are required to be relatively fluid to allow transfer processes to occur. These data are shown in Fig. 8 as the orange bars. Intestinal samples (n = 18) are of origin from the intestinal organs, but are not always specified as either human or epithelial. Samples from the search that satisfied all the criteria of human, epithelial, and intestinal studies (n = 10) were collated to allow investigation of specific types of epithelial surface. Again, in the human intestinal epithelium it is shown that PC, PE, and cholesterol are clearly the most abundant. Peripheral lipids PS and PI each make up around 5–10% of the environment. These both help regulate charge in the membrane, as in the other two categories that were examined, and maintaining a balance of polarity/non-polarity across the membrane helps to only allow desired species to cross the barrier. Human GIT epithelial compositions are shown in Fig. 8 as yellow bars. The data for all three subsets can be found in Table 1.
Bacterial membrane composition
When compared to bacterial composition, there are obvious differences in the presence of lipids that are common to both membrane types and can be seen in Fig. 9. Alongside this, comparisons are drawn to the main bacterial lipids (PE, PG, and CL) as they appear in mammalian cells in Table 2.
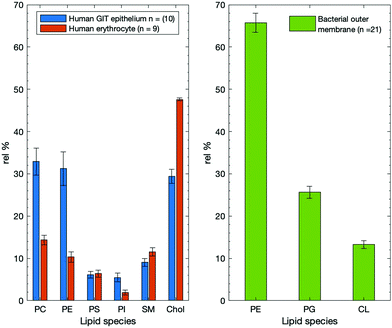 |
| Fig. 9 Figure showing the mean relative percentage lipid compositions of the human GIT epithelium (blue, n = 10), the human erythrocyte membrane (orange, n = 9), and a bacterial outer membrane (green, n = 21). The GIT and erythrocyte membranes are compared according to the common lipid species that are contained within the membrane, while all the lipid species taken from the bacterial membrane search are shown. Values are plotted along with their respective SEM values. | |
Table 2 Lipid composition of bacterial membranes as shown above in Fig. 7 and 9 to show how individual lipid abundance varies between the two different membrane types. Values are given as mean relative % SEM, and shown with the standard deviations as well as the range of the respective data sets
Lipid |
Bacterial (n = 21) |
Mammalian (n = 54) |
Erythrocyte (n = 9) |
Mean rel. (%) |
SEM |
Std dev. |
Mean rel. (%) |
SEM |
Std dev. |
Mean rel. (%) |
SEM |
Std dev. |
PE |
62.6 |
3.5 |
23.0 |
26.1 |
1.5 |
13.2 |
10.3 |
1.3 |
3.7 |
PG |
25.6 |
2.8 |
19.9 |
4.9 |
1.8 |
0.4 |
— |
— |
— |
CL |
13.0 |
2.1 |
13.9 |
4.9 |
0.8 |
3.0 |
— |
— |
— |
It is understood that CL plays a role in the membrane regions that surround proteins and their incorporation into the bilayer, along with non-bilayer lipids such as PE.39,40,145 It has also been reported that PC can account for up to 50% of lipid species in eukaryotes.146 This can go some way towards explaining the lipid split as observed in Fig. 7 where PC dominates, with zwitterionic lipids being argued to play the role of the dominant bilayer-forming lipid in bacterial membranes, leaving PE and CL to provide structural support and phase support around membrane proteins, which would account for the split of lipid species as shown in Fig. 9.
Bacterial membrane composition, has been well reviewed previously; with this in mind, the minor lipids of the bacterial compositions were omitted from this review in order to better highlight the fundamental differences across membrane types147 based on the lipids that tend to dominate. The Sohlenkamp and Geiger (2015) review outlines the presence of how composition varies with species of bacteria, and how E. coli has long served as the model organism; hence, the authors direct you to that work for a comprehensive breakdown of bacterial species. Crucially, the Sohlenkamp review supports the findings gathered through the literature search described above as far as dominant lipid headgroup species are concerned. The question of variation and diversity still remains.
Human erythrocyte membrane lipid composition
In order to further develop understanding of these membrane surfaces, it is important to understand what makes them unique. How does their lipid composition have an impact on function, or instead, is lipid composition optimised according to function? To that end, a lipid composition search was carried out for human erythrocyte membranes. The data for the human erythrocyte compositions are graphically in Fig. 9 and values with their associated SEMs in Table 4. One important note on the erythrocyte data; only 2 of the 9 compositions contained data that related to cholesterol content, and as such it may not be completely representative. The lack of cholesterol data was corrected for as described earlier in the methodology section. The average value taken was found to be in accordance with the literature. While the standard errors and deviations associated with the cholesterol values are unusually small, the lipid values are more representative of the erythrocyte membrane.
While the human GIT epithelia remain higher with respect to the amount of PC and PE, the human erythrocyte membrane contains a far greater proportion of cholesterol. The large proportion of cholesterol within erythrocyte membranes is something that has been understood for some time, so while only 2 articles refer to cholesterol content, these data points should not be excluded. Given that both of these membrane types are human epithelia, the differences are markedly large in terms of their lipid composition. It follows that there is a “geographical” factor involved when membranes regulate lipid species.137,138
Membrane lipid composition comparison
Comparing across all three membranes types shows that lipid variability is abundant. This has been covered in some detail in sections above that explore each individual membrane. The difference in both anatomical geography and function goes some way towards accounting for differences in the amounts of lipid present. Given that cholesterol plays such a role in maintaining layer integrity, it follows that the erythrocyte membrane (in which the shape of the cell is critical for its function needs to have a strictly regulated shape and contains high amounts of cholesterol.148 Conversely, the human GIT epithelium requires substances to pass across it for the absorption of neutraceuticals and as such might be required to be more fluid and permeable, so contains less cholesterol and more PC and PE-like species.35–37
The bacterial OM is reported to be highly asymmetric with the outer leaflet being enriched with lipopolysaccharide (LPS) and largely containing PE.149 The bacterial OM has much more PE as an external membrane component compared to either of the human-born membranes and in the bacterial membrane it is thought that LPS forms mixed bilayers with phospholipids and proteins intercalated into the layer.150 The results of Ishinaga and colleagues are complementary in showing that PE and PG are the dominant OM species, along with cardiolipin.151Fig. 9 (below) shows the lipid compositions of the human GIT epithelium and the human erythrocyte membrane, as well as the bacterial outer membrane.
As summarised above the bacterial OM is PE and PG dominated, while it is clear that irrespective of the environment from which a lipid sample is taken that PC, PE, and Chol are dominant as seen in Fig. 10. In samples of animal origin, a lack of PC lipids are replaced with increased amounts of cholesterol or peripheral lipids such as PS or PI. This is true for animal samples that are epithelial not of the GIT (orange bars) as well as from the animal GIT (green bars). In all cases SM appears to provide a similar supplementary role, as does cardiolipin. The cardiolipin content is only around half of that seen in bacterial outer membranes. PG and PA in all cases are extremely minor membrane components. Given that PG is predominantly a bacterial membrane lipid this is hardly surprising, and there is no need for PG on a charge basis as PS and PI both fulfil these roles. An error bar is missing from the animal relative percentage for PG in Fig. 10 as only a single study quoted PG levels. As a standard deviation and standard error cannot be calculated for a single value, SEM of this value is omitted. The low abundance of PA can be rationalised through its role as a mediator and intermediate in lipid biosynthesis.152 As the simplest membrane lipid, it follows that it would be used as a precursor to other derivatised lipids. It has been reported elsewhere to occur in extremely small amounts within a membrane.153 Other roles of PA include regulation of membrane dynamics as well as lipid signalling.
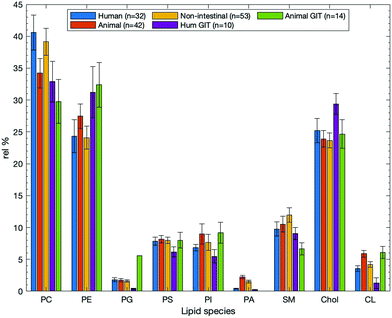 |
| Fig. 10 Grouped bar chart that shows lipid mean lipid composition that varies by sample environment as well as species. Lipid environments are compared according to common lipid types. It should be noted that the animal GIT value for PG has no error bar because there is only value reported. Values are given as mean relative percentage with their associated SEM values. | |
Open questions and further work
In this article, we have highlighted how the phospholipid composition of human epithelial membranes is reflected in the scientific literature. These were compared to bacterial membranes and the human erythrocyte membrane to display that different membranes vary in terms of their composition. When considering specifically the epithelial cell composition of the human GIT, we have shown that the amount of data available when surveying the literature is small, with a gap in the current understanding highlighted. In order to address the reason for such lipid variability as well as the role of lipids in these environments, more information is required.
One question that remains largely unanswered surrounds the need for lipid variability, especially on a small scale. Other publications have sought the answer to this question.154 However, no tangible and concise explanation has been provided, instead attention is only drawn to these problems. The framework that underlies these questions comes from a slightly updated version of the fluid mosaic model that describes separation of the membrane into discrete regions called lipid rafts. This, however, is a topic which remains controversial and the debate concerning lipid rafts is ongoing.28,155 While the dynamics of lipid membranes have been under investigation for years, the subtleties and nuances of the movement of lipids within bilayers remains somewhat elusive. Such aspects are still under contemporary investigation as efforts to understand the asymmetry and roles of lipids continue.156,157
This work shows that many membranes have a majority of zwitterionic, bilayer forming lipids to drive layer self-assembly. Both the human erythrocyte and the different membranes from Fig. 8 are PC, PE and Chol rich. As mentioned previously, the PC and PE are bilayer forming and the cholesterol helps inform layer rigidity through lipid tail intercalation. As previously mentioned, saturation of the hydrocarbon tails also plays a role in the fluidity of the system. Other lipids are responsible for allowing solubilisation of proteins into the membrane, helping with the regulation of charge in the layer and influencing curvature.39,40,145
Given the need for more information on the species that make up biological membranes that this review has identified, some of this can be acquired through more lipidomic studies of GIT epithelia, as well as epithelia at large. Some of the data obtained experimentally and computationally is stored in the Lipid MAPS database which collates structures, spectra, as well as some software and nomenclature tools.158 One recommendation of this review is to establish a more in depth online tool or platform where lipids can be explored through various criteria: based on cell type, cellular region, or bio-geographical location. One such template for this could be taken from a data set that displayed the genetic epidemiology of novel corona virus samples159 which allows various constraints or filters to be applied and is very interactive. Information on composition can also be gained from cell models, both top-down and bottom-up in their design. Having models that can be verified both a posteriori and within the literature is essential for producing a fundamental understanding of the structure of biological interfaces. Through better modelling, more experimental evidence can be obtained, which in turn will provide a more comprehensive method for learning about larger scale structures as well as providing a platform for accurate drug testing models. Such cell mimetics could prove crucial in terms of targeting drug and pro-drug therapies if they can be compositionally accurate.
One weakness of our review is that minor lipid components that may play a crucial role in terms of cell membrane function might have either been excluded or be too small to measure. Some papers only mentioned three or four lipid components, possibly due to only focusing on the major lipid components, or alternatively were either not able to measure or did not have significant resolving power in the techniques used to be able to identify the more peripheral membrane components. Equally, they may have only been sensitive to certain kinds of lipids. As a result, the errors may have been artificially inflated in cases where the values may be more spread out. Further, while the age of some of the publications involved may have had an effect on the experimental capabilities, these do not have any clear bearing on the introduction of errors. The shortcomings of these kinds of studies were consistently observed and the number of lipids reported is assumed to be a product of the time in which the work was conducted. Further, as all sample types taken here were similar, it is not surprising that the they are all compositionally similar, although some differences are accounted for.
There may be something that can be said about the lack in knowledge when compared to areas such as genomics and proteomics, given that lipidomics is still in its infancy; only really beginning to be explored in the 1990s and some 20 or 30 years behind its predecessors. Time could turn out to be a key factor in the volume of information available, as techniques and methodologies are ever improving, so too will the amount of data available. However, when one considers the amount of data available for areas like the composition of bacterial membranes, there is certainly no shortage. The turn of the millennium, and certainly the decade that followed, saw a rise in the identification of bacterial and viral pathogens as a need for concern, but that still doesn’t go all the way to explaining the gap between DNA/bacteria/viruses and phospholipids.160,161
Model biological interfaces are part of a rapidly growing field, and taking advantage of surface sensitive techniques to explore these interfaces are crucial in order to understand how they behave.26 With membranes being so ubiquitous, it is hardly surprising that a huge number of drug targets are a membrane in some form or other.162 Having an understanding of the both the structure and composition of biological membranes on a molecular scale is vital for being able to provide accurate, well informed models. These models are then viable for elucidating complex mechanisms and awareness about how interactions with biologically active compounds can be obtained.
Conclusions
To conclude, this paper has shown that developing a complete understanding of a complete, native biological membrane is a complex task and requires a whole host of parallel streams of work. This comprehensive literature review has shown that PC and PE lipids tend to dominate within humans, as well as the relative proportions of some of the more abundant peripheral lipid species such as PS, PI, SM, and PA. Further, we present for the first time an in depth break down of lipid headgroup composition of membranes from various anatomical environments including epithelia and intestinal samples of both human and animal origin. Cholesterol is often present in high quantities as a structural component as an aid for lipid packing and organisation. The supporting role of PS and PI are rationalised through their role as membrane charge regulators, and PA in very small quantities as an intermediate in lipid biosynthesis. SM is reported as having a critical role in the production of lateral organisation in the bilayer. A need for more work has been identified concerning the human GIT, and in particular its epithelial layer. The use of lipidomic and cell composition studies in tandem with both surface sensitive techniques and cell modelling and mimics could be the key to getting a wider and much more comprehensive understanding.
Author contributions
RTC – conceptualization, data curation, formal analysis, investigation, methodology, software, validation, visualization, writing (original draft), writing (review and editing). RJG – conceptualization, methodology, project administration, supervision, validation, writing (review and editing). RAF – conceptualization, project administration, supervision, validation, writing (review and editing). Contributor role definitions taken from https://casrai.org/credit/.
Abbreviations
Chol | Cholesterol |
CL | Cardiolipin |
FTIR | Fourier transform infrared |
GIT | Gastro-intestinal tract |
NR | Neutron reflectometry |
PA | Phosphatidic acid |
PC | Phosphatidylcholine |
PE | Phosphatidylethanolamine |
PG | Phosphatidylglycerol |
PI | Phosphatidylinositol |
PS | Phosphatidylserine |
SANS | Small angle neutron scattering |
SAXS | Small angle X-ray scattering |
SEM | Standard error of the mean |
SM | Sphingomyelin |
Conflicts of interest
The authors have no conflicts of interest to declare.
Acknowledgements
The authors would like to thank Dr Francesca Greco and Dr Al Edwards at the University of Reading for answering some of our initial questions, giving helping methodology advice, and taking the time to provide feedback on early manuscripts. Medical art and biological drawings for lipids used in figures or the table of contents graphic were used with permission from Servier Medical Art (https://smart.servier.com/, June 2021).
References
-
S. Singer, The fluid mosaic model of membrane structure, Springer, 1977, pp. 443–461 Search PubMed.
- D. M. Engelman, Nature, 2005, 438, 578 CrossRef CAS PubMed.
- F. Gambinossi, B. Mecheri, G. Caminati, M. Nocentini, M. Puggelli and G. Gabrielli, Mater. Sci. Eng., C, 2002, 22, 283–288 CrossRef.
- D. Ding, L. Qin, J. Yang, G. Ren and Y. Wu, Thermochim. Acta, 2014, 576, 36–38 CrossRef CAS.
- X. Yu, S. Chu, A. E. Hagerman and G. A. Lorigan, J. Agric. Food Chem., 2011, 59, 6783–6789 CrossRef CAS PubMed.
- A. Drechsler and F. Separovic, IUBMB Life, 2003, 55, 515–523 CrossRef CAS PubMed.
- K. F. Wang, R. Nagarajan and T. A. Camesano, Colloids Surf., B, 2014, 116, 472–481 CrossRef CAS PubMed.
- M. R. Sanders, L. A. Clifton, R. A. Frazier and R. J. Green, Langmuir, 2016, 32, 2050–2057 CrossRef CAS PubMed.
- J. E. Nielsen, V. A. Bjørnestad and R. Lund, Soft Matter, 2018, 14, 8750–8763 RSC.
- N. Paracini, L. A. Clifton, M. W. Skoda and J. H. Lakey, Proc. Natl. Acad. Sci. U. S. A., 2018, 115, E7587–E7594 CrossRef CAS PubMed.
- O. G. Mouritsen, Eur. J. Lipid Sci. Technol., 2011, 113, 1174–1187 CrossRef CAS PubMed.
- A. Arouri, M. Dathe and A. Blume, Biochim. Biophys. Acta, Biomembr., 2009, 1788, 650–659 CrossRef CAS PubMed.
- S. A. Datta, F. Heinrich, S. Raghunandan, S. Krueger, J. E. Curtis, A. Rein and H. Nanda, J. Mol. Biol., 2011, 406, 205–214 CrossRef CAS PubMed.
- Z. Jiang, F. Heinrich, R. P. McGlinchey, J. M. Gruschus and J. C. Lee, J. Phys. Chem. Lett., 2017, 8, 29–34 CrossRef CAS PubMed.
- S. C. van IJzendoorn, J. Agnetti and A. Gassama-Diagne, Biochim. Biophys. Acta, Biomembr., 2019, 183145 Search PubMed.
- L. A. Clifton, F. Ciesielski, M. W. Skoda, N. Paracini, S. A. Holt and J. H. Lakey, Langmuir, 2016, 32, 3485–3494 CrossRef CAS PubMed.
- A. Shevchenko and K. Simons, Nat. Rev. Mol. Cell Biol., 2010, 11, 593–598 CrossRef CAS PubMed.
- J. Ikenouchi, M. Hirata, S. Yonemura and M. Umeda, J. Cell Sci., 2013, 126, 3585–3592 CAS.
- J. Ikenouchi, Tissue Barriers, 2018, 6, 1–8 CrossRef CAS.
- G. van Meer and K. Simons, J. Cell. Biochem., 1988, 36, 51–58 CrossRef CAS PubMed.
- M. Sud, E. Fahy and D. Cotter,
et al.
, J. Lipid Res., 2002,(Database issue), D527–D532 Search PubMed.
- D. Voelker and G. W. Feigenson,
et al.
, Nat. Rev. Mol. Cell Biol., 2008, 9, 112–124 CrossRef PubMed.
- T. K. Lind, H. Wacklin, J. Schiller, M. Moulin, M. Haertlein, T. G. Pomorski and M. Cardenas, PLoS One, 2015, 10, 1–16 CrossRef PubMed.
- G. van Meer and K. Simons, EMBO J., 1986, 5, 1455–1464 CrossRef CAS.
- F. Prossnigg, A. Hickel, G. Pabst and K. Lohner, Biophys. Chem., 2010, 150, 129–135 CrossRef CAS.
- L. A. Clifton, R. A. Campbell, F. Sebastiani, J. Campos-Teran, J. F. Gonzalez-Martinez, S. Bjorklund, J. Sotres and M. Cardenas, Adv. Colloid Interface Sci., 2020, 277, 102118 CrossRef CAS.
- R. J. Schroeder, S. N. Ahmed, Y. Zhu, E. London and D. A. Brown, J. Biol. Chem., 1998, 273, 1150–1157 CrossRef CAS PubMed.
- I. Levental, K. R. Levental and F. A. Heberle, Trends Cell Biol., 2020, 30, 341–353 CrossRef CAS.
- D. A. Brown and E. London, J. Biol. Chem., 2000, 275, 17221–17224 CrossRef CAS PubMed.
- R. E. Brown, J. Cell Sci., 1998, 111, 1–9 CrossRef CAS.
- R. Tauber, K. Reher, K. Helling and H. Scherer, Biol. Sci. Space, 2002, 16, 22–26 Search PubMed.
- A. Kusumi, I. Koyama-Honda and K. Suzuki, Traffic, 2004, 5, 213–230 CrossRef CAS.
- L. Rajendran and K. Simons, J. Cell Sci., 2005, 118, 1099–1102 CrossRef CAS.
- F. Foglia, M. J. Lawrence and D. J. Barlow, Curr. Opin. Colloid Interface Sci., 2015, 20, 235–243 CrossRef CAS.
- F. De Meyer and B. Smit, Proc. Natl. Acad. Sci. U. S. A., 2009, 106, 3654–3658 CrossRef CAS.
- E. J. Fernandez-Perez, F. J. Sepulveda, C. Peters, D. Bascunan, N. O. Riffo-Lepe, J. Gonzalez-Sanmiguel, S. A. Sanchez, R. W. Peoples, B. Vicente and L. G. Aguayo, Front. Aging Neurosci., 2018, 10, 1–14 CrossRef.
- J. Lee, S.-t. Yang, V. Kiessling and L. K. Tamm, Biophys. J., 2017, 112, 9a CrossRef.
- P. L. Yeagle, Biochim. Biophys. Acta, Rev. Biomembr., 1985, 822(3–4), 267–287 CrossRef CAS.
- D. Marsh, Biophys. J., 2007, 93, 3884–3899 CrossRef CAS PubMed.
-
W. Dowhan, M. Bogdanov and E. Mileykovskaya, Biochemistry of lipids, lipoproteins and membranes, Elsevier, 2008, pp. 1–37 Search PubMed.
- F. Martin-Belmonte, A. Gassama, A. Datta, W. Yu, U. Rescher, V. Gerke and K. Mostov, Cell, 2007, 128, 383–397 CrossRef CAS.
- D. G. Drubin and W. J. Nelson, Cell, 1996, 84, 335–344 CrossRef CAS.
- K. Shin, V. C. Fogg and B. Margolis, Annu. Rev. Cell Dev. Biol., 2006, 22, 207–235 CrossRef CAS PubMed.
- K. Umeda, J. Ikenouchi, S. Katahira-Tayama, K. Furuse, H. Sasaki, M. Nakayama, T. Matsui, S. Tsukita, M. Furuse and S. Tsukita, Cell, 2006, 126, 741–754 CrossRef CAS PubMed.
- J. Connor and A. J. Schroit, Biochemistry, 1987, 26, 5099–5105 CrossRef CAS PubMed.
- P. Biitikofer, Z. W. Lin, D. T. Chiu, B. Lubin and F. A. Kuyperss, J. Biol. Chem., 1990, 265(27), 16035–16038 CrossRef.
- S. Sun, C. Liu, D. R. Melendez, T. Yang and P. S. Cremer, J. Am. Chem. Soc., 2020, 142(30), 13003–13010 CrossRef CAS.
- W. van’t Hof and G. van Meer, Curr. Top. Membr., 1994, 40, 539–563 Search PubMed.
- M. A. Kiselev and D. Lombardo, Biochim. Biophys. Acta, Gen. Subj., 2017, 1861, 3700–3717 CrossRef CAS PubMed.
- J. D. Perlmutter and J. N. Sachs, J. Am. Chem. Soc., 2011, 133, 6563–6577 CrossRef CAS PubMed.
- D. Essaid, V. Rosilio, K. Daghildjian, A. Solgadi, J. Vergnaud, A. Kasselouri and P. Chaminade, Biochim. Biophys. Acta, Biomembr., 2016, 1858, 2725–2736 CrossRef CAS PubMed.
- G. Van Meer, D. R. Voelker and G. W. Feigenson, Nat. Rev. Mol. Cell Biol., 2008, 9, 112–124 CrossRef CAS.
- J. L. Sampaio, M. J. Gerl, C. Klose, C. S. Ejsing, H. Beug, K. Simons and A. Shevchenko, Proc. Natl. Acad. Sci. U. S. A., 2011, 108, 1903–1907 CrossRef CAS.
- M. Jakubec, E. Barias, F. Kryuchkov, L. V. Hjørnevik and Ø. Halskau, ACS Omega, 2019, 4, 21596–21603 CrossRef CAS PubMed.
- G. W. Sewell, Y. A. Hannun, X. Han, G. Koster, J. Bielawski, V. Goss, P. J. Smith, F. Z. Rahman, R. Vega, S. L. Bloom, A. P. Walker, A. D. Postle and A. W. Segal, Int. J. Biochem. Cell Biol., 2012, 44, 1839–1846 CrossRef CAS PubMed.
- A. Stefanko, C. Thiede, G. Ehninger, K. Simons and M. Grzybek, PLoS One, 2017, 12, 1–12 CrossRef PubMed.
- R. Busche, B. Schroder, K. Huber, H. P. Sallmann and G. Breves, J. Comp. Physiol., B, 2007, 177, 135–142 CrossRef CAS PubMed.
- M. G. Schmitz and W. Renooij, Gastroenterology, 1990, 99, 1292–1296 CrossRef CAS.
- L. Mrnka, O. Novakova, F. Novak, E. Tvrzicka and J. Pacha, J. Steroid Biochem. Mol. Biol., 2000, 73, 11–17 CrossRef CAS.
- K. Turnheim, J. Gruber, C. Wachter and V. Ruiz-Gutierrez, Am. J. Physiol.: Cell Physiol., 1999, 277, 83–90 CrossRef.
- H. Weisser and M. Krieg, Prostate, 1997, 30, 41–46 CrossRef CAS.
- S. M. Schwarz, H. E. Bostwick, M. D. Danziger, L. J. Newman and M. S. Medow, Am. J. Physiol.: Gastrointest. Liver Physiol., 1989, 257, 138–144 CrossRef PubMed.
- T. Billington and P. R. Nayudu, Aust. J. Exp. Biol. Med. Sci., 1978, 56, 25–29 CrossRef CAS PubMed.
- S. E. Hancock, M. G. Friedrich, T. W. Mitchell, R. J. Truscott and P. L. Else, GeroScience, 2017, 39, 73–82 CrossRef CAS PubMed.
- C. Tessier, K. Sweers, A. Frajerman, H. Bergaoui, F. Ferreri, C. Delva, N. Lapidus, A. Lamaziere, J. P. Roiser, M. De Hert and P. Nuss, Transl. Psychiatry, 2016, 6(10), e906 CrossRef CAS PubMed.
- C. H. Cortie, A. J. Hulbert, S. E. Hancock, T. W. Mitchell, D. McAndrew and P. L. Else, Exp. Gerontol., 2015, 70, 135–143 CrossRef CAS PubMed.
- V. B. O’Donnell, R. C. Murphy and S. P. Watson, Circ. Res., 2014, 114, 1185–1203 CrossRef PubMed.
- R. Bartz, W. H. Li, B. Venables, J. K. Zehmer, M. R. Roth, R. Welti, R. G. Anderson, P. Liu and K. D. Chapman, J. Lipid Res., 2007, 48, 837–847 CrossRef CAS PubMed.
- P. K. Dudeja, J. M. Harig, K. Ramaswamy and T. A. Brasitus, Am. J. Physiol. - Gastrointest. Liver Physiol., 1989, 257, G809–G817 CrossRef CAS PubMed.
- Y. Wang, S. Hinz, O. Uckermann, P. Honscheid, W. von Schonfels, G. Burmeister, A. Hendricks, J. M. Ackerman, G. B. Baretton, J. Hampe, M. Brosch, C. Schafmayer, A. Shevchenko and S. Zeissig, Biochim. Biophys. Acta, Mol. Cell Biol. Lipids, 2020, 1865, 158579 CrossRef CAS PubMed.
- Y. P. Kang, J. H. Yoon, N. P. Long, G. B. Koo, H. J. Noh, S. J. Oh, S. B. Lee, H. M. Kim, J. Y. Hong, W. J. Lee, S. J. Lee, S. S. Hong, S. W. Kwon and Y. S. Kim, Front. Oncol., 2019, 1865(3), 158579 Search PubMed.
- F. F. Eiriksson, O. Rolfsson, H. M. Ogmundsdottir, G. G. Haraldsson, M. Thorsteinsdottir and S. Halldorsson, Int. J. Biochem. Cell Biol., 2018, 103, 99–104 CrossRef CAS PubMed.
- L. Macchioni, M. Petricciuolo, M. Davidescu, K. Fettucciari, P. Scarpelli, R. Vitale, L. Gatticchi, P. L. Orvietani, A. Marchegiani, P. Marconi, G. Bassotti, A. Corcelli and L. Corazzi, Biochim. Biophys. Acta, Mol. Cell Biol. Lipids, 2018, 1863, 895–908 CrossRef CAS PubMed.
- E. Morel, S. Ghezzal, G. Lucchi, C. Truntzer, J. P. Pais de Barros, F. Simon-Plas, S. Demignot, C. Mineo, P. W. Shaul, A. Leturque, M. Rousset and V. Carriere, Biochim. Biophys. Acta, Mol. Cell Biol. Lipids, 2018, 1863, 199–211 CrossRef CAS PubMed.
- K. Mida, A. Shamay and N. Argov-Argaman, J. Agric. Food Chem., 2012, 60, 10657–10665 CrossRef CAS PubMed.
- B. C. Cohen, A. Shamay and N. Argov-Argaman, PLoS One, 2015, 10, 1–19 Search PubMed.
- R. Zarbock, M. Woischnik, C. Sparr, T. Thurm, S. Kern, E. Kaltenborn, A. Hector, D. Hartl, G. Liebisch, G. Schmitz and M. Griese, BMC Pulm. Med., 2012, 12(1), 1–13 CrossRef PubMed.
- M. G. Marquez, F. Leocata Nieto, M. C. Fernandez-Tome, N. O. Favale and N. Sterin-Speziale, Lipids, 2008, 43, 343–352 CrossRef CAS PubMed.
- M. G. Marquez, N. O. Favale, F. Leocata Nieto, L. G. Pescio and N. Sterin-Speziale, Biochim. Biophys. Acta, Biomembr., 2012, 1818, 491–501 CrossRef CAS PubMed.
- Q. Li, Q. Zhang, M. Wang, S. Zhao, J. Ma, N. Luo, N. Li, Y. Li, G. Xu and J. Li, Clin. Immunol., 2008, 126, 67–80 CrossRef CAS PubMed.
- L. Huang, R. Estrada, M. C. Yappert and D. Borchman, Free Radical Biol. Med., 2006, 41, 1425–1432 CrossRef CAS PubMed.
- I. Diaz-Del Consuelo, Y. Jacques, G. P. Pizzolato, R. H. Guy and F. Falson, Arch. Oral Biol., 2005, 50, 981–987 CrossRef CAS PubMed.
- G. A. Bongiovanni, A. R. Eynard and R. O. Calderon, Mol. Cell. Biochem., 2005, 271, 69–75 CrossRef CAS PubMed.
- R. Prabhu and K. A. Balasubramanian, J. Gastroenterol. Hepatol., 2003, 18, 809–814 CrossRef PubMed.
- R. E. Olsen, B. T. Dragnes, R. Myklebust and E. Ringø, Fish Physiol. Biochem., 2003, 29, 181–192 CrossRef CAS.
- M. Engelke, S. Tykhonova, M. Zorn-Kruppa and H. Diehl, Pharmacol. Toxicol., 2002, 91, 13–21 CrossRef CAS PubMed.
- I. O. Thompson, P. Van der Bijl, C. W. Van Wyk and A. D. Van Eyk, Arch. Oral Biol., 2001, 46, 1091–1098 CrossRef CAS PubMed.
- R. Prabhu and K. A. Balasubramanian, Anal. Biochem., 2001, 289, 157–161 CrossRef CAS PubMed.
- G. A. Fines, J. S. Ballantyne and P. A. Wright, Am. J. Physiol. - Regul. Integr. Comp. Physiol., 2001, 280, 16–24 CrossRef PubMed.
- B. J. Tewes and H. J. Galla, Endothel. J. Endothel. Cell Res., 2001, 8, 207–220 CAS.
- M. Madesh, R. Anup, O. Benard and K. A. Balasubramanian, Free Radical Biol. Med., 1999, 26, 836–843 CrossRef CAS PubMed.
- V. Calderon, A. Lazaro, R. G. Contreras, L. Shoshani, C. Flores-Maldonado, L. Gonzalez-Mariscal, G. Zampighi and M. Cereijido, J. Membr. Biol., 1998, 164, 59–69 CrossRef CAS PubMed.
- J. M. Lopez-Pedrosa, M. I. Torres, M. I. Fernandez, A. Rios and A. Gil, J. Nutr., 1998, 128, 224–233 CrossRef CAS PubMed.
- G. Griffiths, H. E. Jones, C. L. Eaton and A. K. Stobart, Prostate, 1997, 31, 29–36 CrossRef CAS PubMed.
- C. M. Ruiz-Guitierrez and V. Vasquez, Int. J. Gastroenterol., 1997, 58, 161–167 Search PubMed.
- G. S. Schuster, J. F. Erbland, C. A. Lefebvre, G. B. Caughman and K. L. Knoernschild, J. Biomater. Sci., Polym. Ed., 1997, 8, 363–375 CrossRef CAS PubMed.
- H. Weisser and M. Krieg, Prostate, 1998, 36, 235–243 CrossRef CAS PubMed.
- R. Acierno, M. Maffia, P. Sicuro, L. Fiammata, M. Rollo, L. Ronzini and C. Storelli, Comp. Biochem. Physiol., Part A: Mol. Integr. Physiol., 1996, 115, 303–307 CrossRef.
- H. G. Gulcan, R. A. Alvarez, M. B. Maude and R. E. Anderson, Investig. Ophthalmol. Vis. Sci., 1993, 34, 3187–3193 CAS.
- E. Levy, L. Thibault and D. Menard, J. Lipid Res., 1992, 33, 1607–1617 CrossRef CAS.
- V. Ruiz-Gutierrez, A. Cert and J. J. Rios, J. Chromatogr. B: Biomed. Sci. Appl., 1992, 575, 1–6 CrossRef CAS.
- M. A. Perez-Albarsanz, P. Lopez-Aparicio, S. Senar and M. N. Recio, Biochim. Biophys. Acta, Biomembr., 1991, 1066, 124–130 CrossRef CAS.
- M. E. Turini, A. B. Thomson and M. T. Clandinin, Lipids, 1991, 26, 431–440 CrossRef CAS PubMed.
- V. Duranthon, L. Fremont and C. L. Leger, Lipids, 1991, 26, 175–181 CrossRef CAS PubMed.
- R. Christon, J. C. Meslin, J. Thevenoux, A. Linard, C. L. Leger and S. Delpal, Reprod. Nutr. Dev., 1991, 31, 691–701 CrossRef CAS PubMed.
- K. Simons and W. L. Vaz, Annu. Rev. Biophys. Biomol. Struct., 2004, 33, 269–295 CrossRef CAS PubMed.
- R. Schmidt, D. Yonghong and R. Hoffmann, Diagn. Microbiol. Infect. Dis., 2018, 90, 316–323 CrossRef CAS PubMed.
- L. Zheng, Y. Lin, S. Lu, J. Zhang and M. Bogdanov, Biochim. Biophys. Acta, Mol. Cell Biol. Lipids, 2017, 1862, 1404–1413 CrossRef CAS PubMed.
- V. W. Rowlett, V. K. P. S. Mallampalli, A. Karlstaedt, W. Dowhan, H. Taegtmeyer, W. Margolin and H. Vitrac, J. Bacteriol., 2017, 199, 1–22 CrossRef PubMed.
- L. Davydova, S. Bakholdina, M. Barkina, P. Velansky, M. Bogdanov and N. Sanina, Biochimie, 2016, 123, 103–109 CrossRef CAS PubMed.
- H. Benamara, C. Rihouey, I. Abbes, M. A. Ben Mlouka, J. Hardouin, T. Jouenne and S. Alexandre, PLoS One, 2014, 9(9), e108478 CrossRef PubMed.
- N. N. Mishra, A. S. Bayer, T. T. Tran, Y. Shamoo, E. Mileykovskaya, W. Dowhan, Z. Guan and C. A. Arias, PLoS One, 2012, 7, 1–10 Search PubMed.
- D. K. Giles, J. V. Hankins, Z. Guan and M. S. Trent, Mol. Microbiol., 2011, 79, 716–728 CrossRef CAS PubMed.
- R. F. Epand, P. B. Savage and R. M. Epand, Biochim. Biophys. Acta, Biomembr., 2007, 1768, 2500–2509 CrossRef CAS PubMed.
- S. Laurinavicius, R. Kakela, P. Somerharju and D. H. Bamford, Virology, 2004, 322, 328–336 CrossRef CAS PubMed.
- F. R. Rana, C. M. Sultany and J. Blazyk, J. Microbiol. Methods, 1991, 14, 41–51 CrossRef CAS.
- G. V. Marinetti and K. Cattieu, Chem. Phys. Lipids, 1981, 28(3), 241–251 CrossRef CAS.
- I. Contreras, L. Shapiro and S. Henry, J. Bacteriol., 1978, 135, 1130–1136 CrossRef CAS PubMed.
- G. Barrett, M. Horiuchi, M. Paul, R. E. Pratt, N. Nakamura and V. J. Dzau, Proc. Natl. Acad. Sci. U. S. A., 1992, 89, 885–889 CrossRef CAS PubMed.
- W. Yuqian, W. Jianli, L. Ye, W. Biwen, G. Tao and W. Xiaoyuan, Eur. J. Mass Spectrom., 2015, 21, 739–746 CrossRef PubMed.
- R. F. Epand, J. E. Pollard, J. O. Wright, P. B. Savage and R. M. Epand, Antimicrob. Agents Chemother., 2010, 54, 3708–3713 CrossRef CAS PubMed.
- S. Laurinavicius, D. H. Bamford and P. Somerharju, Biochim. Biophys. Acta, Biomembr., 2007, 1768, 2568–2577 CrossRef CAS PubMed.
- G. M. Loyd and N. J. Russell, Can. J. Microbiol., 1984, 30, 1357–1366 CrossRef.
- H. Anwar, M. R. Brown, R. M. Cozens and P. A. Lambert, J. Gen. Microbiol., 1983, 129, 499–507 CAS.
- R. Masilamani, M. B. Cian and Z. D. Dalebroux, Infect. Immun., 2018, 86, 1–15 CrossRef PubMed.
- A. Aloui, M. Mihoub, M. M. Sethom, A. Chatti, M. Feki, N. Kaabachi and A. Landoulsi, Foodborne Pathog. Dis., 2010, 7, 573–583 CrossRef CAS PubMed.
- R. M. Epand and R. F. Epand, Biochim. Biophys. Acta, Biomembr., 2009, 1788, 289–294 CrossRef CAS PubMed.
- H. G. Patel, R. V. Aras, S. S. Katyare and K. R. Dave, Z. Naturforsch., C: J. Biosci., 2000, 55, 770–777 CrossRef CAS PubMed.
- E. Weidekamm, B. Brdiczka and M. Wildermuth, Mol. Biol. Rep., 1978, 4(1), 25–28 CrossRef CAS PubMed.
- L. Li and W. Reserve, Biochem. J., 1991, 274(1), 121–132 CrossRef PubMed.
- Y. Kurumi, Y. Adachi, T. Itoh, H. Kobayashi, T. Nanno and T. Yamamoto, Gastroenterol. Jpn., 1991, 26, 628–632 CrossRef CAS PubMed.
- J. Bramham and F. G. Riddell, J. Inorg. Biochem., 1995, 57, 23–32 CrossRef CAS PubMed.
- J. A. Virtanen, K. H. Cheng and P. Somerharju, Proc. Natl. Acad. Sci. U. S. A., 1998, 95, 4964–4969 CrossRef CAS PubMed.
- E. Faloia, G. G. Garrapa, D. Martarelli, M. A. Camilloni, G. Lucarelli, R. Staffolani, F. Mantero, G. Curatola and L. Mazzanti, Eur. J. Clin. Invest., 1999, 29, 432–437 CrossRef CAS PubMed.
- K. G. Karageuzyan, Neurochem. Res., 1999, 24(9), 1161–1167 CrossRef CAS PubMed.
- V. V. Revin, N. V. Gromova, E. S. Revina, I. P. Grunyushkin, A. Y. Tychkov, A. Y. Samonova, A. N. Kukina, A. A. Moskovkin, J. C. Bourdon and N. Zhelev, Biotechnol. Biotechnol. Equip., 2018, 32, 1236–1250 CrossRef CAS.
- Y. Zhong, H. Tang, Q. Zeng, X. Wang, G. Yi, K. Meng, Y. Mao and X. Mao, Ups. J. Med. Sci., 2012, 117, 390–398 CrossRef PubMed.
- F. D. Kolodgie, H. K. Gold, A. P. Burke, D. R. Fowler, H. S. Kruth, D. K. Weber, A. Farb, L. J. Guerrero, M. Hayase, R. Kutys, J. Narula, A. V. Finn and R. Virmani, N. Engl. J. Med., 2003, 349, 2316–2325 CrossRef CAS PubMed.
- A. Chabanel, M. Flamm, K. L. Sung, M. M. Lee, D. Schachter and S. Chien, Biophys. J., 1983, 44, 171–176 CrossRef CAS PubMed.
- A. Triebl, J. Hartler, M. Trotzmuller and H. C. Kofeler, Biochim. Biophys. Acta, Mol. Cell Biol. Lipids, 2017, 1862, 740–746 CrossRef CAS PubMed.
- J. P. Slotte, Chem. Phys. Lipids, 1999, 102, 13–27 CrossRef CAS.
- M. Porn, M. Ares and J. Slotte, J. Lipid Res., 1993, 34, 1385–1392 CrossRef CAS.
- K. Simons and E. Ikonen, Nature, 1997, 387(6633), 569–572 CrossRef CAS PubMed.
- D. A. Brown and E. London, Biochem. Biophys. Res. Commun., 1997, 240, 1–7 CrossRef CAS PubMed.
-
W. Dowhan and M. Bogdanov, in Biochem. Lipids Lipoproteins Membr., ed. D. E. Vance and J. E. Vance, Elsevier Science, 4th edn, 2002, vol. 36, pp. 1–35 Search PubMed.
- G. Van Meer, D. R. Voelker and G. W. Feigenson, Nat. Rev. Mol. Cell Biol., 2008, 9, 112–124 CrossRef CAS PubMed.
- C. Sohlenkamp and O. Geiger, FEMS Microbiol. Rev., 2015, 40, 133–159 CrossRef PubMed.
- M. Girasole, G. Pompeo, A. Cricenti, A. Congiu-Castellano, F. Andreola, A. Serafino, B. Frazer, G. Boumis and G. Amiconi, Biochim. Biophys. Acta, Biomembr., 2007, 1768, 1268–1276 CrossRef CAS PubMed.
- T. J. Silhavy, N. Ruiz and D. Kahne, Nat. Rev. Microbiol., 2006, 4, 57–66 CrossRef CAS PubMed.
- Y. Kamio and H. Nikaido, Biochemistry, 1976, 15, 2561–2570 CrossRef CAS PubMed.
- M. Ishinaga, R. Kanamoto and M. Kito, J. Biochem., 1979, 86(1), 161–165 CAS.
- R. Thakur, A. Naik, A. Panda and P. Raghu, Front. Cell Dev. Biol., 2019, 7, 1–14 CrossRef PubMed.
- E. E. Kooijman and K. N. Burger, Biochim. Biophys. Acta, Mol. Cell Biol. Lipids, 2009, 1791, 881–888 CrossRef CAS PubMed.
- L. A. Bagatolli, J. H. Ipsen, A. C. Simonsen and O. G. Mouritsen, Prog. Lipid Res., 2010, 49, 378–389 CrossRef CAS PubMed.
- K. Simons and G. Van Meer, Biochemistry, 1988, 27, 6197–6202 CrossRef CAS PubMed.
- H. P. Wacklin, Langmuir, 2011, 27, 7698–7707 CrossRef CAS PubMed.
- M. Chabanon, J. C. Stachowiak and P. Rangamani, Wiley Interdiscip. Rev.: Syst. Biol. Med., 2017, 9, 20–22 Search PubMed.
- E. Fahy, S. Subramaniam, R. C. Murphy, M. Nishijima, C. R. Raetz, T. Shimizu, F. Spener, G. van Meer, M. J. Wakelam and E. A. Dennis, J. Lipid Res., 2009, 50, S9–S14 CrossRef PubMed.
- J. Hadfield, C. Megill, S. M. Bell, J. Huddleston, B. Potter, C. Callender, P. Sagulenko, T. Bedford and R. A. Neher, Bioinformatics, 2018, 34, 4121–4123 CrossRef CAS PubMed.
- R. M. Epand and R. F. Epand, J. Pept. Sci., 2011, 17, 298–305 CrossRef CAS PubMed.
- V. C. Cheng, S. K. Lau, P. C. Woo and Y. Y. Kwok, Clin. Microbiol. Rev., 2007, 20, 660–694 CrossRef CAS PubMed.
- J. P. Overington, B. Al-Lazikani and A. L. Hopkins, Nat. Rev. Drug Discovery, 2006, 5, 993–996 CrossRef CAS PubMed.
Footnote |
† Electronic supplementary information (ESI) available. See DOI: 10.1039/d1sm00703c |
|
This journal is © The Royal Society of Chemistry 2021 |
Click here to see how this site uses Cookies. View our privacy policy here.